User login
Generating Mortality Predictions
The systematic deployment of prediction rules within health systems remains a challenge, although such decision aids have been available for decades.[1, 2] We previously developed and validated a prediction rule for 30‐day mortality in a retrospective cohort, noting that the mortality risk is associated with a number of other clinical events.[3] These relationships suggest risk strata, defined by the predicted probability of 30‐day mortality, and could trigger a number of coordinated care processes proportional to the level of risk.[4] For example, patients within the higher‐risk strata could be considered for placement into an intermediate or intensive care unit (ICU), be monitored more closely by physician and nurse team members for clinical deterioration, be seen by a physician within a few days of hospital discharge, and be considered for advance care planning discussions.[3, 4, 5, 6, 7] Patients within the lower‐risk strata might not need the same intensity of these processes routinely unless some other indication were present.
However attractive this conceptual framework may be, its realization is dependent on the willingness of clinical staff to generate predictions consistently on a substantial portion of the patient population, and on the accuracy of the predictions when the risk factors are determined with some level of uncertainty at the beginning of the hospitalization.[2, 8] Skepticism is justified, because the work involved in completing the prediction rule might be incompatible with existing workflow. A patient might not be scored if the emergency physician lacks time or if technical issues arise with the information system and computation process.[9] There is also a generic concern that the predictions will prove to be less accurate outside of the original study population.[8, 9, 10] A more specific concern for our rule is how well present on admission diagnoses can be determined during the relatively short emergency department or presurgery evaluation period. For example, a final diagnosis of heart failure might not be established until later in the hospitalization, after the results of diagnostic testing and clinical response to treatment are known. Moreover, our retrospective prediction rule requires an assessment of the presence or absence of sepsis and respiratory failure. These diagnoses appear to be susceptible to secular trends in medical record coding practices, suggesting the rule's accuracy might not be stable over time.[11]
We report the feasibility of having emergency physicians and the surgical preparation center team generate mortality predictions before an inpatient bed is assigned. We evaluate and report the accuracy of these prospective predictions.
METHODS
The study population consisted of all patients 18 years of age or less than 100 years who were admitted from the emergency department or assigned an inpatient bed following elective surgery at a tertiary, community teaching hospital in the Midwestern United States from September 1, 2012 through February 15, 2013. Although patients entering the hospital from these 2 pathways would be expected to have different levels of mortality risk, we used the original prediction rule for both because such distinctions were not made in its derivation and validation. Patients were not considered if they were admitted for childbirth or other obstetrical reasons, admitted directly from physician offices, the cardiac catheterization laboratory, hemodialysis unit, or from another hospital. The site institutional review board approved this study.
The implementation process began with presentations to the administrative and medical staff leadership on the accuracy of the retrospectively generated mortality predictions and risk of other adverse events.[3] The chief medical and nursing officers became project champions, secured internal funding for the technical components, and arranged to have 2 project comanagers available. A multidisciplinary task force endorsed the implementation details at biweekly meetings throughout the planning year. The leadership of the emergency department and surgical preparation center committed their colleagues to generate the predictions. The support of the emergency leadership was contingent on the completion of the entire prediction generating process in a very short time (within the time a physician could hold his/her breath). The chief medical officer, with the support of the leadership of the hospitalists and emergency physicians, made the administrative decision that a prediction must be generated prior to the assignment of a hospital room.
During the consensus‐building phase, a Web‐based application was developed to generate the predictions. Emergency physicians and surgical preparation staff were trained on the definitions of the risk factors (see Supporting Information, Appendix, in the online version of this article) and how to use the Web application. Three supporting databases were created. Each midnight, a past medical history database was updated, identifying those who had been discharged from the study hospital in the previous 365 days, and whether or not their diagnoses included atrial fibrillation, leukemia/lymphoma, metastatic cancer, cancer other than leukemia, lymphoma, cognitive disorder, or other neurological conditions (eg, Parkinson's, multiple sclerosis, epilepsy, coma, and stupor). Similarly, a clinical laboratory results database was created and updated real time through an HL7 (Health Level Seven, a standard data exchange format[12]) interface with the laboratory information system for the following tests performed in the preceding 30 days at a hospital‐affiliated facility: hemoglobin, platelet count, white blood count, serum troponin, blood urea nitrogen, serum albumin, serum lactate, arterial pH, arterial partial pressure of oxygen values. The third database, admission‐discharge‐transfer, was created and updated every 15 minutes to identify patients currently in the emergency room or scheduled for surgery. When a patient registration event was added to this database, the Web application created a record, retrieved all relevant data, and displayed the patient name for scoring. When the decision for hospitalization was made, the clinician selected the patient's name and reviewed the pre‐populated medical diagnoses of interest, which could be overwritten based on his/her own assessment (Figure 1A,B). The clinician then indicated (yes, no, or unknown) if the patient currently had or was being treated for each of the following: injury, heart failure, sepsis, respiratory failure, and whether or not the admitting service would be medicine (ie, nonsurgical, nonobstetrical). We considered unknown status to indicate the patient did not have the condition. When laboratory values were not available, a normal value was imputed using a previously developed algorithm.[3] Two additional questions, not used in the current prediction process, were answered to provide data for a future analysis: 1 concerning the change in the patient's condition while in the emergency department and the other concerning the presence of abnormal vital signs. The probability of 30‐day mortality was calculated via the Web application using the risk information supplied and the scoring weights (ie, parameter estimates) provided in the Appendices of our original publication.[3] Predictions were updated every minute as new laboratory values became available, and flagged with an alert if a more severe score resulted.
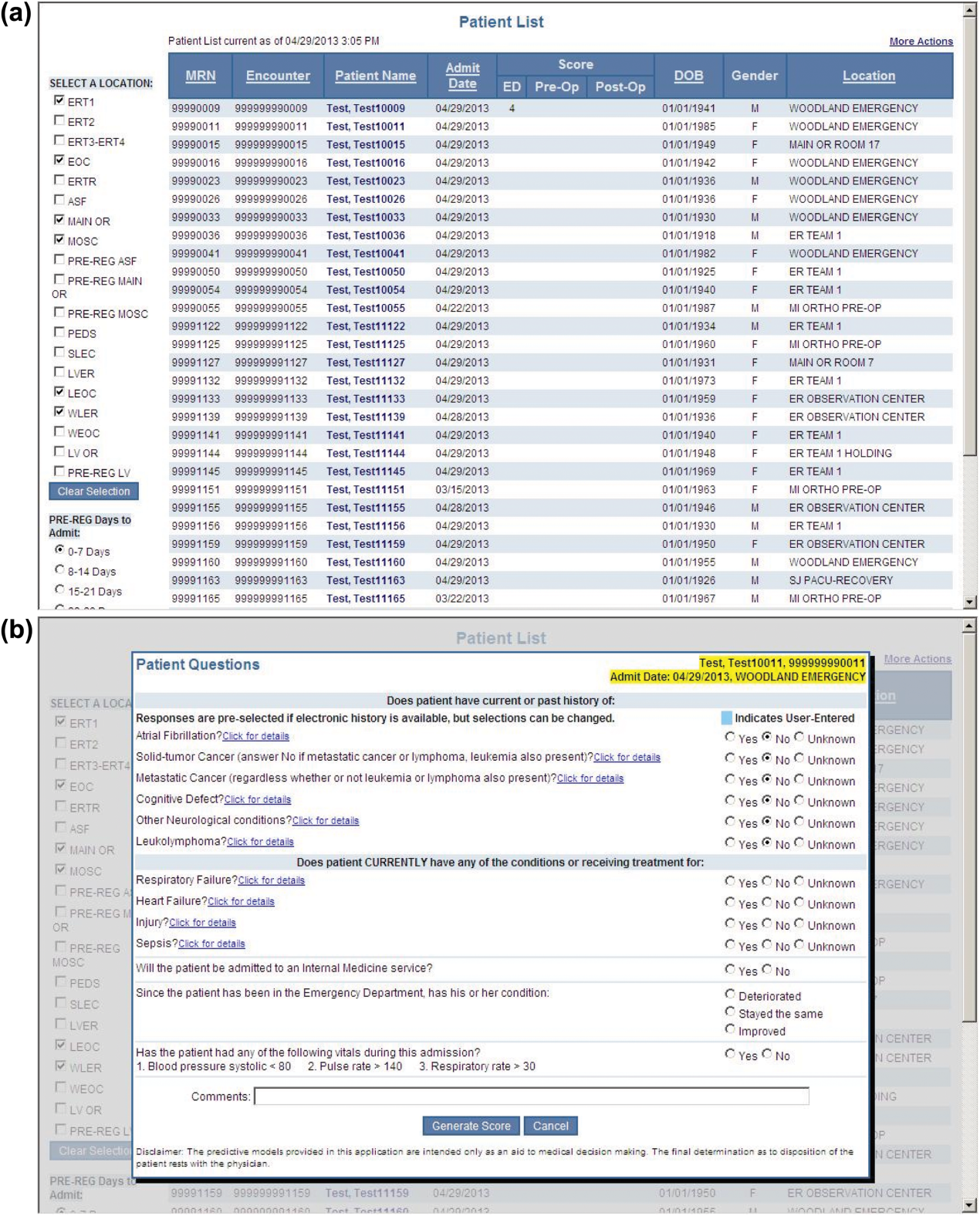
For the analyses of this study, the last prospective prediction viewed by emergency department personnel, a hospital bed manager, or surgical suite staff prior to arrival on the nursing unit is the one referenced as prospective. Once the patient had been discharged from the hospital, we generated a second mortality prediction based on previously published parameter estimates applied to risk factor data ascertained retrospectively as was done in the original article[3]; we subsequently refer to this prediction as retrospective. We will report on the group of patients who had both prospective and retrospective scores (1 patient had a prospective but not retrospective score available).
The prediction scores were made available to the clinical teams gradually during the study period. All scores were viewable by the midpoint of the study for emergency department admissions and near the end of the study for elective‐surgery patients. Only 2 changes in care processes based on level of risk were introduced during the study period. The first required initial placement of patients having a probability of dying of 0.3 or greater into an intensive or intermediate care unit unless the patient or family requested a less aggressive approach. The second occurred in the final 2 months of the study when a large multispecialty practice began routinely arranging for high‐risk patients to be seen within 3 or 7 days of hospital discharge.
