User login
Measuring Agreement After CICU Handoffs
Increasing attention has been paid to the need for effective handoffs between healthcare providers since the Joint Commission identified standardized handoff protocols as a National Patient Safety Goal in 2006.1 Aside from adverse consequences for patients, poor handoffs produce provider uncertainty about care plans.[2, 3] Agreement on clinical information after a handoff is critical because a significant proportion of data is not documented in the medical record, leaving providers reliant on verbal communication.[4, 5, 6] Providers may enter the handoff with differing opinions; however, to mitigate the potential safety consequences of discontinuity of care,[7] the goal should be to achieve consensus about proposed courses of action.
Given the recent focus on improving handoffs, rigorous, outcome‐driven measures of handoff quality are clearly needed, but measuring shift‐to‐shift handoff quality has proved challenging.[8, 9] Previous studies of physician handoffs surveyed receivers for satisfaction,[10, 11] compared reported omissions to audio recordings,[3] and developed evaluation tools for receivers to rate handoffs.[12, 13, 14, 15] None directly assess the underlying goal of a handoff: the transfer of understanding from sender to receiver, enabling safe transfer of patient care responsibility.[16] We therefore chose to measure agreement on patient condition and treatment plans following handoff as an indicator of the quality of the shared clinical understanding formed. Advantages of piloting this approach in the pediatric cardiac intensive care unit (CICU) include the relatively homogenous patient population and small number of medical providers. If effective, the strategy of tool development and evaluation could be generalized to different clinical environments and provider groups.
Our aim was to develop and validate a tool to measure the level of shared clinical understanding regarding the condition and treatment plan of a CICU patient after handoff. The tool we designed was the pediatric cardiology Patient Knowledge Assessment Tool (PKAT), a brief, multiple‐item questionnaire focused on key data elements for individual CICU patients. Although variation in provider opinion helps detect diagnostic or treatment errors,[8] the PKAT is based on the assumption that achieving consensus on clinical status and the next steps of care is the goal of the handoff.
METHODS
Setting
The CICU is a 24‐bed medical and surgical unit in a 500‐bed free standing children's hospital. CICU attending physicians work 12‐ or 24‐hour shifts and supervise front line clinicians (including subspecialty fellows, nurse practitioners, and hospitalists, referred to as clinicians in this article) who work day or night shifts. Handoffs occur twice daily, with no significant differences in handoff practices between the 2 times. Attending physicians (referred to as attendings in this article) conduct parallel but separate handoffs from clinicians. All providers work exclusively in the CICU with the exception of fellows, who rotate monthly.
This study was approved by the institutional review board at The Children's Hospital of Philadelphia. All provider subjects provided informed consent. Consent for patient subjects was waived.
Development of the PKAT
We developed the PKAT content domains based on findings from previous studies,[2, 3] unpublished survey data about handoff omissions in our CICU, and CICU attending expert opinion. Pilot testing included 39 attendings and clinicians involved in 60 handoffs representing a wide variety of admissions. Participants were encouraged to share opinions on tool content and design with study staff. The PKAT (see Supporting Information, Appendix, in the online version of this article) was refined iteratively based on this feedback.
Video Simulation Testing
We used video simulation to test the PKAT for inter‐rater reliability. Nine patient handoff scenarios were written with varying levels of patient complexity and clarity of dialogue. The scenarios were filmed using the same actors and location to minimize variability aside from content. We recruited 10 experienced provider subjects (attendings and senior fellows) to minimize the effect of knowledge deficits. For each simulated handoff, subjects were encouraged to annotate a mock sign‐out sheet, which mimicked the content and format of the CICU sign‐out sheet. After watching all 9 scenarios, subjects completed a PKAT for each handoff from the perspective of the receiver based on the videotape. These standardized conditions allowed for assessment of inter‐rater reliability.
In Situ Testing
We then tested the PKAT in situ in the CICU to assess construct validity. We chose to study the morning handoff because the timing and location are more consistent. We planned to study 90 patient handoffs because the standard practice for testing a new psychometric instrument is to collect 10 observations per item.[17] On study days, 4 providers completed a PKAT for each selected handoff: the sending attending, receiving attending, sending clinician, and receiving clinician.
Study days were scheduled over 2 months to encompass a range of providers. Given the small number of attendings, we did not exclude those who had participated in video simulation testing. On study days, 6 patients were enrolled using stratified sampling to ensure adequate representation of new admissions (ie, admitted within 24 hours). The sending attending received the PKAT forms prior to the handoff. The receiving attending and clinicians received the PKAT after handoff. This difference in administration was due to logistic concerns: sending attendings requested to receive the PKATs earlier because they had to complete all 6 PKATs, whereas other providers completed 3 or fewer per day. Thus, sending attendings could complete the PKAT before or after the handoff, whereas all other participants completed the instrument after the handoff.
To test for construct validity, we gathered data on participating providers and patients, hypothesizing that PKAT agreement levels would decrease in response to less experienced providers or more complex patients. Provider characteristics included previous handoff education and amount of time worked in our CICU. Attending CICU experience was dichotomized into first year versus second or greater year. Clinician experience was dichotomized into first or second month versus third or greater month of CICU service. Each PKAT asked the handoff receiver whether he or she had recently cared for this patient or gathered information prior to handoff (eg, speaking to bedside nurse).
Recorded patient characteristics included age, length of stay, and admission type including neonatal/preoperative observation, postoperative (first 7 days after operation), prolonged postoperative (>7 days after operation), and medical (all others). In recognition of differences in handoffs during the first 24 hours of admission and the right‐skewed length of stay in the CICU, we analyzed length of stay based on the following categories: new admission (<24 hours), days 2 to 7, days 8 to 14, days 15 to 31, and >31 days. Because the number of active medications has been shown to correlate with treatment regimen complexity[18] and physician ratings of illness severity,[19] we recorded this number as a surrogate measure of patient complexity. For analytic purposes, we categorized the number of active medications into quartiles.
Provider subject characteristics and PKAT responses were collected using paper forms and entered into REDCap (Research Electronic Data Capture; REDCap Consortium,
Statistical Analysis
The primary outcome measure was the PKAT agreement level among providers evaluating the same handoff. For the reliability assessment, we calculated agreement across all providers analyzing the simulation videos, expecting that multiple providers should have high agreement for the same scenarios if the instrument has high inter‐rater reliability. For the validity assessment, we calculated agreement for each individual handoff by item and then calculated average levels of agreement for each item across provider and patient characteristics. We analyzed handoffs between attendings and clinicians separately. For items with mutually exclusive responses, simple yes/no agreement was calculated. For items requiring at least 1 response, agreement was coded when both respondents selected at least 1 response in common. For items that did not require a selection, credit was given if both subjects agreed that none of the conditions were present or if they agreed that at least 1 condition was present. In a secondary analysis, we repeated the analyses with unique sender‐receiver pair as the unit of analysis to account for correlation in the pair interaction.
Summary statistics were used to describe provider and patient characteristics. Mean rates of agreement with 95% confidence intervals were calculated for each item. The Wilcoxon rank sum test was used to compare mean results between groups (eg, attendings vs clinicians). A nonparametric test for trend, which is an extension of the Wilcoxon rank sum test,[21] was used to compare mean results across ordered categories (eg, length of stay). All tests of significance were at P<0.05 level and 2‐tailed. All statistical analysis was done using Stata 12 (StataCorp, College Station, TX).
RESULTS
Provider subject types are represented in Table 1. Handoffs between these 29 individuals resulted in 70 unique sender and receiver combinations with a median of 2 PKATs completed per unique sender‐receiver pair (range, 115). Attendings had lower rates of handoff education than clinicians (11% vs 85% for in situ testing participants, P=0.01). Attendings participating in in situ testing had worked in the CICU for a median of 3 years (range, 116 years). Clinicians participating in in situ testing had a median of 3 months of CICU experience (range, 195 months). Providers were 100% compliant with PKAT completion.
Simulation Testing, n=10 | In Situ Testing, n=29 | |
---|---|---|
| ||
Attending physicians | 40% (4) | 31% (9) |
Clinicians | 60% (6) | 69% (20) |
Clinician type | ||
Cardiology | 67% (4) | 35% (7) |
Critical care medicine | 33% (2) | 25% (5) |
CICU nurse practitioner | 25% (5) | |
Anesthesia | 5% (1) | |
Neonatology | 5% (1) | |
Hospitalist | 5% (1) |
Video Simulation Testing
Inter‐rater agreement is shown in Figure 1. Raters achieved perfect agreement for 8/9 questions on at least 1 scenario, supporting high inter‐rater reliability for these items. Some items had particularly high reliability. For example, on item 3, subjects achieved perfect agreement for 5/9 scenarios, making 1 both the median and maximum value. Because item 7 (barriers to transfer) did not demonstrate high inter‐rater agreement, we excluded it from the in situ analysis.
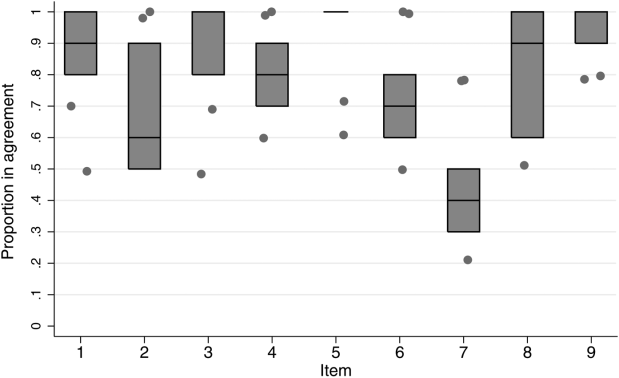
In Situ Testing
Characteristics of patients whose handoffs were selected for in situ testing are listed in Table 2. Because some patients were selected on multiple study days, these 90 handoffs represented 58 unique patients. These 58 patients are representative of the CICU population (data not shown). The number of handoffs studied per patient ranged from 1 to 7 (median 1). A total of 19 patients were included in the study more than once; 13 were included twice.
Characteristic | Categories | Percentage |
---|---|---|
| ||
Age | <1 month | 30 |
112 months | 34 | |
112 years | 28 | |
1318 years | 6 | |
>18 years | 2 | |
Type of admission | Postnatal observation/preoperative | 20 |
Postoperative | 29 | |
Prolonged postoperative (>7 days) | 33 | |
Other admission | 18 | |
CICU days | 1 | 31 |
27 | 22 | |
814 | 10 | |
1531 | 13 | |
>31 | 23 | |
Active medications | <8 | 26 |
811 | 26 | |
1218 | 26 | |
>18 | 23 |
Rates of agreement between handoff pairs, stratified by attending versus clinician, are shown in Table 3. Overall mean levels of agreement ranged from 0.41 to 0.87 (median 0.77). Except for the ratio of pulmonary to systemic blood flow question, there were no significant differences in agreement between attendings as compared to clinicians. When this analysis was repeated with unique sender‐receiver pair as the unit of analysis to account for within‐pair clustering, we obtained qualitatively similar results (data not shown).
PKAT Item | Agreement Level | ||||
---|---|---|---|---|---|
Attending Physician Pair | Clinician Pair | Pa | |||
Mean | 95% CI | Mean | 95% CI | ||
| |||||
Clinical condition | 0.71 | 0.620.81 | 0.78 | 0.690.87 | 0.31 |
Cardiovascular plan | 0.76 | 0.670.85 | 0.68 | 0.580.78 | 0.25 |
Respiratory plan | 0.67 | 0.580.78 | 0.76 | 0.670.85 | 0.26 |
Source of pulmonary blood flow | 0.83 | 0.750.91 | 0.87 | 0.800.94 | 0.53 |
Ratio of pulmonary to systemic flow | 0.67 | 0.570.77 | 0.41 | 0.310.51 | <0.01 |
Anticoagulation indication | 0.79 | 0.700.87 | 0.77 | 0.680.86 | 0.72 |
Active cardiovascular issues | 0.87 | 0.800.94 | 0.76 | 0.670.85 | 0.06 |
Active noncardiovascular issues | 0.80 | 0.720.88 | 0.78 | 0.690.87 | 0.72 |
Both length of stay and increasing number of medications affected agreement levels for PKAT items (Table 4). Increasing length of stay correlated directly with agreement on cardiovascular plan and ratio of pulmonary to systemic flow and inversely with indication for anticoagulation. Increasing number of medications had an inverse correlation with agreement on indication for anticoagulation, active cardiovascular issues, and active noncardiovascular issues.
Item | CICU LOS | No. of Active Medications | |||||||||
---|---|---|---|---|---|---|---|---|---|---|---|
1 Day (n=56) | 27 Days (n=40) | 814 Days (n=18) | 1531 Days (n=24) | >31 Days (n=42) | Pa | 8 (n=46) | 811 (n=46) | 1218 (n=46) | >18 (n=42) | Pa | |
| |||||||||||
Clinical condition | 0.75 | 0.63 | 0.78 | 0.83 | 0.79 | 0.29 | 0.71 | 0.70 | 0.78 | 0.79 | 0.32 |
Cardiovascular plan | 0.59 | 0.73 | 0.67 | 0.79 | 0.86 | <0.01 | 0.63 | 0.72 | 0.63 | 0.81 | 0.16 |
Respiratory plan | 0.68 | 0.78 | 0.61 | 0.83 | 0.69 | 0.79 | 0.67 | 0.72 | 0.78 | 0.69 | 0.68 |
Source of pulmonary blood flow | 0.93 | 0.75 | 0.72 | 0.96 | 0.83 | 0.63 | 0.72 | 0.91 | 0.98 | 0.79 | 0.22 |
Ratio of pulmonary to systemic flow | 0.45 | 0.40 | 0.67 | 0.75 | 0.62 | 0.01 | 0.46 | 0.52 | 0.52 | 0.67 | 0.06 |
Anticoagulation indication | 0.89 | 0.83 | 0.89 | 0.67 | 0.60 | <0.01 | 0.93 | 0.78 | 0.76 | 0.62 | <0.01 |
Active cardiovascular issues | 0.86 | 0.78 | 0.72 | 0.92 | 0.76 | 0.52 | 0.87 | 0.76 | 0.54 | 0.55 | <0.01 |
Active noncardiovascular issues | 0.86 | 0.80 | 0.72 | 0.75 | 0.74 | 0.12 | 0.83 | 0.83 | 0.76 | 0.52 | <0.01 |
In contrast, there were no significant differences in item agreement levels based on provider characteristics, including experience, handoff education, prehandoff preparation, or continuity (data not shown).
CONCLUSIONS
Our results provide initial evidence of reliability and validity of scores for a novel tool, the PKAT, designed to assess providers' shared clinical understanding of a pediatric CICU patient's condition and treatment plan. Because this information should be mutually understood following any handoff, we believe this tool or similar agreement assessments could be used to measure handoff quality across a range of clinical settings. Under the standardized conditions of video simulation, experienced CICU providers achieved high levels of agreement on the PKAT, demonstrating inter‐rater reliability. In situ testing results suggest that the PKAT can validly identify differences in understanding between providers for both routine and complex patients.
The achievement of 100% compliance with in situ testing demonstrates that this type of tool can feasibly be used in a real‐time clinical environment. As expected, mean agreement levels in situ were lower than levels achieved in video simulation. By item, mean levels of agreement for attending and clinician pairs were similar.
Our assessment of PKAT validity demonstrated mixed results. On the one hand, PKAT agreement did not vary significantly by any measured provider characteristics. Consistent with the lack of difference between attendings and clinicians, more experienced providers in both groups did not achieve higher levels of agreement. This finding is surprising, and may illustrate that unmeasured provider characteristics, such as content knowledge, obscure the effects of experience or other measured variables on agreement levels. Alternatively, providing the PKAT to the sending attending prior to the handoff, rather than afterward as for the receiving attendings and clinicians, might have artificially lowered attending agreement levels, concealing a difference due to experience.
On the other hand, construct validity of several items was supported by the difference in agreement levels based on patient characteristics. Agreement levels varied on 5/8 questions as patients became more complex, either defined by length of stay or number of medications. These differences show that agreement on PKAT items responds to changes in handoff complexity, a form of construct validity. Furthermore, these findings suggest that handoffs of more chronic or complex patients may require more attention for components prone to disagreement in these settings. Although complexity and longer length of stay are nonmodifiable risk factors, identifying these handoffs as more susceptible to disagreement provides potential targets for intervention.
It is important to move beyond he said/she said evaluations to assess shared understanding after a handoff, because high fidelity transfer of information is necessary for safe transfer of responsibility. The PKAT addresses this key component of handoff quality in a novel fashion. Although high‐fidelity information transfer may correlate with receiving provider satisfaction, this relationship has not yet been explored. Future studies will evaluate the association between receiver evaluations of handoffs and PKAT agreement, as well as the relationship between PKAT performance and subsequent patient outcomes.
Limitations of this approach include the challenges inherent in reducing a complex understanding of a patient to a multiple‐item instrument. Furthermore, PKAT use may influence handoff content due to the Hawthorne effect. Although our analysis rests on the argument that agreement is the goal of a handoff, some differences of opinion within the care team enrich resilience. Regardless, to maintain continuity of care, providers need to reach agreement on the next steps in a patient's care during the handoff. Because we focused only on agreement, this approach does not compare respondents' answers to a verifiable source of truth, if it exists. Therefore, 2 respondents who agree on the wrong answer receive the same score as 2 who agree on the right answer. Other limitations include using the number of medications as a marker of handoff complexity. Finally, conducting this study in a single CICU limits generalizability. However, we believe that all PKAT items are generalizable to other pediatric CICUs, and that several are generalizable to other pediatric intensive care settings. The approach of measuring shared understanding could be generalized more widely with development of items specific to different clinical settings.
Because the PKAT can be completed and scored quickly, it could be used as a real‐time measure of quality improvement interventions such as the introduction of a standardized handoff protocol. Alternatively, provider pairs could use the PKAT as a final handoff safety check to confirm consensus before transfer of responsibility. The concept of measuring shared clinical understanding could be extended to develop similar instruments for different clinical settings.
Acknowledgements
The authors thank the CICU providers for their enthusiasm for and participation in this study. The authors also thank Margaret Wolff, MD, Newton Buchanan, and the Center for Simulation, Advanced Education and Innovation at The Children's Hospital of Philadelphia for assistance in filming the video scenarios.
Disclosures: Dr. Bates was supported in part by NICHD/T32 HD060550 and NHLBI/T32 HL07915 grant funding. Dr. Metlay was supported by a Mid‐Career Investigator Award in Patient Oriented Research (K24‐AI073957). The authors report no conflicts of interest.
- The published literature on handoffs in hospitals: deficiencies identified in an extensive review. Quality and Safety in Health Care. 2010;19(6):493–497. doi: 10.1136/qshc.2009.033480. , .
- Communication failures in patient sign‐out and suggestions for improvement: a critical incident analysis. Qual Saf Health Care. 2005;14(6):401–407. .
- Consequences of inadequate sign‐out for patient care. Arch Intern Med. 2008;168(16):1755–1760. , , , , .
- Nursing handovers: do we really need them? J Nurs Manag. 2004;12(1):37–42. , , , , , .
- Assessing clinical handover between paramedics and the trauma team. Injury. 2010;41(5):460–464. , , , et al.
- Answering questions on call: Pediatric resident physicians' use of handoffs and other resources. J Hosp Med. 2013;8(6):328–333. , , , , .
- Managing discontinuity in academic medical centers: strategies for a safe and effective resident sign‐out. J Hosp Med. 2006;1(4):257–266. , , , , .
- Patient handoffs: standardized and reliable measurement tools remain elusive. Jt Comm J Qual Patient Saf. 2010;36(2):52–61. , .
- Improving measurement in clinical handover. Qual Saf Health Care. 2009;18(4):272–276. , , , , .
- Adequacy of information transferred at resident sign‐out (inhospital handover of care): a prospective survey. Qual Saf Health Care. 2008;17(1):6–10. , , , .
- Standardized Sign‐out reduces intern perception of medical errors on the general internal medicine ward. Teach Learn Med. 2009;21(2):121–126. , , .
- Hand‐off education and evaluation: piloting the observed simulated hand‐off experience (OSHE). J Gen Intern Med. 2009;25(2):129–134. , , , et al.
- Assessing the quality of patient handoffs at care transitions. Qual Saf Health Care. 2010;19(6):1–5. , , , , .
- Implementing peer evaluation of handoffs: associations with experience and workload. J Hosp Med. 2013;8(3):132–136. , , , , .
- Development of a handoff evaluation tool for shift‐to‐shift physician handoffs: the handoff CEX. J Hosp Med. 2013;8(4):191–200. , , , et al.
- The effects of patient handoff characteristics on subsequent care: a systematic review and areas for future research. Acad Med. 2012;87(8):1105–1124. , .
- Construct validity in organizational behavior. In: Cummings LL, Stawe BM, eds. Research in Organizational Behavior. Vol 2. Greenwich, CT: JAI Press; 1980:3–43. .
- Development and validation of the medication regimen complexity index. Ann Pharmacother. 2004;38(9):1369–1376. , , , , .
- A chronic disease score from automated pharmacy data. J Clin Epidemiol. 1992;45(2):197–203. , , .
- Research electronic data capture (REDCap)—a metadata‐driven methodology and workflow process for providing translational research informatics support. J Biomed Inform. 2009;42(2):377–381. , , , , , .
- A Wilcoxon‐type test for trend. Stat Med. 1985;4(1):87–90. .
Increasing attention has been paid to the need for effective handoffs between healthcare providers since the Joint Commission identified standardized handoff protocols as a National Patient Safety Goal in 2006.1 Aside from adverse consequences for patients, poor handoffs produce provider uncertainty about care plans.[2, 3] Agreement on clinical information after a handoff is critical because a significant proportion of data is not documented in the medical record, leaving providers reliant on verbal communication.[4, 5, 6] Providers may enter the handoff with differing opinions; however, to mitigate the potential safety consequences of discontinuity of care,[7] the goal should be to achieve consensus about proposed courses of action.
Given the recent focus on improving handoffs, rigorous, outcome‐driven measures of handoff quality are clearly needed, but measuring shift‐to‐shift handoff quality has proved challenging.[8, 9] Previous studies of physician handoffs surveyed receivers for satisfaction,[10, 11] compared reported omissions to audio recordings,[3] and developed evaluation tools for receivers to rate handoffs.[12, 13, 14, 15] None directly assess the underlying goal of a handoff: the transfer of understanding from sender to receiver, enabling safe transfer of patient care responsibility.[16] We therefore chose to measure agreement on patient condition and treatment plans following handoff as an indicator of the quality of the shared clinical understanding formed. Advantages of piloting this approach in the pediatric cardiac intensive care unit (CICU) include the relatively homogenous patient population and small number of medical providers. If effective, the strategy of tool development and evaluation could be generalized to different clinical environments and provider groups.
Our aim was to develop and validate a tool to measure the level of shared clinical understanding regarding the condition and treatment plan of a CICU patient after handoff. The tool we designed was the pediatric cardiology Patient Knowledge Assessment Tool (PKAT), a brief, multiple‐item questionnaire focused on key data elements for individual CICU patients. Although variation in provider opinion helps detect diagnostic or treatment errors,[8] the PKAT is based on the assumption that achieving consensus on clinical status and the next steps of care is the goal of the handoff.
METHODS
Setting
The CICU is a 24‐bed medical and surgical unit in a 500‐bed free standing children's hospital. CICU attending physicians work 12‐ or 24‐hour shifts and supervise front line clinicians (including subspecialty fellows, nurse practitioners, and hospitalists, referred to as clinicians in this article) who work day or night shifts. Handoffs occur twice daily, with no significant differences in handoff practices between the 2 times. Attending physicians (referred to as attendings in this article) conduct parallel but separate handoffs from clinicians. All providers work exclusively in the CICU with the exception of fellows, who rotate monthly.
This study was approved by the institutional review board at The Children's Hospital of Philadelphia. All provider subjects provided informed consent. Consent for patient subjects was waived.
Development of the PKAT
We developed the PKAT content domains based on findings from previous studies,[2, 3] unpublished survey data about handoff omissions in our CICU, and CICU attending expert opinion. Pilot testing included 39 attendings and clinicians involved in 60 handoffs representing a wide variety of admissions. Participants were encouraged to share opinions on tool content and design with study staff. The PKAT (see Supporting Information, Appendix, in the online version of this article) was refined iteratively based on this feedback.
Video Simulation Testing
We used video simulation to test the PKAT for inter‐rater reliability. Nine patient handoff scenarios were written with varying levels of patient complexity and clarity of dialogue. The scenarios were filmed using the same actors and location to minimize variability aside from content. We recruited 10 experienced provider subjects (attendings and senior fellows) to minimize the effect of knowledge deficits. For each simulated handoff, subjects were encouraged to annotate a mock sign‐out sheet, which mimicked the content and format of the CICU sign‐out sheet. After watching all 9 scenarios, subjects completed a PKAT for each handoff from the perspective of the receiver based on the videotape. These standardized conditions allowed for assessment of inter‐rater reliability.
In Situ Testing
We then tested the PKAT in situ in the CICU to assess construct validity. We chose to study the morning handoff because the timing and location are more consistent. We planned to study 90 patient handoffs because the standard practice for testing a new psychometric instrument is to collect 10 observations per item.[17] On study days, 4 providers completed a PKAT for each selected handoff: the sending attending, receiving attending, sending clinician, and receiving clinician.
Study days were scheduled over 2 months to encompass a range of providers. Given the small number of attendings, we did not exclude those who had participated in video simulation testing. On study days, 6 patients were enrolled using stratified sampling to ensure adequate representation of new admissions (ie, admitted within 24 hours). The sending attending received the PKAT forms prior to the handoff. The receiving attending and clinicians received the PKAT after handoff. This difference in administration was due to logistic concerns: sending attendings requested to receive the PKATs earlier because they had to complete all 6 PKATs, whereas other providers completed 3 or fewer per day. Thus, sending attendings could complete the PKAT before or after the handoff, whereas all other participants completed the instrument after the handoff.
To test for construct validity, we gathered data on participating providers and patients, hypothesizing that PKAT agreement levels would decrease in response to less experienced providers or more complex patients. Provider characteristics included previous handoff education and amount of time worked in our CICU. Attending CICU experience was dichotomized into first year versus second or greater year. Clinician experience was dichotomized into first or second month versus third or greater month of CICU service. Each PKAT asked the handoff receiver whether he or she had recently cared for this patient or gathered information prior to handoff (eg, speaking to bedside nurse).
Recorded patient characteristics included age, length of stay, and admission type including neonatal/preoperative observation, postoperative (first 7 days after operation), prolonged postoperative (>7 days after operation), and medical (all others). In recognition of differences in handoffs during the first 24 hours of admission and the right‐skewed length of stay in the CICU, we analyzed length of stay based on the following categories: new admission (<24 hours), days 2 to 7, days 8 to 14, days 15 to 31, and >31 days. Because the number of active medications has been shown to correlate with treatment regimen complexity[18] and physician ratings of illness severity,[19] we recorded this number as a surrogate measure of patient complexity. For analytic purposes, we categorized the number of active medications into quartiles.
Provider subject characteristics and PKAT responses were collected using paper forms and entered into REDCap (Research Electronic Data Capture; REDCap Consortium,
Statistical Analysis
The primary outcome measure was the PKAT agreement level among providers evaluating the same handoff. For the reliability assessment, we calculated agreement across all providers analyzing the simulation videos, expecting that multiple providers should have high agreement for the same scenarios if the instrument has high inter‐rater reliability. For the validity assessment, we calculated agreement for each individual handoff by item and then calculated average levels of agreement for each item across provider and patient characteristics. We analyzed handoffs between attendings and clinicians separately. For items with mutually exclusive responses, simple yes/no agreement was calculated. For items requiring at least 1 response, agreement was coded when both respondents selected at least 1 response in common. For items that did not require a selection, credit was given if both subjects agreed that none of the conditions were present or if they agreed that at least 1 condition was present. In a secondary analysis, we repeated the analyses with unique sender‐receiver pair as the unit of analysis to account for correlation in the pair interaction.
Summary statistics were used to describe provider and patient characteristics. Mean rates of agreement with 95% confidence intervals were calculated for each item. The Wilcoxon rank sum test was used to compare mean results between groups (eg, attendings vs clinicians). A nonparametric test for trend, which is an extension of the Wilcoxon rank sum test,[21] was used to compare mean results across ordered categories (eg, length of stay). All tests of significance were at P<0.05 level and 2‐tailed. All statistical analysis was done using Stata 12 (StataCorp, College Station, TX).
RESULTS
Provider subject types are represented in Table 1. Handoffs between these 29 individuals resulted in 70 unique sender and receiver combinations with a median of 2 PKATs completed per unique sender‐receiver pair (range, 115). Attendings had lower rates of handoff education than clinicians (11% vs 85% for in situ testing participants, P=0.01). Attendings participating in in situ testing had worked in the CICU for a median of 3 years (range, 116 years). Clinicians participating in in situ testing had a median of 3 months of CICU experience (range, 195 months). Providers were 100% compliant with PKAT completion.
Simulation Testing, n=10 | In Situ Testing, n=29 | |
---|---|---|
| ||
Attending physicians | 40% (4) | 31% (9) |
Clinicians | 60% (6) | 69% (20) |
Clinician type | ||
Cardiology | 67% (4) | 35% (7) |
Critical care medicine | 33% (2) | 25% (5) |
CICU nurse practitioner | 25% (5) | |
Anesthesia | 5% (1) | |
Neonatology | 5% (1) | |
Hospitalist | 5% (1) |
Video Simulation Testing
Inter‐rater agreement is shown in Figure 1. Raters achieved perfect agreement for 8/9 questions on at least 1 scenario, supporting high inter‐rater reliability for these items. Some items had particularly high reliability. For example, on item 3, subjects achieved perfect agreement for 5/9 scenarios, making 1 both the median and maximum value. Because item 7 (barriers to transfer) did not demonstrate high inter‐rater agreement, we excluded it from the in situ analysis.
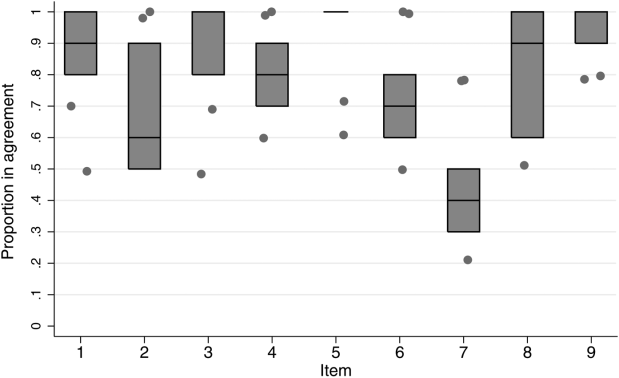
In Situ Testing
Characteristics of patients whose handoffs were selected for in situ testing are listed in Table 2. Because some patients were selected on multiple study days, these 90 handoffs represented 58 unique patients. These 58 patients are representative of the CICU population (data not shown). The number of handoffs studied per patient ranged from 1 to 7 (median 1). A total of 19 patients were included in the study more than once; 13 were included twice.
Characteristic | Categories | Percentage |
---|---|---|
| ||
Age | <1 month | 30 |
112 months | 34 | |
112 years | 28 | |
1318 years | 6 | |
>18 years | 2 | |
Type of admission | Postnatal observation/preoperative | 20 |
Postoperative | 29 | |
Prolonged postoperative (>7 days) | 33 | |
Other admission | 18 | |
CICU days | 1 | 31 |
27 | 22 | |
814 | 10 | |
1531 | 13 | |
>31 | 23 | |
Active medications | <8 | 26 |
811 | 26 | |
1218 | 26 | |
>18 | 23 |
Rates of agreement between handoff pairs, stratified by attending versus clinician, are shown in Table 3. Overall mean levels of agreement ranged from 0.41 to 0.87 (median 0.77). Except for the ratio of pulmonary to systemic blood flow question, there were no significant differences in agreement between attendings as compared to clinicians. When this analysis was repeated with unique sender‐receiver pair as the unit of analysis to account for within‐pair clustering, we obtained qualitatively similar results (data not shown).
