User login
Outcomes Following Implementation of a Hospital-Wide, Multicomponent Delirium Care Pathway
Delirium is an acute disturbance in mental status characterized by fluctuations in cognition and attention that affects more than 2.6 million hospitalized older adults in the United States annually, a rate that is expected to increase as the population ages.1-4 Hospital-acquired delirium is associated with poor outcomes, including prolonged hospital length of stay (LOS), loss of independence, cognitive impairment, and even death.5-10 Individuals who develop delirium do poorly after hospital discharge and are more likely to be readmitted within 30 days.11 Approximately 30% to 40% of hospital-acquired delirium cases are preventable.10,12 However, programs designed to prevent delirium and associated complications, such as increased LOS, have demonstrated variable success.12-14 Many studies are limited by small sample sizes, lack of generalizability to different hospitalized patient populations, poor adherence, or reliance on outside funding.12,13,15-18
Delirium prevention programs face several challenges because delirium could be caused by a variety of risk factors and precipitants.19,20 Some risk factors that occur frequently among hospitalized patients can be mitigated, such as sensory impairment, immobility from physical restraints or urinary catheters, and polypharmacy.20,21 Effective delirium care pathways targeting these risk factors must be multifaceted, interdisciplinary, and interprofessional. Accurate risk assessment is critical to allocate resources to high-risk patients. Delirium affects patients in all medical and surgical disciplines, and often is underdiagnosed.19,22 Comprehensive screening is necessary to identify cases early and track outcomes, and educational efforts must reach all providers in the hospital. These challenges require a systematic, pragmatic approach to change.
The purpose of this study was to evaluate the association between a delirium care pathway and clinical outcomes for hospitalized patients. We hypothesized that this program would be associated with reduced hospital LOS, with secondary benefits to hospitalization costs, odds of 30-day readmission, and delirium rates.
METHODS
Study Design
In this retrospective cohort study, we compared clinical outcomes the year before and after implementation of a delirium care pathway across seven hospital units. The study period spanned October 1, 2015, through February 28, 2019. The study was approved by the University of California, San Francisco Institutional Review Board (#13-12500).
Multicomponent Delirium Care Pathway
The delirium care pathway was developed collaboratively among geriatrics, hospital medicine, neurology, anesthesiology, surgery, and psychiatry services, with an interprofessional team of physicians, nurses, pharmacists, and physical and occupational therapists. This pathway was implemented in units consecutively, approximately every 4 months in the following order: neurosciences, medicine, cardiology, general surgery, specialty surgery, hematology-oncology, and transplant. The same implementation education protocols were performed in each unit. The pathway consisted of several components targeting delirium prevention and management (Appendix Figure 1 and Appendix Figure 2). Systematic screening for delirium was introduced as part of the multicomponent intervention. Nursing staff assessed each patient’s risk of developing delirium at admission using the AWOL score, a validated delirium prediction tool.23 AWOL consists of: patient Age, spelling “World” backwards correctly, Orientation, and assessment of iLlness severity by the nurse. For patients who spoke a language other than English, spelling of “world” backwards was translated to his or her primary language, or if this was not possible, the task was modified to serial 7s (subtracting 7 from 100 in a serial fashion). This modification has been validated for use in other languages.24 Patients at high risk for delirium based on an AWOL score ≥2 received a multidisciplinary intervention with four components: (1) notifying the primary team by pager and electronic medical record (EMR), (2) a nurse-led, evidence-based, nonpharmacologic multicomponent intervention,25 (3) placement of a delirium order set by the physician, and (4) review of medications by the unit pharmacist who adjusted administration timing to occur during waking hours and placed a note in the EMR notifying the primary team of potentially deliriogenic medications. The delirium order set reinforced the nonpharmacologic multicomponent intervention through a nursing order, placed an automatic consult to occupational therapy, and included options to order physical therapy, order speech/language therapy, obtain vital signs three times daily with minimal night interruptions, remove an indwelling bladder catheter, and prescribe melatonin as a sleep aid.
The bedside nurse screened all patients for active delirium every 12-hour shift using the Nursing Delirium Screening Scale (NuDESC) and entered the results into the EMR.23,26 Capturing NuDESC results in the EMR allowed communication across medical providers as well as monitoring of screening adherence. Each nurse received two in-person trainings in staff meetings and one-to-one instruction during the first week of implementation. All nurses were required to complete a 15-minute training module and had the option of completing an additional 1-hour continuing medical education module. If a patient was transferred to the intensive care unit (ICU), delirium was identified through use of the ICU-specific Confusion Assessment Method (CAM-ICU) assessments, which the bedside nurse performed each shift throughout the intervention period.27 Nurses were instructed to call the primary team physician after every positive screen. Before each unit’s implementation start date, physicians with patients on that unit received education through a combination of grand rounds, resident lectures and seminars, and a pocket card on delirium evaluation and management.
Participants and Eligibility Criteria
We included all patients aged ≥50 years hospitalized for >1 day on each hospital unit (Figure). We included adults aged ≥50 years to maximize the number of participants for this study while also capturing a population at risk for delirium. Because the delirium care pathway was unit-based and the pathway was rolled out sequentially across units, only patients who were admitted to and discharged from the same unit were included to better isolate the effect of the pathway. Patients who were transferred to the ICU were only included if they were discharged from the original unit of admission. Only the first hospitalization was included for patients with multiple hospitalizations during the study period.
Patient Characteristics
Patient demographics and clinical data were collected after discharge through Clarity and Vizient electronic databases (Table 1 and Table 2). All Elixhauser comorbidities were included except for the following International Classification of Disease, Tenth Revision, Clinical Modification (ICD-10) codes that overlapped with a delirium diagnosis: G31.2, G93.89, G93.9, G94, R41.0, and R41.82 (Appendix Table 1). Severity of illness was obtained from Vizient, which calculates illness severity based on clinical and claims data (Appendix Table 1).
Delirium Metrics
Delirium screening was introduced as part of the multicomponent intervention, and therefore delirium rates before the intervention could not be determined. Trends in delirium prevalence and incidence after the intervention are reported. Prevalent delirium was defined as a single score of ≥2 on the nurse-administered NuDESC or a positive CAM-ICU at any point during the hospital stay. Incident delirium was identified if the first NuDESC score was negative and any subsequent NuDESC or CAM-ICU score was positive.
Outcomes
The primary study outcome was hospital LOS across all participants. Secondary outcomes included total direct cost and odds of 30-day hospital readmission. Readmissions tracked as part of hospital quality reporting were obtained from Vizient and were not captured if they occurred at another hospital. We also examined rates of safety attendant and restraint use during the study period, defined as the number of safety attendant days or restraint days per 1,000 patient days.
Because previous studies have demonstrated the effectiveness of multicomponent delirium interventions among elderly general medical patients,12 we also investigated these same outcomes in the medicine unit alone.
Statistical Analysis
The date of intervention implementation was determined for each hospital unit, which was defined as time(0) [t(0)]. The 12-month postintervention period was divided into four 3-month epochs to assess for trends. Data were aggregated across the seven units using t(0) as the start date, agnostic to the calendar month. Demographic and clinical characteristics were collected for the 12-months before t(0) and the four 3-month epochs after t(0). Univariate analysis of outcome variables comparing trends across the same epochs were conducted in the same manner, except for the rate of delirium, which was measured after t(0) and therefore could not be compared with the preintervention period.
Multivariable models were adjusted for age, sex, race/ethnicity, admission category, Elixhauser comorbidities, severity of illness quartile, and number days spent in the ICU. Admission category referred to whether the admission was emergent, urgent, or elective/unknown. Because it took 3 months after t(0) for each unit to reach a delirium screening compliance rate of 90%, the intervention was only considered fully implemented after this period. A ramp-up variable was set to 0 for admissions occurring prior to the intervention to t(0), 1/3 for admissions occurring 1 month post intervention, 2/3 for 2 months post intervention, and 1 for admissions occurring 3 to 12 months post intervention. In this way, the coefficient for the ramp-up variable estimated the postintervention versus preintervention effect. Numerical outcomes (LOS, cost) were log transformed to reduce skewness and analyzed using linear models. Coefficients were back-transformed to provide interpretations as proportional change in the median outcomes.
For LOS and readmission, we assessed secular trends by including admission date and admission date squared, in case the trend was nonlinear, as possible predictors; admission date was the specific date—not time from t(0)—to account for secular trends and allow contemporaneous controls in the analysis. To be conservative, we retained secular terms (first considering the quadratic and then the linear) if P <.10. The categorical outcome (30-day readmission) was analyzed using a logistic model. Count variables (delirium, safety attendants, restraints) were analyzed using Poisson regression models with a log link, and coefficients were back-transformed to provide rate ratio interpretations. Because delirium was not measured before t(0), and because the intervention was considered to take 3 months to become fully effective, baseline delirium rates were defined as those in the first 3 months adjusted by the ramp-up variable. For each outcome we included hospital unit, a ramp-up variable (measuring the pre- vs postintervention effect), and their interaction. If there was no statistically significant interaction, we presented the outcome for all units combined. If the interaction was statistically significant, we looked for consistency across units and reported results for all units combined when consistent, along with site-specific results. If the results were not consistent across the units, we provided site-specific results only. All statistical analyses were performed using SAS software, version 9.4 (SAS Institute Inc).
RESULTS
Participant Demographics and Clinical Characteristics
A total of 22,708 individuals were included in this study, with 11,018 in the preintervention period (Table 1 and Table 2). Most patients were cared for on the general surgery unit (n = 5,899), followed by the medicine unit (n = 4,923). The smallest number of patients were cared for on the hematology-oncology unit (n = 1,709). Across the five epochs, patients were of similar age and sex, and spent a similar number of days in the ICU. The population was diverse with regard to race and ethnicity; there were minor differences in admission category. There were also minor differences in severity of illness and some comorbidities between timepoints (Appendix Table 1).
Delirium Metrics
Delirium prevalence was 13.0% during the first epoch post intervention, followed by 12.0%, 11.7%, and 13.0% in the subsequent epochs (P = .91). Incident delirium occurred in 6.1% of patients during the first epoch post intervention, followed by 5.3%, 5.3%, and 5.8% in the subsequent epochs (P = .63).
Primary Outcome
Epoch-level data for LOS before and after the intervention is shown in Appendix Table 2. The mean unadjusted LOS for all units combined did not decrease after the intervention, but in the adjusted model, the mean LOS decreased by 2% after the intervention (P = .0087; Table 3).
Secondary Outcomes
The odds of 30-day readmission decreased by 14% (P = .0002) in the adjusted models for all units combined (Table 3). There was no statistically significant reduction in adjusted total direct hospitalization cost or rate of restraint use. The safety attendant results showed strong effect modification across sites; the site-specific estimates are provided in Appendix Table 3. However, the estimated values all showed reductions, and a number were large and statistically significant.
Medicine Unit Outcomes
On the medicine unit alone, we observed a statistically significant reduction in LOS of 9% after implementation of the delirium care pathway (P = .028) in the adjusted model (Table 3). There was an associated 7% proportional decrease in total direct cost (P = .0002). Reductions in 30-day readmission and safety attendant use did not remain statistically significant in the adjusted models.
DISCUSSION
Implementation of a hospital-wide multicomponent delirium care pathway was associated with reduced hospital LOS and 30-day hospital readmission in a study of 22,708 hospitalized adults at a tertiary care, university hospital in Northern California, encompassing both medical and surgical acute care patients. When evaluating general medicine patients alone, pathway implementation was associated with reductions in LOS and total direct cost. The cost savings of 7% among medical patients translates to median savings of $1,237 per hospitalization. This study—one of the largest to date examining implementation of a hospital-wide delirium care pathway—supports use of a multicomponent delirium care pathway for older adults hospitalized for a range of conditions.
Multicomponent pathways for delirium prevention and management are increasingly being used in hospital settings. The United Kingdom National Institute for Health and Care Excellence guidelines recommend delirium assessment and intervention by a multidisciplinary team within 24 hours of hospital admission for those at risk.25 These guidelines are based on evidence accumulated in clinical studies over the past 30 years suggesting that multicomponent interventions reduce incident delirium by 30% to 40% among medical and surgical patients.12,13,25,28
Although multicomponent delirium care pathways are associated with improved patient outcomes, the specific clinical benefits might vary across patient populations. Here, we found larger reductions in LOS and total direct cost among medicine patients. Medical patients might respond more robustly to nonpharmacologic multicomponent delirium interventions because of differing delirium etiologies (eg, constipation and sleep deprivation in a medical patient vs seizures or encephalitis in a neurosciences patient). Another explanation for the difference observed in total direct cost might be the inclusion of surgical units in the total study population. For example, not all hospital days are equivalent in cost for patients on a surgical unit.29 For patients requiring surgical care, most of the hospitalization cost might be incurred during the initial days of hospitalization, when there are perioperative costs; therefore, reduced LOS might have a lower economic impact.29 Multicomponent, nonpharmacologic delirium interventions encourage discontinuing restraints. As a result, one might expect a need for more frequent safety attendant use and an associated cost increase. However, we found that the estimated unit-specific values for safety attendant use showed reductions, which were large and highly statistically significant. For all units combined and the medicine unit alone, we found that the rate of restraint use decreased, although the change was not statistically significant. It is possible that some of the interventions taught to nurses and physicians as part of care pathway implementation, such as the use of family support for at-risk and delirious patients, led to a reduction in both safety attendants and restraints.
Our study had several strengths. This is one of the largest hospital-based delirium interventions studied, both in terms of its scope across seven diverse medical and surgical hospital units and the number of hospitalized patients studied. This intervention did not require additional staff or creating a specialized ward. Adherence to the pathway, as measured by risk assessment and delirium screening, was high (>90%) 3 months after implementation. This allowed for robust outcome ascertainment. The patient population’s characteristics and rates of delirium were stable over time. Because different hospital units incorporated the multicomponent delirium care pathway at different times, limiting enrollment to patients admitted and discharged from the same unit isolated the analysis to patients exposed to the pathway on each unit. This design also limited potential influence of other hospital quality improvement projects that might have occurred at the same time.
The primary limitation of this study is that screening for delirium was introduced as part of the multicomponent intervention. This decision was made to maximize buy-in from bedside nurses performing delirium screening because this addition to their workflow was explicitly linked to delirium prevention and management measures. Delirium could not be ascertained preintervention from the EMR because it is a clinical diagnosis and is coded inadequately.30 We could only measure the change in delirium metrics after implementation of the delirium care pathway. Because baseline delirium rates before the intervention were not measured systematically, conclusions about the intervention’s association with delirium metrics are limited. All other outcomes were measured before and after the intervention.
Although the comprehensive delirium screening program and high rate of adherence are a methodologic strength of this study, a second limitation is the use of the NuDESC. Our previous research demonstrated that the NuDESC has low sensitivity but high specificity and positive predictive value,26 which might underestimate delirium rates in this study. However, any underestimation should be stable over time and temporal trends should remain meaningful. This could allow more widespread study of delirium among hospitalized individuals. Because this care pathway was hospital-wide, it was important to ensure both consistency of screening and longevity of the initiative, and it was necessary to select a delirium assessment tool that was efficient and validated for nursing implementation. For these reasons, the NuDESC was an appropriate choice.
It is possible that our results could be influenced by unmeasured confounders. For example, although we incorporated Elixhauser medical comorbidities and illness severity into our model, we were unable to adjust for baseline functional status or frailty. Baseline functional status and frailty were not reliably recorded in the EMR, although these are potential confounders when investigating clinical outcomes including hospital readmission.
CONCLUSION
Implementation of a systematic, hospital-wide multicomponent delirium care pathway is associated with reductions in hospital LOS and 30-day readmission. In general medicine units, the reduction in LOS and associated cost savings were robust. These results demonstrate the feasibility and effectiveness of implementing an interprofessional, multidisciplinary multicomponent delirium care pathway through medical center funding to benefit patients and the hospital system.
Acknowledgments
The authors thank the many hospital staff members, especially the nurses, pharmacists, therapists, and patient care assistants, who helped implement the multicomponent delirium care pathway. All persons who have contributed significantly to this work are listed as authors of this work.
1. Bidwell J. Interventions for preventing delirium in hospitalized non-ICU patients: A Cochrane review summary. Int J Nurs Stud. 2017;70:142-143. https://doi.org/ 10.1016/j.ijnurstu.2016.11.010
2. Maldonado JR. Delirium in the acute care setting: characteristics, diagnosis and treatment. Crit Care Clin. 2008;24(4):657-722, vii. https://doi.org/10.1016/j.ccc.2008.05.008
3. Field RR, Wall MH. Delirium: past, present, and future. Semin Cardiothorac Vasc Anesth. 2013;17(3):170-179. https://doi.org/10.1177/1089253213476957
4. Oh ST, Park JY. Postoperative delirium. Korean J Anesthesiol. 2019;72(1):4-12. https://doi.org/10.4097/kja.d.18.00073.1
5. Francis J, Martin D, Kapoor WN. A prospective study of delirium in hospitalized elderly. JAMA. 1990;263(8):1097-1101.
6. Salluh JI, Soares M, Teles JM, et al. Delirium epidemiology in critical care (DECCA): an international study. Crit Care. 2010;14(6):R210. https://doi.org/10.1186/cc9333
7. Ely EW, Shintani A, Truman B, et al. Delirium as a predictor of mortality in mechanically ventilated patients in the intensive care unit. JAMA. 2004;291(14):1753-1762. https://doi.org/
8. McCusker J, Cole MG, Dendukuri N, Belzile E. Does delirium increase hospital stay? J Am Geriatr Soc. 2003;51(11):1539-1546. https://doi.org/10.1001/jama.291.14.1753
9. Inouye SK, Rushing JT, Foreman MD, Palmer RM, Pompei P. Does delirium contribute to poor hospital outcomes? A three-site epidemiologic study. J Gen Intern Med. 1998;13(4):234-242. https://doi.org/10.1046/j.1525-1497.1998.00073.x
10. Siddiqi N, House AO, Holmes JD. Occurrence and outcome of delirium in medical in-patients: a systematic literature review. Age Ageing. 2006;35(4):350-364. https://doi.org/10.1093/ageing/afl005
11. LaHue SC, Douglas VC, Kuo T, et al. Association between inpatient delirium and hospital readmission in patients >/= 65 years of age: a retrospective cohort study. J Hosp Med. 2019;14(4):201-206. https://doi.org/10.12788/jhm.3130
12. Hshieh TT, Yue J, Oh E, et al. Effectiveness of multicomponent nonpharmacological delirium interventions: a meta-analysis. JAMA Intern Med. 2015;175(4):512-520. https://doi.org/10.1001/jamainternmed.2014.7779
13. Inouye SK, Bogardus ST, Jr., Charpentier PA, et al. A multicomponent intervention to prevent delirium in hospitalized older patients. N Engl J Med. 1999;340(9):669-676. https://doi.org/10.1056/NEJM199903043400901
14. Marcantonio ER, Flacker JM, Wright RJ, Resnick NM. Reducing delirium after hip fracture: a randomized trial. J Am Geriatr Soc. 2001;49(5):516-522. https://doi.org/
15. Alhaidari AA, Allen-Narker RA. An evolving approach to delirium: A mixed-methods process evaluation of a hospital-wide delirium program in New Zealand. Australas J Ageing. 2017. https://doi.org/10.1046/j.1532-5415.2001.49108.x
16. Holroyd-Leduc JM, Khandwala F, Sink KM. How can delirium best be prevented and managed in older patients in hospital? CMAJ. 2010;182(5):465-470. https://doi.org/10.1503/cmaj.080519
17. Siddiqi N, Stockdale R, Britton AM, Holmes J. Interventions for preventing delirium in hospitalised patients. Cochrane Database Syst Rev. 2007(2):CD005563. https://doi.org/ 10.1002/14651858.CD005563.pub2
18. Siddiqi N, Harrison JK, Clegg A, et al. Interventions for preventing delirium in hospitalised non-ICU patients. Cochrane Database Syst Rev. 2016;3:CD005563. https://doi.org/10.1002/14651858.CD005563.pub3
19. Inouye SK, Westendorp RG, Saczynski JS. Delirium in elderly people. Lancet. 2014;383(9920):911-922. https://doi.org/10.1016/S0140-6736(13)60688-1
20. Inouye SK, Charpentier PA. Precipitating factors for delirium in hospitalized elderly persons. Predictive model and interrelationship with baseline vulnerability. JAMA. 1996;275(11):852-857.
21. LaHue SC, Liu VX. Loud and clear: sensory impairment, delirium, and functional recovery in critical illness. Am J Respir Crit Care Med. 2016;194(3):252-253. https://doi.org/10.1164/rccm.201602-0372ED
22. Ritter SRF, Cardoso AF, Lins MMP, Zoccoli TLV, Freitas MPD, Camargos EF. Underdiagnosis of delirium in the elderly in acute care hospital settings: lessons not learned. Psychogeriatrics. 2018;18(4):268-275. https://doi.org/10.1111/psyg.12324
23. Douglas VC, Hessler CS, Dhaliwal G, et al. The AWOL tool: derivation and validation of a delirium prediction rule. J Hosp Med. 2013;8(9):493-499. https://doi.org/10.1002/jhm.2062
24. Tombaugh TN, McDowell I, Kristjansson B, Hubley AM. Mini-Mental State Examination (MMSE) and the modified MMSE (3MS): A psychometric comparison and normative data. Psychol Assessment. 1996;8(1):48-59. https://doi.org/10.1037/1040-3590.8.1.48
25. Young J, Murthy L, Westby M, Akunne A, O’Mahony R, Guideline Development Group. Diagnosis, prevention, and management of delirium: summary of NICE guidance. BMJ. 2010;341:c3704. https://doi.org/10.1136/bmj.c3704
26. Hargrave A, Bastiaens J, Bourgeois JA, et al. Validation of a nurse-based delirium-screening tool for hospitalized patients. Psychosomatics. 2017;58(6):594-603. https://doi.org/10.1016/j.psym.2017.05.005
27. Ely EW, Inouye SK, Bernard GR, et al. Delirium in mechanically ventilated patients: validity and reliability of the confusion assessment method for the intensive care unit (CAM-ICU). JAMA. 2001;286(21):2703-2710. https://doi.org/10.1001/jama.286.21.2703
28. Strijbos MJ, Steunenberg B, van der Mast RC, Inouye SK, Schuurmans MJ. Design and methods of the Hospital Elder Life Program (HELP), a multicomponent targeted intervention to prevent delirium in hospitalized older patients: efficacy and cost-effectiveness in Dutch health care. BMC Geriatr. 2013;13:78. https://doi.org/10.1186/1471-2318-13-78
29. Taheri PA, Butz DA, Greenfield LJ. Length of stay has minimal impact on the cost of hospital admission. J Am Coll Surg. 2000;191(2):123-130. https://doi.org/10.1016/s1072-7515(00)00352-5
30. Fong TG, Tulebaev SR, Inouye SK. Delirium in elderly adults: diagnosis, prevention and treatment. Nat Rev Neurol. 2009;5(4):210-220. https://doi.org/10.1038/nrneurol.2009.24
Delirium is an acute disturbance in mental status characterized by fluctuations in cognition and attention that affects more than 2.6 million hospitalized older adults in the United States annually, a rate that is expected to increase as the population ages.1-4 Hospital-acquired delirium is associated with poor outcomes, including prolonged hospital length of stay (LOS), loss of independence, cognitive impairment, and even death.5-10 Individuals who develop delirium do poorly after hospital discharge and are more likely to be readmitted within 30 days.11 Approximately 30% to 40% of hospital-acquired delirium cases are preventable.10,12 However, programs designed to prevent delirium and associated complications, such as increased LOS, have demonstrated variable success.12-14 Many studies are limited by small sample sizes, lack of generalizability to different hospitalized patient populations, poor adherence, or reliance on outside funding.12,13,15-18
Delirium prevention programs face several challenges because delirium could be caused by a variety of risk factors and precipitants.19,20 Some risk factors that occur frequently among hospitalized patients can be mitigated, such as sensory impairment, immobility from physical restraints or urinary catheters, and polypharmacy.20,21 Effective delirium care pathways targeting these risk factors must be multifaceted, interdisciplinary, and interprofessional. Accurate risk assessment is critical to allocate resources to high-risk patients. Delirium affects patients in all medical and surgical disciplines, and often is underdiagnosed.19,22 Comprehensive screening is necessary to identify cases early and track outcomes, and educational efforts must reach all providers in the hospital. These challenges require a systematic, pragmatic approach to change.
The purpose of this study was to evaluate the association between a delirium care pathway and clinical outcomes for hospitalized patients. We hypothesized that this program would be associated with reduced hospital LOS, with secondary benefits to hospitalization costs, odds of 30-day readmission, and delirium rates.
METHODS
Study Design
In this retrospective cohort study, we compared clinical outcomes the year before and after implementation of a delirium care pathway across seven hospital units. The study period spanned October 1, 2015, through February 28, 2019. The study was approved by the University of California, San Francisco Institutional Review Board (#13-12500).
Multicomponent Delirium Care Pathway
The delirium care pathway was developed collaboratively among geriatrics, hospital medicine, neurology, anesthesiology, surgery, and psychiatry services, with an interprofessional team of physicians, nurses, pharmacists, and physical and occupational therapists. This pathway was implemented in units consecutively, approximately every 4 months in the following order: neurosciences, medicine, cardiology, general surgery, specialty surgery, hematology-oncology, and transplant. The same implementation education protocols were performed in each unit. The pathway consisted of several components targeting delirium prevention and management (Appendix Figure 1 and Appendix Figure 2). Systematic screening for delirium was introduced as part of the multicomponent intervention. Nursing staff assessed each patient’s risk of developing delirium at admission using the AWOL score, a validated delirium prediction tool.23 AWOL consists of: patient Age, spelling “World” backwards correctly, Orientation, and assessment of iLlness severity by the nurse. For patients who spoke a language other than English, spelling of “world” backwards was translated to his or her primary language, or if this was not possible, the task was modified to serial 7s (subtracting 7 from 100 in a serial fashion). This modification has been validated for use in other languages.24 Patients at high risk for delirium based on an AWOL score ≥2 received a multidisciplinary intervention with four components: (1) notifying the primary team by pager and electronic medical record (EMR), (2) a nurse-led, evidence-based, nonpharmacologic multicomponent intervention,25 (3) placement of a delirium order set by the physician, and (4) review of medications by the unit pharmacist who adjusted administration timing to occur during waking hours and placed a note in the EMR notifying the primary team of potentially deliriogenic medications. The delirium order set reinforced the nonpharmacologic multicomponent intervention through a nursing order, placed an automatic consult to occupational therapy, and included options to order physical therapy, order speech/language therapy, obtain vital signs three times daily with minimal night interruptions, remove an indwelling bladder catheter, and prescribe melatonin as a sleep aid.
The bedside nurse screened all patients for active delirium every 12-hour shift using the Nursing Delirium Screening Scale (NuDESC) and entered the results into the EMR.23,26 Capturing NuDESC results in the EMR allowed communication across medical providers as well as monitoring of screening adherence. Each nurse received two in-person trainings in staff meetings and one-to-one instruction during the first week of implementation. All nurses were required to complete a 15-minute training module and had the option of completing an additional 1-hour continuing medical education module. If a patient was transferred to the intensive care unit (ICU), delirium was identified through use of the ICU-specific Confusion Assessment Method (CAM-ICU) assessments, which the bedside nurse performed each shift throughout the intervention period.27 Nurses were instructed to call the primary team physician after every positive screen. Before each unit’s implementation start date, physicians with patients on that unit received education through a combination of grand rounds, resident lectures and seminars, and a pocket card on delirium evaluation and management.
Participants and Eligibility Criteria
We included all patients aged ≥50 years hospitalized for >1 day on each hospital unit (Figure). We included adults aged ≥50 years to maximize the number of participants for this study while also capturing a population at risk for delirium. Because the delirium care pathway was unit-based and the pathway was rolled out sequentially across units, only patients who were admitted to and discharged from the same unit were included to better isolate the effect of the pathway. Patients who were transferred to the ICU were only included if they were discharged from the original unit of admission. Only the first hospitalization was included for patients with multiple hospitalizations during the study period.
Patient Characteristics
Patient demographics and clinical data were collected after discharge through Clarity and Vizient electronic databases (Table 1 and Table 2). All Elixhauser comorbidities were included except for the following International Classification of Disease, Tenth Revision, Clinical Modification (ICD-10) codes that overlapped with a delirium diagnosis: G31.2, G93.89, G93.9, G94, R41.0, and R41.82 (Appendix Table 1). Severity of illness was obtained from Vizient, which calculates illness severity based on clinical and claims data (Appendix Table 1).
Delirium Metrics
Delirium screening was introduced as part of the multicomponent intervention, and therefore delirium rates before the intervention could not be determined. Trends in delirium prevalence and incidence after the intervention are reported. Prevalent delirium was defined as a single score of ≥2 on the nurse-administered NuDESC or a positive CAM-ICU at any point during the hospital stay. Incident delirium was identified if the first NuDESC score was negative and any subsequent NuDESC or CAM-ICU score was positive.
Outcomes
The primary study outcome was hospital LOS across all participants. Secondary outcomes included total direct cost and odds of 30-day hospital readmission. Readmissions tracked as part of hospital quality reporting were obtained from Vizient and were not captured if they occurred at another hospital. We also examined rates of safety attendant and restraint use during the study period, defined as the number of safety attendant days or restraint days per 1,000 patient days.
Because previous studies have demonstrated the effectiveness of multicomponent delirium interventions among elderly general medical patients,12 we also investigated these same outcomes in the medicine unit alone.
Statistical Analysis
The date of intervention implementation was determined for each hospital unit, which was defined as time(0) [t(0)]. The 12-month postintervention period was divided into four 3-month epochs to assess for trends. Data were aggregated across the seven units using t(0) as the start date, agnostic to the calendar month. Demographic and clinical characteristics were collected for the 12-months before t(0) and the four 3-month epochs after t(0). Univariate analysis of outcome variables comparing trends across the same epochs were conducted in the same manner, except for the rate of delirium, which was measured after t(0) and therefore could not be compared with the preintervention period.