Statistical Analyses
SAS version 9.3 (SAS Institute Inc., Cary, NC) was used to build the datasets and perform the analyses. Feasibility was evaluated by the number of patients who were candidates for prospective scoring with a score available at the time of admission. The validity was assessed with the primary outcome of death within 30 days from the date of hospital admission, as determined from hospital administrative data and the Social Security Death Index. The primary statistical metric is the area under the receiver operating characteristic curve (AROC) and the corresponding 95% Wald confidence limits. We needed some context for understanding the performance of the prospective predictions, assuming the accuracy could deteriorate due to the instability of the prediction rule over time and/or due to imperfect clinical information at the time the risk factors were determined. Accordingly, we also calculated an AROC based on retrospectively derived covariates (but using the same set of parameter estimates) as done in our original publication so we could gauge the stability of the original prediction rule. However, the motivation was not to determine whether retrospective versus prospective predictions were more accurate, given that only prospective predictions are useful in the context of developing real‐time care processes. Rather, we wanted to know if the prospective predictions would be sufficiently accurate for use in clinical practice. A priori, we assumed the prospective predictions should have an AROC of approximately 0.80. Therefore, a target sample size of 8660 hospitalizations was determined to be adequate to assess validity, assuming a 30‐day mortality rate of 5%, a desired lower 95% confidence boundary for the area under the prospective curve at or above 0.80, with a total confidence interval width of 0.07.[13] Calibration was assessed by comparing the actual proportion of patients dying (with 95% binomial confidence intervals) with the mean predicted mortality level within 5 percentile increments of predicted risk.
Risk Strata
We categorize the probability of 30‐day mortality into strata, with the understanding that the thresholds for defining these are a work in progress. Our hospital currently has 5 strata ranging from level 1 (highest mortality risk) to level 5 (lowest risk). The corresponding thresholds (at probabilities of death of 0.005, 0.02, 0.07, 0.20) were determined by visual inspection of the event rates and slope of curves displayed in Figure 1 of the original publication.[3]
Relationship to Secondary Clinical Outcomes of Interest
The choice of clinical care processes triggered per level of risk may be informed by understanding the frequency of events that increase with the mortality risk. We therefore examined the AROC from logistic regression models for the following outcomes using the prospectively generated probability as an explanatory variable: unplanned transfer to an ICU within the first 24 hours for patients not admitted to an ICU initially, ICU use at some point during the hospitalization, the development of a condition not present on admission (complication), receipt of palliative care by the end of the hospitalization, death during the hospitalization, 30‐day readmission, and death within 180 days. The definition of these outcomes and statistical approach used has been previously reported.[3]
RESULTS
Mortality predictions were generated on demand for 7291 out of 7777 (93.8%) eligible patients admitted from the emergency department, and for 2021 out of 2250 (89.8%) eligible elective surgical cases, for a total of 9312 predictions generated out of a possible 10,027 hospitalizations (92.9%). Table 1 displays the characteristics of the study population. The mean age was 65.2 years and 53.8% were women. The most common risk factors were atrial fibrillation (16.4%) and cancer (14.6%). Orders for a comfort care approach (rather than curative) were entered within 4 hours of admission for 32/9312 patients (0.34%), and 9/9312 (0.1%) were hospice patients on admission.
Risk Factors | No. | Without Imputation | No. | With Imputation |
---|---|---|---|---|
| ||||
Clinical laboratory values within preceding 30 days | ||||
Maximum serum blood urea nitrogen (mg/dL) | 8,484 | 22.7 (17.7) | 9,312 | 22.3 (16.9) |
Minimum hemoglobin, g/dL, | 8,750 | 12.5 (2.4) | 9,312 | 12.4 (2.4) |
Minimum platelet count, 1,000/UL | 8,737 | 224.1 (87.4) | 9,312 | 225.2 (84.7) |
Maximum white blood count, 1,000/UL | 8,750 | 10.3 (5.8) | 9,312 | 10.3 (5.6) |
Maximum serum lactate, mEq/L | 1,749 | 2.2 (1.8) | 9,312 | 0.7 (1.1) |
Minimum serum albumin, g/dL | 4,057 | 3.4 (0.7) | 9,312 | 3.2 (0.5) |
Minimum arterial pH | 509 | 7.36 (0.10) | 9,312 | 7.36 (0.02) |
Minimum arterial pO2, mm Hg | 509 | 73.6 (25.2) | 9,312 | 98.6 (8.4) |
Maximum serum troponin, ng/mL | 3,217 | 0.5 (9.3) | 9,312 | 0.2 (5.4) |
Demographics and diagnoses | ||||
Age, y | 9,312 | 65.2 (17.0) | ||
Female sex | 9,312 | 5,006 (53.8%) | ||
Previous hospitalization within past 365 days | 9,312 | 3,995 (42.9%) | ||
Emergent admission | 9,312 | 7,288 (78.3%) | ||
Admitted to a medicine service | 9,312 | 5,840 (62.7%) | ||
Current or past atrial fibrillation | 9,312 | 1,526 (16.4%) | ||
Current or past cancer without metastases, excluding leukemia or lymphoma | 9,312 | 1,356 (14.6%) | ||
Current or past history of leukemia or lymphoma | 9,312 | 145 (1.6%) | ||
Current or past metastatic cancer | 9,312 | 363 (3.9%) | ||
Current or past cognitive deficiency | 9,312 | 844 (9.1%) | ||
Current or past history of other neurological conditions (eg, Parkinson's disease, multiple sclerosis, epilepsy, coma, stupor, brain damage) | 9,312 | 952 (10.2%) | ||
Injury such as fractures or trauma at the time of admission | 9,312 | 656 (7.0%) | ||
Sepsis at the time of admission | 9,312 | 406 (4.4%) | ||
Heart failure at the time of admission | 9,312 | 776 (8.3%) | ||
Respiratory failure on admission | 9,312 | 557 (6.0%) | ||
Outcomes of interest | ||||
Unplanned transfer to an ICU (for those not admitted to an ICU) within 24 hours of admission | 8,377 | 86 (1.0%) | ||
Ever in an ICU during the hospitalization | 9,312 | 1,267 (13.6%) | ||
Development of a condition not present on admission (complication) | 9,312 | 834 (9.0%) | ||
Within hospital mortality | 9,312 | 188 (2.0%) | ||
Mortality within 30 days of admission | 9,312 | 466 (5.0%) | ||
Mortality within 180 days of admission | 9,312 | 1,070 (11.5%) | ||
Receipt of palliative care by the end of the hospitalization | 9,312 | 314 (3.4%) | ||
Readmitted to the hospital within 30 days of discharge (patients alive at discharge) | 9,124 | 1,302 (14.3%) | ||
Readmitted to the hospital within 30 days of discharge (patients alive on admission) | 9,312 | 1,302 (14.0%) |
Evaluation of Prediction Accuracy
The AROC for 30‐day mortality was 0.850 (95% confidence interval [CI]: 0.833‐0.866) for prospectively collected covariates, and 0.870 (95% CI: 0.855‐0.885) for retrospectively determined risk factors. These AROCs are not substantively different from each other, demonstrating comparable prediction performance. Calibration was excellent, as indicated in Figure 2, in which the predicted level of risk lay within the 95% confidence limits of the actual 30‐day mortality for 19 out of 20 intervals of 5 percentile increments.
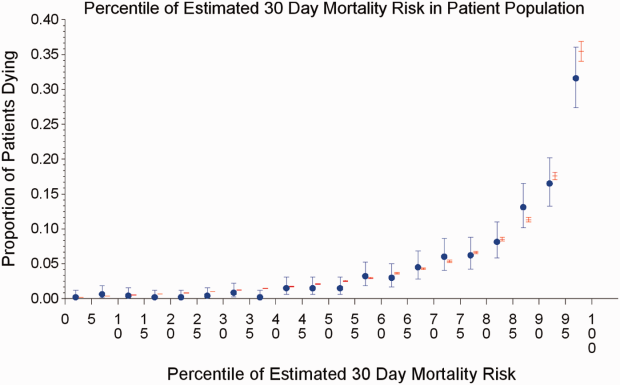
Relationship to Secondary Clinical Outcomes of Interest
The relationship between the prospectively generated probability of dying within 30 days and other events is quantified by the AROC displayed in Table 2. The 30‐day mortality risk has a strong association with the receipt of palliative care by hospital discharge, in‐hospital mortality, and 180‐day mortality, a fair association with the risk for 30‐day readmissions and unplanned transfers to intensive care, and weak associations with receipt of intensive unit care ever within the hospitalization or the development of a new diagnosis that was not present on admission (complication). The frequency of these events per mortality risk strata is shown in Table 3. The level 1 stratum contains a higher frequency of these events, whereas the level 5 stratum contains relatively few, reflecting the Pareto principle by which a relatively small proportion of patients contribute a disproportionate frequency of the events of interest.