PKAT Item | Agreement Level | ||||
---|---|---|---|---|---|
Attending Physician Pair | Clinician Pair | Pa | |||
Mean | 95% CI | Mean | 95% CI | ||
| |||||
Clinical condition | 0.71 | 0.620.81 | 0.78 | 0.690.87 | 0.31 |
Cardiovascular plan | 0.76 | 0.670.85 | 0.68 | 0.580.78 | 0.25 |
Respiratory plan | 0.67 | 0.580.78 | 0.76 | 0.670.85 | 0.26 |
Source of pulmonary blood flow | 0.83 | 0.750.91 | 0.87 | 0.800.94 | 0.53 |
Ratio of pulmonary to systemic flow | 0.67 | 0.570.77 | 0.41 | 0.310.51 | <0.01 |
Anticoagulation indication | 0.79 | 0.700.87 | 0.77 | 0.680.86 | 0.72 |
Active cardiovascular issues | 0.87 | 0.800.94 | 0.76 | 0.670.85 | 0.06 |
Active noncardiovascular issues | 0.80 | 0.720.88 | 0.78 | 0.690.87 | 0.72 |
Both length of stay and increasing number of medications affected agreement levels for PKAT items (Table 4). Increasing length of stay correlated directly with agreement on cardiovascular plan and ratio of pulmonary to systemic flow and inversely with indication for anticoagulation. Increasing number of medications had an inverse correlation with agreement on indication for anticoagulation, active cardiovascular issues, and active noncardiovascular issues.
Item | CICU LOS | No. of Active Medications | |||||||||
---|---|---|---|---|---|---|---|---|---|---|---|
1 Day (n=56) | 27 Days (n=40) | 814 Days (n=18) | 1531 Days (n=24) | >31 Days (n=42) | Pa | 8 (n=46) | 811 (n=46) | 1218 (n=46) | >18 (n=42) | Pa | |
| |||||||||||
Clinical condition | 0.75 | 0.63 | 0.78 | 0.83 | 0.79 | 0.29 | 0.71 | 0.70 | 0.78 | 0.79 | 0.32 |
Cardiovascular plan | 0.59 | 0.73 | 0.67 | 0.79 | 0.86 | <0.01 | 0.63 | 0.72 | 0.63 | 0.81 | 0.16 |
Respiratory plan | 0.68 | 0.78 | 0.61 | 0.83 | 0.69 | 0.79 | 0.67 | 0.72 | 0.78 | 0.69 | 0.68 |
Source of pulmonary blood flow | 0.93 | 0.75 | 0.72 | 0.96 | 0.83 | 0.63 | 0.72 | 0.91 | 0.98 | 0.79 | 0.22 |
Ratio of pulmonary to systemic flow | 0.45 | 0.40 | 0.67 | 0.75 | 0.62 | 0.01 | 0.46 | 0.52 | 0.52 | 0.67 | 0.06 |
Anticoagulation indication | 0.89 | 0.83 | 0.89 | 0.67 | 0.60 | <0.01 | 0.93 | 0.78 | 0.76 | 0.62 | <0.01 |
Active cardiovascular issues | 0.86 | 0.78 | 0.72 | 0.92 | 0.76 | 0.52 | 0.87 | 0.76 | 0.54 | 0.55 | <0.01 |
Active noncardiovascular issues | 0.86 | 0.80 | 0.72 | 0.75 | 0.74 | 0.12 | 0.83 | 0.83 | 0.76 | 0.52 | <0.01 |
In contrast, there were no significant differences in item agreement levels based on provider characteristics, including experience, handoff education, prehandoff preparation, or continuity (data not shown).
CONCLUSIONS
Our results provide initial evidence of reliability and validity of scores for a novel tool, the PKAT, designed to assess providers' shared clinical understanding of a pediatric CICU patient's condition and treatment plan. Because this information should be mutually understood following any handoff, we believe this tool or similar agreement assessments could be used to measure handoff quality across a range of clinical settings. Under the standardized conditions of video simulation, experienced CICU providers achieved high levels of agreement on the PKAT, demonstrating inter‐rater reliability. In situ testing results suggest that the PKAT can validly identify differences in understanding between providers for both routine and complex patients.
The achievement of 100% compliance with in situ testing demonstrates that this type of tool can feasibly be used in a real‐time clinical environment. As expected, mean agreement levels in situ were lower than levels achieved in video simulation. By item, mean levels of agreement for attending and clinician pairs were similar.
Our assessment of PKAT validity demonstrated mixed results. On the one hand, PKAT agreement did not vary significantly by any measured provider characteristics. Consistent with the lack of difference between attendings and clinicians, more experienced providers in both groups did not achieve higher levels of agreement. This finding is surprising, and may illustrate that unmeasured provider characteristics, such as content knowledge, obscure the effects of experience or other measured variables on agreement levels. Alternatively, providing the PKAT to the sending attending prior to the handoff, rather than afterward as for the receiving attendings and clinicians, might have artificially lowered attending agreement levels, concealing a difference due to experience.
On the other hand, construct validity of several items was supported by the difference in agreement levels based on patient characteristics. Agreement levels varied on 5/8 questions as patients became more complex, either defined by length of stay or number of medications. These differences show that agreement on PKAT items responds to changes in handoff complexity, a form of construct validity. Furthermore, these findings suggest that handoffs of more chronic or complex patients may require more attention for components prone to disagreement in these settings. Although complexity and longer length of stay are nonmodifiable risk factors, identifying these handoffs as more susceptible to disagreement provides potential targets for intervention.
It is important to move beyond he said/she said evaluations to assess shared understanding after a handoff, because high fidelity transfer of information is necessary for safe transfer of responsibility. The PKAT addresses this key component of handoff quality in a novel fashion. Although high‐fidelity information transfer may correlate with receiving provider satisfaction, this relationship has not yet been explored. Future studies will evaluate the association between receiver evaluations of handoffs and PKAT agreement, as well as the relationship between PKAT performance and subsequent patient outcomes.
Limitations of this approach include the challenges inherent in reducing a complex understanding of a patient to a multiple‐item instrument. Furthermore, PKAT use may influence handoff content due to the Hawthorne effect. Although our analysis rests on the argument that agreement is the goal of a handoff, some differences of opinion within the care team enrich resilience. Regardless, to maintain continuity of care, providers need to reach agreement on the next steps in a patient's care during the handoff. Because we focused only on agreement, this approach does not compare respondents' answers to a verifiable source of truth, if it exists. Therefore, 2 respondents who agree on the wrong answer receive the same score as 2 who agree on the right answer. Other limitations include using the number of medications as a marker of handoff complexity. Finally, conducting this study in a single CICU limits generalizability. However, we believe that all PKAT items are generalizable to other pediatric CICUs, and that several are generalizable to other pediatric intensive care settings. The approach of measuring shared understanding could be generalized more widely with development of items specific to different clinical settings.
Because the PKAT can be completed and scored quickly, it could be used as a real‐time measure of quality improvement interventions such as the introduction of a standardized handoff protocol. Alternatively, provider pairs could use the PKAT as a final handoff safety check to confirm consensus before transfer of responsibility. The concept of measuring shared clinical understanding could be extended to develop similar instruments for different clinical settings.
Acknowledgements
The authors thank the CICU providers for their enthusiasm for and participation in this study. The authors also thank Margaret Wolff, MD, Newton Buchanan, and the Center for Simulation, Advanced Education and Innovation at The Children's Hospital of Philadelphia for assistance in filming the video scenarios.
Disclosures: Dr. Bates was supported in part by NICHD/T32 HD060550 and NHLBI/T32 HL07915 grant funding. Dr. Metlay was supported by a Mid‐Career Investigator Award in Patient Oriented Research (K24‐AI073957). The authors report no conflicts of interest.
Increasing attention has been paid to the need for effective handoffs between healthcare providers since the Joint Commission identified standardized handoff protocols as a National Patient Safety Goal in 2006.1 Aside from adverse consequences for patients, poor handoffs produce provider uncertainty about care plans.[2, 3] Agreement on clinical information after a handoff is critical because a significant proportion of data is not documented in the medical record, leaving providers reliant on verbal communication.[4, 5, 6] Providers may enter the handoff with differing opinions; however, to mitigate the potential safety consequences of discontinuity of care,[7] the goal should be to achieve consensus about proposed courses of action.
Given the recent focus on improving handoffs, rigorous, outcome‐driven measures of handoff quality are clearly needed, but measuring shift‐to‐shift handoff quality has proved challenging.[8, 9] Previous studies of physician handoffs surveyed receivers for satisfaction,[10, 11] compared reported omissions to audio recordings,[3] and developed evaluation tools for receivers to rate handoffs.[12, 13, 14, 15] None directly assess the underlying goal of a handoff: the transfer of understanding from sender to receiver, enabling safe transfer of patient care responsibility.[16] We therefore chose to measure agreement on patient condition and treatment plans following handoff as an indicator of the quality of the shared clinical understanding formed. Advantages of piloting this approach in the pediatric cardiac intensive care unit (CICU) include the relatively homogenous patient population and small number of medical providers. If effective, the strategy of tool development and evaluation could be generalized to different clinical environments and provider groups.
Our aim was to develop and validate a tool to measure the level of shared clinical understanding regarding the condition and treatment plan of a CICU patient after handoff. The tool we designed was the pediatric cardiology Patient Knowledge Assessment Tool (PKAT), a brief, multiple‐item questionnaire focused on key data elements for individual CICU patients. Although variation in provider opinion helps detect diagnostic or treatment errors,[8] the PKAT is based on the assumption that achieving consensus on clinical status and the next steps of care is the goal of the handoff.
METHODS
Setting
The CICU is a 24‐bed medical and surgical unit in a 500‐bed free standing children's hospital. CICU attending physicians work 12‐ or 24‐hour shifts and supervise front line clinicians (including subspecialty fellows, nurse practitioners, and hospitalists, referred to as clinicians in this article) who work day or night shifts. Handoffs occur twice daily, with no significant differences in handoff practices between the 2 times. Attending physicians (referred to as attendings in this article) conduct parallel but separate handoffs from clinicians. All providers work exclusively in the CICU with the exception of fellows, who rotate monthly.
This study was approved by the institutional review board at The Children's Hospital of Philadelphia. All provider subjects provided informed consent. Consent for patient subjects was waived.
Development of the PKAT
We developed the PKAT content domains based on findings from previous studies,[2, 3] unpublished survey data about handoff omissions in our CICU, and CICU attending expert opinion. Pilot testing included 39 attendings and clinicians involved in 60 handoffs representing a wide variety of admissions. Participants were encouraged to share opinions on tool content and design with study staff. The PKAT (see Supporting Information, Appendix, in the online version of this article) was refined iteratively based on this feedback.
Video Simulation Testing
We used video simulation to test the PKAT for inter‐rater reliability. Nine patient handoff scenarios were written with varying levels of patient complexity and clarity of dialogue. The scenarios were filmed using the same actors and location to minimize variability aside from content. We recruited 10 experienced provider subjects (attendings and senior fellows) to minimize the effect of knowledge deficits. For each simulated handoff, subjects were encouraged to annotate a mock sign‐out sheet, which mimicked the content and format of the CICU sign‐out sheet. After watching all 9 scenarios, subjects completed a PKAT for each handoff from the perspective of the receiver based on the videotape. These standardized conditions allowed for assessment of inter‐rater reliability.
In Situ Testing
We then tested the PKAT in situ in the CICU to assess construct validity. We chose to study the morning handoff because the timing and location are more consistent. We planned to study 90 patient handoffs because the standard practice for testing a new psychometric instrument is to collect 10 observations per item.[17] On study days, 4 providers completed a PKAT for each selected handoff: the sending attending, receiving attending, sending clinician, and receiving clinician.
Study days were scheduled over 2 months to encompass a range of providers. Given the small number of attendings, we did not exclude those who had participated in video simulation testing. On study days, 6 patients were enrolled using stratified sampling to ensure adequate representation of new admissions (ie, admitted within 24 hours). The sending attending received the PKAT forms prior to the handoff. The receiving attending and clinicians received the PKAT after handoff. This difference in administration was due to logistic concerns: sending attendings requested to receive the PKATs earlier because they had to complete all 6 PKATs, whereas other providers completed 3 or fewer per day. Thus, sending attendings could complete the PKAT before or after the handoff, whereas all other participants completed the instrument after the handoff.
To test for construct validity, we gathered data on participating providers and patients, hypothesizing that PKAT agreement levels would decrease in response to less experienced providers or more complex patients. Provider characteristics included previous handoff education and amount of time worked in our CICU. Attending CICU experience was dichotomized into first year versus second or greater year. Clinician experience was dichotomized into first or second month versus third or greater month of CICU service. Each PKAT asked the handoff receiver whether he or she had recently cared for this patient or gathered information prior to handoff (eg, speaking to bedside nurse).
Recorded patient characteristics included age, length of stay, and admission type including neonatal/preoperative observation, postoperative (first 7 days after operation), prolonged postoperative (>7 days after operation), and medical (all others). In recognition of differences in handoffs during the first 24 hours of admission and the right‐skewed length of stay in the CICU, we analyzed length of stay based on the following categories: new admission (<24 hours), days 2 to 7, days 8 to 14, days 15 to 31, and >31 days. Because the number of active medications has been shown to correlate with treatment regimen complexity[18] and physician ratings of illness severity,[19] we recorded this number as a surrogate measure of patient complexity. For analytic purposes, we categorized the number of active medications into quartiles.
Provider subject characteristics and PKAT responses were collected using paper forms and entered into REDCap (Research Electronic Data Capture; REDCap Consortium,
Statistical Analysis
The primary outcome measure was the PKAT agreement level among providers evaluating the same handoff. For the reliability assessment, we calculated agreement across all providers analyzing the simulation videos, expecting that multiple providers should have high agreement for the same scenarios if the instrument has high inter‐rater reliability. For the validity assessment, we calculated agreement for each individual handoff by item and then calculated average levels of agreement for each item across provider and patient characteristics. We analyzed handoffs between attendings and clinicians separately. For items with mutually exclusive responses, simple yes/no agreement was calculated. For items requiring at least 1 response, agreement was coded when both respondents selected at least 1 response in common. For items that did not require a selection, credit was given if both subjects agreed that none of the conditions were present or if they agreed that at least 1 condition was present. In a secondary analysis, we repeated the analyses with unique sender‐receiver pair as the unit of analysis to account for correlation in the pair interaction.
Summary statistics were used to describe provider and patient characteristics. Mean rates of agreement with 95% confidence intervals were calculated for each item. The Wilcoxon rank sum test was used to compare mean results between groups (eg, attendings vs clinicians). A nonparametric test for trend, which is an extension of the Wilcoxon rank sum test,[21] was used to compare mean results across ordered categories (eg, length of stay). All tests of significance were at P<0.05 level and 2‐tailed. All statistical analysis was done using Stata 12 (StataCorp, College Station, TX).
RESULTS
Provider subject types are represented in Table 1. Handoffs between these 29 individuals resulted in 70 unique sender and receiver combinations with a median of 2 PKATs completed per unique sender‐receiver pair (range, 115). Attendings had lower rates of handoff education than clinicians (11% vs 85% for in situ testing participants, P=0.01). Attendings participating in in situ testing had worked in the CICU for a median of 3 years (range, 116 years). Clinicians participating in in situ testing had a median of 3 months of CICU experience (range, 195 months). Providers were 100% compliant with PKAT completion.
Simulation Testing, n=10 | In Situ Testing, n=29 | |
---|---|---|
| ||
Attending physicians | 40% (4) | 31% (9) |
Clinicians | 60% (6) | 69% (20) |
Clinician type | ||
Cardiology | 67% (4) | 35% (7) |
Critical care medicine | 33% (2) | 25% (5) |
CICU nurse practitioner | 25% (5) | |
Anesthesia | 5% (1) | |
Neonatology | 5% (1) | |
Hospitalist | 5% (1) |
Video Simulation Testing
Inter‐rater agreement is shown in Figure 1. Raters achieved perfect agreement for 8/9 questions on at least 1 scenario, supporting high inter‐rater reliability for these items. Some items had particularly high reliability. For example, on item 3, subjects achieved perfect agreement for 5/9 scenarios, making 1 both the median and maximum value. Because item 7 (barriers to transfer) did not demonstrate high inter‐rater agreement, we excluded it from the in situ analysis.
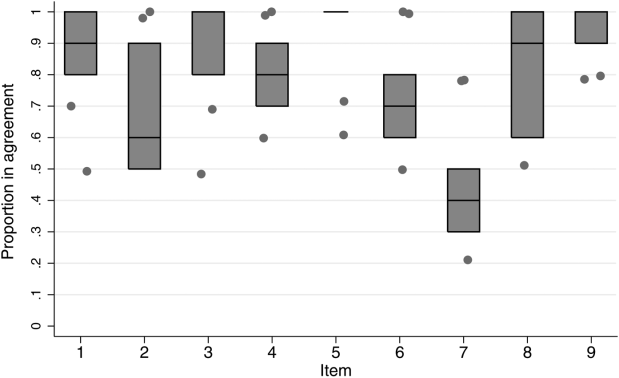
In Situ Testing
Characteristics of patients whose handoffs were selected for in situ testing are listed in Table 2. Because some patients were selected on multiple study days, these 90 handoffs represented 58 unique patients. These 58 patients are representative of the CICU population (data not shown). The number of handoffs studied per patient ranged from 1 to 7 (median 1). A total of 19 patients were included in the study more than once; 13 were included twice.
Characteristic | Categories | Percentage |
---|---|---|
| ||
Age | <1 month | 30 |
112 months | 34 | |
112 years | 28 | |
1318 years | 6 | |
>18 years | 2 | |
Type of admission | Postnatal observation/preoperative | 20 |
Postoperative | 29 | |
Prolonged postoperative (>7 days) | 33 | |
Other admission | 18 | |
CICU days | 1 | 31 |
27 | 22 | |
814 | 10 | |
1531 | 13 | |
>31 | 23 | |
Active medications | <8 | 26 |
811 | 26 | |
1218 | 26 | |
>18 | 23 |
Rates of agreement between handoff pairs, stratified by attending versus clinician, are shown in Table 3. Overall mean levels of agreement ranged from 0.41 to 0.87 (median 0.77). Except for the ratio of pulmonary to systemic blood flow question, there were no significant differences in agreement between attendings as compared to clinicians. When this analysis was repeated with unique sender‐receiver pair as the unit of analysis to account for within‐pair clustering, we obtained qualitatively similar results (data not shown).
PKAT Item | Agreement Level | ||||
---|---|---|---|---|---|
Attending Physician Pair | Clinician Pair | Pa | |||
Mean | 95% CI | Mean | 95% CI | ||
| |||||
Clinical condition | 0.71 | 0.620.81 | 0.78 | 0.690.87 | 0.31 |
Cardiovascular plan | 0.76 | 0.670.85 | 0.68 | 0.580.78 | 0.25 |
Respiratory plan | 0.67 | 0.580.78 | 0.76 | 0.670.85 | 0.26 |
Source of pulmonary blood flow | 0.83 | 0.750.91 | 0.87 | 0.800.94 | 0.53 |
Ratio of pulmonary to systemic flow | 0.67 | 0.570.77 | 0.41 | 0.310.51 | <0.01 |
Anticoagulation indication | 0.79 | 0.700.87 | 0.77 | 0.680.86 | 0.72 |
Active cardiovascular issues | 0.87 | 0.800.94 | 0.76 | 0.670.85 | 0.06 |
Active noncardiovascular issues | 0.80 | 0.720.88 | 0.78 | 0.690.87 | 0.72 |
Both length of stay and increasing number of medications affected agreement levels for PKAT items (Table 4). Increasing length of stay correlated directly with agreement on cardiovascular plan and ratio of pulmonary to systemic flow and inversely with indication for anticoagulation. Increasing number of medications had an inverse correlation with agreement on indication for anticoagulation, active cardiovascular issues, and active noncardiovascular issues.
Item | CICU LOS | No. of Active Medications | |||||||||
---|---|---|---|---|---|---|---|---|---|---|---|
1 Day (n=56) | 27 Days (n=40) | 814 Days (n=18) | 1531 Days (n=24) | >31 Days (n=42) | Pa | 8 (n=46) | 811 (n=46) | 1218 (n=46) | >18 (n=42) | Pa | |
| |||||||||||
Clinical condition | 0.75 | 0.63 | 0.78 | 0.83 | 0.79 | 0.29 | 0.71 | 0.70 | 0.78 | 0.79 | 0.32 |
Cardiovascular plan | 0.59 | 0.73 | 0.67 | 0.79 | 0.86 | <0.01 | 0.63 | 0.72 | 0.63 | 0.81 | 0.16 |
Respiratory plan | 0.68 | 0.78 | 0.61 | 0.83 | 0.69 | 0.79 | 0.67 | 0.72 | 0.78 | 0.69 | 0.68 |
Source of pulmonary blood flow | 0.93 | 0.75 | 0.72 | 0.96 | 0.83 | 0.63 | 0.72 | 0.91 | 0.98 | 0.79 | 0.22 |
Ratio of pulmonary to systemic flow | 0.45 | 0.40 | 0.67 | 0.75 | 0.62 | 0.01 | 0.46 | 0.52 | 0.52 | 0.67 | 0.06 |
Anticoagulation indication | 0.89 | 0.83 | 0.89 | 0.67 | 0.60 | <0.01 | 0.93 | 0.78 | 0.76 | 0.62 | <0.01 |
Active cardiovascular issues | 0.86 | 0.78 | 0.72 | 0.92 | 0.76 | 0.52 | 0.87 | 0.76 | 0.54 | 0.55 | <0.01 |
Active noncardiovascular issues | 0.86 | 0.80 | 0.72 | 0.75 | 0.74 | 0.12 | 0.83 | 0.83 | 0.76 | 0.52 | <0.01 |
In contrast, there were no significant differences in item agreement levels based on provider characteristics, including experience, handoff education, prehandoff preparation, or continuity (data not shown).
CONCLUSIONS
Our results provide initial evidence of reliability and validity of scores for a novel tool, the PKAT, designed to assess providers' shared clinical understanding of a pediatric CICU patient's condition and treatment plan. Because this information should be mutually understood following any handoff, we believe this tool or similar agreement assessments could be used to measure handoff quality across a range of clinical settings. Under the standardized conditions of video simulation, experienced CICU providers achieved high levels of agreement on the PKAT, demonstrating inter‐rater reliability. In situ testing results suggest that the PKAT can validly identify differences in understanding between providers for both routine and complex patients.
The achievement of 100% compliance with in situ testing demonstrates that this type of tool can feasibly be used in a real‐time clinical environment. As expected, mean agreement levels in situ were lower than levels achieved in video simulation. By item, mean levels of agreement for attending and clinician pairs were similar.
Our assessment of PKAT validity demonstrated mixed results. On the one hand, PKAT agreement did not vary significantly by any measured provider characteristics. Consistent with the lack of difference between attendings and clinicians, more experienced providers in both groups did not achieve higher levels of agreement. This finding is surprising, and may illustrate that unmeasured provider characteristics, such as content knowledge, obscure the effects of experience or other measured variables on agreement levels. Alternatively, providing the PKAT to the sending attending prior to the handoff, rather than afterward as for the receiving attendings and clinicians, might have artificially lowered attending agreement levels, concealing a difference due to experience.
On the other hand, construct validity of several items was supported by the difference in agreement levels based on patient characteristics. Agreement levels varied on 5/8 questions as patients became more complex, either defined by length of stay or number of medications. These differences show that agreement on PKAT items responds to changes in handoff complexity, a form of construct validity. Furthermore, these findings suggest that handoffs of more chronic or complex patients may require more attention for components prone to disagreement in these settings. Although complexity and longer length of stay are nonmodifiable risk factors, identifying these handoffs as more susceptible to disagreement provides potential targets for intervention.
It is important to move beyond he said/she said evaluations to assess shared understanding after a handoff, because high fidelity transfer of information is necessary for safe transfer of responsibility. The PKAT addresses this key component of handoff quality in a novel fashion. Although high‐fidelity information transfer may correlate with receiving provider satisfaction, this relationship has not yet been explored. Future studies will evaluate the association between receiver evaluations of handoffs and PKAT agreement, as well as the relationship between PKAT performance and subsequent patient outcomes.
Limitations of this approach include the challenges inherent in reducing a complex understanding of a patient to a multiple‐item instrument. Furthermore, PKAT use may influence handoff content due to the Hawthorne effect. Although our analysis rests on the argument that agreement is the goal of a handoff, some differences of opinion within the care team enrich resilience. Regardless, to maintain continuity of care, providers need to reach agreement on the next steps in a patient's care during the handoff. Because we focused only on agreement, this approach does not compare respondents' answers to a verifiable source of truth, if it exists. Therefore, 2 respondents who agree on the wrong answer receive the same score as 2 who agree on the right answer. Other limitations include using the number of medications as a marker of handoff complexity. Finally, conducting this study in a single CICU limits generalizability. However, we believe that all PKAT items are generalizable to other pediatric CICUs, and that several are generalizable to other pediatric intensive care settings. The approach of measuring shared understanding could be generalized more widely with development of items specific to different clinical settings.
Because the PKAT can be completed and scored quickly, it could be used as a real‐time measure of quality improvement interventions such as the introduction of a standardized handoff protocol. Alternatively, provider pairs could use the PKAT as a final handoff safety check to confirm consensus before transfer of responsibility. The concept of measuring shared clinical understanding could be extended to develop similar instruments for different clinical settings.
Acknowledgements
The authors thank the CICU providers for their enthusiasm for and participation in this study. The authors also thank Margaret Wolff, MD, Newton Buchanan, and the Center for Simulation, Advanced Education and Innovation at The Children's Hospital of Philadelphia for assistance in filming the video scenarios.
Disclosures: Dr. Bates was supported in part by NICHD/T32 HD060550 and NHLBI/T32 HL07915 grant funding. Dr. Metlay was supported by a Mid‐Career Investigator Award in Patient Oriented Research (K24‐AI073957). The authors report no conflicts of interest.
- The published literature on handoffs in hospitals: deficiencies identified in an extensive review. Quality and Safety in Health Care. 2010;19(6):493–497. doi: 10.1136/qshc.2009.033480. , .
- Communication failures in patient sign‐out and suggestions for improvement: a critical incident analysis. Qual Saf Health Care. 2005;14(6):401–407. .
- Consequences of inadequate sign‐out for patient care. Arch Intern Med. 2008;168(16):1755–1760. , , , , .
- Nursing handovers: do we really need them? J Nurs Manag. 2004;12(1):37–42. , , , , , .
- Assessing clinical handover between paramedics and the trauma team. Injury. 2010;41(5):460–464. , , , et al.
- Answering questions on call: Pediatric resident physicians' use of handoffs and other resources. J Hosp Med. 2013;8(6):328–333. , , , , .
- Managing discontinuity in academic medical centers: strategies for a safe and effective resident sign‐out. J Hosp Med. 2006;1(4):257–266. , , , , .
- Patient handoffs: standardized and reliable measurement tools remain elusive. Jt Comm J Qual Patient Saf. 2010;36(2):52–61. , .
- Improving measurement in clinical handover. Qual Saf Health Care. 2009;18(4):272–276. , , , , .
- Adequacy of information transferred at resident sign‐out (inhospital handover of care): a prospective survey. Qual Saf Health Care. 2008;17(1):6–10. , , , .
- Standardized Sign‐out reduces intern perception of medical errors on the general internal medicine ward. Teach Learn Med. 2009;21(2):121–126. , , .
- Hand‐off education and evaluation: piloting the observed simulated hand‐off experience (OSHE). J Gen Intern Med. 2009;25(2):129–134. , , , et al.
- Assessing the quality of patient handoffs at care transitions. Qual Saf Health Care. 2010;19(6):1–5. , , , , .
- Implementing peer evaluation of handoffs: associations with experience and workload. J Hosp Med. 2013;8(3):132–136. , , , , .
- Development of a handoff evaluation tool for shift‐to‐shift physician handoffs: the handoff CEX. J Hosp Med. 2013;8(4):191–200. , , , et al.
- The effects of patient handoff characteristics on subsequent care: a systematic review and areas for future research. Acad Med. 2012;87(8):1105–1124. , .
- Construct validity in organizational behavior. In: Cummings LL, Stawe BM, eds. Research in Organizational Behavior. Vol 2. Greenwich, CT: JAI Press; 1980:3–43. .
- Development and validation of the medication regimen complexity index. Ann Pharmacother. 2004;38(9):1369–1376. , , , , .
- A chronic disease score from automated pharmacy data. J Clin Epidemiol. 1992;45(2):197–203. , , .
- Research electronic data capture (REDCap)—a metadata‐driven methodology and workflow process for providing translational research informatics support. J Biomed Inform. 2009;42(2):377–381. , , , , , .
- A Wilcoxon‐type test for trend. Stat Med. 1985;4(1):87–90. .
- The published literature on handoffs in hospitals: deficiencies identified in an extensive review. Quality and Safety in Health Care. 2010;19(6):493–497. doi: 10.1136/qshc.2009.033480. , .
- Communication failures in patient sign‐out and suggestions for improvement: a critical incident analysis. Qual Saf Health Care. 2005;14(6):401–407. .
- Consequences of inadequate sign‐out for patient care. Arch Intern Med. 2008;168(16):1755–1760. , , , , .
- Nursing handovers: do we really need them? J Nurs Manag. 2004;12(1):37–42. , , , , , .
- Assessing clinical handover between paramedics and the trauma team. Injury. 2010;41(5):460–464. , , , et al.
- Answering questions on call: Pediatric resident physicians' use of handoffs and other resources. J Hosp Med. 2013;8(6):328–333. , , , , .
- Managing discontinuity in academic medical centers: strategies for a safe and effective resident sign‐out. J Hosp Med. 2006;1(4):257–266. , , , , .
- Patient handoffs: standardized and reliable measurement tools remain elusive. Jt Comm J Qual Patient Saf. 2010;36(2):52–61. , .
- Improving measurement in clinical handover. Qual Saf Health Care. 2009;18(4):272–276. , , , , .
- Adequacy of information transferred at resident sign‐out (inhospital handover of care): a prospective survey. Qual Saf Health Care. 2008;17(1):6–10. , , , .
- Standardized Sign‐out reduces intern perception of medical errors on the general internal medicine ward. Teach Learn Med. 2009;21(2):121–126. , , .
- Hand‐off education and evaluation: piloting the observed simulated hand‐off experience (OSHE). J Gen Intern Med. 2009;25(2):129–134. , , , et al.
- Assessing the quality of patient handoffs at care transitions. Qual Saf Health Care. 2010;19(6):1–5. , , , , .
- Implementing peer evaluation of handoffs: associations with experience and workload. J Hosp Med. 2013;8(3):132–136. , , , , .
- Development of a handoff evaluation tool for shift‐to‐shift physician handoffs: the handoff CEX. J Hosp Med. 2013;8(4):191–200. , , , et al.
- The effects of patient handoff characteristics on subsequent care: a systematic review and areas for future research. Acad Med. 2012;87(8):1105–1124. , .
- Construct validity in organizational behavior. In: Cummings LL, Stawe BM, eds. Research in Organizational Behavior. Vol 2. Greenwich, CT: JAI Press; 1980:3–43. .
- Development and validation of the medication regimen complexity index. Ann Pharmacother. 2004;38(9):1369–1376. , , , , .
- A chronic disease score from automated pharmacy data. J Clin Epidemiol. 1992;45(2):197–203. , , .
- Research electronic data capture (REDCap)—a metadata‐driven methodology and workflow process for providing translational research informatics support. J Biomed Inform. 2009;42(2):377–381. , , , , , .
- A Wilcoxon‐type test for trend. Stat Med. 1985;4(1):87–90. .
© 2014 Society of Hospital Medicine
New Strategies to Combat an Old Foe
In the early part of the 20th century, pneumonia was a leading causes of death, particularly among older adults, for whom Osler termed it the Captain of the Men of Death.[1] Mortality rates from severe (bacteremic) pneumonia were typically 80% to 90%, and the introduction of antibacterial therapy in the 1940s reduced that mortality to 10% to 20%. However, as pointed out by Austrian and Gold in a landmark paper in the 1950s, mortality for patients dying within the first 4 to 5 days was not reduced in the postantibiotic era.[2] The survival rates for patients with severe community‐acquired pneumonia minimally improved over the ensuing 50 years, despite the introduction of numerous new antimicrobial drugs and other medical interventions.