Multivariable models were adjusted for age, sex, race/ethnicity, admission category, Elixhauser comorbidities, severity of illness quartile, and number days spent in the ICU. Admission category referred to whether the admission was emergent, urgent, or elective/unknown. Because it took 3 months after t(0) for each unit to reach a delirium screening compliance rate of 90%, the intervention was only considered fully implemented after this period. A ramp-up variable was set to 0 for admissions occurring prior to the intervention to t(0), 1/3 for admissions occurring 1 month post intervention, 2/3 for 2 months post intervention, and 1 for admissions occurring 3 to 12 months post intervention. In this way, the coefficient for the ramp-up variable estimated the postintervention versus preintervention effect. Numerical outcomes (LOS, cost) were log transformed to reduce skewness and analyzed using linear models. Coefficients were back-transformed to provide interpretations as proportional change in the median outcomes.
For LOS and readmission, we assessed secular trends by including admission date and admission date squared, in case the trend was nonlinear, as possible predictors; admission date was the specific date—not time from t(0)—to account for secular trends and allow contemporaneous controls in the analysis. To be conservative, we retained secular terms (first considering the quadratic and then the linear) if P <.10. The categorical outcome (30-day readmission) was analyzed using a logistic model. Count variables (delirium, safety attendants, restraints) were analyzed using Poisson regression models with a log link, and coefficients were back-transformed to provide rate ratio interpretations. Because delirium was not measured before t(0), and because the intervention was considered to take 3 months to become fully effective, baseline delirium rates were defined as those in the first 3 months adjusted by the ramp-up variable. For each outcome we included hospital unit, a ramp-up variable (measuring the pre- vs postintervention effect), and their interaction. If there was no statistically significant interaction, we presented the outcome for all units combined. If the interaction was statistically significant, we looked for consistency across units and reported results for all units combined when consistent, along with site-specific results. If the results were not consistent across the units, we provided site-specific results only. All statistical analyses were performed using SAS software, version 9.4 (SAS Institute Inc).
RESULTS
Participant Demographics and Clinical Characteristics
A total of 22,708 individuals were included in this study, with 11,018 in the preintervention period (Table 1 and Table 2). Most patients were cared for on the general surgery unit (n = 5,899), followed by the medicine unit (n = 4,923). The smallest number of patients were cared for on the hematology-oncology unit (n = 1,709). Across the five epochs, patients were of similar age and sex, and spent a similar number of days in the ICU. The population was diverse with regard to race and ethnicity; there were minor differences in admission category. There were also minor differences in severity of illness and some comorbidities between timepoints (Appendix Table 1).
Delirium Metrics
Delirium prevalence was 13.0% during the first epoch post intervention, followed by 12.0%, 11.7%, and 13.0% in the subsequent epochs (P = .91). Incident delirium occurred in 6.1% of patients during the first epoch post intervention, followed by 5.3%, 5.3%, and 5.8% in the subsequent epochs (P = .63).
Primary Outcome
Epoch-level data for LOS before and after the intervention is shown in Appendix Table 2. The mean unadjusted LOS for all units combined did not decrease after the intervention, but in the adjusted model, the mean LOS decreased by 2% after the intervention (P = .0087; Table 3).
Secondary Outcomes
The odds of 30-day readmission decreased by 14% (P = .0002) in the adjusted models for all units combined (Table 3). There was no statistically significant reduction in adjusted total direct hospitalization cost or rate of restraint use. The safety attendant results showed strong effect modification across sites; the site-specific estimates are provided in Appendix Table 3. However, the estimated values all showed reductions, and a number were large and statistically significant.
Medicine Unit Outcomes
On the medicine unit alone, we observed a statistically significant reduction in LOS of 9% after implementation of the delirium care pathway (P = .028) in the adjusted model (Table 3). There was an associated 7% proportional decrease in total direct cost (P = .0002). Reductions in 30-day readmission and safety attendant use did not remain statistically significant in the adjusted models.
DISCUSSION
Implementation of a hospital-wide multicomponent delirium care pathway was associated with reduced hospital LOS and 30-day hospital readmission in a study of 22,708 hospitalized adults at a tertiary care, university hospital in Northern California, encompassing both medical and surgical acute care patients. When evaluating general medicine patients alone, pathway implementation was associated with reductions in LOS and total direct cost. The cost savings of 7% among medical patients translates to median savings of $1,237 per hospitalization. This study—one of the largest to date examining implementation of a hospital-wide delirium care pathway—supports use of a multicomponent delirium care pathway for older adults hospitalized for a range of conditions.
Multicomponent pathways for delirium prevention and management are increasingly being used in hospital settings. The United Kingdom National Institute for Health and Care Excellence guidelines recommend delirium assessment and intervention by a multidisciplinary team within 24 hours of hospital admission for those at risk.25 These guidelines are based on evidence accumulated in clinical studies over the past 30 years suggesting that multicomponent interventions reduce incident delirium by 30% to 40% among medical and surgical patients.12,13,25,28
Although multicomponent delirium care pathways are associated with improved patient outcomes, the specific clinical benefits might vary across patient populations. Here, we found larger reductions in LOS and total direct cost among medicine patients. Medical patients might respond more robustly to nonpharmacologic multicomponent delirium interventions because of differing delirium etiologies (eg, constipation and sleep deprivation in a medical patient vs seizures or encephalitis in a neurosciences patient). Another explanation for the difference observed in total direct cost might be the inclusion of surgical units in the total study population. For example, not all hospital days are equivalent in cost for patients on a surgical unit.29 For patients requiring surgical care, most of the hospitalization cost might be incurred during the initial days of hospitalization, when there are perioperative costs; therefore, reduced LOS might have a lower economic impact.29 Multicomponent, nonpharmacologic delirium interventions encourage discontinuing restraints. As a result, one might expect a need for more frequent safety attendant use and an associated cost increase. However, we found that the estimated unit-specific values for safety attendant use showed reductions, which were large and highly statistically significant. For all units combined and the medicine unit alone, we found that the rate of restraint use decreased, although the change was not statistically significant. It is possible that some of the interventions taught to nurses and physicians as part of care pathway implementation, such as the use of family support for at-risk and delirious patients, led to a reduction in both safety attendants and restraints.
Our study had several strengths. This is one of the largest hospital-based delirium interventions studied, both in terms of its scope across seven diverse medical and surgical hospital units and the number of hospitalized patients studied. This intervention did not require additional staff or creating a specialized ward. Adherence to the pathway, as measured by risk assessment and delirium screening, was high (>90%) 3 months after implementation. This allowed for robust outcome ascertainment. The patient population’s characteristics and rates of delirium were stable over time. Because different hospital units incorporated the multicomponent delirium care pathway at different times, limiting enrollment to patients admitted and discharged from the same unit isolated the analysis to patients exposed to the pathway on each unit. This design also limited potential influence of other hospital quality improvement projects that might have occurred at the same time.
The primary limitation of this study is that screening for delirium was introduced as part of the multicomponent intervention. This decision was made to maximize buy-in from bedside nurses performing delirium screening because this addition to their workflow was explicitly linked to delirium prevention and management measures. Delirium could not be ascertained preintervention from the EMR because it is a clinical diagnosis and is coded inadequately.30 We could only measure the change in delirium metrics after implementation of the delirium care pathway. Because baseline delirium rates before the intervention were not measured systematically, conclusions about the intervention’s association with delirium metrics are limited. All other outcomes were measured before and after the intervention.
Although the comprehensive delirium screening program and high rate of adherence are a methodologic strength of this study, a second limitation is the use of the NuDESC. Our previous research demonstrated that the NuDESC has low sensitivity but high specificity and positive predictive value,26 which might underestimate delirium rates in this study. However, any underestimation should be stable over time and temporal trends should remain meaningful. This could allow more widespread study of delirium among hospitalized individuals. Because this care pathway was hospital-wide, it was important to ensure both consistency of screening and longevity of the initiative, and it was necessary to select a delirium assessment tool that was efficient and validated for nursing implementation. For these reasons, the NuDESC was an appropriate choice.
It is possible that our results could be influenced by unmeasured confounders. For example, although we incorporated Elixhauser medical comorbidities and illness severity into our model, we were unable to adjust for baseline functional status or frailty. Baseline functional status and frailty were not reliably recorded in the EMR, although these are potential confounders when investigating clinical outcomes including hospital readmission.
CONCLUSION
Implementation of a systematic, hospital-wide multicomponent delirium care pathway is associated with reductions in hospital LOS and 30-day readmission. In general medicine units, the reduction in LOS and associated cost savings were robust. These results demonstrate the feasibility and effectiveness of implementing an interprofessional, multidisciplinary multicomponent delirium care pathway through medical center funding to benefit patients and the hospital system.
Acknowledgments
The authors thank the many hospital staff members, especially the nurses, pharmacists, therapists, and patient care assistants, who helped implement the multicomponent delirium care pathway. All persons who have contributed significantly to this work are listed as authors of this work.
Delirium is an acute disturbance in mental status characterized by fluctuations in cognition and attention that affects more than 2.6 million hospitalized older adults in the United States annually, a rate that is expected to increase as the population ages.1-4 Hospital-acquired delirium is associated with poor outcomes, including prolonged hospital length of stay (LOS), loss of independence, cognitive impairment, and even death.5-10 Individuals who develop delirium do poorly after hospital discharge and are more likely to be readmitted within 30 days.11 Approximately 30% to 40% of hospital-acquired delirium cases are preventable.10,12 However, programs designed to prevent delirium and associated complications, such as increased LOS, have demonstrated variable success.12-14 Many studies are limited by small sample sizes, lack of generalizability to different hospitalized patient populations, poor adherence, or reliance on outside funding.12,13,15-18
Delirium prevention programs face several challenges because delirium could be caused by a variety of risk factors and precipitants.19,20 Some risk factors that occur frequently among hospitalized patients can be mitigated, such as sensory impairment, immobility from physical restraints or urinary catheters, and polypharmacy.20,21 Effective delirium care pathways targeting these risk factors must be multifaceted, interdisciplinary, and interprofessional. Accurate risk assessment is critical to allocate resources to high-risk patients. Delirium affects patients in all medical and surgical disciplines, and often is underdiagnosed.19,22 Comprehensive screening is necessary to identify cases early and track outcomes, and educational efforts must reach all providers in the hospital. These challenges require a systematic, pragmatic approach to change.
The purpose of this study was to evaluate the association between a delirium care pathway and clinical outcomes for hospitalized patients. We hypothesized that this program would be associated with reduced hospital LOS, with secondary benefits to hospitalization costs, odds of 30-day readmission, and delirium rates.
METHODS
Study Design
In this retrospective cohort study, we compared clinical outcomes the year before and after implementation of a delirium care pathway across seven hospital units. The study period spanned October 1, 2015, through February 28, 2019. The study was approved by the University of California, San Francisco Institutional Review Board (#13-12500).
Multicomponent Delirium Care Pathway
The delirium care pathway was developed collaboratively among geriatrics, hospital medicine, neurology, anesthesiology, surgery, and psychiatry services, with an interprofessional team of physicians, nurses, pharmacists, and physical and occupational therapists. This pathway was implemented in units consecutively, approximately every 4 months in the following order: neurosciences, medicine, cardiology, general surgery, specialty surgery, hematology-oncology, and transplant. The same implementation education protocols were performed in each unit. The pathway consisted of several components targeting delirium prevention and management (Appendix Figure 1 and Appendix Figure 2). Systematic screening for delirium was introduced as part of the multicomponent intervention. Nursing staff assessed each patient’s risk of developing delirium at admission using the AWOL score, a validated delirium prediction tool.23 AWOL consists of: patient Age, spelling “World” backwards correctly, Orientation, and assessment of iLlness severity by the nurse. For patients who spoke a language other than English, spelling of “world” backwards was translated to his or her primary language, or if this was not possible, the task was modified to serial 7s (subtracting 7 from 100 in a serial fashion). This modification has been validated for use in other languages.24 Patients at high risk for delirium based on an AWOL score ≥2 received a multidisciplinary intervention with four components: (1) notifying the primary team by pager and electronic medical record (EMR), (2) a nurse-led, evidence-based, nonpharmacologic multicomponent intervention,25 (3) placement of a delirium order set by the physician, and (4) review of medications by the unit pharmacist who adjusted administration timing to occur during waking hours and placed a note in the EMR notifying the primary team of potentially deliriogenic medications. The delirium order set reinforced the nonpharmacologic multicomponent intervention through a nursing order, placed an automatic consult to occupational therapy, and included options to order physical therapy, order speech/language therapy, obtain vital signs three times daily with minimal night interruptions, remove an indwelling bladder catheter, and prescribe melatonin as a sleep aid.
The bedside nurse screened all patients for active delirium every 12-hour shift using the Nursing Delirium Screening Scale (NuDESC) and entered the results into the EMR.23,26 Capturing NuDESC results in the EMR allowed communication across medical providers as well as monitoring of screening adherence. Each nurse received two in-person trainings in staff meetings and one-to-one instruction during the first week of implementation. All nurses were required to complete a 15-minute training module and had the option of completing an additional 1-hour continuing medical education module. If a patient was transferred to the intensive care unit (ICU), delirium was identified through use of the ICU-specific Confusion Assessment Method (CAM-ICU) assessments, which the bedside nurse performed each shift throughout the intervention period.27 Nurses were instructed to call the primary team physician after every positive screen. Before each unit’s implementation start date, physicians with patients on that unit received education through a combination of grand rounds, resident lectures and seminars, and a pocket card on delirium evaluation and management.
Participants and Eligibility Criteria
We included all patients aged ≥50 years hospitalized for >1 day on each hospital unit (Figure). We included adults aged ≥50 years to maximize the number of participants for this study while also capturing a population at risk for delirium. Because the delirium care pathway was unit-based and the pathway was rolled out sequentially across units, only patients who were admitted to and discharged from the same unit were included to better isolate the effect of the pathway. Patients who were transferred to the ICU were only included if they were discharged from the original unit of admission. Only the first hospitalization was included for patients with multiple hospitalizations during the study period.
Patient Characteristics
Patient demographics and clinical data were collected after discharge through Clarity and Vizient electronic databases (Table 1 and Table 2). All Elixhauser comorbidities were included except for the following International Classification of Disease, Tenth Revision, Clinical Modification (ICD-10) codes that overlapped with a delirium diagnosis: G31.2, G93.89, G93.9, G94, R41.0, and R41.82 (Appendix Table 1). Severity of illness was obtained from Vizient, which calculates illness severity based on clinical and claims data (Appendix Table 1).
Delirium Metrics
Delirium screening was introduced as part of the multicomponent intervention, and therefore delirium rates before the intervention could not be determined. Trends in delirium prevalence and incidence after the intervention are reported. Prevalent delirium was defined as a single score of ≥2 on the nurse-administered NuDESC or a positive CAM-ICU at any point during the hospital stay. Incident delirium was identified if the first NuDESC score was negative and any subsequent NuDESC or CAM-ICU score was positive.
Outcomes
The primary study outcome was hospital LOS across all participants. Secondary outcomes included total direct cost and odds of 30-day hospital readmission. Readmissions tracked as part of hospital quality reporting were obtained from Vizient and were not captured if they occurred at another hospital. We also examined rates of safety attendant and restraint use during the study period, defined as the number of safety attendant days or restraint days per 1,000 patient days.
Because previous studies have demonstrated the effectiveness of multicomponent delirium interventions among elderly general medical patients,12 we also investigated these same outcomes in the medicine unit alone.
Statistical Analysis
The date of intervention implementation was determined for each hospital unit, which was defined as time(0) [t(0)]. The 12-month postintervention period was divided into four 3-month epochs to assess for trends. Data were aggregated across the seven units using t(0) as the start date, agnostic to the calendar month. Demographic and clinical characteristics were collected for the 12-months before t(0) and the four 3-month epochs after t(0). Univariate analysis of outcome variables comparing trends across the same epochs were conducted in the same manner, except for the rate of delirium, which was measured after t(0) and therefore could not be compared with the preintervention period.
Multivariable models were adjusted for age, sex, race/ethnicity, admission category, Elixhauser comorbidities, severity of illness quartile, and number days spent in the ICU. Admission category referred to whether the admission was emergent, urgent, or elective/unknown. Because it took 3 months after t(0) for each unit to reach a delirium screening compliance rate of 90%, the intervention was only considered fully implemented after this period. A ramp-up variable was set to 0 for admissions occurring prior to the intervention to t(0), 1/3 for admissions occurring 1 month post intervention, 2/3 for 2 months post intervention, and 1 for admissions occurring 3 to 12 months post intervention. In this way, the coefficient for the ramp-up variable estimated the postintervention versus preintervention effect. Numerical outcomes (LOS, cost) were log transformed to reduce skewness and analyzed using linear models. Coefficients were back-transformed to provide interpretations as proportional change in the median outcomes.
For LOS and readmission, we assessed secular trends by including admission date and admission date squared, in case the trend was nonlinear, as possible predictors; admission date was the specific date—not time from t(0)—to account for secular trends and allow contemporaneous controls in the analysis. To be conservative, we retained secular terms (first considering the quadratic and then the linear) if P <.10. The categorical outcome (30-day readmission) was analyzed using a logistic model. Count variables (delirium, safety attendants, restraints) were analyzed using Poisson regression models with a log link, and coefficients were back-transformed to provide rate ratio interpretations. Because delirium was not measured before t(0), and because the intervention was considered to take 3 months to become fully effective, baseline delirium rates were defined as those in the first 3 months adjusted by the ramp-up variable. For each outcome we included hospital unit, a ramp-up variable (measuring the pre- vs postintervention effect), and their interaction. If there was no statistically significant interaction, we presented the outcome for all units combined. If the interaction was statistically significant, we looked for consistency across units and reported results for all units combined when consistent, along with site-specific results. If the results were not consistent across the units, we provided site-specific results only. All statistical analyses were performed using SAS software, version 9.4 (SAS Institute Inc).
RESULTS
Participant Demographics and Clinical Characteristics
A total of 22,708 individuals were included in this study, with 11,018 in the preintervention period (Table 1 and Table 2). Most patients were cared for on the general surgery unit (n = 5,899), followed by the medicine unit (n = 4,923). The smallest number of patients were cared for on the hematology-oncology unit (n = 1,709). Across the five epochs, patients were of similar age and sex, and spent a similar number of days in the ICU. The population was diverse with regard to race and ethnicity; there were minor differences in admission category. There were also minor differences in severity of illness and some comorbidities between timepoints (Appendix Table 1).
Delirium Metrics
Delirium prevalence was 13.0% during the first epoch post intervention, followed by 12.0%, 11.7%, and 13.0% in the subsequent epochs (P = .91). Incident delirium occurred in 6.1% of patients during the first epoch post intervention, followed by 5.3%, 5.3%, and 5.8% in the subsequent epochs (P = .63).
Primary Outcome
Epoch-level data for LOS before and after the intervention is shown in Appendix Table 2. The mean unadjusted LOS for all units combined did not decrease after the intervention, but in the adjusted model, the mean LOS decreased by 2% after the intervention (P = .0087; Table 3).
Secondary Outcomes
The odds of 30-day readmission decreased by 14% (P = .0002) in the adjusted models for all units combined (Table 3). There was no statistically significant reduction in adjusted total direct hospitalization cost or rate of restraint use. The safety attendant results showed strong effect modification across sites; the site-specific estimates are provided in Appendix Table 3. However, the estimated values all showed reductions, and a number were large and statistically significant.
Medicine Unit Outcomes
On the medicine unit alone, we observed a statistically significant reduction in LOS of 9% after implementation of the delirium care pathway (P = .028) in the adjusted model (Table 3). There was an associated 7% proportional decrease in total direct cost (P = .0002). Reductions in 30-day readmission and safety attendant use did not remain statistically significant in the adjusted models.
DISCUSSION
Implementation of a hospital-wide multicomponent delirium care pathway was associated with reduced hospital LOS and 30-day hospital readmission in a study of 22,708 hospitalized adults at a tertiary care, university hospital in Northern California, encompassing both medical and surgical acute care patients. When evaluating general medicine patients alone, pathway implementation was associated with reductions in LOS and total direct cost. The cost savings of 7% among medical patients translates to median savings of $1,237 per hospitalization. This study—one of the largest to date examining implementation of a hospital-wide delirium care pathway—supports use of a multicomponent delirium care pathway for older adults hospitalized for a range of conditions.
Multicomponent pathways for delirium prevention and management are increasingly being used in hospital settings. The United Kingdom National Institute for Health and Care Excellence guidelines recommend delirium assessment and intervention by a multidisciplinary team within 24 hours of hospital admission for those at risk.25 These guidelines are based on evidence accumulated in clinical studies over the past 30 years suggesting that multicomponent interventions reduce incident delirium by 30% to 40% among medical and surgical patients.12,13,25,28
Although multicomponent delirium care pathways are associated with improved patient outcomes, the specific clinical benefits might vary across patient populations. Here, we found larger reductions in LOS and total direct cost among medicine patients. Medical patients might respond more robustly to nonpharmacologic multicomponent delirium interventions because of differing delirium etiologies (eg, constipation and sleep deprivation in a medical patient vs seizures or encephalitis in a neurosciences patient). Another explanation for the difference observed in total direct cost might be the inclusion of surgical units in the total study population. For example, not all hospital days are equivalent in cost for patients on a surgical unit.29 For patients requiring surgical care, most of the hospitalization cost might be incurred during the initial days of hospitalization, when there are perioperative costs; therefore, reduced LOS might have a lower economic impact.29 Multicomponent, nonpharmacologic delirium interventions encourage discontinuing restraints. As a result, one might expect a need for more frequent safety attendant use and an associated cost increase. However, we found that the estimated unit-specific values for safety attendant use showed reductions, which were large and highly statistically significant. For all units combined and the medicine unit alone, we found that the rate of restraint use decreased, although the change was not statistically significant. It is possible that some of the interventions taught to nurses and physicians as part of care pathway implementation, such as the use of family support for at-risk and delirious patients, led to a reduction in both safety attendants and restraints.
Our study had several strengths. This is one of the largest hospital-based delirium interventions studied, both in terms of its scope across seven diverse medical and surgical hospital units and the number of hospitalized patients studied. This intervention did not require additional staff or creating a specialized ward. Adherence to the pathway, as measured by risk assessment and delirium screening, was high (>90%) 3 months after implementation. This allowed for robust outcome ascertainment. The patient population’s characteristics and rates of delirium were stable over time. Because different hospital units incorporated the multicomponent delirium care pathway at different times, limiting enrollment to patients admitted and discharged from the same unit isolated the analysis to patients exposed to the pathway on each unit. This design also limited potential influence of other hospital quality improvement projects that might have occurred at the same time.
The primary limitation of this study is that screening for delirium was introduced as part of the multicomponent intervention. This decision was made to maximize buy-in from bedside nurses performing delirium screening because this addition to their workflow was explicitly linked to delirium prevention and management measures. Delirium could not be ascertained preintervention from the EMR because it is a clinical diagnosis and is coded inadequately.30 We could only measure the change in delirium metrics after implementation of the delirium care pathway. Because baseline delirium rates before the intervention were not measured systematically, conclusions about the intervention’s association with delirium metrics are limited. All other outcomes were measured before and after the intervention.
Although the comprehensive delirium screening program and high rate of adherence are a methodologic strength of this study, a second limitation is the use of the NuDESC. Our previous research demonstrated that the NuDESC has low sensitivity but high specificity and positive predictive value,26 which might underestimate delirium rates in this study. However, any underestimation should be stable over time and temporal trends should remain meaningful. This could allow more widespread study of delirium among hospitalized individuals. Because this care pathway was hospital-wide, it was important to ensure both consistency of screening and longevity of the initiative, and it was necessary to select a delirium assessment tool that was efficient and validated for nursing implementation. For these reasons, the NuDESC was an appropriate choice.
It is possible that our results could be influenced by unmeasured confounders. For example, although we incorporated Elixhauser medical comorbidities and illness severity into our model, we were unable to adjust for baseline functional status or frailty. Baseline functional status and frailty were not reliably recorded in the EMR, although these are potential confounders when investigating clinical outcomes including hospital readmission.
CONCLUSION
Implementation of a systematic, hospital-wide multicomponent delirium care pathway is associated with reductions in hospital LOS and 30-day readmission. In general medicine units, the reduction in LOS and associated cost savings were robust. These results demonstrate the feasibility and effectiveness of implementing an interprofessional, multidisciplinary multicomponent delirium care pathway through medical center funding to benefit patients and the hospital system.
Acknowledgments
The authors thank the many hospital staff members, especially the nurses, pharmacists, therapists, and patient care assistants, who helped implement the multicomponent delirium care pathway. All persons who have contributed significantly to this work are listed as authors of this work.
1. Bidwell J. Interventions for preventing delirium in hospitalized non-ICU patients: A Cochrane review summary. Int J Nurs Stud. 2017;70:142-143. https://doi.org/ 10.1016/j.ijnurstu.2016.11.010
2. Maldonado JR. Delirium in the acute care setting: characteristics, diagnosis and treatment. Crit Care Clin. 2008;24(4):657-722, vii. https://doi.org/10.1016/j.ccc.2008.05.008
3. Field RR, Wall MH. Delirium: past, present, and future. Semin Cardiothorac Vasc Anesth. 2013;17(3):170-179. https://doi.org/10.1177/1089253213476957
4. Oh ST, Park JY. Postoperative delirium. Korean J Anesthesiol. 2019;72(1):4-12. https://doi.org/10.4097/kja.d.18.00073.1
5. Francis J, Martin D, Kapoor WN. A prospective study of delirium in hospitalized elderly. JAMA. 1990;263(8):1097-1101.
6. Salluh JI, Soares M, Teles JM, et al. Delirium epidemiology in critical care (DECCA): an international study. Crit Care. 2010;14(6):R210. https://doi.org/10.1186/cc9333
7. Ely EW, Shintani A, Truman B, et al. Delirium as a predictor of mortality in mechanically ventilated patients in the intensive care unit. JAMA. 2004;291(14):1753-1762. https://doi.org/
8. McCusker J, Cole MG, Dendukuri N, Belzile E. Does delirium increase hospital stay? J Am Geriatr Soc. 2003;51(11):1539-1546. https://doi.org/10.1001/jama.291.14.1753
9. Inouye SK, Rushing JT, Foreman MD, Palmer RM, Pompei P. Does delirium contribute to poor hospital outcomes? A three-site epidemiologic study. J Gen Intern Med. 1998;13(4):234-242. https://doi.org/10.1046/j.1525-1497.1998.00073.x
10. Siddiqi N, House AO, Holmes JD. Occurrence and outcome of delirium in medical in-patients: a systematic literature review. Age Ageing. 2006;35(4):350-364. https://doi.org/10.1093/ageing/afl005
11. LaHue SC, Douglas VC, Kuo T, et al. Association between inpatient delirium and hospital readmission in patients >/= 65 years of age: a retrospective cohort study. J Hosp Med. 2019;14(4):201-206. https://doi.org/10.12788/jhm.3130
12. Hshieh TT, Yue J, Oh E, et al. Effectiveness of multicomponent nonpharmacological delirium interventions: a meta-analysis. JAMA Intern Med. 2015;175(4):512-520. https://doi.org/10.1001/jamainternmed.2014.7779
13. Inouye SK, Bogardus ST, Jr., Charpentier PA, et al. A multicomponent intervention to prevent delirium in hospitalized older patients. N Engl J Med. 1999;340(9):669-676. https://doi.org/10.1056/NEJM199903043400901
14. Marcantonio ER, Flacker JM, Wright RJ, Resnick NM. Reducing delirium after hip fracture: a randomized trial. J Am Geriatr Soc. 2001;49(5):516-522. https://doi.org/
15. Alhaidari AA, Allen-Narker RA. An evolving approach to delirium: A mixed-methods process evaluation of a hospital-wide delirium program in New Zealand. Australas J Ageing. 2017. https://doi.org/10.1046/j.1532-5415.2001.49108.x
16. Holroyd-Leduc JM, Khandwala F, Sink KM. How can delirium best be prevented and managed in older patients in hospital? CMAJ. 2010;182(5):465-470. https://doi.org/10.1503/cmaj.080519
17. Siddiqi N, Stockdale R, Britton AM, Holmes J. Interventions for preventing delirium in hospitalised patients. Cochrane Database Syst Rev. 2007(2):CD005563. https://doi.org/ 10.1002/14651858.CD005563.pub2
18. Siddiqi N, Harrison JK, Clegg A, et al. Interventions for preventing delirium in hospitalised non-ICU patients. Cochrane Database Syst Rev. 2016;3:CD005563. https://doi.org/10.1002/14651858.CD005563.pub3
19. Inouye SK, Westendorp RG, Saczynski JS. Delirium in elderly people. Lancet. 2014;383(9920):911-922. https://doi.org/10.1016/S0140-6736(13)60688-1
20. Inouye SK, Charpentier PA. Precipitating factors for delirium in hospitalized elderly persons. Predictive model and interrelationship with baseline vulnerability. JAMA. 1996;275(11):852-857.
