User login
Accuracy of GoogleTranslate™
The population of patients in the US with limited English proficiency (LEP)those who speak English less than very well1is substantial and continues to grow.1, 2 Patients with LEP are at risk for lower quality health care overall than their English‐speaking counterparts.38 Professional in‐person interpreters greatly improve spoken communication and quality of care for these patients,4, 9 but their assistance is typically based on the clinical encounter. Particularly if interpreting by phone, interpreters are unlikely to be able to help with materials such as discharge instructions or information sheets meant for family members. Professional written translations of patient educational material help to bridge this gap, allowing clinicians to convey detailed written instructions to patients. However, professional translations must be prepared well in advance of any encounter and can only be used for easily anticipated problems.
The need to translate less common, patient‐specific instructions arises spontaneously in clinical practice, and formally prepared written translations are not useful in these situations. Online translation tools such as GoogleTranslate (available at
We conducted a pilot evaluation of an online translation tool as it relates to detailed, complex patient educational material. Our primary goal was to compare the accuracy of a Spanish translation generated by the online tool to that done by a professional agency. Our secondary goals were: 1) to assess whether sentence word length or complexity mediated the accuracy of GT; and 2) to lay the foundation for a more comprehensive study of the accuracy of online translation tools, with respect to patient educational material.
Methods
Translation Tool and Language Choice
We selected Google Translate (GT) since it is one of the more commonly used online translation tools and because Google is the most widely used search engine in the United States.13 GT uses statistical translation methodology to convert text, documents, and websites between languages; statistical translation involves the following three steps. First, the translation program recognizes a sentence to translate. Second, it compares the words and phrases within that sentence to the billions of words in its library (drawn from bilingual professionally translated documents, such as United Nations proceedings). Third, it uses this comparison to generate a translation combining the words and phrases deemed most equivalent between the source sentence and the target language. If there are multiple sentences, the program recognizes and translates each independently. As the body of bilingual work grows, the program learns and refines its rules automatically.14 In contrast, in rule‐based translation, a program would use manually prespecified rules regarding word choice and grammar to generate a translation.15 We assessed GT's accuracy translating from English to Spanish because Spanish is the predominant non‐English language spoken in the US.1
Document Selection and Preparation
We selected the instruction manual regarding warfarin use prepared by the Agency for Healthcare Research and Quality (AHRQ) for this accuracy evaluation. We selected this manual,16 written at a 6th grade reading level, because a professional Spanish translation was available (completed by ASET International Service, LLC, before and independently of this study), and because patient educational material regarding warfarin has been associated with fewer bleeding events.17 We downloaded the English document on October 19, 2009 and used the GT website to translate it en bloc. We then copied the resulting Spanish output into a text file. The English document and the professional Spanish translation (downloaded the same day) were both converted into text files in the same manner.
Grading Methodology
We scored the translation chosen using both manual and automated evaluation techniques. These techniques are widely used in the machine translation literature and are explained below.
Manual Evaluation: Evaluators, Domains, Scoring
We recruited three nonclinician, bilingual, nativeSpanish‐speaking research assistants as evaluators. The evaluators were all college educated with a Bachelor's degree or higher and were of Mexican, Nicaraguan, and Guatemalan ancestry. Each evaluator received a brief orientation regarding the project, as well as an explanation of the scores, and then proceeded to the blinded evaluation independently.
We asked evaluators to score sentences on Likert scales along five primary domains: fluency, adequacy, meaning, severity, and preference. Fluency and adequacy are well accepted components of machine translation evaluation,18 with fluency being an assessment of grammar and readability ranging from 5 (Perfect fluency; like reading a newspaper) to 1 (No fluency; no appreciable grammar, not understandable) and adequacy being an assessment of information preservation ranging from 5 (100% of information conveyed from the original) to 1 (0% of information conveyed from the original). Given that a sentence can be highly adequate but drastically change the connotation and intent of the sentence (eg, a sentence that contains 75% of the correct words but changes a sentence from take this medication twice a day to take this medication once every two days), we asked evaluators to assess meaning, a measure of connotation and intent maintenance, with scores ranging from 5 (Same meaning as original) to 1 (Totally different meaning from the original).19 Evaluators also assessed severity, a new measure of potential harm if a given sentence was assessed as having errors of any kind, ranging from 5 (Error, no effect on patient care) to 1 (Error, dangerous to patient) with an additional option of N/A (Sentence basically accurate). Finally, evaluators rated a blinded preference (also a new measure) for either of two translated sentences, ranging from Strongly prefer translation #1 to Strongly prefer translation #2. The order of the sentences was random (eg, sometimes the professional translation was first and sometimes the GT translation was). We subsequently converted this to preference for the professional translation, ranging from 5 (Strongly prefer the professional translation) to 1 (Strongly prefer the GT translation) in order to standardize the responses (Figures 1 and 2).
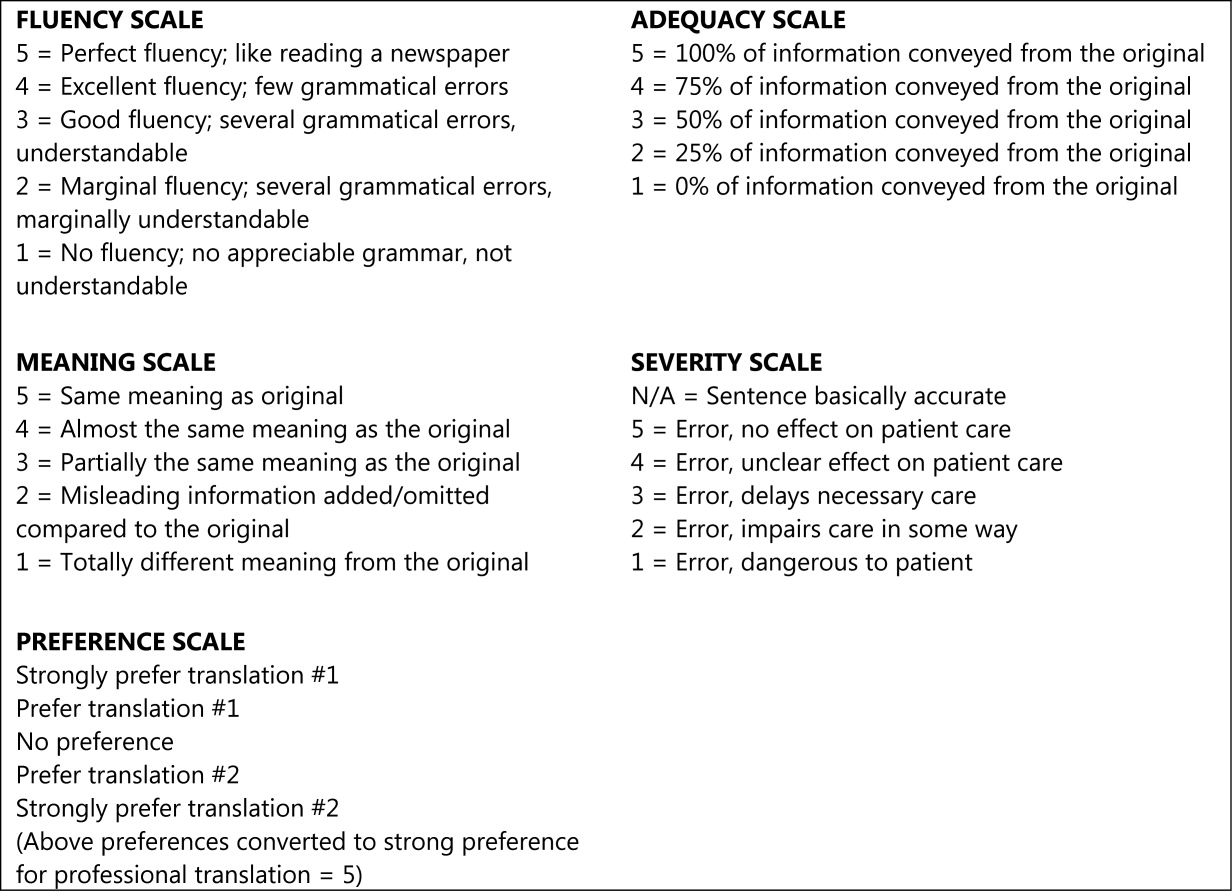
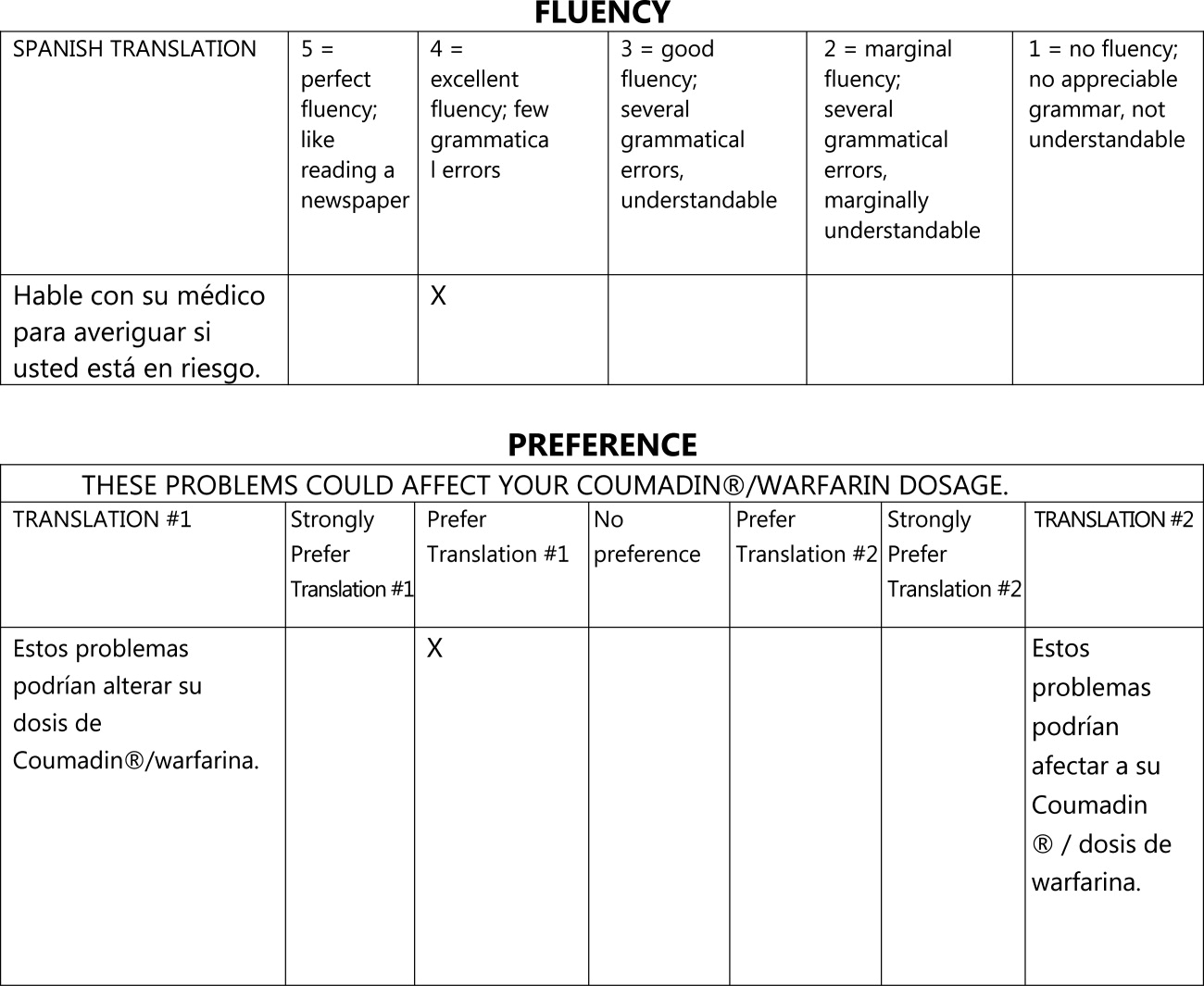
The overall flow of the study is given in Figure 3. Each evaluator initially scored 20 sentences translated by GT and 10 sentences translated professionally along the first four domains. All 30 of these sentences were randomly selected from the original, 263‐sentence pamphlet. For fluency, evaluators had access only to the translated sentence to be scored; for adequacy, meaning, and severity, they had access to both the translated sentence and the original English sentence. Ten of the 30 sentences were further selected randomly for scoring on the preference domain. For these 10 sentences, evaluators compared the GT and professional translations of the same sentence (with the original English sentence available as a reference) and indicated a preference, for any reason, for one translation or the other. Evaluators were blinded to the technique of translation (GT or professional) for all scored sentences and domains. We chose twice as many sentences from the GT preparations for the first four domains to maximize measurements for the translation technology we were evaluating, with the smaller number of professional translations serving as controls.