One promising area for therapeutic intervention relates to the potential adverse effects of the host inflammatory response in the setting of pneumonia. A growing body of literature supports the conclusion that the window of optimal host response may be relatively narrow. Too little response and patients quickly succumb to overwhelming sepsis. Too much response and a patient's hyperactivated inflammatory system can set off a cascade of secondary events, triggering events such as acute lung injury or ischemic heart disease.[3] Studies have also established that the level of inflammation, as measured by biomarkers such as C‐reactive protein, tumor necrosis factor, and interleukins, can identify patients at increased risk of adverse outcomes.[4] Thus, it is logical to ask whether immune modulating therapies can improve outcomes for these patients.
In this issue of the Journal of Hospital Medicine, Shafiq and colleagues completed a systematic review and meta‐analysis of corticosteroid therapy for patients with pneumonia.[5] Updating prior reviews, they included 8 randomized controlled trials, all of which consisted of low‐dose, systemic, steroid therapy as the intervention and standard care as the control arm. The overall quality of the included studies was judged moderate, and the overall size of the pooled data was only 1119 patients. In their analysis, adjunctive steroid therapy did not reduce in‐hospital mortality, with 4 studies demonstrating effect sizes suggesting benefit, 3 studies demonstrating no benefit or harm, and 1 study favoring the nonsteroid arm. In these situations with grossly heterogeneous study results, it seems prudent to avoid overly interpreting pooled results, even if statistical tests for heterogeneity are nonsignificant. The investigators also reported a range of secondary outcomes, noting that hospital length of stay was significantly reduced in the pooled steroid treated arms.
The overall negative finding is clearly disappointing at a time when clinicians are looking for new treatments to improve outcomes for these patients. Pneumonia is a heterogeneous disorder, representing a wide range of microbial pathogens and underlying host risk factors. Current treatment guidelines for patients with community‐acquired pneumonia are largely empirical and do not focus on pathogen identification, host risk factor analysis, or biomarker distributions to select antimicrobial therapy.[6] In this regard, despite being 1 of the oldest conditions for which we have published guidelines for treatment, the treatment approach for pneumonia remains quite antiquated, ignoring recent advances in the incorporation of personalized treatment strategies for other illnesses. We may have reached the limits of one‐size‐fits‐all treatment strategies for hospitalized adults with community‐acquired pneumonia. To improve outcomes further, we need to understand the heterogeneity of the disorder and tailor therapies at an individual level. Rapid point‐of‐care tests for pathogens and host response offer the most promising approach toward this strategy.
It is notable that the majority of studies focus on in‐hospital mortality, even though the impact of steroid therapy may be observed over a longer period of follow‐up. Moreover, although mortality is clearly a relevant outcome, it is not the only patient‐centered outcome of importance. However, other outcomes that are typically assessed, such as length of hospitalization and cost, are not patient‐centered outcomes. These are process measures that reflect physician judgment as much as any patient response to treatment. We need to move the field forward by embracing patient outcomes beyond mortality to optimally evaluate new treatment strategies, particularly because the majority of patients will survive hospitalization for the illness. These outcomes would include time to resolution of major symptoms, such as cough and fatigue, and functional outcomes, including return to work and usual activities. Future comparative efficacy and effectiveness studies in pneumonia need to consider a much wider range of true patient outcomes.[7]
It is increasingly fashionable to adopt cross‐disease approaches toward optimizing patient care, particularly in the hospital. Important initiatives that aim to reduce hospital injuries and improve transitions of care are relatively agnostic to specific disease states. Much of the research agenda of hospital medicine avoids a disease‐specific focus, assuming such disease‐specific approaches are the domain of specialists. Yet, it is worth remembering that much of the progress for medical care can be traced to traditional considerations of disease pathophysiology and empirical studies of risk factors and treatments for specific disease. Hospitalists remain at the front line in dealing with most of the common illnesses that afflict patients. Battling those conditions 1 at a time should be an important component of the broader hospitalist research agenda. One hundred years after Osler charged the medical community to identify new strategies for treating an old enemy, we are still struggling to win the battle.
Disclosure
This work was supported in part by K24‐AI073957 (JPM) from the National Institute of Allergy and Infectious Diseases, National Institutes of Health. The author has no conflicts of interest to report.
- The Principles and Practices of Medicine. 7th ed. New York, London: D. Appleton and Co.; 1909. .
- Pneumococcal bacteremia with special reference to bacteremic pneumococcal pneumonia. Ann Intern Med. 1964;60:759–776. , .
- Acute bacterial pneumonia is associated with the occurrence of acute coronary syndromes. Medicine (Baltimore). 2009;88(3):154–159. , , , et al.
- Understanding the inflammatory cytokine response in pneumonia and sepsis: results of the Genetic and Inflammatory Markers of Sepsis (GenIMS) Study. Arch Intern Med. 2007;167(15):1655–1663. , , , et al.
- Adjuvant steroid therapy in community‐acquired pneumonia: a systematic review and meta‐analysis. J Hosp Med. 2013. , , , , .
- Infectious Diseases Society of America/American Thoracic Society consensus guidelines on the management of community‐acquired pneumonia in adults. Clin Infect Dis. 2007;44(suppl 2):S27–S72. , , , et al.
- Reassessing the design, conduct, and analysis of clinical trials of therapy for community‐acquired pneumonia. Clin Infect Dis. 2008;46(8):1152–1156. .
In the early part of the 20th century, pneumonia was a leading causes of death, particularly among older adults, for whom Osler termed it the Captain of the Men of Death.[1] Mortality rates from severe (bacteremic) pneumonia were typically 80% to 90%, and the introduction of antibacterial therapy in the 1940s reduced that mortality to 10% to 20%. However, as pointed out by Austrian and Gold in a landmark paper in the 1950s, mortality for patients dying within the first 4 to 5 days was not reduced in the postantibiotic era.[2] The survival rates for patients with severe community‐acquired pneumonia minimally improved over the ensuing 50 years, despite the introduction of numerous new antimicrobial drugs and other medical interventions.
One promising area for therapeutic intervention relates to the potential adverse effects of the host inflammatory response in the setting of pneumonia. A growing body of literature supports the conclusion that the window of optimal host response may be relatively narrow. Too little response and patients quickly succumb to overwhelming sepsis. Too much response and a patient's hyperactivated inflammatory system can set off a cascade of secondary events, triggering events such as acute lung injury or ischemic heart disease.[3] Studies have also established that the level of inflammation, as measured by biomarkers such as C‐reactive protein, tumor necrosis factor, and interleukins, can identify patients at increased risk of adverse outcomes.[4] Thus, it is logical to ask whether immune modulating therapies can improve outcomes for these patients.
In this issue of the Journal of Hospital Medicine, Shafiq and colleagues completed a systematic review and meta‐analysis of corticosteroid therapy for patients with pneumonia.[5] Updating prior reviews, they included 8 randomized controlled trials, all of which consisted of low‐dose, systemic, steroid therapy as the intervention and standard care as the control arm. The overall quality of the included studies was judged moderate, and the overall size of the pooled data was only 1119 patients. In their analysis, adjunctive steroid therapy did not reduce in‐hospital mortality, with 4 studies demonstrating effect sizes suggesting benefit, 3 studies demonstrating no benefit or harm, and 1 study favoring the nonsteroid arm. In these situations with grossly heterogeneous study results, it seems prudent to avoid overly interpreting pooled results, even if statistical tests for heterogeneity are nonsignificant. The investigators also reported a range of secondary outcomes, noting that hospital length of stay was significantly reduced in the pooled steroid treated arms.
The overall negative finding is clearly disappointing at a time when clinicians are looking for new treatments to improve outcomes for these patients. Pneumonia is a heterogeneous disorder, representing a wide range of microbial pathogens and underlying host risk factors. Current treatment guidelines for patients with community‐acquired pneumonia are largely empirical and do not focus on pathogen identification, host risk factor analysis, or biomarker distributions to select antimicrobial therapy.[6] In this regard, despite being 1 of the oldest conditions for which we have published guidelines for treatment, the treatment approach for pneumonia remains quite antiquated, ignoring recent advances in the incorporation of personalized treatment strategies for other illnesses. We may have reached the limits of one‐size‐fits‐all treatment strategies for hospitalized adults with community‐acquired pneumonia. To improve outcomes further, we need to understand the heterogeneity of the disorder and tailor therapies at an individual level. Rapid point‐of‐care tests for pathogens and host response offer the most promising approach toward this strategy.
It is notable that the majority of studies focus on in‐hospital mortality, even though the impact of steroid therapy may be observed over a longer period of follow‐up. Moreover, although mortality is clearly a relevant outcome, it is not the only patient‐centered outcome of importance. However, other outcomes that are typically assessed, such as length of hospitalization and cost, are not patient‐centered outcomes. These are process measures that reflect physician judgment as much as any patient response to treatment. We need to move the field forward by embracing patient outcomes beyond mortality to optimally evaluate new treatment strategies, particularly because the majority of patients will survive hospitalization for the illness. These outcomes would include time to resolution of major symptoms, such as cough and fatigue, and functional outcomes, including return to work and usual activities. Future comparative efficacy and effectiveness studies in pneumonia need to consider a much wider range of true patient outcomes.[7]
It is increasingly fashionable to adopt cross‐disease approaches toward optimizing patient care, particularly in the hospital. Important initiatives that aim to reduce hospital injuries and improve transitions of care are relatively agnostic to specific disease states. Much of the research agenda of hospital medicine avoids a disease‐specific focus, assuming such disease‐specific approaches are the domain of specialists. Yet, it is worth remembering that much of the progress for medical care can be traced to traditional considerations of disease pathophysiology and empirical studies of risk factors and treatments for specific disease. Hospitalists remain at the front line in dealing with most of the common illnesses that afflict patients. Battling those conditions 1 at a time should be an important component of the broader hospitalist research agenda. One hundred years after Osler charged the medical community to identify new strategies for treating an old enemy, we are still struggling to win the battle.
Disclosure
This work was supported in part by K24‐AI073957 (JPM) from the National Institute of Allergy and Infectious Diseases, National Institutes of Health. The author has no conflicts of interest to report.
In the early part of the 20th century, pneumonia was a leading causes of death, particularly among older adults, for whom Osler termed it the Captain of the Men of Death.[1] Mortality rates from severe (bacteremic) pneumonia were typically 80% to 90%, and the introduction of antibacterial therapy in the 1940s reduced that mortality to 10% to 20%. However, as pointed out by Austrian and Gold in a landmark paper in the 1950s, mortality for patients dying within the first 4 to 5 days was not reduced in the postantibiotic era.[2] The survival rates for patients with severe community‐acquired pneumonia minimally improved over the ensuing 50 years, despite the introduction of numerous new antimicrobial drugs and other medical interventions.
One promising area for therapeutic intervention relates to the potential adverse effects of the host inflammatory response in the setting of pneumonia. A growing body of literature supports the conclusion that the window of optimal host response may be relatively narrow. Too little response and patients quickly succumb to overwhelming sepsis. Too much response and a patient's hyperactivated inflammatory system can set off a cascade of secondary events, triggering events such as acute lung injury or ischemic heart disease.[3] Studies have also established that the level of inflammation, as measured by biomarkers such as C‐reactive protein, tumor necrosis factor, and interleukins, can identify patients at increased risk of adverse outcomes.[4] Thus, it is logical to ask whether immune modulating therapies can improve outcomes for these patients.
In this issue of the Journal of Hospital Medicine, Shafiq and colleagues completed a systematic review and meta‐analysis of corticosteroid therapy for patients with pneumonia.[5] Updating prior reviews, they included 8 randomized controlled trials, all of which consisted of low‐dose, systemic, steroid therapy as the intervention and standard care as the control arm. The overall quality of the included studies was judged moderate, and the overall size of the pooled data was only 1119 patients. In their analysis, adjunctive steroid therapy did not reduce in‐hospital mortality, with 4 studies demonstrating effect sizes suggesting benefit, 3 studies demonstrating no benefit or harm, and 1 study favoring the nonsteroid arm. In these situations with grossly heterogeneous study results, it seems prudent to avoid overly interpreting pooled results, even if statistical tests for heterogeneity are nonsignificant. The investigators also reported a range of secondary outcomes, noting that hospital length of stay was significantly reduced in the pooled steroid treated arms.
The overall negative finding is clearly disappointing at a time when clinicians are looking for new treatments to improve outcomes for these patients. Pneumonia is a heterogeneous disorder, representing a wide range of microbial pathogens and underlying host risk factors. Current treatment guidelines for patients with community‐acquired pneumonia are largely empirical and do not focus on pathogen identification, host risk factor analysis, or biomarker distributions to select antimicrobial therapy.[6] In this regard, despite being 1 of the oldest conditions for which we have published guidelines for treatment, the treatment approach for pneumonia remains quite antiquated, ignoring recent advances in the incorporation of personalized treatment strategies for other illnesses. We may have reached the limits of one‐size‐fits‐all treatment strategies for hospitalized adults with community‐acquired pneumonia. To improve outcomes further, we need to understand the heterogeneity of the disorder and tailor therapies at an individual level. Rapid point‐of‐care tests for pathogens and host response offer the most promising approach toward this strategy.
It is notable that the majority of studies focus on in‐hospital mortality, even though the impact of steroid therapy may be observed over a longer period of follow‐up. Moreover, although mortality is clearly a relevant outcome, it is not the only patient‐centered outcome of importance. However, other outcomes that are typically assessed, such as length of hospitalization and cost, are not patient‐centered outcomes. These are process measures that reflect physician judgment as much as any patient response to treatment. We need to move the field forward by embracing patient outcomes beyond mortality to optimally evaluate new treatment strategies, particularly because the majority of patients will survive hospitalization for the illness. These outcomes would include time to resolution of major symptoms, such as cough and fatigue, and functional outcomes, including return to work and usual activities. Future comparative efficacy and effectiveness studies in pneumonia need to consider a much wider range of true patient outcomes.[7]
It is increasingly fashionable to adopt cross‐disease approaches toward optimizing patient care, particularly in the hospital. Important initiatives that aim to reduce hospital injuries and improve transitions of care are relatively agnostic to specific disease states. Much of the research agenda of hospital medicine avoids a disease‐specific focus, assuming such disease‐specific approaches are the domain of specialists. Yet, it is worth remembering that much of the progress for medical care can be traced to traditional considerations of disease pathophysiology and empirical studies of risk factors and treatments for specific disease. Hospitalists remain at the front line in dealing with most of the common illnesses that afflict patients. Battling those conditions 1 at a time should be an important component of the broader hospitalist research agenda. One hundred years after Osler charged the medical community to identify new strategies for treating an old enemy, we are still struggling to win the battle.
Disclosure
This work was supported in part by K24‐AI073957 (JPM) from the National Institute of Allergy and Infectious Diseases, National Institutes of Health. The author has no conflicts of interest to report.
- The Principles and Practices of Medicine. 7th ed. New York, London: D. Appleton and Co.; 1909. .
- Pneumococcal bacteremia with special reference to bacteremic pneumococcal pneumonia. Ann Intern Med. 1964;60:759–776. , .
- Acute bacterial pneumonia is associated with the occurrence of acute coronary syndromes. Medicine (Baltimore). 2009;88(3):154–159. , , , et al.
- Understanding the inflammatory cytokine response in pneumonia and sepsis: results of the Genetic and Inflammatory Markers of Sepsis (GenIMS) Study. Arch Intern Med. 2007;167(15):1655–1663. , , , et al.
- Adjuvant steroid therapy in community‐acquired pneumonia: a systematic review and meta‐analysis. J Hosp Med. 2013. , , , , .
- Infectious Diseases Society of America/American Thoracic Society consensus guidelines on the management of community‐acquired pneumonia in adults. Clin Infect Dis. 2007;44(suppl 2):S27–S72. , , , et al.
- Reassessing the design, conduct, and analysis of clinical trials of therapy for community‐acquired pneumonia. Clin Infect Dis. 2008;46(8):1152–1156. .
- The Principles and Practices of Medicine. 7th ed. New York, London: D. Appleton and Co.; 1909. .
- Pneumococcal bacteremia with special reference to bacteremic pneumococcal pneumonia. Ann Intern Med. 1964;60:759–776. , .
- Acute bacterial pneumonia is associated with the occurrence of acute coronary syndromes. Medicine (Baltimore). 2009;88(3):154–159. , , , et al.
- Understanding the inflammatory cytokine response in pneumonia and sepsis: results of the Genetic and Inflammatory Markers of Sepsis (GenIMS) Study. Arch Intern Med. 2007;167(15):1655–1663. , , , et al.
- Adjuvant steroid therapy in community‐acquired pneumonia: a systematic review and meta‐analysis. J Hosp Med. 2013. , , , , .
- Infectious Diseases Society of America/American Thoracic Society consensus guidelines on the management of community‐acquired pneumonia in adults. Clin Infect Dis. 2007;44(suppl 2):S27–S72. , , , et al.
- Reassessing the design, conduct, and analysis of clinical trials of therapy for community‐acquired pneumonia. Clin Infect Dis. 2008;46(8):1152–1156. .
Bed Utilization in the PICU
Patient flow refers to the management and movement of patients in health care settings and is linked to quality, safety, and cost.16 The intensive care unit (ICU) is crucial in patient flow.7, 8 The limited number of beds and the resource‐intensive services and staffing associated with them require that hospitals optimize their utilization, as is increasingly true of all hospital resources. To maximize delivery of services to patients who need them and minimize real and opportunity losses (eg, postponed surgery, diverted transports, or inability to accept patients), patients in ICU beds should receive critical care medicine/nursing services while there and be transferred or discharged when appropriate.
The time between arrival and departure from any area of the hospital, including the ICU, is considered the time when a patient is receiving needed clinical carethe value‐added portion of health care operationsand time waiting to move on to the next step.911 This period includes both necessary logistics (eg, signing out a patient or waiting a reasonable amount of time for room cleaning) and nonvalue‐added time (eg, an excessively long amount of time for room cleaning). Operations management labels nonvalue‐added time as waste, and its reduction is vital for high‐quality health care.9, 12, 13 As in other industries, one important way to understand value versus waste is through direct observation.11, 14 Although operating rooms have been the subject of several published process improvement projects to improve efficiency,1518 inpatient beds have not been the subject of such scrutiny. The objectives of this study were to generate a direct observation method and use it to describe pediatric ICU (PICU) bed utilization from a value‐added perspective.
METHODS
An interdisciplinary work group of physicians, nurses, quality improvement specialists, and 1 operations management expert developed an Excel spreadsheet to categorize hour‐by‐hour status of PICU beds. The clinicians generated a list of 27 activities. A critical care nurse trained in quality improvement piloted the list for 3 separate 4‐hour blocks over 2 weeks adding 18 activities; 2 additional activities were added during the 5 weeks of observation (Table 1). (The recording tool is provided in the Supporting Information Appendix.) Three observers with knowledge of medical terminology (2 third‐year medical students and 1 premedical student with years of experience as an emergency medical technician) were trained over 12 hours to conduct the observations. Prior to the observations, the 3 observers also spent time in the PICU, and terminology used for recordings was reviewed. Interobserver reliability was checked during 3 sets of observation circuits by all 3 observers and the principal investigator, as well as by spot checks during the study.
Activity Description | Activity Code | Total Hours Over 5 Weeks | % Total Hours Over 5 Weeks* | Mean Hours per Week* |
---|---|---|---|---|
| ||||
Ventilated patient | Vent | 8996 | 45 | 1799 |
CCSs not otherwise specified | NOS | 2982 | 15 | 596 |
Neurosurgery patient with ICU needs | NeurosurgICU | 1534 | 8 | 307 |
Room empty and unassigned | Empty‐unassigned | 1511 | 8 | 302 |
Patient on continuous infusion | ContinInfus | 958 | 5 | 192 |
Awaiting floor bed assignment | Floorbedassign | 919 | 5 | 184 |
Patient with arterial line | ArtLine | 508 | 3 | 102 |
Patient on high‐flow nasal cannula | HFNC | 475 | 2 | 95 |
Room cleaning | EVS | 318 | 2 | 64 |
Patient <12 hours after extubation | PostVent | 226 | 1 | 45 |
Patient in OR, bed being held | OR | 210 | 1 | 42 |
Neurosurgery patient, post‐ICU needs | NeurosurgPostICU | 164 | 0.8 | 33 |
No clear ICU need, but no other accepting floor or service | Unclear | 163 | 0.8 | 33 |
Patient at procedure, bed being held | Proced | 133 | 0.7 | 27 |
Patient awaiting a rehabilitation bed | Rehab | 99 | 0.5 | 20 |
Patient with ventriculostomy | Ventriculostomy | 82 | 0.4 | 16 |
Patient eligible to be in NICU | NICU | 76 | 0.4 | 15 |
Patient awaiting social work, case management, prescriptions before discharge | AwaitingOtherServ | 66 | 0.3 | 13 |
Empty bed, assigned to ED patient | Empty‐ED | 40 | 0.2 | 8 |
Empty bed, assigned to incoming transport patient | Empty‐Transport | 37 | 0.2 | 7 |
Patient awaiting transport to another facility | Transport | 37 | 0.2 | 7 |
Patient awaiting consult to determine transfer | Consult | 33 | 0.2 | 7 |
Patient awaiting physician or NP sign‐out to floor before transfer | CallMDNP | 30 | 0.2 | 6 |
PICU room needs a bed for next patient | Bed | 26 | 0.1 | 5 |
Patient eligible to be in CCU | CCU | 24 | 0.1 | 5 |
Patient eligible to be in CICU | CICU | 24 | 0.1 | 5 |
Patient awaiting laboratory result to determine transfer or discharge | LabResult | 21 | 0.1 | 4 |
Patient awaiting a ride home | Ride | 21 | 0.1 | 4 |
Empty bed, assigned to floor patient | Empty‐floor | 19 | 0.1 | 4 |
Patient awaiting nursing report to floor for transfer | Callnurse | 18 | 0.1 | 4 |
Patient eligible to be in PCU | PCU | 18 | 0.1 | 4 |
Patient on cardiac pressor | Pressor | 16 | 0.1 | 3 |
Patient actively coding | Code | 15 | 0.1 | 3 |
Patient on continuous veno‐venous hemofiltration | CVVH | 15 | 0.1 | 3 |
Nursing work needed to enable transfer out | Nursing | 11 | 0.1 | 2 |
Patient awaiting order for transfer to floor | Order | 11 | 0.1 | 2 |
Patient in interventional radiology, bed being held | IR | 10 | 0.1 | 2 |
Patient deceased in PICU room | Deceased | 9 | 0.1 | 2 |
Awaiting radiology result to clear transfer or discharge | RadResult | 9 | 0.1 | 2 |
Patient awaiting a floor bed to be cleaned for transfer out | Floorbedclean | 7 | <0.1 | 1 |
Other logistical need for an empty room | Logistics | 7 | <0.1 | 1 |
Disagreement among services for disposition | Disagreement | 4 | <0.1 | 1 |
Family request to stay in PICU | Family | 3 | <0.1 | 1 |
Awaiting accepting attending/fellow for transfer out | Accept | 1 | <0.1 | <1 |
PICU room needs a crib for next patient | Crib | 1 | <0.1 | <1 |
Patient with preventable reason for being in PICU | Prev | 0 | 0 | 0 |
PICU room needs specialty bed for next patient | SpecialBed | 0 | 0 | 0 |
Total | 19,887 | 100 |
The targeted area included 24 single‐patient rooms. The activity of each bed was recorded hourly. Real‐time recording in to the Excel spreadsheet on a dedicated laptop occurred from 8:00 AM until 11:00 PM. The most visible or critical event was recorded. Although some activities were not mutually exclusive (eg, a patient could be ventilated and on a continuous infusion simultaneously), the objective was to identify when a room was being used for any critical care service, not enumerate all of them. The observers noted overnight events that occurred from 11:00 PM to 8:00 AM in the morning by reviewing the bedside record and talking to the staff to complete each day's 24‐hour recording. The observers also recorded the hospital‐wide census and the census for the other half of the PICU every 4 hours. The observations occurred over 5 noncontiguous weeks between January 2009 and April 2009.
After all observations were complete, activities were classified as critical care services (CCS) or noncritical‐care services (NCCS). NCCSs were further divided into necessary logistics (defined for analysis purposes as the first hour of any NCCS activity) or nonvalue‐added (the second or greater hour of NCCS). A time limit of 1 hour was chosen to define necessary logistics based on a consensus that nonclinical activities optimally would not take more than 1 hour each. We also analyzed results with 2 hours as the cutoff for necessary logistics. Admission, discharge, and transfer records were reviewed to check for returns to the PICU or hospital within 48 hours of transfer or discharge from the PICU.
Analyses were conducted using Microsoft Excel (Microsoft, Redmond, WA) and Stata 10.0 (StataCorp, College Station, TX). The study was approved by the Children's Hospital of Philadelphia Institutional Review Board with waiver of consent.
RESULTS
A total of 824 hours of recordings included 19,887 bed‐hours with 219 unique patients; among them, 2 remained from the first day of recording in January to the last day in April (sample recording in Figure 1). A total of 50 patients (range, 812 per week) stayed for the entirety of each 1‐week observation period. Of the 47 possible activities, 45 of them were recorded for at least 1 hour in the 5 weeks. Overall, 14 activities accounted for 95% of the observed bed‐hours and 31 activities accounted for the remaining 5%. CCS accounted for 82% of observed bed‐hours, NCCS accounted for 10.4%, and empty unassigned accounted for 8% (Figure 2). Using the 1‐hour cutoff for necessary services, 77% of NCCS time was nonvalue‐added, whereas 23% of it was necessary logistics; using the 2‐hour cutoff, 54% was nonvalue‐added, and 46% was necessary logistics.
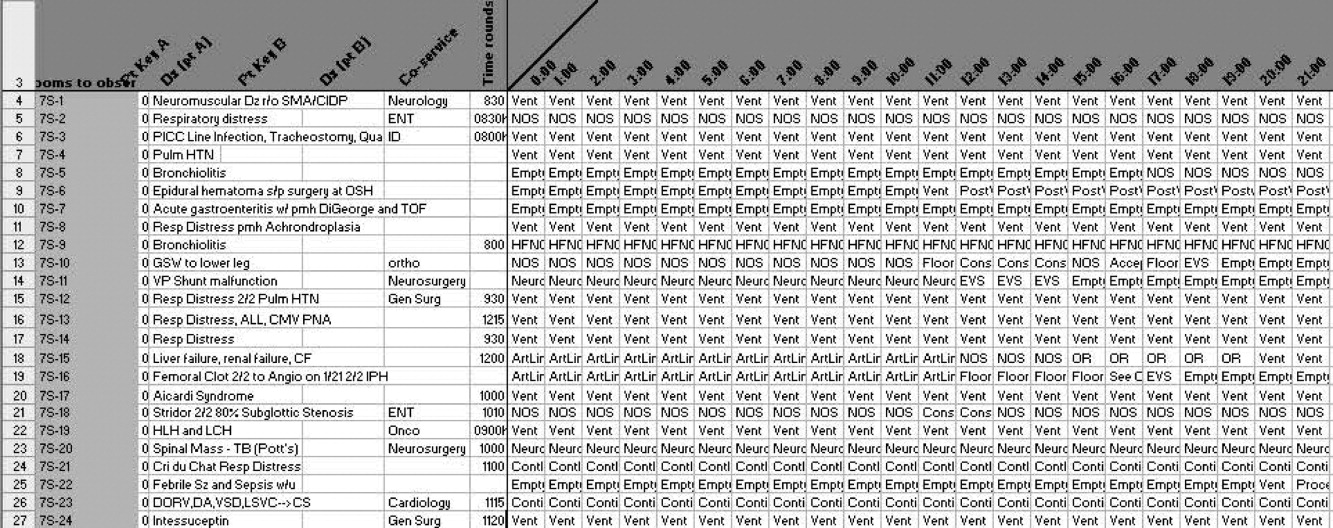
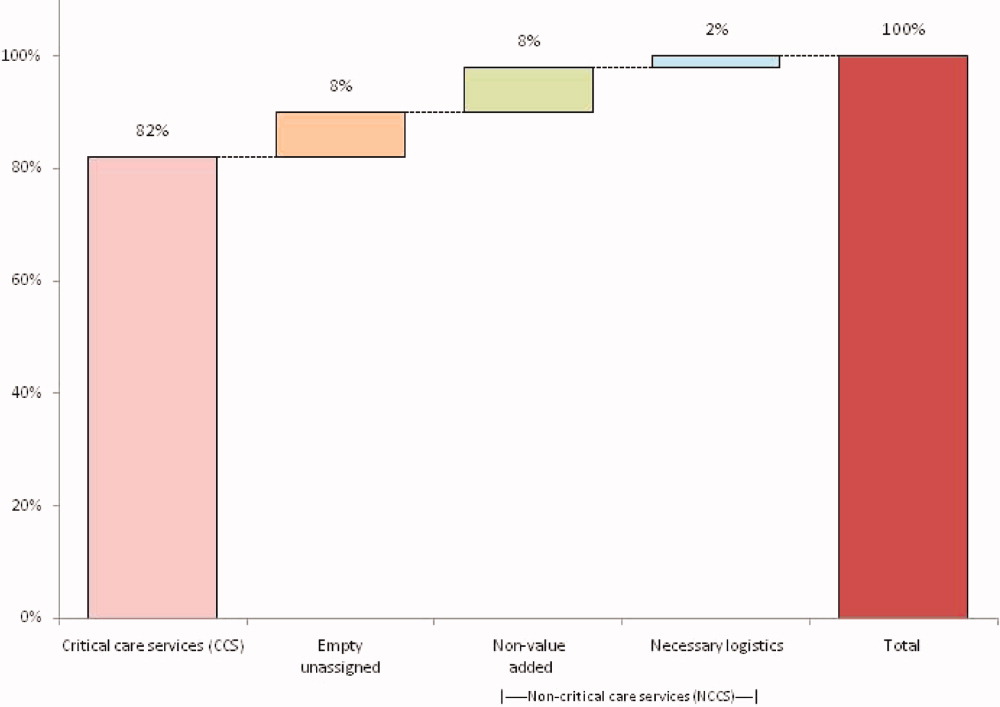
During the observation period, <1% of bed‐hours were used for CCS for overflow patients from the neonatal ICU (NICU), cardiac care unit (CCU), cardiac ICU (CICU), or progressive care unit (PCU; tracheostomy/ventilator unit). Although only 4 patients required transport to a rehabilitation facility, their wait time comprised 99 hours (<1%) of total recordings. Eight patients waited a mean of 2.6 hours for transportation home (maximum, 10 hours).
To demonstrate the cycle of room use, activities were divided into 4 categories: room preparation, critical care services, disposition pending, and postcritical care services (Figure 3). As an example of detailed data revealed by direct observation, we identified 102 instances totaling 919 hours when a patient was waiting for a bed assignment on another floor (5% of all bed‐hours). The mean wait time was 9 hours (range, 188 hours) and the median time was 5.5 hours. There were only 15 instances when floor bed assignment took 1 hour or less, and only 9 instances when it took 12 hours. Similarly, considerable time was spent on cleaning rooms between patients: only 66 of 146 instances of cleaning took 1 hour or less. The mean time for cleaning was 2.2 hours (range, 115), and the median was 2 hours. (There were 136 recorded instances of room cleaning and 10 additional episodes that were not recorded but had to be completed for the room to turnover from one patient to the next, yielding a total of 146 instances of cleaning.)