| |
In‐hospital mortality | 0.841 (0.8140.869) |
180day mortality | 0.836 (0.8250.848) |
Receipt of palliative care by discharge | 0.875 (0.8580.891) |
30day readmission (patients alive at discharge) | 0.649 (0.6340.664) |
Unplanned transfer to an ICU (for those not admitted to an ICU) within 24 hours | 0.643 (0.5900.696) |
Ever in an ICU during the hospitalization | 0.605 (0.5880.621) |
Development of a condition not present on admission (complication) | 0.555 (0.5350.575) |
Risk Strata | 30‐Day Mortality, Count/Cases (%) | Unplanned Transfers to ICU Within 24 Hours, Count/Cases (%) | Diagnosis Not Present on Admission, Complication, Count/Cases (%) | Palliative Status at Discharge, Count/Cases (%) | Death in Hospital, Count/Cases (%) |
---|---|---|---|---|---|
Risk Strata | Ever in ICU, Count/Cases (%) | 30‐Day Readmission, Count/Cases (%) | Death or Readmission Within 30 Days, Count/Cases (%) | 180‐Day Mortality, Count/Cases (%) | |
| |||||
1 | 155/501 (30.9%) | 6/358 (1.7%) | 58/501 (11.6%) | 110/501 (22.0%) | 72/501 (14.4%) |
2 | 166/1,316 (12.6%) | 22/1,166 (1.9%) | 148/1,316 (11.3%) | 121/1,316 (9.2%) | 58/1,316 (4.4%) |
3 | 117/2,977 (3.9%) | 35/2,701 (1.3%) | 271/2,977 (9.1%) | 75/2,977 (2.5%) | 43/2,977 (1.4%) |
4 | 24/3,350 (0.7%) | 20/3,042 (0.7%) | 293/3,350 (8.8%) | 6/3,350 (0.2%) | 13/3,350 (0.4%) |
5 | 4/1,168 (0.3%) | 3/1,110 (0.3%) | 64/1,168 (5.5%) | 2/1,168 (0.2%) | 2/1,168 (0.2%) |
Total | 466/9,312 (5.0%) | 86/8,377 (1.0%) | 834/9,312 (9.0%) | 314/9,312 (3.4%) | 188/9,312 (2.0%) |
1 | 165/501 (32.9%) | 106/429 (24.7%) | 243/501 (48.5%) | 240/501 (47.9%) | |
2 | 213/1,316 (16.2%) | 275/1,258 (21.9%) | 418/1,316 (31.8%) | 403/1,316 (30.6%) | |
3 | 412/2,977 (13.8%) | 521/2,934 (17.8%) | 612/2,977 (20.6%) | 344/2,977 (11.6%) | |
4 | 406/3,350 (12.1%) | 348/3,337 (10.4%) | 368/3,350 (11.0%) | 77/3,350 (2.3%) | |
5 | 71/1,168 (6.1%) | 52/1,166 (4.5%) | 56/1,168 (4.8%) | 6/1,168 (0.5%) | |
Total | 1,267/9,312 (13.6%) | 1,302/9,124 (14.3%) | 1,697/9,312 (18.2%) | 1,070/9,312 (11.5%) |
DISCUSSION
Emergency physicians and surgical preparation center nurses generated predictions by the time of hospital admission for over 90% of the target population during usual workflow, without the addition of staff or resources. The discrimination of the prospectively generated predictions was very good to excellent, with an AROC of 0.850 (95% CI: 0.833‐0.866), similar to that obtained from the retrospective version. Calibration was excellent. The prospectively calculated mortality risk was associated with a number of other events. As shown in Table 3, the differing frequency of events within the risk strata support the development of differing intensities of multidisciplinary strategies according to the level of risk.[5] Our study provides useful experience for others who anticipate generating real‐time predictions. We consider the key reasons for success to be the considerable time spent achieving consensus, the technical development of the Web application, the brief clinician time required for the scoring process, the leadership of the chief medical and nursing officers, and the requirement that a prediction be generated before assignment of a hospital room.
Our study has a number of limitations, some of which were noted in our original publication, and although still relevant, will not be repeated here for space considerations. This is a single‐site study that used a prediction rule developed by the same site, albeit on a patient population 4 to 5 years earlier. It is not known how well the specific rule might perform in other hospital populations; any such use should therefore be accompanied by independent validation studies prior to implementation. Our successful experience should motivate future validation studies. Second, because the prognoses of patients scored from the emergency department are likely to be worse than those of elective surgery patients, our rule should be recalibrated for each subgroup separately. We plan to do this in the near future, as well as consider additional risk factors. Third, the other events of interest might be predicted more accurately if rules specifically developed for each were deployed. The mortality risk by itself is unlikely to be a sufficiently accurate predictor, particularly for complications and intensive care use, for reasons outlined in our original publication.[3] However, the varying levels of events within the higher versus lower strata should be noted by the clinical team as they design their team‐based processes. A follow‐up visit with a physician within a few days of discharge could address the concurrent risk of dying as well as readmission, for example. Finally, it is too early to determine if the availability of mortality predictions from this rule will benefit patients.[2, 8, 10] During the study period, we implemented only 2 new care processes based on the level of risk. This lack of interventions allowed us to evaluate the prediction accuracy with minimal additional confounding, but at the expense of not yet knowing the clinical impact of this work. After the study period, we implemented a number of other interventions and plan on evaluating their effectiveness in the future. We are also considering an evaluation of the potential information gained by updating the predictions throughout the course of the hospitalization.[14]
In conclusion, it is feasible to have a reasonably accurate prediction of mortality risk for most adult patients at the beginning of their hospitalizations. The availability of this prognostic information provides an opportunity to develop proactive care plans for high‐ and low‐risk subsets of patients.
Acknowledgements
The authors acknowledge the technical assistance of Nehal Sanghvi and Ben Sutton in the development of the Web application and related databases, and the support of the Chief Nursing Officer, Joyce Young, RN, PhD, the emergency department medical staff, Mohammad Salameh, MD, David Vandenberg, MD, and the surgical preparation center staff.
Disclosure: Nothing to report.
- Multifactorial index of cardiac risk in noncardiac surgical procedures. N Engl J Med. 1977;297:845–850. , , , et al.
- Methodological standards for the development of clinical decision rules in emergency medicine. Ann Emerg Med. 1999;33:437–447. , .
- Mortality predictions on admission as a context for organizing care activities. J Hosp Med. 2013;8:229–235. , , , , .
- The simple clinical score predicts mortality for 30 days after admission to an acute medical unit. QJM. 2006;99:771–781. , .
- Allocating scare resources in real‐time to reduce heart failure readmissions: a prospective, controlled study. BMJ Qual Saf. 2013;22:998–1005. , , , et al.
- Interventions to decrease hospital readmissions: keys for cost‐effectiveness. JAMA Intern Med. 2013;173:695–698. , .
- A validated value‐based model to improve hospital‐wide perioperative outcomes. Ann Surg. 2010;252:486–498. , , , et.al.
- Why is a good clinical prediction rule so hard to find? Arch Intern Med. 2011;171:1701–1702. , .
- Early detection of impending physiologic deterioration among patients who are not in intensive care: development of predictive models using data from an automated electronic medical record. J Hosp Med. 2012;7(5):388–395. , , , , , .
- Predicting death: an empirical evaluation of predictive tools for mortality. Arch Intern Med. 2011;171:1721–1726. , , .
- Association of diagnostic coding with trends in hospitalizations and mortality of patients with pneumonia, 2003–2009. JAMA. 2012;307:1405–1413. , , , , .
- Health Level Seven International website. Available at: http://www.hl7.org/. Accessed June 21, 2014.
- Bounding sample size projections for the area under a ROC curve. J Stat Plan Inference. 2009;139:711–721. .
- Derivation and validation of a model to predict daily risk of death in hospital. Med Care. 2011;49:734–743. , , , , .
The systematic deployment of prediction rules within health systems remains a challenge, although such decision aids have been available for decades.[1, 2] We previously developed and validated a prediction rule for 30‐day mortality in a retrospective cohort, noting that the mortality risk is associated with a number of other clinical events.[3] These relationships suggest risk strata, defined by the predicted probability of 30‐day mortality, and could trigger a number of coordinated care processes proportional to the level of risk.[4] For example, patients within the higher‐risk strata could be considered for placement into an intermediate or intensive care unit (ICU), be monitored more closely by physician and nurse team members for clinical deterioration, be seen by a physician within a few days of hospital discharge, and be considered for advance care planning discussions.[3, 4, 5, 6, 7] Patients within the lower‐risk strata might not need the same intensity of these processes routinely unless some other indication were present.
However attractive this conceptual framework may be, its realization is dependent on the willingness of clinical staff to generate predictions consistently on a substantial portion of the patient population, and on the accuracy of the predictions when the risk factors are determined with some level of uncertainty at the beginning of the hospitalization.[2, 8] Skepticism is justified, because the work involved in completing the prediction rule might be incompatible with existing workflow. A patient might not be scored if the emergency physician lacks time or if technical issues arise with the information system and computation process.[9] There is also a generic concern that the predictions will prove to be less accurate outside of the original study population.[8, 9, 10] A more specific concern for our rule is how well present on admission diagnoses can be determined during the relatively short emergency department or presurgery evaluation period. For example, a final diagnosis of heart failure might not be established until later in the hospitalization, after the results of diagnostic testing and clinical response to treatment are known. Moreover, our retrospective prediction rule requires an assessment of the presence or absence of sepsis and respiratory failure. These diagnoses appear to be susceptible to secular trends in medical record coding practices, suggesting the rule's accuracy might not be stable over time.[11]
We report the feasibility of having emergency physicians and the surgical preparation center team generate mortality predictions before an inpatient bed is assigned. We evaluate and report the accuracy of these prospective predictions.
METHODS
The study population consisted of all patients 18 years of age or less than 100 years who were admitted from the emergency department or assigned an inpatient bed following elective surgery at a tertiary, community teaching hospital in the Midwestern United States from September 1, 2012 through February 15, 2013. Although patients entering the hospital from these 2 pathways would be expected to have different levels of mortality risk, we used the original prediction rule for both because such distinctions were not made in its derivation and validation. Patients were not considered if they were admitted for childbirth or other obstetrical reasons, admitted directly from physician offices, the cardiac catheterization laboratory, hemodialysis unit, or from another hospital. The site institutional review board approved this study.
The implementation process began with presentations to the administrative and medical staff leadership on the accuracy of the retrospectively generated mortality predictions and risk of other adverse events.[3] The chief medical and nursing officers became project champions, secured internal funding for the technical components, and arranged to have 2 project comanagers available. A multidisciplinary task force endorsed the implementation details at biweekly meetings throughout the planning year. The leadership of the emergency department and surgical preparation center committed their colleagues to generate the predictions. The support of the emergency leadership was contingent on the completion of the entire prediction generating process in a very short time (within the time a physician could hold his/her breath). The chief medical officer, with the support of the leadership of the hospitalists and emergency physicians, made the administrative decision that a prediction must be generated prior to the assignment of a hospital room.