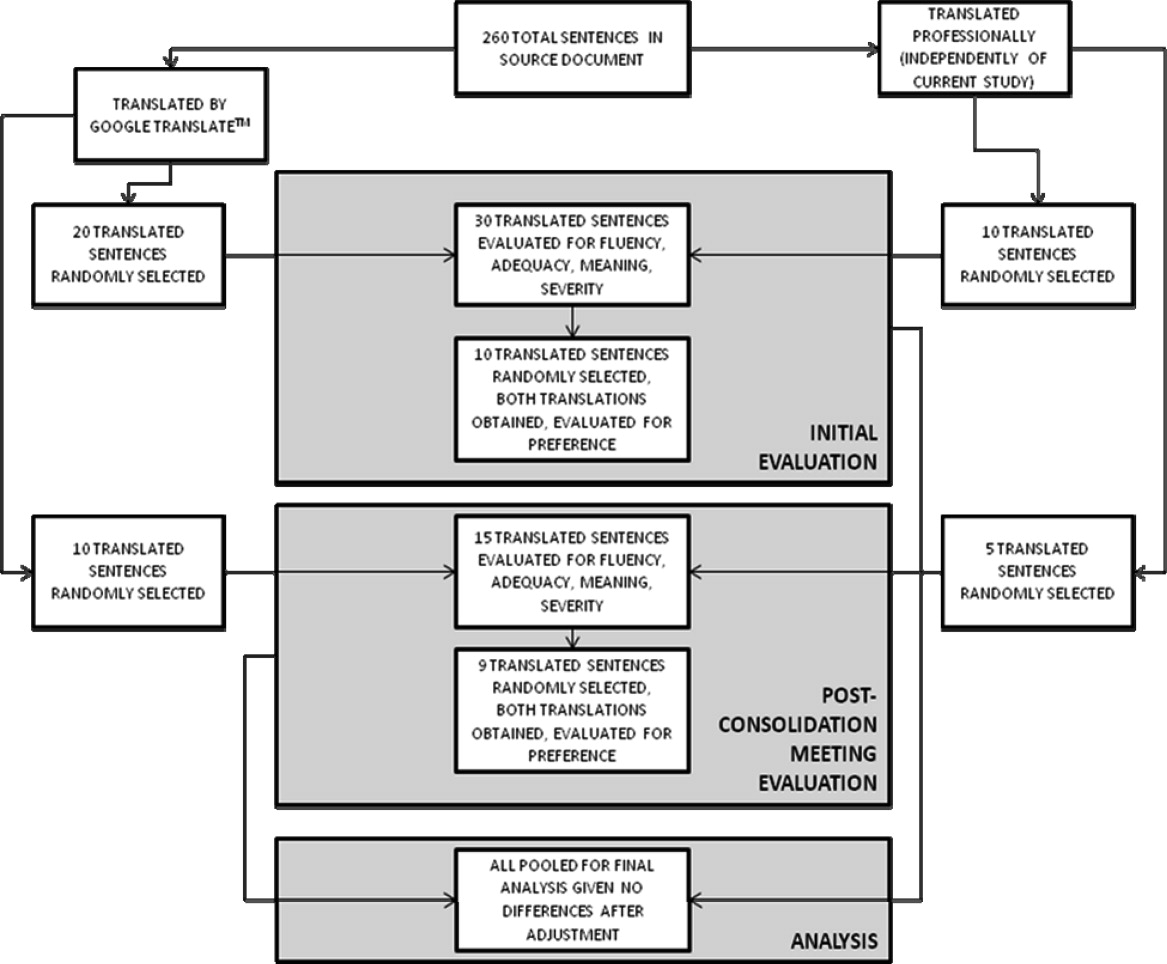
After scoring the first 30 sentences, evaluators met with one of the authors (R.R.K.) to discuss and consolidate their approach to scoring. They then scored an additional 10 GT‐translated sentences and 5 professionally translated sentences for the first four domains, and 9 of these 15 sentences for preference, to see if the meeting changed their scoring approach. These sentences were selected randomly from the original, 263‐sentence pamphlet, excluding the 30 evaluated in the previous step.
Automated Machine Translation Evaluation
Machine translation researchers have developed automated measures allowing the rapid and inexpensive scoring and rescoring of translations. These automated measures supplement more time‐ and resource‐intensive manual evaluations. The automated measures are based upon how well the translation compares to one or, ideally, multiple professionally prepared reference translations. They correlate well with human judgments on the domains above, especially when multiple reference translations are used (increasing the number of reference translations increases the variability allowed for words and phrases in the machine translation, improving the likelihood that differences in score are related to differences in quality rather than differences in translator preference).20 For this study, we used Metric for Evaluation of Translation with Explicit Ordering (METEOR), a machine translation evaluation system that allows additional flexibility for the machine translation in terms of grading individual sentences and being sensitive to synonyms, word stemming, and word order.21 We obtained a METEOR score for each of the GT‐translated sentences using the professional translation as our reference, and assessed correlation between this automated measure and the manual evaluations for the GT sentences, with the aim of assessing the feasibility of using METEOR in future work on patient educational material translation.
Outcomes and Statistical Analysis
We compared the scores assigned to GT‐translated sentences for each of the five manually scored domains as compared to the scores of the professionally translated sentences, as well as the impact of word count and sentence complexity on the scores achieved specifically by the GT‐translated sentences, using clustered linear regression to account for the fact that each of the 45 sentences were scored by each of the three evaluators. Sentences were classified as simple if they contained one or fewer clauses and complex if they contained more than one clause.22 We also assessed interrater reliability for the manual scoring system using intraclass correlation coefficients and repeatability. Repeatability is an estimate of the maximum difference, with 95% confidence, between scores assigned to the same sentence on the same domain by two different evaluators;23 lower scores indicate greater agreement between evaluators. Since we did not have clinical data or a gold standard, we used repeatability to estimate the value above which a difference between two scores might be clinically significant and not simply due to interrater variability.24 Finally, we assessed the correlation of the manual scores with those calculated by the METEOR automated evaluation tool using Pearson correlation coefficients. All analyses were conducted using Stata 11 (College Station, TX).
Results
Sentence Description
A total of 45 sentences were evaluated by the bilingual research assistants. The initial 30 sentences and the subsequent, post‐consolidation meeting 15 sentences were scored similarly in all outcomes, after adjustment for word length and complexity, so we pooled all 45 sentences (as well as the 19 total sentence pairs scored for preference) for the final analysis. Average sentence lengths were 14.2 words, 15.5 words, and 16.6 words for the English source text, professionally translated sentences, and GT‐translated sentences, respectively. Thirty‐three percent of the English source sentences were simple and 67% were complex.
Manual Evaluation Scores
Sentences translated by GT received worse scores on fluency as compared to the professional translations (3.4 vs 4.7, P < 0.0001). Comparisons for adequacy and meaning were not statistically significantly different. GT‐translated sentences contained more errors of any severity as compared to the professional translations (39% vs 22%, P = 0.05), but a similar number of serious, clinically impactful errors (severity scores of 3, 2, or 1; 4% vs 2%, P = 0.61). However, one GT‐translated sentence was considered erroneous with a severity level of 1 (Error, dangerous to patient). This particular sentence was 25 words long and complex in structure in the original English document; all three evaluators considered the GT translation nonsensical (La hemorragia mayor, llame a su mdico, o ir a la emergencia de un hospital habitacin si usted tiene cualquiera de los siguientes: Red N, oscuro, caf o cola de orina de color.) Evaluators had no overall preference for the professional translation (3.2, 95% confidence interval = 2.7 to 3.7, with 3 indicating no preference; P = 0.36) (Table 1).
GoogleTranslate Translation | Professional Translation | P Value | |
---|---|---|---|
| |||
Fluency* | 3.4 | 4.7 | <0.0001 |
Adequacy* | 4.5 | 4.8 | 0.19 |
Meaning* | 4.2 | 4.5 | 0.29 |
Severity | |||
Any error | 39% | 22% | 0.05 |
Serious error | 4% | 2% | 0.61 |
Preference* | 3.2 | 0.36 |
Mediation of Scores by Sentence Length or Complexity
We found that sentence length was not associated with scores for fluency, adequacy, meaning, severity, or preference (P > 0.30 in each case). Complexity, however, was significantly associated with preference: evaluators' preferred the professional translation for complex English sentences while being more ambivalent about simple English sentences (3.6 vs 2.6, P = 0.03).
Interrater Reliability and Repeatability
We assessed the interrater reliability for each domain using intraclass correlation coefficients and repeatability. For fluency, the intraclass correlation was best at 0.70; for adequacy, it was 0.58; for meaning, 0.42; for severity, 0.48; and for preference, 0.37. The repeatability scores were 1.4 for fluency, 0.6 for adequacy, 2.2 for meaning, 1.2 for severity, and 3.8 for preference, indicating that two evaluators might give a sentence almost the same score (at most, 1 point apart from one another) for adequacy, but might have opposite preferences regarding which translation of a sentence was superior.
Correlation with METEOR
Correlation between the first four domains and the METEOR scores were less than in prior studies.21 Fluency correlated best with METEOR at 0.53; adequacy correlated least with METEOR at 0.29. The remaining scores were in‐between. All correlations were statistically significant at P < 0.01 (Table 2).
Correlation with METEOR | P value | |
---|---|---|
| ||
Fluency | 0.53 | <0.0001 |
Adequacy | 0.29 | 0.006 |
Meaning | 0.33 | 0.002 |
Severity | 0.39 | 0.002 |
Discussion
In this preliminary study comparing the accuracy of GT to professional translation for patient educational material, we found that GT was inferior to the professional translation in grammatical fluency but generally preserved the content and sense of the original text. Out of 30 GT sentences assessed, there was one substantially erroneous translation that was considered potentially dangerous. Evaluators preferred the professionally translated sentences for complex sentences, but when the English source sentence was simplecontaining a single clausethis preference disappeared.