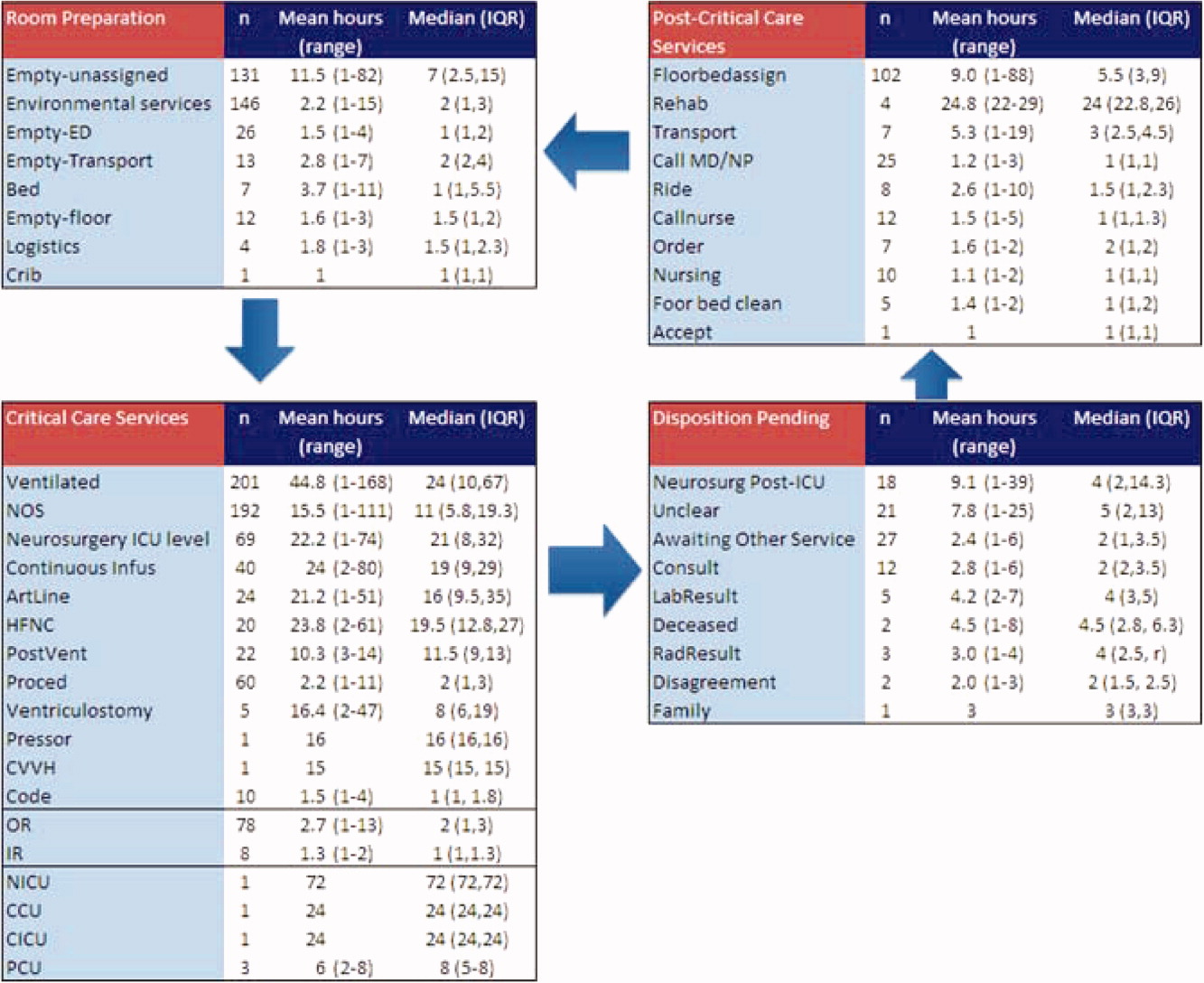
From the 824 hours of recording, we identified 200 hours (25% of time) when there were zero empty unassigned beds available in the section of the PICU being observed. Episodes of full occupancy occurred mostly on weekdays, with 23% of hours of full capacity on Thursdays, 21% on Mondays, and 21% on Wednesdays; only 8% were on Saturdays and <1% on Sundays. These 200 hours fell into 36 separate episodes of complete occupancy, each lasting 122 hours. Each patient, on average, received 3.1 hours of NCCS during each episode of full occupancy (range, 111 hours). Within these 200 hours at capacity, we identified only 15 hours (8%) when all 24 beds were used for CCS. For 72% of the time, there was at least 1 bed with NCCS, and for 37% at least 2 beds. A small portion of the time (7%), the lack of beds was affected by occupancy by patients who should have been in the NICU, CICU, CCU, or PCU.
Data collected through direct observation can be used to understand aggregated and averaged experiences, but also more specific time periods. For example, we identified 1 week with the highest consistent level of occupancy and turnover: March 915 had empty unassigned beds for only 4% of the week. Of the 168 hours in the week, 68 (40%) had full capacity. However, for 90% of the time, at least 1 bed was used for a NCCS. Other analytic options included varying the assumptions around time needed for logistics. Overall, NCCS time on necessary logistics changes from 23% to 46% using 1 hour versus 2 hours as the cutoff. For floor bed assignments, assuming that the first hour of this activity is necessary logistics and any hour thereafter is not, 817 hours were wasted. Even after assuming 2 hours of necessary logistical time (which may also include steps such as nursing and physician sign‐out to the receiving team, often not recorded in the observations), this left 715 hours of NCCS time in which patients waited to be placed elsewhere in the hospital. For room cleaning, because recordings were hourly, but room cleaning could take less time, we performed a sensitivity analysis, converting all 1‐hour recordings to half‐hour recordings to half‐hour recordings (an exaggerated shortening since industry‐standard cleaning may take longer).
Of the 219 patients directly observed, 15 were noted to be waiting for a transfer out of the PICU but experienced a change in disposition before the transfer. On average, these patients waited 8 hours for a floor bed assignment (range, 221) before reverting to a CCS, which then lasted an average of 16.5 hours (range, 149). (Included in this group are 2 patients who experienced this change in disposition twice.) In post hoc review across the 5 weeks, no patients were transferred back to the PICU within 48 hours after being transferred out. During the study period, 19 patients were discharged directly from the PICU (8 to home, 7 by transport to another facility, and 4 to rehabilitation). One patient returned to the hospital (but not the PICU) within 48 hours of being discharged home from the PICU.
During the study period, using the highest census value for recorded for each 24‐hour period and the number of beds available that day, median hospital‐wide occupancy was 93% (interquartile range, 90%96%). During the 35 days of observation, 71% of the days had occupancy >90%, 29% of days had occupancy >95%, and 3% of days had occupancy >100%.
DISCUSSION
In this direct observation of a PICU, we found high usage of beds for delivery of CCS. We identified many episodes in which the half of the PICU we observed was fully occupied (200 of 824 hours), but not necessarily delivering PICU‐level care to all patients. In fact, 75% of the full‐capacity hours had at least 1 patient receiving NCCS and 37% had at least 2. Patients waiting for a floor bed assignment represented nearly 5% of bed‐hours observed (mean 9 hours per patient). That full occupancy was not random, but rather clustered on weekdays, is consistent with other work showing that hospitals are at greater risk for midweek crowding due to the way in which scheduled admissions enter and leave.1925
Our methods provide the basis for operational analysis and improvement to patient flow, such as value stream mapping.9, 26 Process improvement work could be directed to areas of delay uncovered through this analysis and inform clinical and nonclinical management. For example, one of the key problems faced by the PICU was finding floor bed assignments for patients leaving the unit. Simply building more beds in the PICU will not solve this problemand at an estimated cost of $2 million to add a bed, it is likely not an efficient means of responding to poor flow. In these cases, the problem seems to lie downstream, and could suggest shortage of regular floor beds or inefficient bed assignment procedures within the hospital. The output also suggests that variation in nonclinical processes should be a target for improvement, such as time to clean rooms, because variation is known to be a source of nonvalue‐added time in many operations.9, 26 High occupancy on weekdays but low occupancy on weekends also emphasizes the potential for smoothing occupancy to reduce the risk of midweek crowding and to better manage bed utilization and staffing.24, 25
When seeking to reduce nonvalue‐added time, one must weigh the risks of increased efficiency against clinical outcomes. For example, if patients could be transferred out of the PICU faster, would the risk of returns to the PICU be higher? In this study, 15 patients (7%) had a change in disposition from awaiting transfer back to a CCS. The fact that transfers did not happen instantaneously may serve as a safety check to reduce rapid returns, but it is not possible for us to evaluate the reasons why patients did not actually complete the pending transfers. Specifically, we cannot determine whether the patient's clinical status objectively deteriorated, the ICU team made a judgment call to hold the patient, or the floor team refused to accept the transfer. Given this fact, although it appears in this study (and in the health care system more broadly) that there are opportunities to increase efficiency and reduce nonvalue‐added time, it is not realistic (nor advisable) that such time be reduced to zero. Along this line, one must consider separately purely nonclinical functions such as room cleaning and those that include some clinical element, such as time waiting for a patient to be transferred.
Beyond the direct findings of this study, the method should be replicable in other settings and can reveal important information about health care efficiency, capacity, and flexibility. The bottlenecks identified would have been difficult to identify through administrative record review. The exact amount of time to spend on observation may vary from place to place and would depend on the expected variation over time and the level of detail sought. In general, the more common the event and the less variation, the less time needed to observe it.
This study has several limitations that should be considered in terms of interpreting the results and in seeking to reproduce the approach. First, hourly recordings may not be discrete enough for events that took less than 1 hour. To assess the degree to which this would affect our results, we reanalyzed all NCCS by subtracting 30 minutes (0.5 hour) from all recordings, which increased total CCS from 82% to 87% and decreased NCCS by the same 5 percentage points. In a related fashion, our recordings were truncated at the start and end of each 1‐week period, so we could only observe a maximum of 168 hours for any given activity and did not record how long an activity was happening before or after the recordings started or stopped, respectively. Second, each recording could only be for 1 activity per hour. Separate from the level of granularity already noted, this also limits interpretation of critical care activities that may have been simultaneous. However, because the goal of the study was not to describe the provision of critical care services, but rather the times when they were not being delivered, this does not influence our conclusions. For movement of patients, however, we missed instances of physician and nursing calling sign‐out on patients to receiving units, as these events last less than 1 hour (and in the case of surgical patients, generally do not occur as the team provides continuous coverage). The time for such events is then included in other activities. To the extent that this may influence the results, it would increase the perceived time for nonvalue‐added services, but to a limited degree, and never by more than 59 minutes. Third, the overnight hours (11:00 PM to 8:00 AM) were not directly observed, but retrospectively recorded each morning by reviewing the records and discussing the overnight events with the clinical staff. For example, if a patient was intubated at 11:00 PM and at 8:00 AM, the observer would confirm this and record that status for the intervening hours. This is unlikely to result in a substantial impact on the findings, because the overnight hours have a relative degree of stability even for unstable patients in terms of their status of needing or not needing a CCS. Fourth, we did not evaluate the appropriateness of CCS delivered (eg, how long a patient was ventilated). Our definitions for CCS and NCSS were based on Children's Hospital of Philadelphia practices, which may not be the same as those of other facilities. The categorization of CCS was objective for activities such as ventilation or continuous infusion, but was less clear for the not otherwise specified recordings, which represented patients with a complex illness or projected organ, respiratory, cardiac, or neurological failure. These patients were not receiving a specific critical care intervention, but were deemed to need to be in the PICU as opposed to a regular floor (eg, for frequent monitoring of potential respiratory failure). It would also include patients receiving combinations of therapies more efficiently delivered in the PICU. For that, the observers relied on the judgment of clinicians (primarily nurses) to determine whether the patient needed to be in the PICU or not; if no specific reason could be provided, not otherwise specified was applied. These 192 instances accounted for 2982 aggregate bed‐hours (15% of total). It is difficult to judge the direction of bias, because overestimation of need to be in the PICU may be as likely to occur as underestimation. Fifth, the very presence of the observers may have changed behavior. Knowing that they were being observed staff may have acted with greater efficiency than otherwise. We expect that such a finding would lead to less time appearing as necessary logistics or NCCS. Finally, results may not be generalizable to other hospitals or hospital settings. There are clearly important contextual factors, not only for the location but also for the duration. For example, staffing was never an issue during the 5 weeks of observation, but there are locations where an empty bed is not necessarily usable due to lack of staffing. Nonetheless, we believe the results provide a generalizable approach and methodology for other settings (and staffing could be a reason for an empty bed).
In terms of the setting, as noted, we observed one discrete 24‐bed unit, which comprises half of the total PICU. Thus, statements that the PICU was at full capacity must be interpreted in the context that additional rooms may have been available on the other side. Patients are generally admitted alternately to each unit, so the occupancies should parallel each other. We recorded the census every 4 hours for both sides from the electronic system (Sunrise Clinical Manager [SCM]). However, this only accounts for patients physically in beds, not beds held for patients in other locations. Thus, we would expect a discrepancy between direct observation and the SCM value. Through analysis of the entire pediatric intensive care unit,* that part which observed directly, and that which we did not observe directly using census data, we think it reasonable to assert that both units of the total PICU had constrained capacity during the times we directly observed and recorded such constraint on one side.
This study demonstrates the use of direct observation for inpatient settings to learn about resource utilization and identification of value‐added services. PubMed searches for the terms efficiency, flow, process redesign, and time management bring up many more references for operating rooms than for ICUs or inpatient beds. Some examples of ICU‐directed work include videography of an ICU in Australia27 and human factor analysis in ICU nursing.5 Time‐motion studies have also been conducted on clinical staff, such as physicians.28, 29
In conclusion, we found that direct observation provided important insights into the utilization of patient rooms in an important inpatient setting. Data such as these are valuable for clinical and process improvement work, as well as understanding how best to match capacity to patient need. Finally, the methodology is reproducible for other settings and would be an additional tool to measuring and improving the efficiency and value of the health system. When appropriate, this approach can also evaluate the effectiveness of process improvement, help identify and reduce waste,13 and contribute to the growing field that merges operations management with hospital administration and clinical care: in other words, evidence‐based management.30
Acknowledgements
The authors thank Paula Agosto, Patricia Hubbs, Heidi Martin, and Annette Bollig for contributions to the study design.
In comparing direct observation to the SCM count, we found perfect concordance for 110 hours (55%) during which 0 beds were available. For the other 90 hours, SCM reported 1 bed being available in 46 hours (23%), 2 beds being available in 24 hours (12%), 3 beds being available in 17 hours (9%), and 4 beds being available in 3 hours (2%)all while we directly observed 0 beds being available. Thus, cumulatively, 90% of the hours observed with no beds had an SCM report availability of 02 beds; 99% of the time that was 03 beds. Applying this rate of mismatch to the unit that we did not observe directly, SCM reported 0 beds for 46 (23%) of the 200 hours the observation unit was full; SCM reported 1 bed available in 70 hours (35%), 2 beds open in 42 hours (21%), 3 beds open in 26 hours (13%), and 4 beds open in 16 hours (8%). Cumulatively, that is 79% of the time with 02 beds and 92% at 03 beds. From this, we conclude that the combined PICU for both sides was likely functionally full at least 158 of the 200 hours that the side we observed was full (79% 200 hours) and likely had very constrained capacity during the other 42 hours.
- The effect of hospital occupancy on emergency department length of stay and patient disposition.Acad Emerg Med.2003;10:127–133. , , , , .
- The effect of hospital bed occupancy on throughput in the pediatric emergency department.Ann Emerg Med.2009;53:767–776. , , , .
- A Comparison of in‐hospital mortality risk conferred by high hospital occupancy, differences in nurse staffing levels, weekend admission, and seasonal influenza.Medical Care.2010;48:224–232. , , , .
- Intensive care unit occupancy: making room for more patients.Crit Care Med.2009;37:1794–1795. .
- A human factors engineering conceptual framework of nursing workload and patient safety in intensive care units.Intensive Crit Care Nurs.2005;21:284–301. , .
- High level of burnout in intensivists: prevalence and associated factors.Am J Respir Crit Care Med.2007;175:686–692. , , , et al.
- Length of stay and efficiency in pediatric intensive care units.J Pediatr.1998;133:79–85. , , .
- Variability in duration of stay in pediatric intensive care units: a multiinstitutional study.J Pediatr.1996;128:35–44. , .
- Matching Supply with Demand: An Introduction to Operations Management.New York, NY:McGraw‐Hill;2006. , .
- Impact of workload on service time and patient safety: an econometric analysis of hospital operations.Management Science.2009;55:1486–1498. , .
- OPIM 631: Operations Management.Philadelphia, PA:Wharton School, University of Pennsylvania;2008. .
- From waste to value in health care.JAMA.2008;299:568–571. , , .
- Eliminating “waste” in health care.JAMA.2009;302:2481–2482. .
- Toyota Production System: Beyond Large‐scale Production.London, UK:Productivity Press;1995. .
- Interdisciplinary work flow assessment and redesign decreases operating room turnover time and allows for additional caseload.Arch Surg.2006;141:65–69. , .
- Improving operating room efficiency through process redesign.Surgery.2006;140:509–514. , , , .
- Successful strategies for improving operating room efficiency at academic institutions.Anesth Analg.1998year="1998"1998;86:896–906. , , , .
- Efficiency of the operating room suite.Am J Surg.2003;185:244–250. , , .
- Children's hospitals do not acutely respond to high occupancy.Pediatrics.2010;125:974–981. , , , et al.
- Institute for Healthcare Improvement. Smoothing elective surgical admissions. Available at: http://www.ihi.org/IHI/Topics/Flow/Patient Flow/EmergingContent/SmoothingElectiveSurgicalAdmissions.htm. Accessed October 24,2008.
- Boston Hospital Sees Big Impact from Smoothing Elective Schedule.OR Manager. Volume 20, no. 12,2004.
- Rethinking rapid response teams.JAMA.2010;304:1375–1376. , .
- Litvak E, ed.Managing Patient Flow in Hospitals: Strategies and Solutions.2nd ed.Oak Brook, IL:Joint Commission Resources;2009.
- Scheduled admissions and high occupancy at a children's hospital.J Hosp Med.2011;6:81–87. , , , , , .
- Addressing inpatient crowding by smoothing occupancy at children's hospitals.J Hosp Med.2011;6:466–473. , , , et al.
- Learning to See: Value Stream Mapping to Add Value and Eliminate MUDA.Cambridge, MA:Lean Enterprise Institute;1999. , .
- Reshaping ICU ward round practices using video‐reflexive ethnography.Qual Health Res.2008;18:380–390. , , .
- How hospitalists spend their time: insights on efficiency and safety.J Hosp Med.2006;1:88–93. , , .
- Where did the day go? A time‐motion study of hospitalists.J Hosp Med2010;5:323–238. , , , et al.
- Improving patient care by linking evidence‐based medicine and evidence‐based management.JAMA.2007;298:673–676. , , .
Patient flow refers to the management and movement of patients in health care settings and is linked to quality, safety, and cost.16 The intensive care unit (ICU) is crucial in patient flow.7, 8 The limited number of beds and the resource‐intensive services and staffing associated with them require that hospitals optimize their utilization, as is increasingly true of all hospital resources. To maximize delivery of services to patients who need them and minimize real and opportunity losses (eg, postponed surgery, diverted transports, or inability to accept patients), patients in ICU beds should receive critical care medicine/nursing services while there and be transferred or discharged when appropriate.
The time between arrival and departure from any area of the hospital, including the ICU, is considered the time when a patient is receiving needed clinical carethe value‐added portion of health care operationsand time waiting to move on to the next step.911 This period includes both necessary logistics (eg, signing out a patient or waiting a reasonable amount of time for room cleaning) and nonvalue‐added time (eg, an excessively long amount of time for room cleaning). Operations management labels nonvalue‐added time as waste, and its reduction is vital for high‐quality health care.9, 12, 13 As in other industries, one important way to understand value versus waste is through direct observation.11, 14 Although operating rooms have been the subject of several published process improvement projects to improve efficiency,1518 inpatient beds have not been the subject of such scrutiny. The objectives of this study were to generate a direct observation method and use it to describe pediatric ICU (PICU) bed utilization from a value‐added perspective.
METHODS
An interdisciplinary work group of physicians, nurses, quality improvement specialists, and 1 operations management expert developed an Excel spreadsheet to categorize hour‐by‐hour status of PICU beds. The clinicians generated a list of 27 activities. A critical care nurse trained in quality improvement piloted the list for 3 separate 4‐hour blocks over 2 weeks adding 18 activities; 2 additional activities were added during the 5 weeks of observation (Table 1). (The recording tool is provided in the Supporting Information Appendix.) Three observers with knowledge of medical terminology (2 third‐year medical students and 1 premedical student with years of experience as an emergency medical technician) were trained over 12 hours to conduct the observations. Prior to the observations, the 3 observers also spent time in the PICU, and terminology used for recordings was reviewed. Interobserver reliability was checked during 3 sets of observation circuits by all 3 observers and the principal investigator, as well as by spot checks during the study.
Activity Description | Activity Code | Total Hours Over 5 Weeks | % Total Hours Over 5 Weeks* | Mean Hours per Week* |
---|---|---|---|---|
| ||||
Ventilated patient | Vent | 8996 | 45 | 1799 |
CCSs not otherwise specified | NOS | 2982 | 15 | 596 |
Neurosurgery patient with ICU needs | NeurosurgICU | 1534 | 8 | 307 |
Room empty and unassigned | Empty‐unassigned | 1511 | 8 | 302 |
Patient on continuous infusion | ContinInfus | 958 | 5 | 192 |
Awaiting floor bed assignment | Floorbedassign | 919 | 5 | 184 |
Patient with arterial line | ArtLine | 508 | 3 | 102 |
Patient on high‐flow nasal cannula | HFNC | 475 | 2 | 95 |
Room cleaning | EVS | 318 | 2 | 64 |
Patient <12 hours after extubation | PostVent | 226 | 1 | 45 |
Patient in OR, bed being held | OR | 210 | 1 | 42 |
Neurosurgery patient, post‐ICU needs | NeurosurgPostICU | 164 | 0.8 | 33 |
No clear ICU need, but no other accepting floor or service | Unclear | 163 | 0.8 | 33 |
Patient at procedure, bed being held | Proced | 133 | 0.7 | 27 |
Patient awaiting a rehabilitation bed | Rehab | 99 | 0.5 | 20 |
Patient with ventriculostomy | Ventriculostomy | 82 | 0.4 | 16 |
Patient eligible to be in NICU | NICU | 76 | 0.4 | 15 |
Patient awaiting social work, case management, prescriptions before discharge | AwaitingOtherServ | 66 | 0.3 | 13 |
Empty bed, assigned to ED patient | Empty‐ED | 40 | 0.2 | 8 |
Empty bed, assigned to incoming transport patient | Empty‐Transport | 37 | 0.2 | 7 |
Patient awaiting transport to another facility | Transport | 37 | 0.2 | 7 |
Patient awaiting consult to determine transfer | Consult | 33 | 0.2 | 7 |
Patient awaiting physician or NP sign‐out to floor before transfer | CallMDNP | 30 | 0.2 | 6 |
PICU room needs a bed for next patient | Bed | 26 | 0.1 | 5 |
Patient eligible to be in CCU | CCU | 24 | 0.1 | 5 |
Patient eligible to be in CICU | CICU | 24 | 0.1 | 5 |
Patient awaiting laboratory result to determine transfer or discharge | LabResult | 21 | 0.1 | 4 |
Patient awaiting a ride home | Ride | 21 | 0.1 | 4 |
Empty bed, assigned to floor patient | Empty‐floor | 19 | 0.1 | 4 |
Patient awaiting nursing report to floor for transfer | Callnurse | 18 | 0.1 | 4 |
Patient eligible to be in PCU | PCU | 18 | 0.1 | 4 |
Patient on cardiac pressor | Pressor | 16 | 0.1 | 3 |
Patient actively coding | Code | 15 | 0.1 | 3 |
Patient on continuous veno‐venous hemofiltration | CVVH | 15 | 0.1 | 3 |
Nursing work needed to enable transfer out | Nursing | 11 | 0.1 | 2 |
Patient awaiting order for transfer to floor | Order | 11 | 0.1 | 2 |
Patient in interventional radiology, bed being held | IR | 10 | 0.1 | 2 |
Patient deceased in PICU room | Deceased | 9 | 0.1 | 2 |
Awaiting radiology result to clear transfer or discharge | RadResult | 9 | 0.1 | 2 |
Patient awaiting a floor bed to be cleaned for transfer out | Floorbedclean | 7 | <0.1 | 1 |
Other logistical need for an empty room | Logistics | 7 | <0.1 | 1 |
Disagreement among services for disposition | Disagreement | 4 | <0.1 | 1 |
Family request to stay in PICU | Family | 3 | <0.1 | 1 |
Awaiting accepting attending/fellow for transfer out | Accept | 1 | <0.1 | <1 |
PICU room needs a crib for next patient | Crib | 1 | <0.1 | <1 |
Patient with preventable reason for being in PICU | Prev | 0 | 0 | 0 |
PICU room needs specialty bed for next patient | SpecialBed | 0 | 0 | 0 |
Total | 19,887 | 100 |
The targeted area included 24 single‐patient rooms. The activity of each bed was recorded hourly. Real‐time recording in to the Excel spreadsheet on a dedicated laptop occurred from 8:00 AM until 11:00 PM. The most visible or critical event was recorded. Although some activities were not mutually exclusive (eg, a patient could be ventilated and on a continuous infusion simultaneously), the objective was to identify when a room was being used for any critical care service, not enumerate all of them. The observers noted overnight events that occurred from 11:00 PM to 8:00 AM in the morning by reviewing the bedside record and talking to the staff to complete each day's 24‐hour recording. The observers also recorded the hospital‐wide census and the census for the other half of the PICU every 4 hours. The observations occurred over 5 noncontiguous weeks between January 2009 and April 2009.
After all observations were complete, activities were classified as critical care services (CCS) or noncritical‐care services (NCCS). NCCSs were further divided into necessary logistics (defined for analysis purposes as the first hour of any NCCS activity) or nonvalue‐added (the second or greater hour of NCCS). A time limit of 1 hour was chosen to define necessary logistics based on a consensus that nonclinical activities optimally would not take more than 1 hour each. We also analyzed results with 2 hours as the cutoff for necessary logistics. Admission, discharge, and transfer records were reviewed to check for returns to the PICU or hospital within 48 hours of transfer or discharge from the PICU.
Analyses were conducted using Microsoft Excel (Microsoft, Redmond, WA) and Stata 10.0 (StataCorp, College Station, TX). The study was approved by the Children's Hospital of Philadelphia Institutional Review Board with waiver of consent.
RESULTS
A total of 824 hours of recordings included 19,887 bed‐hours with 219 unique patients; among them, 2 remained from the first day of recording in January to the last day in April (sample recording in Figure 1). A total of 50 patients (range, 812 per week) stayed for the entirety of each 1‐week observation period. Of the 47 possible activities, 45 of them were recorded for at least 1 hour in the 5 weeks. Overall, 14 activities accounted for 95% of the observed bed‐hours and 31 activities accounted for the remaining 5%. CCS accounted for 82% of observed bed‐hours, NCCS accounted for 10.4%, and empty unassigned accounted for 8% (Figure 2). Using the 1‐hour cutoff for necessary services, 77% of NCCS time was nonvalue‐added, whereas 23% of it was necessary logistics; using the 2‐hour cutoff, 54% was nonvalue‐added, and 46% was necessary logistics.
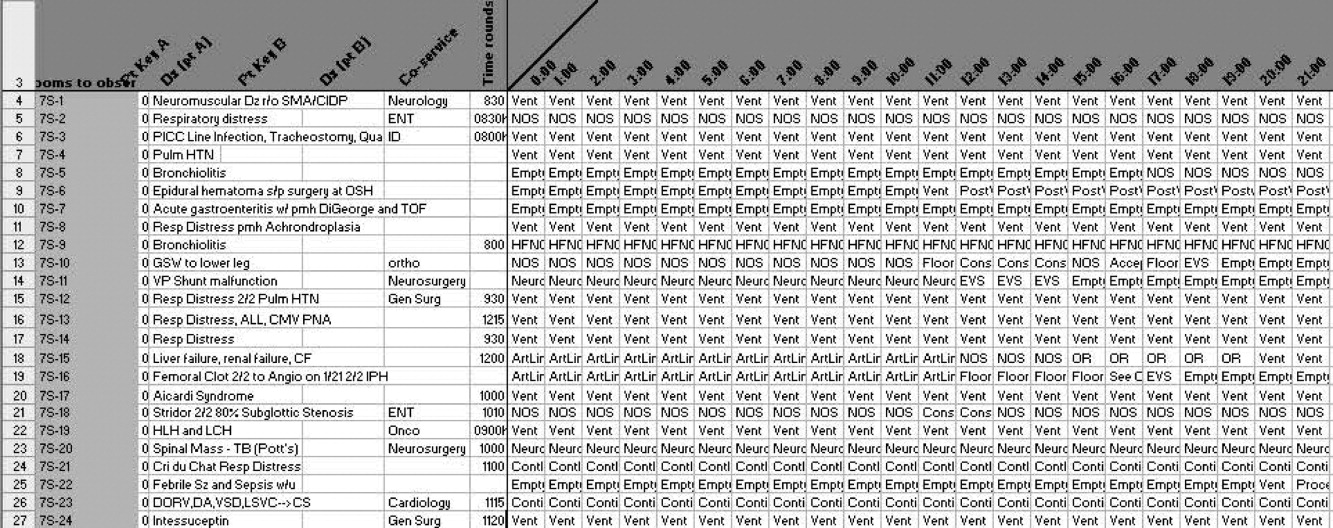
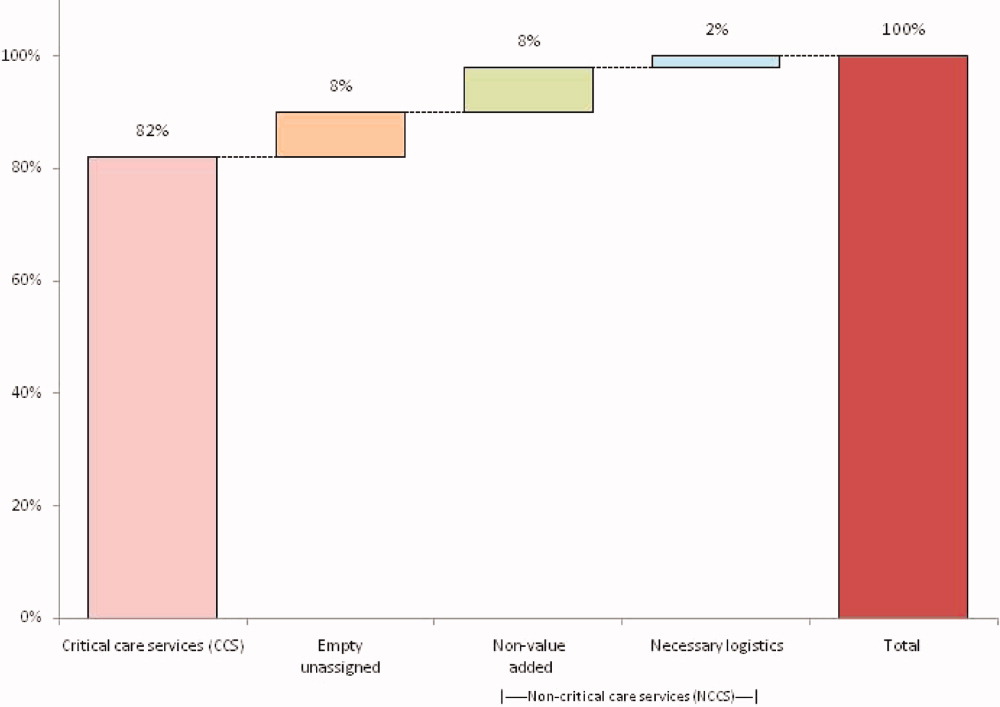
During the observation period, <1% of bed‐hours were used for CCS for overflow patients from the neonatal ICU (NICU), cardiac care unit (CCU), cardiac ICU (CICU), or progressive care unit (PCU; tracheostomy/ventilator unit). Although only 4 patients required transport to a rehabilitation facility, their wait time comprised 99 hours (<1%) of total recordings. Eight patients waited a mean of 2.6 hours for transportation home (maximum, 10 hours).
To demonstrate the cycle of room use, activities were divided into 4 categories: room preparation, critical care services, disposition pending, and postcritical care services (Figure 3). As an example of detailed data revealed by direct observation, we identified 102 instances totaling 919 hours when a patient was waiting for a bed assignment on another floor (5% of all bed‐hours). The mean wait time was 9 hours (range, 188 hours) and the median time was 5.5 hours. There were only 15 instances when floor bed assignment took 1 hour or less, and only 9 instances when it took 12 hours. Similarly, considerable time was spent on cleaning rooms between patients: only 66 of 146 instances of cleaning took 1 hour or less. The mean time for cleaning was 2.2 hours (range, 115), and the median was 2 hours. (There were 136 recorded instances of room cleaning and 10 additional episodes that were not recorded but had to be completed for the room to turnover from one patient to the next, yielding a total of 146 instances of cleaning.)