During the consensus‐building phase, a Web‐based application was developed to generate the predictions. Emergency physicians and surgical preparation staff were trained on the definitions of the risk factors (see Supporting Information, Appendix, in the online version of this article) and how to use the Web application. Three supporting databases were created. Each midnight, a past medical history database was updated, identifying those who had been discharged from the study hospital in the previous 365 days, and whether or not their diagnoses included atrial fibrillation, leukemia/lymphoma, metastatic cancer, cancer other than leukemia, lymphoma, cognitive disorder, or other neurological conditions (eg, Parkinson's, multiple sclerosis, epilepsy, coma, and stupor). Similarly, a clinical laboratory results database was created and updated real time through an HL7 (Health Level Seven, a standard data exchange format[12]) interface with the laboratory information system for the following tests performed in the preceding 30 days at a hospital‐affiliated facility: hemoglobin, platelet count, white blood count, serum troponin, blood urea nitrogen, serum albumin, serum lactate, arterial pH, arterial partial pressure of oxygen values. The third database, admission‐discharge‐transfer, was created and updated every 15 minutes to identify patients currently in the emergency room or scheduled for surgery. When a patient registration event was added to this database, the Web application created a record, retrieved all relevant data, and displayed the patient name for scoring. When the decision for hospitalization was made, the clinician selected the patient's name and reviewed the pre‐populated medical diagnoses of interest, which could be overwritten based on his/her own assessment (Figure 1A,B). The clinician then indicated (yes, no, or unknown) if the patient currently had or was being treated for each of the following: injury, heart failure, sepsis, respiratory failure, and whether or not the admitting service would be medicine (ie, nonsurgical, nonobstetrical). We considered unknown status to indicate the patient did not have the condition. When laboratory values were not available, a normal value was imputed using a previously developed algorithm.[3] Two additional questions, not used in the current prediction process, were answered to provide data for a future analysis: 1 concerning the change in the patient's condition while in the emergency department and the other concerning the presence of abnormal vital signs. The probability of 30‐day mortality was calculated via the Web application using the risk information supplied and the scoring weights (ie, parameter estimates) provided in the Appendices of our original publication.[3] Predictions were updated every minute as new laboratory values became available, and flagged with an alert if a more severe score resulted.
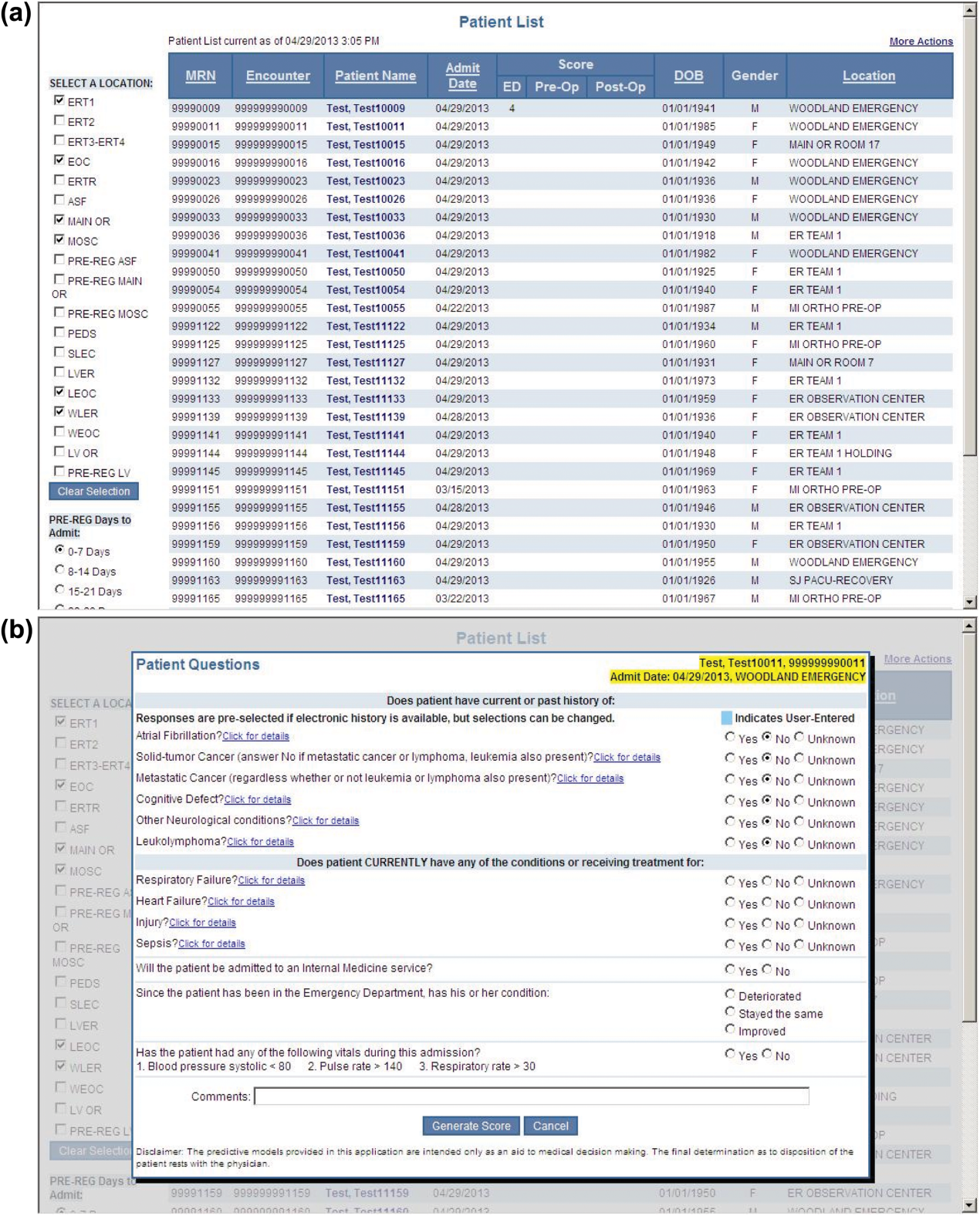
For the analyses of this study, the last prospective prediction viewed by emergency department personnel, a hospital bed manager, or surgical suite staff prior to arrival on the nursing unit is the one referenced as prospective. Once the patient had been discharged from the hospital, we generated a second mortality prediction based on previously published parameter estimates applied to risk factor data ascertained retrospectively as was done in the original article[3]; we subsequently refer to this prediction as retrospective. We will report on the group of patients who had both prospective and retrospective scores (1 patient had a prospective but not retrospective score available).
The prediction scores were made available to the clinical teams gradually during the study period. All scores were viewable by the midpoint of the study for emergency department admissions and near the end of the study for elective‐surgery patients. Only 2 changes in care processes based on level of risk were introduced during the study period. The first required initial placement of patients having a probability of dying of 0.3 or greater into an intensive or intermediate care unit unless the patient or family requested a less aggressive approach. The second occurred in the final 2 months of the study when a large multispecialty practice began routinely arranging for high‐risk patients to be seen within 3 or 7 days of hospital discharge.
Statistical Analyses
SAS version 9.3 (SAS Institute Inc., Cary, NC) was used to build the datasets and perform the analyses. Feasibility was evaluated by the number of patients who were candidates for prospective scoring with a score available at the time of admission. The validity was assessed with the primary outcome of death within 30 days from the date of hospital admission, as determined from hospital administrative data and the Social Security Death Index. The primary statistical metric is the area under the receiver operating characteristic curve (AROC) and the corresponding 95% Wald confidence limits. We needed some context for understanding the performance of the prospective predictions, assuming the accuracy could deteriorate due to the instability of the prediction rule over time and/or due to imperfect clinical information at the time the risk factors were determined. Accordingly, we also calculated an AROC based on retrospectively derived covariates (but using the same set of parameter estimates) as done in our original publication so we could gauge the stability of the original prediction rule. However, the motivation was not to determine whether retrospective versus prospective predictions were more accurate, given that only prospective predictions are useful in the context of developing real‐time care processes. Rather, we wanted to know if the prospective predictions would be sufficiently accurate for use in clinical practice. A priori, we assumed the prospective predictions should have an AROC of approximately 0.80. Therefore, a target sample size of 8660 hospitalizations was determined to be adequate to assess validity, assuming a 30‐day mortality rate of 5%, a desired lower 95% confidence boundary for the area under the prospective curve at or above 0.80, with a total confidence interval width of 0.07.[13] Calibration was assessed by comparing the actual proportion of patients dying (with 95% binomial confidence intervals) with the mean predicted mortality level within 5 percentile increments of predicted risk.
Risk Strata
We categorize the probability of 30‐day mortality into strata, with the understanding that the thresholds for defining these are a work in progress. Our hospital currently has 5 strata ranging from level 1 (highest mortality risk) to level 5 (lowest risk). The corresponding thresholds (at probabilities of death of 0.005, 0.02, 0.07, 0.20) were determined by visual inspection of the event rates and slope of curves displayed in Figure 1 of the original publication.[3]
Relationship to Secondary Clinical Outcomes of Interest
The choice of clinical care processes triggered per level of risk may be informed by understanding the frequency of events that increase with the mortality risk. We therefore examined the AROC from logistic regression models for the following outcomes using the prospectively generated probability as an explanatory variable: unplanned transfer to an ICU within the first 24 hours for patients not admitted to an ICU initially, ICU use at some point during the hospitalization, the development of a condition not present on admission (complication), receipt of palliative care by the end of the hospitalization, death during the hospitalization, 30‐day readmission, and death within 180 days. The definition of these outcomes and statistical approach used has been previously reported.[3]
RESULTS
Mortality predictions were generated on demand for 7291 out of 7777 (93.8%) eligible patients admitted from the emergency department, and for 2021 out of 2250 (89.8%) eligible elective surgical cases, for a total of 9312 predictions generated out of a possible 10,027 hospitalizations (92.9%). Table 1 displays the characteristics of the study population. The mean age was 65.2 years and 53.8% were women. The most common risk factors were atrial fibrillation (16.4%) and cancer (14.6%). Orders for a comfort care approach (rather than curative) were entered within 4 hours of admission for 32/9312 patients (0.34%), and 9/9312 (0.1%) were hospice patients on admission.
