User login
Prevention of Inpatient Hypoglycemia
Insulin therapy in the hospital setting can cause hypoglycemia, which may lead to increased mortality and length of stay (LOS).[1, 2, 3] Hypoglycemia is associated with cardiovascular, cerebrovascular, and patient fall events.[4, 5] The Centers for Medicare and Medicaid Services have designated both severe hypoglycemia (SH) with harm and diabetic ketoacidosis as hospital acquired conditions (HAC) or never events. The Society for Hospital Medicine (SHM) defines SH in the hospital as a blood glucose (BG) <40 mg/dL. Minimizing episodes of SH is important for patient health outcomes, patient safety, and for healthcare facilities' safety metrics.
Many factors contribute to SH including excessive insulin doses, medication errors, inappropriate timing of insulin doses with food intake, changes in nutritional status, impaired renal function, and changes in medications such as steroids.[6] As part of a multiyear project in patient safety, an inpatient hypoglycemia alert algorithm was developed based on a multivariate analysis of individual patient demographic, pharmacy, laboratory, and glucometric data. The algorithm was previously shown to have a 75% sensitivity to predict episodes of SH.[7] In this study, we tested whether a predictive real‐time informatics hypoglycemia alert based on the tested algorithm, along with trained nurses, would result in a decreased frequency of SH events compared to usual care. We hypothesized that this alert would result in a reduction of SH events in those patients at high risk for hypoglycemia.
METHODS
Study Design and Population
This prospective cohort‐intervention study involved inpatients admitted to Barnes‐Jewish Hospital in St. Louis, Missouri, the academic hospital of Washington University School of Medicine (WUSM), from August 2011 through December 2011. Fourteen floors, including 10 internal medicine and 4 cardiology medicine floors, were selected based upon a high frequency of severe hypoglycemic events noted in 2010. Six of the internal medicine floors were designated as intervention floors, and 8 were designated as control floors, including the 4 cardiology units. The study population consisted of patients receiving diabetic medications on study floors who had a BG <90 mg/dL during their hospital stay (Figure 1). The study was approved by the WUSM institutional review board and included a waiver of consent for individual patients.
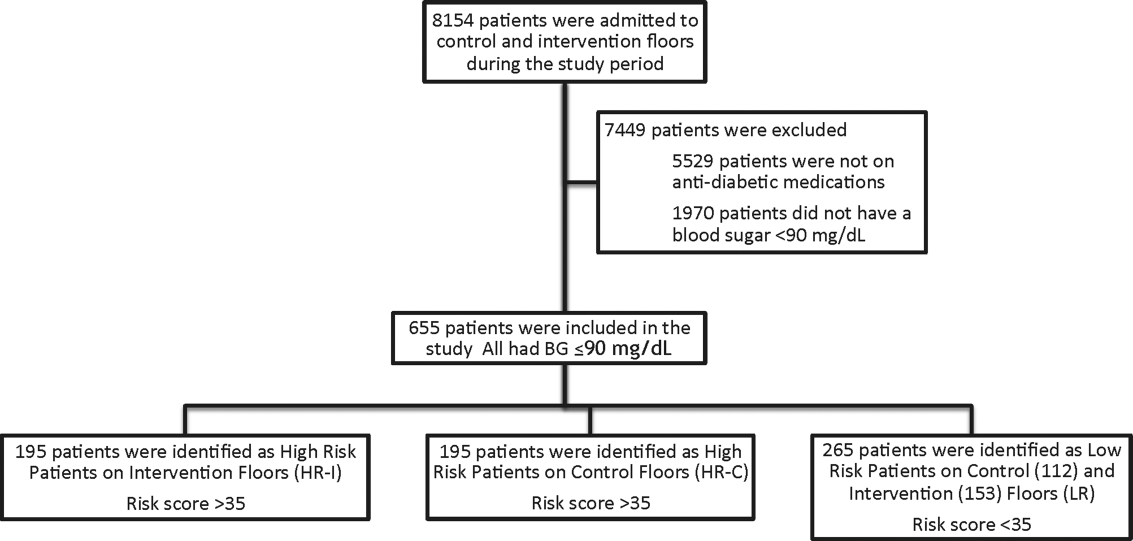
The pharmacy informatics system was programmed with the previously developed hypoglycemia alert to prospectively identify those patients at high risk of hypoglycemia based on real‐time patient information.[7] Patients were identified as high risk on study floors if insulin or an oral antihyperglycemic agent was prescribed and if their hypoglycemia informatics generated risk score was >35 within 24 hours of having a capillary or venous BG<90 mg/dL. The risk score of 35 corresponded to a 50% sensitivity for a subsequent BG <60 mg/dL and a 75% sensitivity for a BG <40 mg/dL. Patients who generated an alert once during their hospital stay were assigned to 1 of 3 categories based on their admission division and risk score: high‐risk intervention (HR‐I), high‐risk control (HR‐C), or low risk (LR). LR patients also had a BG <90 mg/dL during their stay, but a risk score of <35.
The electronic alert for HR‐I patients was sent by pager to division‐specific charge nurses. Fourteen charge nurses on intervention divisions were trained to assess the alert, interview the patient, identify an alternate dosing strategy, and collaborate with the patient's physicians. HR‐C patients were identified on control divisions based on the same criteria as intervention patients, but no alert was generated. Control patients' charts were reviewed and evaluated upon discharge by the research team‐certified diabetes nurse educator to determine whether the treating physician had identified the SH risk and had changed insulin orders.
Nurses and physicians caring for patients on study divisions provided informed consent to participate in the study. Nurses' satisfaction with the alert process and physician interaction was assessed with a collaboration scale that was completed after each alert (see Supporting Information, Appendix A, in the online version of this article).[8]
Alert Development Process
The alert equation algorithm was developed at Barnes‐Jewish Hospital after a retrospective analysis of hospital glucometric data, including capillary and venous BG measurements, and demographic and pharmacy data over a 6‐month time period.[9] The analysis identified factors that were independently associated with hypoglycemia and used these variables in a mathematical model to achieve a 50% sensitivity to predict a subsequent BG of <60 mg/dL and a 75% sensitivity to predict SH.[7] Table 1 outlines the variables in the model and provides the risk‐score equation used to generate an alert.
Variable | Description of Variable |
---|---|
Body weight | Patients at a lower weight were at an increased risk. The variable had a linear response, and 3 levels were used to modify the risk equation: <69 kg, 7079 kg, and >80 kg. |
Creatinine clearance | Patients with a lower creatinine clearance were at an increased risk. This variable had a linear response, and 2 levels were used to modify the risk equation: <48 mL/min or >48 mL/min. |
Basal insulin dose | Increased risk was noted at a doses of basal insulin >0.25 U/kg. |
Basal‐only dosing | Dosing of basal insulin without meal‐time insulin conferred increased risk. |
Nonstandard insulin therapy | The use of 70/30 insulin was associated with increased risk. |
Oral diabetic therapy | Use of sulfonylureas was associated with increased risk. |
Risk score equation | (Value <60)=0.055+1.062 * (Basal <0.25 U/kg)+1.234 * (Basal 0.25 U/kg) & minus;0.294 * (Weight <6069 kg)0.540 * (Weight 7079 kg)0.786 * (Weight 80 kg) & minus;0.389 * (Creatinine Clearance <3847) 0.680 * (Creatinine Clearance 48) 0.239 * (Sliding Yes) 0.556*(Meal Yes)+0.951 * (Sliding and Meal)+0.336 * (Sulfonylurea Yes) Score=100 * (Exp (Value <60)/(1+Exp (Value <60)) |
The alert used a BG cutoff of 90 mg/dL in accordance with the American College of Endocrinology Hospital Guideline. Although current guidelines from the SHM recommend keeping BG values >100 mg/dL for patient safety, our analysis found that the cutoff of 90 mg/dL had better sensitivity and specificity than the <100 mg/dL guideline for the risk algorithm.[10, 11]
Nurse and Physician Training
Charge nurses received 5 hours of hyperglycemia management training in 3 sessions utilizing a structured curriculum. Session 1 included a pretest followed by diabetes management education. Session 2 was devoted to an interactive workshop utilizing case‐based scenarios of diabetes management problems and hypoglycemia prevention. The final session provided instructions on the electronic alert communication process. Nurses were empowered with tools for effective communication practices using the situation‐background‐assessment‐recommendation (SBAR) technique.[12]
Physicians, including hospitalists and medicine residents on intervention and control floors, took a pretest, received a 1‐hour lecture, and completed the same curriculum of case‐based scenarios in an online self‐directed learning module. Physicians did not receive SBAR training. Both nurses and physicians received pocket cards with insulin management guidelines developed by our research team to ensure that all clinicians had common prescribing practices.[13]
Outcomes
The primary outcome was the incidence of SH occurring in HR‐I versus HR‐C patients. Secondary outcomes included: episodes of SH in LR study patients, incidence of BG<60 mg/dL frequency of transfer to a higher level of care, incidence of severe hyperglycemia defined as BG >299 mg/dL, frequency that high‐risk patient's orders were changed to reduce hypoglycemia risk in response to the alert‐intervention process, LOS, mortality, and a nurse‐physician collaboration scale score.[14]
Statistical Analysis
Demographic and clinical metrics were compared between HR‐I and HR‐C patients to evaluate potential sources of bias. These included age, weight, serum creatinine, creatinine clearance (measured by Cockcroft‐Gault), hemoglobin A1c (HbA1c) if available, LOS, gender, admitting diagnosis, type of diabetes, and Charlson Comorbidity Index score. The alert risk‐score was also compared between intervention and control floors. Two‐tailed t tests assessed differences between the study groups on normally distributed variables, whereas Wilcoxon rank sum tests were used for non‐normally distributed variables, and 2 tests were used for categorical variables. Two‐tailed Fisher exact tests compared the prevalence of hypoglycemia thresholds between the study groups. 2 analysis was used to compare the proportion of patients who experienced a BG >299 mg/dL between intervention and controls and the proportion of orders changed in HR‐I versus HR‐C patients. Logistic regression was used to test the association of nurse collaboration score with the likelihood of orders being changed.
Based on previous research, we estimated a 48% rate of hypoglycemia <60 mg/dL in HR‐C patients on control floors.[7] We calculated a sample size of 195 subjects in each high‐risk group as the number needed for the intervention to produce a clinically meaningful reduction in hypoglycemia of 25% on the intervention floor compared to the control floors with 90% power.
RESULTS
Study Cohort and Patient Characteristics
One hundred ninety‐five patients who met criteria for high‐risk status were enrolled on HR‐I floors and HR‐C floors for a total of 390 high‐risk patients. During the same time period, 265 LR patients were identified on intervention (153 patients) and control (112 patients) floors. The HR‐I patients were similar to the HR‐C patients by baseline demographics, as shown in Table 2. HbA1c was not available on all patients, but the mean HbA1c in the HR‐I group was 7.93% versus 7.40% in the HR‐C group (P=0.048). The Charlson Comorbidity Index score was significantly different between the high‐risk groups (HR‐I: 6.48 vs HR‐C: 7.48, P=0.002), indicating that the HR‐C patients had more comorbidities.[15] There were significant differences in 2 of the 3 most common admitting diagnoses between groups, with more HR‐C patients admitted for circulatory system diseases (HR‐C: 22.3% vs HR‐I: 4.4%, P=0.001), and more HR‐I patients admitted for digestive system diseases (HR‐I: 13.7% vs HR‐C: 3.3%, P<0.001). The proportion of patients with preexisting type 2 diabetes did not differ by intervention status (HR‐I: 89.8% vs HR‐C: 92.0%, P=0.462).
Demographic | HR‐I, Mean SD/Frequency (%), N=195 | HR‐C, Mean, SD/Frequency (%), N=195 | Low Risk, Mean, SD/Frequency (%), N=265 | P Value* |
---|---|---|---|---|
| ||||
Age, y | 60.2 (15.1) | 60.3 (16.9) | 61.0 (13.8) | 0.940 |
Weight, kg | 84.9 (31.9) | 80.8 (26.6) | 93.6 (28.7) | 0.173 |
Serum creatinine, mg/dL | 2.06 (2.56) | 2.03 (1.87) | 1.89 (2.17) | 0.910 |
Creatinine clearance, mL/min | 50.6 (29.8) | 45.4 (27.1) | 55.5 (29.3) | 0.077 |
Hemoglobin A1c, n (%) with data | 7.93 (2.46), n=130 (67%) | 7.40 (1.75), n=115 (59%) | 6.65 (2.05), n=152 (57%) | 0.048 |
Risk score | 52 (11) | 54 (11) | 26 (6) | 0.111 |
Length of stay, median, d | 5.83 | 5.88 | 5.79 | 0.664 |
Male gender | 84 (43.1%) | 98 (50.3%) | 145 (54.7%) | 0.155 |
Type 2 diabetes | 167 (89.8%) | 172 (92.0%) | 219 (95.6%) | 0.462 |
Charlson Comorbidity Index score | 6.48 (3.06) | 7.48 (3.28) | 6.66 (3.24) | 0.002 |
Admit diagnosis endocrine, nutritional, metabolic diseases, and immunity disorders (codes 240279) | 17 (9.3%) | 10 (5.4%) | 11 (4.3%) | 0.153 |
Admit diagnosis disease of circulatory system (codes 390459) | 8 (4.4%) | 41 (22.3%) | 26 (10.1%) | <0.001 |
Admit diagnosis disease of digestive system (codes 520579) | 25 (13.7%) | 6 (3.3%) | 27 (10.5%) | <0.001 |
Admit diagnosis diseases of the genitourinary system (codes 580629) | 6 (3.3%) | 4 (2.2%) | 15 (5.8%) | 0.510 |
Admit diagnosis reported only as signs, symptoms, or ill‐defined conditions (codes 780799) | 77 (42.3%) | 92 (50.0%) | 121 (46.9%) | 0.140 |
Study Outcomes
The rate of hypoglycemia was compared between 195 HR‐I and 195 HR‐C patients, and it should be noted that each patient could generate only 1 episode of hypoglycemia during an admission. As shown in Table 3, the incidence of a BG <60 mg/dL was significantly lower in the HR‐I patients versus the HR‐C patients (13.3% vs 26.7%, P=0.002) as was the incidence of a BG <40 mg/dL (3.1% HR‐I vs 9.7% HR‐C, P=0.012). This represents a decrease of 50% in moderate hypoglycemia (BG <60 mg/dL) and a decrease of 68% in SH (BG <40 mg/dL) between HR‐I and HR‐C patients. Severe hyperglycemia occurrences were not significantly different between intervention and control floors at 28% each.
Alerted Patients Glucose Threshold | HR‐I (%), N=195 | HR‐C (%), N=195 | Low Risk (%), N=265 | P Value* |
---|---|---|---|---|
| ||||
With BG <40 mg/dL | 6 (3.1%) | 19 (9.7%) | 10 (3.8%) | 0.012 |
With BG <60 mg/dL | 26 (13.3%) | 51 (26.7%) | 50 (18.9%) | 0.002 |
With BG >299 mg/dL | 53 (28.0%) | 53 (27.9%) | 29 (11.9%) | 0.974 |
The sensitivity, specificity, and predictive values of the alert for BG thresholds of <40 mg/dL and <60 mg/dL are presented in Table 4. On control floors, the alert exhibited a modest sensitivity and high negative predictive value for BG <40 mg/dL. Sensitivity for a BG <40 mg/dL was 76% and 51.5% for BG <60 mg/dL. The alert was developed with a 50% sensitivity for a BG of <60 mg/dL, and the sensitivities calculated on control floors were consistent with the original modeling. The predictive value of an LR classification was 98.2% for not having a BG <40 mg/dL. The predictive value of a positive alert was 9.7% for BG <40 mg/dL.