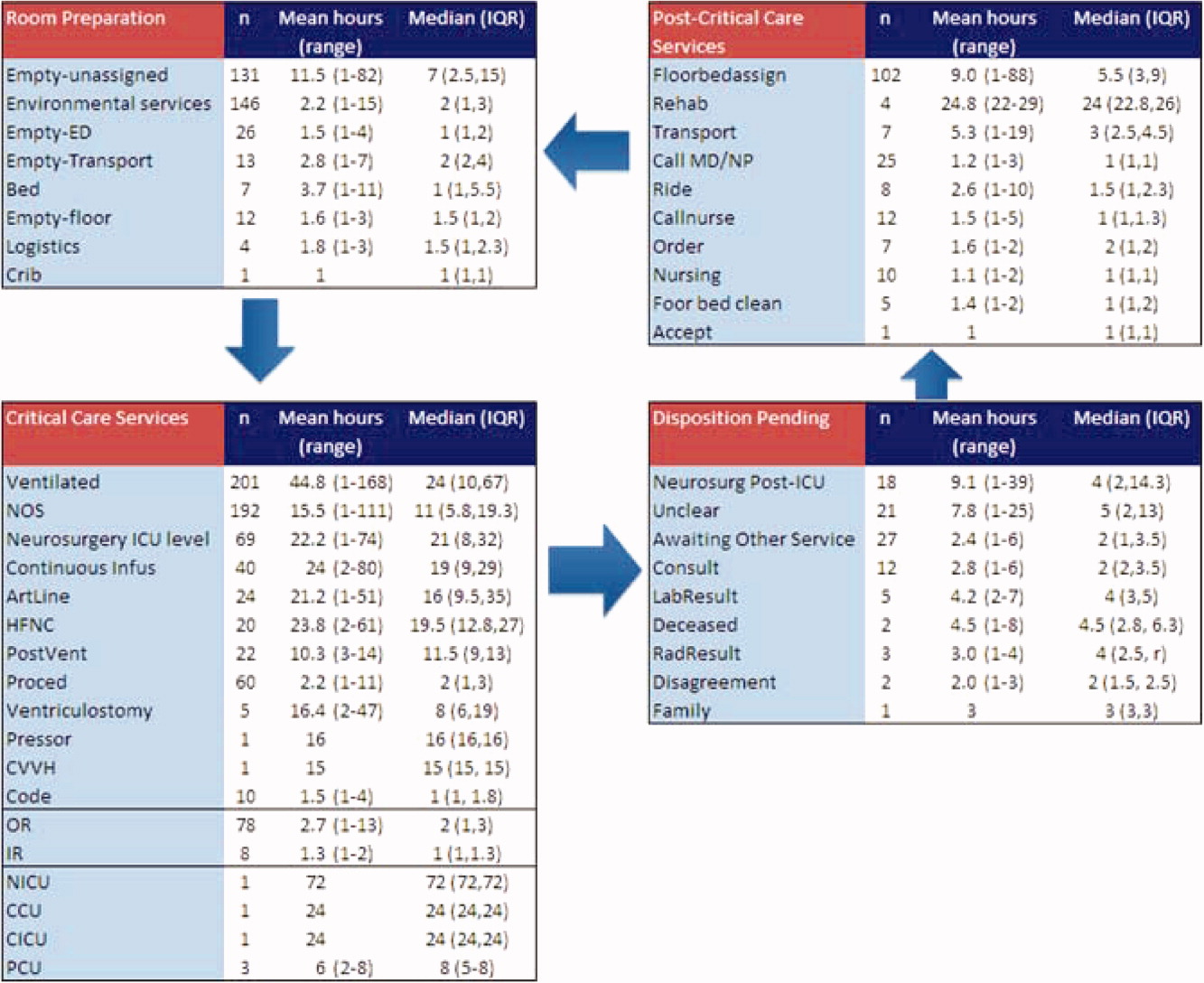
From the 824 hours of recording, we identified 200 hours (25% of time) when there were zero empty unassigned beds available in the section of the PICU being observed. Episodes of full occupancy occurred mostly on weekdays, with 23% of hours of full capacity on Thursdays, 21% on Mondays, and 21% on Wednesdays; only 8% were on Saturdays and <1% on Sundays. These 200 hours fell into 36 separate episodes of complete occupancy, each lasting 122 hours. Each patient, on average, received 3.1 hours of NCCS during each episode of full occupancy (range, 111 hours). Within these 200 hours at capacity, we identified only 15 hours (8%) when all 24 beds were used for CCS. For 72% of the time, there was at least 1 bed with NCCS, and for 37% at least 2 beds. A small portion of the time (7%), the lack of beds was affected by occupancy by patients who should have been in the NICU, CICU, CCU, or PCU.
Data collected through direct observation can be used to understand aggregated and averaged experiences, but also more specific time periods. For example, we identified 1 week with the highest consistent level of occupancy and turnover: March 915 had empty unassigned beds for only 4% of the week. Of the 168 hours in the week, 68 (40%) had full capacity. However, for 90% of the time, at least 1 bed was used for a NCCS. Other analytic options included varying the assumptions around time needed for logistics. Overall, NCCS time on necessary logistics changes from 23% to 46% using 1 hour versus 2 hours as the cutoff. For floor bed assignments, assuming that the first hour of this activity is necessary logistics and any hour thereafter is not, 817 hours were wasted. Even after assuming 2 hours of necessary logistical time (which may also include steps such as nursing and physician sign‐out to the receiving team, often not recorded in the observations), this left 715 hours of NCCS time in which patients waited to be placed elsewhere in the hospital. For room cleaning, because recordings were hourly, but room cleaning could take less time, we performed a sensitivity analysis, converting all 1‐hour recordings to half‐hour recordings to half‐hour recordings (an exaggerated shortening since industry‐standard cleaning may take longer).
Of the 219 patients directly observed, 15 were noted to be waiting for a transfer out of the PICU but experienced a change in disposition before the transfer. On average, these patients waited 8 hours for a floor bed assignment (range, 221) before reverting to a CCS, which then lasted an average of 16.5 hours (range, 149). (Included in this group are 2 patients who experienced this change in disposition twice.) In post hoc review across the 5 weeks, no patients were transferred back to the PICU within 48 hours after being transferred out. During the study period, 19 patients were discharged directly from the PICU (8 to home, 7 by transport to another facility, and 4 to rehabilitation). One patient returned to the hospital (but not the PICU) within 48 hours of being discharged home from the PICU.
During the study period, using the highest census value for recorded for each 24‐hour period and the number of beds available that day, median hospital‐wide occupancy was 93% (interquartile range, 90%96%). During the 35 days of observation, 71% of the days had occupancy >90%, 29% of days had occupancy >95%, and 3% of days had occupancy >100%.
DISCUSSION
In this direct observation of a PICU, we found high usage of beds for delivery of CCS. We identified many episodes in which the half of the PICU we observed was fully occupied (200 of 824 hours), but not necessarily delivering PICU‐level care to all patients. In fact, 75% of the full‐capacity hours had at least 1 patient receiving NCCS and 37% had at least 2. Patients waiting for a floor bed assignment represented nearly 5% of bed‐hours observed (mean 9 hours per patient). That full occupancy was not random, but rather clustered on weekdays, is consistent with other work showing that hospitals are at greater risk for midweek crowding due to the way in which scheduled admissions enter and leave.1925
Our methods provide the basis for operational analysis and improvement to patient flow, such as value stream mapping.9, 26 Process improvement work could be directed to areas of delay uncovered through this analysis and inform clinical and nonclinical management. For example, one of the key problems faced by the PICU was finding floor bed assignments for patients leaving the unit. Simply building more beds in the PICU will not solve this problemand at an estimated cost of $2 million to add a bed, it is likely not an efficient means of responding to poor flow. In these cases, the problem seems to lie downstream, and could suggest shortage of regular floor beds or inefficient bed assignment procedures within the hospital. The output also suggests that variation in nonclinical processes should be a target for improvement, such as time to clean rooms, because variation is known to be a source of nonvalue‐added time in many operations.9, 26 High occupancy on weekdays but low occupancy on weekends also emphasizes the potential for smoothing occupancy to reduce the risk of midweek crowding and to better manage bed utilization and staffing.24, 25
When seeking to reduce nonvalue‐added time, one must weigh the risks of increased efficiency against clinical outcomes. For example, if patients could be transferred out of the PICU faster, would the risk of returns to the PICU be higher? In this study, 15 patients (7%) had a change in disposition from awaiting transfer back to a CCS. The fact that transfers did not happen instantaneously may serve as a safety check to reduce rapid returns, but it is not possible for us to evaluate the reasons why patients did not actually complete the pending transfers. Specifically, we cannot determine whether the patient's clinical status objectively deteriorated, the ICU team made a judgment call to hold the patient, or the floor team refused to accept the transfer. Given this fact, although it appears in this study (and in the health care system more broadly) that there are opportunities to increase efficiency and reduce nonvalue‐added time, it is not realistic (nor advisable) that such time be reduced to zero. Along this line, one must consider separately purely nonclinical functions such as room cleaning and those that include some clinical element, such as time waiting for a patient to be transferred.
Beyond the direct findings of this study, the method should be replicable in other settings and can reveal important information about health care efficiency, capacity, and flexibility. The bottlenecks identified would have been difficult to identify through administrative record review. The exact amount of time to spend on observation may vary from place to place and would depend on the expected variation over time and the level of detail sought. In general, the more common the event and the less variation, the less time needed to observe it.
This study has several limitations that should be considered in terms of interpreting the results and in seeking to reproduce the approach. First, hourly recordings may not be discrete enough for events that took less than 1 hour. To assess the degree to which this would affect our results, we reanalyzed all NCCS by subtracting 30 minutes (0.5 hour) from all recordings, which increased total CCS from 82% to 87% and decreased NCCS by the same 5 percentage points. In a related fashion, our recordings were truncated at the start and end of each 1‐week period, so we could only observe a maximum of 168 hours for any given activity and did not record how long an activity was happening before or after the recordings started or stopped, respectively. Second, each recording could only be for 1 activity per hour. Separate from the level of granularity already noted, this also limits interpretation of critical care activities that may have been simultaneous. However, because the goal of the study was not to describe the provision of critical care services, but rather the times when they were not being delivered, this does not influence our conclusions. For movement of patients, however, we missed instances of physician and nursing calling sign‐out on patients to receiving units, as these events last less than 1 hour (and in the case of surgical patients, generally do not occur as the team provides continuous coverage). The time for such events is then included in other activities. To the extent that this may influence the results, it would increase the perceived time for nonvalue‐added services, but to a limited degree, and never by more than 59 minutes. Third, the overnight hours (11:00 PM to 8:00 AM) were not directly observed, but retrospectively recorded each morning by reviewing the records and discussing the overnight events with the clinical staff. For example, if a patient was intubated at 11:00 PM and at 8:00 AM, the observer would confirm this and record that status for the intervening hours. This is unlikely to result in a substantial impact on the findings, because the overnight hours have a relative degree of stability even for unstable patients in terms of their status of needing or not needing a CCS. Fourth, we did not evaluate the appropriateness of CCS delivered (eg, how long a patient was ventilated). Our definitions for CCS and NCSS were based on Children's Hospital of Philadelphia practices, which may not be the same as those of other facilities. The categorization of CCS was objective for activities such as ventilation or continuous infusion, but was less clear for the not otherwise specified recordings, which represented patients with a complex illness or projected organ, respiratory, cardiac, or neurological failure. These patients were not receiving a specific critical care intervention, but were deemed to need to be in the PICU as opposed to a regular floor (eg, for frequent monitoring of potential respiratory failure). It would also include patients receiving combinations of therapies more efficiently delivered in the PICU. For that, the observers relied on the judgment of clinicians (primarily nurses) to determine whether the patient needed to be in the PICU or not; if no specific reason could be provided, not otherwise specified was applied. These 192 instances accounted for 2982 aggregate bed‐hours (15% of total). It is difficult to judge the direction of bias, because overestimation of need to be in the PICU may be as likely to occur as underestimation. Fifth, the very presence of the observers may have changed behavior. Knowing that they were being observed staff may have acted with greater efficiency than otherwise. We expect that such a finding would lead to less time appearing as necessary logistics or NCCS. Finally, results may not be generalizable to other hospitals or hospital settings. There are clearly important contextual factors, not only for the location but also for the duration. For example, staffing was never an issue during the 5 weeks of observation, but there are locations where an empty bed is not necessarily usable due to lack of staffing. Nonetheless, we believe the results provide a generalizable approach and methodology for other settings (and staffing could be a reason for an empty bed).
In terms of the setting, as noted, we observed one discrete 24‐bed unit, which comprises half of the total PICU. Thus, statements that the PICU was at full capacity must be interpreted in the context that additional rooms may have been available on the other side. Patients are generally admitted alternately to each unit, so the occupancies should parallel each other. We recorded the census every 4 hours for both sides from the electronic system (Sunrise Clinical Manager [SCM]). However, this only accounts for patients physically in beds, not beds held for patients in other locations. Thus, we would expect a discrepancy between direct observation and the SCM value. Through analysis of the entire pediatric intensive care unit,* that part which observed directly, and that which we did not observe directly using census data, we think it reasonable to assert that both units of the total PICU had constrained capacity during the times we directly observed and recorded such constraint on one side.
This study demonstrates the use of direct observation for inpatient settings to learn about resource utilization and identification of value‐added services. PubMed searches for the terms efficiency, flow, process redesign, and time management bring up many more references for operating rooms than for ICUs or inpatient beds. Some examples of ICU‐directed work include videography of an ICU in Australia27 and human factor analysis in ICU nursing.5 Time‐motion studies have also been conducted on clinical staff, such as physicians.28, 29
In conclusion, we found that direct observation provided important insights into the utilization of patient rooms in an important inpatient setting. Data such as these are valuable for clinical and process improvement work, as well as understanding how best to match capacity to patient need. Finally, the methodology is reproducible for other settings and would be an additional tool to measuring and improving the efficiency and value of the health system. When appropriate, this approach can also evaluate the effectiveness of process improvement, help identify and reduce waste,13 and contribute to the growing field that merges operations management with hospital administration and clinical care: in other words, evidence‐based management.30
Acknowledgements
The authors thank Paula Agosto, Patricia Hubbs, Heidi Martin, and Annette Bollig for contributions to the study design.
In comparing direct observation to the SCM count, we found perfect concordance for 110 hours (55%) during which 0 beds were available. For the other 90 hours, SCM reported 1 bed being available in 46 hours (23%), 2 beds being available in 24 hours (12%), 3 beds being available in 17 hours (9%), and 4 beds being available in 3 hours (2%)all while we directly observed 0 beds being available. Thus, cumulatively, 90% of the hours observed with no beds had an SCM report availability of 02 beds; 99% of the time that was 03 beds. Applying this rate of mismatch to the unit that we did not observe directly, SCM reported 0 beds for 46 (23%) of the 200 hours the observation unit was full; SCM reported 1 bed available in 70 hours (35%), 2 beds open in 42 hours (21%), 3 beds open in 26 hours (13%), and 4 beds open in 16 hours (8%). Cumulatively, that is 79% of the time with 02 beds and 92% at 03 beds. From this, we conclude that the combined PICU for both sides was likely functionally full at least 158 of the 200 hours that the side we observed was full (79% 200 hours) and likely had very constrained capacity during the other 42 hours.
Patient flow refers to the management and movement of patients in health care settings and is linked to quality, safety, and cost.16 The intensive care unit (ICU) is crucial in patient flow.7, 8 The limited number of beds and the resource‐intensive services and staffing associated with them require that hospitals optimize their utilization, as is increasingly true of all hospital resources. To maximize delivery of services to patients who need them and minimize real and opportunity losses (eg, postponed surgery, diverted transports, or inability to accept patients), patients in ICU beds should receive critical care medicine/nursing services while there and be transferred or discharged when appropriate.
The time between arrival and departure from any area of the hospital, including the ICU, is considered the time when a patient is receiving needed clinical carethe value‐added portion of health care operationsand time waiting to move on to the next step.911 This period includes both necessary logistics (eg, signing out a patient or waiting a reasonable amount of time for room cleaning) and nonvalue‐added time (eg, an excessively long amount of time for room cleaning). Operations management labels nonvalue‐added time as waste, and its reduction is vital for high‐quality health care.9, 12, 13 As in other industries, one important way to understand value versus waste is through direct observation.11, 14 Although operating rooms have been the subject of several published process improvement projects to improve efficiency,1518 inpatient beds have not been the subject of such scrutiny. The objectives of this study were to generate a direct observation method and use it to describe pediatric ICU (PICU) bed utilization from a value‐added perspective.
METHODS
An interdisciplinary work group of physicians, nurses, quality improvement specialists, and 1 operations management expert developed an Excel spreadsheet to categorize hour‐by‐hour status of PICU beds. The clinicians generated a list of 27 activities. A critical care nurse trained in quality improvement piloted the list for 3 separate 4‐hour blocks over 2 weeks adding 18 activities; 2 additional activities were added during the 5 weeks of observation (Table 1). (The recording tool is provided in the Supporting Information Appendix.) Three observers with knowledge of medical terminology (2 third‐year medical students and 1 premedical student with years of experience as an emergency medical technician) were trained over 12 hours to conduct the observations. Prior to the observations, the 3 observers also spent time in the PICU, and terminology used for recordings was reviewed. Interobserver reliability was checked during 3 sets of observation circuits by all 3 observers and the principal investigator, as well as by spot checks during the study.
Activity Description | Activity Code | Total Hours Over 5 Weeks | % Total Hours Over 5 Weeks* | Mean Hours per Week* |
---|---|---|---|---|
| ||||
Ventilated patient | Vent | 8996 | 45 | 1799 |
CCSs not otherwise specified | NOS | 2982 | 15 | 596 |
Neurosurgery patient with ICU needs | NeurosurgICU | 1534 | 8 | 307 |
Room empty and unassigned | Empty‐unassigned | 1511 | 8 | 302 |
Patient on continuous infusion | ContinInfus | 958 | 5 | 192 |
Awaiting floor bed assignment | Floorbedassign | 919 | 5 | 184 |
Patient with arterial line | ArtLine | 508 | 3 | 102 |
Patient on high‐flow nasal cannula | HFNC | 475 | 2 | 95 |
Room cleaning | EVS | 318 | 2 | 64 |
Patient <12 hours after extubation | PostVent | 226 | 1 | 45 |
Patient in OR, bed being held | OR | 210 | 1 | 42 |
Neurosurgery patient, post‐ICU needs | NeurosurgPostICU | 164 | 0.8 | 33 |
No clear ICU need, but no other accepting floor or service | Unclear | 163 | 0.8 | 33 |
Patient at procedure, bed being held | Proced | 133 | 0.7 | 27 |
Patient awaiting a rehabilitation bed | Rehab | 99 | 0.5 | 20 |
Patient with ventriculostomy | Ventriculostomy | 82 | 0.4 | 16 |
Patient eligible to be in NICU | NICU | 76 | 0.4 | 15 |
Patient awaiting social work, case management, prescriptions before discharge | AwaitingOtherServ | 66 | 0.3 | 13 |
Empty bed, assigned to ED patient | Empty‐ED | 40 | 0.2 | 8 |
Empty bed, assigned to incoming transport patient | Empty‐Transport | 37 | 0.2 | 7 |
Patient awaiting transport to another facility | Transport | 37 | 0.2 | 7 |
Patient awaiting consult to determine transfer | Consult | 33 | 0.2 | 7 |
Patient awaiting physician or NP sign‐out to floor before transfer | CallMDNP | 30 | 0.2 | 6 |
PICU room needs a bed for next patient | Bed | 26 | 0.1 | 5 |
Patient eligible to be in CCU | CCU | 24 | 0.1 | 5 |
Patient eligible to be in CICU | CICU | 24 | 0.1 | 5 |
Patient awaiting laboratory result to determine transfer or discharge | LabResult | 21 | 0.1 | 4 |
Patient awaiting a ride home | Ride | 21 | 0.1 | 4 |
Empty bed, assigned to floor patient | Empty‐floor | 19 | 0.1 | 4 |
Patient awaiting nursing report to floor for transfer | Callnurse | 18 | 0.1 | 4 |
Patient eligible to be in PCU | PCU | 18 | 0.1 | 4 |
Patient on cardiac pressor | Pressor | 16 | 0.1 | 3 |
Patient actively coding | Code | 15 | 0.1 | 3 |
Patient on continuous veno‐venous hemofiltration | CVVH | 15 | 0.1 | 3 |
Nursing work needed to enable transfer out | Nursing | 11 | 0.1 | 2 |
Patient awaiting order for transfer to floor | Order | 11 | 0.1 | 2 |
Patient in interventional radiology, bed being held | IR | 10 | 0.1 | 2 |
Patient deceased in PICU room | Deceased | 9 | 0.1 | 2 |
Awaiting radiology result to clear transfer or discharge | RadResult | 9 | 0.1 | 2 |
Patient awaiting a floor bed to be cleaned for transfer out | Floorbedclean | 7 | <0.1 | 1 |
Other logistical need for an empty room | Logistics | 7 | <0.1 | 1 |
Disagreement among services for disposition | Disagreement | 4 | <0.1 | 1 |
Family request to stay in PICU | Family | 3 | <0.1 | 1 |
Awaiting accepting attending/fellow for transfer out | Accept | 1 | <0.1 | <1 |
PICU room needs a crib for next patient | Crib | 1 | <0.1 | <1 |
Patient with preventable reason for being in PICU | Prev | 0 | 0 | 0 |
PICU room needs specialty bed for next patient | SpecialBed | 0 | 0 | 0 |
Total | 19,887 | 100 |
The targeted area included 24 single‐patient rooms. The activity of each bed was recorded hourly. Real‐time recording in to the Excel spreadsheet on a dedicated laptop occurred from 8:00 AM until 11:00 PM. The most visible or critical event was recorded. Although some activities were not mutually exclusive (eg, a patient could be ventilated and on a continuous infusion simultaneously), the objective was to identify when a room was being used for any critical care service, not enumerate all of them. The observers noted overnight events that occurred from 11:00 PM to 8:00 AM in the morning by reviewing the bedside record and talking to the staff to complete each day's 24‐hour recording. The observers also recorded the hospital‐wide census and the census for the other half of the PICU every 4 hours. The observations occurred over 5 noncontiguous weeks between January 2009 and April 2009.
After all observations were complete, activities were classified as critical care services (CCS) or noncritical‐care services (NCCS). NCCSs were further divided into necessary logistics (defined for analysis purposes as the first hour of any NCCS activity) or nonvalue‐added (the second or greater hour of NCCS). A time limit of 1 hour was chosen to define necessary logistics based on a consensus that nonclinical activities optimally would not take more than 1 hour each. We also analyzed results with 2 hours as the cutoff for necessary logistics. Admission, discharge, and transfer records were reviewed to check for returns to the PICU or hospital within 48 hours of transfer or discharge from the PICU.
Analyses were conducted using Microsoft Excel (Microsoft, Redmond, WA) and Stata 10.0 (StataCorp, College Station, TX). The study was approved by the Children's Hospital of Philadelphia Institutional Review Board with waiver of consent.
RESULTS
A total of 824 hours of recordings included 19,887 bed‐hours with 219 unique patients; among them, 2 remained from the first day of recording in January to the last day in April (sample recording in Figure 1). A total of 50 patients (range, 812 per week) stayed for the entirety of each 1‐week observation period. Of the 47 possible activities, 45 of them were recorded for at least 1 hour in the 5 weeks. Overall, 14 activities accounted for 95% of the observed bed‐hours and 31 activities accounted for the remaining 5%. CCS accounted for 82% of observed bed‐hours, NCCS accounted for 10.4%, and empty unassigned accounted for 8% (Figure 2). Using the 1‐hour cutoff for necessary services, 77% of NCCS time was nonvalue‐added, whereas 23% of it was necessary logistics; using the 2‐hour cutoff, 54% was nonvalue‐added, and 46% was necessary logistics.
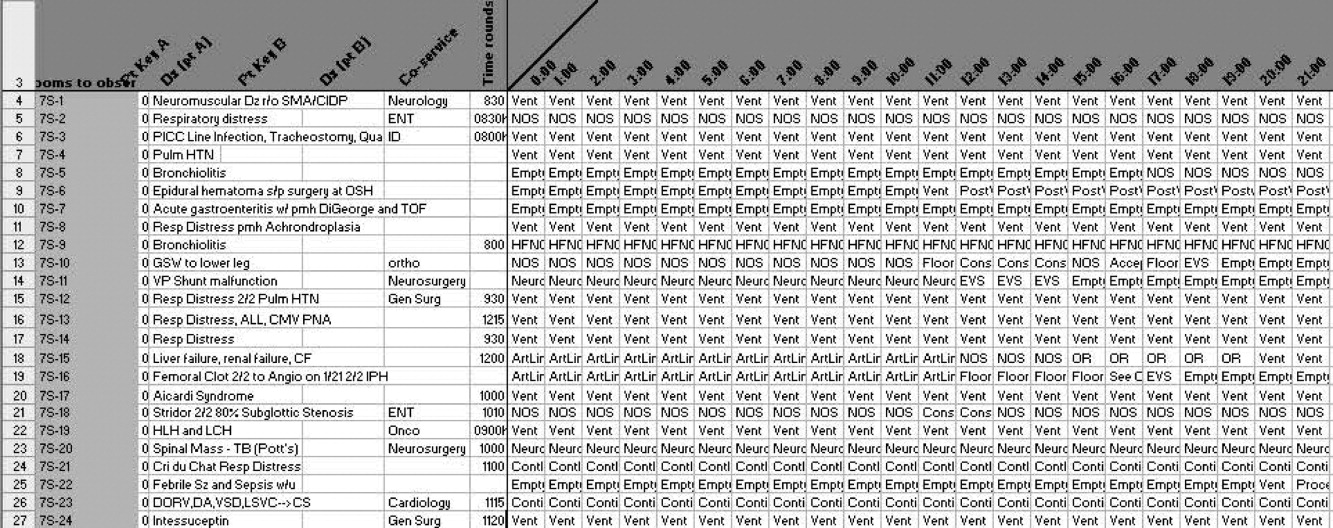
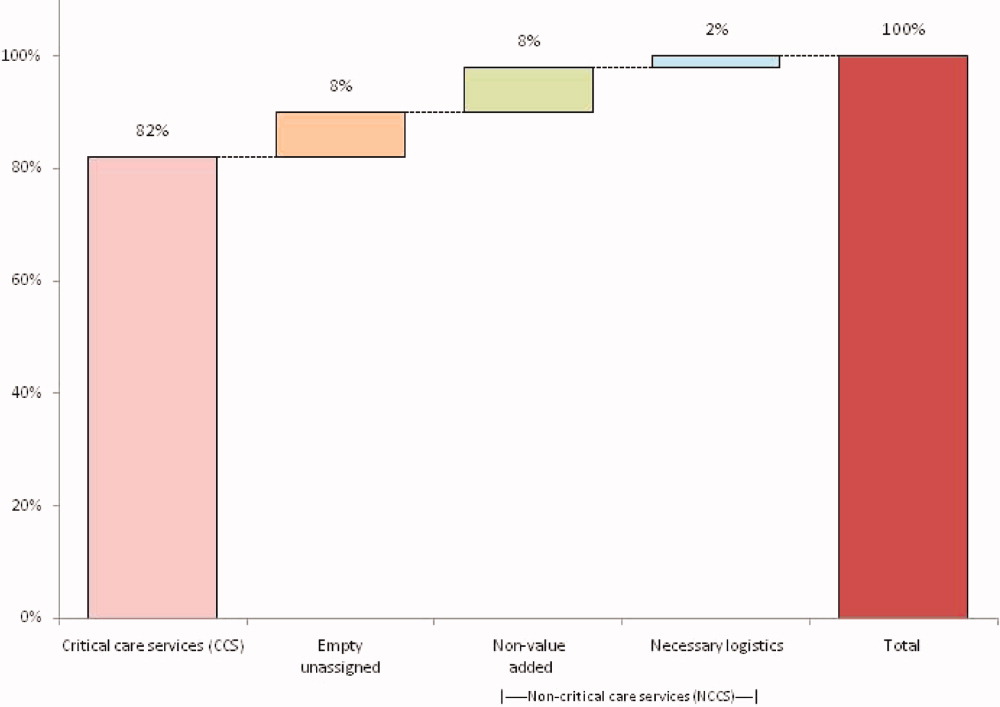
During the observation period, <1% of bed‐hours were used for CCS for overflow patients from the neonatal ICU (NICU), cardiac care unit (CCU), cardiac ICU (CICU), or progressive care unit (PCU; tracheostomy/ventilator unit). Although only 4 patients required transport to a rehabilitation facility, their wait time comprised 99 hours (<1%) of total recordings. Eight patients waited a mean of 2.6 hours for transportation home (maximum, 10 hours).
To demonstrate the cycle of room use, activities were divided into 4 categories: room preparation, critical care services, disposition pending, and postcritical care services (Figure 3). As an example of detailed data revealed by direct observation, we identified 102 instances totaling 919 hours when a patient was waiting for a bed assignment on another floor (5% of all bed‐hours). The mean wait time was 9 hours (range, 188 hours) and the median time was 5.5 hours. There were only 15 instances when floor bed assignment took 1 hour or less, and only 9 instances when it took 12 hours. Similarly, considerable time was spent on cleaning rooms between patients: only 66 of 146 instances of cleaning took 1 hour or less. The mean time for cleaning was 2.2 hours (range, 115), and the median was 2 hours. (There were 136 recorded instances of room cleaning and 10 additional episodes that were not recorded but had to be completed for the room to turnover from one patient to the next, yielding a total of 146 instances of cleaning.)