Like Sharif and Tse,12 we found that for information not arranged in sentences, automated translation sometimes produced nonsensical sentences. In our study, these resulted from an English sentence fragment followed by a bulleted list; in their study, the nonsensical translations resulted from pharmacy labels. The difference in frequency of these errors between our studies may have resulted partly from the translation tool evaluated (GT vs programs used by pharmacies in the Bronx), but may have also been due to our use of machine translation for complete sentencesthe purpose for which it is optimally designed. The hypothesis that machine translations of clinical information are most understandable when used for simple, complete sentences concurs with the methodology used by these tools and requires further study.
GT has the potential to be very useful to clinicians, particularly for those instances when the communication required is both spontaneous and routine or noncritical. For example, in the inpatient setting, patients could communicate diet and other nonclinical requests, as well as ask or answer simple, short questions when the interpreter is not available. In such situations, the low cost and ease of using online translations and machine translation more generally may help to circumvent the tendency of clinicians to get by with inadequate language skills or to avoid communication altogether.25 If used wisely, GT and other online tools could supplement the use of standardized translations and professional interpreters in helping clinicians to overcome language barriers and linguistic inertia, though this will require further assessment.
Ours is a pilot study, and while it suggests a more promising way to use online translation tools, significant further evaluation is required regarding accuracy and applicability prior to widespread use of any machine translation tools for patient care. The document we utilized for evaluation was a professionally translated patient educational brochure provided to individuals starting a complex medication. As online translation tools would most likely not be used in this setting, but rather for spontaneous and less critical patient‐specific instructions, further testing of GT as applied to such scenarios should be considered. Second, we only evaluated GT for English translated into Spanish; its usefulness in other languages will need to be evaluated. It also remains to be seen how easily GT translations will be understood by patients, who may have variable medical understanding and educational attainment as compared to our evaluators. Finally, in this evaluation, we only assessed automated written translation, not automated spoken translation services such as those now available on cellular phones and other mobile devices.11 The latter are based upon translation software with an additional speech recognition interface. These applications may prove to be even more useful than online translation, but the speech recognition component will add an additional layer of potential error and these applications will need to be evaluated on their own merits.
The domains chosen for this study had only moderate interrater reliability as assessed by intraclass correlation and repeatability, with meaning and preference scoring particularly poorly. The latter domains in particular will require more thorough assessment before routine use in online translation assessment. The variability in all domains may have resulted partly from the choice of nonclinicians of different ancestral backgrounds as evaluators. However, this variability is likely better representative of the wide range of patient backgrounds. Because our evaluators were not professional translators, we asked a professional interpreter to grade all sentences to assess the quality of their evaluation. While the interpreter noted slightly fewer errors among the professionally translated sentences (13% vs 22%) and slightly more errors among the GT‐translated sentences (50% vs 39%), and preferred the professional translation slightly more (3.8 vs 3.2), his scores for all of the other measures were almost identical, increasing our confidence in our primary findings (Appendix A). Additionally, since statistical translation is conducted sentence by sentence, in our study evaluators only scored translations at the sentence level. The accuracy of GT for whole paragraphs or entire documents will need to be assessed separately. The correlation between METEOR and the manual evaluation scores was less than in prior studies; while inexpensive to assess, METEOR will have to be recalibrated in optimal circumstanceswith several reference translations available rather than just onebefore it can be used to supplement the assessment of new languages, new materials, other translation technologies, and improvements in a given technology over time for patient educational material.
In summary, GT scored worse in grammar but similarly in content and sense to the professional translation, committing one critical error in translating a complex, fragmented sentence as nonsense. We believe that, with further study and judicious use, GT has the potential to substantially improve clinicians' communication with patients with limited English proficiency in the area of brief spontaneous patient‐specific information, supplementing well the role that professional spoken interpretation and standardized written translations already play.
- Language use and English‐speaking ability: 2000. In:Census 2000 Brief.Washington, DC:US Census Bureau;2003. p. 2. http://www.census.gov/prod/2003pubs/c2kbr‐29.pdf. , .
- The need for more research on language barriers in health care: a proposed research agenda.Milbank Q.2006;84(1):111–133. , , , , .
- Language proficiency and adverse events in US hospitals: a pilot study.Int J Qual Health Care.2007;19(2):60–67. , , , .
- The impact of medical interpreter services on the quality of health care: a systematic review.Med Care Res Rev.2005;62(3):255–299. .
- Errors in medical interpretation and their potential clinical consequences in pediatric encounters.Pediatrics.2003;111(1):6–14. , , , et al.
- The effect of English language proficiency on length of stay and in‐hospital mortality.J Gen Intern Med.2004;19(3):221–228. , , , et al.
- Influence of language barriers on outcomes of hospital care for general medicine inpatients.J Hosp Med.2010;5(5):276–282. , , , .
- Hospitals, language, and culture: a snapshot of the nation. In:Los Angeles, CA:The California Endowment, the Joint Commission;2007. p.51–52. http://www.jointcommission.org/assets/1/6/hlc_paper.pdf. , .
- Do professional interpreters improve clinical care for patients with limited English proficiency? A systematic review of the literature.Health Serv Res.2007;42(2):727–754. , , , .
- Google's Computing Power Refines Translation Tool.New York Times; March 9,2010. Accessed March 24, 2010. http://www.nytimes.com/2010/03/09/technology/09translate.html?_r=1. .
- New York Times; March 20,2010. Accessed March 24, 2010. http://www.nytimes.com/2010/03/21/opinion/21bellos.html. , Translator.
- Accuracy of computer‐generated, Spanish‐language medicine labels.Pediatrics.2010;125(5):960–965. , .
- Nielsen NetRatings Search Engine Ratings.SearchEngineWatch; August 22,2006. Accessed March 24, 2010. http://searchenginewatch.com/2156451. .
- Google.Google Translate Help;2010. Accessed March 24, 2010. http://translate.google.com/support/?hl=en.
- Chapter 4: Basic strategies. In:An Introduction to Machine Translation;1992. Accessed April 22, 2010. http://www.hutchinsweb.me.uk/IntroMT‐4.pdf , .
- Your Guide to Coumadin®/Warfarin Therapy.Agency for Healthcare Research and Quality; August 21,2008. Accessed October 19, 2009. http://www.ahrq.gov/consumer/btpills.htm. .
- Patient reported receipt of medication instructions for warfarin is associated with reduced risk of serious bleeding events.J Gen Intern Med.2008;23(10):1589–1594. , , , et al.
- The ARPA MT evaluation methodologies: evolution, lessons, and future approaches. In: Proceedings of AMTA, 1994, Columbia, MD; October1994. , , .
- Overview of the IWSLT 2005 evaluation campaign. In: Proceedings of IWSLT 2005, Pittsburgh, PA; October2005. , .
- BLEU: a method for automatic evaluation of machine translation. In: ACL‐2002: 40th Annual Meeting of the Association for Computational Linguistics.2002:311–318. , , , .
- METEOR: an automatic metric for MT evaluation with high levels of correlation with human judgments. In: Proceedings of the Second Workshop on Statistical Machine Translation at ACL, Prague, Czech Republic; June2007. , .
- The Structure of a Sentence.Ottawa:The Writing Centre, University of Ottawa;2007. .
- Statistical methods for assessing agreement between two methods of clinical measurement.Lancet.1986;1(8476):307–310. , .
- Measurement, reproducibility, and validity. In:Epidemiologic Methods 203.San Francisco:Department of Biostatistics and Epidemiology, University of California;2009. .
- Getting by: underuse of interpreters by resident physicians.J Gen Intern Med.2009;24(2):256–262. , , , , .
The population of patients in the US with limited English proficiency (LEP)those who speak English less than very well1is substantial and continues to grow.1, 2 Patients with LEP are at risk for lower quality health care overall than their English‐speaking counterparts.38 Professional in‐person interpreters greatly improve spoken communication and quality of care for these patients,4, 9 but their assistance is typically based on the clinical encounter. Particularly if interpreting by phone, interpreters are unlikely to be able to help with materials such as discharge instructions or information sheets meant for family members. Professional written translations of patient educational material help to bridge this gap, allowing clinicians to convey detailed written instructions to patients. However, professional translations must be prepared well in advance of any encounter and can only be used for easily anticipated problems.
The need to translate less common, patient‐specific instructions arises spontaneously in clinical practice, and formally prepared written translations are not useful in these situations. Online translation tools such as GoogleTranslate (available at
We conducted a pilot evaluation of an online translation tool as it relates to detailed, complex patient educational material. Our primary goal was to compare the accuracy of a Spanish translation generated by the online tool to that done by a professional agency. Our secondary goals were: 1) to assess whether sentence word length or complexity mediated the accuracy of GT; and 2) to lay the foundation for a more comprehensive study of the accuracy of online translation tools, with respect to patient educational material.
Methods
Translation Tool and Language Choice
We selected Google Translate (GT) since it is one of the more commonly used online translation tools and because Google is the most widely used search engine in the United States.13 GT uses statistical translation methodology to convert text, documents, and websites between languages; statistical translation involves the following three steps. First, the translation program recognizes a sentence to translate. Second, it compares the words and phrases within that sentence to the billions of words in its library (drawn from bilingual professionally translated documents, such as United Nations proceedings). Third, it uses this comparison to generate a translation combining the words and phrases deemed most equivalent between the source sentence and the target language. If there are multiple sentences, the program recognizes and translates each independently. As the body of bilingual work grows, the program learns and refines its rules automatically.14 In contrast, in rule‐based translation, a program would use manually prespecified rules regarding word choice and grammar to generate a translation.15 We assessed GT's accuracy translating from English to Spanish because Spanish is the predominant non‐English language spoken in the US.1
Document Selection and Preparation
We selected the instruction manual regarding warfarin use prepared by the Agency for Healthcare Research and Quality (AHRQ) for this accuracy evaluation. We selected this manual,16 written at a 6th grade reading level, because a professional Spanish translation was available (completed by ASET International Service, LLC, before and independently of this study), and because patient educational material regarding warfarin has been associated with fewer bleeding events.17 We downloaded the English document on October 19, 2009 and used the GT website to translate it en bloc. We then copied the resulting Spanish output into a text file. The English document and the professional Spanish translation (downloaded the same day) were both converted into text files in the same manner.
Grading Methodology
We scored the translation chosen using both manual and automated evaluation techniques. These techniques are widely used in the machine translation literature and are explained below.
Manual Evaluation: Evaluators, Domains, Scoring
We recruited three nonclinician, bilingual, nativeSpanish‐speaking research assistants as evaluators. The evaluators were all college educated with a Bachelor's degree or higher and were of Mexican, Nicaraguan, and Guatemalan ancestry. Each evaluator received a brief orientation regarding the project, as well as an explanation of the scores, and then proceeded to the blinded evaluation independently.