Variable | 40 mg/dL Threshold | 60 mg/dL Threshold |
---|---|---|
| ||
Sensitivity: probability of an alert given BG <40 or 60 mg/dL | 76.0% | 51.5% |
Specificity: probability of no alert given BG >40 or 60 mg/dL | 64.6% | 66.0% |
Positive predictive value | 9.7% | 26.7% |
Negative predictive value (nonalerted patients identified as low risk) | 98.2% | 85.0% |
There was no significant difference in mortality (P=0.726), transfer to a higher level of care (P=0.296), or LOS between the 2 groups (HR‐I: 5.83 days vs HR‐C: 5.88 days, P=0.664). However, patients with a BG <40 mg/dL had an LOS of 12.2 days (N=45) versus 8.1 days for those without an SH event (N=610), which was statistically significant (P=0.005). There was no increase in the incidence of BG >299 mg/dL in the HR‐I versus HR‐C groups (P=0.53).
Nurse‐physician satisfaction with the alert process was evaluated using a collaboration scale completed after each alert.[8] Of the 195 hypoglycemia alerts, there were 167 (85.6%) nurse and 25 (12.8%) physician collaboration scales completed. Scores were similar among nurses (average 1.52) and physicians (average 1.72), reflecting positive experiences with collaboration. Orders were changed in 40.7% HR‐I patients in response to the collaboration, but in only 20.5% of HR‐C patients after the initial BG of <90 mg/dL occurred. A change in orders constituted a modification consistent with lowering the risk of hypoglycemia and included discontinuing an oral antidiabetic agent, lowering the dose of insulin, and rarely the addition of dextrose‐containing fluids. The most common change in orders was a reduction in the total dose of insulin. A difference in orders changed was partially explained by the collaboration score; a 1‐unit increase in the score correlated to an odds ratio of 2.10 that the orders would be changed (P=0.002).
DISCUSSION
Hospitals are accountable for safe and effective care of patients with hyperglycemia, which includes prevention of medication‐induced hypoglycemia. We have developed a predictive informatics hypoglycemia risk alert that, when tested in a real‐world situation, significantly reduced the rate of SH in hospitalized patients without increasing severe hyperglycemia. The alert algorithm correctly identified patients who were at high risk for hypoglycemia and allowed caretakers the opportunity to lower that risk. The positive predictive value of the alert was low but acceptable at 9.7%, owing to the overall low rate of hypoglycemia in the patient population.
The alert model tested involved 3 components for success: the automated alert, trained charge nurse responders, and an interaction between the nurse responder and the care provider. HR‐I patients were interviewed and assessed for problems associated with oral intake, dietary habits, medication compliance, and hypoglycemia at home prior to communicating with physicians. The extensive training and proficiency in patient assessment and SBAR communication process required by nurses was paramount in the success of the alert. However, the alert provided a definitive risk assessment that was actionable, versus more global instruction, which has not had the same impact in risk reduction. Based on feedback collected from nurses at the study end, they felt the alert process was within their scope of practice and was not unduly burdensome. They also found that the training in diabetes management and SBAR communication techniques, in addition to the alert system, were useful in protecting patients from medication harm.
Physicians for HR‐C patients missed many opportunities to effectively intervene and thereby reduce the likelihood of an SH event. Our assumption is that the clinicians did not ascertain the risk of SH, which was reflected by the fact that orders were changed in 40.7% of HR‐I patients versus only 20.5% in the HR‐C group. Having alerts go directly to nurses rather than physicians permitted inclusion of additional information, such as caloric intake and testing schedules, so that changes in orders would have greater context, and the importance of mild hypoglycemia would not be overlooked.[16] Glycemic control is challenging for providers in the inpatient setting, as there is little time to test and titrate doses of insulin to achieve control. Tight glycemic control has become the primary focus of diabetes management in the outpatient setting to reduce long‐term risks of microvascular complications.[17, 18] However, establishing glycemic targets in the inpatient setting has been difficult because the risk for hypoglycemia increases with tighter control.[19, 20] Inpatient hypoglycemia has been associated with increased mortality, particularly in critically ill patients.[21, 22] Many factors contribute to hypoglycemia including low creatinine clearance, low body weight, untested insulin doses, errors in insulin administration, unexpected dietary changes, changes in medications affecting BG levels, poor communication during times of patient transfer to different care teams, and poor coordination of BG testing with insulin administration at meal times. A multifaceted approach aimed at improving both clinician and nurse awareness, and providing real‐time risk assessment is clearly required to insure patient safety.[6, 13, 23, 24]
There are significant economic benefits to avoiding SH in the hospital given the adverse outcomes associated with HACs and the extra cost associated with these conditions. In hospitalized patients, hypoglycemia worsens outcomes leading to higher costs due to longer LOS (by 3 days), higher inpatient charges (38.9%), and higher risk of discharge to a skilled nursing facility.[1, 3, 25, 26] Conversely, improved glycemic control can reduce surgical site infections, perioperative morbidity, and hospital LOS.[27] The high prevalence of insulin use among inpatients, many of whom have high‐risk characteristics, creates a milieu for both hyper‐ and hypoglycemia. Other groups have described a drop in hypoglycemia rates related to the use of standardized diabetes order sets and nurse and physician education, but this is the first study that used informatics in a prospective manner to identify patients who are at high risk for developing hypoglycemia and then specifically targeted those patients.[28] The alert process was modeled after a similar alert developed in our institution for identifying medicine patients at risk for sepsis.[29] Given the paucity of data related to inpatient glycemia risk reduction, this study is particularly relevant for improving patient safety.
The major limitation of this study is that it was not randomized at the patient level. Patients were assigned to intervention and control groups based on their occupancy on specific hospital floors to avoid treatment bias. Bias was assessed due to this nonrandom assignment by comparing demographic and clinical factors of HR patients between intervention and control floors, and found significant differences in HbA1c and admitting diagnosis. As the control group had lower HbA1c values than the intervention group, and it is known from the Diabetes Control and Complications Trial and Action to Control Cardiovascular Risk in Diabetes trial that lower HbA1c increases the risk of hypoglycemia, our results may be biased by the level of glucose control on admission.[30, 31] Admitting diagnoses differed significantly between intervention and control patients as did the Charlson Comorbidity Index score; however, the hypoglycemia alert system does not include patient diagnoses or comorbidities, and as such provided equipoise with regard to risk reduction regardless of presenting illness. This study included trained nurses, which may be beyond the scope of every institution and thereby limit the effectiveness of the alert in reducing risk. However, as a result of this study, the alert was expanded to other acute care floors at our hospital as well as other hospitals in the Barnes‐Jewish Hospital system.
In summary, this study showed a 68% decrease in episodes of SH in a high‐risk patient cohort on diabetic medications using a hypoglycemia alert system. The results of this study demonstrate the validity of a systems‐based approach to reduce SH in high‐risk inpatients.
Disclosures
This work was funded by the Barnes‐Jewish Hospital Foundation The authors report no conflicts of interest.
- Hypoglycaemia is associated with increased length of stay and mortality in people with diabetes who are hospitalized. Diabet Med. 2012;29:e445–e448. , , , , , .
- Hypoglycemia as a predictor of mortality in hospitalized elderly patients. Arch Intern Med. 2003;163:1825–1829. , , , et al.
- Hypoglycemia and clinical outcomes in patients with diabetes hospitalized in the general ward. Diabetes Care. 2009;32:1153–1157. , , , , , .
- Association of hypoglycemia and cardiac ischemia: a study based on continuous glucose monitoring. Diabetes Care. 2003;26:1485–1489. , , , , .
- Diabetes‐related complications, glycemic control, and falls in older adults. Diabetes Care. 2008;31:391–396. , , , et al.
- Inpatient insulin orders: are patients getting what is prescribed? J Hosp Med. 2011;9:526–529. , , , , .
- Prediction and prevention of treatment‐related inpatient hypoglycemia. J Diabetes Sci Technol. 2012;6:302–309. , , , .
- Collaboration between nurses and physicians. Image J Nurs Sch. 1988;20:145–149. , .
- Glucometrics—assessing the quality of inpatient glucose management. Diabetes Technol Ther. 2005;8:560–569. , , , et al.
- ACE/ADA Task Force on Inpatient Diabetes. American College of Endocrinology and American Diabetes Association consensus statement on inpatient diabetes and glycemic control. Diabetes Care. 2006;29:1955–1962.
- American Association of Clinical Endocrinologists and American Diabetes Association consensus statement on inpatient glycemic control. Endocr Pract. 2009;15:353–369. , , , et al.
- Role‐play using SBAR technique to improve observed communication skills in senior nursing students. J Nurs Educ. 2011;50:79–87. .
- SHM Glycemic Control Task Force. Workbook for improvement: improving glycemic control, preventing hypoglycemia, and optimizing care of the inpatient with hyperglycemia and diabetes. Society of Hospital Medicine website, Glycemic Control Quality Improvement Resource Room. Available at: http://www.hospitalmedicine.org. Accessed on February 12, 2011.
- An internet service supporting quality assessment of inpatient glycemic control. J Diabetes Sci and Technol. 2008;2:402–408. , .
- Updating and validating the Charlson Comorbidity Index and score for risk adjustment in hospital discharge abstracts using data from 6 countries. Am J Epidemiol. 2011;173:676–682. , , , et al.
- Glycemic management in medical and surgical patients in the non‐ICU setting. Curr Diab Rep. 2013;13:96–106. , , .
- The Diabetes Control and Complications Trial Research Group. The effect of intensive treatment of diabetes on the development and progression of long‐term complications in insulin‐dependent diabetes mellitus. N Engl J Med. 1993;329:977–986.
- UK Prospective Diabetes Study Group. Intensive blood‐glucose control with sulphonylureas or insulin compared with conventional treatment and risk of complications in patients with type 2 diabetes. Lancet. 1998;352:837–853.
- Hypoglycemia in diabetes. Diabetes Care. 2003;26:1902–1912. , , .
- Hypoglycemia: still the limiting factor in the glycemic management of diabetes. Endocr Pract. 2008;14:750–756. .
- for the NICE‐SUGAR Study Investigators. Hypoglycemia and risk of death in critically ill patients. N Engl J Med. 2012;367:1108–1118. , , , et al.;
- Increased mortality of patients with diabetes reporting severe hypoglycemia. Diabetes Care. 2012;35:1897–1901. , , , , , .
- Risk factors for inpatient hypoglycemia during subcutaneous insulin therapy in non‐critically ill patients with type 2 diabetes. J Diabetes Sci Technol. 2012;6:1022–1029. , , , et al.
- Minimizing hypoglycemia in the wake of a tight glycemic control protocol in hospitalized patients. J Nurs Care Qual. 2010;25:255–260. , , .
- The economic and quality of life impact of hypoglycemia. Eur J Health Econ. 2005;6:197–202. , , , .
- Economic and clinical impact of inpatient diabetic hypoglycemia. Endocr Pract. 2009;15:302–312. , , , , , .
- Mild hypoglycemia is strongly associated with increased intensive care unit length of stay. Ann Intensive Care. 2011;49:1–49. , , , et al.
- Implementing and evaluating a multicomponent inpatient diabetes management program: putting research into practice. Jt Comm J Qual Patient Saf. 2012;38:195–206. , , , et al.
- Implementation of a real‐time computerized sepsis alert in nonintensive care unit patients. Crit Care Med. 2011;39:469–473. , , , et al.
- The Diabetes Control and Complications Trial Research Group. Epidemiology of severe hypoglycemia in the Diabetes Control and Complications Trial. Am J Med. 1991;90:450–459.
- The effects of baseline characteristics, glycemia treatment approach, and glycated hemoglobin concentration on the risk of severe hypoglycaemia: post hoc epidemiological analysis of the ACCORD study. BMJ. 2010;340:1–12. , , , et al.
Insulin therapy in the hospital setting can cause hypoglycemia, which may lead to increased mortality and length of stay (LOS).[1, 2, 3] Hypoglycemia is associated with cardiovascular, cerebrovascular, and patient fall events.[4, 5] The Centers for Medicare and Medicaid Services have designated both severe hypoglycemia (SH) with harm and diabetic ketoacidosis as hospital acquired conditions (HAC) or never events. The Society for Hospital Medicine (SHM) defines SH in the hospital as a blood glucose (BG) <40 mg/dL. Minimizing episodes of SH is important for patient health outcomes, patient safety, and for healthcare facilities' safety metrics.
Many factors contribute to SH including excessive insulin doses, medication errors, inappropriate timing of insulin doses with food intake, changes in nutritional status, impaired renal function, and changes in medications such as steroids.[6] As part of a multiyear project in patient safety, an inpatient hypoglycemia alert algorithm was developed based on a multivariate analysis of individual patient demographic, pharmacy, laboratory, and glucometric data. The algorithm was previously shown to have a 75% sensitivity to predict episodes of SH.[7] In this study, we tested whether a predictive real‐time informatics hypoglycemia alert based on the tested algorithm, along with trained nurses, would result in a decreased frequency of SH events compared to usual care. We hypothesized that this alert would result in a reduction of SH events in those patients at high risk for hypoglycemia.
METHODS
Study Design and Population
This prospective cohort‐intervention study involved inpatients admitted to Barnes‐Jewish Hospital in St. Louis, Missouri, the academic hospital of Washington University School of Medicine (WUSM), from August 2011 through December 2011. Fourteen floors, including 10 internal medicine and 4 cardiology medicine floors, were selected based upon a high frequency of severe hypoglycemic events noted in 2010. Six of the internal medicine floors were designated as intervention floors, and 8 were designated as control floors, including the 4 cardiology units. The study population consisted of patients receiving diabetic medications on study floors who had a BG <90 mg/dL during their hospital stay (Figure 1). The study was approved by the WUSM institutional review board and included a waiver of consent for individual patients.