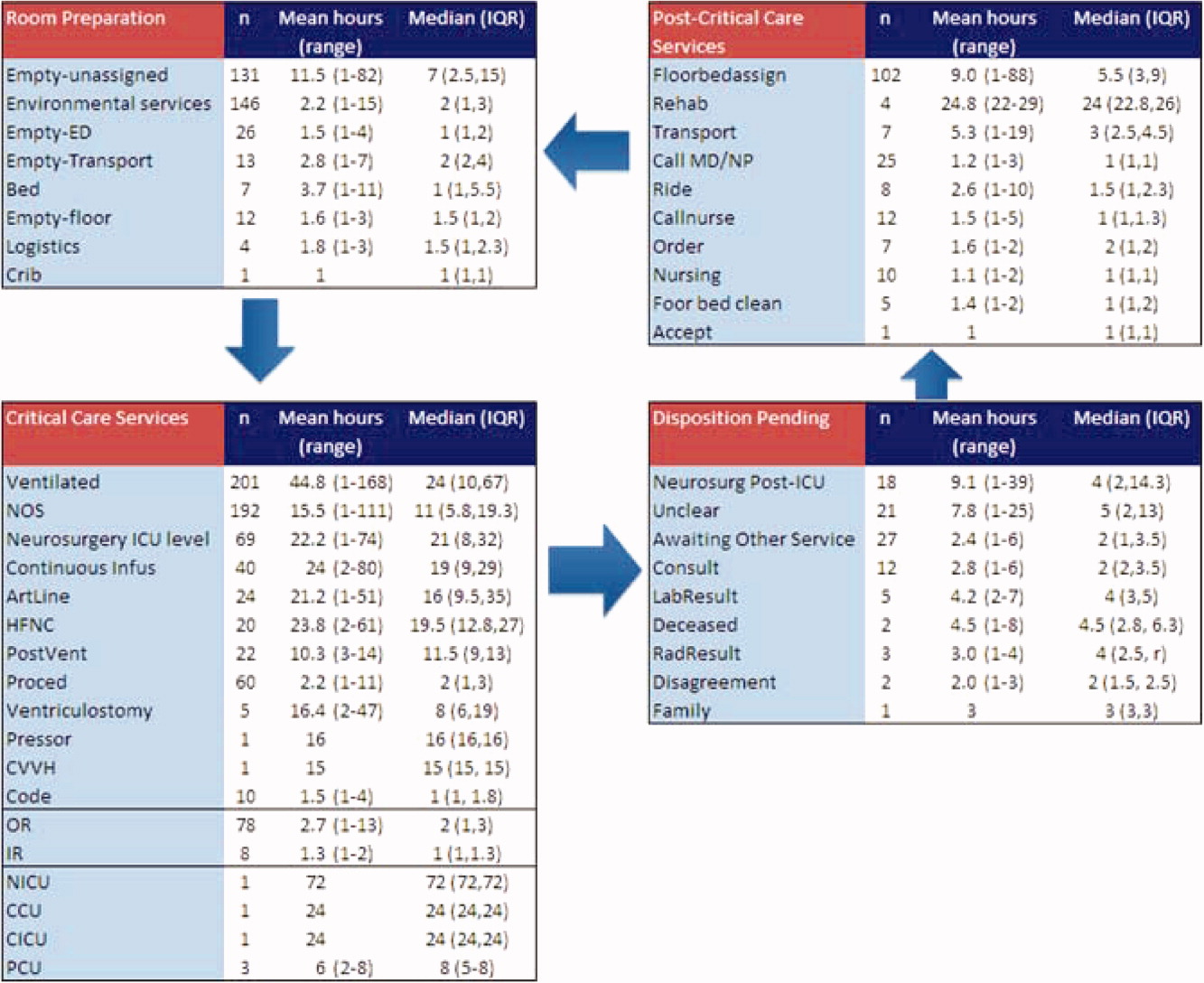
From the 824 hours of recording, we identified 200 hours (25% of time) when there were zero empty unassigned beds available in the section of the PICU being observed. Episodes of full occupancy occurred mostly on weekdays, with 23% of hours of full capacity on Thursdays, 21% on Mondays, and 21% on Wednesdays; only 8% were on Saturdays and <1% on Sundays. These 200 hours fell into 36 separate episodes of complete occupancy, each lasting 122 hours. Each patient, on average, received 3.1 hours of NCCS during each episode of full occupancy (range, 111 hours). Within these 200 hours at capacity, we identified only 15 hours (8%) when all 24 beds were used for CCS. For 72% of the time, there was at least 1 bed with NCCS, and for 37% at least 2 beds. A small portion of the time (7%), the lack of beds was affected by occupancy by patients who should have been in the NICU, CICU, CCU, or PCU.
Data collected through direct observation can be used to understand aggregated and averaged experiences, but also more specific time periods. For example, we identified 1 week with the highest consistent level of occupancy and turnover: March 915 had empty unassigned beds for only 4% of the week. Of the 168 hours in the week, 68 (40%) had full capacity. However, for 90% of the time, at least 1 bed was used for a NCCS. Other analytic options included varying the assumptions around time needed for logistics. Overall, NCCS time on necessary logistics changes from 23% to 46% using 1 hour versus 2 hours as the cutoff. For floor bed assignments, assuming that the first hour of this activity is necessary logistics and any hour thereafter is not, 817 hours were wasted. Even after assuming 2 hours of necessary logistical time (which may also include steps such as nursing and physician sign‐out to the receiving team, often not recorded in the observations), this left 715 hours of NCCS time in which patients waited to be placed elsewhere in the hospital. For room cleaning, because recordings were hourly, but room cleaning could take less time, we performed a sensitivity analysis, converting all 1‐hour recordings to half‐hour recordings to half‐hour recordings (an exaggerated shortening since industry‐standard cleaning may take longer).
Of the 219 patients directly observed, 15 were noted to be waiting for a transfer out of the PICU but experienced a change in disposition before the transfer. On average, these patients waited 8 hours for a floor bed assignment (range, 221) before reverting to a CCS, which then lasted an average of 16.5 hours (range, 149). (Included in this group are 2 patients who experienced this change in disposition twice.) In post hoc review across the 5 weeks, no patients were transferred back to the PICU within 48 hours after being transferred out. During the study period, 19 patients were discharged directly from the PICU (8 to home, 7 by transport to another facility, and 4 to rehabilitation). One patient returned to the hospital (but not the PICU) within 48 hours of being discharged home from the PICU.
During the study period, using the highest census value for recorded for each 24‐hour period and the number of beds available that day, median hospital‐wide occupancy was 93% (interquartile range, 90%96%). During the 35 days of observation, 71% of the days had occupancy >90%, 29% of days had occupancy >95%, and 3% of days had occupancy >100%.
DISCUSSION
In this direct observation of a PICU, we found high usage of beds for delivery of CCS. We identified many episodes in which the half of the PICU we observed was fully occupied (200 of 824 hours), but not necessarily delivering PICU‐level care to all patients. In fact, 75% of the full‐capacity hours had at least 1 patient receiving NCCS and 37% had at least 2. Patients waiting for a floor bed assignment represented nearly 5% of bed‐hours observed (mean 9 hours per patient). That full occupancy was not random, but rather clustered on weekdays, is consistent with other work showing that hospitals are at greater risk for midweek crowding due to the way in which scheduled admissions enter and leave.1925
Our methods provide the basis for operational analysis and improvement to patient flow, such as value stream mapping.9, 26 Process improvement work could be directed to areas of delay uncovered through this analysis and inform clinical and nonclinical management. For example, one of the key problems faced by the PICU was finding floor bed assignments for patients leaving the unit. Simply building more beds in the PICU will not solve this problemand at an estimated cost of $2 million to add a bed, it is likely not an efficient means of responding to poor flow. In these cases, the problem seems to lie downstream, and could suggest shortage of regular floor beds or inefficient bed assignment procedures within the hospital. The output also suggests that variation in nonclinical processes should be a target for improvement, such as time to clean rooms, because variation is known to be a source of nonvalue‐added time in many operations.9, 26 High occupancy on weekdays but low occupancy on weekends also emphasizes the potential for smoothing occupancy to reduce the risk of midweek crowding and to better manage bed utilization and staffing.24, 25
When seeking to reduce nonvalue‐added time, one must weigh the risks of increased efficiency against clinical outcomes. For example, if patients could be transferred out of the PICU faster, would the risk of returns to the PICU be higher? In this study, 15 patients (7%) had a change in disposition from awaiting transfer back to a CCS. The fact that transfers did not happen instantaneously may serve as a safety check to reduce rapid returns, but it is not possible for us to evaluate the reasons why patients did not actually complete the pending transfers. Specifically, we cannot determine whether the patient's clinical status objectively deteriorated, the ICU team made a judgment call to hold the patient, or the floor team refused to accept the transfer. Given this fact, although it appears in this study (and in the health care system more broadly) that there are opportunities to increase efficiency and reduce nonvalue‐added time, it is not realistic (nor advisable) that such time be reduced to zero. Along this line, one must consider separately purely nonclinical functions such as room cleaning and those that include some clinical element, such as time waiting for a patient to be transferred.
Beyond the direct findings of this study, the method should be replicable in other settings and can reveal important information about health care efficiency, capacity, and flexibility. The bottlenecks identified would have been difficult to identify through administrative record review. The exact amount of time to spend on observation may vary from place to place and would depend on the expected variation over time and the level of detail sought. In general, the more common the event and the less variation, the less time needed to observe it.
This study has several limitations that should be considered in terms of interpreting the results and in seeking to reproduce the approach. First, hourly recordings may not be discrete enough for events that took less than 1 hour. To assess the degree to which this would affect our results, we reanalyzed all NCCS by subtracting 30 minutes (0.5 hour) from all recordings, which increased total CCS from 82% to 87% and decreased NCCS by the same 5 percentage points. In a related fashion, our recordings were truncated at the start and end of each 1‐week period, so we could only observe a maximum of 168 hours for any given activity and did not record how long an activity was happening before or after the recordings started or stopped, respectively. Second, each recording could only be for 1 activity per hour. Separate from the level of granularity already noted, this also limits interpretation of critical care activities that may have been simultaneous. However, because the goal of the study was not to describe the provision of critical care services, but rather the times when they were not being delivered, this does not influence our conclusions. For movement of patients, however, we missed instances of physician and nursing calling sign‐out on patients to receiving units, as these events last less than 1 hour (and in the case of surgical patients, generally do not occur as the team provides continuous coverage). The time for such events is then included in other activities. To the extent that this may influence the results, it would increase the perceived time for nonvalue‐added services, but to a limited degree, and never by more than 59 minutes. Third, the overnight hours (11:00 PM to 8:00 AM) were not directly observed, but retrospectively recorded each morning by reviewing the records and discussing the overnight events with the clinical staff. For example, if a patient was intubated at 11:00 PM and at 8:00 AM, the observer would confirm this and record that status for the intervening hours. This is unlikely to result in a substantial impact on the findings, because the overnight hours have a relative degree of stability even for unstable patients in terms of their status of needing or not needing a CCS. Fourth, we did not evaluate the appropriateness of CCS delivered (eg, how long a patient was ventilated). Our definitions for CCS and NCSS were based on Children's Hospital of Philadelphia practices, which may not be the same as those of other facilities. The categorization of CCS was objective for activities such as ventilation or continuous infusion, but was less clear for the not otherwise specified recordings, which represented patients with a complex illness or projected organ, respiratory, cardiac, or neurological failure. These patients were not receiving a specific critical care intervention, but were deemed to need to be in the PICU as opposed to a regular floor (eg, for frequent monitoring of potential respiratory failure). It would also include patients receiving combinations of therapies more efficiently delivered in the PICU. For that, the observers relied on the judgment of clinicians (primarily nurses) to determine whether the patient needed to be in the PICU or not; if no specific reason could be provided, not otherwise specified was applied. These 192 instances accounted for 2982 aggregate bed‐hours (15% of total). It is difficult to judge the direction of bias, because overestimation of need to be in the PICU may be as likely to occur as underestimation. Fifth, the very presence of the observers may have changed behavior. Knowing that they were being observed staff may have acted with greater efficiency than otherwise. We expect that such a finding would lead to less time appearing as necessary logistics or NCCS. Finally, results may not be generalizable to other hospitals or hospital settings. There are clearly important contextual factors, not only for the location but also for the duration. For example, staffing was never an issue during the 5 weeks of observation, but there are locations where an empty bed is not necessarily usable due to lack of staffing. Nonetheless, we believe the results provide a generalizable approach and methodology for other settings (and staffing could be a reason for an empty bed).
In terms of the setting, as noted, we observed one discrete 24‐bed unit, which comprises half of the total PICU. Thus, statements that the PICU was at full capacity must be interpreted in the context that additional rooms may have been available on the other side. Patients are generally admitted alternately to each unit, so the occupancies should parallel each other. We recorded the census every 4 hours for both sides from the electronic system (Sunrise Clinical Manager [SCM]). However, this only accounts for patients physically in beds, not beds held for patients in other locations. Thus, we would expect a discrepancy between direct observation and the SCM value. Through analysis of the entire pediatric intensive care unit,* that part which observed directly, and that which we did not observe directly using census data, we think it reasonable to assert that both units of the total PICU had constrained capacity during the times we directly observed and recorded such constraint on one side.
This study demonstrates the use of direct observation for inpatient settings to learn about resource utilization and identification of value‐added services. PubMed searches for the terms efficiency, flow, process redesign, and time management bring up many more references for operating rooms than for ICUs or inpatient beds. Some examples of ICU‐directed work include videography of an ICU in Australia27 and human factor analysis in ICU nursing.5 Time‐motion studies have also been conducted on clinical staff, such as physicians.28, 29
In conclusion, we found that direct observation provided important insights into the utilization of patient rooms in an important inpatient setting. Data such as these are valuable for clinical and process improvement work, as well as understanding how best to match capacity to patient need. Finally, the methodology is reproducible for other settings and would be an additional tool to measuring and improving the efficiency and value of the health system. When appropriate, this approach can also evaluate the effectiveness of process improvement, help identify and reduce waste,13 and contribute to the growing field that merges operations management with hospital administration and clinical care: in other words, evidence‐based management.30
Acknowledgements
The authors thank Paula Agosto, Patricia Hubbs, Heidi Martin, and Annette Bollig for contributions to the study design.
In comparing direct observation to the SCM count, we found perfect concordance for 110 hours (55%) during which 0 beds were available. For the other 90 hours, SCM reported 1 bed being available in 46 hours (23%), 2 beds being available in 24 hours (12%), 3 beds being available in 17 hours (9%), and 4 beds being available in 3 hours (2%)all while we directly observed 0 beds being available. Thus, cumulatively, 90% of the hours observed with no beds had an SCM report availability of 02 beds; 99% of the time that was 03 beds. Applying this rate of mismatch to the unit that we did not observe directly, SCM reported 0 beds for 46 (23%) of the 200 hours the observation unit was full; SCM reported 1 bed available in 70 hours (35%), 2 beds open in 42 hours (21%), 3 beds open in 26 hours (13%), and 4 beds open in 16 hours (8%). Cumulatively, that is 79% of the time with 02 beds and 92% at 03 beds. From this, we conclude that the combined PICU for both sides was likely functionally full at least 158 of the 200 hours that the side we observed was full (79% 200 hours) and likely had very constrained capacity during the other 42 hours.
- The effect of hospital occupancy on emergency department length of stay and patient disposition.Acad Emerg Med.2003;10:127–133. , , , , .
- The effect of hospital bed occupancy on throughput in the pediatric emergency department.Ann Emerg Med.2009;53:767–776. , , , .
- A Comparison of in‐hospital mortality risk conferred by high hospital occupancy, differences in nurse staffing levels, weekend admission, and seasonal influenza.Medical Care.2010;48:224–232. , , , .
- Intensive care unit occupancy: making room for more patients.Crit Care Med.2009;37:1794–1795. .
- A human factors engineering conceptual framework of nursing workload and patient safety in intensive care units.Intensive Crit Care Nurs.2005;21:284–301. , .
- High level of burnout in intensivists: prevalence and associated factors.Am J Respir Crit Care Med.2007;175:686–692. , , , et al.
- Length of stay and efficiency in pediatric intensive care units.J Pediatr.1998;133:79–85. , , .
- Variability in duration of stay in pediatric intensive care units: a multiinstitutional study.J Pediatr.1996;128:35–44. , .
- Matching Supply with Demand: An Introduction to Operations Management.New York, NY:McGraw‐Hill;2006. , .
- Impact of workload on service time and patient safety: an econometric analysis of hospital operations.Management Science.2009;55:1486–1498. , .
- OPIM 631: Operations Management.Philadelphia, PA:Wharton School, University of Pennsylvania;2008. .
- From waste to value in health care.JAMA.2008;299:568–571. , , .
- Eliminating “waste” in health care.JAMA.2009;302:2481–2482. .
- Toyota Production System: Beyond Large‐scale Production.London, UK:Productivity Press;1995. .
- Interdisciplinary work flow assessment and redesign decreases operating room turnover time and allows for additional caseload.Arch Surg.2006;141:65–69. , .
- Improving operating room efficiency through process redesign.Surgery.2006;140:509–514. , , , .
- Successful strategies for improving operating room efficiency at academic institutions.Anesth Analg.1998year="1998"1998;86:896–906. , , , .
- Efficiency of the operating room suite.Am J Surg.2003;185:244–250. , , .
- Children's hospitals do not acutely respond to high occupancy.Pediatrics.2010;125:974–981. , , , et al.
- Institute for Healthcare Improvement. Smoothing elective surgical admissions. Available at: http://www.ihi.org/IHI/Topics/Flow/Patient Flow/EmergingContent/SmoothingElectiveSurgicalAdmissions.htm. Accessed October 24,2008.
- Boston Hospital Sees Big Impact from Smoothing Elective Schedule.OR Manager. Volume 20, no. 12,2004.
- Rethinking rapid response teams.JAMA.2010;304:1375–1376. , .
- Litvak E, ed.Managing Patient Flow in Hospitals: Strategies and Solutions.2nd ed.Oak Brook, IL:Joint Commission Resources;2009.
- Scheduled admissions and high occupancy at a children's hospital.J Hosp Med.2011;6:81–87. , , , , , .
- Addressing inpatient crowding by smoothing occupancy at children's hospitals.J Hosp Med.2011;6:466–473. , , , et al.
- Learning to See: Value Stream Mapping to Add Value and Eliminate MUDA.Cambridge, MA:Lean Enterprise Institute;1999. , .
- Reshaping ICU ward round practices using video‐reflexive ethnography.Qual Health Res.2008;18:380–390. , , .
- How hospitalists spend their time: insights on efficiency and safety.J Hosp Med.2006;1:88–93. , , .
- Where did the day go? A time‐motion study of hospitalists.J Hosp Med2010;5:323–238. , , , et al.
- Improving patient care by linking evidence‐based medicine and evidence‐based management.JAMA.2007;298:673–676. , , .
- The effect of hospital occupancy on emergency department length of stay and patient disposition.Acad Emerg Med.2003;10:127–133. , , , , .
- The effect of hospital bed occupancy on throughput in the pediatric emergency department.Ann Emerg Med.2009;53:767–776. , , , .
- A Comparison of in‐hospital mortality risk conferred by high hospital occupancy, differences in nurse staffing levels, weekend admission, and seasonal influenza.Medical Care.2010;48:224–232. , , , .
- Intensive care unit occupancy: making room for more patients.Crit Care Med.2009;37:1794–1795. .
- A human factors engineering conceptual framework of nursing workload and patient safety in intensive care units.Intensive Crit Care Nurs.2005;21:284–301. , .
- High level of burnout in intensivists: prevalence and associated factors.Am J Respir Crit Care Med.2007;175:686–692. , , , et al.
- Length of stay and efficiency in pediatric intensive care units.J Pediatr.1998;133:79–85. , , .
- Variability in duration of stay in pediatric intensive care units: a multiinstitutional study.J Pediatr.1996;128:35–44. , .
- Matching Supply with Demand: An Introduction to Operations Management.New York, NY:McGraw‐Hill;2006. , .
- Impact of workload on service time and patient safety: an econometric analysis of hospital operations.Management Science.2009;55:1486–1498. , .
- OPIM 631: Operations Management.Philadelphia, PA:Wharton School, University of Pennsylvania;2008. .
- From waste to value in health care.JAMA.2008;299:568–571. , , .
- Eliminating “waste” in health care.JAMA.2009;302:2481–2482. .
- Toyota Production System: Beyond Large‐scale Production.London, UK:Productivity Press;1995. .
- Interdisciplinary work flow assessment and redesign decreases operating room turnover time and allows for additional caseload.Arch Surg.2006;141:65–69. , .
- Improving operating room efficiency through process redesign.Surgery.2006;140:509–514. , , , .
- Successful strategies for improving operating room efficiency at academic institutions.Anesth Analg.1998year="1998"1998;86:896–906. , , , .
- Efficiency of the operating room suite.Am J Surg.2003;185:244–250. , , .
- Children's hospitals do not acutely respond to high occupancy.Pediatrics.2010;125:974–981. , , , et al.
- Institute for Healthcare Improvement. Smoothing elective surgical admissions. Available at: http://www.ihi.org/IHI/Topics/Flow/Patient Flow/EmergingContent/SmoothingElectiveSurgicalAdmissions.htm. Accessed October 24,2008.
- Boston Hospital Sees Big Impact from Smoothing Elective Schedule.OR Manager. Volume 20, no. 12,2004.
- Rethinking rapid response teams.JAMA.2010;304:1375–1376. , .
- Litvak E, ed.Managing Patient Flow in Hospitals: Strategies and Solutions.2nd ed.Oak Brook, IL:Joint Commission Resources;2009.
- Scheduled admissions and high occupancy at a children's hospital.J Hosp Med.2011;6:81–87. , , , , , .
- Addressing inpatient crowding by smoothing occupancy at children's hospitals.J Hosp Med.2011;6:466–473. , , , et al.
- Learning to See: Value Stream Mapping to Add Value and Eliminate MUDA.Cambridge, MA:Lean Enterprise Institute;1999. , .
- Reshaping ICU ward round practices using video‐reflexive ethnography.Qual Health Res.2008;18:380–390. , , .
- How hospitalists spend their time: insights on efficiency and safety.J Hosp Med.2006;1:88–93. , , .
- Where did the day go? A time‐motion study of hospitalists.J Hosp Med2010;5:323–238. , , , et al.
- Improving patient care by linking evidence‐based medicine and evidence‐based management.JAMA.2007;298:673–676. , , .
Copyright © 2011 Society of Hospital Medicine
Scheduled Admissions and Occupancy
Patient flow in a hospital refers to the management and movement of patients through the facility. Optimizing patient flow is considered of great importance to improvement of quality (including safety, efficiency, timeliness, equity, effectiveness, and patient‐centeredness), as well as finance, staff satisfaction, education and overall healthcare value.18 Central to concerns about patient flow at hospitals is occupancy, which is the census (number of patients at a point in time) divided by the bed capacity. Occupancy that is too high is associated with challenges to quality and access,913 while occupancy that is too low may underutilize resources and be costly.14, 15 Occupancy is determined by the pattern of admission and discharge, thus including length of stay (LOS) as a factor. While all related, admissions, census, occupancy, and LOS convey different aspects of hospital operations and may point to different opportunities to improve patient flow.
Variability in patient flow over time has been noted as a common occurrence in adult hospitals, due to uneven patterns of scheduled (elective) admissions, as well as uncontrollable variability of emergent admissions.2, 45, 16 Typically very few patients are scheduled to enter hospitals over weekends. In addition, when the admission is expected to be 5 days or less, clinical and operational staff may schedule those admissions early in the week to avoid patients staying the weekend. This artificial variability has been shown to lead to uneven levels of occupancy, with crowding on some days of the week more than others.2, 45, 16 As hospital crowding adversely affects access to emergent and elective care, quality and safety of care, and patient and staff satisfaction, many groups are focusing attention on patient flow and strategies to avoid high occupancy.19, 17 This is true for children's hospitals, as well, particularly as these scarce resources have ever increasing demand placed on them.1820
Patient flow improvements can be made by increasing efficiency of throughput, primarily measured by decreased LOS, or by addressing artificial variability in how hospital beds are used. As children's hospitals have short LOSs and are relatively efficient (as measured by standardized LOS ratios), we sought to evaluate how much artificial variability was active at 1 large children's hospital. We did this to both evaluate flow at 1 institution and to create methodology for other hospitals to use in order to better understand and improve their flow.
Our specific aims were to describe daily and monthly variability in admission, discharge, LOS, and occupancy patterns at a large children's hospital and assess the relationship between scheduled admissions and occupancy.
Methods
This retrospective administrative data analysis was performed with admission‐discharge‐transfer (ADT) data for inpatient admissions from one urban, tertiary‐care children's hospital for the period July 1, 2007 to June 30, 2008. The dataset included the date and time of all arrivals and departures from all inpatient units (including observation‐status patients), as entered by the unit clerks into the electronic ADT system. The dataset also included categorization of the admission as emergent, urgent, or elective (hereafter referred to as scheduled.) Registration staff entered these codes at or prior to admission. Using the timestamps, LOS was calculated by subtracting admission date and time from discharge date and time. An SAS macro was applied to the timestamps to calculate a hospital census for every hour of each calendar day. Peak census figures were extracted for each day. Occupancy was calculated as census over number of beds in use (monthly average). Data for the hospital's peak daily census and occupancy were utilized to analyze patterns of occupancy by day of week and month of year. To express variability, coefficient of variation (CV) (standard deviation [SD] divided by its mean) was used, as it is helpful when samples sizes are different.21
Analysis of number of admissions per day of week and month by type was performed with descriptive statistics and t‐tests for significant differences across seasons. We calculated a measure of patient hours generated by day of admission based on the LOS generated by each admission, in which the average number of admissions for each day of the week was multiplied by the average LOS (in hours) for those admissions. In order to remove outliers and focus on patients whose occupancy would affect weekly variation, we analyzed in detail the admissions with LOS 30 days and 7 days, respectively.
Statistical analyses were performed with SAS 9.2 (SAS Institute, Cary, NC), Stata 10.0 (StataCorp, College Station, TX) and Microsoft Excel (Microsoft, Redmond, WA). The study was approved by the Human Subjects Committee of the hospital's Institutional Review Board.
Results
A total of 22,310 patients were admitted over the period July 1, 2007 to June 30, 2008, including 4957 (22%) coded as scheduled and 17,353 (78%) coded as emergent. (Only 200 patients were registered as urgent and these were recoded as emergent for this analysis). Details on admission types and discharging departments are provided in Table 1. Overall, mean LOS was 5.6 days (median 2.29 days). For patients with LOS 30 days, mean LOS was 3.88 days (median 2.22 days). For patients staying 7 days, mean LOS was 2.4 days (median 1.98 days). Among patients with LOS 7 days, mean LOS for scheduled patients was longer for those admitted on Monday than on any other weekday (2.49 vs. 2.08 days, P < 0.0001). In contrast, mean LOS for emergent patients was longer for patients admitted on Friday and Saturday than the rest of the week (2.57 vs. 2.44 days, P < 0.0001).
All | Scheduled | Emergent | |
---|---|---|---|
| |||
Total Admissions, n (%)* | 22,310 | 4957 (22) | 17,353 (78) |
Median LOS (days) | 2.29 | 1.93 | 2.50 |
Mean LOS (days) (95% CI) | 5.60 (5.41, 5.79) | 4.20 (3.95, 4.45) | 5.78 (5.596.0) |
% Patients with LOS 30 days (%) | 97 | 98 | 96 |
% Patients with LOS 7 days (%) | 84 | 89 | 83 |
Medical patients at discharge, n (%) | 16,586 (74) | 2363 (48) | 14,403 (83) |
Surgical patients at discharge, n (%) | 4276 (19) | 2450 (49) | 1826 (10.5) |
Critical care patients at discharge (NICU, PICU, CICU), n (%) | 1433 (6) | 140 (3) | 1293 (7.5) |
Total admissions per month (Figure 1) averaged 1937 in October through April and 1751 in May through September (P = 0.03). Variation in the number of emergent and scheduled patients over months of the year were similar (CV 10% for each), but emergent admissions did decrease in summer (mean 1299 for June‐September vs. 1520 for the rest of the year, P = 0.003). Conversely, scheduled admissions remained relatively stable all year‐long: mean 423 per month for May through September vs. mean 413 per month for October through April (P = 0.48). Even just the summer months of June‐August, when school‐age children are on vacation, were not significantly different from other months (440 vs. 404, P = 0.2).
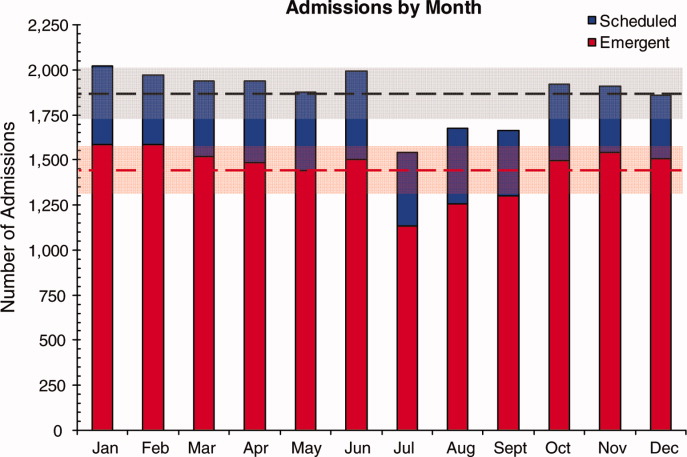
Variation in volume of admissions was large over days of the week, driven primarily by the pattern of scheduled admissions (CV 65.3%), which dropped off completely on weekends (Table 2, Figure 2). In contrast, there was much less variation in the number of emergent admissions across days of the week (CV 12%). For both emergent and scheduled admissions, more patients came in on Mondays than any other day of the week, but even more so for scheduled patients. While emergent admissions did decline on weekends, it was driven primarily by a decrease in physician referrals (ie, direct admission) from clinics (mean 7.48 per weekday vs. 0.73 per weekend day for the entire year, P < 0.001), while emergency department (ED) admissions remained relatively stable (mean 35.8 per weekday vs. 32.7 per weekend day, P = 0.08). Emergency transports were also stable (mean 7.15 per weekday vs. 6.49 per weekend day, P = 0.10).
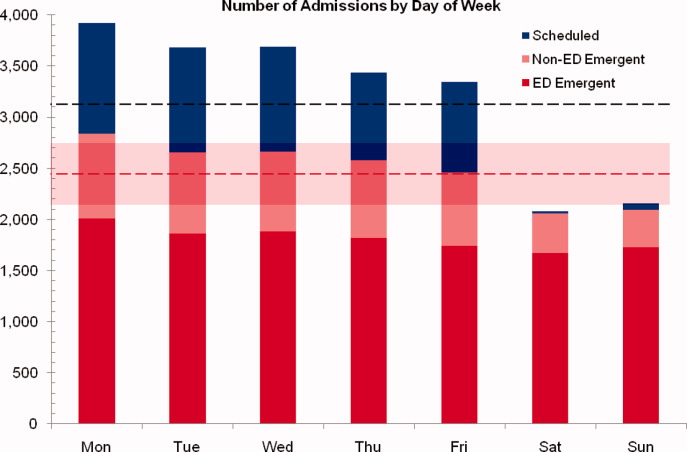
All (%) | Scheduled (%) | Emergent (%) | |
---|---|---|---|
| |||
CV on admissions by month | 8 | 10 | 10 |
CV on admissions over days of week (including weekends) | 24 | 65 | 12 |
CV on admissions over days of week (excluding weekends) | 6 | 10 | 5 |
CV on monthly occupancy over 12 months | 4 | 14 | 2 |
Although scheduled patients contributed less to the hospital's overall occupancy, they conferred most of the variability by day of week. Over the days of the week, variation for scheduled occupancy was nearly twice that for emergent occupancy (CV 19% vs. 10%). Within the higher‐volume period of October to April, the differential was even more evident (CV 19% for scheduled occupancy versus 6% for emergent).
For scheduled patients with LOS 30 days (98% of scheduled patients), Mondays and Tuesdays together accounted for 42.5% of admission volume and 44.7% of the patient‐hours generated. For scheduled patients with LOS 7 days (89% of scheduled patients), Mondays and Tuesdays together accounted for 42% of admission volume and 45.2% of the patient‐hours generated. This combined impact of volume and LOS from admissions earlier in the week (restricted to patients with LOS 7days) is displayed graphically in Figure 3, which depicts the unevenness of scheduled admissions and their time in the hospital, with many patients overlapping in the middle of the week. Together with the more steady flow of emergent patients, this variability in scheduled occupancy contributed to mid‐week crowding, with higher risk of the hospital being >90% and >95% occupied on Wednesday through Friday (Figure 4). Detailed hourly analysis (not displayed) showed this risk to be highest from Wednesday afternoon to Friday afternoon. Due to higher emergent census, certain months also had a higher risk of high occupancy at daily peak. For example, while the entire year had 50% to 60% of Wednesdays and Thursdays with occupancy >90%, during the months of November through February, 70% to 85% of those days had occupancy at that level or higher (all these patterns were seen for both stays with LOS 30 days and 7days).