21. LaHue SC, Liu VX. Loud and clear: sensory impairment, delirium, and functional recovery in critical illness. Am J Respir Crit Care Med. 2016;194(3):252-253. https://doi.org/10.1164/rccm.201602-0372ED
22. Ritter SRF, Cardoso AF, Lins MMP, Zoccoli TLV, Freitas MPD, Camargos EF. Underdiagnosis of delirium in the elderly in acute care hospital settings: lessons not learned. Psychogeriatrics. 2018;18(4):268-275. https://doi.org/10.1111/psyg.12324
23. Douglas VC, Hessler CS, Dhaliwal G, et al. The AWOL tool: derivation and validation of a delirium prediction rule. J Hosp Med. 2013;8(9):493-499. https://doi.org/10.1002/jhm.2062
24. Tombaugh TN, McDowell I, Kristjansson B, Hubley AM. Mini-Mental State Examination (MMSE) and the modified MMSE (3MS): A psychometric comparison and normative data. Psychol Assessment. 1996;8(1):48-59. https://doi.org/10.1037/1040-3590.8.1.48
25. Young J, Murthy L, Westby M, Akunne A, O’Mahony R, Guideline Development Group. Diagnosis, prevention, and management of delirium: summary of NICE guidance. BMJ. 2010;341:c3704. https://doi.org/10.1136/bmj.c3704
26. Hargrave A, Bastiaens J, Bourgeois JA, et al. Validation of a nurse-based delirium-screening tool for hospitalized patients. Psychosomatics. 2017;58(6):594-603. https://doi.org/10.1016/j.psym.2017.05.005
27. Ely EW, Inouye SK, Bernard GR, et al. Delirium in mechanically ventilated patients: validity and reliability of the confusion assessment method for the intensive care unit (CAM-ICU). JAMA. 2001;286(21):2703-2710. https://doi.org/10.1001/jama.286.21.2703
28. Strijbos MJ, Steunenberg B, van der Mast RC, Inouye SK, Schuurmans MJ. Design and methods of the Hospital Elder Life Program (HELP), a multicomponent targeted intervention to prevent delirium in hospitalized older patients: efficacy and cost-effectiveness in Dutch health care. BMC Geriatr. 2013;13:78. https://doi.org/10.1186/1471-2318-13-78
29. Taheri PA, Butz DA, Greenfield LJ. Length of stay has minimal impact on the cost of hospital admission. J Am Coll Surg. 2000;191(2):123-130. https://doi.org/10.1016/s1072-7515(00)00352-5
30. Fong TG, Tulebaev SR, Inouye SK. Delirium in elderly adults: diagnosis, prevention and treatment. Nat Rev Neurol. 2009;5(4):210-220. https://doi.org/10.1038/nrneurol.2009.24
1. Bidwell J. Interventions for preventing delirium in hospitalized non-ICU patients: A Cochrane review summary. Int J Nurs Stud. 2017;70:142-143. https://doi.org/ 10.1016/j.ijnurstu.2016.11.010
2. Maldonado JR. Delirium in the acute care setting: characteristics, diagnosis and treatment. Crit Care Clin. 2008;24(4):657-722, vii. https://doi.org/10.1016/j.ccc.2008.05.008
3. Field RR, Wall MH. Delirium: past, present, and future. Semin Cardiothorac Vasc Anesth. 2013;17(3):170-179. https://doi.org/10.1177/1089253213476957
4. Oh ST, Park JY. Postoperative delirium. Korean J Anesthesiol. 2019;72(1):4-12. https://doi.org/10.4097/kja.d.18.00073.1
5. Francis J, Martin D, Kapoor WN. A prospective study of delirium in hospitalized elderly. JAMA. 1990;263(8):1097-1101.
6. Salluh JI, Soares M, Teles JM, et al. Delirium epidemiology in critical care (DECCA): an international study. Crit Care. 2010;14(6):R210. https://doi.org/10.1186/cc9333
7. Ely EW, Shintani A, Truman B, et al. Delirium as a predictor of mortality in mechanically ventilated patients in the intensive care unit. JAMA. 2004;291(14):1753-1762. https://doi.org/
8. McCusker J, Cole MG, Dendukuri N, Belzile E. Does delirium increase hospital stay? J Am Geriatr Soc. 2003;51(11):1539-1546. https://doi.org/10.1001/jama.291.14.1753
9. Inouye SK, Rushing JT, Foreman MD, Palmer RM, Pompei P. Does delirium contribute to poor hospital outcomes? A three-site epidemiologic study. J Gen Intern Med. 1998;13(4):234-242. https://doi.org/10.1046/j.1525-1497.1998.00073.x
10. Siddiqi N, House AO, Holmes JD. Occurrence and outcome of delirium in medical in-patients: a systematic literature review. Age Ageing. 2006;35(4):350-364. https://doi.org/10.1093/ageing/afl005
11. LaHue SC, Douglas VC, Kuo T, et al. Association between inpatient delirium and hospital readmission in patients >/= 65 years of age: a retrospective cohort study. J Hosp Med. 2019;14(4):201-206. https://doi.org/10.12788/jhm.3130
12. Hshieh TT, Yue J, Oh E, et al. Effectiveness of multicomponent nonpharmacological delirium interventions: a meta-analysis. JAMA Intern Med. 2015;175(4):512-520. https://doi.org/10.1001/jamainternmed.2014.7779
13. Inouye SK, Bogardus ST, Jr., Charpentier PA, et al. A multicomponent intervention to prevent delirium in hospitalized older patients. N Engl J Med. 1999;340(9):669-676. https://doi.org/10.1056/NEJM199903043400901
14. Marcantonio ER, Flacker JM, Wright RJ, Resnick NM. Reducing delirium after hip fracture: a randomized trial. J Am Geriatr Soc. 2001;49(5):516-522. https://doi.org/
15. Alhaidari AA, Allen-Narker RA. An evolving approach to delirium: A mixed-methods process evaluation of a hospital-wide delirium program in New Zealand. Australas J Ageing. 2017. https://doi.org/10.1046/j.1532-5415.2001.49108.x
16. Holroyd-Leduc JM, Khandwala F, Sink KM. How can delirium best be prevented and managed in older patients in hospital? CMAJ. 2010;182(5):465-470. https://doi.org/10.1503/cmaj.080519
17. Siddiqi N, Stockdale R, Britton AM, Holmes J. Interventions for preventing delirium in hospitalised patients. Cochrane Database Syst Rev. 2007(2):CD005563. https://doi.org/ 10.1002/14651858.CD005563.pub2
18. Siddiqi N, Harrison JK, Clegg A, et al. Interventions for preventing delirium in hospitalised non-ICU patients. Cochrane Database Syst Rev. 2016;3:CD005563. https://doi.org/10.1002/14651858.CD005563.pub3
19. Inouye SK, Westendorp RG, Saczynski JS. Delirium in elderly people. Lancet. 2014;383(9920):911-922. https://doi.org/10.1016/S0140-6736(13)60688-1
20. Inouye SK, Charpentier PA. Precipitating factors for delirium in hospitalized elderly persons. Predictive model and interrelationship with baseline vulnerability. JAMA. 1996;275(11):852-857.
21. LaHue SC, Liu VX. Loud and clear: sensory impairment, delirium, and functional recovery in critical illness. Am J Respir Crit Care Med. 2016;194(3):252-253. https://doi.org/10.1164/rccm.201602-0372ED
22. Ritter SRF, Cardoso AF, Lins MMP, Zoccoli TLV, Freitas MPD, Camargos EF. Underdiagnosis of delirium in the elderly in acute care hospital settings: lessons not learned. Psychogeriatrics. 2018;18(4):268-275. https://doi.org/10.1111/psyg.12324
23. Douglas VC, Hessler CS, Dhaliwal G, et al. The AWOL tool: derivation and validation of a delirium prediction rule. J Hosp Med. 2013;8(9):493-499. https://doi.org/10.1002/jhm.2062
24. Tombaugh TN, McDowell I, Kristjansson B, Hubley AM. Mini-Mental State Examination (MMSE) and the modified MMSE (3MS): A psychometric comparison and normative data. Psychol Assessment. 1996;8(1):48-59. https://doi.org/10.1037/1040-3590.8.1.48
25. Young J, Murthy L, Westby M, Akunne A, O’Mahony R, Guideline Development Group. Diagnosis, prevention, and management of delirium: summary of NICE guidance. BMJ. 2010;341:c3704. https://doi.org/10.1136/bmj.c3704
26. Hargrave A, Bastiaens J, Bourgeois JA, et al. Validation of a nurse-based delirium-screening tool for hospitalized patients. Psychosomatics. 2017;58(6):594-603. https://doi.org/10.1016/j.psym.2017.05.005
27. Ely EW, Inouye SK, Bernard GR, et al. Delirium in mechanically ventilated patients: validity and reliability of the confusion assessment method for the intensive care unit (CAM-ICU). JAMA. 2001;286(21):2703-2710. https://doi.org/10.1001/jama.286.21.2703
28. Strijbos MJ, Steunenberg B, van der Mast RC, Inouye SK, Schuurmans MJ. Design and methods of the Hospital Elder Life Program (HELP), a multicomponent targeted intervention to prevent delirium in hospitalized older patients: efficacy and cost-effectiveness in Dutch health care. BMC Geriatr. 2013;13:78. https://doi.org/10.1186/1471-2318-13-78
29. Taheri PA, Butz DA, Greenfield LJ. Length of stay has minimal impact on the cost of hospital admission. J Am Coll Surg. 2000;191(2):123-130. https://doi.org/10.1016/s1072-7515(00)00352-5
30. Fong TG, Tulebaev SR, Inouye SK. Delirium in elderly adults: diagnosis, prevention and treatment. Nat Rev Neurol. 2009;5(4):210-220. https://doi.org/10.1038/nrneurol.2009.24
© 2021 Society of Hospital Medicine
Appropriate Reconciliation of Cardiovascular Medications After Elective Surgery and Postdischarge Acute Hospital and Ambulatory Visits
Medication reconciliation at hospital discharge is a critical component of the posthospital transition of care.1 Effective reconciliation involves a clear process for documenting a current medication list, identifying and resolving discrepancies, and then documenting decisions and instructions around which medications should be continued, modified, or stopped.2 Existing studies3-5 suggest that medication discrepancies are common during hospital discharge transitions of care and lead to preventable adverse drug events, patient disability, and increased healthcare utilization following hospital discharge, including physician office visits, emergency department (ED) visits, and hospitalizations.6-8
While the majority of studies of medication discrepancies have been conducted in general medical patients, few have examined how gaps in discharge medication reconciliation might affect surgical patients.9,10 Two prior studies9,10 suggest that medication discrepancies may occur more frequently for surgical patients, compared with medical patients, particularly discrepancies in reordering home medications postoperatively, raising patient safety concerns for more than 50 million patients hospitalized for surgery each year.11 In particular, little is known about the appropriate discharge reconciliation of chronic cardiovascular medications, such as beta-blockers, renin-angiotensin system inhibitors, and statins in surgical patients, despite perioperative practice guidelines recommending continuation or rapid reinitiation of these medications after noncardiac surgery.12 Problems with chronic cardiovascular medications have been implicated as major contributors to ED visits and hospitalizations for adverse drug events,13,14 further highlighting the importance of safe and appropriate management of these medications.
To better understand the current state and impact of postoperative discharge medication reconciliation of chronic cardiovascular medications in surgical patients, we examined (1) the appropriate discharge reconciliation of 4 cardiovascular medication classes, and (2) the associations between the appropriate discharge reconciliation of these medication classes and postdischarge acute hospital and ambulatory visits in patients hospitalized for elective noncardiac surgery at an academic medical center.
METHODS
Study Design and Patient Selection
We performed a retrospective analysis of data collected as part of a cohort study of hospitalized surgical patients admitted between January 2007 and December 2011. The original study was designed to assess the impact of a social marketing intervention on guideline-appropriate perioperative beta-blocker use in surgical patients. The study was conducted at 1 academic medical center that had 2 campuses with full noncardiac operative facilities and a 600-bed total capacity. Both sites had preoperative clinics, and patients were recruited by review of preoperative clinic records. Institutional review boards responsible for all sites approved the study.
For this analysis, we included adults (age >18 years) undergoing elective noncardiac surgery, who were expected to remain hospitalized for at least 1 day and were taking antiplatelet agents (aspirin, aspirin-dipyridamole, or clopidogrel), beta-blockers, renin-angiotensin system inhibitors (angiotensin-converting-enzyme inhibitors or angiotensin-receptor blockers), or statin lipid-lowering agents.
Data Collection
Data Sources. We collected data from a structured review of medical records as well as from discharge abstract information obtained from administrative data systems. Data regarding patient demographics (age, sex, and race/ethnicity), medical history, preoperative cardiovascular medications, surgical procedure and service, and attending surgeon were obtained from a medical record review of comprehensive preoperative clinic evaluations. Data regarding complications during hospitalization were obtained from medical record review and administrative data (Supplement for International Classification of Diseases, Ninth Revision codes).15 Research assistants abstracting data were trained by using a comprehensive reference manual providing specific criteria for classifying chart abstraction data. Research assistants also were directly observed during initial chart abstractions and underwent random chart validation audits by a senior investigator to ensure consistency. Any discrepancies in coding were resolved by consensus among senior investigators.
Definition of Key Predictor: Appropriate Reconciliation. We abstracted discharge medication lists from the electronic medical record. We defined the appropriate reconciliation of cardiovascular medications at discharge as documentation in discharge instructions, medication reconciliation tools, or discharge summaries that a preadmission cardiovascular medication was being continued at discharge, or, if the medication was not continued, documentation of a new contraindication to the medication or complication precluding its use during hospitalization. Medication continuity was considered appropriate if it was continued at discharge irrespective of changes in dosage. By using this measure for individual medications, we also assessed appropriate reconciliation as an “all-or-none” complete versus incomplete measure (appropriate reconciliation of all preoperative cardiovascular medication classes the patient was taking).16
Definition of Outcomes. Our coprimary outcomes were acute hospital visits (ED visits or hospitalizations) and unplanned ambulatory visits (primary care or surgical) at 30 days after surgery. Postoperative ambulatory visits that were not planned prior to surgery were defined as unplanned. Outcomes were ascertained by patient reports during follow-up telephone questionnaires administered by trained research staff and verified by medical record review.
Definition of Covariates. Using these data, we calculated a Revised Cardiac Risk Index (RCRI) score,17 which estimates the risk of perioperative cardiac complications in patients undergoing surgery. Through chart abstraction data supplemented by diagnosis codes from administrative data, we also constructed variables indicating occurrences of postoperative complications anytime during hospitalization that might pose contraindications to continuation of the 4 cardiovascular medication classes studied. For example, if a chart indicated that the patient had an acute rise in creatinine (elevation of baseline creatinine by 50% or absolute rise of 1 mg/dL in patients with baseline creatinine greater than 3 mg/dL) during hospitalization and a preoperative renin-angiotensin system inhibitor was not prescribed at discharge, we would have considered discontinuation appropriate. Other complications we abstracted were hypotension (systolic blood pressure less than 90 mmHg) for beta-blockers and renin-angiotensin system inhibitors, bradycardia (heart rate less than 50 bpm) for beta-blockers, acute kidney injury (defined above) and hyperkalemia for renin-angiotensin system inhibitors, and bleeding (any site) for antiplatelet agents.
Statistical Analysis
We used χ2 and Kruskal-Wallis tests to compare baseline patient characteristics. To assess associations between appropriate medication reconciliation and patient outcomes, we used multilevel mixed-effects logistic regression to account for the clustering of patients by the attending surgeon. We adjusted for baseline patient demographics, surgical service, the number of baseline cardiovascular medications, and individual RCRI criteria. We constructed separate models for all-or-none appropriate reconciliation and for each individual medication class.
As a sensitivity analysis, we constructed similar models by using a simplified definition of appropriate reconciliation based entirely on medication continuity (continued or not continued at discharge) without taking potential contraindications during hospitalization into account. For complete versus incomplete reconciliation, we also constructed models with an interaction term between the number of baseline cardiovascular medications and appropriate medication reconciliation to test the hypothesis that inappropriate reconciliation would be more likely with an increasing number of preoperative cardiovascular medications. Because this interaction term was not statistically significant, we did not include it in the final models for ease of reporting and interpretability. We performed all statistical analyses using Stata 14 (StataCorp, LLC, College Station, Texas), and used 2-sided statistical tests and a P value of less than .05 to define statistical significance.
RESULTS
Patient Characteristics
A total of 849 patients were enrolled, of which 752 (88.6%) were taking at least 1 of the specified cardiovascular medications in the preoperative period. Their mean age was 61.5; 50.9% were male, 72.6% were non-Hispanic white, and 89.4% had RCRI scores of 0 or 1 (Table 1). The majority (63.8%) were undergoing general surgery, orthopedic surgery, or neurosurgery procedures. In the preoperative period, 327 (43.5%) patients were taking antiplatelet agents, 624 (83.0%) were taking beta-blockers, 361 (48.0%) were taking renin-angiotensin system inhibitors, and 406 (54.0%) were taking statins (Table 2). Among patients taking antiplatelet agents, 271 (82.9%) were taking aspirin alone, 21 (6.4%) were taking clopidogrel alone, and 35 (10.7%) were taking dual antiplatelet therapy with aspirin and clopidogrel. Nearly three-quarters of the patients (551, 73.3%) were taking medications from 2 or more classes, and the proportion of patients with inappropriate reconciliation increased with the number of preoperative cardiovascular medications.
Patients with and without appropriate reconciliation of all preoperative cardiovascular medications were similar in age, sex, and race/ethnicity (Table 1). Patients with inappropriate reconciliation of at least 1 medication were more likely to be on the urology and renal/liver transplant surgical services, have higher RCRI scores, and be taking antiplatelet agents, statins, renin-angiotensin system inhibitors, and 3 or more cardiovascular medications in the preoperative period.
Appropriate Medication Reconciliation
Four hundred thirty-six patients (58.0%) had their baseline cardiovascular medications appropriately reconciled. Among all patients with appropriately reconciled medications, 1 (0.2%) had beta-blockers discontinued due to a documented episode of hypotension; 17 (3.9%) had renin-angiotensin system inhibitors discontinued due to episodes of acute kidney injury, hypotension, or hyperkalemia; and 1 (0.2%) had antiplatelet agents discontinued due to bleeding. For individual medications, appropriate reconciliation between the preoperative and discharge periods occurred for 156 of the 327 patients on antiplatelet agents (47.7%), 507 of the 624 patients on beta-blockers (81.3%), 259 of the 361 patients on renin-angiotensin system inhibitors (71.8%), and 302 of the 406 patients on statins (74.4%; Table 2).
Associations Between Medication Reconciliation and Outcomes
Thirty-day outcome data on acute hospital visits were available for 679 (90.3%) patients. Of these, 146 (21.5%) were seen in the ED or were hospitalized, and 111 (16.3%) were seen for an unplanned primary care or surgical outpatient visit at 30 days after surgery. Patients with incomplete outcome data were more likely to have complete medication reconciliation compared with those with complete outcome data (71.2% vs 56.6%, P = 0.02). As shown in Table 3, the proportion of patients with 30-day acute hospital visits was nonstatistically significantly lower in patients with complete medication reconciliation (20.8% vs 22.4%, P = 0.63) and the appropriate reconciliation of beta-blockers (21.9% vs 23.6%, P = 0.71) and renin-angiotensin system inhibitors (19.6% vs 20.0%, P = 0.93), and nonsignificantly higher with the appropriate reconciliation of antiplatelet agents (23.9% vs 19.9%, P = 0.40). Acute hospital visits were statistically significantly lower with the appropriate reconciliation of statins (17.9% vs 31.9%, P = 0.004).
Sensitivity Analysis
Overall, 430 (57.2%) patients had complete cardiovascular medication continuity without considering potential contraindications during hospitalization. Associations between medication continuity and acute hospital and ambulatory visits were similar to the primary analyses.
DISCUSSION
In this study of 752 patients hospitalized for elective noncardiac surgery, we found significant gaps in the appropriate reconciliation of commonly prescribed cardiovascular medications, with inappropriate discontinuation ranging from 18.8% to 52.3% for individual medications. Unplanned postdischarge healthcare utilization was high, with acute hospital visits documented in 21.5% of patients and unplanned ambulatory visits in 16.3% at 30 days after surgery. However, medication reconciliation gaps were not consistently associated with ED visits, hospitalizations, or unplanned ambulatory visits.
Our finding of large gaps in postoperative medication reconciliation is consistent with existing studies of medication reconciliation in surgical patients.9,10,18 One study found medication discrepancies in 40.2% of postoperative patients receiving usual care and discrepancies judged to have the potential to cause harm (such as the omission of beta-blockers) in 29.9%.9 Consistent with our findings, this study also found that most postoperative medication discrepancies were omissions in reordering home medications, though at a rate somewhat higher than those seen in medical patients at discharge.5 While hospitalization by itself increases the risk of unintentional discontinuation of chronic medications,3 our results, along with existing literature, suggest that the risk for omission of chronic medications is unacceptably high.
We also found significant variation in reconciliation among cardiovascular medications, with appropriate reconciliation occurring least frequently for antiplatelet agents and most frequently for beta-blockers. The low rates of appropriate reconciliation for antiplatelet agents may be attributable to deliberate withholding of antiplatelet therapy in the postoperative period based on clinical assessments of surgical bleeding risk in the absence of active bleeding. Perioperative management of antiplatelet agents for noncardiac surgery remains an unclear and controversial topic, which may also contribute to the variation noted.19 Conversely, beta-blockers demonstrated high rates of preoperative use (over 80% of patients) and appropriate reconciliation. Both findings are likely attributable in part to the timing of the study, which began prior to the publication of the Perioperative Ischemic Evaluation trial, which more definitively demonstrated the potential harms of perioperative beta-blocker therapy.20
Despite a high proportion of patients with discontinuous medications at discharge, we found no associations between the appropriate reconciliation of beta-blockers, renin-angiotensin system inhibitors, and antiplatelet agents and acute hospital or ambulatory visits in the first 30 days after discharge. One explanation for this discrepancy is that, although we focused on cardiovascular medications commonly implicated in acute hospital visits, the vast majority of patients in our study had low perioperative cardiovascular risk as assessed by the RCRI. Previous studies have demonstrated that the benefit of perioperative beta-blocker therapy is predominantly in patients with moderate to high perioperative cardiovascular risk.21,22 It is possible that the detrimental effects of the discontinuation of chronic cardiovascular medications are more prominent in populations at a higher risk of perioperative cardiovascular complications or that complications will occur later than 30 days after discharge. Similarly, while the benefits of continuation of renin-angiotensin system inhibitors are less clear,23 few patients in our cohort had a history of congestive heart failure (6.3%) or coronary artery disease (13.0%), 2 conditions in which the impact of perioperative discontinuation of renin-angiotensin inhibitor or beta-blocker therapy would likely be more pronounced.24,25 An additional explanation for the lack of associations is that, while multiple studies have demonstrated that medication errors are common, the proportion of errors with the potential for harm is much lower, and the proportion that causes actual harm is lower still.5,26,27 Thus, while we likely captured high-severity medication errors leading to acute hospital or unplanned ambulatory visits, we would not have captured medication errors with lower severity clinical consequences that did not result in medical encounters.
We did find an association between the continuation of statin therapy and reduced ED visits and hospitalizations. This finding is supported by previous studies of patients undergoing noncardiac surgery, including 1 demonstrating an association between immediate postoperative statin therapy and reduced in-hospital mortality28 and another study demonstrating an association between postoperative statin therapy and reductions in a composite endpoint of 30-day mortality, atrial fibrillation, and nonfatal myocardial infarction.29 Alternatively, this finding could reflect the effects of unaddressed confounding by factors contributing to statin discontinuation and poor health outcomes leading to acute hospital visits, such as acute elevations in liver enzymes.
Our study has important implications for patients undergoing elective noncardiac surgery and the healthcare providers caring for them. First, inappropriate omissions of chronic cardiovascular medications at discharge are common; clinicians should increase their general awareness and focus on appropriately reconciling these medications, for even if our results do not connect medication discontinuity to readmissions or unexpected clinical encounters, their impact on patients’ understanding of their medications remains a potential concern. Second, the overall high rates of unplanned postdischarge healthcare utilization in this study highlight the need for close postdischarge monitoring of patients undergoing elective surgical procedures and for further research to identify preventable etiologies of postdischarge healthcare utilization in this population. Third, further study is needed to identify specific patient populations and medication classes, in which appropriate reconciliation is associated with patient outcomes that may benefit from more intensive discharge medication reconciliation interventions.
Our study has limitations. First, the majority of patients in this single-center study were at low risk of perioperative cardiovascular events, and our results may not be generalizable to higher-risk patients undergoing elective surgery. Second, discharge reconciliation was based on documentation of medication reconciliation and not on patient-reported medication adherence. In addition, the ability to judge the accuracy of discharge medication reconciliation is in part dependent on the accuracy of the admission medication reconciliation. Thus, although we used preoperative medication regimens documented during preadmission visits to comprehensive preoperative clinics for comparison, discrepancies in these preoperative regimens could have affected our analysis of appropriate discharge reconciliation. Third, inadequate documentation of clinical reasons for discontinuing medications may have led to residual confounding by indication in our observational study. Finally, the outcomes available to us may have been relatively insensitive to other adverse effects of medication discontinuity, such as patient symptoms (eg, angina severity), patient awareness of medications, or work placed on primary care physicians needing to “clean up” erroneous medication lists.
In conclusion, gaps in appropriate discharge reconciliation of chronic cardiovascular medications were common but not consistently associated with postdischarge acute hospital or unplanned ambulatory visits in a relatively low-risk cohort of patients undergoing elective surgery. While appropriate medication reconciliation should always be a priority, further study is needed to identify medication reconciliation approaches associated with postdischarge healthcare utilization and other patient outcomes.
Disclosure
Dr. Lee reports receiving grant support from the Health Resources and Services Administration (T32HP19025). Dr. Vittinghoff reports receiving grant support from the Agency for Healthcare Research and Quality. Dr. Auerbach and Dr. Fleischmann report receiving grant support from the National Institutes of Health. Dr. Auerbach also reports receiving honorarium as Editor-in-Chief of the Journal of Hospital Medicine. Dr. Corbett reports receiving grant and travel support from Simon Fraser University. The remaining authors have no disclosures to report.
1. The Joint Commission. National Patient Safety Goals. 2016; https://www.jointcommission.org/standards_information/npsgs.aspx. Accessed June 21, 2016.
2. Institute for Healthcare Improvement. Medication Reconciliation to Prevent Adverse Drug Events. 2016; http://www.ihi.org/topics/ADEsMedicationReconciliation/Pages/default.aspx. Accessed June 24, 2016.
3. Bell CM, Brener SS, Gunraj N, et al. Association of ICU or hospital admission with unintentional discontinuation of medications for chronic diseases. JAMA. 2011;306(8):840-847. PubMed
4. Coleman EA, Smith JD, Raha D, Min SJ. Posthospital medication discrepancies: prevalence and contributing factors. Arch Intern Med. 2005;165(16):1842-1847. PubMed
5. Wong JD, Bajcar JM, Wong GG, et al. Medication reconciliation at hospital discharge: evaluating discrepancies. Ann Pharmacother. 2008;42(10):1373-1379. PubMed
6. Forster AJ, Clark HD, Menard A, et al. Adverse events among medical patients after discharge from hospital. CMAJ. 2004;170(3):345-349. PubMed
7. Forster AJ, Murff HJ, Peterson JF, Gandhi TK, Bates DW. The incidence and severity of adverse events affecting patients after discharge from the hospital. Ann Intern Med. 2003;138(3):161-167. PubMed
8. Forster AJ, Murff HJ, Peterson JF, Gandhi TK, Bates DW. Adverse drug events occurring following hospital discharge. JGIM. 2005;20(4):317-323. PubMed
9. Kwan Y, Fernandes OA, Nagge JJ, et al. Pharmacist medication assessments in a surgical preadmission clinic. Arch Intern Med. 2007;167(10):1034-1040. PubMed
10. Unroe KT, Pfeiffenberger T, Riegelhaupt S, Jastrzembski J, Lokhnygina Y, Colon-Emeric C. Inpatient Medication Reconciliation at Admission and Discharge: A Retrospective Cohort Study of Age and Other Risk Factors for Medication Discrepancies. Am J Geriatr Pharmacother. 2010;8(2):115-126. PubMed
11. CDC - National Center for Health Statistics. Fast Stats: Inpatient Surgery. http://www.cdc.gov/nchs/fastats/inpatient-surgery.htm. Accessed on June 24, 2016.
12. Fleisher LA, Fleischmann KE, Auerbach AD, et al. 2014 ACC/AHA guideline on perioperative cardiovascular evaluation and management of patients undergoing noncardiac surgery: a report of the American College of Cardiology/American Heart Association Task Force on Practice Guidelines. Circulation. 2014;130(24):e278-e333. PubMed
13. Budnitz DS, Lovegrove MC, Shehab N, Richards CL. Emergency hospitalizations for adverse drug events in older Americans. N Engl J Med. 2011;365(21):2002-2012. PubMed
14. Budnitz DS, Pollock DA, Weidenbach KN, Mendelsohn AB, Schroeder TJ, Annest JL. National surveillance of emergency department visits for outpatient adverse drug events. JAMA. 2006;296(15):1858-1866. PubMed
15. Bozic KJ, Maselli J, Pekow PS, Lindenauer PK, Vail TP, Auerbach AD. The influence of procedure volumes and standardization of care on quality and efficiency in total joint replacement surgery. J Bone Joint Surg Am. 2010;92(16):2643-2652. PubMed
16. Nolan T, Berwick DM. All-or-none measurement raises the bar on performance. JAMA. 2006;295(10):1168-1170. PubMed
17. Lee TH, Marcantonio ER, Mangione CM, et al. Derivation and prospective validation of a simple index for prediction of cardiac risk of major noncardiac surgery. Circulation. 1999;100(10):1043-1049. PubMed
18. Gonzalez-Garcia L, Salmeron-Garcia A, Garcia-Lirola MA, Moya-Roldan S, Belda-Rustarazo S, Cabeza-Barrera J. Medication reconciliation at admission to surgical departments. J Eval Clin Pract. 2016;22(1):20-25. PubMed
19. Devereaux PJ, Mrkobrada M, Sessler DI, et al. Aspirin in patients undergoing noncardiac surgery. N Engl J Med. 2014;370(16):1494-1503. PubMed
20. Devereaux PJ, Yang H, Yusuf S, et al. Effects of extended-release metoprolol succinate in patients undergoing non-cardiac surgery (POISE trial): a randomised controlled trial. Lancet. 2008;371(9627):1839-1847. PubMed
21. Lindenauer PK, Pekow P, Wang K, Mamidi DK, Gutierrez B, Benjamin EM. Perioperative beta-blocker therapy and mortality after major noncardiac surgery. N Engl J Med. 2005;353(4):349-361. PubMed
22. London MJ, Hur K, Schwartz GG, Henderson WG. Association of perioperative beta-blockade with mortality and cardiovascular morbidity following major noncardiac surgery. JAMA. 2013;309(16):1704-1713. PubMed
23. Rosenman DJ, McDonald FS, Ebbert JO, Erwin PJ, LaBella M, Montori VM. Clinical consequences of withholding versus administering renin-angiotensin-aldosterone system antagonists in the preoperative period. J Hosp Med. 2008;3(4):319-325. PubMed
24. Andersson C, Merie C, Jorgensen M, et al. Association of beta-blocker therapy with risks of adverse cardiovascular events and deaths in patients with ischemic heart disease undergoing noncardiac surgery: a Danish nationwide cohort study. JAMA Intern Med. 2014;174(3):336-344. PubMed
25. Yancy CW, Jessup M, Bozkurt B, et al. 2013 ACCF/AHA Guideline for the Management of Heart Failure A Report of the American College of Cardiology Foundation/American Heart Association Task Force on Practice Guidelines. Circulation. 2013;128(16):E240-E327. PubMed
26. Kwan JL, Lo L, Sampson M, Shojania KG. Medication reconciliation during transitions of care as a patient safety strategy: a systematic review. Ann Intern Med. 2013;158(5 Pt 2):397-403. PubMed
27. Tam VC, Knowles SR, Cornish PL, Fine N, Marchesano R, Etchells EE. Frequency, type and clinical importance of medication history errors at admission to hospital: a systematic review. CMAJ. 2005;173(5):510-515. PubMed
28. Lindenauer PK, Pekow P, Wang K, Gutierrez B, Benjamin EM. Lipid-lowering therapy and in-hospital mortality following major noncardiac surgery. JAMA. 2004;291(17):2092-2099. PubMed
29. Raju MG, Pachika A, Punnam SR, et al. Statin Therapy in the Reduction of Cardiovascular Events in Patients Undergoing Intermediate-Risk Noncardiac, Nonvascular Surgery. Clin Cardiol. 2013;36(8):456-461. PubMed
Medication reconciliation at hospital discharge is a critical component of the posthospital transition of care.1 Effective reconciliation involves a clear process for documenting a current medication list, identifying and resolving discrepancies, and then documenting decisions and instructions around which medications should be continued, modified, or stopped.2 Existing studies3-5 suggest that medication discrepancies are common during hospital discharge transitions of care and lead to preventable adverse drug events, patient disability, and increased healthcare utilization following hospital discharge, including physician office visits, emergency department (ED) visits, and hospitalizations.6-8
While the majority of studies of medication discrepancies have been conducted in general medical patients, few have examined how gaps in discharge medication reconciliation might affect surgical patients.9,10 Two prior studies9,10 suggest that medication discrepancies may occur more frequently for surgical patients, compared with medical patients, particularly discrepancies in reordering home medications postoperatively, raising patient safety concerns for more than 50 million patients hospitalized for surgery each year.11 In particular, little is known about the appropriate discharge reconciliation of chronic cardiovascular medications, such as beta-blockers, renin-angiotensin system inhibitors, and statins in surgical patients, despite perioperative practice guidelines recommending continuation or rapid reinitiation of these medications after noncardiac surgery.12 Problems with chronic cardiovascular medications have been implicated as major contributors to ED visits and hospitalizations for adverse drug events,13,14 further highlighting the importance of safe and appropriate management of these medications.