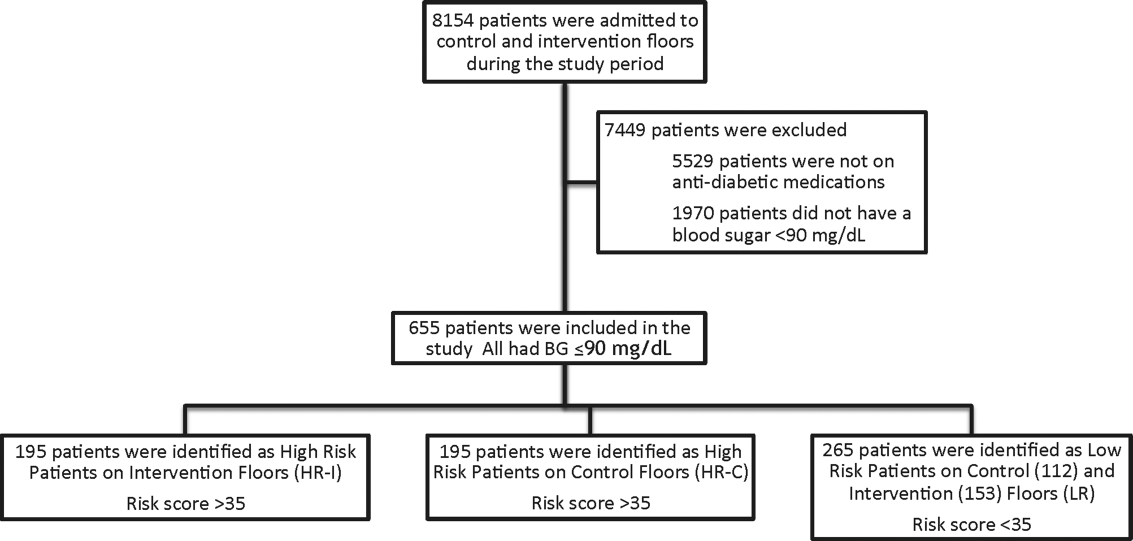
The pharmacy informatics system was programmed with the previously developed hypoglycemia alert to prospectively identify those patients at high risk of hypoglycemia based on real‐time patient information.[7] Patients were identified as high risk on study floors if insulin or an oral antihyperglycemic agent was prescribed and if their hypoglycemia informatics generated risk score was >35 within 24 hours of having a capillary or venous BG<90 mg/dL. The risk score of 35 corresponded to a 50% sensitivity for a subsequent BG <60 mg/dL and a 75% sensitivity for a BG <40 mg/dL. Patients who generated an alert once during their hospital stay were assigned to 1 of 3 categories based on their admission division and risk score: high‐risk intervention (HR‐I), high‐risk control (HR‐C), or low risk (LR). LR patients also had a BG <90 mg/dL during their stay, but a risk score of <35.
The electronic alert for HR‐I patients was sent by pager to division‐specific charge nurses. Fourteen charge nurses on intervention divisions were trained to assess the alert, interview the patient, identify an alternate dosing strategy, and collaborate with the patient's physicians. HR‐C patients were identified on control divisions based on the same criteria as intervention patients, but no alert was generated. Control patients' charts were reviewed and evaluated upon discharge by the research team‐certified diabetes nurse educator to determine whether the treating physician had identified the SH risk and had changed insulin orders.
Nurses and physicians caring for patients on study divisions provided informed consent to participate in the study. Nurses' satisfaction with the alert process and physician interaction was assessed with a collaboration scale that was completed after each alert (see Supporting Information, Appendix A, in the online version of this article).[8]
Alert Development Process
The alert equation algorithm was developed at Barnes‐Jewish Hospital after a retrospective analysis of hospital glucometric data, including capillary and venous BG measurements, and demographic and pharmacy data over a 6‐month time period.[9] The analysis identified factors that were independently associated with hypoglycemia and used these variables in a mathematical model to achieve a 50% sensitivity to predict a subsequent BG of <60 mg/dL and a 75% sensitivity to predict SH.[7] Table 1 outlines the variables in the model and provides the risk‐score equation used to generate an alert.
Variable | Description of Variable |
---|---|
Body weight | Patients at a lower weight were at an increased risk. The variable had a linear response, and 3 levels were used to modify the risk equation: <69 kg, 7079 kg, and >80 kg. |
Creatinine clearance | Patients with a lower creatinine clearance were at an increased risk. This variable had a linear response, and 2 levels were used to modify the risk equation: <48 mL/min or >48 mL/min. |
Basal insulin dose | Increased risk was noted at a doses of basal insulin >0.25 U/kg. |
Basal‐only dosing | Dosing of basal insulin without meal‐time insulin conferred increased risk. |
Nonstandard insulin therapy | The use of 70/30 insulin was associated with increased risk. |
Oral diabetic therapy | Use of sulfonylureas was associated with increased risk. |
Risk score equation | (Value <60)=0.055+1.062 * (Basal <0.25 U/kg)+1.234 * (Basal 0.25 U/kg) & minus;0.294 * (Weight <6069 kg)0.540 * (Weight 7079 kg)0.786 * (Weight 80 kg) & minus;0.389 * (Creatinine Clearance <3847) 0.680 * (Creatinine Clearance 48) 0.239 * (Sliding Yes) 0.556*(Meal Yes)+0.951 * (Sliding and Meal)+0.336 * (Sulfonylurea Yes) Score=100 * (Exp (Value <60)/(1+Exp (Value <60)) |
The alert used a BG cutoff of 90 mg/dL in accordance with the American College of Endocrinology Hospital Guideline. Although current guidelines from the SHM recommend keeping BG values >100 mg/dL for patient safety, our analysis found that the cutoff of 90 mg/dL had better sensitivity and specificity than the <100 mg/dL guideline for the risk algorithm.[10, 11]
Nurse and Physician Training
Charge nurses received 5 hours of hyperglycemia management training in 3 sessions utilizing a structured curriculum. Session 1 included a pretest followed by diabetes management education. Session 2 was devoted to an interactive workshop utilizing case‐based scenarios of diabetes management problems and hypoglycemia prevention. The final session provided instructions on the electronic alert communication process. Nurses were empowered with tools for effective communication practices using the situation‐background‐assessment‐recommendation (SBAR) technique.[12]
Physicians, including hospitalists and medicine residents on intervention and control floors, took a pretest, received a 1‐hour lecture, and completed the same curriculum of case‐based scenarios in an online self‐directed learning module. Physicians did not receive SBAR training. Both nurses and physicians received pocket cards with insulin management guidelines developed by our research team to ensure that all clinicians had common prescribing practices.[13]
Outcomes
The primary outcome was the incidence of SH occurring in HR‐I versus HR‐C patients. Secondary outcomes included: episodes of SH in LR study patients, incidence of BG<60 mg/dL frequency of transfer to a higher level of care, incidence of severe hyperglycemia defined as BG >299 mg/dL, frequency that high‐risk patient's orders were changed to reduce hypoglycemia risk in response to the alert‐intervention process, LOS, mortality, and a nurse‐physician collaboration scale score.[14]
Statistical Analysis
Demographic and clinical metrics were compared between HR‐I and HR‐C patients to evaluate potential sources of bias. These included age, weight, serum creatinine, creatinine clearance (measured by Cockcroft‐Gault), hemoglobin A1c (HbA1c) if available, LOS, gender, admitting diagnosis, type of diabetes, and Charlson Comorbidity Index score. The alert risk‐score was also compared between intervention and control floors. Two‐tailed t tests assessed differences between the study groups on normally distributed variables, whereas Wilcoxon rank sum tests were used for non‐normally distributed variables, and 2 tests were used for categorical variables. Two‐tailed Fisher exact tests compared the prevalence of hypoglycemia thresholds between the study groups. 2 analysis was used to compare the proportion of patients who experienced a BG >299 mg/dL between intervention and controls and the proportion of orders changed in HR‐I versus HR‐C patients. Logistic regression was used to test the association of nurse collaboration score with the likelihood of orders being changed.
Based on previous research, we estimated a 48% rate of hypoglycemia <60 mg/dL in HR‐C patients on control floors.[7] We calculated a sample size of 195 subjects in each high‐risk group as the number needed for the intervention to produce a clinically meaningful reduction in hypoglycemia of 25% on the intervention floor compared to the control floors with 90% power.
RESULTS
Study Cohort and Patient Characteristics
One hundred ninety‐five patients who met criteria for high‐risk status were enrolled on HR‐I floors and HR‐C floors for a total of 390 high‐risk patients. During the same time period, 265 LR patients were identified on intervention (153 patients) and control (112 patients) floors. The HR‐I patients were similar to the HR‐C patients by baseline demographics, as shown in Table 2. HbA1c was not available on all patients, but the mean HbA1c in the HR‐I group was 7.93% versus 7.40% in the HR‐C group (P=0.048). The Charlson Comorbidity Index score was significantly different between the high‐risk groups (HR‐I: 6.48 vs HR‐C: 7.48, P=0.002), indicating that the HR‐C patients had more comorbidities.[15] There were significant differences in 2 of the 3 most common admitting diagnoses between groups, with more HR‐C patients admitted for circulatory system diseases (HR‐C: 22.3% vs HR‐I: 4.4%, P=0.001), and more HR‐I patients admitted for digestive system diseases (HR‐I: 13.7% vs HR‐C: 3.3%, P<0.001). The proportion of patients with preexisting type 2 diabetes did not differ by intervention status (HR‐I: 89.8% vs HR‐C: 92.0%, P=0.462).
Demographic | HR‐I, Mean SD/Frequency (%), N=195 | HR‐C, Mean, SD/Frequency (%), N=195 | Low Risk, Mean, SD/Frequency (%), N=265 | P Value* |
---|---|---|---|---|
| ||||
Age, y | 60.2 (15.1) | 60.3 (16.9) | 61.0 (13.8) | 0.940 |
Weight, kg | 84.9 (31.9) | 80.8 (26.6) | 93.6 (28.7) | 0.173 |
Serum creatinine, mg/dL | 2.06 (2.56) | 2.03 (1.87) | 1.89 (2.17) | 0.910 |
Creatinine clearance, mL/min | 50.6 (29.8) | 45.4 (27.1) | 55.5 (29.3) | 0.077 |
Hemoglobin A1c, n (%) with data | 7.93 (2.46), n=130 (67%) | 7.40 (1.75), n=115 (59%) | 6.65 (2.05), n=152 (57%) | 0.048 |
Risk score | 52 (11) | 54 (11) | 26 (6) | 0.111 |
Length of stay, median, d | 5.83 | 5.88 | 5.79 | 0.664 |
Male gender | 84 (43.1%) | 98 (50.3%) | 145 (54.7%) | 0.155 |
Type 2 diabetes | 167 (89.8%) | 172 (92.0%) | 219 (95.6%) | 0.462 |
Charlson Comorbidity Index score | 6.48 (3.06) | 7.48 (3.28) | 6.66 (3.24) | 0.002 |
Admit diagnosis endocrine, nutritional, metabolic diseases, and immunity disorders (codes 240279) | 17 (9.3%) | 10 (5.4%) | 11 (4.3%) | 0.153 |
Admit diagnosis disease of circulatory system (codes 390459) | 8 (4.4%) | 41 (22.3%) | 26 (10.1%) | <0.001 |
Admit diagnosis disease of digestive system (codes 520579) | 25 (13.7%) | 6 (3.3%) | 27 (10.5%) | <0.001 |
Admit diagnosis diseases of the genitourinary system (codes 580629) | 6 (3.3%) | 4 (2.2%) | 15 (5.8%) | 0.510 |
Admit diagnosis reported only as signs, symptoms, or ill‐defined conditions (codes 780799) | 77 (42.3%) | 92 (50.0%) | 121 (46.9%) | 0.140 |
Study Outcomes
The rate of hypoglycemia was compared between 195 HR‐I and 195 HR‐C patients, and it should be noted that each patient could generate only 1 episode of hypoglycemia during an admission. As shown in Table 3, the incidence of a BG <60 mg/dL was significantly lower in the HR‐I patients versus the HR‐C patients (13.3% vs 26.7%, P=0.002) as was the incidence of a BG <40 mg/dL (3.1% HR‐I vs 9.7% HR‐C, P=0.012). This represents a decrease of 50% in moderate hypoglycemia (BG <60 mg/dL) and a decrease of 68% in SH (BG <40 mg/dL) between HR‐I and HR‐C patients. Severe hyperglycemia occurrences were not significantly different between intervention and control floors at 28% each.
Alerted Patients Glucose Threshold | HR‐I (%), N=195 | HR‐C (%), N=195 | Low Risk (%), N=265 | P Value* |
---|---|---|---|---|
| ||||
With BG <40 mg/dL | 6 (3.1%) | 19 (9.7%) | 10 (3.8%) | 0.012 |
With BG <60 mg/dL | 26 (13.3%) | 51 (26.7%) | 50 (18.9%) | 0.002 |
With BG >299 mg/dL | 53 (28.0%) | 53 (27.9%) | 29 (11.9%) | 0.974 |
The sensitivity, specificity, and predictive values of the alert for BG thresholds of <40 mg/dL and <60 mg/dL are presented in Table 4. On control floors, the alert exhibited a modest sensitivity and high negative predictive value for BG <40 mg/dL. Sensitivity for a BG <40 mg/dL was 76% and 51.5% for BG <60 mg/dL. The alert was developed with a 50% sensitivity for a BG of <60 mg/dL, and the sensitivities calculated on control floors were consistent with the original modeling. The predictive value of an LR classification was 98.2% for not having a BG <40 mg/dL. The predictive value of a positive alert was 9.7% for BG <40 mg/dL.
Variable | 40 mg/dL Threshold | 60 mg/dL Threshold |
---|---|---|
| ||
Sensitivity: probability of an alert given BG <40 or 60 mg/dL | 76.0% | 51.5% |
Specificity: probability of no alert given BG >40 or 60 mg/dL | 64.6% | 66.0% |
Positive predictive value | 9.7% | 26.7% |
Negative predictive value (nonalerted patients identified as low risk) | 98.2% | 85.0% |
There was no significant difference in mortality (P=0.726), transfer to a higher level of care (P=0.296), or LOS between the 2 groups (HR‐I: 5.83 days vs HR‐C: 5.88 days, P=0.664). However, patients with a BG <40 mg/dL had an LOS of 12.2 days (N=45) versus 8.1 days for those without an SH event (N=610), which was statistically significant (P=0.005). There was no increase in the incidence of BG >299 mg/dL in the HR‐I versus HR‐C groups (P=0.53).
Nurse‐physician satisfaction with the alert process was evaluated using a collaboration scale completed after each alert.[8] Of the 195 hypoglycemia alerts, there were 167 (85.6%) nurse and 25 (12.8%) physician collaboration scales completed. Scores were similar among nurses (average 1.52) and physicians (average 1.72), reflecting positive experiences with collaboration. Orders were changed in 40.7% HR‐I patients in response to the collaboration, but in only 20.5% of HR‐C patients after the initial BG of <90 mg/dL occurred. A change in orders constituted a modification consistent with lowering the risk of hypoglycemia and included discontinuing an oral antidiabetic agent, lowering the dose of insulin, and rarely the addition of dextrose‐containing fluids. The most common change in orders was a reduction in the total dose of insulin. A difference in orders changed was partially explained by the collaboration score; a 1‐unit increase in the score correlated to an odds ratio of 2.10 that the orders would be changed (P=0.002).
DISCUSSION
Hospitals are accountable for safe and effective care of patients with hyperglycemia, which includes prevention of medication‐induced hypoglycemia. We have developed a predictive informatics hypoglycemia risk alert that, when tested in a real‐world situation, significantly reduced the rate of SH in hospitalized patients without increasing severe hyperglycemia. The alert algorithm correctly identified patients who were at high risk for hypoglycemia and allowed caretakers the opportunity to lower that risk. The positive predictive value of the alert was low but acceptable at 9.7%, owing to the overall low rate of hypoglycemia in the patient population.
The alert model tested involved 3 components for success: the automated alert, trained charge nurse responders, and an interaction between the nurse responder and the care provider. HR‐I patients were interviewed and assessed for problems associated with oral intake, dietary habits, medication compliance, and hypoglycemia at home prior to communicating with physicians. The extensive training and proficiency in patient assessment and SBAR communication process required by nurses was paramount in the success of the alert. However, the alert provided a definitive risk assessment that was actionable, versus more global instruction, which has not had the same impact in risk reduction. Based on feedback collected from nurses at the study end, they felt the alert process was within their scope of practice and was not unduly burdensome. They also found that the training in diabetes management and SBAR communication techniques, in addition to the alert system, were useful in protecting patients from medication harm.