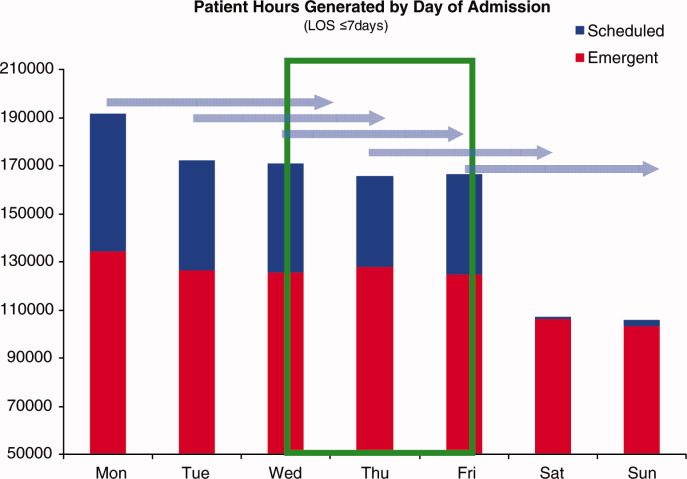
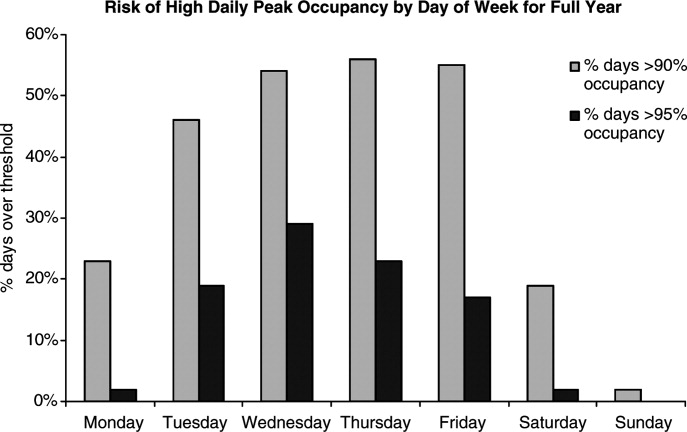
Discussion
In this study, we found that a large children's hospital was frequently at high occupancy in certain months and on certain days more than others, driven largely by predictable seasonal increases in emergent admissions and variability in scheduled admissions by day of week, respectively. Patient‐hours generated by day of admission varied as a result of both volume and LOS, both of which were larger in the early part of the week and diminished as the week progressed for scheduled admissions. But, the cumulative effect of many admissions with relatively‐longer LOS on Monday through Wednesday contributed to high occupancy on Wednesday afternoon to Friday morning, underscoring the importance of admission patterns on census later in the week. Our finding that the occupancy of scheduled patientsthe result of both the admission pattern and their LOSis also highly variable suggests that managing the inflow of scheduled patients could decrease crowding on weekdays, assure a consistent supply of capacity for regular admissions and surges, and improve the value of the delivery system.18 This inflow management would ideally consider both admissions and associated LOS, since rescheduling patients with a longer LOS (eg, 34 days) would have a greater impact on occupancy than rescheduling patients with a shorter LOS (eg, 12 days).
Not surprisingly, total admissions decreased in summer months, especially in July and August, due primarily to fewer emergent admissions. In fact, scheduled admissions per month remained relatively stable over the entire year. The decrease in summer emergent admissions may present an opportunity to stepwise shift a proportion of scheduled admissions from the spring and fall into the summer months, and winter into spring and fall, to alleviate crowding in the winter (Figure 1). Assuming clinical conditions, families and staff members were amenable to this change, hospitals with similar patterns could use this method to reduce the crowding (eg, days over 90% or 95% occupancy) that occurs in the winter.
Using patient‐hours (or days) generated by day of admission, it is evident that admission of more and longer‐stay patients at the start of the week contributes to higher occupancy later in the week (Figure 4). Mid‐week crowding could potentially contribute to a number of operational issues, including delays of new admissions, decreases in physician referrals and patient satisfaction, and an increased use of nontraditional beds (eg, treatment rooms, playrooms, doubling up single rooms) that lead to excessive patient to staff ratios and burnout for clinical staff.
The reasons for these patterns of admissions may include clinician or patient preference to avoid weekend admissions, lack of availability of particular services or resources on weekends, or concerns about safety and efficiency (due to relatively lower staffing on weekends in many hospitals).2230 While clinicians may prefer to avoid additional work on weekends, there are benefits to smoothing occupancy, including less risk of excessive work mid‐week and potential revenue opportunities. In addition, when contrasted with the estimated $1 million to $2 million cost per bed of construction, the marginal cost of staffing to provide safe, high‐quality care on weekends is dramatically lower than that of adding more beds (when faced with mid‐week crowding and unused weekend capacity). In addition, empty beds also do not generate revenue to cover fixed or variable costs, meaning that hospitals are not matching revenue to cost when there is unused capacity due to artificial variability.15, 31 Hospitals looking to make greater use of weekends, however, must be sensitive to staff concerns and the organizational dynamics of 7‐day operations, including the risk for burn‐out and attrition. Such factors should not be perceived as insurmountable barriers, particularly in light of opportunities for flexible scheduling and gain‐sharing.
Patients' and parents' preferences may partially drive admitting patterns, but a reasonable proportion of them may prefer to minimize the number of work and school days missed by being admitted near or on weekends. For example, an expected 3‐day admission could start on Friday and end on Sunday or Monday, rather than the current practice which appears to be to admit on Monday and discharge before the weekend. This may not only meet preferences among some parents to avoid missing work or school, but also by consideration of educational outcomes for hospitalized children.32
In addition, higher mean LOS for emergent patients on the weekends suggests that some services are currently unavailable on weekends to treat patients who are admitted on Fridays through Sundays.2, 25, 29, 33 More even staffing and provision of diagnostic and therapeutic services on weekends (eg, advanced radiology, consult, and laboratory services) would not only remove the barrier to weekend occupancy, it would also improve efficiency, timeliness, patient‐centeredness, and potentially effectiveness and safety for emergent patients. Running hospitals at full functionality on only 5 days of the week means that 2 out of 7 days puts patients at risk for disparate care, which may be appearing in this analysis as prolonged LOS due to lack of services over the weekenda pattern reported in the literature for adult hospitals.
Operations management and queuing theory suggest that systems function well up to 85% to 90% of capacity.34 Hospitals that plan ahead and ensure a buffer for unscheduled admissions during months or days when that demand is known to rise are less likely to cross into high occupancy. On the other hand, hospitals that do not anticipate increases in unscheduled admissions are more likely to encounter excess capacity problems.35 Aligning incentives with all staff can assist in this planning and maximize control of capacity.
Adopting the use of CV in health care operations would also be of value as a way to better express and track variation in admissions, occupancy, and discharges. Since different patient populations, different units, different hospitals, and different months have different scales, SD is not easily comparable across these settings. CV allows for comparison of variation by normalizing on the mean. In this study, it clearly differentiated the variation in elective admissions (CV 65%) over days of the week from the relative stability of emergent admissions (CV 12%). As variability and its management are important to operations, quality control, and quality improvement, use of CV can play an important role in hospital management and health services research. As days with high levels of activity may put more stress on the system, tracking this variation could lead to improvements in quality and value.
This study has several limitations. Data were analyzed for 1 children's hospital, so the analysis may or may not generally apply to other hospitals. However, in a separate study, we analyzed data from the Pediatric Health Information System database, and observed similar patterns.18 In addition, the proportion of elective patients shown in this study was similar to the national data in Kids Inpatient Database (KID, about 15% of all admissions elective).36 Moreover, the methods are reproducible for other settings, which would be useful to clinical and hospital leadership. Second, the trends depicted in the data only reflected data for one year. Third, coding of the admission as emergent or elective was done by registrars at or before arrival and may not reflect actual clinical need. In addition, those admissions coded as elective included a heterogeneous population (eg, chemotherapy to research studies).
Further studies should analyze trends for other hospitals and evaluate the effect of high peak census and high levels of variation with quality, safety, efficiency, patient satisfaction, financial, and educational outcomes for those receiving care, working, or learning at hospitals. In addition, a qualitative study that develops insights into clinician and patient/parent preferences would help answer questions in regard to usage of weekends for scheduled patients.
Conclusions
Scheduled admissions drive most variability in day‐to‐day occupancy despite the fact that they are a smaller proportion of the inpatient population. Variation in scheduled admissions by day of week provides hospitals with an opportunity to address crowding without adding beds or delaying admissions. Rather, fully utilizing capacity by smoothing occupancy over all days of the week can reduce the risk of high occupancy and thereby improve accessibility and patient/parent satisfaction. While family and staff preferences need to be considered, some combination of within‐week smoothing and shifting admissions towards summer are likely to achieve dramatic improvements in patient flow without large expenditures of capital. The key, then, is to ensure that organizational dynamic factors support these changes, so that staff members do not become stressed working at a 7‐day facility. Taken together, these strategies would better match revenue to capacity, and ultimately increase the quality and value of healthcare operations.
Acknowledgements
Authors' contributions: Study concept and design: Fieldston, Ragavan. Analysis and interpretation of data: Ragavan, Fieldston, Jayaraman, Pati. Drafting of the manuscript: Ragavan, Fieldston. Critical Revision of the manuscript for important intellectual content: Fieldston, Ragavan, Pati, Metlay. Statistical analysis: Fieldston, Jayaraman, Ragavan, Allebach. Study supervision: Fieldston, Pati, Metlay.
Additional contributions: The authors the fellows and faculty of the Robert Wood Johnson Foundation Clinical Scholars Program at the University of Pennsylvania and members of its Community Advisory Board for their suggestions to this work. They also wish to thank Tracy Kish, Jennifer Massenburg, and Brian Smith for assistance with access to and interpretation of hospital census and bed capacity data.
- Relationship between resident workload and self‐perceived learning on inpatient medicine wards: a longitudinal study.BMC Med Educ.2006;6(1):35. , , , , , .
- AHA Solutions, Patient Flow Challenges Assessment 2009. Chicago, IL.2009.
- Patient flow in hospitals: understanding and controlling it better.Front Health Serv Manage.2004;20:3–15. , .
- Managing Variability in Patient Flow is the Key to Improving Access to Care, Nursing Staffing, Quality of Care, and Reducing Its Cost. Paper presented at: Institute of Medicine; June 24,2004. .
- Managing unnecessary variability in patient demand to reduce nursing stress and improve patient safety.Jt Comm J Qual Patient Saf.2005;31(6):330–338. , , , , , .
- Developing models for patient flow and daily surge capacity research.Acad Emerg Med.2006;13(11):1109–1113. , , .
- Institute for Healthcare Improvement, Flow initiatives. 2008. Available at: http://www.ihi.org/IHI/Topics/Flow. Accessed June2010.
- Hospital workload and adverse events.Med Care.2007;45(5):448–455. , , , et al.
- Impact of admission‐day crowding on the length of stay of pediatric hospitalizations.Pediatrics.2008;121(4):e718–730. , , , , .
- Emergency department crowding, Part 1: concept, causes, and moral consequences.Ann Emerg Med.2009;53(5):605–611. , , , , .
- Emergency department overcrowding and ambulance diversion: the impact and potential solutions of extended boarding of admitted patients in the emergency department.J Emerg Med.2006;30(3):351–356. , .
- A comparison of in‐hospital mortality risk conferred by high hospital occupancy, differences in nurse staffing levels, weekend admission, and seasonal influenza.Medical Care.2010;48(3):224–232. , , , .
- The effect of hospital bed occupancy on throughput in the pediatric emergency department.Ann Emerg Med.2009;53(6):767–776.e763. . , , .
- Interpreting the Volume‐Outcome Relationship in the Context of Health Care Quality: Workshop Summary.Washington, DC:National Academies Press;2000. .
- Uncertain demand, the structure of hospital costs, and the cost of empty hospital beds.J Health Econ.1995;14(3):291–317. , .
- Variability in surgical caseload and access to intensive care services.Anesthesiology.2003;98(6):1491–1496. , , , et al.
- Effects of hospital care environment on patient mortality and nurse outcomes.J Nurs Adm.2009;39(7/8):S45–S51. , , , , .
- Children's hospitals do not acutely respond to high occupancy.Pediatrics.2010;125:974–981. , , , et al.
- Easing the strain on a pediatric tertiary care center: use of a redistribution system.Arch Pediatr Adolesc Med.2007;161(9):870–876. , .
- Lengths of stay and costs associated with Children's Hospitals.Pediatrics.2005;115:839–844. , , .
- Matching Supply with Demand: An introduction to operations management.New York:McGraw‐Hill;2006. , .
- Do hospitals provide lower quality care on weekends?Health Serv Res.2007;42:1589–1612. .
- Waiting for urgent procedures on the weekend among emergently hospitalized patients.Am J Med.2004;117:175–181. , .
- Mortality among patients admitted to hospitals on weekends as compared with weekdays.N Engl J Med.2001;345:663–668. , .
- Enhanced weekend service: an affordable means to increased hospital procedure volume.CMAJ.2005;172(4):503–504. , .
- Hospital deaths and weekend admissions‐how do we leap across a chasm?Clin Nurse Spec.2002;16:91–92. .
- Weekend birth and higher neonatal mortality: a problem of patient acuity or quality of care?J Obstet Gynecol Neonatal Nurs.2003;32(6):724–733. , .
- Weekend versus weekday admission and mortality from myocardial infarction.N Engl J Med.2007;356:1099–1109. , , , et al.
- Weekend admission and treatment of patients with renal colic: a case of avoidable variation?Urology.2009;73(4):720–724. .
- Characteristics of weekday and weekend hospital admissions.HCUP Statistical Brief.2010;87. http://www.hcup‐us.ahrq.gov/reports/statbriefs/sb87.pdf. , , .
- Hospital Costs and the Cost of Empty Hospital Beds (NBER Working Paper No. W3872).1991. , .
- The effects of timing of pediatric knee ligament surgery on short‐term academic performance in school‐aged athletes.Am J Sports Med.2009;37(9):1684–1691. , , .
- Institute for Healthcare Improvement, Smoothing Elective Surgical Admissions. Available at: http://www.ihi.org/IHI/Topics/Flow/PatientFlow/EmergingContent/SmoothingElectiveSurgicalAdmissions.htm. Accessed June2010.
- http://www.ihi.org/IHI/Topics/Flow. Accessed June2010. . Institute for Healthcare Improvement, Patient Flow Comments. Available at:
- OPIM 631: Operations Management.Wharton School, University of Pennsylvania.Philadelphia, PA.2008. .
- Agency for Healthcare Research and Quality. HCUP Databases, Healthcare Cost and Utilization Project (HCUP). 2008. Available at: www.hcup‐us.ahrq.gov/kidoverview.jsp. Accessed June2010.
Patient flow in a hospital refers to the management and movement of patients through the facility. Optimizing patient flow is considered of great importance to improvement of quality (including safety, efficiency, timeliness, equity, effectiveness, and patient‐centeredness), as well as finance, staff satisfaction, education and overall healthcare value.18 Central to concerns about patient flow at hospitals is occupancy, which is the census (number of patients at a point in time) divided by the bed capacity. Occupancy that is too high is associated with challenges to quality and access,913 while occupancy that is too low may underutilize resources and be costly.14, 15 Occupancy is determined by the pattern of admission and discharge, thus including length of stay (LOS) as a factor. While all related, admissions, census, occupancy, and LOS convey different aspects of hospital operations and may point to different opportunities to improve patient flow.
Variability in patient flow over time has been noted as a common occurrence in adult hospitals, due to uneven patterns of scheduled (elective) admissions, as well as uncontrollable variability of emergent admissions.2, 45, 16 Typically very few patients are scheduled to enter hospitals over weekends. In addition, when the admission is expected to be 5 days or less, clinical and operational staff may schedule those admissions early in the week to avoid patients staying the weekend. This artificial variability has been shown to lead to uneven levels of occupancy, with crowding on some days of the week more than others.2, 45, 16 As hospital crowding adversely affects access to emergent and elective care, quality and safety of care, and patient and staff satisfaction, many groups are focusing attention on patient flow and strategies to avoid high occupancy.19, 17 This is true for children's hospitals, as well, particularly as these scarce resources have ever increasing demand placed on them.1820
Patient flow improvements can be made by increasing efficiency of throughput, primarily measured by decreased LOS, or by addressing artificial variability in how hospital beds are used. As children's hospitals have short LOSs and are relatively efficient (as measured by standardized LOS ratios), we sought to evaluate how much artificial variability was active at 1 large children's hospital. We did this to both evaluate flow at 1 institution and to create methodology for other hospitals to use in order to better understand and improve their flow.
Our specific aims were to describe daily and monthly variability in admission, discharge, LOS, and occupancy patterns at a large children's hospital and assess the relationship between scheduled admissions and occupancy.
Methods
This retrospective administrative data analysis was performed with admission‐discharge‐transfer (ADT) data for inpatient admissions from one urban, tertiary‐care children's hospital for the period July 1, 2007 to June 30, 2008. The dataset included the date and time of all arrivals and departures from all inpatient units (including observation‐status patients), as entered by the unit clerks into the electronic ADT system. The dataset also included categorization of the admission as emergent, urgent, or elective (hereafter referred to as scheduled.) Registration staff entered these codes at or prior to admission. Using the timestamps, LOS was calculated by subtracting admission date and time from discharge date and time. An SAS macro was applied to the timestamps to calculate a hospital census for every hour of each calendar day. Peak census figures were extracted for each day. Occupancy was calculated as census over number of beds in use (monthly average). Data for the hospital's peak daily census and occupancy were utilized to analyze patterns of occupancy by day of week and month of year. To express variability, coefficient of variation (CV) (standard deviation [SD] divided by its mean) was used, as it is helpful when samples sizes are different.21
Analysis of number of admissions per day of week and month by type was performed with descriptive statistics and t‐tests for significant differences across seasons. We calculated a measure of patient hours generated by day of admission based on the LOS generated by each admission, in which the average number of admissions for each day of the week was multiplied by the average LOS (in hours) for those admissions. In order to remove outliers and focus on patients whose occupancy would affect weekly variation, we analyzed in detail the admissions with LOS 30 days and 7 days, respectively.
Statistical analyses were performed with SAS 9.2 (SAS Institute, Cary, NC), Stata 10.0 (StataCorp, College Station, TX) and Microsoft Excel (Microsoft, Redmond, WA). The study was approved by the Human Subjects Committee of the hospital's Institutional Review Board.
Results
A total of 22,310 patients were admitted over the period July 1, 2007 to June 30, 2008, including 4957 (22%) coded as scheduled and 17,353 (78%) coded as emergent. (Only 200 patients were registered as urgent and these were recoded as emergent for this analysis). Details on admission types and discharging departments are provided in Table 1. Overall, mean LOS was 5.6 days (median 2.29 days). For patients with LOS 30 days, mean LOS was 3.88 days (median 2.22 days). For patients staying 7 days, mean LOS was 2.4 days (median 1.98 days). Among patients with LOS 7 days, mean LOS for scheduled patients was longer for those admitted on Monday than on any other weekday (2.49 vs. 2.08 days, P < 0.0001). In contrast, mean LOS for emergent patients was longer for patients admitted on Friday and Saturday than the rest of the week (2.57 vs. 2.44 days, P < 0.0001).
All | Scheduled | Emergent | |
---|---|---|---|
| |||
Total Admissions, n (%)* | 22,310 | 4957 (22) | 17,353 (78) |
Median LOS (days) | 2.29 | 1.93 | 2.50 |
Mean LOS (days) (95% CI) | 5.60 (5.41, 5.79) | 4.20 (3.95, 4.45) | 5.78 (5.596.0) |
% Patients with LOS 30 days (%) | 97 | 98 | 96 |
% Patients with LOS 7 days (%) | 84 | 89 | 83 |
Medical patients at discharge, n (%) | 16,586 (74) | 2363 (48) | 14,403 (83) |
Surgical patients at discharge, n (%) | 4276 (19) | 2450 (49) | 1826 (10.5) |
Critical care patients at discharge (NICU, PICU, CICU), n (%) | 1433 (6) | 140 (3) | 1293 (7.5) |
Total admissions per month (Figure 1) averaged 1937 in October through April and 1751 in May through September (P = 0.03). Variation in the number of emergent and scheduled patients over months of the year were similar (CV 10% for each), but emergent admissions did decrease in summer (mean 1299 for June‐September vs. 1520 for the rest of the year, P = 0.003). Conversely, scheduled admissions remained relatively stable all year‐long: mean 423 per month for May through September vs. mean 413 per month for October through April (P = 0.48). Even just the summer months of June‐August, when school‐age children are on vacation, were not significantly different from other months (440 vs. 404, P = 0.2).
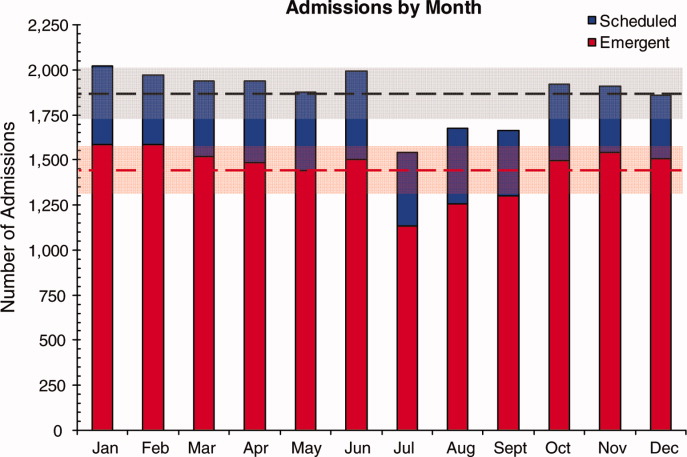
Variation in volume of admissions was large over days of the week, driven primarily by the pattern of scheduled admissions (CV 65.3%), which dropped off completely on weekends (Table 2, Figure 2). In contrast, there was much less variation in the number of emergent admissions across days of the week (CV 12%). For both emergent and scheduled admissions, more patients came in on Mondays than any other day of the week, but even more so for scheduled patients. While emergent admissions did decline on weekends, it was driven primarily by a decrease in physician referrals (ie, direct admission) from clinics (mean 7.48 per weekday vs. 0.73 per weekend day for the entire year, P < 0.001), while emergency department (ED) admissions remained relatively stable (mean 35.8 per weekday vs. 32.7 per weekend day, P = 0.08). Emergency transports were also stable (mean 7.15 per weekday vs. 6.49 per weekend day, P = 0.10).
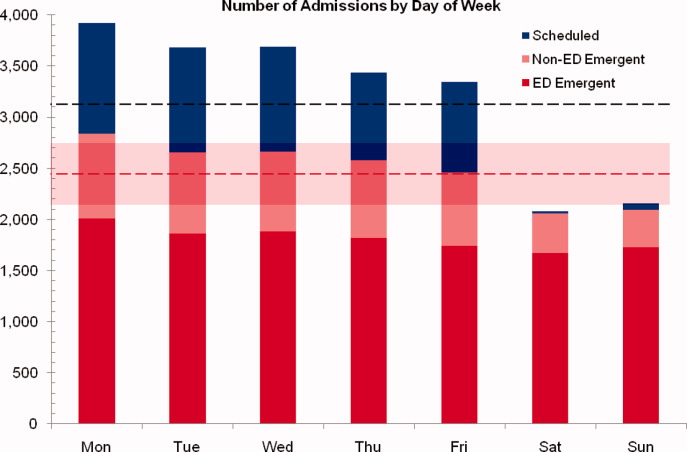
All (%) | Scheduled (%) | Emergent (%) | |
---|---|---|---|
| |||
CV on admissions by month | 8 | 10 | 10 |
CV on admissions over days of week (including weekends) | 24 | 65 | 12 |
CV on admissions over days of week (excluding weekends) | 6 | 10 | 5 |
CV on monthly occupancy over 12 months | 4 | 14 | 2 |
Although scheduled patients contributed less to the hospital's overall occupancy, they conferred most of the variability by day of week. Over the days of the week, variation for scheduled occupancy was nearly twice that for emergent occupancy (CV 19% vs. 10%). Within the higher‐volume period of October to April, the differential was even more evident (CV 19% for scheduled occupancy versus 6% for emergent).
For scheduled patients with LOS 30 days (98% of scheduled patients), Mondays and Tuesdays together accounted for 42.5% of admission volume and 44.7% of the patient‐hours generated. For scheduled patients with LOS 7 days (89% of scheduled patients), Mondays and Tuesdays together accounted for 42% of admission volume and 45.2% of the patient‐hours generated. This combined impact of volume and LOS from admissions earlier in the week (restricted to patients with LOS 7days) is displayed graphically in Figure 3, which depicts the unevenness of scheduled admissions and their time in the hospital, with many patients overlapping in the middle of the week. Together with the more steady flow of emergent patients, this variability in scheduled occupancy contributed to mid‐week crowding, with higher risk of the hospital being >90% and >95% occupied on Wednesday through Friday (Figure 4). Detailed hourly analysis (not displayed) showed this risk to be highest from Wednesday afternoon to Friday afternoon. Due to higher emergent census, certain months also had a higher risk of high occupancy at daily peak. For example, while the entire year had 50% to 60% of Wednesdays and Thursdays with occupancy >90%, during the months of November through February, 70% to 85% of those days had occupancy at that level or higher (all these patterns were seen for both stays with LOS 30 days and 7days).
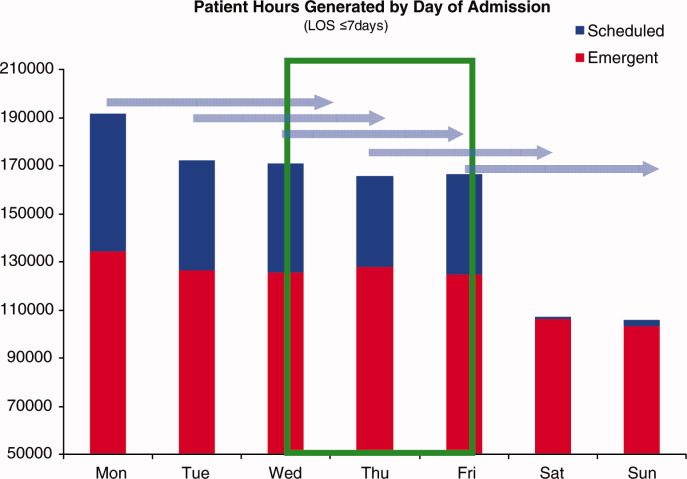
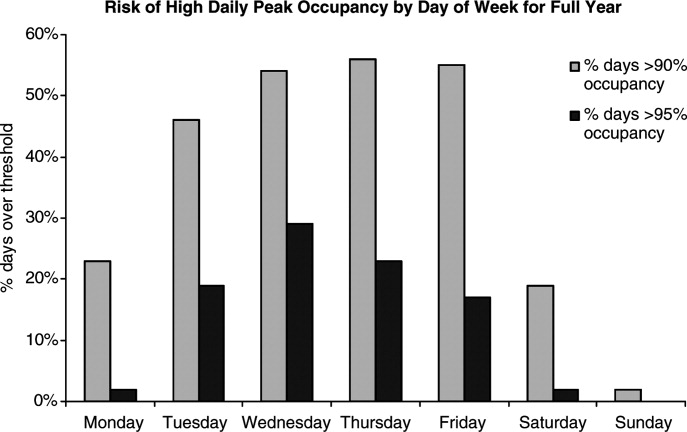
Discussion
In this study, we found that a large children's hospital was frequently at high occupancy in certain months and on certain days more than others, driven largely by predictable seasonal increases in emergent admissions and variability in scheduled admissions by day of week, respectively. Patient‐hours generated by day of admission varied as a result of both volume and LOS, both of which were larger in the early part of the week and diminished as the week progressed for scheduled admissions. But, the cumulative effect of many admissions with relatively‐longer LOS on Monday through Wednesday contributed to high occupancy on Wednesday afternoon to Friday morning, underscoring the importance of admission patterns on census later in the week. Our finding that the occupancy of scheduled patientsthe result of both the admission pattern and their LOSis also highly variable suggests that managing the inflow of scheduled patients could decrease crowding on weekdays, assure a consistent supply of capacity for regular admissions and surges, and improve the value of the delivery system.18 This inflow management would ideally consider both admissions and associated LOS, since rescheduling patients with a longer LOS (eg, 34 days) would have a greater impact on occupancy than rescheduling patients with a shorter LOS (eg, 12 days).
Not surprisingly, total admissions decreased in summer months, especially in July and August, due primarily to fewer emergent admissions. In fact, scheduled admissions per month remained relatively stable over the entire year. The decrease in summer emergent admissions may present an opportunity to stepwise shift a proportion of scheduled admissions from the spring and fall into the summer months, and winter into spring and fall, to alleviate crowding in the winter (Figure 1). Assuming clinical conditions, families and staff members were amenable to this change, hospitals with similar patterns could use this method to reduce the crowding (eg, days over 90% or 95% occupancy) that occurs in the winter.
Using patient‐hours (or days) generated by day of admission, it is evident that admission of more and longer‐stay patients at the start of the week contributes to higher occupancy later in the week (Figure 4). Mid‐week crowding could potentially contribute to a number of operational issues, including delays of new admissions, decreases in physician referrals and patient satisfaction, and an increased use of nontraditional beds (eg, treatment rooms, playrooms, doubling up single rooms) that lead to excessive patient to staff ratios and burnout for clinical staff.
The reasons for these patterns of admissions may include clinician or patient preference to avoid weekend admissions, lack of availability of particular services or resources on weekends, or concerns about safety and efficiency (due to relatively lower staffing on weekends in many hospitals).2230 While clinicians may prefer to avoid additional work on weekends, there are benefits to smoothing occupancy, including less risk of excessive work mid‐week and potential revenue opportunities. In addition, when contrasted with the estimated $1 million to $2 million cost per bed of construction, the marginal cost of staffing to provide safe, high‐quality care on weekends is dramatically lower than that of adding more beds (when faced with mid‐week crowding and unused weekend capacity). In addition, empty beds also do not generate revenue to cover fixed or variable costs, meaning that hospitals are not matching revenue to cost when there is unused capacity due to artificial variability.15, 31 Hospitals looking to make greater use of weekends, however, must be sensitive to staff concerns and the organizational dynamics of 7‐day operations, including the risk for burn‐out and attrition. Such factors should not be perceived as insurmountable barriers, particularly in light of opportunities for flexible scheduling and gain‐sharing.
Patients' and parents' preferences may partially drive admitting patterns, but a reasonable proportion of them may prefer to minimize the number of work and school days missed by being admitted near or on weekends. For example, an expected 3‐day admission could start on Friday and end on Sunday or Monday, rather than the current practice which appears to be to admit on Monday and discharge before the weekend. This may not only meet preferences among some parents to avoid missing work or school, but also by consideration of educational outcomes for hospitalized children.32
In addition, higher mean LOS for emergent patients on the weekends suggests that some services are currently unavailable on weekends to treat patients who are admitted on Fridays through Sundays.2, 25, 29, 33 More even staffing and provision of diagnostic and therapeutic services on weekends (eg, advanced radiology, consult, and laboratory services) would not only remove the barrier to weekend occupancy, it would also improve efficiency, timeliness, patient‐centeredness, and potentially effectiveness and safety for emergent patients. Running hospitals at full functionality on only 5 days of the week means that 2 out of 7 days puts patients at risk for disparate care, which may be appearing in this analysis as prolonged LOS due to lack of services over the weekenda pattern reported in the literature for adult hospitals.
Operations management and queuing theory suggest that systems function well up to 85% to 90% of capacity.34 Hospitals that plan ahead and ensure a buffer for unscheduled admissions during months or days when that demand is known to rise are less likely to cross into high occupancy. On the other hand, hospitals that do not anticipate increases in unscheduled admissions are more likely to encounter excess capacity problems.35 Aligning incentives with all staff can assist in this planning and maximize control of capacity.
Adopting the use of CV in health care operations would also be of value as a way to better express and track variation in admissions, occupancy, and discharges. Since different patient populations, different units, different hospitals, and different months have different scales, SD is not easily comparable across these settings. CV allows for comparison of variation by normalizing on the mean. In this study, it clearly differentiated the variation in elective admissions (CV 65%) over days of the week from the relative stability of emergent admissions (CV 12%). As variability and its management are important to operations, quality control, and quality improvement, use of CV can play an important role in hospital management and health services research. As days with high levels of activity may put more stress on the system, tracking this variation could lead to improvements in quality and value.
This study has several limitations. Data were analyzed for 1 children's hospital, so the analysis may or may not generally apply to other hospitals. However, in a separate study, we analyzed data from the Pediatric Health Information System database, and observed similar patterns.18 In addition, the proportion of elective patients shown in this study was similar to the national data in Kids Inpatient Database (KID, about 15% of all admissions elective).36 Moreover, the methods are reproducible for other settings, which would be useful to clinical and hospital leadership. Second, the trends depicted in the data only reflected data for one year. Third, coding of the admission as emergent or elective was done by registrars at or before arrival and may not reflect actual clinical need. In addition, those admissions coded as elective included a heterogeneous population (eg, chemotherapy to research studies).