To better understand the current state and impact of postoperative discharge medication reconciliation of chronic cardiovascular medications in surgical patients, we examined (1) the appropriate discharge reconciliation of 4 cardiovascular medication classes, and (2) the associations between the appropriate discharge reconciliation of these medication classes and postdischarge acute hospital and ambulatory visits in patients hospitalized for elective noncardiac surgery at an academic medical center.
METHODS
Study Design and Patient Selection
We performed a retrospective analysis of data collected as part of a cohort study of hospitalized surgical patients admitted between January 2007 and December 2011. The original study was designed to assess the impact of a social marketing intervention on guideline-appropriate perioperative beta-blocker use in surgical patients. The study was conducted at 1 academic medical center that had 2 campuses with full noncardiac operative facilities and a 600-bed total capacity. Both sites had preoperative clinics, and patients were recruited by review of preoperative clinic records. Institutional review boards responsible for all sites approved the study.
For this analysis, we included adults (age >18 years) undergoing elective noncardiac surgery, who were expected to remain hospitalized for at least 1 day and were taking antiplatelet agents (aspirin, aspirin-dipyridamole, or clopidogrel), beta-blockers, renin-angiotensin system inhibitors (angiotensin-converting-enzyme inhibitors or angiotensin-receptor blockers), or statin lipid-lowering agents.
Data Collection
Data Sources. We collected data from a structured review of medical records as well as from discharge abstract information obtained from administrative data systems. Data regarding patient demographics (age, sex, and race/ethnicity), medical history, preoperative cardiovascular medications, surgical procedure and service, and attending surgeon were obtained from a medical record review of comprehensive preoperative clinic evaluations. Data regarding complications during hospitalization were obtained from medical record review and administrative data (Supplement for International Classification of Diseases, Ninth Revision codes).15 Research assistants abstracting data were trained by using a comprehensive reference manual providing specific criteria for classifying chart abstraction data. Research assistants also were directly observed during initial chart abstractions and underwent random chart validation audits by a senior investigator to ensure consistency. Any discrepancies in coding were resolved by consensus among senior investigators.
Definition of Key Predictor: Appropriate Reconciliation. We abstracted discharge medication lists from the electronic medical record. We defined the appropriate reconciliation of cardiovascular medications at discharge as documentation in discharge instructions, medication reconciliation tools, or discharge summaries that a preadmission cardiovascular medication was being continued at discharge, or, if the medication was not continued, documentation of a new contraindication to the medication or complication precluding its use during hospitalization. Medication continuity was considered appropriate if it was continued at discharge irrespective of changes in dosage. By using this measure for individual medications, we also assessed appropriate reconciliation as an “all-or-none” complete versus incomplete measure (appropriate reconciliation of all preoperative cardiovascular medication classes the patient was taking).16
Definition of Outcomes. Our coprimary outcomes were acute hospital visits (ED visits or hospitalizations) and unplanned ambulatory visits (primary care or surgical) at 30 days after surgery. Postoperative ambulatory visits that were not planned prior to surgery were defined as unplanned. Outcomes were ascertained by patient reports during follow-up telephone questionnaires administered by trained research staff and verified by medical record review.
Definition of Covariates. Using these data, we calculated a Revised Cardiac Risk Index (RCRI) score,17 which estimates the risk of perioperative cardiac complications in patients undergoing surgery. Through chart abstraction data supplemented by diagnosis codes from administrative data, we also constructed variables indicating occurrences of postoperative complications anytime during hospitalization that might pose contraindications to continuation of the 4 cardiovascular medication classes studied. For example, if a chart indicated that the patient had an acute rise in creatinine (elevation of baseline creatinine by 50% or absolute rise of 1 mg/dL in patients with baseline creatinine greater than 3 mg/dL) during hospitalization and a preoperative renin-angiotensin system inhibitor was not prescribed at discharge, we would have considered discontinuation appropriate. Other complications we abstracted were hypotension (systolic blood pressure less than 90 mmHg) for beta-blockers and renin-angiotensin system inhibitors, bradycardia (heart rate less than 50 bpm) for beta-blockers, acute kidney injury (defined above) and hyperkalemia for renin-angiotensin system inhibitors, and bleeding (any site) for antiplatelet agents.
Statistical Analysis
We used χ2 and Kruskal-Wallis tests to compare baseline patient characteristics. To assess associations between appropriate medication reconciliation and patient outcomes, we used multilevel mixed-effects logistic regression to account for the clustering of patients by the attending surgeon. We adjusted for baseline patient demographics, surgical service, the number of baseline cardiovascular medications, and individual RCRI criteria. We constructed separate models for all-or-none appropriate reconciliation and for each individual medication class.
As a sensitivity analysis, we constructed similar models by using a simplified definition of appropriate reconciliation based entirely on medication continuity (continued or not continued at discharge) without taking potential contraindications during hospitalization into account. For complete versus incomplete reconciliation, we also constructed models with an interaction term between the number of baseline cardiovascular medications and appropriate medication reconciliation to test the hypothesis that inappropriate reconciliation would be more likely with an increasing number of preoperative cardiovascular medications. Because this interaction term was not statistically significant, we did not include it in the final models for ease of reporting and interpretability. We performed all statistical analyses using Stata 14 (StataCorp, LLC, College Station, Texas), and used 2-sided statistical tests and a P value of less than .05 to define statistical significance.
RESULTS
Patient Characteristics
A total of 849 patients were enrolled, of which 752 (88.6%) were taking at least 1 of the specified cardiovascular medications in the preoperative period. Their mean age was 61.5; 50.9% were male, 72.6% were non-Hispanic white, and 89.4% had RCRI scores of 0 or 1 (Table 1). The majority (63.8%) were undergoing general surgery, orthopedic surgery, or neurosurgery procedures. In the preoperative period, 327 (43.5%) patients were taking antiplatelet agents, 624 (83.0%) were taking beta-blockers, 361 (48.0%) were taking renin-angiotensin system inhibitors, and 406 (54.0%) were taking statins (Table 2). Among patients taking antiplatelet agents, 271 (82.9%) were taking aspirin alone, 21 (6.4%) were taking clopidogrel alone, and 35 (10.7%) were taking dual antiplatelet therapy with aspirin and clopidogrel. Nearly three-quarters of the patients (551, 73.3%) were taking medications from 2 or more classes, and the proportion of patients with inappropriate reconciliation increased with the number of preoperative cardiovascular medications.
Patients with and without appropriate reconciliation of all preoperative cardiovascular medications were similar in age, sex, and race/ethnicity (Table 1). Patients with inappropriate reconciliation of at least 1 medication were more likely to be on the urology and renal/liver transplant surgical services, have higher RCRI scores, and be taking antiplatelet agents, statins, renin-angiotensin system inhibitors, and 3 or more cardiovascular medications in the preoperative period.
Appropriate Medication Reconciliation
Four hundred thirty-six patients (58.0%) had their baseline cardiovascular medications appropriately reconciled. Among all patients with appropriately reconciled medications, 1 (0.2%) had beta-blockers discontinued due to a documented episode of hypotension; 17 (3.9%) had renin-angiotensin system inhibitors discontinued due to episodes of acute kidney injury, hypotension, or hyperkalemia; and 1 (0.2%) had antiplatelet agents discontinued due to bleeding. For individual medications, appropriate reconciliation between the preoperative and discharge periods occurred for 156 of the 327 patients on antiplatelet agents (47.7%), 507 of the 624 patients on beta-blockers (81.3%), 259 of the 361 patients on renin-angiotensin system inhibitors (71.8%), and 302 of the 406 patients on statins (74.4%; Table 2).
Associations Between Medication Reconciliation and Outcomes
Thirty-day outcome data on acute hospital visits were available for 679 (90.3%) patients. Of these, 146 (21.5%) were seen in the ED or were hospitalized, and 111 (16.3%) were seen for an unplanned primary care or surgical outpatient visit at 30 days after surgery. Patients with incomplete outcome data were more likely to have complete medication reconciliation compared with those with complete outcome data (71.2% vs 56.6%, P = 0.02). As shown in Table 3, the proportion of patients with 30-day acute hospital visits was nonstatistically significantly lower in patients with complete medication reconciliation (20.8% vs 22.4%, P = 0.63) and the appropriate reconciliation of beta-blockers (21.9% vs 23.6%, P = 0.71) and renin-angiotensin system inhibitors (19.6% vs 20.0%, P = 0.93), and nonsignificantly higher with the appropriate reconciliation of antiplatelet agents (23.9% vs 19.9%, P = 0.40). Acute hospital visits were statistically significantly lower with the appropriate reconciliation of statins (17.9% vs 31.9%, P = 0.004).
Sensitivity Analysis
Overall, 430 (57.2%) patients had complete cardiovascular medication continuity without considering potential contraindications during hospitalization. Associations between medication continuity and acute hospital and ambulatory visits were similar to the primary analyses.
DISCUSSION
In this study of 752 patients hospitalized for elective noncardiac surgery, we found significant gaps in the appropriate reconciliation of commonly prescribed cardiovascular medications, with inappropriate discontinuation ranging from 18.8% to 52.3% for individual medications. Unplanned postdischarge healthcare utilization was high, with acute hospital visits documented in 21.5% of patients and unplanned ambulatory visits in 16.3% at 30 days after surgery. However, medication reconciliation gaps were not consistently associated with ED visits, hospitalizations, or unplanned ambulatory visits.
Our finding of large gaps in postoperative medication reconciliation is consistent with existing studies of medication reconciliation in surgical patients.9,10,18 One study found medication discrepancies in 40.2% of postoperative patients receiving usual care and discrepancies judged to have the potential to cause harm (such as the omission of beta-blockers) in 29.9%.9 Consistent with our findings, this study also found that most postoperative medication discrepancies were omissions in reordering home medications, though at a rate somewhat higher than those seen in medical patients at discharge.5 While hospitalization by itself increases the risk of unintentional discontinuation of chronic medications,3 our results, along with existing literature, suggest that the risk for omission of chronic medications is unacceptably high.
We also found significant variation in reconciliation among cardiovascular medications, with appropriate reconciliation occurring least frequently for antiplatelet agents and most frequently for beta-blockers. The low rates of appropriate reconciliation for antiplatelet agents may be attributable to deliberate withholding of antiplatelet therapy in the postoperative period based on clinical assessments of surgical bleeding risk in the absence of active bleeding. Perioperative management of antiplatelet agents for noncardiac surgery remains an unclear and controversial topic, which may also contribute to the variation noted.19 Conversely, beta-blockers demonstrated high rates of preoperative use (over 80% of patients) and appropriate reconciliation. Both findings are likely attributable in part to the timing of the study, which began prior to the publication of the Perioperative Ischemic Evaluation trial, which more definitively demonstrated the potential harms of perioperative beta-blocker therapy.20
Despite a high proportion of patients with discontinuous medications at discharge, we found no associations between the appropriate reconciliation of beta-blockers, renin-angiotensin system inhibitors, and antiplatelet agents and acute hospital or ambulatory visits in the first 30 days after discharge. One explanation for this discrepancy is that, although we focused on cardiovascular medications commonly implicated in acute hospital visits, the vast majority of patients in our study had low perioperative cardiovascular risk as assessed by the RCRI. Previous studies have demonstrated that the benefit of perioperative beta-blocker therapy is predominantly in patients with moderate to high perioperative cardiovascular risk.21,22 It is possible that the detrimental effects of the discontinuation of chronic cardiovascular medications are more prominent in populations at a higher risk of perioperative cardiovascular complications or that complications will occur later than 30 days after discharge. Similarly, while the benefits of continuation of renin-angiotensin system inhibitors are less clear,23 few patients in our cohort had a history of congestive heart failure (6.3%) or coronary artery disease (13.0%), 2 conditions in which the impact of perioperative discontinuation of renin-angiotensin inhibitor or beta-blocker therapy would likely be more pronounced.24,25 An additional explanation for the lack of associations is that, while multiple studies have demonstrated that medication errors are common, the proportion of errors with the potential for harm is much lower, and the proportion that causes actual harm is lower still.5,26,27 Thus, while we likely captured high-severity medication errors leading to acute hospital or unplanned ambulatory visits, we would not have captured medication errors with lower severity clinical consequences that did not result in medical encounters.
We did find an association between the continuation of statin therapy and reduced ED visits and hospitalizations. This finding is supported by previous studies of patients undergoing noncardiac surgery, including 1 demonstrating an association between immediate postoperative statin therapy and reduced in-hospital mortality28 and another study demonstrating an association between postoperative statin therapy and reductions in a composite endpoint of 30-day mortality, atrial fibrillation, and nonfatal myocardial infarction.29 Alternatively, this finding could reflect the effects of unaddressed confounding by factors contributing to statin discontinuation and poor health outcomes leading to acute hospital visits, such as acute elevations in liver enzymes.
Our study has important implications for patients undergoing elective noncardiac surgery and the healthcare providers caring for them. First, inappropriate omissions of chronic cardiovascular medications at discharge are common; clinicians should increase their general awareness and focus on appropriately reconciling these medications, for even if our results do not connect medication discontinuity to readmissions or unexpected clinical encounters, their impact on patients’ understanding of their medications remains a potential concern. Second, the overall high rates of unplanned postdischarge healthcare utilization in this study highlight the need for close postdischarge monitoring of patients undergoing elective surgical procedures and for further research to identify preventable etiologies of postdischarge healthcare utilization in this population. Third, further study is needed to identify specific patient populations and medication classes, in which appropriate reconciliation is associated with patient outcomes that may benefit from more intensive discharge medication reconciliation interventions.
Our study has limitations. First, the majority of patients in this single-center study were at low risk of perioperative cardiovascular events, and our results may not be generalizable to higher-risk patients undergoing elective surgery. Second, discharge reconciliation was based on documentation of medication reconciliation and not on patient-reported medication adherence. In addition, the ability to judge the accuracy of discharge medication reconciliation is in part dependent on the accuracy of the admission medication reconciliation. Thus, although we used preoperative medication regimens documented during preadmission visits to comprehensive preoperative clinics for comparison, discrepancies in these preoperative regimens could have affected our analysis of appropriate discharge reconciliation. Third, inadequate documentation of clinical reasons for discontinuing medications may have led to residual confounding by indication in our observational study. Finally, the outcomes available to us may have been relatively insensitive to other adverse effects of medication discontinuity, such as patient symptoms (eg, angina severity), patient awareness of medications, or work placed on primary care physicians needing to “clean up” erroneous medication lists.
In conclusion, gaps in appropriate discharge reconciliation of chronic cardiovascular medications were common but not consistently associated with postdischarge acute hospital or unplanned ambulatory visits in a relatively low-risk cohort of patients undergoing elective surgery. While appropriate medication reconciliation should always be a priority, further study is needed to identify medication reconciliation approaches associated with postdischarge healthcare utilization and other patient outcomes.
Disclosure
Dr. Lee reports receiving grant support from the Health Resources and Services Administration (T32HP19025). Dr. Vittinghoff reports receiving grant support from the Agency for Healthcare Research and Quality. Dr. Auerbach and Dr. Fleischmann report receiving grant support from the National Institutes of Health. Dr. Auerbach also reports receiving honorarium as Editor-in-Chief of the Journal of Hospital Medicine. Dr. Corbett reports receiving grant and travel support from Simon Fraser University. The remaining authors have no disclosures to report.
Medication reconciliation at hospital discharge is a critical component of the posthospital transition of care.1 Effective reconciliation involves a clear process for documenting a current medication list, identifying and resolving discrepancies, and then documenting decisions and instructions around which medications should be continued, modified, or stopped.2 Existing studies3-5 suggest that medication discrepancies are common during hospital discharge transitions of care and lead to preventable adverse drug events, patient disability, and increased healthcare utilization following hospital discharge, including physician office visits, emergency department (ED) visits, and hospitalizations.6-8
While the majority of studies of medication discrepancies have been conducted in general medical patients, few have examined how gaps in discharge medication reconciliation might affect surgical patients.9,10 Two prior studies9,10 suggest that medication discrepancies may occur more frequently for surgical patients, compared with medical patients, particularly discrepancies in reordering home medications postoperatively, raising patient safety concerns for more than 50 million patients hospitalized for surgery each year.11 In particular, little is known about the appropriate discharge reconciliation of chronic cardiovascular medications, such as beta-blockers, renin-angiotensin system inhibitors, and statins in surgical patients, despite perioperative practice guidelines recommending continuation or rapid reinitiation of these medications after noncardiac surgery.12 Problems with chronic cardiovascular medications have been implicated as major contributors to ED visits and hospitalizations for adverse drug events,13,14 further highlighting the importance of safe and appropriate management of these medications.
To better understand the current state and impact of postoperative discharge medication reconciliation of chronic cardiovascular medications in surgical patients, we examined (1) the appropriate discharge reconciliation of 4 cardiovascular medication classes, and (2) the associations between the appropriate discharge reconciliation of these medication classes and postdischarge acute hospital and ambulatory visits in patients hospitalized for elective noncardiac surgery at an academic medical center.
METHODS
Study Design and Patient Selection
We performed a retrospective analysis of data collected as part of a cohort study of hospitalized surgical patients admitted between January 2007 and December 2011. The original study was designed to assess the impact of a social marketing intervention on guideline-appropriate perioperative beta-blocker use in surgical patients. The study was conducted at 1 academic medical center that had 2 campuses with full noncardiac operative facilities and a 600-bed total capacity. Both sites had preoperative clinics, and patients were recruited by review of preoperative clinic records. Institutional review boards responsible for all sites approved the study.
For this analysis, we included adults (age >18 years) undergoing elective noncardiac surgery, who were expected to remain hospitalized for at least 1 day and were taking antiplatelet agents (aspirin, aspirin-dipyridamole, or clopidogrel), beta-blockers, renin-angiotensin system inhibitors (angiotensin-converting-enzyme inhibitors or angiotensin-receptor blockers), or statin lipid-lowering agents.
Data Collection
Data Sources. We collected data from a structured review of medical records as well as from discharge abstract information obtained from administrative data systems. Data regarding patient demographics (age, sex, and race/ethnicity), medical history, preoperative cardiovascular medications, surgical procedure and service, and attending surgeon were obtained from a medical record review of comprehensive preoperative clinic evaluations. Data regarding complications during hospitalization were obtained from medical record review and administrative data (Supplement for International Classification of Diseases, Ninth Revision codes).15 Research assistants abstracting data were trained by using a comprehensive reference manual providing specific criteria for classifying chart abstraction data. Research assistants also were directly observed during initial chart abstractions and underwent random chart validation audits by a senior investigator to ensure consistency. Any discrepancies in coding were resolved by consensus among senior investigators.
Definition of Key Predictor: Appropriate Reconciliation. We abstracted discharge medication lists from the electronic medical record. We defined the appropriate reconciliation of cardiovascular medications at discharge as documentation in discharge instructions, medication reconciliation tools, or discharge summaries that a preadmission cardiovascular medication was being continued at discharge, or, if the medication was not continued, documentation of a new contraindication to the medication or complication precluding its use during hospitalization. Medication continuity was considered appropriate if it was continued at discharge irrespective of changes in dosage. By using this measure for individual medications, we also assessed appropriate reconciliation as an “all-or-none” complete versus incomplete measure (appropriate reconciliation of all preoperative cardiovascular medication classes the patient was taking).16
Definition of Outcomes. Our coprimary outcomes were acute hospital visits (ED visits or hospitalizations) and unplanned ambulatory visits (primary care or surgical) at 30 days after surgery. Postoperative ambulatory visits that were not planned prior to surgery were defined as unplanned. Outcomes were ascertained by patient reports during follow-up telephone questionnaires administered by trained research staff and verified by medical record review.
Definition of Covariates. Using these data, we calculated a Revised Cardiac Risk Index (RCRI) score,17 which estimates the risk of perioperative cardiac complications in patients undergoing surgery. Through chart abstraction data supplemented by diagnosis codes from administrative data, we also constructed variables indicating occurrences of postoperative complications anytime during hospitalization that might pose contraindications to continuation of the 4 cardiovascular medication classes studied. For example, if a chart indicated that the patient had an acute rise in creatinine (elevation of baseline creatinine by 50% or absolute rise of 1 mg/dL in patients with baseline creatinine greater than 3 mg/dL) during hospitalization and a preoperative renin-angiotensin system inhibitor was not prescribed at discharge, we would have considered discontinuation appropriate. Other complications we abstracted were hypotension (systolic blood pressure less than 90 mmHg) for beta-blockers and renin-angiotensin system inhibitors, bradycardia (heart rate less than 50 bpm) for beta-blockers, acute kidney injury (defined above) and hyperkalemia for renin-angiotensin system inhibitors, and bleeding (any site) for antiplatelet agents.
Statistical Analysis
We used χ2 and Kruskal-Wallis tests to compare baseline patient characteristics. To assess associations between appropriate medication reconciliation and patient outcomes, we used multilevel mixed-effects logistic regression to account for the clustering of patients by the attending surgeon. We adjusted for baseline patient demographics, surgical service, the number of baseline cardiovascular medications, and individual RCRI criteria. We constructed separate models for all-or-none appropriate reconciliation and for each individual medication class.
As a sensitivity analysis, we constructed similar models by using a simplified definition of appropriate reconciliation based entirely on medication continuity (continued or not continued at discharge) without taking potential contraindications during hospitalization into account. For complete versus incomplete reconciliation, we also constructed models with an interaction term between the number of baseline cardiovascular medications and appropriate medication reconciliation to test the hypothesis that inappropriate reconciliation would be more likely with an increasing number of preoperative cardiovascular medications. Because this interaction term was not statistically significant, we did not include it in the final models for ease of reporting and interpretability. We performed all statistical analyses using Stata 14 (StataCorp, LLC, College Station, Texas), and used 2-sided statistical tests and a P value of less than .05 to define statistical significance.
RESULTS
Patient Characteristics
A total of 849 patients were enrolled, of which 752 (88.6%) were taking at least 1 of the specified cardiovascular medications in the preoperative period. Their mean age was 61.5; 50.9% were male, 72.6% were non-Hispanic white, and 89.4% had RCRI scores of 0 or 1 (Table 1). The majority (63.8%) were undergoing general surgery, orthopedic surgery, or neurosurgery procedures. In the preoperative period, 327 (43.5%) patients were taking antiplatelet agents, 624 (83.0%) were taking beta-blockers, 361 (48.0%) were taking renin-angiotensin system inhibitors, and 406 (54.0%) were taking statins (Table 2). Among patients taking antiplatelet agents, 271 (82.9%) were taking aspirin alone, 21 (6.4%) were taking clopidogrel alone, and 35 (10.7%) were taking dual antiplatelet therapy with aspirin and clopidogrel. Nearly three-quarters of the patients (551, 73.3%) were taking medications from 2 or more classes, and the proportion of patients with inappropriate reconciliation increased with the number of preoperative cardiovascular medications.
Patients with and without appropriate reconciliation of all preoperative cardiovascular medications were similar in age, sex, and race/ethnicity (Table 1). Patients with inappropriate reconciliation of at least 1 medication were more likely to be on the urology and renal/liver transplant surgical services, have higher RCRI scores, and be taking antiplatelet agents, statins, renin-angiotensin system inhibitors, and 3 or more cardiovascular medications in the preoperative period.
Appropriate Medication Reconciliation
Four hundred thirty-six patients (58.0%) had their baseline cardiovascular medications appropriately reconciled. Among all patients with appropriately reconciled medications, 1 (0.2%) had beta-blockers discontinued due to a documented episode of hypotension; 17 (3.9%) had renin-angiotensin system inhibitors discontinued due to episodes of acute kidney injury, hypotension, or hyperkalemia; and 1 (0.2%) had antiplatelet agents discontinued due to bleeding. For individual medications, appropriate reconciliation between the preoperative and discharge periods occurred for 156 of the 327 patients on antiplatelet agents (47.7%), 507 of the 624 patients on beta-blockers (81.3%), 259 of the 361 patients on renin-angiotensin system inhibitors (71.8%), and 302 of the 406 patients on statins (74.4%; Table 2).
Associations Between Medication Reconciliation and Outcomes
Thirty-day outcome data on acute hospital visits were available for 679 (90.3%) patients. Of these, 146 (21.5%) were seen in the ED or were hospitalized, and 111 (16.3%) were seen for an unplanned primary care or surgical outpatient visit at 30 days after surgery. Patients with incomplete outcome data were more likely to have complete medication reconciliation compared with those with complete outcome data (71.2% vs 56.6%, P = 0.02). As shown in Table 3, the proportion of patients with 30-day acute hospital visits was nonstatistically significantly lower in patients with complete medication reconciliation (20.8% vs 22.4%, P = 0.63) and the appropriate reconciliation of beta-blockers (21.9% vs 23.6%, P = 0.71) and renin-angiotensin system inhibitors (19.6% vs 20.0%, P = 0.93), and nonsignificantly higher with the appropriate reconciliation of antiplatelet agents (23.9% vs 19.9%, P = 0.40). Acute hospital visits were statistically significantly lower with the appropriate reconciliation of statins (17.9% vs 31.9%, P = 0.004).
Sensitivity Analysis
Overall, 430 (57.2%) patients had complete cardiovascular medication continuity without considering potential contraindications during hospitalization. Associations between medication continuity and acute hospital and ambulatory visits were similar to the primary analyses.
DISCUSSION
In this study of 752 patients hospitalized for elective noncardiac surgery, we found significant gaps in the appropriate reconciliation of commonly prescribed cardiovascular medications, with inappropriate discontinuation ranging from 18.8% to 52.3% for individual medications. Unplanned postdischarge healthcare utilization was high, with acute hospital visits documented in 21.5% of patients and unplanned ambulatory visits in 16.3% at 30 days after surgery. However, medication reconciliation gaps were not consistently associated with ED visits, hospitalizations, or unplanned ambulatory visits.
Our finding of large gaps in postoperative medication reconciliation is consistent with existing studies of medication reconciliation in surgical patients.9,10,18 One study found medication discrepancies in 40.2% of postoperative patients receiving usual care and discrepancies judged to have the potential to cause harm (such as the omission of beta-blockers) in 29.9%.9 Consistent with our findings, this study also found that most postoperative medication discrepancies were omissions in reordering home medications, though at a rate somewhat higher than those seen in medical patients at discharge.5 While hospitalization by itself increases the risk of unintentional discontinuation of chronic medications,3 our results, along with existing literature, suggest that the risk for omission of chronic medications is unacceptably high.
We also found significant variation in reconciliation among cardiovascular medications, with appropriate reconciliation occurring least frequently for antiplatelet agents and most frequently for beta-blockers. The low rates of appropriate reconciliation for antiplatelet agents may be attributable to deliberate withholding of antiplatelet therapy in the postoperative period based on clinical assessments of surgical bleeding risk in the absence of active bleeding. Perioperative management of antiplatelet agents for noncardiac surgery remains an unclear and controversial topic, which may also contribute to the variation noted.19 Conversely, beta-blockers demonstrated high rates of preoperative use (over 80% of patients) and appropriate reconciliation. Both findings are likely attributable in part to the timing of the study, which began prior to the publication of the Perioperative Ischemic Evaluation trial, which more definitively demonstrated the potential harms of perioperative beta-blocker therapy.20
Despite a high proportion of patients with discontinuous medications at discharge, we found no associations between the appropriate reconciliation of beta-blockers, renin-angiotensin system inhibitors, and antiplatelet agents and acute hospital or ambulatory visits in the first 30 days after discharge. One explanation for this discrepancy is that, although we focused on cardiovascular medications commonly implicated in acute hospital visits, the vast majority of patients in our study had low perioperative cardiovascular risk as assessed by the RCRI. Previous studies have demonstrated that the benefit of perioperative beta-blocker therapy is predominantly in patients with moderate to high perioperative cardiovascular risk.21,22 It is possible that the detrimental effects of the discontinuation of chronic cardiovascular medications are more prominent in populations at a higher risk of perioperative cardiovascular complications or that complications will occur later than 30 days after discharge. Similarly, while the benefits of continuation of renin-angiotensin system inhibitors are less clear,23 few patients in our cohort had a history of congestive heart failure (6.3%) or coronary artery disease (13.0%), 2 conditions in which the impact of perioperative discontinuation of renin-angiotensin inhibitor or beta-blocker therapy would likely be more pronounced.24,25 An additional explanation for the lack of associations is that, while multiple studies have demonstrated that medication errors are common, the proportion of errors with the potential for harm is much lower, and the proportion that causes actual harm is lower still.5,26,27 Thus, while we likely captured high-severity medication errors leading to acute hospital or unplanned ambulatory visits, we would not have captured medication errors with lower severity clinical consequences that did not result in medical encounters.