Physicians for HR‐C patients missed many opportunities to effectively intervene and thereby reduce the likelihood of an SH event. Our assumption is that the clinicians did not ascertain the risk of SH, which was reflected by the fact that orders were changed in 40.7% of HR‐I patients versus only 20.5% in the HR‐C group. Having alerts go directly to nurses rather than physicians permitted inclusion of additional information, such as caloric intake and testing schedules, so that changes in orders would have greater context, and the importance of mild hypoglycemia would not be overlooked.[16] Glycemic control is challenging for providers in the inpatient setting, as there is little time to test and titrate doses of insulin to achieve control. Tight glycemic control has become the primary focus of diabetes management in the outpatient setting to reduce long‐term risks of microvascular complications.[17, 18] However, establishing glycemic targets in the inpatient setting has been difficult because the risk for hypoglycemia increases with tighter control.[19, 20] Inpatient hypoglycemia has been associated with increased mortality, particularly in critically ill patients.[21, 22] Many factors contribute to hypoglycemia including low creatinine clearance, low body weight, untested insulin doses, errors in insulin administration, unexpected dietary changes, changes in medications affecting BG levels, poor communication during times of patient transfer to different care teams, and poor coordination of BG testing with insulin administration at meal times. A multifaceted approach aimed at improving both clinician and nurse awareness, and providing real‐time risk assessment is clearly required to insure patient safety.[6, 13, 23, 24]
There are significant economic benefits to avoiding SH in the hospital given the adverse outcomes associated with HACs and the extra cost associated with these conditions. In hospitalized patients, hypoglycemia worsens outcomes leading to higher costs due to longer LOS (by 3 days), higher inpatient charges (38.9%), and higher risk of discharge to a skilled nursing facility.[1, 3, 25, 26] Conversely, improved glycemic control can reduce surgical site infections, perioperative morbidity, and hospital LOS.[27] The high prevalence of insulin use among inpatients, many of whom have high‐risk characteristics, creates a milieu for both hyper‐ and hypoglycemia. Other groups have described a drop in hypoglycemia rates related to the use of standardized diabetes order sets and nurse and physician education, but this is the first study that used informatics in a prospective manner to identify patients who are at high risk for developing hypoglycemia and then specifically targeted those patients.[28] The alert process was modeled after a similar alert developed in our institution for identifying medicine patients at risk for sepsis.[29] Given the paucity of data related to inpatient glycemia risk reduction, this study is particularly relevant for improving patient safety.
The major limitation of this study is that it was not randomized at the patient level. Patients were assigned to intervention and control groups based on their occupancy on specific hospital floors to avoid treatment bias. Bias was assessed due to this nonrandom assignment by comparing demographic and clinical factors of HR patients between intervention and control floors, and found significant differences in HbA1c and admitting diagnosis. As the control group had lower HbA1c values than the intervention group, and it is known from the Diabetes Control and Complications Trial and Action to Control Cardiovascular Risk in Diabetes trial that lower HbA1c increases the risk of hypoglycemia, our results may be biased by the level of glucose control on admission.[30, 31] Admitting diagnoses differed significantly between intervention and control patients as did the Charlson Comorbidity Index score; however, the hypoglycemia alert system does not include patient diagnoses or comorbidities, and as such provided equipoise with regard to risk reduction regardless of presenting illness. This study included trained nurses, which may be beyond the scope of every institution and thereby limit the effectiveness of the alert in reducing risk. However, as a result of this study, the alert was expanded to other acute care floors at our hospital as well as other hospitals in the Barnes‐Jewish Hospital system.
In summary, this study showed a 68% decrease in episodes of SH in a high‐risk patient cohort on diabetic medications using a hypoglycemia alert system. The results of this study demonstrate the validity of a systems‐based approach to reduce SH in high‐risk inpatients.
Disclosures
This work was funded by the Barnes‐Jewish Hospital Foundation The authors report no conflicts of interest.
Insulin therapy in the hospital setting can cause hypoglycemia, which may lead to increased mortality and length of stay (LOS).[1, 2, 3] Hypoglycemia is associated with cardiovascular, cerebrovascular, and patient fall events.[4, 5] The Centers for Medicare and Medicaid Services have designated both severe hypoglycemia (SH) with harm and diabetic ketoacidosis as hospital acquired conditions (HAC) or never events. The Society for Hospital Medicine (SHM) defines SH in the hospital as a blood glucose (BG) <40 mg/dL. Minimizing episodes of SH is important for patient health outcomes, patient safety, and for healthcare facilities' safety metrics.
Many factors contribute to SH including excessive insulin doses, medication errors, inappropriate timing of insulin doses with food intake, changes in nutritional status, impaired renal function, and changes in medications such as steroids.[6] As part of a multiyear project in patient safety, an inpatient hypoglycemia alert algorithm was developed based on a multivariate analysis of individual patient demographic, pharmacy, laboratory, and glucometric data. The algorithm was previously shown to have a 75% sensitivity to predict episodes of SH.[7] In this study, we tested whether a predictive real‐time informatics hypoglycemia alert based on the tested algorithm, along with trained nurses, would result in a decreased frequency of SH events compared to usual care. We hypothesized that this alert would result in a reduction of SH events in those patients at high risk for hypoglycemia.
METHODS
Study Design and Population
This prospective cohort‐intervention study involved inpatients admitted to Barnes‐Jewish Hospital in St. Louis, Missouri, the academic hospital of Washington University School of Medicine (WUSM), from August 2011 through December 2011. Fourteen floors, including 10 internal medicine and 4 cardiology medicine floors, were selected based upon a high frequency of severe hypoglycemic events noted in 2010. Six of the internal medicine floors were designated as intervention floors, and 8 were designated as control floors, including the 4 cardiology units. The study population consisted of patients receiving diabetic medications on study floors who had a BG <90 mg/dL during their hospital stay (Figure 1). The study was approved by the WUSM institutional review board and included a waiver of consent for individual patients.
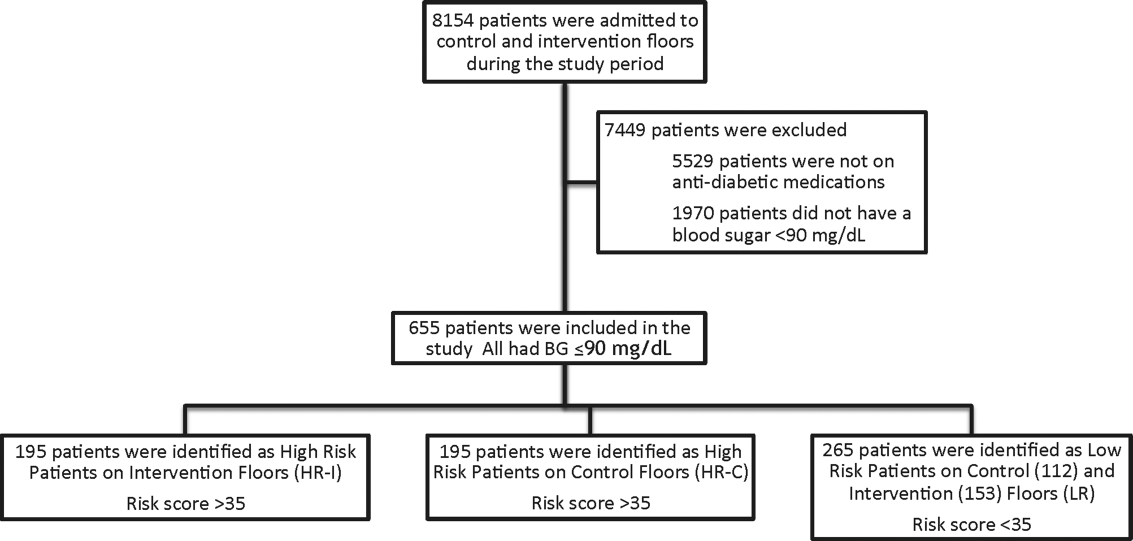
The pharmacy informatics system was programmed with the previously developed hypoglycemia alert to prospectively identify those patients at high risk of hypoglycemia based on real‐time patient information.[7] Patients were identified as high risk on study floors if insulin or an oral antihyperglycemic agent was prescribed and if their hypoglycemia informatics generated risk score was >35 within 24 hours of having a capillary or venous BG<90 mg/dL. The risk score of 35 corresponded to a 50% sensitivity for a subsequent BG <60 mg/dL and a 75% sensitivity for a BG <40 mg/dL. Patients who generated an alert once during their hospital stay were assigned to 1 of 3 categories based on their admission division and risk score: high‐risk intervention (HR‐I), high‐risk control (HR‐C), or low risk (LR). LR patients also had a BG <90 mg/dL during their stay, but a risk score of <35.
The electronic alert for HR‐I patients was sent by pager to division‐specific charge nurses. Fourteen charge nurses on intervention divisions were trained to assess the alert, interview the patient, identify an alternate dosing strategy, and collaborate with the patient's physicians. HR‐C patients were identified on control divisions based on the same criteria as intervention patients, but no alert was generated. Control patients' charts were reviewed and evaluated upon discharge by the research team‐certified diabetes nurse educator to determine whether the treating physician had identified the SH risk and had changed insulin orders.
Nurses and physicians caring for patients on study divisions provided informed consent to participate in the study. Nurses' satisfaction with the alert process and physician interaction was assessed with a collaboration scale that was completed after each alert (see Supporting Information, Appendix A, in the online version of this article).[8]
Alert Development Process
The alert equation algorithm was developed at Barnes‐Jewish Hospital after a retrospective analysis of hospital glucometric data, including capillary and venous BG measurements, and demographic and pharmacy data over a 6‐month time period.[9] The analysis identified factors that were independently associated with hypoglycemia and used these variables in a mathematical model to achieve a 50% sensitivity to predict a subsequent BG of <60 mg/dL and a 75% sensitivity to predict SH.[7] Table 1 outlines the variables in the model and provides the risk‐score equation used to generate an alert.
Variable | Description of Variable |
---|---|
Body weight | Patients at a lower weight were at an increased risk. The variable had a linear response, and 3 levels were used to modify the risk equation: <69 kg, 7079 kg, and >80 kg. |
Creatinine clearance | Patients with a lower creatinine clearance were at an increased risk. This variable had a linear response, and 2 levels were used to modify the risk equation: <48 mL/min or >48 mL/min. |
Basal insulin dose | Increased risk was noted at a doses of basal insulin >0.25 U/kg. |
Basal‐only dosing | Dosing of basal insulin without meal‐time insulin conferred increased risk. |
Nonstandard insulin therapy | The use of 70/30 insulin was associated with increased risk. |
Oral diabetic therapy | Use of sulfonylureas was associated with increased risk. |
Risk score equation | (Value <60)=0.055+1.062 * (Basal <0.25 U/kg)+1.234 * (Basal 0.25 U/kg) & minus;0.294 * (Weight <6069 kg)0.540 * (Weight 7079 kg)0.786 * (Weight 80 kg) & minus;0.389 * (Creatinine Clearance <3847) 0.680 * (Creatinine Clearance 48) 0.239 * (Sliding Yes) 0.556*(Meal Yes)+0.951 * (Sliding and Meal)+0.336 * (Sulfonylurea Yes) Score=100 * (Exp (Value <60)/(1+Exp (Value <60)) |
The alert used a BG cutoff of 90 mg/dL in accordance with the American College of Endocrinology Hospital Guideline. Although current guidelines from the SHM recommend keeping BG values >100 mg/dL for patient safety, our analysis found that the cutoff of 90 mg/dL had better sensitivity and specificity than the <100 mg/dL guideline for the risk algorithm.[10, 11]
Nurse and Physician Training
Charge nurses received 5 hours of hyperglycemia management training in 3 sessions utilizing a structured curriculum. Session 1 included a pretest followed by diabetes management education. Session 2 was devoted to an interactive workshop utilizing case‐based scenarios of diabetes management problems and hypoglycemia prevention. The final session provided instructions on the electronic alert communication process. Nurses were empowered with tools for effective communication practices using the situation‐background‐assessment‐recommendation (SBAR) technique.[12]
Physicians, including hospitalists and medicine residents on intervention and control floors, took a pretest, received a 1‐hour lecture, and completed the same curriculum of case‐based scenarios in an online self‐directed learning module. Physicians did not receive SBAR training. Both nurses and physicians received pocket cards with insulin management guidelines developed by our research team to ensure that all clinicians had common prescribing practices.[13]
Outcomes
The primary outcome was the incidence of SH occurring in HR‐I versus HR‐C patients. Secondary outcomes included: episodes of SH in LR study patients, incidence of BG<60 mg/dL frequency of transfer to a higher level of care, incidence of severe hyperglycemia defined as BG >299 mg/dL, frequency that high‐risk patient's orders were changed to reduce hypoglycemia risk in response to the alert‐intervention process, LOS, mortality, and a nurse‐physician collaboration scale score.[14]
Statistical Analysis
Demographic and clinical metrics were compared between HR‐I and HR‐C patients to evaluate potential sources of bias. These included age, weight, serum creatinine, creatinine clearance (measured by Cockcroft‐Gault), hemoglobin A1c (HbA1c) if available, LOS, gender, admitting diagnosis, type of diabetes, and Charlson Comorbidity Index score. The alert risk‐score was also compared between intervention and control floors. Two‐tailed t tests assessed differences between the study groups on normally distributed variables, whereas Wilcoxon rank sum tests were used for non‐normally distributed variables, and 2 tests were used for categorical variables. Two‐tailed Fisher exact tests compared the prevalence of hypoglycemia thresholds between the study groups. 2 analysis was used to compare the proportion of patients who experienced a BG >299 mg/dL between intervention and controls and the proportion of orders changed in HR‐I versus HR‐C patients. Logistic regression was used to test the association of nurse collaboration score with the likelihood of orders being changed.
Based on previous research, we estimated a 48% rate of hypoglycemia <60 mg/dL in HR‐C patients on control floors.[7] We calculated a sample size of 195 subjects in each high‐risk group as the number needed for the intervention to produce a clinically meaningful reduction in hypoglycemia of 25% on the intervention floor compared to the control floors with 90% power.
RESULTS
Study Cohort and Patient Characteristics
One hundred ninety‐five patients who met criteria for high‐risk status were enrolled on HR‐I floors and HR‐C floors for a total of 390 high‐risk patients. During the same time period, 265 LR patients were identified on intervention (153 patients) and control (112 patients) floors. The HR‐I patients were similar to the HR‐C patients by baseline demographics, as shown in Table 2. HbA1c was not available on all patients, but the mean HbA1c in the HR‐I group was 7.93% versus 7.40% in the HR‐C group (P=0.048). The Charlson Comorbidity Index score was significantly different between the high‐risk groups (HR‐I: 6.48 vs HR‐C: 7.48, P=0.002), indicating that the HR‐C patients had more comorbidities.[15] There were significant differences in 2 of the 3 most common admitting diagnoses between groups, with more HR‐C patients admitted for circulatory system diseases (HR‐C: 22.3% vs HR‐I: 4.4%, P=0.001), and more HR‐I patients admitted for digestive system diseases (HR‐I: 13.7% vs HR‐C: 3.3%, P<0.001). The proportion of patients with preexisting type 2 diabetes did not differ by intervention status (HR‐I: 89.8% vs HR‐C: 92.0%, P=0.462).