Further studies should analyze trends for other hospitals and evaluate the effect of high peak census and high levels of variation with quality, safety, efficiency, patient satisfaction, financial, and educational outcomes for those receiving care, working, or learning at hospitals. In addition, a qualitative study that develops insights into clinician and patient/parent preferences would help answer questions in regard to usage of weekends for scheduled patients.
Conclusions
Scheduled admissions drive most variability in day‐to‐day occupancy despite the fact that they are a smaller proportion of the inpatient population. Variation in scheduled admissions by day of week provides hospitals with an opportunity to address crowding without adding beds or delaying admissions. Rather, fully utilizing capacity by smoothing occupancy over all days of the week can reduce the risk of high occupancy and thereby improve accessibility and patient/parent satisfaction. While family and staff preferences need to be considered, some combination of within‐week smoothing and shifting admissions towards summer are likely to achieve dramatic improvements in patient flow without large expenditures of capital. The key, then, is to ensure that organizational dynamic factors support these changes, so that staff members do not become stressed working at a 7‐day facility. Taken together, these strategies would better match revenue to capacity, and ultimately increase the quality and value of healthcare operations.
Acknowledgements
Authors' contributions: Study concept and design: Fieldston, Ragavan. Analysis and interpretation of data: Ragavan, Fieldston, Jayaraman, Pati. Drafting of the manuscript: Ragavan, Fieldston. Critical Revision of the manuscript for important intellectual content: Fieldston, Ragavan, Pati, Metlay. Statistical analysis: Fieldston, Jayaraman, Ragavan, Allebach. Study supervision: Fieldston, Pati, Metlay.
Additional contributions: The authors the fellows and faculty of the Robert Wood Johnson Foundation Clinical Scholars Program at the University of Pennsylvania and members of its Community Advisory Board for their suggestions to this work. They also wish to thank Tracy Kish, Jennifer Massenburg, and Brian Smith for assistance with access to and interpretation of hospital census and bed capacity data.
Patient flow in a hospital refers to the management and movement of patients through the facility. Optimizing patient flow is considered of great importance to improvement of quality (including safety, efficiency, timeliness, equity, effectiveness, and patient‐centeredness), as well as finance, staff satisfaction, education and overall healthcare value.18 Central to concerns about patient flow at hospitals is occupancy, which is the census (number of patients at a point in time) divided by the bed capacity. Occupancy that is too high is associated with challenges to quality and access,913 while occupancy that is too low may underutilize resources and be costly.14, 15 Occupancy is determined by the pattern of admission and discharge, thus including length of stay (LOS) as a factor. While all related, admissions, census, occupancy, and LOS convey different aspects of hospital operations and may point to different opportunities to improve patient flow.
Variability in patient flow over time has been noted as a common occurrence in adult hospitals, due to uneven patterns of scheduled (elective) admissions, as well as uncontrollable variability of emergent admissions.2, 45, 16 Typically very few patients are scheduled to enter hospitals over weekends. In addition, when the admission is expected to be 5 days or less, clinical and operational staff may schedule those admissions early in the week to avoid patients staying the weekend. This artificial variability has been shown to lead to uneven levels of occupancy, with crowding on some days of the week more than others.2, 45, 16 As hospital crowding adversely affects access to emergent and elective care, quality and safety of care, and patient and staff satisfaction, many groups are focusing attention on patient flow and strategies to avoid high occupancy.19, 17 This is true for children's hospitals, as well, particularly as these scarce resources have ever increasing demand placed on them.1820
Patient flow improvements can be made by increasing efficiency of throughput, primarily measured by decreased LOS, or by addressing artificial variability in how hospital beds are used. As children's hospitals have short LOSs and are relatively efficient (as measured by standardized LOS ratios), we sought to evaluate how much artificial variability was active at 1 large children's hospital. We did this to both evaluate flow at 1 institution and to create methodology for other hospitals to use in order to better understand and improve their flow.
Our specific aims were to describe daily and monthly variability in admission, discharge, LOS, and occupancy patterns at a large children's hospital and assess the relationship between scheduled admissions and occupancy.
Methods
This retrospective administrative data analysis was performed with admission‐discharge‐transfer (ADT) data for inpatient admissions from one urban, tertiary‐care children's hospital for the period July 1, 2007 to June 30, 2008. The dataset included the date and time of all arrivals and departures from all inpatient units (including observation‐status patients), as entered by the unit clerks into the electronic ADT system. The dataset also included categorization of the admission as emergent, urgent, or elective (hereafter referred to as scheduled.) Registration staff entered these codes at or prior to admission. Using the timestamps, LOS was calculated by subtracting admission date and time from discharge date and time. An SAS macro was applied to the timestamps to calculate a hospital census for every hour of each calendar day. Peak census figures were extracted for each day. Occupancy was calculated as census over number of beds in use (monthly average). Data for the hospital's peak daily census and occupancy were utilized to analyze patterns of occupancy by day of week and month of year. To express variability, coefficient of variation (CV) (standard deviation [SD] divided by its mean) was used, as it is helpful when samples sizes are different.21
Analysis of number of admissions per day of week and month by type was performed with descriptive statistics and t‐tests for significant differences across seasons. We calculated a measure of patient hours generated by day of admission based on the LOS generated by each admission, in which the average number of admissions for each day of the week was multiplied by the average LOS (in hours) for those admissions. In order to remove outliers and focus on patients whose occupancy would affect weekly variation, we analyzed in detail the admissions with LOS 30 days and 7 days, respectively.
Statistical analyses were performed with SAS 9.2 (SAS Institute, Cary, NC), Stata 10.0 (StataCorp, College Station, TX) and Microsoft Excel (Microsoft, Redmond, WA). The study was approved by the Human Subjects Committee of the hospital's Institutional Review Board.
Results
A total of 22,310 patients were admitted over the period July 1, 2007 to June 30, 2008, including 4957 (22%) coded as scheduled and 17,353 (78%) coded as emergent. (Only 200 patients were registered as urgent and these were recoded as emergent for this analysis). Details on admission types and discharging departments are provided in Table 1. Overall, mean LOS was 5.6 days (median 2.29 days). For patients with LOS 30 days, mean LOS was 3.88 days (median 2.22 days). For patients staying 7 days, mean LOS was 2.4 days (median 1.98 days). Among patients with LOS 7 days, mean LOS for scheduled patients was longer for those admitted on Monday than on any other weekday (2.49 vs. 2.08 days, P < 0.0001). In contrast, mean LOS for emergent patients was longer for patients admitted on Friday and Saturday than the rest of the week (2.57 vs. 2.44 days, P < 0.0001).
All | Scheduled | Emergent | |
---|---|---|---|
| |||
Total Admissions, n (%)* | 22,310 | 4957 (22) | 17,353 (78) |
Median LOS (days) | 2.29 | 1.93 | 2.50 |
Mean LOS (days) (95% CI) | 5.60 (5.41, 5.79) | 4.20 (3.95, 4.45) | 5.78 (5.596.0) |
% Patients with LOS 30 days (%) | 97 | 98 | 96 |
% Patients with LOS 7 days (%) | 84 | 89 | 83 |
Medical patients at discharge, n (%) | 16,586 (74) | 2363 (48) | 14,403 (83) |
Surgical patients at discharge, n (%) | 4276 (19) | 2450 (49) | 1826 (10.5) |
Critical care patients at discharge (NICU, PICU, CICU), n (%) | 1433 (6) | 140 (3) | 1293 (7.5) |
Total admissions per month (Figure 1) averaged 1937 in October through April and 1751 in May through September (P = 0.03). Variation in the number of emergent and scheduled patients over months of the year were similar (CV 10% for each), but emergent admissions did decrease in summer (mean 1299 for June‐September vs. 1520 for the rest of the year, P = 0.003). Conversely, scheduled admissions remained relatively stable all year‐long: mean 423 per month for May through September vs. mean 413 per month for October through April (P = 0.48). Even just the summer months of June‐August, when school‐age children are on vacation, were not significantly different from other months (440 vs. 404, P = 0.2).
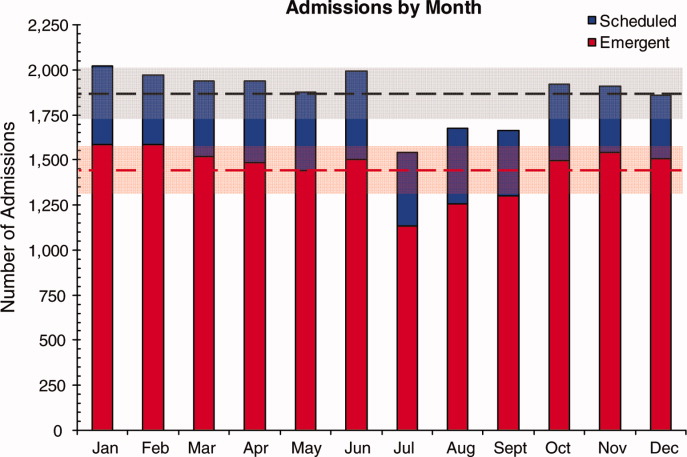
Variation in volume of admissions was large over days of the week, driven primarily by the pattern of scheduled admissions (CV 65.3%), which dropped off completely on weekends (Table 2, Figure 2). In contrast, there was much less variation in the number of emergent admissions across days of the week (CV 12%). For both emergent and scheduled admissions, more patients came in on Mondays than any other day of the week, but even more so for scheduled patients. While emergent admissions did decline on weekends, it was driven primarily by a decrease in physician referrals (ie, direct admission) from clinics (mean 7.48 per weekday vs. 0.73 per weekend day for the entire year, P < 0.001), while emergency department (ED) admissions remained relatively stable (mean 35.8 per weekday vs. 32.7 per weekend day, P = 0.08). Emergency transports were also stable (mean 7.15 per weekday vs. 6.49 per weekend day, P = 0.10).
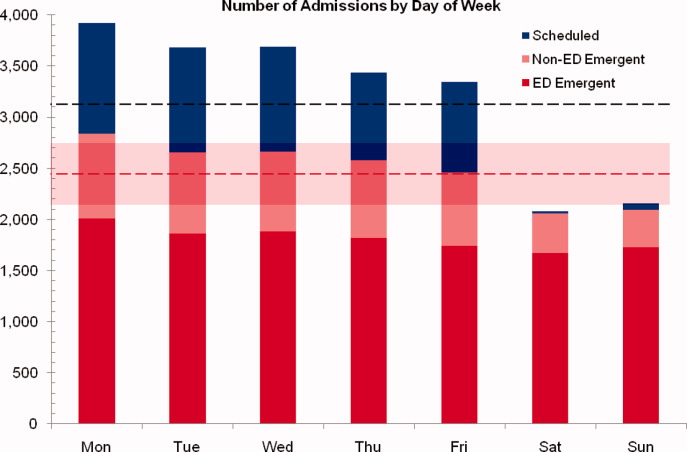
All (%) | Scheduled (%) | Emergent (%) | |
---|---|---|---|
| |||
CV on admissions by month | 8 | 10 | 10 |
CV on admissions over days of week (including weekends) | 24 | 65 | 12 |
CV on admissions over days of week (excluding weekends) | 6 | 10 | 5 |
CV on monthly occupancy over 12 months | 4 | 14 | 2 |
Although scheduled patients contributed less to the hospital's overall occupancy, they conferred most of the variability by day of week. Over the days of the week, variation for scheduled occupancy was nearly twice that for emergent occupancy (CV 19% vs. 10%). Within the higher‐volume period of October to April, the differential was even more evident (CV 19% for scheduled occupancy versus 6% for emergent).
For scheduled patients with LOS 30 days (98% of scheduled patients), Mondays and Tuesdays together accounted for 42.5% of admission volume and 44.7% of the patient‐hours generated. For scheduled patients with LOS 7 days (89% of scheduled patients), Mondays and Tuesdays together accounted for 42% of admission volume and 45.2% of the patient‐hours generated. This combined impact of volume and LOS from admissions earlier in the week (restricted to patients with LOS 7days) is displayed graphically in Figure 3, which depicts the unevenness of scheduled admissions and their time in the hospital, with many patients overlapping in the middle of the week. Together with the more steady flow of emergent patients, this variability in scheduled occupancy contributed to mid‐week crowding, with higher risk of the hospital being >90% and >95% occupied on Wednesday through Friday (Figure 4). Detailed hourly analysis (not displayed) showed this risk to be highest from Wednesday afternoon to Friday afternoon. Due to higher emergent census, certain months also had a higher risk of high occupancy at daily peak. For example, while the entire year had 50% to 60% of Wednesdays and Thursdays with occupancy >90%, during the months of November through February, 70% to 85% of those days had occupancy at that level or higher (all these patterns were seen for both stays with LOS 30 days and 7days).
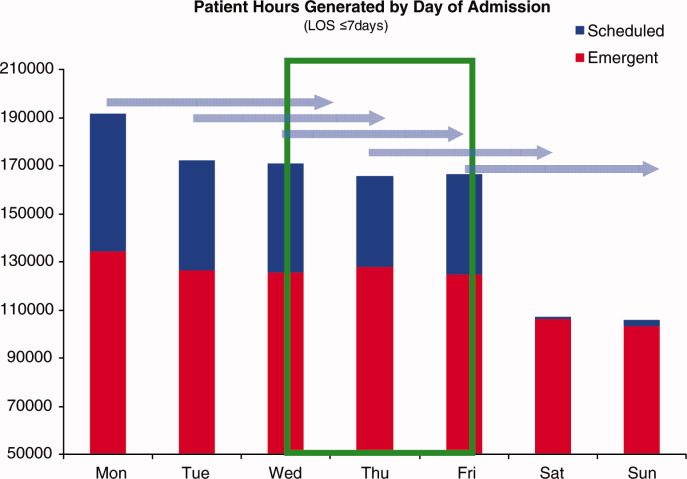
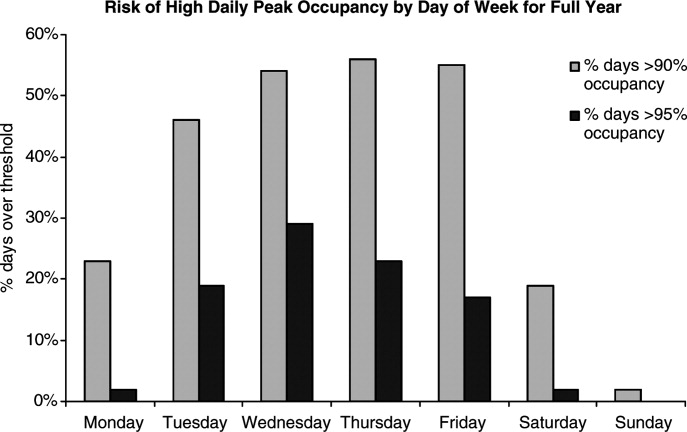
Discussion
In this study, we found that a large children's hospital was frequently at high occupancy in certain months and on certain days more than others, driven largely by predictable seasonal increases in emergent admissions and variability in scheduled admissions by day of week, respectively. Patient‐hours generated by day of admission varied as a result of both volume and LOS, both of which were larger in the early part of the week and diminished as the week progressed for scheduled admissions. But, the cumulative effect of many admissions with relatively‐longer LOS on Monday through Wednesday contributed to high occupancy on Wednesday afternoon to Friday morning, underscoring the importance of admission patterns on census later in the week. Our finding that the occupancy of scheduled patientsthe result of both the admission pattern and their LOSis also highly variable suggests that managing the inflow of scheduled patients could decrease crowding on weekdays, assure a consistent supply of capacity for regular admissions and surges, and improve the value of the delivery system.18 This inflow management would ideally consider both admissions and associated LOS, since rescheduling patients with a longer LOS (eg, 34 days) would have a greater impact on occupancy than rescheduling patients with a shorter LOS (eg, 12 days).
Not surprisingly, total admissions decreased in summer months, especially in July and August, due primarily to fewer emergent admissions. In fact, scheduled admissions per month remained relatively stable over the entire year. The decrease in summer emergent admissions may present an opportunity to stepwise shift a proportion of scheduled admissions from the spring and fall into the summer months, and winter into spring and fall, to alleviate crowding in the winter (Figure 1). Assuming clinical conditions, families and staff members were amenable to this change, hospitals with similar patterns could use this method to reduce the crowding (eg, days over 90% or 95% occupancy) that occurs in the winter.
Using patient‐hours (or days) generated by day of admission, it is evident that admission of more and longer‐stay patients at the start of the week contributes to higher occupancy later in the week (Figure 4). Mid‐week crowding could potentially contribute to a number of operational issues, including delays of new admissions, decreases in physician referrals and patient satisfaction, and an increased use of nontraditional beds (eg, treatment rooms, playrooms, doubling up single rooms) that lead to excessive patient to staff ratios and burnout for clinical staff.
The reasons for these patterns of admissions may include clinician or patient preference to avoid weekend admissions, lack of availability of particular services or resources on weekends, or concerns about safety and efficiency (due to relatively lower staffing on weekends in many hospitals).2230 While clinicians may prefer to avoid additional work on weekends, there are benefits to smoothing occupancy, including less risk of excessive work mid‐week and potential revenue opportunities. In addition, when contrasted with the estimated $1 million to $2 million cost per bed of construction, the marginal cost of staffing to provide safe, high‐quality care on weekends is dramatically lower than that of adding more beds (when faced with mid‐week crowding and unused weekend capacity). In addition, empty beds also do not generate revenue to cover fixed or variable costs, meaning that hospitals are not matching revenue to cost when there is unused capacity due to artificial variability.15, 31 Hospitals looking to make greater use of weekends, however, must be sensitive to staff concerns and the organizational dynamics of 7‐day operations, including the risk for burn‐out and attrition. Such factors should not be perceived as insurmountable barriers, particularly in light of opportunities for flexible scheduling and gain‐sharing.
Patients' and parents' preferences may partially drive admitting patterns, but a reasonable proportion of them may prefer to minimize the number of work and school days missed by being admitted near or on weekends. For example, an expected 3‐day admission could start on Friday and end on Sunday or Monday, rather than the current practice which appears to be to admit on Monday and discharge before the weekend. This may not only meet preferences among some parents to avoid missing work or school, but also by consideration of educational outcomes for hospitalized children.32
In addition, higher mean LOS for emergent patients on the weekends suggests that some services are currently unavailable on weekends to treat patients who are admitted on Fridays through Sundays.2, 25, 29, 33 More even staffing and provision of diagnostic and therapeutic services on weekends (eg, advanced radiology, consult, and laboratory services) would not only remove the barrier to weekend occupancy, it would also improve efficiency, timeliness, patient‐centeredness, and potentially effectiveness and safety for emergent patients. Running hospitals at full functionality on only 5 days of the week means that 2 out of 7 days puts patients at risk for disparate care, which may be appearing in this analysis as prolonged LOS due to lack of services over the weekenda pattern reported in the literature for adult hospitals.
Operations management and queuing theory suggest that systems function well up to 85% to 90% of capacity.34 Hospitals that plan ahead and ensure a buffer for unscheduled admissions during months or days when that demand is known to rise are less likely to cross into high occupancy. On the other hand, hospitals that do not anticipate increases in unscheduled admissions are more likely to encounter excess capacity problems.35 Aligning incentives with all staff can assist in this planning and maximize control of capacity.
Adopting the use of CV in health care operations would also be of value as a way to better express and track variation in admissions, occupancy, and discharges. Since different patient populations, different units, different hospitals, and different months have different scales, SD is not easily comparable across these settings. CV allows for comparison of variation by normalizing on the mean. In this study, it clearly differentiated the variation in elective admissions (CV 65%) over days of the week from the relative stability of emergent admissions (CV 12%). As variability and its management are important to operations, quality control, and quality improvement, use of CV can play an important role in hospital management and health services research. As days with high levels of activity may put more stress on the system, tracking this variation could lead to improvements in quality and value.
This study has several limitations. Data were analyzed for 1 children's hospital, so the analysis may or may not generally apply to other hospitals. However, in a separate study, we analyzed data from the Pediatric Health Information System database, and observed similar patterns.18 In addition, the proportion of elective patients shown in this study was similar to the national data in Kids Inpatient Database (KID, about 15% of all admissions elective).36 Moreover, the methods are reproducible for other settings, which would be useful to clinical and hospital leadership. Second, the trends depicted in the data only reflected data for one year. Third, coding of the admission as emergent or elective was done by registrars at or before arrival and may not reflect actual clinical need. In addition, those admissions coded as elective included a heterogeneous population (eg, chemotherapy to research studies).
Further studies should analyze trends for other hospitals and evaluate the effect of high peak census and high levels of variation with quality, safety, efficiency, patient satisfaction, financial, and educational outcomes for those receiving care, working, or learning at hospitals. In addition, a qualitative study that develops insights into clinician and patient/parent preferences would help answer questions in regard to usage of weekends for scheduled patients.
Conclusions
Scheduled admissions drive most variability in day‐to‐day occupancy despite the fact that they are a smaller proportion of the inpatient population. Variation in scheduled admissions by day of week provides hospitals with an opportunity to address crowding without adding beds or delaying admissions. Rather, fully utilizing capacity by smoothing occupancy over all days of the week can reduce the risk of high occupancy and thereby improve accessibility and patient/parent satisfaction. While family and staff preferences need to be considered, some combination of within‐week smoothing and shifting admissions towards summer are likely to achieve dramatic improvements in patient flow without large expenditures of capital. The key, then, is to ensure that organizational dynamic factors support these changes, so that staff members do not become stressed working at a 7‐day facility. Taken together, these strategies would better match revenue to capacity, and ultimately increase the quality and value of healthcare operations.
Acknowledgements
Authors' contributions: Study concept and design: Fieldston, Ragavan. Analysis and interpretation of data: Ragavan, Fieldston, Jayaraman, Pati. Drafting of the manuscript: Ragavan, Fieldston. Critical Revision of the manuscript for important intellectual content: Fieldston, Ragavan, Pati, Metlay. Statistical analysis: Fieldston, Jayaraman, Ragavan, Allebach. Study supervision: Fieldston, Pati, Metlay.
Additional contributions: The authors the fellows and faculty of the Robert Wood Johnson Foundation Clinical Scholars Program at the University of Pennsylvania and members of its Community Advisory Board for their suggestions to this work. They also wish to thank Tracy Kish, Jennifer Massenburg, and Brian Smith for assistance with access to and interpretation of hospital census and bed capacity data.
- Relationship between resident workload and self‐perceived learning on inpatient medicine wards: a longitudinal study.BMC Med Educ.2006;6(1):35. , , , , , .
- AHA Solutions, Patient Flow Challenges Assessment 2009. Chicago, IL.2009.
- Patient flow in hospitals: understanding and controlling it better.Front Health Serv Manage.2004;20:3–15. , .
- Managing Variability in Patient Flow is the Key to Improving Access to Care, Nursing Staffing, Quality of Care, and Reducing Its Cost. Paper presented at: Institute of Medicine; June 24,2004. .
- Managing unnecessary variability in patient demand to reduce nursing stress and improve patient safety.Jt Comm J Qual Patient Saf.2005;31(6):330–338. , , , , , .
- Developing models for patient flow and daily surge capacity research.Acad Emerg Med.2006;13(11):1109–1113. , , .
- Institute for Healthcare Improvement, Flow initiatives. 2008. Available at: http://www.ihi.org/IHI/Topics/Flow. Accessed June2010.
- Hospital workload and adverse events.Med Care.2007;45(5):448–455. , , , et al.
- Impact of admission‐day crowding on the length of stay of pediatric hospitalizations.Pediatrics.2008;121(4):e718–730. , , , , .
- Emergency department crowding, Part 1: concept, causes, and moral consequences.Ann Emerg Med.2009;53(5):605–611. , , , , .
- Emergency department overcrowding and ambulance diversion: the impact and potential solutions of extended boarding of admitted patients in the emergency department.J Emerg Med.2006;30(3):351–356. , .
- A comparison of in‐hospital mortality risk conferred by high hospital occupancy, differences in nurse staffing levels, weekend admission, and seasonal influenza.Medical Care.2010;48(3):224–232. , , , .
- The effect of hospital bed occupancy on throughput in the pediatric emergency department.Ann Emerg Med.2009;53(6):767–776.e763. . , , .
- Interpreting the Volume‐Outcome Relationship in the Context of Health Care Quality: Workshop Summary.Washington, DC:National Academies Press;2000. .
- Uncertain demand, the structure of hospital costs, and the cost of empty hospital beds.J Health Econ.1995;14(3):291–317. , .
- Variability in surgical caseload and access to intensive care services.Anesthesiology.2003;98(6):1491–1496. , , , et al.
- Effects of hospital care environment on patient mortality and nurse outcomes.J Nurs Adm.2009;39(7/8):S45–S51. , , , , .
- Children's hospitals do not acutely respond to high occupancy.Pediatrics.2010;125:974–981. , , , et al.
- Easing the strain on a pediatric tertiary care center: use of a redistribution system.Arch Pediatr Adolesc Med.2007;161(9):870–876. , .
- Lengths of stay and costs associated with Children's Hospitals.Pediatrics.2005;115:839–844. , , .
- Matching Supply with Demand: An introduction to operations management.New York:McGraw‐Hill;2006. , .
- Do hospitals provide lower quality care on weekends?Health Serv Res.2007;42:1589–1612. .
- Waiting for urgent procedures on the weekend among emergently hospitalized patients.Am J Med.2004;117:175–181. , .
- Mortality among patients admitted to hospitals on weekends as compared with weekdays.N Engl J Med.2001;345:663–668. , .
- Enhanced weekend service: an affordable means to increased hospital procedure volume.CMAJ.2005;172(4):503–504. , .
- Hospital deaths and weekend admissions‐how do we leap across a chasm?Clin Nurse Spec.2002;16:91–92. .
- Weekend birth and higher neonatal mortality: a problem of patient acuity or quality of care?J Obstet Gynecol Neonatal Nurs.2003;32(6):724–733. , .
- Weekend versus weekday admission and mortality from myocardial infarction.N Engl J Med.2007;356:1099–1109. , , , et al.
- Weekend admission and treatment of patients with renal colic: a case of avoidable variation?Urology.2009;73(4):720–724. .
- Characteristics of weekday and weekend hospital admissions.HCUP Statistical Brief.2010;87. http://www.hcup‐us.ahrq.gov/reports/statbriefs/sb87.pdf. , , .
- Hospital Costs and the Cost of Empty Hospital Beds (NBER Working Paper No. W3872).1991. , .
- The effects of timing of pediatric knee ligament surgery on short‐term academic performance in school‐aged athletes.Am J Sports Med.2009;37(9):1684–1691. , , .
- Institute for Healthcare Improvement, Smoothing Elective Surgical Admissions. Available at: http://www.ihi.org/IHI/Topics/Flow/PatientFlow/EmergingContent/SmoothingElectiveSurgicalAdmissions.htm. Accessed June2010.
- http://www.ihi.org/IHI/Topics/Flow. Accessed June2010. . Institute for Healthcare Improvement, Patient Flow Comments. Available at:
- OPIM 631: Operations Management.Wharton School, University of Pennsylvania.Philadelphia, PA.2008. .
- Agency for Healthcare Research and Quality. HCUP Databases, Healthcare Cost and Utilization Project (HCUP). 2008. Available at: www.hcup‐us.ahrq.gov/kidoverview.jsp. Accessed June2010.
- Relationship between resident workload and self‐perceived learning on inpatient medicine wards: a longitudinal study.BMC Med Educ.2006;6(1):35. , , , , , .
- AHA Solutions, Patient Flow Challenges Assessment 2009. Chicago, IL.2009.
- Patient flow in hospitals: understanding and controlling it better.Front Health Serv Manage.2004;20:3–15. , .
- Managing Variability in Patient Flow is the Key to Improving Access to Care, Nursing Staffing, Quality of Care, and Reducing Its Cost. Paper presented at: Institute of Medicine; June 24,2004. .
- Managing unnecessary variability in patient demand to reduce nursing stress and improve patient safety.Jt Comm J Qual Patient Saf.2005;31(6):330–338. , , , , , .
- Developing models for patient flow and daily surge capacity research.Acad Emerg Med.2006;13(11):1109–1113. , , .
- Institute for Healthcare Improvement, Flow initiatives. 2008. Available at: http://www.ihi.org/IHI/Topics/Flow. Accessed June2010.
- Hospital workload and adverse events.Med Care.2007;45(5):448–455. , , , et al.
- Impact of admission‐day crowding on the length of stay of pediatric hospitalizations.Pediatrics.2008;121(4):e718–730. , , , , .
- Emergency department crowding, Part 1: concept, causes, and moral consequences.Ann Emerg Med.2009;53(5):605–611. , , , , .
- Emergency department overcrowding and ambulance diversion: the impact and potential solutions of extended boarding of admitted patients in the emergency department.J Emerg Med.2006;30(3):351–356. , .
- A comparison of in‐hospital mortality risk conferred by high hospital occupancy, differences in nurse staffing levels, weekend admission, and seasonal influenza.Medical Care.2010;48(3):224–232. , , , .
- The effect of hospital bed occupancy on throughput in the pediatric emergency department.Ann Emerg Med.2009;53(6):767–776.e763. . , , .
- Interpreting the Volume‐Outcome Relationship in the Context of Health Care Quality: Workshop Summary.Washington, DC:National Academies Press;2000. .
- Uncertain demand, the structure of hospital costs, and the cost of empty hospital beds.J Health Econ.1995;14(3):291–317. , .
- Variability in surgical caseload and access to intensive care services.Anesthesiology.2003;98(6):1491–1496. , , , et al.
- Effects of hospital care environment on patient mortality and nurse outcomes.J Nurs Adm.2009;39(7/8):S45–S51. , , , , .
- Children's hospitals do not acutely respond to high occupancy.Pediatrics.2010;125:974–981. , , , et al.
- Easing the strain on a pediatric tertiary care center: use of a redistribution system.Arch Pediatr Adolesc Med.2007;161(9):870–876. , .
- Lengths of stay and costs associated with Children's Hospitals.Pediatrics.2005;115:839–844. , , .
- Matching Supply with Demand: An introduction to operations management.New York:McGraw‐Hill;2006. , .
- Do hospitals provide lower quality care on weekends?Health Serv Res.2007;42:1589–1612. .
- Waiting for urgent procedures on the weekend among emergently hospitalized patients.Am J Med.2004;117:175–181. , .
- Mortality among patients admitted to hospitals on weekends as compared with weekdays.N Engl J Med.2001;345:663–668. , .
- Enhanced weekend service: an affordable means to increased hospital procedure volume.CMAJ.2005;172(4):503–504. , .
- Hospital deaths and weekend admissions‐how do we leap across a chasm?Clin Nurse Spec.2002;16:91–92. .
- Weekend birth and higher neonatal mortality: a problem of patient acuity or quality of care?J Obstet Gynecol Neonatal Nurs.2003;32(6):724–733. , .
- Weekend versus weekday admission and mortality from myocardial infarction.N Engl J Med.2007;356:1099–1109. , , , et al.
- Weekend admission and treatment of patients with renal colic: a case of avoidable variation?Urology.2009;73(4):720–724. .
- Characteristics of weekday and weekend hospital admissions.HCUP Statistical Brief.2010;87. http://www.hcup‐us.ahrq.gov/reports/statbriefs/sb87.pdf. , , .
- Hospital Costs and the Cost of Empty Hospital Beds (NBER Working Paper No. W3872).1991. , .
- The effects of timing of pediatric knee ligament surgery on short‐term academic performance in school‐aged athletes.Am J Sports Med.2009;37(9):1684–1691. , , .
- Institute for Healthcare Improvement, Smoothing Elective Surgical Admissions. Available at: http://www.ihi.org/IHI/Topics/Flow/PatientFlow/EmergingContent/SmoothingElectiveSurgicalAdmissions.htm. Accessed June2010.
- http://www.ihi.org/IHI/Topics/Flow. Accessed June2010. . Institute for Healthcare Improvement, Patient Flow Comments. Available at:
- OPIM 631: Operations Management.Wharton School, University of Pennsylvania.Philadelphia, PA.2008. .
- Agency for Healthcare Research and Quality. HCUP Databases, Healthcare Cost and Utilization Project (HCUP). 2008. Available at: www.hcup‐us.ahrq.gov/kidoverview.jsp. Accessed June2010.
Copyright © 2010 Society of Hospital Medicine