We asked evaluators to score sentences on Likert scales along five primary domains: fluency, adequacy, meaning, severity, and preference. Fluency and adequacy are well accepted components of machine translation evaluation,18 with fluency being an assessment of grammar and readability ranging from 5 (Perfect fluency; like reading a newspaper) to 1 (No fluency; no appreciable grammar, not understandable) and adequacy being an assessment of information preservation ranging from 5 (100% of information conveyed from the original) to 1 (0% of information conveyed from the original). Given that a sentence can be highly adequate but drastically change the connotation and intent of the sentence (eg, a sentence that contains 75% of the correct words but changes a sentence from take this medication twice a day to take this medication once every two days), we asked evaluators to assess meaning, a measure of connotation and intent maintenance, with scores ranging from 5 (Same meaning as original) to 1 (Totally different meaning from the original).19 Evaluators also assessed severity, a new measure of potential harm if a given sentence was assessed as having errors of any kind, ranging from 5 (Error, no effect on patient care) to 1 (Error, dangerous to patient) with an additional option of N/A (Sentence basically accurate). Finally, evaluators rated a blinded preference (also a new measure) for either of two translated sentences, ranging from Strongly prefer translation #1 to Strongly prefer translation #2. The order of the sentences was random (eg, sometimes the professional translation was first and sometimes the GT translation was). We subsequently converted this to preference for the professional translation, ranging from 5 (Strongly prefer the professional translation) to 1 (Strongly prefer the GT translation) in order to standardize the responses (Figures 1 and 2).
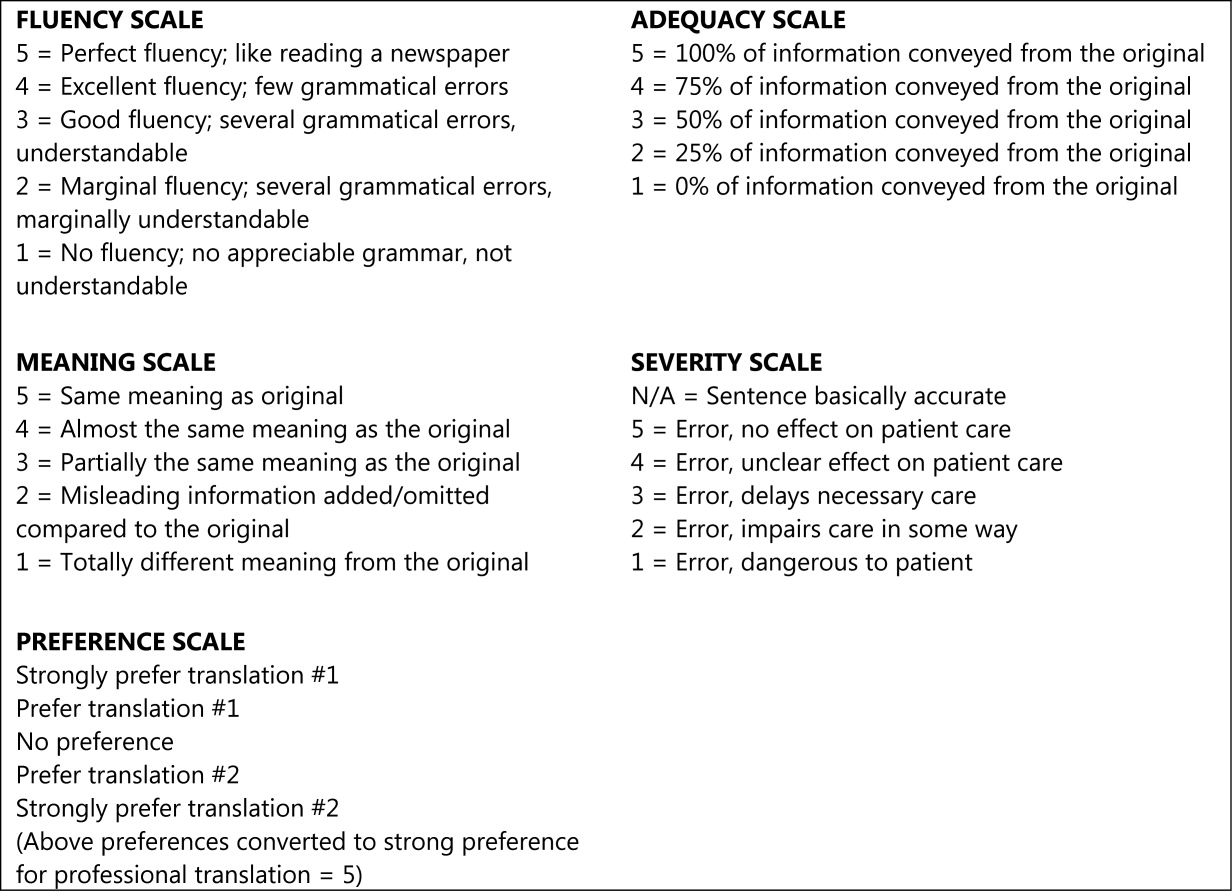
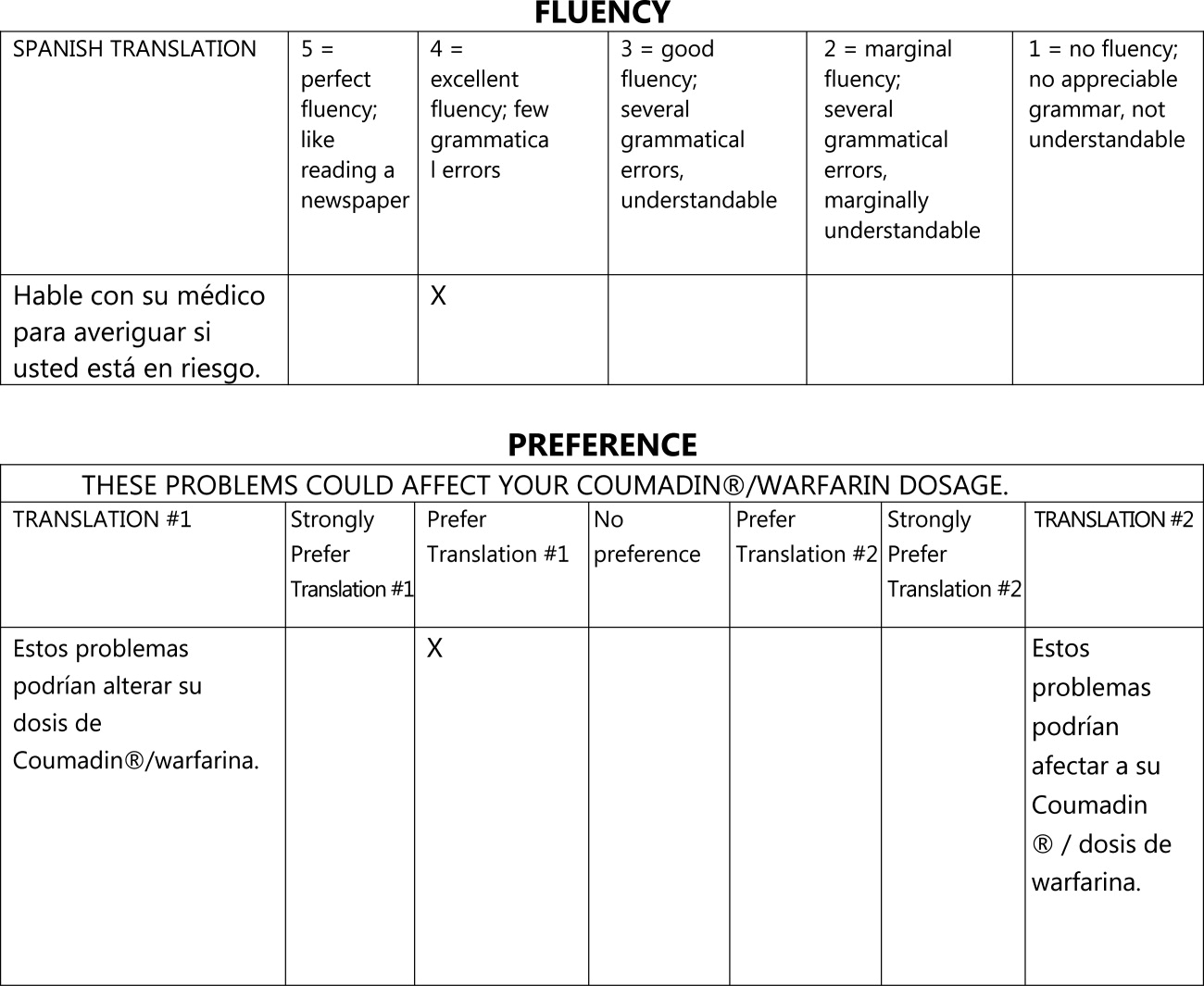
The overall flow of the study is given in Figure 3. Each evaluator initially scored 20 sentences translated by GT and 10 sentences translated professionally along the first four domains. All 30 of these sentences were randomly selected from the original, 263‐sentence pamphlet. For fluency, evaluators had access only to the translated sentence to be scored; for adequacy, meaning, and severity, they had access to both the translated sentence and the original English sentence. Ten of the 30 sentences were further selected randomly for scoring on the preference domain. For these 10 sentences, evaluators compared the GT and professional translations of the same sentence (with the original English sentence available as a reference) and indicated a preference, for any reason, for one translation or the other. Evaluators were blinded to the technique of translation (GT or professional) for all scored sentences and domains. We chose twice as many sentences from the GT preparations for the first four domains to maximize measurements for the translation technology we were evaluating, with the smaller number of professional translations serving as controls.
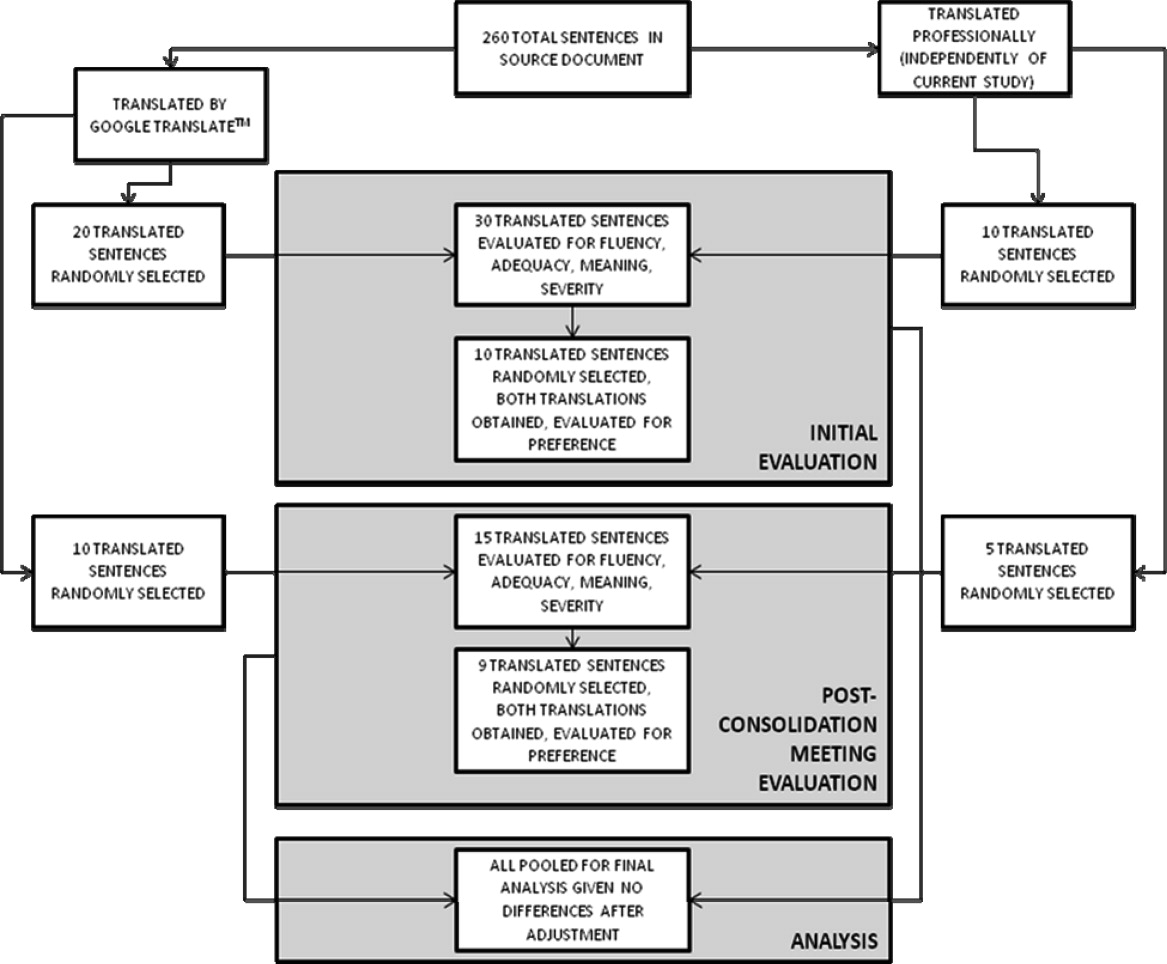
After scoring the first 30 sentences, evaluators met with one of the authors (R.R.K.) to discuss and consolidate their approach to scoring. They then scored an additional 10 GT‐translated sentences and 5 professionally translated sentences for the first four domains, and 9 of these 15 sentences for preference, to see if the meeting changed their scoring approach. These sentences were selected randomly from the original, 263‐sentence pamphlet, excluding the 30 evaluated in the previous step.