Demographic | HR‐I, Mean SD/Frequency (%), N=195 | HR‐C, Mean, SD/Frequency (%), N=195 | Low Risk, Mean, SD/Frequency (%), N=265 | P Value* |
---|---|---|---|---|
| ||||
Age, y | 60.2 (15.1) | 60.3 (16.9) | 61.0 (13.8) | 0.940 |
Weight, kg | 84.9 (31.9) | 80.8 (26.6) | 93.6 (28.7) | 0.173 |
Serum creatinine, mg/dL | 2.06 (2.56) | 2.03 (1.87) | 1.89 (2.17) | 0.910 |
Creatinine clearance, mL/min | 50.6 (29.8) | 45.4 (27.1) | 55.5 (29.3) | 0.077 |
Hemoglobin A1c, n (%) with data | 7.93 (2.46), n=130 (67%) | 7.40 (1.75), n=115 (59%) | 6.65 (2.05), n=152 (57%) | 0.048 |
Risk score | 52 (11) | 54 (11) | 26 (6) | 0.111 |
Length of stay, median, d | 5.83 | 5.88 | 5.79 | 0.664 |
Male gender | 84 (43.1%) | 98 (50.3%) | 145 (54.7%) | 0.155 |
Type 2 diabetes | 167 (89.8%) | 172 (92.0%) | 219 (95.6%) | 0.462 |
Charlson Comorbidity Index score | 6.48 (3.06) | 7.48 (3.28) | 6.66 (3.24) | 0.002 |
Admit diagnosis endocrine, nutritional, metabolic diseases, and immunity disorders (codes 240279) | 17 (9.3%) | 10 (5.4%) | 11 (4.3%) | 0.153 |
Admit diagnosis disease of circulatory system (codes 390459) | 8 (4.4%) | 41 (22.3%) | 26 (10.1%) | <0.001 |
Admit diagnosis disease of digestive system (codes 520579) | 25 (13.7%) | 6 (3.3%) | 27 (10.5%) | <0.001 |
Admit diagnosis diseases of the genitourinary system (codes 580629) | 6 (3.3%) | 4 (2.2%) | 15 (5.8%) | 0.510 |
Admit diagnosis reported only as signs, symptoms, or ill‐defined conditions (codes 780799) | 77 (42.3%) | 92 (50.0%) | 121 (46.9%) | 0.140 |
Study Outcomes
The rate of hypoglycemia was compared between 195 HR‐I and 195 HR‐C patients, and it should be noted that each patient could generate only 1 episode of hypoglycemia during an admission. As shown in Table 3, the incidence of a BG <60 mg/dL was significantly lower in the HR‐I patients versus the HR‐C patients (13.3% vs 26.7%, P=0.002) as was the incidence of a BG <40 mg/dL (3.1% HR‐I vs 9.7% HR‐C, P=0.012). This represents a decrease of 50% in moderate hypoglycemia (BG <60 mg/dL) and a decrease of 68% in SH (BG <40 mg/dL) between HR‐I and HR‐C patients. Severe hyperglycemia occurrences were not significantly different between intervention and control floors at 28% each.
Alerted Patients Glucose Threshold | HR‐I (%), N=195 | HR‐C (%), N=195 | Low Risk (%), N=265 | P Value* |
---|---|---|---|---|
| ||||
With BG <40 mg/dL | 6 (3.1%) | 19 (9.7%) | 10 (3.8%) | 0.012 |
With BG <60 mg/dL | 26 (13.3%) | 51 (26.7%) | 50 (18.9%) | 0.002 |
With BG >299 mg/dL | 53 (28.0%) | 53 (27.9%) | 29 (11.9%) | 0.974 |
The sensitivity, specificity, and predictive values of the alert for BG thresholds of <40 mg/dL and <60 mg/dL are presented in Table 4. On control floors, the alert exhibited a modest sensitivity and high negative predictive value for BG <40 mg/dL. Sensitivity for a BG <40 mg/dL was 76% and 51.5% for BG <60 mg/dL. The alert was developed with a 50% sensitivity for a BG of <60 mg/dL, and the sensitivities calculated on control floors were consistent with the original modeling. The predictive value of an LR classification was 98.2% for not having a BG <40 mg/dL. The predictive value of a positive alert was 9.7% for BG <40 mg/dL.
Variable | 40 mg/dL Threshold | 60 mg/dL Threshold |
---|---|---|
| ||
Sensitivity: probability of an alert given BG <40 or 60 mg/dL | 76.0% | 51.5% |
Specificity: probability of no alert given BG >40 or 60 mg/dL | 64.6% | 66.0% |
Positive predictive value | 9.7% | 26.7% |
Negative predictive value (nonalerted patients identified as low risk) | 98.2% | 85.0% |
There was no significant difference in mortality (P=0.726), transfer to a higher level of care (P=0.296), or LOS between the 2 groups (HR‐I: 5.83 days vs HR‐C: 5.88 days, P=0.664). However, patients with a BG <40 mg/dL had an LOS of 12.2 days (N=45) versus 8.1 days for those without an SH event (N=610), which was statistically significant (P=0.005). There was no increase in the incidence of BG >299 mg/dL in the HR‐I versus HR‐C groups (P=0.53).
Nurse‐physician satisfaction with the alert process was evaluated using a collaboration scale completed after each alert.[8] Of the 195 hypoglycemia alerts, there were 167 (85.6%) nurse and 25 (12.8%) physician collaboration scales completed. Scores were similar among nurses (average 1.52) and physicians (average 1.72), reflecting positive experiences with collaboration. Orders were changed in 40.7% HR‐I patients in response to the collaboration, but in only 20.5% of HR‐C patients after the initial BG of <90 mg/dL occurred. A change in orders constituted a modification consistent with lowering the risk of hypoglycemia and included discontinuing an oral antidiabetic agent, lowering the dose of insulin, and rarely the addition of dextrose‐containing fluids. The most common change in orders was a reduction in the total dose of insulin. A difference in orders changed was partially explained by the collaboration score; a 1‐unit increase in the score correlated to an odds ratio of 2.10 that the orders would be changed (P=0.002).
DISCUSSION
Hospitals are accountable for safe and effective care of patients with hyperglycemia, which includes prevention of medication‐induced hypoglycemia. We have developed a predictive informatics hypoglycemia risk alert that, when tested in a real‐world situation, significantly reduced the rate of SH in hospitalized patients without increasing severe hyperglycemia. The alert algorithm correctly identified patients who were at high risk for hypoglycemia and allowed caretakers the opportunity to lower that risk. The positive predictive value of the alert was low but acceptable at 9.7%, owing to the overall low rate of hypoglycemia in the patient population.
The alert model tested involved 3 components for success: the automated alert, trained charge nurse responders, and an interaction between the nurse responder and the care provider. HR‐I patients were interviewed and assessed for problems associated with oral intake, dietary habits, medication compliance, and hypoglycemia at home prior to communicating with physicians. The extensive training and proficiency in patient assessment and SBAR communication process required by nurses was paramount in the success of the alert. However, the alert provided a definitive risk assessment that was actionable, versus more global instruction, which has not had the same impact in risk reduction. Based on feedback collected from nurses at the study end, they felt the alert process was within their scope of practice and was not unduly burdensome. They also found that the training in diabetes management and SBAR communication techniques, in addition to the alert system, were useful in protecting patients from medication harm.
Physicians for HR‐C patients missed many opportunities to effectively intervene and thereby reduce the likelihood of an SH event. Our assumption is that the clinicians did not ascertain the risk of SH, which was reflected by the fact that orders were changed in 40.7% of HR‐I patients versus only 20.5% in the HR‐C group. Having alerts go directly to nurses rather than physicians permitted inclusion of additional information, such as caloric intake and testing schedules, so that changes in orders would have greater context, and the importance of mild hypoglycemia would not be overlooked.[16] Glycemic control is challenging for providers in the inpatient setting, as there is little time to test and titrate doses of insulin to achieve control. Tight glycemic control has become the primary focus of diabetes management in the outpatient setting to reduce long‐term risks of microvascular complications.[17, 18] However, establishing glycemic targets in the inpatient setting has been difficult because the risk for hypoglycemia increases with tighter control.[19, 20] Inpatient hypoglycemia has been associated with increased mortality, particularly in critically ill patients.[21, 22] Many factors contribute to hypoglycemia including low creatinine clearance, low body weight, untested insulin doses, errors in insulin administration, unexpected dietary changes, changes in medications affecting BG levels, poor communication during times of patient transfer to different care teams, and poor coordination of BG testing with insulin administration at meal times. A multifaceted approach aimed at improving both clinician and nurse awareness, and providing real‐time risk assessment is clearly required to insure patient safety.[6, 13, 23, 24]
There are significant economic benefits to avoiding SH in the hospital given the adverse outcomes associated with HACs and the extra cost associated with these conditions. In hospitalized patients, hypoglycemia worsens outcomes leading to higher costs due to longer LOS (by 3 days), higher inpatient charges (38.9%), and higher risk of discharge to a skilled nursing facility.[1, 3, 25, 26] Conversely, improved glycemic control can reduce surgical site infections, perioperative morbidity, and hospital LOS.[27] The high prevalence of insulin use among inpatients, many of whom have high‐risk characteristics, creates a milieu for both hyper‐ and hypoglycemia. Other groups have described a drop in hypoglycemia rates related to the use of standardized diabetes order sets and nurse and physician education, but this is the first study that used informatics in a prospective manner to identify patients who are at high risk for developing hypoglycemia and then specifically targeted those patients.[28] The alert process was modeled after a similar alert developed in our institution for identifying medicine patients at risk for sepsis.[29] Given the paucity of data related to inpatient glycemia risk reduction, this study is particularly relevant for improving patient safety.
The major limitation of this study is that it was not randomized at the patient level. Patients were assigned to intervention and control groups based on their occupancy on specific hospital floors to avoid treatment bias. Bias was assessed due to this nonrandom assignment by comparing demographic and clinical factors of HR patients between intervention and control floors, and found significant differences in HbA1c and admitting diagnosis. As the control group had lower HbA1c values than the intervention group, and it is known from the Diabetes Control and Complications Trial and Action to Control Cardiovascular Risk in Diabetes trial that lower HbA1c increases the risk of hypoglycemia, our results may be biased by the level of glucose control on admission.[30, 31] Admitting diagnoses differed significantly between intervention and control patients as did the Charlson Comorbidity Index score; however, the hypoglycemia alert system does not include patient diagnoses or comorbidities, and as such provided equipoise with regard to risk reduction regardless of presenting illness. This study included trained nurses, which may be beyond the scope of every institution and thereby limit the effectiveness of the alert in reducing risk. However, as a result of this study, the alert was expanded to other acute care floors at our hospital as well as other hospitals in the Barnes‐Jewish Hospital system.
In summary, this study showed a 68% decrease in episodes of SH in a high‐risk patient cohort on diabetic medications using a hypoglycemia alert system. The results of this study demonstrate the validity of a systems‐based approach to reduce SH in high‐risk inpatients.
Disclosures
This work was funded by the Barnes‐Jewish Hospital Foundation The authors report no conflicts of interest.
- Hypoglycaemia is associated with increased length of stay and mortality in people with diabetes who are hospitalized. Diabet Med. 2012;29:e445–e448. , , , , , .
- Hypoglycemia as a predictor of mortality in hospitalized elderly patients. Arch Intern Med. 2003;163:1825–1829. , , , et al.
- Hypoglycemia and clinical outcomes in patients with diabetes hospitalized in the general ward. Diabetes Care. 2009;32:1153–1157. , , , , , .
- Association of hypoglycemia and cardiac ischemia: a study based on continuous glucose monitoring. Diabetes Care. 2003;26:1485–1489. , , , , .
- Diabetes‐related complications, glycemic control, and falls in older adults. Diabetes Care. 2008;31:391–396. , , , et al.
- Inpatient insulin orders: are patients getting what is prescribed? J Hosp Med. 2011;9:526–529. , , , , .
- Prediction and prevention of treatment‐related inpatient hypoglycemia. J Diabetes Sci Technol. 2012;6:302–309. , , , .
- Collaboration between nurses and physicians. Image J Nurs Sch. 1988;20:145–149. , .
- Glucometrics—assessing the quality of inpatient glucose management. Diabetes Technol Ther. 2005;8:560–569. , , , et al.
- ACE/ADA Task Force on Inpatient Diabetes. American College of Endocrinology and American Diabetes Association consensus statement on inpatient diabetes and glycemic control. Diabetes Care. 2006;29:1955–1962.
- American Association of Clinical Endocrinologists and American Diabetes Association consensus statement on inpatient glycemic control. Endocr Pract. 2009;15:353–369. , , , et al.
- Role‐play using SBAR technique to improve observed communication skills in senior nursing students. J Nurs Educ. 2011;50:79–87. .
- SHM Glycemic Control Task Force. Workbook for improvement: improving glycemic control, preventing hypoglycemia, and optimizing care of the inpatient with hyperglycemia and diabetes. Society of Hospital Medicine website, Glycemic Control Quality Improvement Resource Room. Available at: http://www.hospitalmedicine.org. Accessed on February 12, 2011.
- An internet service supporting quality assessment of inpatient glycemic control. J Diabetes Sci and Technol. 2008;2:402–408. , .
- Updating and validating the Charlson Comorbidity Index and score for risk adjustment in hospital discharge abstracts using data from 6 countries. Am J Epidemiol. 2011;173:676–682. , , , et al.
- Glycemic management in medical and surgical patients in the non‐ICU setting. Curr Diab Rep. 2013;13:96–106. , , .
- The Diabetes Control and Complications Trial Research Group. The effect of intensive treatment of diabetes on the development and progression of long‐term complications in insulin‐dependent diabetes mellitus. N Engl J Med. 1993;329:977–986.
- UK Prospective Diabetes Study Group. Intensive blood‐glucose control with sulphonylureas or insulin compared with conventional treatment and risk of complications in patients with type 2 diabetes. Lancet. 1998;352:837–853.
- Hypoglycemia in diabetes. Diabetes Care. 2003;26:1902–1912. , , .
- Hypoglycemia: still the limiting factor in the glycemic management of diabetes. Endocr Pract. 2008;14:750–756. .
- for the NICE‐SUGAR Study Investigators. Hypoglycemia and risk of death in critically ill patients. N Engl J Med. 2012;367:1108–1118. , , , et al.;
- Increased mortality of patients with diabetes reporting severe hypoglycemia. Diabetes Care. 2012;35:1897–1901. , , , , , .
- Risk factors for inpatient hypoglycemia during subcutaneous insulin therapy in non‐critically ill patients with type 2 diabetes. J Diabetes Sci Technol. 2012;6:1022–1029. , , , et al.
- Minimizing hypoglycemia in the wake of a tight glycemic control protocol in hospitalized patients. J Nurs Care Qual. 2010;25:255–260. , , .
- The economic and quality of life impact of hypoglycemia. Eur J Health Econ. 2005;6:197–202. , , , .
- Economic and clinical impact of inpatient diabetic hypoglycemia. Endocr Pract. 2009;15:302–312. , , , , , .
- Mild hypoglycemia is strongly associated with increased intensive care unit length of stay. Ann Intensive Care. 2011;49:1–49. , , , et al.
- Implementing and evaluating a multicomponent inpatient diabetes management program: putting research into practice. Jt Comm J Qual Patient Saf. 2012;38:195–206. , , , et al.
- Implementation of a real‐time computerized sepsis alert in nonintensive care unit patients. Crit Care Med. 2011;39:469–473. , , , et al.