Risk Factors | No. | Without Imputation | No. | With Imputation |
---|---|---|---|---|
| ||||
Clinical laboratory values within preceding 30 days | ||||
Maximum serum blood urea nitrogen (mg/dL) | 8,484 | 22.7 (17.7) | 9,312 | 22.3 (16.9) |
Minimum hemoglobin, g/dL, | 8,750 | 12.5 (2.4) | 9,312 | 12.4 (2.4) |
Minimum platelet count, 1,000/UL | 8,737 | 224.1 (87.4) | 9,312 | 225.2 (84.7) |
Maximum white blood count, 1,000/UL | 8,750 | 10.3 (5.8) | 9,312 | 10.3 (5.6) |
Maximum serum lactate, mEq/L | 1,749 | 2.2 (1.8) | 9,312 | 0.7 (1.1) |
Minimum serum albumin, g/dL | 4,057 | 3.4 (0.7) | 9,312 | 3.2 (0.5) |
Minimum arterial pH | 509 | 7.36 (0.10) | 9,312 | 7.36 (0.02) |
Minimum arterial pO2, mm Hg | 509 | 73.6 (25.2) | 9,312 | 98.6 (8.4) |
Maximum serum troponin, ng/mL | 3,217 | 0.5 (9.3) | 9,312 | 0.2 (5.4) |
Demographics and diagnoses | ||||
Age, y | 9,312 | 65.2 (17.0) | ||
Female sex | 9,312 | 5,006 (53.8%) | ||
Previous hospitalization within past 365 days | 9,312 | 3,995 (42.9%) | ||
Emergent admission | 9,312 | 7,288 (78.3%) | ||
Admitted to a medicine service | 9,312 | 5,840 (62.7%) | ||
Current or past atrial fibrillation | 9,312 | 1,526 (16.4%) | ||
Current or past cancer without metastases, excluding leukemia or lymphoma | 9,312 | 1,356 (14.6%) | ||
Current or past history of leukemia or lymphoma | 9,312 | 145 (1.6%) | ||
Current or past metastatic cancer | 9,312 | 363 (3.9%) | ||
Current or past cognitive deficiency | 9,312 | 844 (9.1%) | ||
Current or past history of other neurological conditions (eg, Parkinson's disease, multiple sclerosis, epilepsy, coma, stupor, brain damage) | 9,312 | 952 (10.2%) | ||
Injury such as fractures or trauma at the time of admission | 9,312 | 656 (7.0%) | ||
Sepsis at the time of admission | 9,312 | 406 (4.4%) | ||
Heart failure at the time of admission | 9,312 | 776 (8.3%) | ||
Respiratory failure on admission | 9,312 | 557 (6.0%) | ||
Outcomes of interest | ||||
Unplanned transfer to an ICU (for those not admitted to an ICU) within 24 hours of admission | 8,377 | 86 (1.0%) | ||
Ever in an ICU during the hospitalization | 9,312 | 1,267 (13.6%) | ||
Development of a condition not present on admission (complication) | 9,312 | 834 (9.0%) | ||
Within hospital mortality | 9,312 | 188 (2.0%) | ||
Mortality within 30 days of admission | 9,312 | 466 (5.0%) | ||
Mortality within 180 days of admission | 9,312 | 1,070 (11.5%) | ||
Receipt of palliative care by the end of the hospitalization | 9,312 | 314 (3.4%) | ||
Readmitted to the hospital within 30 days of discharge (patients alive at discharge) | 9,124 | 1,302 (14.3%) | ||
Readmitted to the hospital within 30 days of discharge (patients alive on admission) | 9,312 | 1,302 (14.0%) |
Evaluation of Prediction Accuracy
The AROC for 30‐day mortality was 0.850 (95% confidence interval [CI]: 0.833‐0.866) for prospectively collected covariates, and 0.870 (95% CI: 0.855‐0.885) for retrospectively determined risk factors. These AROCs are not substantively different from each other, demonstrating comparable prediction performance. Calibration was excellent, as indicated in Figure 2, in which the predicted level of risk lay within the 95% confidence limits of the actual 30‐day mortality for 19 out of 20 intervals of 5 percentile increments.
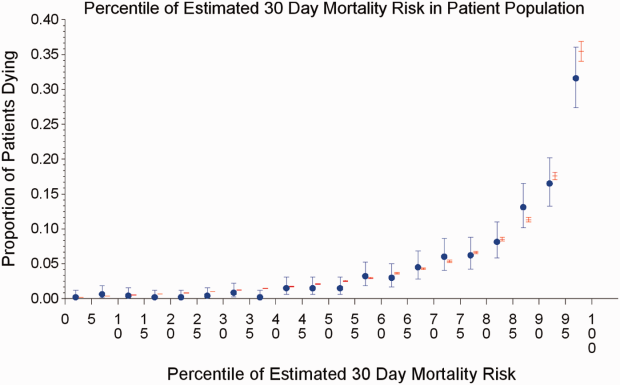
Relationship to Secondary Clinical Outcomes of Interest
The relationship between the prospectively generated probability of dying within 30 days and other events is quantified by the AROC displayed in Table 2. The 30‐day mortality risk has a strong association with the receipt of palliative care by hospital discharge, in‐hospital mortality, and 180‐day mortality, a fair association with the risk for 30‐day readmissions and unplanned transfers to intensive care, and weak associations with receipt of intensive unit care ever within the hospitalization or the development of a new diagnosis that was not present on admission (complication). The frequency of these events per mortality risk strata is shown in Table 3. The level 1 stratum contains a higher frequency of these events, whereas the level 5 stratum contains relatively few, reflecting the Pareto principle by which a relatively small proportion of patients contribute a disproportionate frequency of the events of interest.
| |
In‐hospital mortality | 0.841 (0.8140.869) |
180day mortality | 0.836 (0.8250.848) |
Receipt of palliative care by discharge | 0.875 (0.8580.891) |
30day readmission (patients alive at discharge) | 0.649 (0.6340.664) |
Unplanned transfer to an ICU (for those not admitted to an ICU) within 24 hours | 0.643 (0.5900.696) |
Ever in an ICU during the hospitalization | 0.605 (0.5880.621) |
Development of a condition not present on admission (complication) | 0.555 (0.5350.575) |
Risk Strata | 30‐Day Mortality, Count/Cases (%) | Unplanned Transfers to ICU Within 24 Hours, Count/Cases (%) | Diagnosis Not Present on Admission, Complication, Count/Cases (%) | Palliative Status at Discharge, Count/Cases (%) | Death in Hospital, Count/Cases (%) |
---|---|---|---|---|---|
Risk Strata | Ever in ICU, Count/Cases (%) | 30‐Day Readmission, Count/Cases (%) | Death or Readmission Within 30 Days, Count/Cases (%) | 180‐Day Mortality, Count/Cases (%) | |
| |||||
1 | 155/501 (30.9%) | 6/358 (1.7%) | 58/501 (11.6%) | 110/501 (22.0%) | 72/501 (14.4%) |
2 | 166/1,316 (12.6%) | 22/1,166 (1.9%) | 148/1,316 (11.3%) | 121/1,316 (9.2%) | 58/1,316 (4.4%) |
3 | 117/2,977 (3.9%) | 35/2,701 (1.3%) | 271/2,977 (9.1%) | 75/2,977 (2.5%) | 43/2,977 (1.4%) |
4 | 24/3,350 (0.7%) | 20/3,042 (0.7%) | 293/3,350 (8.8%) | 6/3,350 (0.2%) | 13/3,350 (0.4%) |
5 | 4/1,168 (0.3%) | 3/1,110 (0.3%) | 64/1,168 (5.5%) | 2/1,168 (0.2%) | 2/1,168 (0.2%) |
Total | 466/9,312 (5.0%) | 86/8,377 (1.0%) | 834/9,312 (9.0%) | 314/9,312 (3.4%) | 188/9,312 (2.0%) |
1 | 165/501 (32.9%) | 106/429 (24.7%) | 243/501 (48.5%) | 240/501 (47.9%) | |
2 | 213/1,316 (16.2%) | 275/1,258 (21.9%) | 418/1,316 (31.8%) | 403/1,316 (30.6%) | |
3 | 412/2,977 (13.8%) | 521/2,934 (17.8%) | 612/2,977 (20.6%) | 344/2,977 (11.6%) | |
4 | 406/3,350 (12.1%) | 348/3,337 (10.4%) | 368/3,350 (11.0%) | 77/3,350 (2.3%) | |
5 | 71/1,168 (6.1%) | 52/1,166 (4.5%) | 56/1,168 (4.8%) | 6/1,168 (0.5%) | |
Total | 1,267/9,312 (13.6%) | 1,302/9,124 (14.3%) | 1,697/9,312 (18.2%) | 1,070/9,312 (11.5%) |
DISCUSSION
Emergency physicians and surgical preparation center nurses generated predictions by the time of hospital admission for over 90% of the target population during usual workflow, without the addition of staff or resources. The discrimination of the prospectively generated predictions was very good to excellent, with an AROC of 0.850 (95% CI: 0.833‐0.866), similar to that obtained from the retrospective version. Calibration was excellent. The prospectively calculated mortality risk was associated with a number of other events. As shown in Table 3, the differing frequency of events within the risk strata support the development of differing intensities of multidisciplinary strategies according to the level of risk.[5] Our study provides useful experience for others who anticipate generating real‐time predictions. We consider the key reasons for success to be the considerable time spent achieving consensus, the technical development of the Web application, the brief clinician time required for the scoring process, the leadership of the chief medical and nursing officers, and the requirement that a prediction be generated before assignment of a hospital room.