We did find an association between the continuation of statin therapy and reduced ED visits and hospitalizations. This finding is supported by previous studies of patients undergoing noncardiac surgery, including 1 demonstrating an association between immediate postoperative statin therapy and reduced in-hospital mortality28 and another study demonstrating an association between postoperative statin therapy and reductions in a composite endpoint of 30-day mortality, atrial fibrillation, and nonfatal myocardial infarction.29 Alternatively, this finding could reflect the effects of unaddressed confounding by factors contributing to statin discontinuation and poor health outcomes leading to acute hospital visits, such as acute elevations in liver enzymes.
Our study has important implications for patients undergoing elective noncardiac surgery and the healthcare providers caring for them. First, inappropriate omissions of chronic cardiovascular medications at discharge are common; clinicians should increase their general awareness and focus on appropriately reconciling these medications, for even if our results do not connect medication discontinuity to readmissions or unexpected clinical encounters, their impact on patients’ understanding of their medications remains a potential concern. Second, the overall high rates of unplanned postdischarge healthcare utilization in this study highlight the need for close postdischarge monitoring of patients undergoing elective surgical procedures and for further research to identify preventable etiologies of postdischarge healthcare utilization in this population. Third, further study is needed to identify specific patient populations and medication classes, in which appropriate reconciliation is associated with patient outcomes that may benefit from more intensive discharge medication reconciliation interventions.
Our study has limitations. First, the majority of patients in this single-center study were at low risk of perioperative cardiovascular events, and our results may not be generalizable to higher-risk patients undergoing elective surgery. Second, discharge reconciliation was based on documentation of medication reconciliation and not on patient-reported medication adherence. In addition, the ability to judge the accuracy of discharge medication reconciliation is in part dependent on the accuracy of the admission medication reconciliation. Thus, although we used preoperative medication regimens documented during preadmission visits to comprehensive preoperative clinics for comparison, discrepancies in these preoperative regimens could have affected our analysis of appropriate discharge reconciliation. Third, inadequate documentation of clinical reasons for discontinuing medications may have led to residual confounding by indication in our observational study. Finally, the outcomes available to us may have been relatively insensitive to other adverse effects of medication discontinuity, such as patient symptoms (eg, angina severity), patient awareness of medications, or work placed on primary care physicians needing to “clean up” erroneous medication lists.
In conclusion, gaps in appropriate discharge reconciliation of chronic cardiovascular medications were common but not consistently associated with postdischarge acute hospital or unplanned ambulatory visits in a relatively low-risk cohort of patients undergoing elective surgery. While appropriate medication reconciliation should always be a priority, further study is needed to identify medication reconciliation approaches associated with postdischarge healthcare utilization and other patient outcomes.
Disclosure
Dr. Lee reports receiving grant support from the Health Resources and Services Administration (T32HP19025). Dr. Vittinghoff reports receiving grant support from the Agency for Healthcare Research and Quality. Dr. Auerbach and Dr. Fleischmann report receiving grant support from the National Institutes of Health. Dr. Auerbach also reports receiving honorarium as Editor-in-Chief of the Journal of Hospital Medicine. Dr. Corbett reports receiving grant and travel support from Simon Fraser University. The remaining authors have no disclosures to report.
1. The Joint Commission. National Patient Safety Goals. 2016; https://www.jointcommission.org/standards_information/npsgs.aspx. Accessed June 21, 2016.
2. Institute for Healthcare Improvement. Medication Reconciliation to Prevent Adverse Drug Events. 2016; http://www.ihi.org/topics/ADEsMedicationReconciliation/Pages/default.aspx. Accessed June 24, 2016.
3. Bell CM, Brener SS, Gunraj N, et al. Association of ICU or hospital admission with unintentional discontinuation of medications for chronic diseases. JAMA. 2011;306(8):840-847. PubMed
4. Coleman EA, Smith JD, Raha D, Min SJ. Posthospital medication discrepancies: prevalence and contributing factors. Arch Intern Med. 2005;165(16):1842-1847. PubMed
5. Wong JD, Bajcar JM, Wong GG, et al. Medication reconciliation at hospital discharge: evaluating discrepancies. Ann Pharmacother. 2008;42(10):1373-1379. PubMed
6. Forster AJ, Clark HD, Menard A, et al. Adverse events among medical patients after discharge from hospital. CMAJ. 2004;170(3):345-349. PubMed
7. Forster AJ, Murff HJ, Peterson JF, Gandhi TK, Bates DW. The incidence and severity of adverse events affecting patients after discharge from the hospital. Ann Intern Med. 2003;138(3):161-167. PubMed
8. Forster AJ, Murff HJ, Peterson JF, Gandhi TK, Bates DW. Adverse drug events occurring following hospital discharge. JGIM. 2005;20(4):317-323. PubMed
9. Kwan Y, Fernandes OA, Nagge JJ, et al. Pharmacist medication assessments in a surgical preadmission clinic. Arch Intern Med. 2007;167(10):1034-1040. PubMed
10. Unroe KT, Pfeiffenberger T, Riegelhaupt S, Jastrzembski J, Lokhnygina Y, Colon-Emeric C. Inpatient Medication Reconciliation at Admission and Discharge: A Retrospective Cohort Study of Age and Other Risk Factors for Medication Discrepancies. Am J Geriatr Pharmacother. 2010;8(2):115-126. PubMed
11. CDC - National Center for Health Statistics. Fast Stats: Inpatient Surgery. http://www.cdc.gov/nchs/fastats/inpatient-surgery.htm. Accessed on June 24, 2016.
12. Fleisher LA, Fleischmann KE, Auerbach AD, et al. 2014 ACC/AHA guideline on perioperative cardiovascular evaluation and management of patients undergoing noncardiac surgery: a report of the American College of Cardiology/American Heart Association Task Force on Practice Guidelines. Circulation. 2014;130(24):e278-e333. PubMed
13. Budnitz DS, Lovegrove MC, Shehab N, Richards CL. Emergency hospitalizations for adverse drug events in older Americans. N Engl J Med. 2011;365(21):2002-2012. PubMed
14. Budnitz DS, Pollock DA, Weidenbach KN, Mendelsohn AB, Schroeder TJ, Annest JL. National surveillance of emergency department visits for outpatient adverse drug events. JAMA. 2006;296(15):1858-1866. PubMed
15. Bozic KJ, Maselli J, Pekow PS, Lindenauer PK, Vail TP, Auerbach AD. The influence of procedure volumes and standardization of care on quality and efficiency in total joint replacement surgery. J Bone Joint Surg Am. 2010;92(16):2643-2652. PubMed
16. Nolan T, Berwick DM. All-or-none measurement raises the bar on performance. JAMA. 2006;295(10):1168-1170. PubMed
17. Lee TH, Marcantonio ER, Mangione CM, et al. Derivation and prospective validation of a simple index for prediction of cardiac risk of major noncardiac surgery. Circulation. 1999;100(10):1043-1049. PubMed
18. Gonzalez-Garcia L, Salmeron-Garcia A, Garcia-Lirola MA, Moya-Roldan S, Belda-Rustarazo S, Cabeza-Barrera J. Medication reconciliation at admission to surgical departments. J Eval Clin Pract. 2016;22(1):20-25. PubMed
19. Devereaux PJ, Mrkobrada M, Sessler DI, et al. Aspirin in patients undergoing noncardiac surgery. N Engl J Med. 2014;370(16):1494-1503. PubMed
20. Devereaux PJ, Yang H, Yusuf S, et al. Effects of extended-release metoprolol succinate in patients undergoing non-cardiac surgery (POISE trial): a randomised controlled trial. Lancet. 2008;371(9627):1839-1847. PubMed
21. Lindenauer PK, Pekow P, Wang K, Mamidi DK, Gutierrez B, Benjamin EM. Perioperative beta-blocker therapy and mortality after major noncardiac surgery. N Engl J Med. 2005;353(4):349-361. PubMed
22. London MJ, Hur K, Schwartz GG, Henderson WG. Association of perioperative beta-blockade with mortality and cardiovascular morbidity following major noncardiac surgery. JAMA. 2013;309(16):1704-1713. PubMed
23. Rosenman DJ, McDonald FS, Ebbert JO, Erwin PJ, LaBella M, Montori VM. Clinical consequences of withholding versus administering renin-angiotensin-aldosterone system antagonists in the preoperative period. J Hosp Med. 2008;3(4):319-325. PubMed
24. Andersson C, Merie C, Jorgensen M, et al. Association of beta-blocker therapy with risks of adverse cardiovascular events and deaths in patients with ischemic heart disease undergoing noncardiac surgery: a Danish nationwide cohort study. JAMA Intern Med. 2014;174(3):336-344. PubMed
25. Yancy CW, Jessup M, Bozkurt B, et al. 2013 ACCF/AHA Guideline for the Management of Heart Failure A Report of the American College of Cardiology Foundation/American Heart Association Task Force on Practice Guidelines. Circulation. 2013;128(16):E240-E327. PubMed
26. Kwan JL, Lo L, Sampson M, Shojania KG. Medication reconciliation during transitions of care as a patient safety strategy: a systematic review. Ann Intern Med. 2013;158(5 Pt 2):397-403. PubMed
27. Tam VC, Knowles SR, Cornish PL, Fine N, Marchesano R, Etchells EE. Frequency, type and clinical importance of medication history errors at admission to hospital: a systematic review. CMAJ. 2005;173(5):510-515. PubMed
28. Lindenauer PK, Pekow P, Wang K, Gutierrez B, Benjamin EM. Lipid-lowering therapy and in-hospital mortality following major noncardiac surgery. JAMA. 2004;291(17):2092-2099. PubMed
29. Raju MG, Pachika A, Punnam SR, et al. Statin Therapy in the Reduction of Cardiovascular Events in Patients Undergoing Intermediate-Risk Noncardiac, Nonvascular Surgery. Clin Cardiol. 2013;36(8):456-461. PubMed
1. The Joint Commission. National Patient Safety Goals. 2016; https://www.jointcommission.org/standards_information/npsgs.aspx. Accessed June 21, 2016.
2. Institute for Healthcare Improvement. Medication Reconciliation to Prevent Adverse Drug Events. 2016; http://www.ihi.org/topics/ADEsMedicationReconciliation/Pages/default.aspx. Accessed June 24, 2016.
3. Bell CM, Brener SS, Gunraj N, et al. Association of ICU or hospital admission with unintentional discontinuation of medications for chronic diseases. JAMA. 2011;306(8):840-847. PubMed
4. Coleman EA, Smith JD, Raha D, Min SJ. Posthospital medication discrepancies: prevalence and contributing factors. Arch Intern Med. 2005;165(16):1842-1847. PubMed
5. Wong JD, Bajcar JM, Wong GG, et al. Medication reconciliation at hospital discharge: evaluating discrepancies. Ann Pharmacother. 2008;42(10):1373-1379. PubMed
6. Forster AJ, Clark HD, Menard A, et al. Adverse events among medical patients after discharge from hospital. CMAJ. 2004;170(3):345-349. PubMed
7. Forster AJ, Murff HJ, Peterson JF, Gandhi TK, Bates DW. The incidence and severity of adverse events affecting patients after discharge from the hospital. Ann Intern Med. 2003;138(3):161-167. PubMed
8. Forster AJ, Murff HJ, Peterson JF, Gandhi TK, Bates DW. Adverse drug events occurring following hospital discharge. JGIM. 2005;20(4):317-323. PubMed
9. Kwan Y, Fernandes OA, Nagge JJ, et al. Pharmacist medication assessments in a surgical preadmission clinic. Arch Intern Med. 2007;167(10):1034-1040. PubMed
10. Unroe KT, Pfeiffenberger T, Riegelhaupt S, Jastrzembski J, Lokhnygina Y, Colon-Emeric C. Inpatient Medication Reconciliation at Admission and Discharge: A Retrospective Cohort Study of Age and Other Risk Factors for Medication Discrepancies. Am J Geriatr Pharmacother. 2010;8(2):115-126. PubMed
11. CDC - National Center for Health Statistics. Fast Stats: Inpatient Surgery. http://www.cdc.gov/nchs/fastats/inpatient-surgery.htm. Accessed on June 24, 2016.
12. Fleisher LA, Fleischmann KE, Auerbach AD, et al. 2014 ACC/AHA guideline on perioperative cardiovascular evaluation and management of patients undergoing noncardiac surgery: a report of the American College of Cardiology/American Heart Association Task Force on Practice Guidelines. Circulation. 2014;130(24):e278-e333. PubMed
13. Budnitz DS, Lovegrove MC, Shehab N, Richards CL. Emergency hospitalizations for adverse drug events in older Americans. N Engl J Med. 2011;365(21):2002-2012. PubMed
14. Budnitz DS, Pollock DA, Weidenbach KN, Mendelsohn AB, Schroeder TJ, Annest JL. National surveillance of emergency department visits for outpatient adverse drug events. JAMA. 2006;296(15):1858-1866. PubMed
15. Bozic KJ, Maselli J, Pekow PS, Lindenauer PK, Vail TP, Auerbach AD. The influence of procedure volumes and standardization of care on quality and efficiency in total joint replacement surgery. J Bone Joint Surg Am. 2010;92(16):2643-2652. PubMed
16. Nolan T, Berwick DM. All-or-none measurement raises the bar on performance. JAMA. 2006;295(10):1168-1170. PubMed
17. Lee TH, Marcantonio ER, Mangione CM, et al. Derivation and prospective validation of a simple index for prediction of cardiac risk of major noncardiac surgery. Circulation. 1999;100(10):1043-1049. PubMed
18. Gonzalez-Garcia L, Salmeron-Garcia A, Garcia-Lirola MA, Moya-Roldan S, Belda-Rustarazo S, Cabeza-Barrera J. Medication reconciliation at admission to surgical departments. J Eval Clin Pract. 2016;22(1):20-25. PubMed
19. Devereaux PJ, Mrkobrada M, Sessler DI, et al. Aspirin in patients undergoing noncardiac surgery. N Engl J Med. 2014;370(16):1494-1503. PubMed
20. Devereaux PJ, Yang H, Yusuf S, et al. Effects of extended-release metoprolol succinate in patients undergoing non-cardiac surgery (POISE trial): a randomised controlled trial. Lancet. 2008;371(9627):1839-1847. PubMed
21. Lindenauer PK, Pekow P, Wang K, Mamidi DK, Gutierrez B, Benjamin EM. Perioperative beta-blocker therapy and mortality after major noncardiac surgery. N Engl J Med. 2005;353(4):349-361. PubMed
22. London MJ, Hur K, Schwartz GG, Henderson WG. Association of perioperative beta-blockade with mortality and cardiovascular morbidity following major noncardiac surgery. JAMA. 2013;309(16):1704-1713. PubMed
23. Rosenman DJ, McDonald FS, Ebbert JO, Erwin PJ, LaBella M, Montori VM. Clinical consequences of withholding versus administering renin-angiotensin-aldosterone system antagonists in the preoperative period. J Hosp Med. 2008;3(4):319-325. PubMed
24. Andersson C, Merie C, Jorgensen M, et al. Association of beta-blocker therapy with risks of adverse cardiovascular events and deaths in patients with ischemic heart disease undergoing noncardiac surgery: a Danish nationwide cohort study. JAMA Intern Med. 2014;174(3):336-344. PubMed
25. Yancy CW, Jessup M, Bozkurt B, et al. 2013 ACCF/AHA Guideline for the Management of Heart Failure A Report of the American College of Cardiology Foundation/American Heart Association Task Force on Practice Guidelines. Circulation. 2013;128(16):E240-E327. PubMed
26. Kwan JL, Lo L, Sampson M, Shojania KG. Medication reconciliation during transitions of care as a patient safety strategy: a systematic review. Ann Intern Med. 2013;158(5 Pt 2):397-403. PubMed
27. Tam VC, Knowles SR, Cornish PL, Fine N, Marchesano R, Etchells EE. Frequency, type and clinical importance of medication history errors at admission to hospital: a systematic review. CMAJ. 2005;173(5):510-515. PubMed
28. Lindenauer PK, Pekow P, Wang K, Gutierrez B, Benjamin EM. Lipid-lowering therapy and in-hospital mortality following major noncardiac surgery. JAMA. 2004;291(17):2092-2099. PubMed
29. Raju MG, Pachika A, Punnam SR, et al. Statin Therapy in the Reduction of Cardiovascular Events in Patients Undergoing Intermediate-Risk Noncardiac, Nonvascular Surgery. Clin Cardiol. 2013;36(8):456-461. PubMed
© 2017 Society of Hospital Medicine
Caring Wisely: A Program to Support Frontline Clinicians and Staff in Improving Healthcare Delivery and Reducing Costs
© 2017 Society of Hospital Medicine
Strategies are needed to empower frontline clinicians to work with organizational leadership to reduce healthcare costs and improve high-value care. Caring Wisely® is a program developed by the University of California, San Francisco’s (UCSF) Center for Healthcare Value (CHV), aimed at engaging frontline clinicians and staff, connecting them with implementation experts, and supporting the development of targeted interventions to improve value. Financial savings from the program more than cover program costs. Caring Wisely® provides an institutional model for implementing robust interventions to address areas of low-value care.
Launched in 2013, the annual Caring Wisely® program consists of 3 stages for identifying projects that meet the following criteria:
- Potential to measurably reduce UCSF Health’s costs of care without transferring costs to patients, insurers, or other providers
- Plan for ensuring that health outcomes are maintained or improved
- Envision disseminating the intervention within and beyond UCSF
- Demonstrate commitment and engagement of clinical leadership and frontline staff.
The first stage is the Ideas Contest, a UCSF Health-wide call (to learn more about UCSF Health: https://www.ucsf.edu/sites/default/files/052516_About_UCSF.pdf) to identify areas that may be targeted to reduce unnecessary services, inefficiencies, and healthcare costs. We use a crowdsourcing platform—Open Proposals—to solicit the best ideas from frontline clinicians and staff.1 Open Proposals is a secure, web-based platform for transparent and collaborative proposal development that displays threads of comments, responses, and revisions, and allows submissions to be “liked.” Open Proposals is managed by the UCSF Clinical and Translational Science Institute, funded by the National Center for Advancing Translational Sciences (Grant Number UL1 TR000004) at the National Institutes of Health. Using institutional e-mail lists for faculty, staff and residents, as well as described at monthly managers and directors meetings, the Ideas Contest is announced each year by the Chief Medical Officer and the CHV leadership. The Caring Wisely® Executive Steering Committee, which consists of CHV and senior UCSF Health system leaders, selects the top 5-10 ideas based on the above criteria. Each winning idea receives a $100 gift certificate for a popular restaurant in San Francisco, and the list of winners is announced to the entire UCSF community.
The second stage is the Request for Proposals. The Caring Wisely® program solicits proposals that outline implementation plans to target specific areas identified through the Ideas Contest. Finalists from the Ideas Contest are encouraged to submit proposals that address the problem they identified, but anyone affiliated with UCSF Health may submit a proposal on a winning idea. There is an approximately 4-week open submission period during which applicants submit brief 2-page proposals on the Open Proposal platform. This is followed by a period of optimization that leverages the social media aspect of the Open Proposals platform in which the UCSF Health community asks clarifying questions, make suggestions, and modifications can be made to the proposals. All submissions receive written feedback from at least one Steering Committee member. In addition, the Caring Wisely® Director directly invites relevant UCSF colleagues, administrators, or program leaders to comment on proposals and make suggestions for improvement. Plans for assessing financial and health care delivery impacts are developed in collaboration with the UCSF Health Finance department. UCSF Health managers and leaders who are stakeholders in project proposal areas are consulted to provide input and finalize proposal plans, including the identification of existing personnel who can support and drive the project forward. Proposers use this feedback to revise their applications throughout this stage.
The third stage is Project Implementation. The Caring Wisely® Executive Steering Committee selects up to 3 winners from the submitted proposals. Using the program criteria above, each project is scored independently, discussed in committee, and rescored to identify the top proposals. Each selected project receives a maximum budget of $50,000 that can be used for project materials, activities, and salary support for project leaders or staff. In addition to funding, each project team receives input from the implementation science team to co-develop and implement the intervention with a goal of creating a first-test-of-change within 3-6 months. A key feature of Caring Wisely® is the partnership between project teams and the Caring Wisely® implementation team, which includes a director, program manager, data analysts, and implementation scientists (Table 1).
The $150,000 administrative budget for the Caring Wisely® program provides 20% support of the medical director, 50% support of a program manager/analyst, and 10% support of an implementation scientist. Approximately 5% support is donated from additional senior implementation scientists and various UCSF Health experts based on project needs. To make most efficient use of the Caring Wisely® program staff time with the project teams, there is a weekly 60-90 minute works-in-progress session attended by all 3 teams with a rotating schedule for lead presenter during the first 6 months; these meetings occur every 2-3 weeks during the second 6 months. Caring Wisely® program staff and the implementation scientist are also available for 1:1 meetings as needed. The Caring Wisely® Executive Steering Committee is not paid and meets for 90 minutes quarterly. Custom reports and modifications of the electronic health record are provided by the UCSF Health clinical informatics department as part of their operating budget.
The collaboration between the project teams and the implementation science team is guided by the Consolidated Framework for Implementation Research (CFIR)2 and PRECEDE-PROCEED model—a logic model and evaluation tool that is based on a composite of individual behavior change theory and social ecology.3 Table 2 illustrates how we weave PRECEDE-PROCEED and Plan-Do-Study-Act frameworks into project design and strategy. Each funded team is required to submit an end-of-year progress report.
Cost and cost savings estimates were based on administrative financial data obtained through the assistance of the Decision Support Services unit of the Finance Department of UCSF Health. All costs reflect direct institutional costs, rather than charges. For some projects, costs are directly available through computerized dashboards that provide year-to-year comparisons of specific costs of materials, supplies, and services (eg, blood transfusion reduction, surgical supplies project, OR efficiency program). This same dashboard also allows calculation of CMI-adjusted direct costs of hospital care by service line, as used in the perioperative pathways program evaluation. In other cases, the Decision Support Services and/or Caring Wisely® program manager created custom cost reports based on the key performance indicator (eg, nebulizer therapy costs consist of medication costs plus respiratory therapist time; CT scan utilization for suspected pulmonary embolus in emergency department; and antimicrobial utilization for suspected neonatal sepsis).
Ongoing monitoring and sustainability of Caring Wisely® projects is supported by the Caring Wisely® program leaders. Monitoring of ongoing cost savings is based on automated service-line level dashboards related to cost, utilization, and quality outcomes with quarterly updates provided to the Caring Wisely® Steering Committee. Depending on the project or program, appropriate UCSF Health senior leaders determine the level of support within their departments that is required to sustain the program(s). Ongoing monitoring of each program is also included in the strategic deployment visibility room with regular rounding by senior health system executives.
Since 2013, there have been 3 complete Caring Wisely® cycles. The Ideas Contest generated more than 75 ideas in each of the past 3 cycles, ranging from eliminating redundant laboratory or radiological studies to reducing linen and food waste. We received between 13-20 full proposals in each of the request for proposal stages, and 9 projects have been implemented, 3 in each year. Funded projects have been led by a variety of individuals including physicians, nurses, pharmacists, administrators and residents, and topics have ranged from reducing overutilization of tests, supplies and treatments, to improving patient throughput during the perioperative period (Table 3). Estimated cumulative savings to date from Caring Wisely® projects has exceeded $4 million, based on the four projects shown in Table 4. The IV-to-PO switch program and the neonatal sepsis risk prediction project (Table 3) have been successful in reducing unnecessary utilization, but cost and savings estimates are not yet finalized. Three funded projects were equivocal in cost savings but were successful in their primary aims: (1) increasing the appropriateness of CT scan ordering for suspected pulmonary embolus; (2) shortening operating room turnover times; and (3) implementing a postoperative debrief program for the systematic documentation of safety events, waste, and inefficiencies related to surgery.
We developed an innovative program that reduces hospital costs through crowdsourcing of ideas from frontline clinicians and staff, and by connecting these ideas to project and implementation science teams. At a time when healthcare costs have reached unsustainable levels, the Caring Wisely® program provides a process for healthcare personnel to make a positive impact on healthcare costs in areas under their direct control. Through the Open Proposals platform, we have tapped a growing desire among frontline providers to reduce medical waste.
A key criterion for the Caring Wisely® program is to propose changes that reduce cost without adversely affect healthcare quality or outcomes. While this is an important consideration in selecting projects, there is limited power to detect many of the most clinically relevant outcomes. We find this acceptable because many of the sponsored Caring Wisely® project goals were to increase compliance with evidence-based practice guidelines and reduce harms associated with unnecessary treatments (eg, blood transfusion, nebulizer therapy, CT scan, antimicrobial therapy). Selected balancing metrics for each project are reported by established quality and safety programs at UCSF Health, but we acknowledge that many factors that can affect these clinical outcomes are not related to the cost-reduction intervention and are not possible to control outside of a clinical research study. Therefore, any response to changes in these outcome and balancing measures requires further analysis beyond the Caring Wisely® project alone.
We believe one of the key factors in the success of the Caring Wisely® program is the application of implementation science principles to the intervention design strategies (Table 1). These principles included stakeholder engagement, behavior change theory, market (target audience) segmentation, and process measurement and feedback. Because we are conducting this program in an academic health center, resident and fellow education and engagement are also critical to success. In each project, we utilize the PRECEDE model as a guide to ensure that each intervention design includes complementary elements of effective behavior change, intended to increase awareness and motivation to change, to make change “easy,” and to reinforce change(Table 2).3
The Caring Wisely® program—itself a multifaceted intervention—embodies the same PRECEDE dimensions we apply to each specific project. The Ideas Contest serves as a tool for increasing awareness, attitudes, and motivation across the clinical enterprise for reducing healthcare costs. The support provided to the project teams by the Caring Wisely® program is an enabling factor that makes it “easier” for frontline teams to design and implement interventions with a greater likelihood of achieving early success. Timely measurement and feedback of results to the hospital leadership and broadcasting to the larger community reinforces the support of the program at both the leadership and frontline levels.
Collaboration between project teams and the Caring Wisely® program also provides frontline clinicians and staff with practical experience and lessons that they can apply to future improvement work. Project teams learn implementation science principles such as constructing a pragmatic theoretical framework to guide implementation design using CFIR model.2 Incorporating multiple, rapid-cycle tests of change allows teams to modify and adapt final interventions as they learn how the target audience and environment responds to specific intervention components. Access to real-time, actionable data and a data analyst is essential to rapid cycle adaptation that allows teams to focus on specific units or providers. We also find that cross-fertilization between project teams working in different areas helps to share resources and minimize duplication of efforts from the clinical and staff champions. Partnering with UCSF Health system leaders at every phase of project development—from proposal selection, development, and final evaluation of results—enhances sustainable transition of successful projects into clinical operations.
The costs and coordination for the first cycle of Caring Wisely® were supported by the UCSF Center for Healthcare Value. Upon completion of the evaluation of the first cycle, UCSF Health agreed to fund the program going forward, with the expectation that Caring Wisely would continue to achieve direct cost-savings for the organization. The Caring Wisely team provides a final report each year detailing the impact of each project on utilization and associated costs. Currently, program costs are approximately $150,000 for the Caring Wisely program leaders, staff, and other resources, and $50,000 for each of 3 projects for a total program cost of $300,000 per year. Projects included in the first three cycles have already saved more than $4 million, representing a strong return on investment. This program could be a model for other academic health centers to engage frontline clinicians and staff in addressing healthcare costs, and lends itself to being scaled-up into a multi-system collaborative.
LIST OF ABBREVIATIONS
UCSF—University of California, San Francisco; PRECEDE—Predisposing, Reinforcing, and Enabling Constructs in Educational Diagnosis and Evaluation; PROCEED—Policy, Regulatory and Organizational Constructs in Educational and Environmental Development
Acknowledgments
Other participants in blood transfusion reduction project (D. Johnson, K. Curcione); IV-to-PO Switch (C. Tsourounis, A. Pollock); Surgical Supply Cost Reduction (C. Zygourakis); Perioperative Efficiency (L. Hampson); CT for PE Risk Prediction (E. Weber); ERAS Pathways (L. Chen); Neonatal Sepsis Risk Prediction (T. Newman); Post-Operative Debrief (S. Imershein). Caring Wisely Executive Steering Committee (J. Adler, S. Antrum, A Auerbach, J. Bennan, M. Blum, C. Ritchie, C. Tsourounis). This Center for Healthcare Value is funded in part by a grant from the Grove Foundation. We appreciate additional review and comments to the manuscript provided by George Sawaya and Adams Dudley.
Disclosures
Christopher Moriates has accepted royalties from McGraw-Hill for textbook, Understanding Value-Based Healthcare. Alvin Rajkomar has received fees as a research adviser from Google, Inc.
1. Kahlon M, Yuan L, Gologorskaya O, Johnston SC. Crowdsourcing the CTSA innovation mission. Clin Transl Sci. 2014;7:89-92. PubMed
2. Damschroder LJ, Aron DC, Keith RE, Kirsh SR, Alexander JA, Lowery JC. Fostering implementation of health services research findings into practice: a consolidated framework for advancing implementation science. Implement Sci. 2009;4:50. PubMed
3. Green LW and Kreuter. Health Program Planning: An Educational and Ecological Approach. 4th Ed. McGraw-Hill. New York, NY. 2005.
4. Zygourakis CC, Valencia V, Moriates C et al. Association between surgeon scorecard use and operating room costs. JAMA Surg. 2016 Dec 7. doi: 10.1001/jamasurg.2016.4674. [Epub ahead of print] PubMed
© 2017 Society of Hospital Medicine
Strategies are needed to empower frontline clinicians to work with organizational leadership to reduce healthcare costs and improve high-value care. Caring Wisely® is a program developed by the University of California, San Francisco’s (UCSF) Center for Healthcare Value (CHV), aimed at engaging frontline clinicians and staff, connecting them with implementation experts, and supporting the development of targeted interventions to improve value. Financial savings from the program more than cover program costs. Caring Wisely® provides an institutional model for implementing robust interventions to address areas of low-value care.
Launched in 2013, the annual Caring Wisely® program consists of 3 stages for identifying projects that meet the following criteria:
- Potential to measurably reduce UCSF Health’s costs of care without transferring costs to patients, insurers, or other providers
- Plan for ensuring that health outcomes are maintained or improved
- Envision disseminating the intervention within and beyond UCSF
- Demonstrate commitment and engagement of clinical leadership and frontline staff.