- The Diabetes Control and Complications Trial Research Group. Epidemiology of severe hypoglycemia in the Diabetes Control and Complications Trial. Am J Med. 1991;90:450–459.
- The effects of baseline characteristics, glycemia treatment approach, and glycated hemoglobin concentration on the risk of severe hypoglycaemia: post hoc epidemiological analysis of the ACCORD study. BMJ. 2010;340:1–12. , , , et al.
- Hypoglycaemia is associated with increased length of stay and mortality in people with diabetes who are hospitalized. Diabet Med. 2012;29:e445–e448. , , , , , .
- Hypoglycemia as a predictor of mortality in hospitalized elderly patients. Arch Intern Med. 2003;163:1825–1829. , , , et al.
- Hypoglycemia and clinical outcomes in patients with diabetes hospitalized in the general ward. Diabetes Care. 2009;32:1153–1157. , , , , , .
- Association of hypoglycemia and cardiac ischemia: a study based on continuous glucose monitoring. Diabetes Care. 2003;26:1485–1489. , , , , .
- Diabetes‐related complications, glycemic control, and falls in older adults. Diabetes Care. 2008;31:391–396. , , , et al.
- Inpatient insulin orders: are patients getting what is prescribed? J Hosp Med. 2011;9:526–529. , , , , .
- Prediction and prevention of treatment‐related inpatient hypoglycemia. J Diabetes Sci Technol. 2012;6:302–309. , , , .
- Collaboration between nurses and physicians. Image J Nurs Sch. 1988;20:145–149. , .
- Glucometrics—assessing the quality of inpatient glucose management. Diabetes Technol Ther. 2005;8:560–569. , , , et al.
- ACE/ADA Task Force on Inpatient Diabetes. American College of Endocrinology and American Diabetes Association consensus statement on inpatient diabetes and glycemic control. Diabetes Care. 2006;29:1955–1962.
- American Association of Clinical Endocrinologists and American Diabetes Association consensus statement on inpatient glycemic control. Endocr Pract. 2009;15:353–369. , , , et al.
- Role‐play using SBAR technique to improve observed communication skills in senior nursing students. J Nurs Educ. 2011;50:79–87. .
- SHM Glycemic Control Task Force. Workbook for improvement: improving glycemic control, preventing hypoglycemia, and optimizing care of the inpatient with hyperglycemia and diabetes. Society of Hospital Medicine website, Glycemic Control Quality Improvement Resource Room. Available at: http://www.hospitalmedicine.org. Accessed on February 12, 2011.
- An internet service supporting quality assessment of inpatient glycemic control. J Diabetes Sci and Technol. 2008;2:402–408. , .
- Updating and validating the Charlson Comorbidity Index and score for risk adjustment in hospital discharge abstracts using data from 6 countries. Am J Epidemiol. 2011;173:676–682. , , , et al.
- Glycemic management in medical and surgical patients in the non‐ICU setting. Curr Diab Rep. 2013;13:96–106. , , .
- The Diabetes Control and Complications Trial Research Group. The effect of intensive treatment of diabetes on the development and progression of long‐term complications in insulin‐dependent diabetes mellitus. N Engl J Med. 1993;329:977–986.
- UK Prospective Diabetes Study Group. Intensive blood‐glucose control with sulphonylureas or insulin compared with conventional treatment and risk of complications in patients with type 2 diabetes. Lancet. 1998;352:837–853.
- Hypoglycemia in diabetes. Diabetes Care. 2003;26:1902–1912. , , .
- Hypoglycemia: still the limiting factor in the glycemic management of diabetes. Endocr Pract. 2008;14:750–756. .
- for the NICE‐SUGAR Study Investigators. Hypoglycemia and risk of death in critically ill patients. N Engl J Med. 2012;367:1108–1118. , , , et al.;
- Increased mortality of patients with diabetes reporting severe hypoglycemia. Diabetes Care. 2012;35:1897–1901. , , , , , .
- Risk factors for inpatient hypoglycemia during subcutaneous insulin therapy in non‐critically ill patients with type 2 diabetes. J Diabetes Sci Technol. 2012;6:1022–1029. , , , et al.
- Minimizing hypoglycemia in the wake of a tight glycemic control protocol in hospitalized patients. J Nurs Care Qual. 2010;25:255–260. , , .
- The economic and quality of life impact of hypoglycemia. Eur J Health Econ. 2005;6:197–202. , , , .
- Economic and clinical impact of inpatient diabetic hypoglycemia. Endocr Pract. 2009;15:302–312. , , , , , .
- Mild hypoglycemia is strongly associated with increased intensive care unit length of stay. Ann Intensive Care. 2011;49:1–49. , , , et al.
- Implementing and evaluating a multicomponent inpatient diabetes management program: putting research into practice. Jt Comm J Qual Patient Saf. 2012;38:195–206. , , , et al.
- Implementation of a real‐time computerized sepsis alert in nonintensive care unit patients. Crit Care Med. 2011;39:469–473. , , , et al.
- The Diabetes Control and Complications Trial Research Group. Epidemiology of severe hypoglycemia in the Diabetes Control and Complications Trial. Am J Med. 1991;90:450–459.
- The effects of baseline characteristics, glycemia treatment approach, and glycated hemoglobin concentration on the risk of severe hypoglycaemia: post hoc epidemiological analysis of the ACCORD study. BMJ. 2010;340:1–12. , , , et al.
© 2014 Society of Hospital Medicine
Hospital Reporting of Glomerular Filtration Rate
Chronic kidney disease is increasingly recognized as a significant public health issue, especially as our population ages. In the United States, it is estimated that 19.2 million individuals have chronic kidney disease (CKD), with an increasing prevalence in the elderly.1 CKD is associated with a higher mortality rate, as well as an increased risk of having several comorbidities, including anemia, coronary artery disease, and congestive heart failure.24 Early recognition, intervention, and management of patients with CKD by physicians has been shown to slow progression of disease and decrease complications.57 In the hospital setting, patients with CKD are at increased risk of medication dosing errors and acute renal failure (ARF).810
Serum creatinine is the most commonly used laboratory marker for assessing renal function. However, creatinine level is an imprecise measure of overall renal function, especially in older patients. The most recent National Kidney Foundation/ Kidney Disease Outcomes Quality Initiative (K/DOQI) guidelines recommend laboratory reporting of a calculated estimate of GFR.11 Equations used to calculate estimated GFR in adults, including the Cockcroft‐Gault (C‐G) equation, have been shown to provide an estimate of renal function, which can be used to clinically stratify varying levels of impaired renal function.11 Several studies have demonstrated that recognition of CKD by physicians is low in various clinical settings, especially in elderly patients.1215 Compliance with renal‐dose medication guidelines has also frequently been noted to be poor.16, 17
The investigators conducted a chart review study before and after reporting of estimated GFR to physicians in a hospital setting to assess the effect on physician recognition of CKD, the primary outcome. Secondary outcomes included the effect of reporting GFR on physician prescribing behaviors at the time of hospital discharge, including dosing of renal‐dosed antibiotics and use of nonsteroidal anti‐inflammatory (NSAID) and cyclooxygenase type 2 inhibitor (COX‐2) medications.
METHODS
This study was a retrospective chart review, with a prospective chart review as a comparison. Patients selected were admitted to a general medical floor in a 900‐bed academic medical center over the 2 years from 2002 to 2004. Computerized databases of laboratory values and weights obtained during hospitalization were used to select patients who fulfilled the following criteria: age > 65 years, all creatinine values during hospitalization < 1.6 mg/dL, and calculated estimated creatinine clearance (CrCl) < 60 mL/min using the Cockcroft‐Gault (C‐G) formula. The C‐G equation was developed for estimating CrCl and has also been extensively tested as a predictor of GFR. K/DOQI guidelines identify the C‐G equation as the most frequently used equation to estimate GFR in adults.11 To ensure steady‐state renal function, patients were excluded if creatinine varied by more than 0.4 mg/dL during their hospitalization. Based on an anticipated CKD recognition rate of 24%,13 our study sample size was selected to detect a 13% difference in the primary end point between the pre‐ and postintervention groups with 80% power. The study was approved by the institutional review board of the medical school.
Patient charts were reviewed with data obtained from the medical record, including physician notes, discharge summaries, orders, medication lists, and discharge prescriptions. Physician recognition was defined by documentation of CKD, calculated CrCl, or GFR in the physician notes or discharge summary. Charts were reviewed for diagnosis of hypertension (HTN) or diabetes (DM), and discharge medications including NSAID and COX‐2 medications and use and correct dosing of antibiotics requiring dose adjustment in patients with decreased GFR. Aspirin was not included as an NSAID.
For the prospective chart review portion, patients were selected at the time of admission on the basis of the same criteria. A notification was placed in the chart prominently listing the patient's estimated GFR calculated using the C‐G equation. Also included was a list of the stages of chronic renal disease based on the most recent K/DOQI guidelines11 and recommendations on dosing of select renal‐dosed antibiotics. Patients were again excluded if creatinine varied more than 0.4 mg/dL during their hospitalization.
Data Analysis
For statistical analysis, the association between recognition of CKD and the chart intervention, unadjusted for covariates, was evaluated using a contingency table. Additionally, the associations between recognition of CKD and other patient covariatessex, diabetes, hypertension, estimated GFRwere analyzed both individually and jointly. For individual covariate analysis, Fisher's exact test was used in all tests for association. For joint analysis, a set of relevant covariates was determined by stepwise logistic regression. The association of CKD recognition and the intervention was again analyzed using logistic regression while adjusting for this set of relevant covariates.
Finally, an analysis of appropriate medication prescribing at the time of hospital discharge was carried out to assess the effect of reporting estimated GFR. Prescription of NSAID or COX‐2 medications and correct dosing of renal‐dosed antibiotics at discharge were analyzed separately. As in the exploratory covariate analysis, Fisher's exact test for association was used.
RESULTS
Study Population
Characteristics of the study cohort are summarized in Table 1. The pre‐ and postintervention groups had 260 and 198 patients, respectively. Most were female. Average age, serum creatinine, and estimated GFR were similar in both groups.
Characteristics | Preintervention | Postintervention |
---|---|---|
| ||
Total number | 260 | 198 |
Age (years) | 81.1 6.6 | 82 6.8 |
Sex (female) | 199 (76.5) | 168 (84.8) |
Serum creatinine (mg/dL) | 0.98 0.2 | 0.9 0.2 |
C‐G CrCl (mL/min) | 41.5 10.2 | 41.4 9.3 |
DM | 58 (22.3) | 63 (31.8) |
HTN | 190 (73.1) | 152 (76.7) |
Physician recognition of CKD | 10 (3.9) | 25 (12.6) |
NSAID or COX‐2 prescribed at discharge | 35 (13.5) | 21 (10.6) |
Antibiotic requiring renal‐dose adjustment prescribed at discharge | 50 (19.2) | 29 (14.2) |
Correct dosing of renal‐dosed antibiotic at discharge* | 28 (56.0) | 18 (62.1) |
Effect of Intervention on Recognition of CKD
Table 1 shows the number of patients recognized by physicians as having CKD in both groups. Prior to the study intervention, CKD was recognized in only 10 of 260 patients (3.9%), and following the intervention, rates increased to 25 of 198 patients (12.6%; P .001).
The results of the stepwise logistic regression of the covariates on CKD recognition showed that CKD recognition was modeled best with diabetes and lower estimated GFR. This corresponded well with the results of the individual covariate analyses. Thus, the primary outcome was again modeled by the intervention and the covariates diabetes and lower estimated GFR. With the addition of the covariates, the intervention was still a significant predictor of CKD recognition (P = .001), with an odds ratio of 4.07 (95% CI = (1.83,9.01)).
Effect of Intervention on Medication Prescribed at Hospital Discharge
Table 1 shows the number of patients discharged on NSAID/COX‐2 medications and renal‐dosed antibiotics in both the pre‐ and postintervention groups. Physicians prescribed NSAID/COX‐2 medications in 13.5% of patients preintervention and in 10.6% postintervention (P = .10). Overall, 12% of patients were discharged on a NSAID/COX‐2 medication. Reporting of estimated GFR did not have a significant effect on correct dosing of antibiotics at discharge (P = .81). Overall, 40% of renal‐dosed antibiotics were dosed incorrectly at the time of discharge.
DISCUSSION
This study has confirmed the findings of other investigators that significant CKD is underdiagnosed by physicians, especially in elderly patients with creatinine values within the normal laboratory range.13, 14 Investigators have demonstrated improved documentation of CKD with reporting of creatinine clearance and other simple educational interventions in an outpatient setting.13 In this study, reporting of estimated GFR did result in a significantly higher rate of recognition, but the overall rate was still very low in both groups (3.9%‐12.6%).
Although physician recognition of CKD did increase with the reporting of estimated GFR, this study found no significant impact on prescribing behaviors. Previous studies have shown an association between documentation of specific diagnoses and appropriate physician management.15, 18 However, the current data suggest that simply reporting GFR and increasing physician recognition of CKD may not lead to a significant decrease in medication dosing errors and that more extensive educational measures may be required.
Hospitalist physicians are increasingly serving as the primary caregivers for an aging population of hospitalized patients, and it is imperative that physicians recognize decreased GFR in elderly patients. Clearly, medication dosing errors are occurring in these patients, increasing the risk of adverse drug reactions.19 Elderly patients with renal impairment are also at increased risk of ARF while hospitalized.9, 10 Recognition of CKD by inpatient physicians identifies those patients who require preventive measures including maintenance of adequate hydration and avoidance of hypotension and nephrotoxic agents. Prevention of ARF in these patients has important clinical implications, as the mortality of patients is higher for elderly patients who develop hospital‐acquired ARF than for those presenting with community‐acquired ARF.20 Development of ARF has also been shown to increase length of hospitalization.21 Hospitalist physicians can also use the period of hospitalization as an opportunity to identify patients at risk of progressive CKD and in need of close follow‐up and possible referral to a nephrologist.
This study had several limitations. It was performed at a single institution, and therefore results may not be generalizable to all medical centers. The primary outcome of CKD documentation is an imperfect measure of recognition. The fact that chart documentation of CKD increased following the intervention suggests that documentation is associated with recognition, although it may be an underestimate. The effects of reporting estimated GFR on other secondary outcomes, including dosing of other medications, prevention of ARF, and length of hospital stay were not examined and deserve further investigation. The C‐G equation was chosen to calculate estimated GFR. There may be some advantage to using the Modification of Diet in Renal Disease equation as an alternative, but it is unclear if this is true in elderly female patients, who made up most of our study population.2225 Although using a prediction equation is clearly superior to using creatinine measurement solely to assess renal function in patients, further study is needed to identify the most accurate and effective formula for calculating estimated GFR in elderly patients.
The low rate of recognition of CKD by physicians found in this and other studies demonstrates the strong need for improvement in this area. Low recognition of CKD and a high rate of medication dosing errors despite reporting of the estimated GFR suggest that simply reporting GFR in addition to creatinine level is not sufficient. Further research is indicated to identify pragmatic educational tools and feedback mechanisms that effectively improve inpatient physician recognition of CKD and decrease medication dosing errors in elderly hospitalized patients.
Acknowledgements
The authors thank Christina Bennett for her assistance with data collection and Brian Waterman, MPH, of Waterman Research LLC, St. Louis, Missouri, for his assistance with statistical analyses.
- Prevalence of chronic kidney disease and decreased kidney function in the adult US population: Third National Health and Nutrition Examination Survey.Am J Kidney Dis.2003;41:1–12. , , , , .