Our study has a number of limitations, some of which were noted in our original publication, and although still relevant, will not be repeated here for space considerations. This is a single‐site study that used a prediction rule developed by the same site, albeit on a patient population 4 to 5 years earlier. It is not known how well the specific rule might perform in other hospital populations; any such use should therefore be accompanied by independent validation studies prior to implementation. Our successful experience should motivate future validation studies. Second, because the prognoses of patients scored from the emergency department are likely to be worse than those of elective surgery patients, our rule should be recalibrated for each subgroup separately. We plan to do this in the near future, as well as consider additional risk factors. Third, the other events of interest might be predicted more accurately if rules specifically developed for each were deployed. The mortality risk by itself is unlikely to be a sufficiently accurate predictor, particularly for complications and intensive care use, for reasons outlined in our original publication.[3] However, the varying levels of events within the higher versus lower strata should be noted by the clinical team as they design their team‐based processes. A follow‐up visit with a physician within a few days of discharge could address the concurrent risk of dying as well as readmission, for example. Finally, it is too early to determine if the availability of mortality predictions from this rule will benefit patients.[2, 8, 10] During the study period, we implemented only 2 new care processes based on the level of risk. This lack of interventions allowed us to evaluate the prediction accuracy with minimal additional confounding, but at the expense of not yet knowing the clinical impact of this work. After the study period, we implemented a number of other interventions and plan on evaluating their effectiveness in the future. We are also considering an evaluation of the potential information gained by updating the predictions throughout the course of the hospitalization.[14]
In conclusion, it is feasible to have a reasonably accurate prediction of mortality risk for most adult patients at the beginning of their hospitalizations. The availability of this prognostic information provides an opportunity to develop proactive care plans for high‐ and low‐risk subsets of patients.
Acknowledgements
The authors acknowledge the technical assistance of Nehal Sanghvi and Ben Sutton in the development of the Web application and related databases, and the support of the Chief Nursing Officer, Joyce Young, RN, PhD, the emergency department medical staff, Mohammad Salameh, MD, David Vandenberg, MD, and the surgical preparation center staff.
Disclosure: Nothing to report.
The systematic deployment of prediction rules within health systems remains a challenge, although such decision aids have been available for decades.[1, 2] We previously developed and validated a prediction rule for 30‐day mortality in a retrospective cohort, noting that the mortality risk is associated with a number of other clinical events.[3] These relationships suggest risk strata, defined by the predicted probability of 30‐day mortality, and could trigger a number of coordinated care processes proportional to the level of risk.[4] For example, patients within the higher‐risk strata could be considered for placement into an intermediate or intensive care unit (ICU), be monitored more closely by physician and nurse team members for clinical deterioration, be seen by a physician within a few days of hospital discharge, and be considered for advance care planning discussions.[3, 4, 5, 6, 7] Patients within the lower‐risk strata might not need the same intensity of these processes routinely unless some other indication were present.
However attractive this conceptual framework may be, its realization is dependent on the willingness of clinical staff to generate predictions consistently on a substantial portion of the patient population, and on the accuracy of the predictions when the risk factors are determined with some level of uncertainty at the beginning of the hospitalization.[2, 8] Skepticism is justified, because the work involved in completing the prediction rule might be incompatible with existing workflow. A patient might not be scored if the emergency physician lacks time or if technical issues arise with the information system and computation process.[9] There is also a generic concern that the predictions will prove to be less accurate outside of the original study population.[8, 9, 10] A more specific concern for our rule is how well present on admission diagnoses can be determined during the relatively short emergency department or presurgery evaluation period. For example, a final diagnosis of heart failure might not be established until later in the hospitalization, after the results of diagnostic testing and clinical response to treatment are known. Moreover, our retrospective prediction rule requires an assessment of the presence or absence of sepsis and respiratory failure. These diagnoses appear to be susceptible to secular trends in medical record coding practices, suggesting the rule's accuracy might not be stable over time.[11]
We report the feasibility of having emergency physicians and the surgical preparation center team generate mortality predictions before an inpatient bed is assigned. We evaluate and report the accuracy of these prospective predictions.
METHODS
The study population consisted of all patients 18 years of age or less than 100 years who were admitted from the emergency department or assigned an inpatient bed following elective surgery at a tertiary, community teaching hospital in the Midwestern United States from September 1, 2012 through February 15, 2013. Although patients entering the hospital from these 2 pathways would be expected to have different levels of mortality risk, we used the original prediction rule for both because such distinctions were not made in its derivation and validation. Patients were not considered if they were admitted for childbirth or other obstetrical reasons, admitted directly from physician offices, the cardiac catheterization laboratory, hemodialysis unit, or from another hospital. The site institutional review board approved this study.
The implementation process began with presentations to the administrative and medical staff leadership on the accuracy of the retrospectively generated mortality predictions and risk of other adverse events.[3] The chief medical and nursing officers became project champions, secured internal funding for the technical components, and arranged to have 2 project comanagers available. A multidisciplinary task force endorsed the implementation details at biweekly meetings throughout the planning year. The leadership of the emergency department and surgical preparation center committed their colleagues to generate the predictions. The support of the emergency leadership was contingent on the completion of the entire prediction generating process in a very short time (within the time a physician could hold his/her breath). The chief medical officer, with the support of the leadership of the hospitalists and emergency physicians, made the administrative decision that a prediction must be generated prior to the assignment of a hospital room.
During the consensus‐building phase, a Web‐based application was developed to generate the predictions. Emergency physicians and surgical preparation staff were trained on the definitions of the risk factors (see Supporting Information, Appendix, in the online version of this article) and how to use the Web application. Three supporting databases were created. Each midnight, a past medical history database was updated, identifying those who had been discharged from the study hospital in the previous 365 days, and whether or not their diagnoses included atrial fibrillation, leukemia/lymphoma, metastatic cancer, cancer other than leukemia, lymphoma, cognitive disorder, or other neurological conditions (eg, Parkinson's, multiple sclerosis, epilepsy, coma, and stupor). Similarly, a clinical laboratory results database was created and updated real time through an HL7 (Health Level Seven, a standard data exchange format[12]) interface with the laboratory information system for the following tests performed in the preceding 30 days at a hospital‐affiliated facility: hemoglobin, platelet count, white blood count, serum troponin, blood urea nitrogen, serum albumin, serum lactate, arterial pH, arterial partial pressure of oxygen values. The third database, admission‐discharge‐transfer, was created and updated every 15 minutes to identify patients currently in the emergency room or scheduled for surgery. When a patient registration event was added to this database, the Web application created a record, retrieved all relevant data, and displayed the patient name for scoring. When the decision for hospitalization was made, the clinician selected the patient's name and reviewed the pre‐populated medical diagnoses of interest, which could be overwritten based on his/her own assessment (Figure 1A,B). The clinician then indicated (yes, no, or unknown) if the patient currently had or was being treated for each of the following: injury, heart failure, sepsis, respiratory failure, and whether or not the admitting service would be medicine (ie, nonsurgical, nonobstetrical). We considered unknown status to indicate the patient did not have the condition. When laboratory values were not available, a normal value was imputed using a previously developed algorithm.[3] Two additional questions, not used in the current prediction process, were answered to provide data for a future analysis: 1 concerning the change in the patient's condition while in the emergency department and the other concerning the presence of abnormal vital signs. The probability of 30‐day mortality was calculated via the Web application using the risk information supplied and the scoring weights (ie, parameter estimates) provided in the Appendices of our original publication.[3] Predictions were updated every minute as new laboratory values became available, and flagged with an alert if a more severe score resulted.
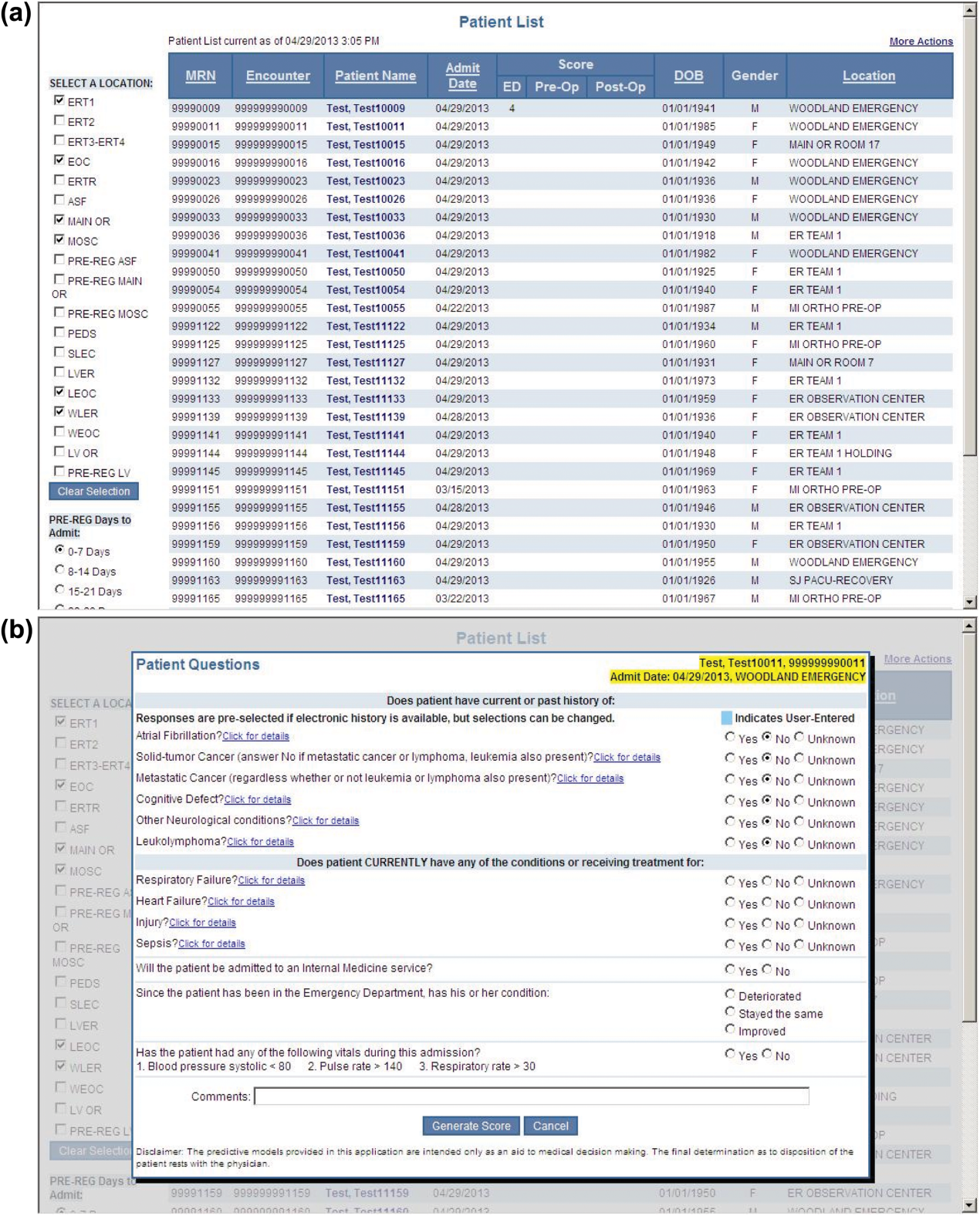
For the analyses of this study, the last prospective prediction viewed by emergency department personnel, a hospital bed manager, or surgical suite staff prior to arrival on the nursing unit is the one referenced as prospective. Once the patient had been discharged from the hospital, we generated a second mortality prediction based on previously published parameter estimates applied to risk factor data ascertained retrospectively as was done in the original article[3]; we subsequently refer to this prediction as retrospective. We will report on the group of patients who had both prospective and retrospective scores (1 patient had a prospective but not retrospective score available).