Automated Machine Translation Evaluation
Machine translation researchers have developed automated measures allowing the rapid and inexpensive scoring and rescoring of translations. These automated measures supplement more time‐ and resource‐intensive manual evaluations. The automated measures are based upon how well the translation compares to one or, ideally, multiple professionally prepared reference translations. They correlate well with human judgments on the domains above, especially when multiple reference translations are used (increasing the number of reference translations increases the variability allowed for words and phrases in the machine translation, improving the likelihood that differences in score are related to differences in quality rather than differences in translator preference).20 For this study, we used Metric for Evaluation of Translation with Explicit Ordering (METEOR), a machine translation evaluation system that allows additional flexibility for the machine translation in terms of grading individual sentences and being sensitive to synonyms, word stemming, and word order.21 We obtained a METEOR score for each of the GT‐translated sentences using the professional translation as our reference, and assessed correlation between this automated measure and the manual evaluations for the GT sentences, with the aim of assessing the feasibility of using METEOR in future work on patient educational material translation.
Outcomes and Statistical Analysis
We compared the scores assigned to GT‐translated sentences for each of the five manually scored domains as compared to the scores of the professionally translated sentences, as well as the impact of word count and sentence complexity on the scores achieved specifically by the GT‐translated sentences, using clustered linear regression to account for the fact that each of the 45 sentences were scored by each of the three evaluators. Sentences were classified as simple if they contained one or fewer clauses and complex if they contained more than one clause.22 We also assessed interrater reliability for the manual scoring system using intraclass correlation coefficients and repeatability. Repeatability is an estimate of the maximum difference, with 95% confidence, between scores assigned to the same sentence on the same domain by two different evaluators;23 lower scores indicate greater agreement between evaluators. Since we did not have clinical data or a gold standard, we used repeatability to estimate the value above which a difference between two scores might be clinically significant and not simply due to interrater variability.24 Finally, we assessed the correlation of the manual scores with those calculated by the METEOR automated evaluation tool using Pearson correlation coefficients. All analyses were conducted using Stata 11 (College Station, TX).
Results
Sentence Description
A total of 45 sentences were evaluated by the bilingual research assistants. The initial 30 sentences and the subsequent, post‐consolidation meeting 15 sentences were scored similarly in all outcomes, after adjustment for word length and complexity, so we pooled all 45 sentences (as well as the 19 total sentence pairs scored for preference) for the final analysis. Average sentence lengths were 14.2 words, 15.5 words, and 16.6 words for the English source text, professionally translated sentences, and GT‐translated sentences, respectively. Thirty‐three percent of the English source sentences were simple and 67% were complex.
Manual Evaluation Scores
Sentences translated by GT received worse scores on fluency as compared to the professional translations (3.4 vs 4.7, P < 0.0001). Comparisons for adequacy and meaning were not statistically significantly different. GT‐translated sentences contained more errors of any severity as compared to the professional translations (39% vs 22%, P = 0.05), but a similar number of serious, clinically impactful errors (severity scores of 3, 2, or 1; 4% vs 2%, P = 0.61). However, one GT‐translated sentence was considered erroneous with a severity level of 1 (Error, dangerous to patient). This particular sentence was 25 words long and complex in structure in the original English document; all three evaluators considered the GT translation nonsensical (La hemorragia mayor, llame a su mdico, o ir a la emergencia de un hospital habitacin si usted tiene cualquiera de los siguientes: Red N, oscuro, caf o cola de orina de color.) Evaluators had no overall preference for the professional translation (3.2, 95% confidence interval = 2.7 to 3.7, with 3 indicating no preference; P = 0.36) (Table 1).
GoogleTranslate Translation | Professional Translation | P Value | |
---|---|---|---|
| |||
Fluency* | 3.4 | 4.7 | <0.0001 |
Adequacy* | 4.5 | 4.8 | 0.19 |
Meaning* | 4.2 | 4.5 | 0.29 |
Severity | |||
Any error | 39% | 22% | 0.05 |
Serious error | 4% | 2% | 0.61 |
Preference* | 3.2 | 0.36 |
Mediation of Scores by Sentence Length or Complexity
We found that sentence length was not associated with scores for fluency, adequacy, meaning, severity, or preference (P > 0.30 in each case). Complexity, however, was significantly associated with preference: evaluators' preferred the professional translation for complex English sentences while being more ambivalent about simple English sentences (3.6 vs 2.6, P = 0.03).
Interrater Reliability and Repeatability
We assessed the interrater reliability for each domain using intraclass correlation coefficients and repeatability. For fluency, the intraclass correlation was best at 0.70; for adequacy, it was 0.58; for meaning, 0.42; for severity, 0.48; and for preference, 0.37. The repeatability scores were 1.4 for fluency, 0.6 for adequacy, 2.2 for meaning, 1.2 for severity, and 3.8 for preference, indicating that two evaluators might give a sentence almost the same score (at most, 1 point apart from one another) for adequacy, but might have opposite preferences regarding which translation of a sentence was superior.
Correlation with METEOR
Correlation between the first four domains and the METEOR scores were less than in prior studies.21 Fluency correlated best with METEOR at 0.53; adequacy correlated least with METEOR at 0.29. The remaining scores were in‐between. All correlations were statistically significant at P < 0.01 (Table 2).
Correlation with METEOR | P value | |
---|---|---|
| ||
Fluency | 0.53 | <0.0001 |
Adequacy | 0.29 | 0.006 |
Meaning | 0.33 | 0.002 |
Severity | 0.39 | 0.002 |
Discussion
In this preliminary study comparing the accuracy of GT to professional translation for patient educational material, we found that GT was inferior to the professional translation in grammatical fluency but generally preserved the content and sense of the original text. Out of 30 GT sentences assessed, there was one substantially erroneous translation that was considered potentially dangerous. Evaluators preferred the professionally translated sentences for complex sentences, but when the English source sentence was simplecontaining a single clausethis preference disappeared.
Like Sharif and Tse,12 we found that for information not arranged in sentences, automated translation sometimes produced nonsensical sentences. In our study, these resulted from an English sentence fragment followed by a bulleted list; in their study, the nonsensical translations resulted from pharmacy labels. The difference in frequency of these errors between our studies may have resulted partly from the translation tool evaluated (GT vs programs used by pharmacies in the Bronx), but may have also been due to our use of machine translation for complete sentencesthe purpose for which it is optimally designed. The hypothesis that machine translations of clinical information are most understandable when used for simple, complete sentences concurs with the methodology used by these tools and requires further study.
GT has the potential to be very useful to clinicians, particularly for those instances when the communication required is both spontaneous and routine or noncritical. For example, in the inpatient setting, patients could communicate diet and other nonclinical requests, as well as ask or answer simple, short questions when the interpreter is not available. In such situations, the low cost and ease of using online translations and machine translation more generally may help to circumvent the tendency of clinicians to get by with inadequate language skills or to avoid communication altogether.25 If used wisely, GT and other online tools could supplement the use of standardized translations and professional interpreters in helping clinicians to overcome language barriers and linguistic inertia, though this will require further assessment.
Ours is a pilot study, and while it suggests a more promising way to use online translation tools, significant further evaluation is required regarding accuracy and applicability prior to widespread use of any machine translation tools for patient care. The document we utilized for evaluation was a professionally translated patient educational brochure provided to individuals starting a complex medication. As online translation tools would most likely not be used in this setting, but rather for spontaneous and less critical patient‐specific instructions, further testing of GT as applied to such scenarios should be considered. Second, we only evaluated GT for English translated into Spanish; its usefulness in other languages will need to be evaluated. It also remains to be seen how easily GT translations will be understood by patients, who may have variable medical understanding and educational attainment as compared to our evaluators. Finally, in this evaluation, we only assessed automated written translation, not automated spoken translation services such as those now available on cellular phones and other mobile devices.11 The latter are based upon translation software with an additional speech recognition interface. These applications may prove to be even more useful than online translation, but the speech recognition component will add an additional layer of potential error and these applications will need to be evaluated on their own merits.
The domains chosen for this study had only moderate interrater reliability as assessed by intraclass correlation and repeatability, with meaning and preference scoring particularly poorly. The latter domains in particular will require more thorough assessment before routine use in online translation assessment. The variability in all domains may have resulted partly from the choice of nonclinicians of different ancestral backgrounds as evaluators. However, this variability is likely better representative of the wide range of patient backgrounds. Because our evaluators were not professional translators, we asked a professional interpreter to grade all sentences to assess the quality of their evaluation. While the interpreter noted slightly fewer errors among the professionally translated sentences (13% vs 22%) and slightly more errors among the GT‐translated sentences (50% vs 39%), and preferred the professional translation slightly more (3.8 vs 3.2), his scores for all of the other measures were almost identical, increasing our confidence in our primary findings (Appendix A). Additionally, since statistical translation is conducted sentence by sentence, in our study evaluators only scored translations at the sentence level. The accuracy of GT for whole paragraphs or entire documents will need to be assessed separately. The correlation between METEOR and the manual evaluation scores was less than in prior studies; while inexpensive to assess, METEOR will have to be recalibrated in optimal circumstanceswith several reference translations available rather than just onebefore it can be used to supplement the assessment of new languages, new materials, other translation technologies, and improvements in a given technology over time for patient educational material.
In summary, GT scored worse in grammar but similarly in content and sense to the professional translation, committing one critical error in translating a complex, fragmented sentence as nonsense. We believe that, with further study and judicious use, GT has the potential to substantially improve clinicians' communication with patients with limited English proficiency in the area of brief spontaneous patient‐specific information, supplementing well the role that professional spoken interpretation and standardized written translations already play.