- Anemia: an early complication of chronic renal insufficiencyAm J Kidney Dis.2001;38:803–812. , , , et al.
- Mild renal insufficiency is associated with increased cardiovascular mortality: The Hoorn StudyKidney Int.2002;62:1402–1407. , , , et al.
- Chronic kidney disease and the risks of death, cardiovascular events, and hospitalization.N Engl J Med.2004;351:1296–1305. , , , , .
- The timing of specialist evaluation in chronic kidney disease and mortalityAnn Intern Med.2002;137:479‐486. , , , et al.
- The effect of intensive treatment of diabetes on the development and progression of long‐term complications in insulin‐dependent diabetes mellitus.The Diabetes Control and Complications Trial Research Group.N Engl J Med.1993;329:977–986.
- A more accurate method to estimate glomerular filtration rate from serum creatinine: a new prediction equation. Modification of Diet in Renal Disease Study Group.Ann Intern Med.1999;130:461–470. , , , , , .
- Calculation of the estimated creatinine clearance in avoiding drug dosing errors in the older patientAm J Med Sci.2001;322:133–136. , , .
- Acute renal failure in hospitalized patients: part I.Ann Pharmacother.2002;36:1261–1267. , .
- Acute renal failure in hospitalized patients: part II.Ann Pharmacother.2002;36:1430–1442. , .
- K/DOQI clinical practice guidelines for chronic kidney disease: evaluation, classification, and stratification.Am J Kid Dis.2002;39:S1–266. .
- An analysis of discharge drug prescribing amongst elderly patients with renal impairmentPostgrad Med J.1998;74:420–422. , .
- Detection of chronic kidney disease with laboratory reporting of estimated glomerular filtration rate and an educational programArch Intern Med.2004;164:1788–1792. , , , et al.
- Screening for renal disease using serum creatinine: who are we missing?Nephrol Dial Transplant.2001;16:1042–1046. , , , .
- Early detection and treatment of renal disease in hospitalized diabetic and hypertensive patients: important differences between practice and published guidelines.Am J Kidney Dis.1997;29:368–375. , , , .
- Compliance with dosing guidelines in patients with chronic kidney diseaseAnn Pharmacother.2004;38:853–858. , , , .
- Evaluation of dosage adjustment in patients with renal impairmentIntern Med J.2003;33:10–13. , , , , .
- Current physician screening and treatment of hypercholesterolemic patients.Am J Med Sci.1993;306:124–128. , , .
- Concealed renal insufficiency and adverse drug reactions in elderly hospitalized patients.Arch Intern Med.2005;165:790–795. , , , , , .
- Prognosis of ARF in hospitalized elderly patients.Am J Kidney Dis2004;44:410–409. , , , .
- Epidemiology of acute renal failure: a prospective, multicenter, community‐based study. Madrid Acute Renal Failure Study Group.Kidney Int.1996;50:811–818. , .
- Performance of the modification of diet in renal disease and Cockcroft‐Gault equations in the estimation of GFR in health and in chronic kidney disease.J Am Soc Nephrol.2005;16:459–466. , , , , .
- Predictive performance of the modification of diet in renal disease and Cockcroft‐Gault equations for estimating renal function.J Am Soc Nephrol.2005;16:763–773. , , , , .
- Can creatinine clearance be accurately predicted by formulae in octogenarian in‐patients?QJM.2004;97:281–287. , , , , , .
- Estimation of glomerular filtration rate in older patients with chronic renal insufficiency: is the modification of diet in renal disease formula an improvement?J Am Geriatr Soc.2003;51:1012–1017. , , , , , .
Chronic kidney disease is increasingly recognized as a significant public health issue, especially as our population ages. In the United States, it is estimated that 19.2 million individuals have chronic kidney disease (CKD), with an increasing prevalence in the elderly.1 CKD is associated with a higher mortality rate, as well as an increased risk of having several comorbidities, including anemia, coronary artery disease, and congestive heart failure.24 Early recognition, intervention, and management of patients with CKD by physicians has been shown to slow progression of disease and decrease complications.57 In the hospital setting, patients with CKD are at increased risk of medication dosing errors and acute renal failure (ARF).810
Serum creatinine is the most commonly used laboratory marker for assessing renal function. However, creatinine level is an imprecise measure of overall renal function, especially in older patients. The most recent National Kidney Foundation/ Kidney Disease Outcomes Quality Initiative (K/DOQI) guidelines recommend laboratory reporting of a calculated estimate of GFR.11 Equations used to calculate estimated GFR in adults, including the Cockcroft‐Gault (C‐G) equation, have been shown to provide an estimate of renal function, which can be used to clinically stratify varying levels of impaired renal function.11 Several studies have demonstrated that recognition of CKD by physicians is low in various clinical settings, especially in elderly patients.1215 Compliance with renal‐dose medication guidelines has also frequently been noted to be poor.16, 17
The investigators conducted a chart review study before and after reporting of estimated GFR to physicians in a hospital setting to assess the effect on physician recognition of CKD, the primary outcome. Secondary outcomes included the effect of reporting GFR on physician prescribing behaviors at the time of hospital discharge, including dosing of renal‐dosed antibiotics and use of nonsteroidal anti‐inflammatory (NSAID) and cyclooxygenase type 2 inhibitor (COX‐2) medications.
METHODS
This study was a retrospective chart review, with a prospective chart review as a comparison. Patients selected were admitted to a general medical floor in a 900‐bed academic medical center over the 2 years from 2002 to 2004. Computerized databases of laboratory values and weights obtained during hospitalization were used to select patients who fulfilled the following criteria: age > 65 years, all creatinine values during hospitalization < 1.6 mg/dL, and calculated estimated creatinine clearance (CrCl) < 60 mL/min using the Cockcroft‐Gault (C‐G) formula. The C‐G equation was developed for estimating CrCl and has also been extensively tested as a predictor of GFR. K/DOQI guidelines identify the C‐G equation as the most frequently used equation to estimate GFR in adults.11 To ensure steady‐state renal function, patients were excluded if creatinine varied by more than 0.4 mg/dL during their hospitalization. Based on an anticipated CKD recognition rate of 24%,13 our study sample size was selected to detect a 13% difference in the primary end point between the pre‐ and postintervention groups with 80% power. The study was approved by the institutional review board of the medical school.
Patient charts were reviewed with data obtained from the medical record, including physician notes, discharge summaries, orders, medication lists, and discharge prescriptions. Physician recognition was defined by documentation of CKD, calculated CrCl, or GFR in the physician notes or discharge summary. Charts were reviewed for diagnosis of hypertension (HTN) or diabetes (DM), and discharge medications including NSAID and COX‐2 medications and use and correct dosing of antibiotics requiring dose adjustment in patients with decreased GFR. Aspirin was not included as an NSAID.
For the prospective chart review portion, patients were selected at the time of admission on the basis of the same criteria. A notification was placed in the chart prominently listing the patient's estimated GFR calculated using the C‐G equation. Also included was a list of the stages of chronic renal disease based on the most recent K/DOQI guidelines11 and recommendations on dosing of select renal‐dosed antibiotics. Patients were again excluded if creatinine varied more than 0.4 mg/dL during their hospitalization.
Data Analysis
For statistical analysis, the association between recognition of CKD and the chart intervention, unadjusted for covariates, was evaluated using a contingency table. Additionally, the associations between recognition of CKD and other patient covariatessex, diabetes, hypertension, estimated GFRwere analyzed both individually and jointly. For individual covariate analysis, Fisher's exact test was used in all tests for association. For joint analysis, a set of relevant covariates was determined by stepwise logistic regression. The association of CKD recognition and the intervention was again analyzed using logistic regression while adjusting for this set of relevant covariates.
Finally, an analysis of appropriate medication prescribing at the time of hospital discharge was carried out to assess the effect of reporting estimated GFR. Prescription of NSAID or COX‐2 medications and correct dosing of renal‐dosed antibiotics at discharge were analyzed separately. As in the exploratory covariate analysis, Fisher's exact test for association was used.
RESULTS
Study Population
Characteristics of the study cohort are summarized in Table 1. The pre‐ and postintervention groups had 260 and 198 patients, respectively. Most were female. Average age, serum creatinine, and estimated GFR were similar in both groups.
Characteristics | Preintervention | Postintervention |
---|---|---|
| ||
Total number | 260 | 198 |
Age (years) | 81.1 6.6 | 82 6.8 |
Sex (female) | 199 (76.5) | 168 (84.8) |
Serum creatinine (mg/dL) | 0.98 0.2 | 0.9 0.2 |
C‐G CrCl (mL/min) | 41.5 10.2 | 41.4 9.3 |
DM | 58 (22.3) | 63 (31.8) |
HTN | 190 (73.1) | 152 (76.7) |
Physician recognition of CKD | 10 (3.9) | 25 (12.6) |
NSAID or COX‐2 prescribed at discharge | 35 (13.5) | 21 (10.6) |
Antibiotic requiring renal‐dose adjustment prescribed at discharge | 50 (19.2) | 29 (14.2) |
Correct dosing of renal‐dosed antibiotic at discharge* | 28 (56.0) | 18 (62.1) |
Effect of Intervention on Recognition of CKD
Table 1 shows the number of patients recognized by physicians as having CKD in both groups. Prior to the study intervention, CKD was recognized in only 10 of 260 patients (3.9%), and following the intervention, rates increased to 25 of 198 patients (12.6%; P .001).
The results of the stepwise logistic regression of the covariates on CKD recognition showed that CKD recognition was modeled best with diabetes and lower estimated GFR. This corresponded well with the results of the individual covariate analyses. Thus, the primary outcome was again modeled by the intervention and the covariates diabetes and lower estimated GFR. With the addition of the covariates, the intervention was still a significant predictor of CKD recognition (P = .001), with an odds ratio of 4.07 (95% CI = (1.83,9.01)).
Effect of Intervention on Medication Prescribed at Hospital Discharge
Table 1 shows the number of patients discharged on NSAID/COX‐2 medications and renal‐dosed antibiotics in both the pre‐ and postintervention groups. Physicians prescribed NSAID/COX‐2 medications in 13.5% of patients preintervention and in 10.6% postintervention (P = .10). Overall, 12% of patients were discharged on a NSAID/COX‐2 medication. Reporting of estimated GFR did not have a significant effect on correct dosing of antibiotics at discharge (P = .81). Overall, 40% of renal‐dosed antibiotics were dosed incorrectly at the time of discharge.
DISCUSSION
This study has confirmed the findings of other investigators that significant CKD is underdiagnosed by physicians, especially in elderly patients with creatinine values within the normal laboratory range.13, 14 Investigators have demonstrated improved documentation of CKD with reporting of creatinine clearance and other simple educational interventions in an outpatient setting.13 In this study, reporting of estimated GFR did result in a significantly higher rate of recognition, but the overall rate was still very low in both groups (3.9%‐12.6%).
Although physician recognition of CKD did increase with the reporting of estimated GFR, this study found no significant impact on prescribing behaviors. Previous studies have shown an association between documentation of specific diagnoses and appropriate physician management.15, 18 However, the current data suggest that simply reporting GFR and increasing physician recognition of CKD may not lead to a significant decrease in medication dosing errors and that more extensive educational measures may be required.
Hospitalist physicians are increasingly serving as the primary caregivers for an aging population of hospitalized patients, and it is imperative that physicians recognize decreased GFR in elderly patients. Clearly, medication dosing errors are occurring in these patients, increasing the risk of adverse drug reactions.19 Elderly patients with renal impairment are also at increased risk of ARF while hospitalized.9, 10 Recognition of CKD by inpatient physicians identifies those patients who require preventive measures including maintenance of adequate hydration and avoidance of hypotension and nephrotoxic agents. Prevention of ARF in these patients has important clinical implications, as the mortality of patients is higher for elderly patients who develop hospital‐acquired ARF than for those presenting with community‐acquired ARF.20 Development of ARF has also been shown to increase length of hospitalization.21 Hospitalist physicians can also use the period of hospitalization as an opportunity to identify patients at risk of progressive CKD and in need of close follow‐up and possible referral to a nephrologist.
This study had several limitations. It was performed at a single institution, and therefore results may not be generalizable to all medical centers. The primary outcome of CKD documentation is an imperfect measure of recognition. The fact that chart documentation of CKD increased following the intervention suggests that documentation is associated with recognition, although it may be an underestimate. The effects of reporting estimated GFR on other secondary outcomes, including dosing of other medications, prevention of ARF, and length of hospital stay were not examined and deserve further investigation. The C‐G equation was chosen to calculate estimated GFR. There may be some advantage to using the Modification of Diet in Renal Disease equation as an alternative, but it is unclear if this is true in elderly female patients, who made up most of our study population.2225 Although using a prediction equation is clearly superior to using creatinine measurement solely to assess renal function in patients, further study is needed to identify the most accurate and effective formula for calculating estimated GFR in elderly patients.
The low rate of recognition of CKD by physicians found in this and other studies demonstrates the strong need for improvement in this area. Low recognition of CKD and a high rate of medication dosing errors despite reporting of the estimated GFR suggest that simply reporting GFR in addition to creatinine level is not sufficient. Further research is indicated to identify pragmatic educational tools and feedback mechanisms that effectively improve inpatient physician recognition of CKD and decrease medication dosing errors in elderly hospitalized patients.
Acknowledgements
The authors thank Christina Bennett for her assistance with data collection and Brian Waterman, MPH, of Waterman Research LLC, St. Louis, Missouri, for his assistance with statistical analyses.
Chronic kidney disease is increasingly recognized as a significant public health issue, especially as our population ages. In the United States, it is estimated that 19.2 million individuals have chronic kidney disease (CKD), with an increasing prevalence in the elderly.1 CKD is associated with a higher mortality rate, as well as an increased risk of having several comorbidities, including anemia, coronary artery disease, and congestive heart failure.24 Early recognition, intervention, and management of patients with CKD by physicians has been shown to slow progression of disease and decrease complications.57 In the hospital setting, patients with CKD are at increased risk of medication dosing errors and acute renal failure (ARF).810
Serum creatinine is the most commonly used laboratory marker for assessing renal function. However, creatinine level is an imprecise measure of overall renal function, especially in older patients. The most recent National Kidney Foundation/ Kidney Disease Outcomes Quality Initiative (K/DOQI) guidelines recommend laboratory reporting of a calculated estimate of GFR.11 Equations used to calculate estimated GFR in adults, including the Cockcroft‐Gault (C‐G) equation, have been shown to provide an estimate of renal function, which can be used to clinically stratify varying levels of impaired renal function.11 Several studies have demonstrated that recognition of CKD by physicians is low in various clinical settings, especially in elderly patients.1215 Compliance with renal‐dose medication guidelines has also frequently been noted to be poor.16, 17
The investigators conducted a chart review study before and after reporting of estimated GFR to physicians in a hospital setting to assess the effect on physician recognition of CKD, the primary outcome. Secondary outcomes included the effect of reporting GFR on physician prescribing behaviors at the time of hospital discharge, including dosing of renal‐dosed antibiotics and use of nonsteroidal anti‐inflammatory (NSAID) and cyclooxygenase type 2 inhibitor (COX‐2) medications.