The prediction scores were made available to the clinical teams gradually during the study period. All scores were viewable by the midpoint of the study for emergency department admissions and near the end of the study for elective‐surgery patients. Only 2 changes in care processes based on level of risk were introduced during the study period. The first required initial placement of patients having a probability of dying of 0.3 or greater into an intensive or intermediate care unit unless the patient or family requested a less aggressive approach. The second occurred in the final 2 months of the study when a large multispecialty practice began routinely arranging for high‐risk patients to be seen within 3 or 7 days of hospital discharge.
Statistical Analyses
SAS version 9.3 (SAS Institute Inc., Cary, NC) was used to build the datasets and perform the analyses. Feasibility was evaluated by the number of patients who were candidates for prospective scoring with a score available at the time of admission. The validity was assessed with the primary outcome of death within 30 days from the date of hospital admission, as determined from hospital administrative data and the Social Security Death Index. The primary statistical metric is the area under the receiver operating characteristic curve (AROC) and the corresponding 95% Wald confidence limits. We needed some context for understanding the performance of the prospective predictions, assuming the accuracy could deteriorate due to the instability of the prediction rule over time and/or due to imperfect clinical information at the time the risk factors were determined. Accordingly, we also calculated an AROC based on retrospectively derived covariates (but using the same set of parameter estimates) as done in our original publication so we could gauge the stability of the original prediction rule. However, the motivation was not to determine whether retrospective versus prospective predictions were more accurate, given that only prospective predictions are useful in the context of developing real‐time care processes. Rather, we wanted to know if the prospective predictions would be sufficiently accurate for use in clinical practice. A priori, we assumed the prospective predictions should have an AROC of approximately 0.80. Therefore, a target sample size of 8660 hospitalizations was determined to be adequate to assess validity, assuming a 30‐day mortality rate of 5%, a desired lower 95% confidence boundary for the area under the prospective curve at or above 0.80, with a total confidence interval width of 0.07.[13] Calibration was assessed by comparing the actual proportion of patients dying (with 95% binomial confidence intervals) with the mean predicted mortality level within 5 percentile increments of predicted risk.
Risk Strata
We categorize the probability of 30‐day mortality into strata, with the understanding that the thresholds for defining these are a work in progress. Our hospital currently has 5 strata ranging from level 1 (highest mortality risk) to level 5 (lowest risk). The corresponding thresholds (at probabilities of death of 0.005, 0.02, 0.07, 0.20) were determined by visual inspection of the event rates and slope of curves displayed in Figure 1 of the original publication.[3]
Relationship to Secondary Clinical Outcomes of Interest
The choice of clinical care processes triggered per level of risk may be informed by understanding the frequency of events that increase with the mortality risk. We therefore examined the AROC from logistic regression models for the following outcomes using the prospectively generated probability as an explanatory variable: unplanned transfer to an ICU within the first 24 hours for patients not admitted to an ICU initially, ICU use at some point during the hospitalization, the development of a condition not present on admission (complication), receipt of palliative care by the end of the hospitalization, death during the hospitalization, 30‐day readmission, and death within 180 days. The definition of these outcomes and statistical approach used has been previously reported.[3]
RESULTS
Mortality predictions were generated on demand for 7291 out of 7777 (93.8%) eligible patients admitted from the emergency department, and for 2021 out of 2250 (89.8%) eligible elective surgical cases, for a total of 9312 predictions generated out of a possible 10,027 hospitalizations (92.9%). Table 1 displays the characteristics of the study population. The mean age was 65.2 years and 53.8% were women. The most common risk factors were atrial fibrillation (16.4%) and cancer (14.6%). Orders for a comfort care approach (rather than curative) were entered within 4 hours of admission for 32/9312 patients (0.34%), and 9/9312 (0.1%) were hospice patients on admission.
Risk Factors | No. | Without Imputation | No. | With Imputation |
---|---|---|---|---|
| ||||
Clinical laboratory values within preceding 30 days | ||||
Maximum serum blood urea nitrogen (mg/dL) | 8,484 | 22.7 (17.7) | 9,312 | 22.3 (16.9) |
Minimum hemoglobin, g/dL, | 8,750 | 12.5 (2.4) | 9,312 | 12.4 (2.4) |
Minimum platelet count, 1,000/UL | 8,737 | 224.1 (87.4) | 9,312 | 225.2 (84.7) |
Maximum white blood count, 1,000/UL | 8,750 | 10.3 (5.8) | 9,312 | 10.3 (5.6) |
Maximum serum lactate, mEq/L | 1,749 | 2.2 (1.8) | 9,312 | 0.7 (1.1) |
Minimum serum albumin, g/dL | 4,057 | 3.4 (0.7) | 9,312 | 3.2 (0.5) |
Minimum arterial pH | 509 | 7.36 (0.10) | 9,312 | 7.36 (0.02) |
Minimum arterial pO2, mm Hg | 509 | 73.6 (25.2) | 9,312 | 98.6 (8.4) |
Maximum serum troponin, ng/mL | 3,217 | 0.5 (9.3) | 9,312 | 0.2 (5.4) |
Demographics and diagnoses | ||||
Age, y | 9,312 | 65.2 (17.0) | ||
Female sex | 9,312 | 5,006 (53.8%) | ||
Previous hospitalization within past 365 days | 9,312 | 3,995 (42.9%) | ||
Emergent admission | 9,312 | 7,288 (78.3%) | ||
Admitted to a medicine service | 9,312 | 5,840 (62.7%) | ||
Current or past atrial fibrillation | 9,312 | 1,526 (16.4%) | ||
Current or past cancer without metastases, excluding leukemia or lymphoma | 9,312 | 1,356 (14.6%) | ||
Current or past history of leukemia or lymphoma | 9,312 | 145 (1.6%) | ||
Current or past metastatic cancer | 9,312 | 363 (3.9%) | ||
Current or past cognitive deficiency | 9,312 | 844 (9.1%) | ||
Current or past history of other neurological conditions (eg, Parkinson's disease, multiple sclerosis, epilepsy, coma, stupor, brain damage) | 9,312 | 952 (10.2%) | ||
Injury such as fractures or trauma at the time of admission | 9,312 | 656 (7.0%) | ||
Sepsis at the time of admission | 9,312 | 406 (4.4%) | ||
Heart failure at the time of admission | 9,312 | 776 (8.3%) | ||
Respiratory failure on admission | 9,312 | 557 (6.0%) | ||
Outcomes of interest | ||||
Unplanned transfer to an ICU (for those not admitted to an ICU) within 24 hours of admission | 8,377 | 86 (1.0%) | ||
Ever in an ICU during the hospitalization | 9,312 | 1,267 (13.6%) | ||
Development of a condition not present on admission (complication) | 9,312 | 834 (9.0%) | ||
Within hospital mortality | 9,312 | 188 (2.0%) | ||
Mortality within 30 days of admission | 9,312 | 466 (5.0%) | ||
Mortality within 180 days of admission | 9,312 | 1,070 (11.5%) | ||
Receipt of palliative care by the end of the hospitalization | 9,312 | 314 (3.4%) | ||
Readmitted to the hospital within 30 days of discharge (patients alive at discharge) | 9,124 | 1,302 (14.3%) | ||
Readmitted to the hospital within 30 days of discharge (patients alive on admission) | 9,312 | 1,302 (14.0%) |
Evaluation of Prediction Accuracy
The AROC for 30‐day mortality was 0.850 (95% confidence interval [CI]: 0.833‐0.866) for prospectively collected covariates, and 0.870 (95% CI: 0.855‐0.885) for retrospectively determined risk factors. These AROCs are not substantively different from each other, demonstrating comparable prediction performance. Calibration was excellent, as indicated in Figure 2, in which the predicted level of risk lay within the 95% confidence limits of the actual 30‐day mortality for 19 out of 20 intervals of 5 percentile increments.