The population of patients in the US with limited English proficiency (LEP)those who speak English less than very well1is substantial and continues to grow.1, 2 Patients with LEP are at risk for lower quality health care overall than their English‐speaking counterparts.38 Professional in‐person interpreters greatly improve spoken communication and quality of care for these patients,4, 9 but their assistance is typically based on the clinical encounter. Particularly if interpreting by phone, interpreters are unlikely to be able to help with materials such as discharge instructions or information sheets meant for family members. Professional written translations of patient educational material help to bridge this gap, allowing clinicians to convey detailed written instructions to patients. However, professional translations must be prepared well in advance of any encounter and can only be used for easily anticipated problems.
The need to translate less common, patient‐specific instructions arises spontaneously in clinical practice, and formally prepared written translations are not useful in these situations. Online translation tools such as GoogleTranslate (available at
We conducted a pilot evaluation of an online translation tool as it relates to detailed, complex patient educational material. Our primary goal was to compare the accuracy of a Spanish translation generated by the online tool to that done by a professional agency. Our secondary goals were: 1) to assess whether sentence word length or complexity mediated the accuracy of GT; and 2) to lay the foundation for a more comprehensive study of the accuracy of online translation tools, with respect to patient educational material.
Methods
Translation Tool and Language Choice
We selected Google Translate (GT) since it is one of the more commonly used online translation tools and because Google is the most widely used search engine in the United States.13 GT uses statistical translation methodology to convert text, documents, and websites between languages; statistical translation involves the following three steps. First, the translation program recognizes a sentence to translate. Second, it compares the words and phrases within that sentence to the billions of words in its library (drawn from bilingual professionally translated documents, such as United Nations proceedings). Third, it uses this comparison to generate a translation combining the words and phrases deemed most equivalent between the source sentence and the target language. If there are multiple sentences, the program recognizes and translates each independently. As the body of bilingual work grows, the program learns and refines its rules automatically.14 In contrast, in rule‐based translation, a program would use manually prespecified rules regarding word choice and grammar to generate a translation.15 We assessed GT's accuracy translating from English to Spanish because Spanish is the predominant non‐English language spoken in the US.1
Document Selection and Preparation
We selected the instruction manual regarding warfarin use prepared by the Agency for Healthcare Research and Quality (AHRQ) for this accuracy evaluation. We selected this manual,16 written at a 6th grade reading level, because a professional Spanish translation was available (completed by ASET International Service, LLC, before and independently of this study), and because patient educational material regarding warfarin has been associated with fewer bleeding events.17 We downloaded the English document on October 19, 2009 and used the GT website to translate it en bloc. We then copied the resulting Spanish output into a text file. The English document and the professional Spanish translation (downloaded the same day) were both converted into text files in the same manner.
Grading Methodology
We scored the translation chosen using both manual and automated evaluation techniques. These techniques are widely used in the machine translation literature and are explained below.
Manual Evaluation: Evaluators, Domains, Scoring
We recruited three nonclinician, bilingual, nativeSpanish‐speaking research assistants as evaluators. The evaluators were all college educated with a Bachelor's degree or higher and were of Mexican, Nicaraguan, and Guatemalan ancestry. Each evaluator received a brief orientation regarding the project, as well as an explanation of the scores, and then proceeded to the blinded evaluation independently.
We asked evaluators to score sentences on Likert scales along five primary domains: fluency, adequacy, meaning, severity, and preference. Fluency and adequacy are well accepted components of machine translation evaluation,18 with fluency being an assessment of grammar and readability ranging from 5 (Perfect fluency; like reading a newspaper) to 1 (No fluency; no appreciable grammar, not understandable) and adequacy being an assessment of information preservation ranging from 5 (100% of information conveyed from the original) to 1 (0% of information conveyed from the original). Given that a sentence can be highly adequate but drastically change the connotation and intent of the sentence (eg, a sentence that contains 75% of the correct words but changes a sentence from take this medication twice a day to take this medication once every two days), we asked evaluators to assess meaning, a measure of connotation and intent maintenance, with scores ranging from 5 (Same meaning as original) to 1 (Totally different meaning from the original).19 Evaluators also assessed severity, a new measure of potential harm if a given sentence was assessed as having errors of any kind, ranging from 5 (Error, no effect on patient care) to 1 (Error, dangerous to patient) with an additional option of N/A (Sentence basically accurate). Finally, evaluators rated a blinded preference (also a new measure) for either of two translated sentences, ranging from Strongly prefer translation #1 to Strongly prefer translation #2. The order of the sentences was random (eg, sometimes the professional translation was first and sometimes the GT translation was). We subsequently converted this to preference for the professional translation, ranging from 5 (Strongly prefer the professional translation) to 1 (Strongly prefer the GT translation) in order to standardize the responses (Figures 1 and 2).
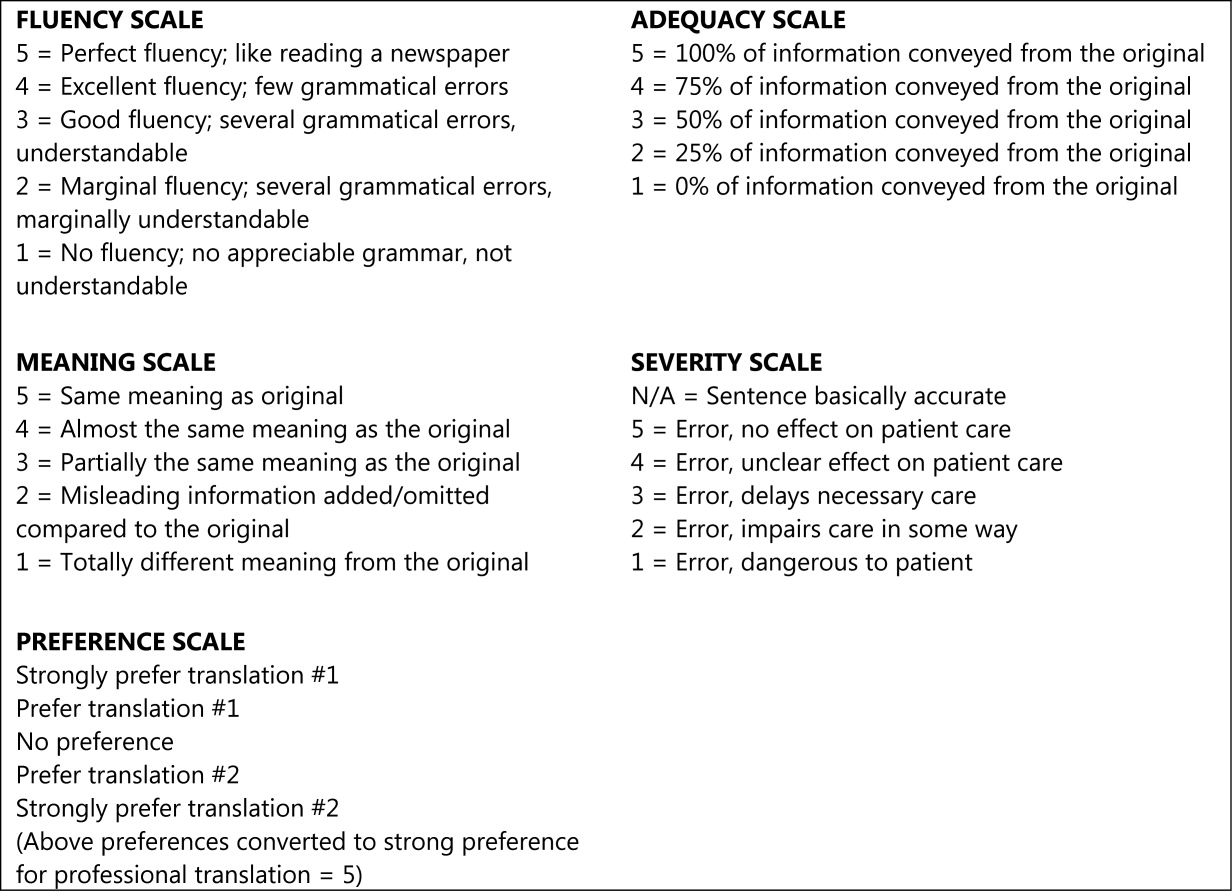
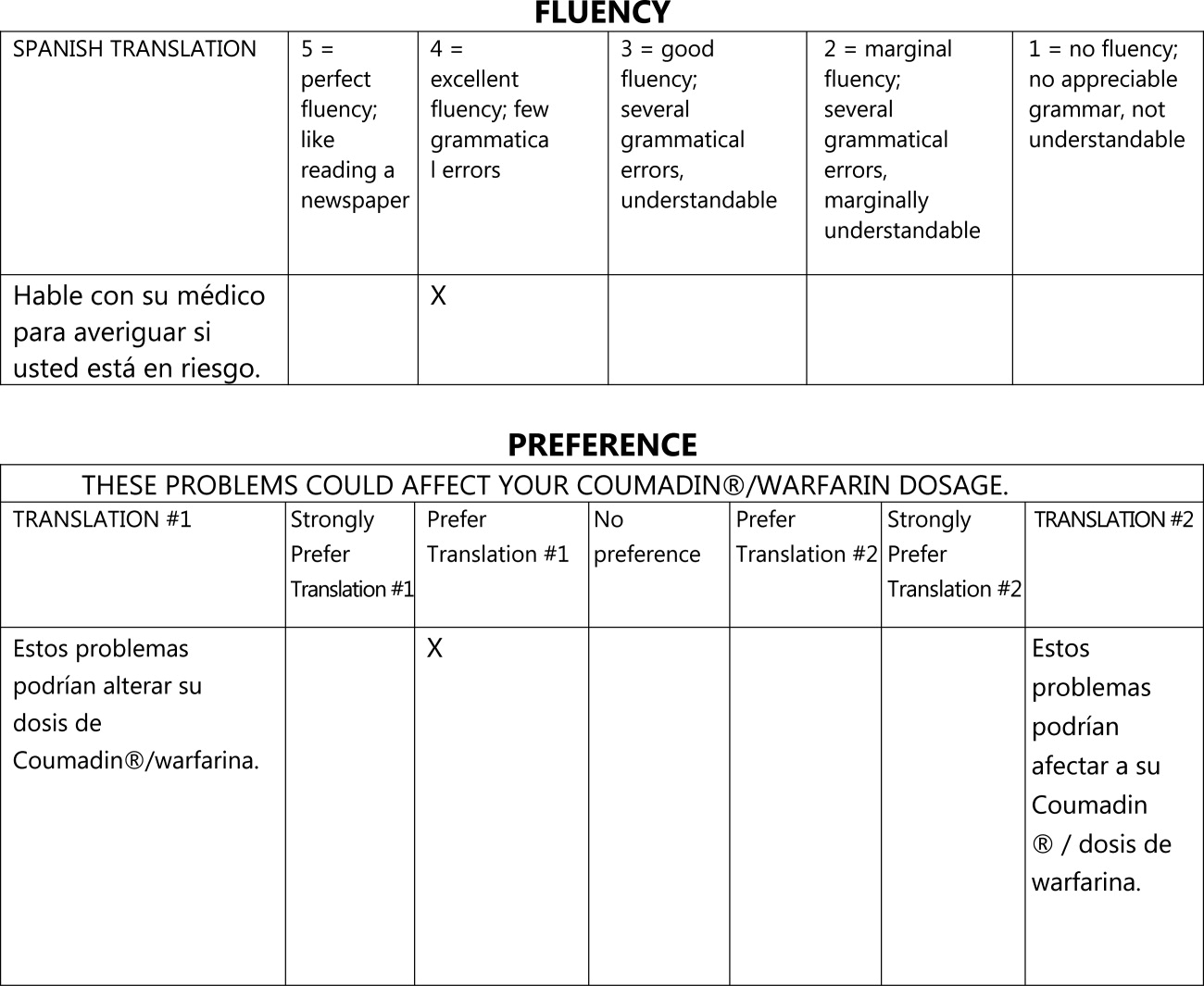
The overall flow of the study is given in Figure 3. Each evaluator initially scored 20 sentences translated by GT and 10 sentences translated professionally along the first four domains. All 30 of these sentences were randomly selected from the original, 263‐sentence pamphlet. For fluency, evaluators had access only to the translated sentence to be scored; for adequacy, meaning, and severity, they had access to both the translated sentence and the original English sentence. Ten of the 30 sentences were further selected randomly for scoring on the preference domain. For these 10 sentences, evaluators compared the GT and professional translations of the same sentence (with the original English sentence available as a reference) and indicated a preference, for any reason, for one translation or the other. Evaluators were blinded to the technique of translation (GT or professional) for all scored sentences and domains. We chose twice as many sentences from the GT preparations for the first four domains to maximize measurements for the translation technology we were evaluating, with the smaller number of professional translations serving as controls.