METHODS
This study was a retrospective chart review, with a prospective chart review as a comparison. Patients selected were admitted to a general medical floor in a 900‐bed academic medical center over the 2 years from 2002 to 2004. Computerized databases of laboratory values and weights obtained during hospitalization were used to select patients who fulfilled the following criteria: age > 65 years, all creatinine values during hospitalization < 1.6 mg/dL, and calculated estimated creatinine clearance (CrCl) < 60 mL/min using the Cockcroft‐Gault (C‐G) formula. The C‐G equation was developed for estimating CrCl and has also been extensively tested as a predictor of GFR. K/DOQI guidelines identify the C‐G equation as the most frequently used equation to estimate GFR in adults.11 To ensure steady‐state renal function, patients were excluded if creatinine varied by more than 0.4 mg/dL during their hospitalization. Based on an anticipated CKD recognition rate of 24%,13 our study sample size was selected to detect a 13% difference in the primary end point between the pre‐ and postintervention groups with 80% power. The study was approved by the institutional review board of the medical school.
Patient charts were reviewed with data obtained from the medical record, including physician notes, discharge summaries, orders, medication lists, and discharge prescriptions. Physician recognition was defined by documentation of CKD, calculated CrCl, or GFR in the physician notes or discharge summary. Charts were reviewed for diagnosis of hypertension (HTN) or diabetes (DM), and discharge medications including NSAID and COX‐2 medications and use and correct dosing of antibiotics requiring dose adjustment in patients with decreased GFR. Aspirin was not included as an NSAID.
For the prospective chart review portion, patients were selected at the time of admission on the basis of the same criteria. A notification was placed in the chart prominently listing the patient's estimated GFR calculated using the C‐G equation. Also included was a list of the stages of chronic renal disease based on the most recent K/DOQI guidelines11 and recommendations on dosing of select renal‐dosed antibiotics. Patients were again excluded if creatinine varied more than 0.4 mg/dL during their hospitalization.
Data Analysis
For statistical analysis, the association between recognition of CKD and the chart intervention, unadjusted for covariates, was evaluated using a contingency table. Additionally, the associations between recognition of CKD and other patient covariatessex, diabetes, hypertension, estimated GFRwere analyzed both individually and jointly. For individual covariate analysis, Fisher's exact test was used in all tests for association. For joint analysis, a set of relevant covariates was determined by stepwise logistic regression. The association of CKD recognition and the intervention was again analyzed using logistic regression while adjusting for this set of relevant covariates.
Finally, an analysis of appropriate medication prescribing at the time of hospital discharge was carried out to assess the effect of reporting estimated GFR. Prescription of NSAID or COX‐2 medications and correct dosing of renal‐dosed antibiotics at discharge were analyzed separately. As in the exploratory covariate analysis, Fisher's exact test for association was used.
RESULTS
Study Population
Characteristics of the study cohort are summarized in Table 1. The pre‐ and postintervention groups had 260 and 198 patients, respectively. Most were female. Average age, serum creatinine, and estimated GFR were similar in both groups.
Characteristics | Preintervention | Postintervention |
---|---|---|
| ||
Total number | 260 | 198 |
Age (years) | 81.1 6.6 | 82 6.8 |
Sex (female) | 199 (76.5) | 168 (84.8) |
Serum creatinine (mg/dL) | 0.98 0.2 | 0.9 0.2 |
C‐G CrCl (mL/min) | 41.5 10.2 | 41.4 9.3 |
DM | 58 (22.3) | 63 (31.8) |
HTN | 190 (73.1) | 152 (76.7) |
Physician recognition of CKD | 10 (3.9) | 25 (12.6) |
NSAID or COX‐2 prescribed at discharge | 35 (13.5) | 21 (10.6) |
Antibiotic requiring renal‐dose adjustment prescribed at discharge | 50 (19.2) | 29 (14.2) |
Correct dosing of renal‐dosed antibiotic at discharge* | 28 (56.0) | 18 (62.1) |
Effect of Intervention on Recognition of CKD
Table 1 shows the number of patients recognized by physicians as having CKD in both groups. Prior to the study intervention, CKD was recognized in only 10 of 260 patients (3.9%), and following the intervention, rates increased to 25 of 198 patients (12.6%; P .001).
The results of the stepwise logistic regression of the covariates on CKD recognition showed that CKD recognition was modeled best with diabetes and lower estimated GFR. This corresponded well with the results of the individual covariate analyses. Thus, the primary outcome was again modeled by the intervention and the covariates diabetes and lower estimated GFR. With the addition of the covariates, the intervention was still a significant predictor of CKD recognition (P = .001), with an odds ratio of 4.07 (95% CI = (1.83,9.01)).
Effect of Intervention on Medication Prescribed at Hospital Discharge
Table 1 shows the number of patients discharged on NSAID/COX‐2 medications and renal‐dosed antibiotics in both the pre‐ and postintervention groups. Physicians prescribed NSAID/COX‐2 medications in 13.5% of patients preintervention and in 10.6% postintervention (P = .10). Overall, 12% of patients were discharged on a NSAID/COX‐2 medication. Reporting of estimated GFR did not have a significant effect on correct dosing of antibiotics at discharge (P = .81). Overall, 40% of renal‐dosed antibiotics were dosed incorrectly at the time of discharge.
DISCUSSION
This study has confirmed the findings of other investigators that significant CKD is underdiagnosed by physicians, especially in elderly patients with creatinine values within the normal laboratory range.13, 14 Investigators have demonstrated improved documentation of CKD with reporting of creatinine clearance and other simple educational interventions in an outpatient setting.13 In this study, reporting of estimated GFR did result in a significantly higher rate of recognition, but the overall rate was still very low in both groups (3.9%‐12.6%).
Although physician recognition of CKD did increase with the reporting of estimated GFR, this study found no significant impact on prescribing behaviors. Previous studies have shown an association between documentation of specific diagnoses and appropriate physician management.15, 18 However, the current data suggest that simply reporting GFR and increasing physician recognition of CKD may not lead to a significant decrease in medication dosing errors and that more extensive educational measures may be required.
Hospitalist physicians are increasingly serving as the primary caregivers for an aging population of hospitalized patients, and it is imperative that physicians recognize decreased GFR in elderly patients. Clearly, medication dosing errors are occurring in these patients, increasing the risk of adverse drug reactions.19 Elderly patients with renal impairment are also at increased risk of ARF while hospitalized.9, 10 Recognition of CKD by inpatient physicians identifies those patients who require preventive measures including maintenance of adequate hydration and avoidance of hypotension and nephrotoxic agents. Prevention of ARF in these patients has important clinical implications, as the mortality of patients is higher for elderly patients who develop hospital‐acquired ARF than for those presenting with community‐acquired ARF.20 Development of ARF has also been shown to increase length of hospitalization.21 Hospitalist physicians can also use the period of hospitalization as an opportunity to identify patients at risk of progressive CKD and in need of close follow‐up and possible referral to a nephrologist.
This study had several limitations. It was performed at a single institution, and therefore results may not be generalizable to all medical centers. The primary outcome of CKD documentation is an imperfect measure of recognition. The fact that chart documentation of CKD increased following the intervention suggests that documentation is associated with recognition, although it may be an underestimate. The effects of reporting estimated GFR on other secondary outcomes, including dosing of other medications, prevention of ARF, and length of hospital stay were not examined and deserve further investigation. The C‐G equation was chosen to calculate estimated GFR. There may be some advantage to using the Modification of Diet in Renal Disease equation as an alternative, but it is unclear if this is true in elderly female patients, who made up most of our study population.2225 Although using a prediction equation is clearly superior to using creatinine measurement solely to assess renal function in patients, further study is needed to identify the most accurate and effective formula for calculating estimated GFR in elderly patients.
The low rate of recognition of CKD by physicians found in this and other studies demonstrates the strong need for improvement in this area. Low recognition of CKD and a high rate of medication dosing errors despite reporting of the estimated GFR suggest that simply reporting GFR in addition to creatinine level is not sufficient. Further research is indicated to identify pragmatic educational tools and feedback mechanisms that effectively improve inpatient physician recognition of CKD and decrease medication dosing errors in elderly hospitalized patients.
Acknowledgements
The authors thank Christina Bennett for her assistance with data collection and Brian Waterman, MPH, of Waterman Research LLC, St. Louis, Missouri, for his assistance with statistical analyses.
- Prevalence of chronic kidney disease and decreased kidney function in the adult US population: Third National Health and Nutrition Examination Survey.Am J Kidney Dis.2003;41:1–12. , , , , .
- Anemia: an early complication of chronic renal insufficiencyAm J Kidney Dis.2001;38:803–812. , , , et al.
- Mild renal insufficiency is associated with increased cardiovascular mortality: The Hoorn StudyKidney Int.2002;62:1402–1407. , , , et al.
- Chronic kidney disease and the risks of death, cardiovascular events, and hospitalization.N Engl J Med.2004;351:1296–1305. , , , , .
- The timing of specialist evaluation in chronic kidney disease and mortalityAnn Intern Med.2002;137:479‐486. , , , et al.
- The effect of intensive treatment of diabetes on the development and progression of long‐term complications in insulin‐dependent diabetes mellitus.The Diabetes Control and Complications Trial Research Group.N Engl J Med.1993;329:977–986.
- A more accurate method to estimate glomerular filtration rate from serum creatinine: a new prediction equation. Modification of Diet in Renal Disease Study Group.Ann Intern Med.1999;130:461–470. , , , , , .
- Calculation of the estimated creatinine clearance in avoiding drug dosing errors in the older patientAm J Med Sci.2001;322:133–136. , , .
- Acute renal failure in hospitalized patients: part I.Ann Pharmacother.2002;36:1261–1267. , .
- Acute renal failure in hospitalized patients: part II.Ann Pharmacother.2002;36:1430–1442. , .
- K/DOQI clinical practice guidelines for chronic kidney disease: evaluation, classification, and stratification.Am J Kid Dis.2002;39:S1–266. .
- An analysis of discharge drug prescribing amongst elderly patients with renal impairmentPostgrad Med J.1998;74:420–422. , .
- Detection of chronic kidney disease with laboratory reporting of estimated glomerular filtration rate and an educational programArch Intern Med.2004;164:1788–1792. , , , et al.
- Screening for renal disease using serum creatinine: who are we missing?Nephrol Dial Transplant.2001;16:1042–1046. , , , .
- Early detection and treatment of renal disease in hospitalized diabetic and hypertensive patients: important differences between practice and published guidelines.Am J Kidney Dis.1997;29:368–375. , , , .
- Compliance with dosing guidelines in patients with chronic kidney diseaseAnn Pharmacother.2004;38:853–858. , , , .
- Evaluation of dosage adjustment in patients with renal impairmentIntern Med J.2003;33:10–13. , , , , .
- Current physician screening and treatment of hypercholesterolemic patients.Am J Med Sci.1993;306:124–128. , , .
- Concealed renal insufficiency and adverse drug reactions in elderly hospitalized patients.Arch Intern Med.2005;165:790–795. , , , , , .
- Prognosis of ARF in hospitalized elderly patients.Am J Kidney Dis2004;44:410–409. , , , .
- Epidemiology of acute renal failure: a prospective, multicenter, community‐based study. Madrid Acute Renal Failure Study Group.Kidney Int.1996;50:811–818. , .
- Performance of the modification of diet in renal disease and Cockcroft‐Gault equations in the estimation of GFR in health and in chronic kidney disease.J Am Soc Nephrol.2005;16:459–466. , , , , .
- Predictive performance of the modification of diet in renal disease and Cockcroft‐Gault equations for estimating renal function.J Am Soc Nephrol.2005;16:763–773. , , , , .
- Can creatinine clearance be accurately predicted by formulae in octogenarian in‐patients?QJM.2004;97:281–287. , , , , , .
- Estimation of glomerular filtration rate in older patients with chronic renal insufficiency: is the modification of diet in renal disease formula an improvement?J Am Geriatr Soc.2003;51:1012–1017. , , , , , .
- Prevalence of chronic kidney disease and decreased kidney function in the adult US population: Third National Health and Nutrition Examination Survey.Am J Kidney Dis.2003;41:1–12. , , , , .
- Anemia: an early complication of chronic renal insufficiencyAm J Kidney Dis.2001;38:803–812. , , , et al.
- Mild renal insufficiency is associated with increased cardiovascular mortality: The Hoorn StudyKidney Int.2002;62:1402–1407. , , , et al.
- Chronic kidney disease and the risks of death, cardiovascular events, and hospitalization.N Engl J Med.2004;351:1296–1305. , , , , .
- The timing of specialist evaluation in chronic kidney disease and mortalityAnn Intern Med.2002;137:479‐486. , , , et al.
- The effect of intensive treatment of diabetes on the development and progression of long‐term complications in insulin‐dependent diabetes mellitus.The Diabetes Control and Complications Trial Research Group.N Engl J Med.1993;329:977–986.
- A more accurate method to estimate glomerular filtration rate from serum creatinine: a new prediction equation. Modification of Diet in Renal Disease Study Group.Ann Intern Med.1999;130:461–470. , , , , , .
- Calculation of the estimated creatinine clearance in avoiding drug dosing errors in the older patientAm J Med Sci.2001;322:133–136. , , .
- Acute renal failure in hospitalized patients: part I.Ann Pharmacother.2002;36:1261–1267. , .
- Acute renal failure in hospitalized patients: part II.Ann Pharmacother.2002;36:1430–1442. , .
- K/DOQI clinical practice guidelines for chronic kidney disease: evaluation, classification, and stratification.Am J Kid Dis.2002;39:S1–266. .
- An analysis of discharge drug prescribing amongst elderly patients with renal impairmentPostgrad Med J.1998;74:420–422. , .
- Detection of chronic kidney disease with laboratory reporting of estimated glomerular filtration rate and an educational programArch Intern Med.2004;164:1788–1792. , , , et al.
- Screening for renal disease using serum creatinine: who are we missing?Nephrol Dial Transplant.2001;16:1042–1046. , , , .
- Early detection and treatment of renal disease in hospitalized diabetic and hypertensive patients: important differences between practice and published guidelines.Am J Kidney Dis.1997;29:368–375. , , , .
- Compliance with dosing guidelines in patients with chronic kidney diseaseAnn Pharmacother.2004;38:853–858. , , , .
- Evaluation of dosage adjustment in patients with renal impairmentIntern Med J.2003;33:10–13. , , , , .
- Current physician screening and treatment of hypercholesterolemic patients.Am J Med Sci.1993;306:124–128. , , .
- Concealed renal insufficiency and adverse drug reactions in elderly hospitalized patients.Arch Intern Med.2005;165:790–795. , , , , , .
- Prognosis of ARF in hospitalized elderly patients.Am J Kidney Dis2004;44:410–409. , , , .
- Epidemiology of acute renal failure: a prospective, multicenter, community‐based study. Madrid Acute Renal Failure Study Group.Kidney Int.1996;50:811–818. , .
- Performance of the modification of diet in renal disease and Cockcroft‐Gault equations in the estimation of GFR in health and in chronic kidney disease.J Am Soc Nephrol.2005;16:459–466. , , , , .
- Predictive performance of the modification of diet in renal disease and Cockcroft‐Gault equations for estimating renal function.J Am Soc Nephrol.2005;16:763–773. , , , , .
- Can creatinine clearance be accurately predicted by formulae in octogenarian in‐patients?QJM.2004;97:281–287. , , , , , .
- Estimation of glomerular filtration rate in older patients with chronic renal insufficiency: is the modification of diet in renal disease formula an improvement?J Am Geriatr Soc.2003;51:1012–1017. , , , , , .
Copyright © 2007 Society of Hospital Medicine