The first stage is the Ideas Contest, a UCSF Health-wide call (to learn more about UCSF Health: https://www.ucsf.edu/sites/default/files/052516_About_UCSF.pdf) to identify areas that may be targeted to reduce unnecessary services, inefficiencies, and healthcare costs. We use a crowdsourcing platform—Open Proposals—to solicit the best ideas from frontline clinicians and staff.1 Open Proposals is a secure, web-based platform for transparent and collaborative proposal development that displays threads of comments, responses, and revisions, and allows submissions to be “liked.” Open Proposals is managed by the UCSF Clinical and Translational Science Institute, funded by the National Center for Advancing Translational Sciences (Grant Number UL1 TR000004) at the National Institutes of Health. Using institutional e-mail lists for faculty, staff and residents, as well as described at monthly managers and directors meetings, the Ideas Contest is announced each year by the Chief Medical Officer and the CHV leadership. The Caring Wisely® Executive Steering Committee, which consists of CHV and senior UCSF Health system leaders, selects the top 5-10 ideas based on the above criteria. Each winning idea receives a $100 gift certificate for a popular restaurant in San Francisco, and the list of winners is announced to the entire UCSF community.
The second stage is the Request for Proposals. The Caring Wisely® program solicits proposals that outline implementation plans to target specific areas identified through the Ideas Contest. Finalists from the Ideas Contest are encouraged to submit proposals that address the problem they identified, but anyone affiliated with UCSF Health may submit a proposal on a winning idea. There is an approximately 4-week open submission period during which applicants submit brief 2-page proposals on the Open Proposal platform. This is followed by a period of optimization that leverages the social media aspect of the Open Proposals platform in which the UCSF Health community asks clarifying questions, make suggestions, and modifications can be made to the proposals. All submissions receive written feedback from at least one Steering Committee member. In addition, the Caring Wisely® Director directly invites relevant UCSF colleagues, administrators, or program leaders to comment on proposals and make suggestions for improvement. Plans for assessing financial and health care delivery impacts are developed in collaboration with the UCSF Health Finance department. UCSF Health managers and leaders who are stakeholders in project proposal areas are consulted to provide input and finalize proposal plans, including the identification of existing personnel who can support and drive the project forward. Proposers use this feedback to revise their applications throughout this stage.
The third stage is Project Implementation. The Caring Wisely® Executive Steering Committee selects up to 3 winners from the submitted proposals. Using the program criteria above, each project is scored independently, discussed in committee, and rescored to identify the top proposals. Each selected project receives a maximum budget of $50,000 that can be used for project materials, activities, and salary support for project leaders or staff. In addition to funding, each project team receives input from the implementation science team to co-develop and implement the intervention with a goal of creating a first-test-of-change within 3-6 months. A key feature of Caring Wisely® is the partnership between project teams and the Caring Wisely® implementation team, which includes a director, program manager, data analysts, and implementation scientists (Table 1).
The $150,000 administrative budget for the Caring Wisely® program provides 20% support of the medical director, 50% support of a program manager/analyst, and 10% support of an implementation scientist. Approximately 5% support is donated from additional senior implementation scientists and various UCSF Health experts based on project needs. To make most efficient use of the Caring Wisely® program staff time with the project teams, there is a weekly 60-90 minute works-in-progress session attended by all 3 teams with a rotating schedule for lead presenter during the first 6 months; these meetings occur every 2-3 weeks during the second 6 months. Caring Wisely® program staff and the implementation scientist are also available for 1:1 meetings as needed. The Caring Wisely® Executive Steering Committee is not paid and meets for 90 minutes quarterly. Custom reports and modifications of the electronic health record are provided by the UCSF Health clinical informatics department as part of their operating budget.
The collaboration between the project teams and the implementation science team is guided by the Consolidated Framework for Implementation Research (CFIR)2 and PRECEDE-PROCEED model—a logic model and evaluation tool that is based on a composite of individual behavior change theory and social ecology.3 Table 2 illustrates how we weave PRECEDE-PROCEED and Plan-Do-Study-Act frameworks into project design and strategy. Each funded team is required to submit an end-of-year progress report.
Cost and cost savings estimates were based on administrative financial data obtained through the assistance of the Decision Support Services unit of the Finance Department of UCSF Health. All costs reflect direct institutional costs, rather than charges. For some projects, costs are directly available through computerized dashboards that provide year-to-year comparisons of specific costs of materials, supplies, and services (eg, blood transfusion reduction, surgical supplies project, OR efficiency program). This same dashboard also allows calculation of CMI-adjusted direct costs of hospital care by service line, as used in the perioperative pathways program evaluation. In other cases, the Decision Support Services and/or Caring Wisely® program manager created custom cost reports based on the key performance indicator (eg, nebulizer therapy costs consist of medication costs plus respiratory therapist time; CT scan utilization for suspected pulmonary embolus in emergency department; and antimicrobial utilization for suspected neonatal sepsis).
Ongoing monitoring and sustainability of Caring Wisely® projects is supported by the Caring Wisely® program leaders. Monitoring of ongoing cost savings is based on automated service-line level dashboards related to cost, utilization, and quality outcomes with quarterly updates provided to the Caring Wisely® Steering Committee. Depending on the project or program, appropriate UCSF Health senior leaders determine the level of support within their departments that is required to sustain the program(s). Ongoing monitoring of each program is also included in the strategic deployment visibility room with regular rounding by senior health system executives.
Since 2013, there have been 3 complete Caring Wisely® cycles. The Ideas Contest generated more than 75 ideas in each of the past 3 cycles, ranging from eliminating redundant laboratory or radiological studies to reducing linen and food waste. We received between 13-20 full proposals in each of the request for proposal stages, and 9 projects have been implemented, 3 in each year. Funded projects have been led by a variety of individuals including physicians, nurses, pharmacists, administrators and residents, and topics have ranged from reducing overutilization of tests, supplies and treatments, to improving patient throughput during the perioperative period (Table 3). Estimated cumulative savings to date from Caring Wisely® projects has exceeded $4 million, based on the four projects shown in Table 4. The IV-to-PO switch program and the neonatal sepsis risk prediction project (Table 3) have been successful in reducing unnecessary utilization, but cost and savings estimates are not yet finalized. Three funded projects were equivocal in cost savings but were successful in their primary aims: (1) increasing the appropriateness of CT scan ordering for suspected pulmonary embolus; (2) shortening operating room turnover times; and (3) implementing a postoperative debrief program for the systematic documentation of safety events, waste, and inefficiencies related to surgery.
We developed an innovative program that reduces hospital costs through crowdsourcing of ideas from frontline clinicians and staff, and by connecting these ideas to project and implementation science teams. At a time when healthcare costs have reached unsustainable levels, the Caring Wisely® program provides a process for healthcare personnel to make a positive impact on healthcare costs in areas under their direct control. Through the Open Proposals platform, we have tapped a growing desire among frontline providers to reduce medical waste.
A key criterion for the Caring Wisely® program is to propose changes that reduce cost without adversely affect healthcare quality or outcomes. While this is an important consideration in selecting projects, there is limited power to detect many of the most clinically relevant outcomes. We find this acceptable because many of the sponsored Caring Wisely® project goals were to increase compliance with evidence-based practice guidelines and reduce harms associated with unnecessary treatments (eg, blood transfusion, nebulizer therapy, CT scan, antimicrobial therapy). Selected balancing metrics for each project are reported by established quality and safety programs at UCSF Health, but we acknowledge that many factors that can affect these clinical outcomes are not related to the cost-reduction intervention and are not possible to control outside of a clinical research study. Therefore, any response to changes in these outcome and balancing measures requires further analysis beyond the Caring Wisely® project alone.
We believe one of the key factors in the success of the Caring Wisely® program is the application of implementation science principles to the intervention design strategies (Table 1). These principles included stakeholder engagement, behavior change theory, market (target audience) segmentation, and process measurement and feedback. Because we are conducting this program in an academic health center, resident and fellow education and engagement are also critical to success. In each project, we utilize the PRECEDE model as a guide to ensure that each intervention design includes complementary elements of effective behavior change, intended to increase awareness and motivation to change, to make change “easy,” and to reinforce change(Table 2).3
The Caring Wisely® program—itself a multifaceted intervention—embodies the same PRECEDE dimensions we apply to each specific project. The Ideas Contest serves as a tool for increasing awareness, attitudes, and motivation across the clinical enterprise for reducing healthcare costs. The support provided to the project teams by the Caring Wisely® program is an enabling factor that makes it “easier” for frontline teams to design and implement interventions with a greater likelihood of achieving early success. Timely measurement and feedback of results to the hospital leadership and broadcasting to the larger community reinforces the support of the program at both the leadership and frontline levels.
Collaboration between project teams and the Caring Wisely® program also provides frontline clinicians and staff with practical experience and lessons that they can apply to future improvement work. Project teams learn implementation science principles such as constructing a pragmatic theoretical framework to guide implementation design using CFIR model.2 Incorporating multiple, rapid-cycle tests of change allows teams to modify and adapt final interventions as they learn how the target audience and environment responds to specific intervention components. Access to real-time, actionable data and a data analyst is essential to rapid cycle adaptation that allows teams to focus on specific units or providers. We also find that cross-fertilization between project teams working in different areas helps to share resources and minimize duplication of efforts from the clinical and staff champions. Partnering with UCSF Health system leaders at every phase of project development—from proposal selection, development, and final evaluation of results—enhances sustainable transition of successful projects into clinical operations.
The costs and coordination for the first cycle of Caring Wisely® were supported by the UCSF Center for Healthcare Value. Upon completion of the evaluation of the first cycle, UCSF Health agreed to fund the program going forward, with the expectation that Caring Wisely would continue to achieve direct cost-savings for the organization. The Caring Wisely team provides a final report each year detailing the impact of each project on utilization and associated costs. Currently, program costs are approximately $150,000 for the Caring Wisely program leaders, staff, and other resources, and $50,000 for each of 3 projects for a total program cost of $300,000 per year. Projects included in the first three cycles have already saved more than $4 million, representing a strong return on investment. This program could be a model for other academic health centers to engage frontline clinicians and staff in addressing healthcare costs, and lends itself to being scaled-up into a multi-system collaborative.
LIST OF ABBREVIATIONS
UCSF—University of California, San Francisco; PRECEDE—Predisposing, Reinforcing, and Enabling Constructs in Educational Diagnosis and Evaluation; PROCEED—Policy, Regulatory and Organizational Constructs in Educational and Environmental Development
Acknowledgments
Other participants in blood transfusion reduction project (D. Johnson, K. Curcione); IV-to-PO Switch (C. Tsourounis, A. Pollock); Surgical Supply Cost Reduction (C. Zygourakis); Perioperative Efficiency (L. Hampson); CT for PE Risk Prediction (E. Weber); ERAS Pathways (L. Chen); Neonatal Sepsis Risk Prediction (T. Newman); Post-Operative Debrief (S. Imershein). Caring Wisely Executive Steering Committee (J. Adler, S. Antrum, A Auerbach, J. Bennan, M. Blum, C. Ritchie, C. Tsourounis). This Center for Healthcare Value is funded in part by a grant from the Grove Foundation. We appreciate additional review and comments to the manuscript provided by George Sawaya and Adams Dudley.
Disclosures
Christopher Moriates has accepted royalties from McGraw-Hill for textbook, Understanding Value-Based Healthcare. Alvin Rajkomar has received fees as a research adviser from Google, Inc.
© 2017 Society of Hospital Medicine
Strategies are needed to empower frontline clinicians to work with organizational leadership to reduce healthcare costs and improve high-value care. Caring Wisely® is a program developed by the University of California, San Francisco’s (UCSF) Center for Healthcare Value (CHV), aimed at engaging frontline clinicians and staff, connecting them with implementation experts, and supporting the development of targeted interventions to improve value. Financial savings from the program more than cover program costs. Caring Wisely® provides an institutional model for implementing robust interventions to address areas of low-value care.
Launched in 2013, the annual Caring Wisely® program consists of 3 stages for identifying projects that meet the following criteria:
- Potential to measurably reduce UCSF Health’s costs of care without transferring costs to patients, insurers, or other providers
- Plan for ensuring that health outcomes are maintained or improved
- Envision disseminating the intervention within and beyond UCSF
- Demonstrate commitment and engagement of clinical leadership and frontline staff.
The first stage is the Ideas Contest, a UCSF Health-wide call (to learn more about UCSF Health: https://www.ucsf.edu/sites/default/files/052516_About_UCSF.pdf) to identify areas that may be targeted to reduce unnecessary services, inefficiencies, and healthcare costs. We use a crowdsourcing platform—Open Proposals—to solicit the best ideas from frontline clinicians and staff.1 Open Proposals is a secure, web-based platform for transparent and collaborative proposal development that displays threads of comments, responses, and revisions, and allows submissions to be “liked.” Open Proposals is managed by the UCSF Clinical and Translational Science Institute, funded by the National Center for Advancing Translational Sciences (Grant Number UL1 TR000004) at the National Institutes of Health. Using institutional e-mail lists for faculty, staff and residents, as well as described at monthly managers and directors meetings, the Ideas Contest is announced each year by the Chief Medical Officer and the CHV leadership. The Caring Wisely® Executive Steering Committee, which consists of CHV and senior UCSF Health system leaders, selects the top 5-10 ideas based on the above criteria. Each winning idea receives a $100 gift certificate for a popular restaurant in San Francisco, and the list of winners is announced to the entire UCSF community.
The second stage is the Request for Proposals. The Caring Wisely® program solicits proposals that outline implementation plans to target specific areas identified through the Ideas Contest. Finalists from the Ideas Contest are encouraged to submit proposals that address the problem they identified, but anyone affiliated with UCSF Health may submit a proposal on a winning idea. There is an approximately 4-week open submission period during which applicants submit brief 2-page proposals on the Open Proposal platform. This is followed by a period of optimization that leverages the social media aspect of the Open Proposals platform in which the UCSF Health community asks clarifying questions, make suggestions, and modifications can be made to the proposals. All submissions receive written feedback from at least one Steering Committee member. In addition, the Caring Wisely® Director directly invites relevant UCSF colleagues, administrators, or program leaders to comment on proposals and make suggestions for improvement. Plans for assessing financial and health care delivery impacts are developed in collaboration with the UCSF Health Finance department. UCSF Health managers and leaders who are stakeholders in project proposal areas are consulted to provide input and finalize proposal plans, including the identification of existing personnel who can support and drive the project forward. Proposers use this feedback to revise their applications throughout this stage.
The third stage is Project Implementation. The Caring Wisely® Executive Steering Committee selects up to 3 winners from the submitted proposals. Using the program criteria above, each project is scored independently, discussed in committee, and rescored to identify the top proposals. Each selected project receives a maximum budget of $50,000 that can be used for project materials, activities, and salary support for project leaders or staff. In addition to funding, each project team receives input from the implementation science team to co-develop and implement the intervention with a goal of creating a first-test-of-change within 3-6 months. A key feature of Caring Wisely® is the partnership between project teams and the Caring Wisely® implementation team, which includes a director, program manager, data analysts, and implementation scientists (Table 1).
The $150,000 administrative budget for the Caring Wisely® program provides 20% support of the medical director, 50% support of a program manager/analyst, and 10% support of an implementation scientist. Approximately 5% support is donated from additional senior implementation scientists and various UCSF Health experts based on project needs. To make most efficient use of the Caring Wisely® program staff time with the project teams, there is a weekly 60-90 minute works-in-progress session attended by all 3 teams with a rotating schedule for lead presenter during the first 6 months; these meetings occur every 2-3 weeks during the second 6 months. Caring Wisely® program staff and the implementation scientist are also available for 1:1 meetings as needed. The Caring Wisely® Executive Steering Committee is not paid and meets for 90 minutes quarterly. Custom reports and modifications of the electronic health record are provided by the UCSF Health clinical informatics department as part of their operating budget.
The collaboration between the project teams and the implementation science team is guided by the Consolidated Framework for Implementation Research (CFIR)2 and PRECEDE-PROCEED model—a logic model and evaluation tool that is based on a composite of individual behavior change theory and social ecology.3 Table 2 illustrates how we weave PRECEDE-PROCEED and Plan-Do-Study-Act frameworks into project design and strategy. Each funded team is required to submit an end-of-year progress report.
Cost and cost savings estimates were based on administrative financial data obtained through the assistance of the Decision Support Services unit of the Finance Department of UCSF Health. All costs reflect direct institutional costs, rather than charges. For some projects, costs are directly available through computerized dashboards that provide year-to-year comparisons of specific costs of materials, supplies, and services (eg, blood transfusion reduction, surgical supplies project, OR efficiency program). This same dashboard also allows calculation of CMI-adjusted direct costs of hospital care by service line, as used in the perioperative pathways program evaluation. In other cases, the Decision Support Services and/or Caring Wisely® program manager created custom cost reports based on the key performance indicator (eg, nebulizer therapy costs consist of medication costs plus respiratory therapist time; CT scan utilization for suspected pulmonary embolus in emergency department; and antimicrobial utilization for suspected neonatal sepsis).
Ongoing monitoring and sustainability of Caring Wisely® projects is supported by the Caring Wisely® program leaders. Monitoring of ongoing cost savings is based on automated service-line level dashboards related to cost, utilization, and quality outcomes with quarterly updates provided to the Caring Wisely® Steering Committee. Depending on the project or program, appropriate UCSF Health senior leaders determine the level of support within their departments that is required to sustain the program(s). Ongoing monitoring of each program is also included in the strategic deployment visibility room with regular rounding by senior health system executives.
Since 2013, there have been 3 complete Caring Wisely® cycles. The Ideas Contest generated more than 75 ideas in each of the past 3 cycles, ranging from eliminating redundant laboratory or radiological studies to reducing linen and food waste. We received between 13-20 full proposals in each of the request for proposal stages, and 9 projects have been implemented, 3 in each year. Funded projects have been led by a variety of individuals including physicians, nurses, pharmacists, administrators and residents, and topics have ranged from reducing overutilization of tests, supplies and treatments, to improving patient throughput during the perioperative period (Table 3). Estimated cumulative savings to date from Caring Wisely® projects has exceeded $4 million, based on the four projects shown in Table 4. The IV-to-PO switch program and the neonatal sepsis risk prediction project (Table 3) have been successful in reducing unnecessary utilization, but cost and savings estimates are not yet finalized. Three funded projects were equivocal in cost savings but were successful in their primary aims: (1) increasing the appropriateness of CT scan ordering for suspected pulmonary embolus; (2) shortening operating room turnover times; and (3) implementing a postoperative debrief program for the systematic documentation of safety events, waste, and inefficiencies related to surgery.
We developed an innovative program that reduces hospital costs through crowdsourcing of ideas from frontline clinicians and staff, and by connecting these ideas to project and implementation science teams. At a time when healthcare costs have reached unsustainable levels, the Caring Wisely® program provides a process for healthcare personnel to make a positive impact on healthcare costs in areas under their direct control. Through the Open Proposals platform, we have tapped a growing desire among frontline providers to reduce medical waste.
A key criterion for the Caring Wisely® program is to propose changes that reduce cost without adversely affect healthcare quality or outcomes. While this is an important consideration in selecting projects, there is limited power to detect many of the most clinically relevant outcomes. We find this acceptable because many of the sponsored Caring Wisely® project goals were to increase compliance with evidence-based practice guidelines and reduce harms associated with unnecessary treatments (eg, blood transfusion, nebulizer therapy, CT scan, antimicrobial therapy). Selected balancing metrics for each project are reported by established quality and safety programs at UCSF Health, but we acknowledge that many factors that can affect these clinical outcomes are not related to the cost-reduction intervention and are not possible to control outside of a clinical research study. Therefore, any response to changes in these outcome and balancing measures requires further analysis beyond the Caring Wisely® project alone.
We believe one of the key factors in the success of the Caring Wisely® program is the application of implementation science principles to the intervention design strategies (Table 1). These principles included stakeholder engagement, behavior change theory, market (target audience) segmentation, and process measurement and feedback. Because we are conducting this program in an academic health center, resident and fellow education and engagement are also critical to success. In each project, we utilize the PRECEDE model as a guide to ensure that each intervention design includes complementary elements of effective behavior change, intended to increase awareness and motivation to change, to make change “easy,” and to reinforce change(Table 2).3
The Caring Wisely® program—itself a multifaceted intervention—embodies the same PRECEDE dimensions we apply to each specific project. The Ideas Contest serves as a tool for increasing awareness, attitudes, and motivation across the clinical enterprise for reducing healthcare costs. The support provided to the project teams by the Caring Wisely® program is an enabling factor that makes it “easier” for frontline teams to design and implement interventions with a greater likelihood of achieving early success. Timely measurement and feedback of results to the hospital leadership and broadcasting to the larger community reinforces the support of the program at both the leadership and frontline levels.
Collaboration between project teams and the Caring Wisely® program also provides frontline clinicians and staff with practical experience and lessons that they can apply to future improvement work. Project teams learn implementation science principles such as constructing a pragmatic theoretical framework to guide implementation design using CFIR model.2 Incorporating multiple, rapid-cycle tests of change allows teams to modify and adapt final interventions as they learn how the target audience and environment responds to specific intervention components. Access to real-time, actionable data and a data analyst is essential to rapid cycle adaptation that allows teams to focus on specific units or providers. We also find that cross-fertilization between project teams working in different areas helps to share resources and minimize duplication of efforts from the clinical and staff champions. Partnering with UCSF Health system leaders at every phase of project development—from proposal selection, development, and final evaluation of results—enhances sustainable transition of successful projects into clinical operations.
The costs and coordination for the first cycle of Caring Wisely® were supported by the UCSF Center for Healthcare Value. Upon completion of the evaluation of the first cycle, UCSF Health agreed to fund the program going forward, with the expectation that Caring Wisely would continue to achieve direct cost-savings for the organization. The Caring Wisely team provides a final report each year detailing the impact of each project on utilization and associated costs. Currently, program costs are approximately $150,000 for the Caring Wisely program leaders, staff, and other resources, and $50,000 for each of 3 projects for a total program cost of $300,000 per year. Projects included in the first three cycles have already saved more than $4 million, representing a strong return on investment. This program could be a model for other academic health centers to engage frontline clinicians and staff in addressing healthcare costs, and lends itself to being scaled-up into a multi-system collaborative.
LIST OF ABBREVIATIONS
UCSF—University of California, San Francisco; PRECEDE—Predisposing, Reinforcing, and Enabling Constructs in Educational Diagnosis and Evaluation; PROCEED—Policy, Regulatory and Organizational Constructs in Educational and Environmental Development
Acknowledgments
Other participants in blood transfusion reduction project (D. Johnson, K. Curcione); IV-to-PO Switch (C. Tsourounis, A. Pollock); Surgical Supply Cost Reduction (C. Zygourakis); Perioperative Efficiency (L. Hampson); CT for PE Risk Prediction (E. Weber); ERAS Pathways (L. Chen); Neonatal Sepsis Risk Prediction (T. Newman); Post-Operative Debrief (S. Imershein). Caring Wisely Executive Steering Committee (J. Adler, S. Antrum, A Auerbach, J. Bennan, M. Blum, C. Ritchie, C. Tsourounis). This Center for Healthcare Value is funded in part by a grant from the Grove Foundation. We appreciate additional review and comments to the manuscript provided by George Sawaya and Adams Dudley.
Disclosures
Christopher Moriates has accepted royalties from McGraw-Hill for textbook, Understanding Value-Based Healthcare. Alvin Rajkomar has received fees as a research adviser from Google, Inc.
1. Kahlon M, Yuan L, Gologorskaya O, Johnston SC. Crowdsourcing the CTSA innovation mission. Clin Transl Sci. 2014;7:89-92. PubMed
2. Damschroder LJ, Aron DC, Keith RE, Kirsh SR, Alexander JA, Lowery JC. Fostering implementation of health services research findings into practice: a consolidated framework for advancing implementation science. Implement Sci. 2009;4:50. PubMed
3. Green LW and Kreuter. Health Program Planning: An Educational and Ecological Approach. 4th Ed. McGraw-Hill. New York, NY. 2005.
4. Zygourakis CC, Valencia V, Moriates C et al. Association between surgeon scorecard use and operating room costs. JAMA Surg. 2016 Dec 7. doi: 10.1001/jamasurg.2016.4674. [Epub ahead of print] PubMed
1. Kahlon M, Yuan L, Gologorskaya O, Johnston SC. Crowdsourcing the CTSA innovation mission. Clin Transl Sci. 2014;7:89-92. PubMed
2. Damschroder LJ, Aron DC, Keith RE, Kirsh SR, Alexander JA, Lowery JC. Fostering implementation of health services research findings into practice: a consolidated framework for advancing implementation science. Implement Sci. 2009;4:50. PubMed
3. Green LW and Kreuter. Health Program Planning: An Educational and Ecological Approach. 4th Ed. McGraw-Hill. New York, NY. 2005.
4. Zygourakis CC, Valencia V, Moriates C et al. Association between surgeon scorecard use and operating room costs. JAMA Surg. 2016 Dec 7. doi: 10.1001/jamasurg.2016.4674. [Epub ahead of print] PubMed
Readmissions in Primary Care Clinics
Reducing hospital readmissions is a national healthcare priority. In October 2012, the Centers for Medicare and Medicaid Services (CMS) enacted financial penalties on hospitals with higher than average risk‐adjusted readmissions, offering an incentive pool of $850 million in the first year.[1] As a result, a wide range of activities to understand and reduce readmissions among patients with congestive heart failure (CHF), acute myocardial infarction (AMI), and pneumonia have emerged.[2, 3, 4, 5] Some have proposed that to effectively reduce hospital readmissions, a community of inpatient and outpatient providers and local support organizations must coordinate efforts.[6, 7] In fact, CMS has recognized the important role of community support groups and outpatient providers in safe discharge transitions in 2 ways. First, in 2011 CMS launched the Community‐based Care Transitions Program to fund community‐based organizations to assist Medicare patients with care transitions.[8] Second, CMS introduced 2 new reimbursement codes for primary care providers (PCPs) to perform care coordination immediately after hospital discharge.[9] Both of these new payment programs represent an evolving perspective that reducing hospital readmissions requires active participation among outpatient partners.
As leaders of the outpatient care team, PCPs play a significant role in reducing hospital readmissions. One way in which PCPs can begin to understand the magnitude of the issue within their clinic is to evaluate the clinic's 30‐day readmission rates. Currently, CMS calculates readmission rates at the hospital level. However, understanding these rates at the clinic level is critical for developing strategies for improvement across the care continuum. Our current understanding of effective outpatient interventions to reduce hospital readmission is limited.[3] As clinics introduce and refine strategies to reduce readmissions, tracking the impact of these strategies on readmission rates will be critical for identifying effective outpatient interventions. Clinics with similar patient case‐mix can also benchmark readmission rates, sharing best practices from clinics with lower‐than‐expected rates.
There are no available studies or proposed methodologies to guide primary care clinics in calculating their 30‐day readmission rates. A particularly difficult challenge is obtaining the admission information when patients may be admitted to 1 of several area hospitals. For large integrated delivery networks where primary care patients are relatively loyal to the network of physicians and hospitals, an opportunity exists to explore the data. Furthermore, variations in readmission rates across primary care specialties (such as internal medicine and family practice) are not well understood. In this study, we set out to develop a methodology for calculating all‐cause 30‐day hospital readmission rates at the level of individual primary care practices and to identify factors associated with variations in these rates.
METHODS
Study Design
We conducted a retrospective observational study of adult primary care patients at the University of California, San Francisco (UCSF) who were hospitalized at UCSF Medical Center between July 1, 2009 and June 30, 2012. UCSF Medical Center is comprised of Moffitt‐Long Hospital (a 600‐bed facility) and UCSF‐Mount Zion Hospital (a 90‐bed facility) located in San Francisco, CA. The patient population was limited to adults ages 18 and over with a PCP at UCSF. UCSF has 7 adult primary care clinics: General Internal Medicine (IM), Family Practice (FP), Women's Health, Geriatrics, a combined IM/FP clinic, Human Immunodeficiency Virus (HIV) Primary Care, and a Concierge Internal Medicine clinic staffed by IM physicians. Between 2009 and 2011, all clinics completed the process of enpanelment, or defining the population of patients for which each PCP and the clinic is responsible. We obtained the list of patient and PCP assignments at each of the 7 clinics across the time period of study. We then obtained UCSF Medical Center hospital claims data for this group, including dates of admission and discharge, patient age, sex, race/ethnicity, language, insurance, admitting service, diagnosis codes, information on intensive care unit stay, and discharge disposition. Hospital claims data are housed in Transition Systems International (Boston, MA) administrative databases, a cost‐accounting system that collects data abstracted from patient charts upon discharge from UCSF Medical Center.
All‐cause 30‐day hospital readmission rates were calculated for each primary care clinic, using an adaptation of the CMS definition. First, we defined index discharges as the first discharge for an individual patient in any given 30‐day interval. Only 1 index discharge is flagged for each 30‐day interval. The first admission within 30 days after the index discharge was flagged as the readmission. Consistent with CMS methodology, only the first readmission in the 30‐day period was counted. We included all inpatient and observation status admissions and excluded patients who died during the index encounter, left against medical advice, or transferred to another acute care hospital after the index encounter.
We used secondary diagnosis codes in the administrative data to classify comorbidities by the Elixhauser methodology.[10] All but 1 adult primary care clinic at UCSF are faculty‐only clinics, whereby the assigned PCP is an attending physician. In the general internal medicine clinic, an attending or a resident can serve as the PCP, and approximately 20% of IM clinic patients have a resident as their PCP. For the IM clinic, we classified patients' PCP as attending, resident, or departed. The latter category refers to patients whose PCPs were residents who had graduated or faculty who had departed and had not been assigned to a new PCP prior to the index admission or readmission.
Statistical Analysis
We built a model to predict readmissions using the demographic and clinical variables with [2] P<0.20 in an initial bivariate analysis, and then removed, with backward selection, the least significant variables until only those with P0.05 remained. Age, log‐length of stay (LOS), and intensive care unit stay were forced in the model, as studies evaluating factors related to readmissions have often included these as important covariates.[11, 12, 13, 14] Results were expressed as adjusted odds ratio (OR) with 95% confidence interval (CI). All analyses were carried out using SAS version 9.2 (SAS Institute, Inc., Cary, NC).
This study was exempt from review by the institutional review board of UCSF.