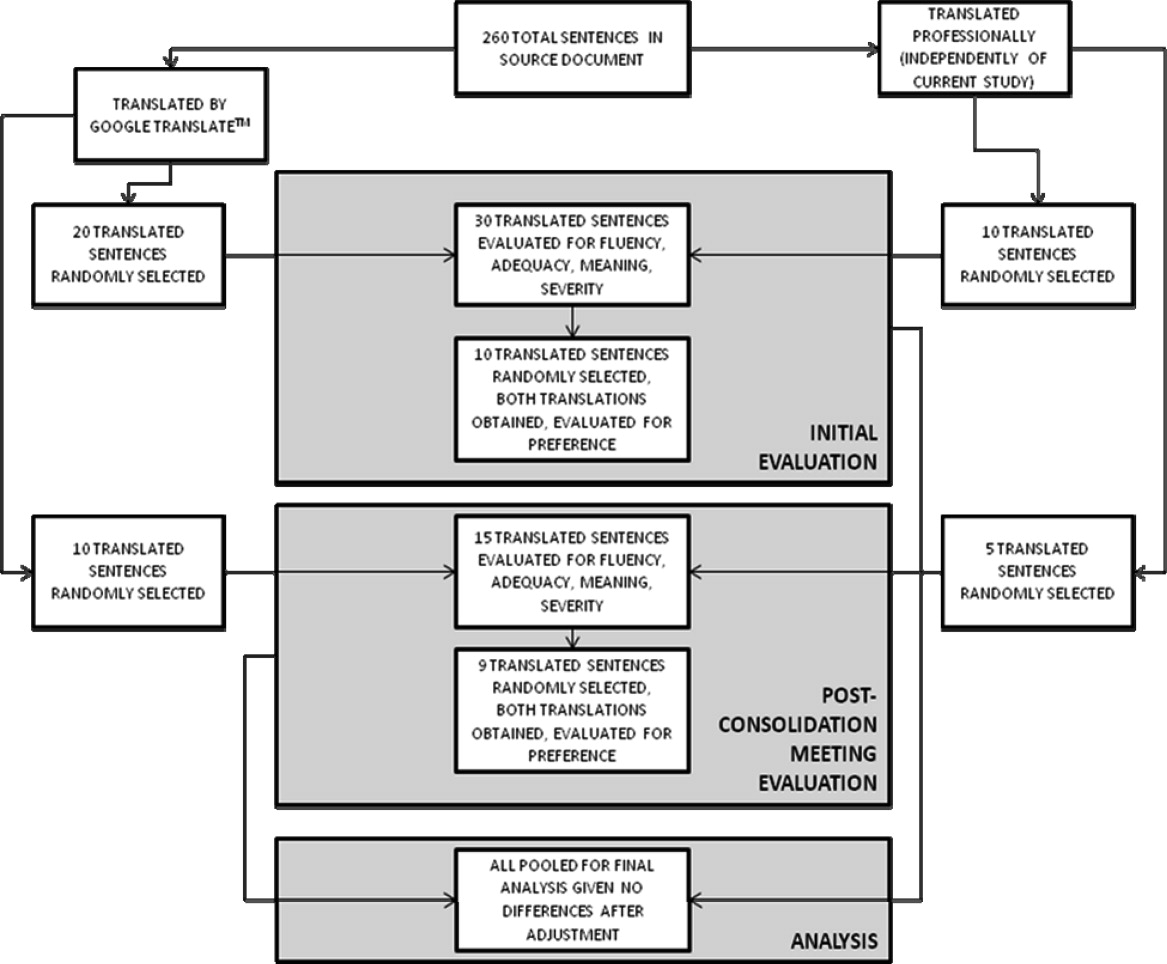
After scoring the first 30 sentences, evaluators met with one of the authors (R.R.K.) to discuss and consolidate their approach to scoring. They then scored an additional 10 GT‐translated sentences and 5 professionally translated sentences for the first four domains, and 9 of these 15 sentences for preference, to see if the meeting changed their scoring approach. These sentences were selected randomly from the original, 263‐sentence pamphlet, excluding the 30 evaluated in the previous step.
Automated Machine Translation Evaluation
Machine translation researchers have developed automated measures allowing the rapid and inexpensive scoring and rescoring of translations. These automated measures supplement more time‐ and resource‐intensive manual evaluations. The automated measures are based upon how well the translation compares to one or, ideally, multiple professionally prepared reference translations. They correlate well with human judgments on the domains above, especially when multiple reference translations are used (increasing the number of reference translations increases the variability allowed for words and phrases in the machine translation, improving the likelihood that differences in score are related to differences in quality rather than differences in translator preference).20 For this study, we used Metric for Evaluation of Translation with Explicit Ordering (METEOR), a machine translation evaluation system that allows additional flexibility for the machine translation in terms of grading individual sentences and being sensitive to synonyms, word stemming, and word order.21 We obtained a METEOR score for each of the GT‐translated sentences using the professional translation as our reference, and assessed correlation between this automated measure and the manual evaluations for the GT sentences, with the aim of assessing the feasibility of using METEOR in future work on patient educational material translation.
Outcomes and Statistical Analysis
We compared the scores assigned to GT‐translated sentences for each of the five manually scored domains as compared to the scores of the professionally translated sentences, as well as the impact of word count and sentence complexity on the scores achieved specifically by the GT‐translated sentences, using clustered linear regression to account for the fact that each of the 45 sentences were scored by each of the three evaluators. Sentences were classified as simple if they contained one or fewer clauses and complex if they contained more than one clause.22 We also assessed interrater reliability for the manual scoring system using intraclass correlation coefficients and repeatability. Repeatability is an estimate of the maximum difference, with 95% confidence, between scores assigned to the same sentence on the same domain by two different evaluators;23 lower scores indicate greater agreement between evaluators. Since we did not have clinical data or a gold standard, we used repeatability to estimate the value above which a difference between two scores might be clinically significant and not simply due to interrater variability.24 Finally, we assessed the correlation of the manual scores with those calculated by the METEOR automated evaluation tool using Pearson correlation coefficients. All analyses were conducted using Stata 11 (College Station, TX).
Results
Sentence Description
A total of 45 sentences were evaluated by the bilingual research assistants. The initial 30 sentences and the subsequent, post‐consolidation meeting 15 sentences were scored similarly in all outcomes, after adjustment for word length and complexity, so we pooled all 45 sentences (as well as the 19 total sentence pairs scored for preference) for the final analysis. Average sentence lengths were 14.2 words, 15.5 words, and 16.6 words for the English source text, professionally translated sentences, and GT‐translated sentences, respectively. Thirty‐three percent of the English source sentences were simple and 67% were complex.
Manual Evaluation Scores
Sentences translated by GT received worse scores on fluency as compared to the professional translations (3.4 vs 4.7, P < 0.0001). Comparisons for adequacy and meaning were not statistically significantly different. GT‐translated sentences contained more errors of any severity as compared to the professional translations (39% vs 22%, P = 0.05), but a similar number of serious, clinically impactful errors (severity scores of 3, 2, or 1; 4% vs 2%, P = 0.61). However, one GT‐translated sentence was considered erroneous with a severity level of 1 (Error, dangerous to patient). This particular sentence was 25 words long and complex in structure in the original English document; all three evaluators considered the GT translation nonsensical (La hemorragia mayor, llame a su mdico, o ir a la emergencia de un hospital habitacin si usted tiene cualquiera de los siguientes: Red N, oscuro, caf o cola de orina de color.) Evaluators had no overall preference for the professional translation (3.2, 95% confidence interval = 2.7 to 3.7, with 3 indicating no preference; P = 0.36) (Table 1).
GoogleTranslate Translation | Professional Translation | P Value | |
---|---|---|---|
| |||
Fluency* | 3.4 | 4.7 | <0.0001 |
Adequacy* | 4.5 | 4.8 | 0.19 |
Meaning* | 4.2 | 4.5 | 0.29 |
Severity | |||
Any error | 39% | 22% | 0.05 |
Serious error | 4% | 2% | 0.61 |
Preference* | 3.2 | 0.36 |
Mediation of Scores by Sentence Length or Complexity
We found that sentence length was not associated with scores for fluency, adequacy, meaning, severity, or preference (P > 0.30 in each case). Complexity, however, was significantly associated with preference: evaluators' preferred the professional translation for complex English sentences while being more ambivalent about simple English sentences (3.6 vs 2.6, P = 0.03).
Interrater Reliability and Repeatability
We assessed the interrater reliability for each domain using intraclass correlation coefficients and repeatability. For fluency, the intraclass correlation was best at 0.70; for adequacy, it was 0.58; for meaning, 0.42; for severity, 0.48; and for preference, 0.37. The repeatability scores were 1.4 for fluency, 0.6 for adequacy, 2.2 for meaning, 1.2 for severity, and 3.8 for preference, indicating that two evaluators might give a sentence almost the same score (at most, 1 point apart from one another) for adequacy, but might have opposite preferences regarding which translation of a sentence was superior.
Correlation with METEOR
Correlation between the first four domains and the METEOR scores were less than in prior studies.21 Fluency correlated best with METEOR at 0.53; adequacy correlated least with METEOR at 0.29. The remaining scores were in‐between. All correlations were statistically significant at P < 0.01 (Table 2).
Correlation with METEOR | P value | |
---|---|---|
| ||
Fluency | 0.53 | <0.0001 |
Adequacy | 0.29 | 0.006 |
Meaning | 0.33 | 0.002 |
Severity | 0.39 | 0.002 |
Discussion
In this preliminary study comparing the accuracy of GT to professional translation for patient educational material, we found that GT was inferior to the professional translation in grammatical fluency but generally preserved the content and sense of the original text. Out of 30 GT sentences assessed, there was one substantially erroneous translation that was considered potentially dangerous. Evaluators preferred the professionally translated sentences for complex sentences, but when the English source sentence was simplecontaining a single clausethis preference disappeared.