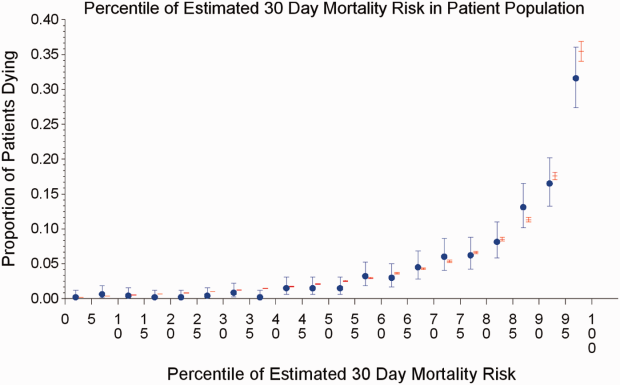
Relationship to Secondary Clinical Outcomes of Interest
The relationship between the prospectively generated probability of dying within 30 days and other events is quantified by the AROC displayed in Table 2. The 30‐day mortality risk has a strong association with the receipt of palliative care by hospital discharge, in‐hospital mortality, and 180‐day mortality, a fair association with the risk for 30‐day readmissions and unplanned transfers to intensive care, and weak associations with receipt of intensive unit care ever within the hospitalization or the development of a new diagnosis that was not present on admission (complication). The frequency of these events per mortality risk strata is shown in Table 3. The level 1 stratum contains a higher frequency of these events, whereas the level 5 stratum contains relatively few, reflecting the Pareto principle by which a relatively small proportion of patients contribute a disproportionate frequency of the events of interest.
| |
In‐hospital mortality | 0.841 (0.8140.869) |
180day mortality | 0.836 (0.8250.848) |
Receipt of palliative care by discharge | 0.875 (0.8580.891) |
30day readmission (patients alive at discharge) | 0.649 (0.6340.664) |
Unplanned transfer to an ICU (for those not admitted to an ICU) within 24 hours | 0.643 (0.5900.696) |
Ever in an ICU during the hospitalization | 0.605 (0.5880.621) |
Development of a condition not present on admission (complication) | 0.555 (0.5350.575) |
Risk Strata | 30‐Day Mortality, Count/Cases (%) | Unplanned Transfers to ICU Within 24 Hours, Count/Cases (%) | Diagnosis Not Present on Admission, Complication, Count/Cases (%) | Palliative Status at Discharge, Count/Cases (%) | Death in Hospital, Count/Cases (%) |
---|---|---|---|---|---|
Risk Strata | Ever in ICU, Count/Cases (%) | 30‐Day Readmission, Count/Cases (%) | Death or Readmission Within 30 Days, Count/Cases (%) | 180‐Day Mortality, Count/Cases (%) | |
| |||||
1 | 155/501 (30.9%) | 6/358 (1.7%) | 58/501 (11.6%) | 110/501 (22.0%) | 72/501 (14.4%) |
2 | 166/1,316 (12.6%) | 22/1,166 (1.9%) | 148/1,316 (11.3%) | 121/1,316 (9.2%) | 58/1,316 (4.4%) |
3 | 117/2,977 (3.9%) | 35/2,701 (1.3%) | 271/2,977 (9.1%) | 75/2,977 (2.5%) | 43/2,977 (1.4%) |
4 | 24/3,350 (0.7%) | 20/3,042 (0.7%) | 293/3,350 (8.8%) | 6/3,350 (0.2%) | 13/3,350 (0.4%) |
5 | 4/1,168 (0.3%) | 3/1,110 (0.3%) | 64/1,168 (5.5%) | 2/1,168 (0.2%) | 2/1,168 (0.2%) |
Total | 466/9,312 (5.0%) | 86/8,377 (1.0%) | 834/9,312 (9.0%) | 314/9,312 (3.4%) | 188/9,312 (2.0%) |
1 | 165/501 (32.9%) | 106/429 (24.7%) | 243/501 (48.5%) | 240/501 (47.9%) | |
2 | 213/1,316 (16.2%) | 275/1,258 (21.9%) | 418/1,316 (31.8%) | 403/1,316 (30.6%) | |
3 | 412/2,977 (13.8%) | 521/2,934 (17.8%) | 612/2,977 (20.6%) | 344/2,977 (11.6%) | |
4 | 406/3,350 (12.1%) | 348/3,337 (10.4%) | 368/3,350 (11.0%) | 77/3,350 (2.3%) | |
5 | 71/1,168 (6.1%) | 52/1,166 (4.5%) | 56/1,168 (4.8%) | 6/1,168 (0.5%) | |
Total | 1,267/9,312 (13.6%) | 1,302/9,124 (14.3%) | 1,697/9,312 (18.2%) | 1,070/9,312 (11.5%) |
DISCUSSION
Emergency physicians and surgical preparation center nurses generated predictions by the time of hospital admission for over 90% of the target population during usual workflow, without the addition of staff or resources. The discrimination of the prospectively generated predictions was very good to excellent, with an AROC of 0.850 (95% CI: 0.833‐0.866), similar to that obtained from the retrospective version. Calibration was excellent. The prospectively calculated mortality risk was associated with a number of other events. As shown in Table 3, the differing frequency of events within the risk strata support the development of differing intensities of multidisciplinary strategies according to the level of risk.[5] Our study provides useful experience for others who anticipate generating real‐time predictions. We consider the key reasons for success to be the considerable time spent achieving consensus, the technical development of the Web application, the brief clinician time required for the scoring process, the leadership of the chief medical and nursing officers, and the requirement that a prediction be generated before assignment of a hospital room.
Our study has a number of limitations, some of which were noted in our original publication, and although still relevant, will not be repeated here for space considerations. This is a single‐site study that used a prediction rule developed by the same site, albeit on a patient population 4 to 5 years earlier. It is not known how well the specific rule might perform in other hospital populations; any such use should therefore be accompanied by independent validation studies prior to implementation. Our successful experience should motivate future validation studies. Second, because the prognoses of patients scored from the emergency department are likely to be worse than those of elective surgery patients, our rule should be recalibrated for each subgroup separately. We plan to do this in the near future, as well as consider additional risk factors. Third, the other events of interest might be predicted more accurately if rules specifically developed for each were deployed. The mortality risk by itself is unlikely to be a sufficiently accurate predictor, particularly for complications and intensive care use, for reasons outlined in our original publication.[3] However, the varying levels of events within the higher versus lower strata should be noted by the clinical team as they design their team‐based processes. A follow‐up visit with a physician within a few days of discharge could address the concurrent risk of dying as well as readmission, for example. Finally, it is too early to determine if the availability of mortality predictions from this rule will benefit patients.[2, 8, 10] During the study period, we implemented only 2 new care processes based on the level of risk. This lack of interventions allowed us to evaluate the prediction accuracy with minimal additional confounding, but at the expense of not yet knowing the clinical impact of this work. After the study period, we implemented a number of other interventions and plan on evaluating their effectiveness in the future. We are also considering an evaluation of the potential information gained by updating the predictions throughout the course of the hospitalization.[14]
In conclusion, it is feasible to have a reasonably accurate prediction of mortality risk for most adult patients at the beginning of their hospitalizations. The availability of this prognostic information provides an opportunity to develop proactive care plans for high‐ and low‐risk subsets of patients.
Acknowledgements
The authors acknowledge the technical assistance of Nehal Sanghvi and Ben Sutton in the development of the Web application and related databases, and the support of the Chief Nursing Officer, Joyce Young, RN, PhD, the emergency department medical staff, Mohammad Salameh, MD, David Vandenberg, MD, and the surgical preparation center staff.
Disclosure: Nothing to report.
- Multifactorial index of cardiac risk in noncardiac surgical procedures. N Engl J Med. 1977;297:845–850. , , , et al.
- Methodological standards for the development of clinical decision rules in emergency medicine. Ann Emerg Med. 1999;33:437–447. , .
- Mortality predictions on admission as a context for organizing care activities. J Hosp Med. 2013;8:229–235. , , , , .
- The simple clinical score predicts mortality for 30 days after admission to an acute medical unit. QJM. 2006;99:771–781. , .
- Allocating scare resources in real‐time to reduce heart failure readmissions: a prospective, controlled study. BMJ Qual Saf. 2013;22:998–1005. , , , et al.
- Interventions to decrease hospital readmissions: keys for cost‐effectiveness. JAMA Intern Med. 2013;173:695–698. , .
- A validated value‐based model to improve hospital‐wide perioperative outcomes. Ann Surg. 2010;252:486–498. , , , et.al.
- Why is a good clinical prediction rule so hard to find? Arch Intern Med. 2011;171:1701–1702. , .
- Early detection of impending physiologic deterioration among patients who are not in intensive care: development of predictive models using data from an automated electronic medical record. J Hosp Med. 2012;7(5):388–395. , , , , , .
- Predicting death: an empirical evaluation of predictive tools for mortality. Arch Intern Med. 2011;171:1721–1726. , , .
- Association of diagnostic coding with trends in hospitalizations and mortality of patients with pneumonia, 2003–2009. JAMA. 2012;307:1405–1413. , , , , .
- Health Level Seven International website. Available at: http://www.hl7.org/. Accessed June 21, 2014.
- Bounding sample size projections for the area under a ROC curve. J Stat Plan Inference. 2009;139:711–721. .
- Derivation and validation of a model to predict daily risk of death in hospital. Med Care. 2011;49:734–743. , , , , .
- Multifactorial index of cardiac risk in noncardiac surgical procedures. N Engl J Med. 1977;297:845–850. , , , et al.
- Methodological standards for the development of clinical decision rules in emergency medicine. Ann Emerg Med. 1999;33:437–447. , .
- Mortality predictions on admission as a context for organizing care activities. J Hosp Med. 2013;8:229–235. , , , , .
- The simple clinical score predicts mortality for 30 days after admission to an acute medical unit. QJM. 2006;99:771–781. , .
- Allocating scare resources in real‐time to reduce heart failure readmissions: a prospective, controlled study. BMJ Qual Saf. 2013;22:998–1005. , , , et al.
- Interventions to decrease hospital readmissions: keys for cost‐effectiveness. JAMA Intern Med. 2013;173:695–698. , .
- A validated value‐based model to improve hospital‐wide perioperative outcomes. Ann Surg. 2010;252:486–498. , , , et.al.
- Why is a good clinical prediction rule so hard to find? Arch Intern Med. 2011;171:1701–1702. , .
- Early detection of impending physiologic deterioration among patients who are not in intensive care: development of predictive models using data from an automated electronic medical record. J Hosp Med. 2012;7(5):388–395. , , , , , .
- Predicting death: an empirical evaluation of predictive tools for mortality. Arch Intern Med. 2011;171:1721–1726. , , .
- Association of diagnostic coding with trends in hospitalizations and mortality of patients with pneumonia, 2003–2009. JAMA. 2012;307:1405–1413. , , , , .
- Health Level Seven International website. Available at: http://www.hl7.org/. Accessed June 21, 2014.
- Bounding sample size projections for the area under a ROC curve. J Stat Plan Inference. 2009;139:711–721. .
- Derivation and validation of a model to predict daily risk of death in hospital. Med Care. 2011;49:734–743. , , , , .
© 2014 Society of Hospital Medicine