RESULTS
During the study period, there were 12,564 discharges from UCSF Medical Center for primary care patients belonging to the 7 clinics. Of these, 8685 were index discharges and 1032 were readmissions within 30 days. Table 1 shows the characteristics of the patients who had at least 1 admission during the study period. In all but 2 clinics (HIV Primary Care and Concierge Internal Medicine), there were more women hospitalized than men. Age and gender differences across clinics are consistent with the patient populations served by these clinics, with Women's Health having more female patients and younger patients hospitalized compared to Geriatrics.
General Internal Medicine | Family Practice | Women's Health | Geriatrics | Combined IM/FP | HIV Primary Care | Concierge IM | |
---|---|---|---|---|---|---|---|
| |||||||
Panel size | 26,521 | 10,495 | 8,526 | 190 | 4,109 | 1,039 | 706 |
FTE attending MD and NP providers | 18.8 | 7.3 | 4.5 | 1.7 | 5.0 | 2.3 | 2.1 |
Mean panel size per FTE | 1,311 | 1,448 | 1,895 | 112 | 822 | 452 | 336 |
Clinic visits in FY 2012 | 50,362 | 20,647 | 11,014 | 4,425 | 8,120 | 4,182 | 1,713 |
No. of index discharges | 5,388 | 1,204 | 983 | 409 | 339 | 249 | 113 |
No. of readmissions | 718 | 104 | 76 | 56 | 30 | 37 | 11 |
No. of patients discharged during study period | 4,063 | 1,003 | 818 | 289* | 290 | 185 | 84 |
% Male | 40.1% | 33.9% | 4.3% | 35.3% | 33.8% | 69.7% | 52.4% |
Average age (SD), y | 60.4 (18.6) | 52.0 (19.0) | 47.0 (15.7) | 83.2 (6.9) | 46.3 (16.9) | 49.5 (9.9) | 64.5 (15.6) |
Age range, y | 19104 | 1896 | 1997 | 6399 | 1890 | 2273 | 2092 |
Race | |||||||
% White | 42.2% | 41.3% | 58.8% | 61.9% | 49.3% | 61.0% | 91.7% |
% Black | 16.3% | 8.5% | 7.5% | 7.3% | 10.0% | 27.6% | 0.0% |
% Asian | 23.6% | 31.4% | 19.0% | 17.0% | 16.6% | 2.2% | 4.8% |
% Native America/ Alaskan Native | 0.7% | 1.6% | 0.7% | 0.7% | 0.7% | 0.5% | 0.0% |
% Other | 16.3% | 15.7% | 13.1% | 12.5% | 18.3% | 8.1% | 2.4% |
Not available | 1.0% | 1.6% | 1.0% | 0.7% | 5.2% | 0.5% | 1.2% |
Ethnicity | |||||||
% Hispanic | 8.8% | 10.6% | 5.0% | 6.2% | 8.3% | 4.9% | 0.0% |
% Non‐Hispanic | 73.8% | 74.5% | 81.8% | 73.0% | 74.5% | 84.9% | 75.0% |
Not available | 17.4% | 15.0% | 13.2% | 20.8% | 17.2% | 10.3% | 25.0% |
Language | |||||||
% English | 69.7% | 75.6% | 88.8% | 69.6% | 85.9% | 89.7% | 89.3% |
% Spanish | 3.8% | 1.9% | 0.6% | 4.2% | 1.0% | 0.5% | 0.0% |
% Chinese (Mandarin or Cantonese) | 8.0% | 6.7% | 1.2% | 4.8% | 2.1% | 0.0% | 0.0% |
% Russian | 1.7% | 0.6% | 0.1% | 0.4% | 1.0% | 0.0% | 0.0% |
% Vietnamese | 1.0% | 1.4% | 0.0% | 0.4% | 0.0% | 0.0% | 0.0% |
% Other | 11.7% | 9.3% | 5.6% | 18.0% | 6.9% | 8.1% | 4.8% |
Not available | 4.1% | 4.6% | 3.7% | 2.8% | 3.1% | 1.6% | 6.0% |
Insurance type | |||||||
% Private | 35.4% | 58.7% | 77.4% | 10.7% | 63.8% | 36.8% | 66.7% |
% Medicare | 22.0% | 17.2% | 12.0% | 67.8% | 14.8% | 8.7% | 33.3% |
% Medicaid | 15.5% | 12.5% | 3.1% | 1.4% | 12.8% | 24.3% | 0.0% |
% Dual eligible | 24.4% | 8.8% | 5.4% | 19.4% | 6.6% | 27.6% | 0.0% |
% Self‐pay/other | 2.6% | 2.9% | 2.2% | 0.7% | 2.1% | 2.7% | 0.0% |
All‐cause 30‐day readmission rates varied across practices, with HIV Primary Care being the highest at 14.9%, followed by Geriatrics at 13.7%, General Internal Medicine at 13.3%, Concierge Internal Medicine at 9.7%, combined IM/FP at 8.9%, Family Practice at 8.6%, and Women's Health at 7.7% (Figure 1). Despite HIV Primary Care having the highest readmission rate, the number of index discharges during the 3‐year period was relatively low (249) compared to General Internal Medicine (5388). For the index admission, the top 5 admitting services were medicine, obstetrics, cardiology, orthopedic surgery, and general surgery (Table 2). Medicine was the primary admitting service for patients in the following clinics: General Internal Medicine, Geriatrics, HIV Primary Care, and Concierge Internal Medicine. Obstetrics was the primary admitting service for patients in the Family Practice, Women's Health, and combined IM/FP clinics. Vaginal delivery was the top discharge diagnosis‐related group (DRG) for patients in the General Internal Medicine, Family Practice, Women's Health, and combined IM/FP clinics. The top discharge DRG was urinary tract infection for Geriatrics, HIV for the HIV clinic (13.6%), and chemotherapy (8.0%) for Concierge Internal Medicine. Average LOS varied from 4.7 days for patients in the HIV Primary Care clinic to 2.8 days for patients in the Concierge Internal Medicine clinic. Average LOS was 3.4, 3.1, and 3.2 days in the Family Practice, Women's Health, and Geriatrics clinics, respectively, and 3.8 days in the General Internal Medicine and combined IM/FP clinics. For all clinics except Geriatrics, the majority of patients were discharged home without home health. A larger proportion of patients in the Geriatrics clinic were discharged home with home health or discharged to a skilled nursing facility.
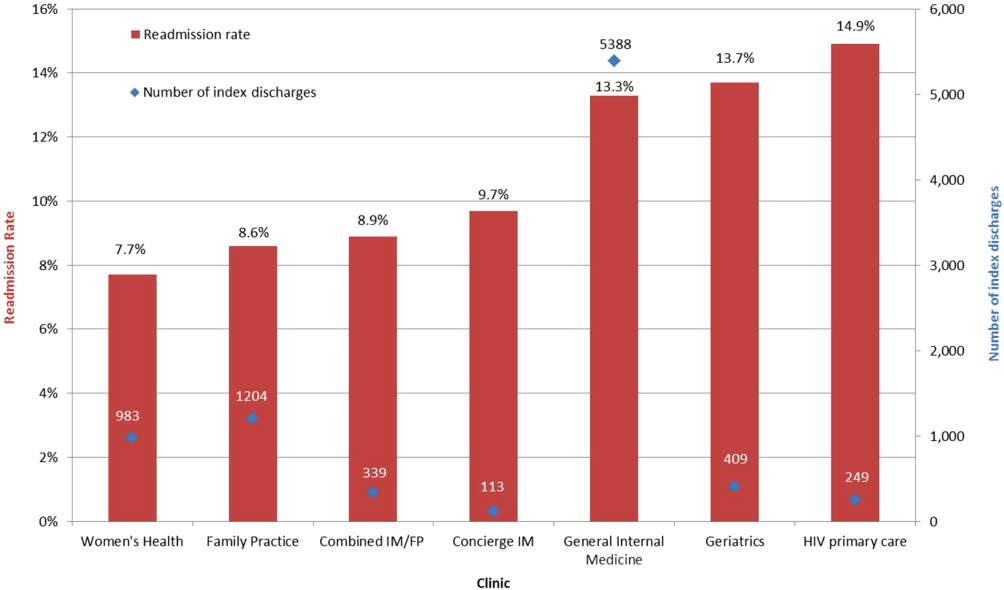
General Internal Medicine, N=5,388 | Family Practice, N=1,204 | Women's Health, N=983 | Geriatrics, N=409 | Combined IM/FP, N=339 | HIV Primary Care, N=249 | Concierge IM, N=113 | |
---|---|---|---|---|---|---|---|
| |||||||
Top 5 admitting services | |||||||
Medicine | 41.8% | 25.0% | 15.2% | 56.4% | 23.5% | 59.7% | 19.0% |
Obstetrics | 7.5% | 24.7% | 38.7% | 0.0% | 35.6% | 2.1% | 9.0% |
Cardiology | 15.1% | 10.9% | 6.2% | 16.5% | 10.3% | 6.5% | 17.0% |
Orthopedic surgery | 8.3% | 8.3% | 8.2% | 7.3% | 5.3% | 4.8% | 12.0% |
Adult general surgery | 7.2% | 9.8% | 10.0% | 6.0% | 5.0% | 5.8% | 7.0% |
Top 5 discharge diagnoses | |||||||
Vaginal delivery | 3.5% | 13.6% | 20.6% | 0.0% | 17.9% | 0.3% | 3.0% |
Major joint replacement | 3.5% | 3.4% | 4.8% | 4.3% | 3.8% | 0.7% | 5.0% |
Vaginal delivery with complications | 1.3% | 5.0% | 6.9% | 0.0% | 4.1% | 1.0% | 2.0% |
Simple pneumonia and pleurisy | 2.8% | 1.2% | 0.6% | 3.8% | 0.3% | 2.1% | 0.0% |
Urinary tract infection | 2.0% | 1.5% | 1.4% | 6.2% | 0.9% | 2.4% | 1.0% |
Discharge disposition | |||||||
% Home | 69.1% | 74.6% | 76.6% | 47.8% | 69.9% | 78.6% | 68.4% |
% Home with home health | 19.4% | 16.5% | 17.8% | 24.1% | 21.7% | 11.8% | 18.8% |
% Skilled nursing facility | 7.2% | 4.9% | 3.0% | 18.9% | 3.3% | 5.0% | 0.8% |
% Other | 4.3% | 4.0% | 2.6% | 9.2% | 5.1% | 4.6% | 12.0% |
Average length of stay (SD) | 3.8 (5.6) | 3.4 (7.5) | 3.1 (3.7) | 3.2 (3.7) | 3.8 (5.2) | 4.7 (6.5) | 2.8 (2.8) |
% Discharges with ICU stay | 11.0% | 10.6% | 5.9% | 8.5% | 9.2% | 11.1% | 15.8% |
Factors associated with variation in readmission rates included: male gender (OR: 1.21, 95% CI: 1.051.40), Medicare (OR: 1.31, 95% CI: 1.051.64; Ref=private) and dual‐eligible Medicare‐Medicaid insurance (OR: 1.26, 95% CI: 1.011.56), unknown primary language (OR: 0.06, 95% CI: 0.020.25; Ref=English), and the following comorbidities: peripheral vascular disease, renal failure, lymphoma, fluid and electrolyte disorders, and anemia. Multivariable logistic regression modeling results are listed in Table 3. Patients having a resident PCP showed no increased odds of readmission (OR: 1.13, 95% CI: 0.931.37; Ref=attending PCP). However, patients with a graduated resident PCP or departed faculty PCP awaiting transfer to a new PCP had an OR of 1.59 (95% CI: 1.162.17) compared with having a current faculty PCP. The C‐statistic for this model was 0.67.
Adjusted Odds Ratio (95% CI) | |
---|---|
| |
Age | |
<65 years | Ref |
>65 years | 0.91 (0.751.10) |
Clinic | |
General Internal Medicine | 1.24 (0.991.56) |
Family Practice | Ref |
Women's Health | 1.13 (0.821.56) |
Geriatrics | 1.20 (0.841.74) |
Combined Internal Medicine/Family Practice | 1.13 (0.731.74) |
HIV Primary Care | 1.24 (0.811.89) |
Concierge Internal Medicine | 1.06 (0.542.07) |
Sex | |
Female | Ref |
Male | 1.21 (1.051.40) |
Insurance | |
Private | Ref |
Medicaid | 1.09 (0.871.37) |
Medicare | 1.31 (1.051.64) |
Dual eligible MedicareMedicaid | 1.26 (1.011.56) |
Self/other | 1.20 (0.741.94) |
Language | |
English | Ref |
Spanish | 1.02 (0.701.47) |
Chinese | 1.13 (0.871.47) |
Other | 0.87 (0.701.08) |
Unknown | 0.06 (0.020.25) |
Comorbidities | |
Pulmonary circulation disease | 1.51 (0.982.32) |
Peripheral vascular disease | 1.50 (1.141.98) |
Renal failure | 1.31 (1.091.58) |
Lymphoma | 2.50 (1.434.36) |
Fluid and electrolyte disorders | 1.27 (1.071.52) |
Deficiency anemia | 1.25 (1.041.51) |
Physician | |
Attending | Ref |
Resident | 1.13 (0.931.37) |
Departed PCP (internal medicine only) | 1.59 (1.162.17) |
ICU stay | 1.03 (0.831.28) |
LOS (log) | 1.02 (0.981.06) |
Admitting service | |
Medicine | Ref |
Obstetrics | 0.41 (0.300.56) |
Cardiology | 1.12 (0.911.37) |
Orthopedic surgery | 0.36 (0.250.51) |
Adult general surgery | 0.64 (0.470.87) |
Other services | 0.76 (0.630.92) |
DISCUSSION
In this study we introduce a complementary way to view hospital readmissions, from the perspective of primary care practices. We found variation in readmission rates across primary care practices. After controlling for admitting service, clinic, provider, and patient factors, the specific characteristics of male gender, patients with Medicare or Medicare with Medicaid, and patients in our General Internal Medicine clinic with a departed PCP were independent risk factors for hospital readmission. Patients with specific comorbidities were also at increased risk for admissions, including those with peripheral vascular disease, renal failure, lymphoma, fluid and electrolyte disorders, and anemia. Because this is the first study viewing readmissions from the perspective of primary care practices, our findings are unique in the literature. However, hospital‐based studies have found similar relationships between readmission rates and these specific comorbidities.[11, 12, 15] Unlike other studies, our study cohort did not show CHF as an independent risk factor. We hypothesize this is because in 2008, UCSF Medical Center introduced an inpatient‐based intervention to reduce readmissions in patients with heart failure. By 2011, the readmission rates of patients with heart failure (primary or secondary diagnoses) had dropped by 30%. The success of this program, focused only on patients with heart failure, likely affected our analysis of comorbidities as risk factors for readmissions.
Models developed to predict hospital readmissions, overall and for specific disease conditions, have inconsistently identified predictive factors, and there is not a specific set of variables that dominate.[16, 17, 18, 19] A recent review of readmission risk prediction models suggested that models that take into account psychosocial factors such as social support, substance abuse, and functional status improved model performance.[16] We hypothesize that the reason why male gender was significant in our model may be related to lack of social support, especially among those who may be single or widowed. Other studies have also showed male gender as a predictor for hospital readmissions.[14, 20]
People with Medicare as the primary payor or Medicare with Medicaid also tended to have higher risk of readmission. We believe that this may be a proxy for the combined effect of age, multiple comorbidities, and psychosocial factors. In a multicenter study of general medicine inpatients, Medicare, but not age, was also found to be a predictive variable.[21] Unlike other studies, our study did not find Medicaid alone as a significant predictive variable for readmissions.[15, 21] One explanation may be that in hospital‐based studies, people with Medicaid who are at high risk for readmission may be high‐risk because they do not have a PCP or good access to outpatient care. In our study, all patients have a PCP in 1 of the UCSF clinics, and access to care is improved with this established relationship. These other studies did not examine Medicare‐Medicaid dual eligibility status. Our results are consistent with a national study on avoidable hospital admissions that showed the dual eligibility population experiencing 60% higher avoidable admission rates compared to the Medicaid‐only population.[22]
An interesting finding was that there were no statistically significant differences in readmissions among patients who report a language other than English as their primary language. Although language barriers and health literacy can affect a patient's ability to understand discharge instructions, the use of translators at UCSF Medical Center may have decreased the risk of readmissions. For a small number of patients whose primary language was not recorded (unknown language in our model), they appeared to have a lower risk of readmissions. Language preferences are recorded by our admitting staff during the process of admission. This step may have been skipped after hours or if the patient was not able to answer the question. However, we do not have a good hypothesis as to why these patients may have a reduced risk of readmissions.
Having a departed PCP in the General Internal Medicine clinic was an independent predictor of readmissions. These patients have access to primary care; they can schedule acute appointments with covering providers for new medical issues or follow‐up of chronic conditions. However, until they are transferred to a new PCP, they do not have a provider who is proactively managing their preventive and chronic disease care, including follow‐up and coordinating care after hospital discharge. Our study is not the first to suggest adverse outcomes for patients who are in transition from 1 primary care provider to the next.[23] Studies conducted in General Internal Medicine clinics have shown missed opportunities for cancer screening and overlooked test results during the transition period,[24] and as many as one‐fifth of patients whom residents identified as high‐risk were lost to follow‐up.[25] However, our study is the first to show the link between PCP transition in a teaching clinic and hospital readmissions. This finding underscores the importance of continuity of care in the optimal management of patients following hospital discharge.
There are several limitations to this study. First, our study only considers hospitalizations to UCSF Medical Center, potentially undercounting readmissions to area hospitals. Because our study population are patients with PCPs at UCSF, these patients tend to seek specialty and acute care at UCSF Medical Center as well. We obtained payor data from our medical group (Hill Physicians), which covers our largest private payors, to understand whether our results can be applied on a global basis. We found that in 87.4% of index admissions with readmissions from January 1, 2010 through May 30, 2012, the readmission occurred at UCSF Medical Center. We conducted a similar analysis with CMS data from October 1, 2008 to June 30, 2011, the latest data we have from CMS. For patients with UCSF PCPs and a diagnosis of AMI or CHF, 100% were readmitted back to UCSF Medical Center. For patients with UCSF PCPs and a diagnosis of pneumonia, 89% were readmitted back to UCSF Medical Center. Given that only a small percentage of patients with PCPs at UCSF present for readmission at other area hospitals, we believe that limiting our analysis to UCSF Medical Center is reasonable.
In our study, we did not remove vaginal deliveries or Caesarian sections prior to building the model. Primary care physicians and their clinic leadership are accustomed to taking a population‐health perspective. We anticipate they would be interested in designing interventions and addressing readmission for the entire primary care panel. Although readmissions after delivery are not frequent, they can still occur, and interventions should not necessarily exclude this population. We did run a sensitivity analysis by removing vaginal delivery and Caesarian sections from the analysis. As expected, readmission rates for all practices increased except for Geriatrics. However, the independent predictors of readmissions did not change.
Our study is based on a population of patients at 1 urban academic medical center and may not be generalizable across all delivery systems. Our population is racially and ethnically diverse, and many do not speak English as their primary language. The study also spans different types of primary care clinics, capturing a wide range of ages and case mix. As PCP assignments fluctuate over time, there may be errors with PCP attribution in the UCSF Medical Center data systems. We do not believe errors in PCP attribution would differ across primary care practices. Because the primary care practices' performance reports on quality measures are based on PCP assignment, each clinic regularly updates their clinic panels and has specific protocols to address errors with PCP attribution.
Finally, our study includes only the variables that we were able to extract from administrative claims. Other explanatory variables that have been suggested as important for evaluation, such as social support, functional assessment, access to care, hospital discharge process, and posthospitalization follow‐up, were not included. Each of these could be explored in future studies.
This study offers a unique perspective of hospital readmission by introducing a new methodology for primary care clinics to calculate and evaluate their all‐cause 30‐day readmission rates. Although this methodology is not intended to provide real‐time feedback to clinics on readmitted patients, it opens the door for benchmarking based on specific case‐mix indices. Another direction for future research is to design robust evaluations of the impact of interventions, both inpatient and outpatient, on primary care clinic readmission rates. Finally, future research should replicate this analysis across teaching clinics to identify whether provider turnover is a consistent independent predictor of hospital readmissions.
This study also has implications for inpatient interventions. For example, discharging physicians may consider proactively identifying whether the patient has a continuous primary care provider. Patients who are in between PCPs may need closer follow‐up after discharge, until they re‐establish with a new PCP. This can be accomplished through a postdischarge clinic visit, either run by inpatient providers or covering physicians in the primary care clinic. In addition, the discharging physician may work with the case manager to increase the level of care coordination. The case manager could contact the primary care clinic and proactively ask for immediate PCP re‐assignment. Once a new PCP has been identified, the discharging physician could consider a warm hand‐off. With a warm hand‐off, the new PCP may feel more comfortable managing problems that may arise after hospital discharge, and especially before the first outpatient visit with the new PCP. Future research can test whether these interventions could effectively reduce hospital readmissions across a broad primary care population.
CONCLUSION
Primary care providers and their clinics play an important role in managing population health, decreasing healthcare spending, and keeping patients out of the hospital. In this study, we introduce a tool in which primary care clinics can begin to understand their hospital readmission rates. This may be particularly valuable as primary care providers enter global payment arrangements such as accountable care organizations or bundled payments and are responsible for a population of patients across the continuum of care. We found significant variation in readmission rates between different primary care practices, but much of this variation appears to be due to differences between practices in patient demographics, comorbidities, and hospitalization factors. Our study is the first to show the association between provider transitions and higher hospital readmissions. Continuity of care is critical for the optimal management of patients following hospital discharge. More attention will need to be focused on providing good continuity outpatient care for patients at high risk for readmissions.
Acknowledgements
The authors acknowledge Janelle Lee, MBA, MHA, DrPH, for her assistance with extracting the hospital claims data at UCSF Medical Center.
Disclosure: Dr. Tang and Ms. Maselli were supported by a University of California, Center for Health Quality and Innovation grant. The authors report no conflicts of interest.
- Medicare's new hospital value‐based purchasing program is likely to have only a small impact on hospital payments. Health Aff. 2012;31:1932–1940. , .
- Contemporary evidence about hospital strategies for reducing 30‐day readmissions. J Am Coll Cardiol. 2012;60:607–614. , , , et al.
- Interventions to reduce 30‐day rehospitalization: a systematic review. Ann Intern Med. 2011;155:520–528. , , , , .
- Reducing hospital readmissions: lessons from top‐performing hospitals. The Commonwealth Fund Synthesis Report. Available at: http://www.commonwealthfund.org/publications/case‐studies/2011/apr/reducing‐hospital‐readmissions. Published April 6, 2011. Accessed February 8, 2013. , , .
- Effective Interventions to Reduce Rehospitalizations: A Survey of the Published Evidence. Cambridge, MA: Institute for Healthcare Improvement; 2009. , .
- Recasting readmissions by placing the hospital role in community context. JAMA. 2013;309:351–352. , , .
- Hospitalists and care transitions: the divorce of inpatient and outpatient care. Health Affairs. 2008;27:1315–1327. , , , .
- Center for Medicare and Medicaid Innovation. Community‐based Care Transitions Program. Available at: http://innovation.cms.gov/initiatives/CCTP/#collapse‐tableDetails. Accessed February 8, 2013.
- Medicare's transitional care payment—a step toward the medical home. N Engl J Med. 2013;368:692–694. , , .
- Volume thresholds and hospital characteristics in the United States. Health Aff (Millwood). 2003;22:167–177. , , .
- Thirty‐day rehospitalizations after acute myocardial infarction: a cohort study. Ann Intern Med. 2012;157:11–18. , , , , , .
- Rehospitalizations among patients in the Medicare fee‐for‐service program. N Engl J Med. 2009;360:1418–1428. , , .
- Associations between reduced hospital length of stay and 30‐day readmission rate and mortality: 14‐year experience in 129 Veterans Affairs hospitals. Ann Intern Med. 2012;157:837–845. , , , et al.
- Readmission after hospitalization for congestive heart failure among Medicare beneficiaries. Arch Intern Med. 1997;157:99–104. , , , et al.
- Redefining readmission risk factors for general medicine patients. J Hosp Med. 2011;6:54–60. , , , .
- Risk prediction models for hospital readmission: a systematic review. JAMA. 2011;206:1688–1698. , , , et al.
- Statistical models and patient predictors of readmission for heart failure: a systematic review. Arch Intern Med. 2008;168:1371–1386. , , , et al.
- Statistical models and patient predictors of readmission for acute myocardial infarction: a systematic review. Circ Cardiovasc Qual Outcomes. 2009;2:500–507. , , , .
- Predictors of hospital readmission after stroke: a systematic review. Stroke. 2010;41:2525–2533. , , , et al.
- Risk factors for 30‐day hospital readmission in patients ≥65 years of age. Proc (Bayl Univ Med Cent). 2008;21:363–372. , , , , .
- Hospital readmission in general medicine patients: a prediction model. J Gen Intern Med. 2010;25:211–219. , , , et al.
- Users of Medicaid home and community‐based services are especially vulnerable to costly avoidable hospital admissions. Health Affairs. 2012;31:1167–1175. , , .
- Academic year‐end transfers of outpatients from outgoing to incoming residents: an unaddressed patient safety issue. JAMA. 2009;302:1327–1329. , .
- The revolving door of resident continuity practice: identifying gaps in transitions of care. J Gen Intern Med. 2011;26:995–998. , , , , , .
- Outcomes for resident‐identified high‐risk patients and resident perspectives of year‐end continuity clinic handoffs. J Gen Intern Med. 2012;27:1438–1444. , , , et al.
Reducing hospital readmissions is a national healthcare priority. In October 2012, the Centers for Medicare and Medicaid Services (CMS) enacted financial penalties on hospitals with higher than average risk‐adjusted readmissions, offering an incentive pool of $850 million in the first year.[1] As a result, a wide range of activities to understand and reduce readmissions among patients with congestive heart failure (CHF), acute myocardial infarction (AMI), and pneumonia have emerged.[2, 3, 4, 5] Some have proposed that to effectively reduce hospital readmissions, a community of inpatient and outpatient providers and local support organizations must coordinate efforts.[6, 7] In fact, CMS has recognized the important role of community support groups and outpatient providers in safe discharge transitions in 2 ways. First, in 2011 CMS launched the Community‐based Care Transitions Program to fund community‐based organizations to assist Medicare patients with care transitions.[8] Second, CMS introduced 2 new reimbursement codes for primary care providers (PCPs) to perform care coordination immediately after hospital discharge.[9] Both of these new payment programs represent an evolving perspective that reducing hospital readmissions requires active participation among outpatient partners.
As leaders of the outpatient care team, PCPs play a significant role in reducing hospital readmissions. One way in which PCPs can begin to understand the magnitude of the issue within their clinic is to evaluate the clinic's 30‐day readmission rates. Currently, CMS calculates readmission rates at the hospital level. However, understanding these rates at the clinic level is critical for developing strategies for improvement across the care continuum. Our current understanding of effective outpatient interventions to reduce hospital readmission is limited.[3] As clinics introduce and refine strategies to reduce readmissions, tracking the impact of these strategies on readmission rates will be critical for identifying effective outpatient interventions. Clinics with similar patient case‐mix can also benchmark readmission rates, sharing best practices from clinics with lower‐than‐expected rates.
There are no available studies or proposed methodologies to guide primary care clinics in calculating their 30‐day readmission rates. A particularly difficult challenge is obtaining the admission information when patients may be admitted to 1 of several area hospitals. For large integrated delivery networks where primary care patients are relatively loyal to the network of physicians and hospitals, an opportunity exists to explore the data. Furthermore, variations in readmission rates across primary care specialties (such as internal medicine and family practice) are not well understood. In this study, we set out to develop a methodology for calculating all‐cause 30‐day hospital readmission rates at the level of individual primary care practices and to identify factors associated with variations in these rates.
METHODS
Study Design
We conducted a retrospective observational study of adult primary care patients at the University of California, San Francisco (UCSF) who were hospitalized at UCSF Medical Center between July 1, 2009 and June 30, 2012. UCSF Medical Center is comprised of Moffitt‐Long Hospital (a 600‐bed facility) and UCSF‐Mount Zion Hospital (a 90‐bed facility) located in San Francisco, CA. The patient population was limited to adults ages 18 and over with a PCP at UCSF. UCSF has 7 adult primary care clinics: General Internal Medicine (IM), Family Practice (FP), Women's Health, Geriatrics, a combined IM/FP clinic, Human Immunodeficiency Virus (HIV) Primary Care, and a Concierge Internal Medicine clinic staffed by IM physicians. Between 2009 and 2011, all clinics completed the process of enpanelment, or defining the population of patients for which each PCP and the clinic is responsible. We obtained the list of patient and PCP assignments at each of the 7 clinics across the time period of study. We then obtained UCSF Medical Center hospital claims data for this group, including dates of admission and discharge, patient age, sex, race/ethnicity, language, insurance, admitting service, diagnosis codes, information on intensive care unit stay, and discharge disposition. Hospital claims data are housed in Transition Systems International (Boston, MA) administrative databases, a cost‐accounting system that collects data abstracted from patient charts upon discharge from UCSF Medical Center.
All‐cause 30‐day hospital readmission rates were calculated for each primary care clinic, using an adaptation of the CMS definition. First, we defined index discharges as the first discharge for an individual patient in any given 30‐day interval. Only 1 index discharge is flagged for each 30‐day interval. The first admission within 30 days after the index discharge was flagged as the readmission. Consistent with CMS methodology, only the first readmission in the 30‐day period was counted. We included all inpatient and observation status admissions and excluded patients who died during the index encounter, left against medical advice, or transferred to another acute care hospital after the index encounter.
We used secondary diagnosis codes in the administrative data to classify comorbidities by the Elixhauser methodology.[10] All but 1 adult primary care clinic at UCSF are faculty‐only clinics, whereby the assigned PCP is an attending physician. In the general internal medicine clinic, an attending or a resident can serve as the PCP, and approximately 20% of IM clinic patients have a resident as their PCP. For the IM clinic, we classified patients' PCP as attending, resident, or departed. The latter category refers to patients whose PCPs were residents who had graduated or faculty who had departed and had not been assigned to a new PCP prior to the index admission or readmission.