Like Sharif and Tse,12 we found that for information not arranged in sentences, automated translation sometimes produced nonsensical sentences. In our study, these resulted from an English sentence fragment followed by a bulleted list; in their study, the nonsensical translations resulted from pharmacy labels. The difference in frequency of these errors between our studies may have resulted partly from the translation tool evaluated (GT vs programs used by pharmacies in the Bronx), but may have also been due to our use of machine translation for complete sentencesthe purpose for which it is optimally designed. The hypothesis that machine translations of clinical information are most understandable when used for simple, complete sentences concurs with the methodology used by these tools and requires further study.
GT has the potential to be very useful to clinicians, particularly for those instances when the communication required is both spontaneous and routine or noncritical. For example, in the inpatient setting, patients could communicate diet and other nonclinical requests, as well as ask or answer simple, short questions when the interpreter is not available. In such situations, the low cost and ease of using online translations and machine translation more generally may help to circumvent the tendency of clinicians to get by with inadequate language skills or to avoid communication altogether.25 If used wisely, GT and other online tools could supplement the use of standardized translations and professional interpreters in helping clinicians to overcome language barriers and linguistic inertia, though this will require further assessment.
Ours is a pilot study, and while it suggests a more promising way to use online translation tools, significant further evaluation is required regarding accuracy and applicability prior to widespread use of any machine translation tools for patient care. The document we utilized for evaluation was a professionally translated patient educational brochure provided to individuals starting a complex medication. As online translation tools would most likely not be used in this setting, but rather for spontaneous and less critical patient‐specific instructions, further testing of GT as applied to such scenarios should be considered. Second, we only evaluated GT for English translated into Spanish; its usefulness in other languages will need to be evaluated. It also remains to be seen how easily GT translations will be understood by patients, who may have variable medical understanding and educational attainment as compared to our evaluators. Finally, in this evaluation, we only assessed automated written translation, not automated spoken translation services such as those now available on cellular phones and other mobile devices.11 The latter are based upon translation software with an additional speech recognition interface. These applications may prove to be even more useful than online translation, but the speech recognition component will add an additional layer of potential error and these applications will need to be evaluated on their own merits.
The domains chosen for this study had only moderate interrater reliability as assessed by intraclass correlation and repeatability, with meaning and preference scoring particularly poorly. The latter domains in particular will require more thorough assessment before routine use in online translation assessment. The variability in all domains may have resulted partly from the choice of nonclinicians of different ancestral backgrounds as evaluators. However, this variability is likely better representative of the wide range of patient backgrounds. Because our evaluators were not professional translators, we asked a professional interpreter to grade all sentences to assess the quality of their evaluation. While the interpreter noted slightly fewer errors among the professionally translated sentences (13% vs 22%) and slightly more errors among the GT‐translated sentences (50% vs 39%), and preferred the professional translation slightly more (3.8 vs 3.2), his scores for all of the other measures were almost identical, increasing our confidence in our primary findings (Appendix A). Additionally, since statistical translation is conducted sentence by sentence, in our study evaluators only scored translations at the sentence level. The accuracy of GT for whole paragraphs or entire documents will need to be assessed separately. The correlation between METEOR and the manual evaluation scores was less than in prior studies; while inexpensive to assess, METEOR will have to be recalibrated in optimal circumstanceswith several reference translations available rather than just onebefore it can be used to supplement the assessment of new languages, new materials, other translation technologies, and improvements in a given technology over time for patient educational material.
In summary, GT scored worse in grammar but similarly in content and sense to the professional translation, committing one critical error in translating a complex, fragmented sentence as nonsense. We believe that, with further study and judicious use, GT has the potential to substantially improve clinicians' communication with patients with limited English proficiency in the area of brief spontaneous patient‐specific information, supplementing well the role that professional spoken interpretation and standardized written translations already play.
- Language use and English‐speaking ability: 2000. In:Census 2000 Brief.Washington, DC:US Census Bureau;2003. p. 2. http://www.census.gov/prod/2003pubs/c2kbr‐29.pdf. , .
- The need for more research on language barriers in health care: a proposed research agenda.Milbank Q.2006;84(1):111–133. , , , , .
- Language proficiency and adverse events in US hospitals: a pilot study.Int J Qual Health Care.2007;19(2):60–67. , , , .
- The impact of medical interpreter services on the quality of health care: a systematic review.Med Care Res Rev.2005;62(3):255–299. .
- Errors in medical interpretation and their potential clinical consequences in pediatric encounters.Pediatrics.2003;111(1):6–14. , , , et al.
- The effect of English language proficiency on length of stay and in‐hospital mortality.J Gen Intern Med.2004;19(3):221–228. , , , et al.
- Influence of language barriers on outcomes of hospital care for general medicine inpatients.J Hosp Med.2010;5(5):276–282. , , , .
- Hospitals, language, and culture: a snapshot of the nation. In:Los Angeles, CA:The California Endowment, the Joint Commission;2007. p.51–52. http://www.jointcommission.org/assets/1/6/hlc_paper.pdf. , .
- Do professional interpreters improve clinical care for patients with limited English proficiency? A systematic review of the literature.Health Serv Res.2007;42(2):727–754. , , , .
- Google's Computing Power Refines Translation Tool.New York Times; March 9,2010. Accessed March 24, 2010. http://www.nytimes.com/2010/03/09/technology/09translate.html?_r=1. .
- New York Times; March 20,2010. Accessed March 24, 2010. http://www.nytimes.com/2010/03/21/opinion/21bellos.html. , Translator.
- Accuracy of computer‐generated, Spanish‐language medicine labels.Pediatrics.2010;125(5):960–965. , .
- Nielsen NetRatings Search Engine Ratings.SearchEngineWatch; August 22,2006. Accessed March 24, 2010. http://searchenginewatch.com/2156451. .
- Google.Google Translate Help;2010. Accessed March 24, 2010. http://translate.google.com/support/?hl=en.
- Chapter 4: Basic strategies. In:An Introduction to Machine Translation;1992. Accessed April 22, 2010. http://www.hutchinsweb.me.uk/IntroMT‐4.pdf , .
- Your Guide to Coumadin®/Warfarin Therapy.Agency for Healthcare Research and Quality; August 21,2008. Accessed October 19, 2009. http://www.ahrq.gov/consumer/btpills.htm. .
- Patient reported receipt of medication instructions for warfarin is associated with reduced risk of serious bleeding events.J Gen Intern Med.2008;23(10):1589–1594. , , , et al.
- The ARPA MT evaluation methodologies: evolution, lessons, and future approaches. In: Proceedings of AMTA, 1994, Columbia, MD; October1994. , , .
- Overview of the IWSLT 2005 evaluation campaign. In: Proceedings of IWSLT 2005, Pittsburgh, PA; October2005. , .
- BLEU: a method for automatic evaluation of machine translation. In: ACL‐2002: 40th Annual Meeting of the Association for Computational Linguistics.2002:311–318. , , , .
- METEOR: an automatic metric for MT evaluation with high levels of correlation with human judgments. In: Proceedings of the Second Workshop on Statistical Machine Translation at ACL, Prague, Czech Republic; June2007. , .
- The Structure of a Sentence.Ottawa:The Writing Centre, University of Ottawa;2007. .
- Statistical methods for assessing agreement between two methods of clinical measurement.Lancet.1986;1(8476):307–310. , .
- Measurement, reproducibility, and validity. In:Epidemiologic Methods 203.San Francisco:Department of Biostatistics and Epidemiology, University of California;2009. .
- Getting by: underuse of interpreters by resident physicians.J Gen Intern Med.2009;24(2):256–262. , , , , .
- Language use and English‐speaking ability: 2000. In:Census 2000 Brief.Washington, DC:US Census Bureau;2003. p. 2. http://www.census.gov/prod/2003pubs/c2kbr‐29.pdf. , .
- The need for more research on language barriers in health care: a proposed research agenda.Milbank Q.2006;84(1):111–133. , , , , .
- Language proficiency and adverse events in US hospitals: a pilot study.Int J Qual Health Care.2007;19(2):60–67. , , , .
- The impact of medical interpreter services on the quality of health care: a systematic review.Med Care Res Rev.2005;62(3):255–299. .
- Errors in medical interpretation and their potential clinical consequences in pediatric encounters.Pediatrics.2003;111(1):6–14. , , , et al.
- The effect of English language proficiency on length of stay and in‐hospital mortality.J Gen Intern Med.2004;19(3):221–228. , , , et al.
- Influence of language barriers on outcomes of hospital care for general medicine inpatients.J Hosp Med.2010;5(5):276–282. , , , .
- Hospitals, language, and culture: a snapshot of the nation. In:Los Angeles, CA:The California Endowment, the Joint Commission;2007. p.51–52. http://www.jointcommission.org/assets/1/6/hlc_paper.pdf. , .
- Do professional interpreters improve clinical care for patients with limited English proficiency? A systematic review of the literature.Health Serv Res.2007;42(2):727–754. , , , .
- Google's Computing Power Refines Translation Tool.New York Times; March 9,2010. Accessed March 24, 2010. http://www.nytimes.com/2010/03/09/technology/09translate.html?_r=1. .
- New York Times; March 20,2010. Accessed March 24, 2010. http://www.nytimes.com/2010/03/21/opinion/21bellos.html. , Translator.
- Accuracy of computer‐generated, Spanish‐language medicine labels.Pediatrics.2010;125(5):960–965. , .
- Nielsen NetRatings Search Engine Ratings.SearchEngineWatch; August 22,2006. Accessed March 24, 2010. http://searchenginewatch.com/2156451. .
- Google.Google Translate Help;2010. Accessed March 24, 2010. http://translate.google.com/support/?hl=en.
- Chapter 4: Basic strategies. In:An Introduction to Machine Translation;1992. Accessed April 22, 2010. http://www.hutchinsweb.me.uk/IntroMT‐4.pdf , .
- Your Guide to Coumadin®/Warfarin Therapy.Agency for Healthcare Research and Quality; August 21,2008. Accessed October 19, 2009. http://www.ahrq.gov/consumer/btpills.htm. .
- Patient reported receipt of medication instructions for warfarin is associated with reduced risk of serious bleeding events.J Gen Intern Med.2008;23(10):1589–1594. , , , et al.
- The ARPA MT evaluation methodologies: evolution, lessons, and future approaches. In: Proceedings of AMTA, 1994, Columbia, MD; October1994. , , .
- Overview of the IWSLT 2005 evaluation campaign. In: Proceedings of IWSLT 2005, Pittsburgh, PA; October2005. , .
- BLEU: a method for automatic evaluation of machine translation. In: ACL‐2002: 40th Annual Meeting of the Association for Computational Linguistics.2002:311–318. , , , .
- METEOR: an automatic metric for MT evaluation with high levels of correlation with human judgments. In: Proceedings of the Second Workshop on Statistical Machine Translation at ACL, Prague, Czech Republic; June2007. , .
- The Structure of a Sentence.Ottawa:The Writing Centre, University of Ottawa;2007. .
- Statistical methods for assessing agreement between two methods of clinical measurement.Lancet.1986;1(8476):307–310. , .
- Measurement, reproducibility, and validity. In:Epidemiologic Methods 203.San Francisco:Department of Biostatistics and Epidemiology, University of California;2009. .
- Getting by: underuse of interpreters by resident physicians.J Gen Intern Med.2009;24(2):256–262. , , , , .
Copyright © 2011 Society of Hospital Medicine