Statistical Analysis
We built a model to predict readmissions using the demographic and clinical variables with [2] P<0.20 in an initial bivariate analysis, and then removed, with backward selection, the least significant variables until only those with P0.05 remained. Age, log‐length of stay (LOS), and intensive care unit stay were forced in the model, as studies evaluating factors related to readmissions have often included these as important covariates.[11, 12, 13, 14] Results were expressed as adjusted odds ratio (OR) with 95% confidence interval (CI). All analyses were carried out using SAS version 9.2 (SAS Institute, Inc., Cary, NC).
This study was exempt from review by the institutional review board of UCSF.
RESULTS
During the study period, there were 12,564 discharges from UCSF Medical Center for primary care patients belonging to the 7 clinics. Of these, 8685 were index discharges and 1032 were readmissions within 30 days. Table 1 shows the characteristics of the patients who had at least 1 admission during the study period. In all but 2 clinics (HIV Primary Care and Concierge Internal Medicine), there were more women hospitalized than men. Age and gender differences across clinics are consistent with the patient populations served by these clinics, with Women's Health having more female patients and younger patients hospitalized compared to Geriatrics.
General Internal Medicine | Family Practice | Women's Health | Geriatrics | Combined IM/FP | HIV Primary Care | Concierge IM | |
---|---|---|---|---|---|---|---|
| |||||||
Panel size | 26,521 | 10,495 | 8,526 | 190 | 4,109 | 1,039 | 706 |
FTE attending MD and NP providers | 18.8 | 7.3 | 4.5 | 1.7 | 5.0 | 2.3 | 2.1 |
Mean panel size per FTE | 1,311 | 1,448 | 1,895 | 112 | 822 | 452 | 336 |
Clinic visits in FY 2012 | 50,362 | 20,647 | 11,014 | 4,425 | 8,120 | 4,182 | 1,713 |
No. of index discharges | 5,388 | 1,204 | 983 | 409 | 339 | 249 | 113 |
No. of readmissions | 718 | 104 | 76 | 56 | 30 | 37 | 11 |
No. of patients discharged during study period | 4,063 | 1,003 | 818 | 289* | 290 | 185 | 84 |
% Male | 40.1% | 33.9% | 4.3% | 35.3% | 33.8% | 69.7% | 52.4% |
Average age (SD), y | 60.4 (18.6) | 52.0 (19.0) | 47.0 (15.7) | 83.2 (6.9) | 46.3 (16.9) | 49.5 (9.9) | 64.5 (15.6) |
Age range, y | 19104 | 1896 | 1997 | 6399 | 1890 | 2273 | 2092 |
Race | |||||||
% White | 42.2% | 41.3% | 58.8% | 61.9% | 49.3% | 61.0% | 91.7% |
% Black | 16.3% | 8.5% | 7.5% | 7.3% | 10.0% | 27.6% | 0.0% |
% Asian | 23.6% | 31.4% | 19.0% | 17.0% | 16.6% | 2.2% | 4.8% |
% Native America/ Alaskan Native | 0.7% | 1.6% | 0.7% | 0.7% | 0.7% | 0.5% | 0.0% |
% Other | 16.3% | 15.7% | 13.1% | 12.5% | 18.3% | 8.1% | 2.4% |
Not available | 1.0% | 1.6% | 1.0% | 0.7% | 5.2% | 0.5% | 1.2% |
Ethnicity | |||||||
% Hispanic | 8.8% | 10.6% | 5.0% | 6.2% | 8.3% | 4.9% | 0.0% |
% Non‐Hispanic | 73.8% | 74.5% | 81.8% | 73.0% | 74.5% | 84.9% | 75.0% |
Not available | 17.4% | 15.0% | 13.2% | 20.8% | 17.2% | 10.3% | 25.0% |
Language | |||||||
% English | 69.7% | 75.6% | 88.8% | 69.6% | 85.9% | 89.7% | 89.3% |
% Spanish | 3.8% | 1.9% | 0.6% | 4.2% | 1.0% | 0.5% | 0.0% |
% Chinese (Mandarin or Cantonese) | 8.0% | 6.7% | 1.2% | 4.8% | 2.1% | 0.0% | 0.0% |
% Russian | 1.7% | 0.6% | 0.1% | 0.4% | 1.0% | 0.0% | 0.0% |
% Vietnamese | 1.0% | 1.4% | 0.0% | 0.4% | 0.0% | 0.0% | 0.0% |
% Other | 11.7% | 9.3% | 5.6% | 18.0% | 6.9% | 8.1% | 4.8% |
Not available | 4.1% | 4.6% | 3.7% | 2.8% | 3.1% | 1.6% | 6.0% |
Insurance type | |||||||
% Private | 35.4% | 58.7% | 77.4% | 10.7% | 63.8% | 36.8% | 66.7% |
% Medicare | 22.0% | 17.2% | 12.0% | 67.8% | 14.8% | 8.7% | 33.3% |
% Medicaid | 15.5% | 12.5% | 3.1% | 1.4% | 12.8% | 24.3% | 0.0% |
% Dual eligible | 24.4% | 8.8% | 5.4% | 19.4% | 6.6% | 27.6% | 0.0% |
% Self‐pay/other | 2.6% | 2.9% | 2.2% | 0.7% | 2.1% | 2.7% | 0.0% |
All‐cause 30‐day readmission rates varied across practices, with HIV Primary Care being the highest at 14.9%, followed by Geriatrics at 13.7%, General Internal Medicine at 13.3%, Concierge Internal Medicine at 9.7%, combined IM/FP at 8.9%, Family Practice at 8.6%, and Women's Health at 7.7% (Figure 1). Despite HIV Primary Care having the highest readmission rate, the number of index discharges during the 3‐year period was relatively low (249) compared to General Internal Medicine (5388). For the index admission, the top 5 admitting services were medicine, obstetrics, cardiology, orthopedic surgery, and general surgery (Table 2). Medicine was the primary admitting service for patients in the following clinics: General Internal Medicine, Geriatrics, HIV Primary Care, and Concierge Internal Medicine. Obstetrics was the primary admitting service for patients in the Family Practice, Women's Health, and combined IM/FP clinics. Vaginal delivery was the top discharge diagnosis‐related group (DRG) for patients in the General Internal Medicine, Family Practice, Women's Health, and combined IM/FP clinics. The top discharge DRG was urinary tract infection for Geriatrics, HIV for the HIV clinic (13.6%), and chemotherapy (8.0%) for Concierge Internal Medicine. Average LOS varied from 4.7 days for patients in the HIV Primary Care clinic to 2.8 days for patients in the Concierge Internal Medicine clinic. Average LOS was 3.4, 3.1, and 3.2 days in the Family Practice, Women's Health, and Geriatrics clinics, respectively, and 3.8 days in the General Internal Medicine and combined IM/FP clinics. For all clinics except Geriatrics, the majority of patients were discharged home without home health. A larger proportion of patients in the Geriatrics clinic were discharged home with home health or discharged to a skilled nursing facility.
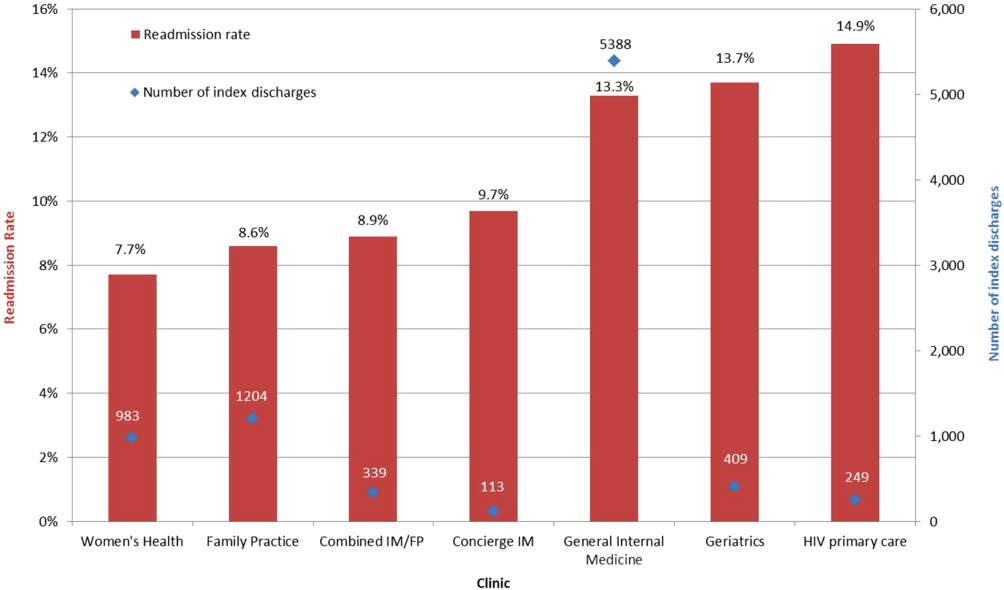
General Internal Medicine, N=5,388 | Family Practice, N=1,204 | Women's Health, N=983 | Geriatrics, N=409 | Combined IM/FP, N=339 | HIV Primary Care, N=249 | Concierge IM, N=113 | |
---|---|---|---|---|---|---|---|
| |||||||
Top 5 admitting services | |||||||
Medicine | 41.8% | 25.0% | 15.2% | 56.4% | 23.5% | 59.7% | 19.0% |
Obstetrics | 7.5% | 24.7% | 38.7% | 0.0% | 35.6% | 2.1% | 9.0% |
Cardiology | 15.1% | 10.9% | 6.2% | 16.5% | 10.3% | 6.5% | 17.0% |
Orthopedic surgery | 8.3% | 8.3% | 8.2% | 7.3% | 5.3% | 4.8% | 12.0% |
Adult general surgery | 7.2% | 9.8% | 10.0% | 6.0% | 5.0% | 5.8% | 7.0% |
Top 5 discharge diagnoses | |||||||
Vaginal delivery | 3.5% | 13.6% | 20.6% | 0.0% | 17.9% | 0.3% | 3.0% |
Major joint replacement | 3.5% | 3.4% | 4.8% | 4.3% | 3.8% | 0.7% | 5.0% |
Vaginal delivery with complications | 1.3% | 5.0% | 6.9% | 0.0% | 4.1% | 1.0% | 2.0% |
Simple pneumonia and pleurisy | 2.8% | 1.2% | 0.6% | 3.8% | 0.3% | 2.1% | 0.0% |
Urinary tract infection | 2.0% | 1.5% | 1.4% | 6.2% | 0.9% | 2.4% | 1.0% |
Discharge disposition | |||||||
% Home | 69.1% | 74.6% | 76.6% | 47.8% | 69.9% | 78.6% | 68.4% |
% Home with home health | 19.4% | 16.5% | 17.8% | 24.1% | 21.7% | 11.8% | 18.8% |
% Skilled nursing facility | 7.2% | 4.9% | 3.0% | 18.9% | 3.3% | 5.0% | 0.8% |
% Other | 4.3% | 4.0% | 2.6% | 9.2% | 5.1% | 4.6% | 12.0% |
Average length of stay (SD) | 3.8 (5.6) | 3.4 (7.5) | 3.1 (3.7) | 3.2 (3.7) | 3.8 (5.2) | 4.7 (6.5) | 2.8 (2.8) |
% Discharges with ICU stay | 11.0% | 10.6% | 5.9% | 8.5% | 9.2% | 11.1% | 15.8% |
Factors associated with variation in readmission rates included: male gender (OR: 1.21, 95% CI: 1.051.40), Medicare (OR: 1.31, 95% CI: 1.051.64; Ref=private) and dual‐eligible Medicare‐Medicaid insurance (OR: 1.26, 95% CI: 1.011.56), unknown primary language (OR: 0.06, 95% CI: 0.020.25; Ref=English), and the following comorbidities: peripheral vascular disease, renal failure, lymphoma, fluid and electrolyte disorders, and anemia. Multivariable logistic regression modeling results are listed in Table 3. Patients having a resident PCP showed no increased odds of readmission (OR: 1.13, 95% CI: 0.931.37; Ref=attending PCP). However, patients with a graduated resident PCP or departed faculty PCP awaiting transfer to a new PCP had an OR of 1.59 (95% CI: 1.162.17) compared with having a current faculty PCP. The C‐statistic for this model was 0.67.
Adjusted Odds Ratio (95% CI) | |
---|---|
| |
Age | |
<65 years | Ref |
>65 years | 0.91 (0.751.10) |
Clinic | |
General Internal Medicine | 1.24 (0.991.56) |
Family Practice | Ref |
Women's Health | 1.13 (0.821.56) |
Geriatrics | 1.20 (0.841.74) |
Combined Internal Medicine/Family Practice | 1.13 (0.731.74) |
HIV Primary Care | 1.24 (0.811.89) |
Concierge Internal Medicine | 1.06 (0.542.07) |
Sex | |
Female | Ref |
Male | 1.21 (1.051.40) |
Insurance | |
Private | Ref |
Medicaid | 1.09 (0.871.37) |
Medicare | 1.31 (1.051.64) |
Dual eligible MedicareMedicaid | 1.26 (1.011.56) |
Self/other | 1.20 (0.741.94) |
Language | |
English | Ref |
Spanish | 1.02 (0.701.47) |
Chinese | 1.13 (0.871.47) |
Other | 0.87 (0.701.08) |
Unknown | 0.06 (0.020.25) |
Comorbidities | |
Pulmonary circulation disease | 1.51 (0.982.32) |
Peripheral vascular disease | 1.50 (1.141.98) |
Renal failure | 1.31 (1.091.58) |
Lymphoma | 2.50 (1.434.36) |
Fluid and electrolyte disorders | 1.27 (1.071.52) |
Deficiency anemia | 1.25 (1.041.51) |
Physician | |
Attending | Ref |
Resident | 1.13 (0.931.37) |
Departed PCP (internal medicine only) | 1.59 (1.162.17) |
ICU stay | 1.03 (0.831.28) |
LOS (log) | 1.02 (0.981.06) |
Admitting service | |
Medicine | Ref |
Obstetrics | 0.41 (0.300.56) |
Cardiology | 1.12 (0.911.37) |
Orthopedic surgery | 0.36 (0.250.51) |
Adult general surgery | 0.64 (0.470.87) |
Other services | 0.76 (0.630.92) |
DISCUSSION
In this study we introduce a complementary way to view hospital readmissions, from the perspective of primary care practices. We found variation in readmission rates across primary care practices. After controlling for admitting service, clinic, provider, and patient factors, the specific characteristics of male gender, patients with Medicare or Medicare with Medicaid, and patients in our General Internal Medicine clinic with a departed PCP were independent risk factors for hospital readmission. Patients with specific comorbidities were also at increased risk for admissions, including those with peripheral vascular disease, renal failure, lymphoma, fluid and electrolyte disorders, and anemia. Because this is the first study viewing readmissions from the perspective of primary care practices, our findings are unique in the literature. However, hospital‐based studies have found similar relationships between readmission rates and these specific comorbidities.[11, 12, 15] Unlike other studies, our study cohort did not show CHF as an independent risk factor. We hypothesize this is because in 2008, UCSF Medical Center introduced an inpatient‐based intervention to reduce readmissions in patients with heart failure. By 2011, the readmission rates of patients with heart failure (primary or secondary diagnoses) had dropped by 30%. The success of this program, focused only on patients with heart failure, likely affected our analysis of comorbidities as risk factors for readmissions.
Models developed to predict hospital readmissions, overall and for specific disease conditions, have inconsistently identified predictive factors, and there is not a specific set of variables that dominate.[16, 17, 18, 19] A recent review of readmission risk prediction models suggested that models that take into account psychosocial factors such as social support, substance abuse, and functional status improved model performance.[16] We hypothesize that the reason why male gender was significant in our model may be related to lack of social support, especially among those who may be single or widowed. Other studies have also showed male gender as a predictor for hospital readmissions.[14, 20]
People with Medicare as the primary payor or Medicare with Medicaid also tended to have higher risk of readmission. We believe that this may be a proxy for the combined effect of age, multiple comorbidities, and psychosocial factors. In a multicenter study of general medicine inpatients, Medicare, but not age, was also found to be a predictive variable.[21] Unlike other studies, our study did not find Medicaid alone as a significant predictive variable for readmissions.[15, 21] One explanation may be that in hospital‐based studies, people with Medicaid who are at high risk for readmission may be high‐risk because they do not have a PCP or good access to outpatient care. In our study, all patients have a PCP in 1 of the UCSF clinics, and access to care is improved with this established relationship. These other studies did not examine Medicare‐Medicaid dual eligibility status. Our results are consistent with a national study on avoidable hospital admissions that showed the dual eligibility population experiencing 60% higher avoidable admission rates compared to the Medicaid‐only population.[22]
An interesting finding was that there were no statistically significant differences in readmissions among patients who report a language other than English as their primary language. Although language barriers and health literacy can affect a patient's ability to understand discharge instructions, the use of translators at UCSF Medical Center may have decreased the risk of readmissions. For a small number of patients whose primary language was not recorded (unknown language in our model), they appeared to have a lower risk of readmissions. Language preferences are recorded by our admitting staff during the process of admission. This step may have been skipped after hours or if the patient was not able to answer the question. However, we do not have a good hypothesis as to why these patients may have a reduced risk of readmissions.
Having a departed PCP in the General Internal Medicine clinic was an independent predictor of readmissions. These patients have access to primary care; they can schedule acute appointments with covering providers for new medical issues or follow‐up of chronic conditions. However, until they are transferred to a new PCP, they do not have a provider who is proactively managing their preventive and chronic disease care, including follow‐up and coordinating care after hospital discharge. Our study is not the first to suggest adverse outcomes for patients who are in transition from 1 primary care provider to the next.[23] Studies conducted in General Internal Medicine clinics have shown missed opportunities for cancer screening and overlooked test results during the transition period,[24] and as many as one‐fifth of patients whom residents identified as high‐risk were lost to follow‐up.[25] However, our study is the first to show the link between PCP transition in a teaching clinic and hospital readmissions. This finding underscores the importance of continuity of care in the optimal management of patients following hospital discharge.
There are several limitations to this study. First, our study only considers hospitalizations to UCSF Medical Center, potentially undercounting readmissions to area hospitals. Because our study population are patients with PCPs at UCSF, these patients tend to seek specialty and acute care at UCSF Medical Center as well. We obtained payor data from our medical group (Hill Physicians), which covers our largest private payors, to understand whether our results can be applied on a global basis. We found that in 87.4% of index admissions with readmissions from January 1, 2010 through May 30, 2012, the readmission occurred at UCSF Medical Center. We conducted a similar analysis with CMS data from October 1, 2008 to June 30, 2011, the latest data we have from CMS. For patients with UCSF PCPs and a diagnosis of AMI or CHF, 100% were readmitted back to UCSF Medical Center. For patients with UCSF PCPs and a diagnosis of pneumonia, 89% were readmitted back to UCSF Medical Center. Given that only a small percentage of patients with PCPs at UCSF present for readmission at other area hospitals, we believe that limiting our analysis to UCSF Medical Center is reasonable.
In our study, we did not remove vaginal deliveries or Caesarian sections prior to building the model. Primary care physicians and their clinic leadership are accustomed to taking a population‐health perspective. We anticipate they would be interested in designing interventions and addressing readmission for the entire primary care panel. Although readmissions after delivery are not frequent, they can still occur, and interventions should not necessarily exclude this population. We did run a sensitivity analysis by removing vaginal delivery and Caesarian sections from the analysis. As expected, readmission rates for all practices increased except for Geriatrics. However, the independent predictors of readmissions did not change.
Our study is based on a population of patients at 1 urban academic medical center and may not be generalizable across all delivery systems. Our population is racially and ethnically diverse, and many do not speak English as their primary language. The study also spans different types of primary care clinics, capturing a wide range of ages and case mix. As PCP assignments fluctuate over time, there may be errors with PCP attribution in the UCSF Medical Center data systems. We do not believe errors in PCP attribution would differ across primary care practices. Because the primary care practices' performance reports on quality measures are based on PCP assignment, each clinic regularly updates their clinic panels and has specific protocols to address errors with PCP attribution.
Finally, our study includes only the variables that we were able to extract from administrative claims. Other explanatory variables that have been suggested as important for evaluation, such as social support, functional assessment, access to care, hospital discharge process, and posthospitalization follow‐up, were not included. Each of these could be explored in future studies.
This study offers a unique perspective of hospital readmission by introducing a new methodology for primary care clinics to calculate and evaluate their all‐cause 30‐day readmission rates. Although this methodology is not intended to provide real‐time feedback to clinics on readmitted patients, it opens the door for benchmarking based on specific case‐mix indices. Another direction for future research is to design robust evaluations of the impact of interventions, both inpatient and outpatient, on primary care clinic readmission rates. Finally, future research should replicate this analysis across teaching clinics to identify whether provider turnover is a consistent independent predictor of hospital readmissions.
This study also has implications for inpatient interventions. For example, discharging physicians may consider proactively identifying whether the patient has a continuous primary care provider. Patients who are in between PCPs may need closer follow‐up after discharge, until they re‐establish with a new PCP. This can be accomplished through a postdischarge clinic visit, either run by inpatient providers or covering physicians in the primary care clinic. In addition, the discharging physician may work with the case manager to increase the level of care coordination. The case manager could contact the primary care clinic and proactively ask for immediate PCP re‐assignment. Once a new PCP has been identified, the discharging physician could consider a warm hand‐off. With a warm hand‐off, the new PCP may feel more comfortable managing problems that may arise after hospital discharge, and especially before the first outpatient visit with the new PCP. Future research can test whether these interventions could effectively reduce hospital readmissions across a broad primary care population.
CONCLUSION
Primary care providers and their clinics play an important role in managing population health, decreasing healthcare spending, and keeping patients out of the hospital. In this study, we introduce a tool in which primary care clinics can begin to understand their hospital readmission rates. This may be particularly valuable as primary care providers enter global payment arrangements such as accountable care organizations or bundled payments and are responsible for a population of patients across the continuum of care. We found significant variation in readmission rates between different primary care practices, but much of this variation appears to be due to differences between practices in patient demographics, comorbidities, and hospitalization factors. Our study is the first to show the association between provider transitions and higher hospital readmissions. Continuity of care is critical for the optimal management of patients following hospital discharge. More attention will need to be focused on providing good continuity outpatient care for patients at high risk for readmissions.
Acknowledgements
The authors acknowledge Janelle Lee, MBA, MHA, DrPH, for her assistance with extracting the hospital claims data at UCSF Medical Center.
Disclosure: Dr. Tang and Ms. Maselli were supported by a University of California, Center for Health Quality and Innovation grant. The authors report no conflicts of interest.
Reducing hospital readmissions is a national healthcare priority. In October 2012, the Centers for Medicare and Medicaid Services (CMS) enacted financial penalties on hospitals with higher than average risk‐adjusted readmissions, offering an incentive pool of $850 million in the first year.[1] As a result, a wide range of activities to understand and reduce readmissions among patients with congestive heart failure (CHF), acute myocardial infarction (AMI), and pneumonia have emerged.[2, 3, 4, 5] Some have proposed that to effectively reduce hospital readmissions, a community of inpatient and outpatient providers and local support organizations must coordinate efforts.[6, 7] In fact, CMS has recognized the important role of community support groups and outpatient providers in safe discharge transitions in 2 ways. First, in 2011 CMS launched the Community‐based Care Transitions Program to fund community‐based organizations to assist Medicare patients with care transitions.[8] Second, CMS introduced 2 new reimbursement codes for primary care providers (PCPs) to perform care coordination immediately after hospital discharge.[9] Both of these new payment programs represent an evolving perspective that reducing hospital readmissions requires active participation among outpatient partners.
As leaders of the outpatient care team, PCPs play a significant role in reducing hospital readmissions. One way in which PCPs can begin to understand the magnitude of the issue within their clinic is to evaluate the clinic's 30‐day readmission rates. Currently, CMS calculates readmission rates at the hospital level. However, understanding these rates at the clinic level is critical for developing strategies for improvement across the care continuum. Our current understanding of effective outpatient interventions to reduce hospital readmission is limited.[3] As clinics introduce and refine strategies to reduce readmissions, tracking the impact of these strategies on readmission rates will be critical for identifying effective outpatient interventions. Clinics with similar patient case‐mix can also benchmark readmission rates, sharing best practices from clinics with lower‐than‐expected rates.
There are no available studies or proposed methodologies to guide primary care clinics in calculating their 30‐day readmission rates. A particularly difficult challenge is obtaining the admission information when patients may be admitted to 1 of several area hospitals. For large integrated delivery networks where primary care patients are relatively loyal to the network of physicians and hospitals, an opportunity exists to explore the data. Furthermore, variations in readmission rates across primary care specialties (such as internal medicine and family practice) are not well understood. In this study, we set out to develop a methodology for calculating all‐cause 30‐day hospital readmission rates at the level of individual primary care practices and to identify factors associated with variations in these rates.
METHODS
Study Design
We conducted a retrospective observational study of adult primary care patients at the University of California, San Francisco (UCSF) who were hospitalized at UCSF Medical Center between July 1, 2009 and June 30, 2012. UCSF Medical Center is comprised of Moffitt‐Long Hospital (a 600‐bed facility) and UCSF‐Mount Zion Hospital (a 90‐bed facility) located in San Francisco, CA. The patient population was limited to adults ages 18 and over with a PCP at UCSF. UCSF has 7 adult primary care clinics: General Internal Medicine (IM), Family Practice (FP), Women's Health, Geriatrics, a combined IM/FP clinic, Human Immunodeficiency Virus (HIV) Primary Care, and a Concierge Internal Medicine clinic staffed by IM physicians. Between 2009 and 2011, all clinics completed the process of enpanelment, or defining the population of patients for which each PCP and the clinic is responsible. We obtained the list of patient and PCP assignments at each of the 7 clinics across the time period of study. We then obtained UCSF Medical Center hospital claims data for this group, including dates of admission and discharge, patient age, sex, race/ethnicity, language, insurance, admitting service, diagnosis codes, information on intensive care unit stay, and discharge disposition. Hospital claims data are housed in Transition Systems International (Boston, MA) administrative databases, a cost‐accounting system that collects data abstracted from patient charts upon discharge from UCSF Medical Center.
All‐cause 30‐day hospital readmission rates were calculated for each primary care clinic, using an adaptation of the CMS definition. First, we defined index discharges as the first discharge for an individual patient in any given 30‐day interval. Only 1 index discharge is flagged for each 30‐day interval. The first admission within 30 days after the index discharge was flagged as the readmission. Consistent with CMS methodology, only the first readmission in the 30‐day period was counted. We included all inpatient and observation status admissions and excluded patients who died during the index encounter, left against medical advice, or transferred to another acute care hospital after the index encounter.
We used secondary diagnosis codes in the administrative data to classify comorbidities by the Elixhauser methodology.[10] All but 1 adult primary care clinic at UCSF are faculty‐only clinics, whereby the assigned PCP is an attending physician. In the general internal medicine clinic, an attending or a resident can serve as the PCP, and approximately 20% of IM clinic patients have a resident as their PCP. For the IM clinic, we classified patients' PCP as attending, resident, or departed. The latter category refers to patients whose PCPs were residents who had graduated or faculty who had departed and had not been assigned to a new PCP prior to the index admission or readmission.
Statistical Analysis
We built a model to predict readmissions using the demographic and clinical variables with [2] P<0.20 in an initial bivariate analysis, and then removed, with backward selection, the least significant variables until only those with P0.05 remained. Age, log‐length of stay (LOS), and intensive care unit stay were forced in the model, as studies evaluating factors related to readmissions have often included these as important covariates.[11, 12, 13, 14] Results were expressed as adjusted odds ratio (OR) with 95% confidence interval (CI). All analyses were carried out using SAS version 9.2 (SAS Institute, Inc., Cary, NC).
This study was exempt from review by the institutional review board of UCSF.
RESULTS
During the study period, there were 12,564 discharges from UCSF Medical Center for primary care patients belonging to the 7 clinics. Of these, 8685 were index discharges and 1032 were readmissions within 30 days. Table 1 shows the characteristics of the patients who had at least 1 admission during the study period. In all but 2 clinics (HIV Primary Care and Concierge Internal Medicine), there were more women hospitalized than men. Age and gender differences across clinics are consistent with the patient populations served by these clinics, with Women's Health having more female patients and younger patients hospitalized compared to Geriatrics.
General Internal Medicine | Family Practice | Women's Health | Geriatrics | Combined IM/FP | HIV Primary Care | Concierge IM | |
---|---|---|---|---|---|---|---|
| |||||||
Panel size | 26,521 | 10,495 | 8,526 | 190 | 4,109 | 1,039 | 706 |
FTE attending MD and NP providers | 18.8 | 7.3 | 4.5 | 1.7 | 5.0 | 2.3 | 2.1 |
Mean panel size per FTE | 1,311 | 1,448 | 1,895 | 112 | 822 | 452 | 336 |
Clinic visits in FY 2012 | 50,362 | 20,647 | 11,014 | 4,425 | 8,120 | 4,182 | 1,713 |
No. of index discharges | 5,388 | 1,204 | 983 | 409 | 339 | 249 | 113 |
No. of readmissions | 718 | 104 | 76 | 56 | 30 | 37 | 11 |
No. of patients discharged during study period | 4,063 | 1,003 | 818 | 289* | 290 | 185 | 84 |
% Male | 40.1% | 33.9% | 4.3% | 35.3% | 33.8% | 69.7% | 52.4% |
Average age (SD), y | 60.4 (18.6) | 52.0 (19.0) | 47.0 (15.7) | 83.2 (6.9) | 46.3 (16.9) | 49.5 (9.9) | 64.5 (15.6) |
Age range, y | 19104 | 1896 | 1997 | 6399 | 1890 | 2273 | 2092 |
Race | |||||||
% White | 42.2% | 41.3% | 58.8% | 61.9% | 49.3% | 61.0% | 91.7% |
% Black | 16.3% | 8.5% | 7.5% | 7.3% | 10.0% | 27.6% | 0.0% |
% Asian | 23.6% | 31.4% | 19.0% | 17.0% | 16.6% | 2.2% | 4.8% |
% Native America/ Alaskan Native | 0.7% | 1.6% | 0.7% | 0.7% | 0.7% | 0.5% | 0.0% |
% Other | 16.3% | 15.7% | 13.1% | 12.5% | 18.3% | 8.1% | 2.4% |
Not available | 1.0% | 1.6% | 1.0% | 0.7% | 5.2% | 0.5% | 1.2% |
Ethnicity | |||||||
% Hispanic | 8.8% | 10.6% | 5.0% | 6.2% | 8.3% | 4.9% | 0.0% |
% Non‐Hispanic | 73.8% | 74.5% | 81.8% | 73.0% | 74.5% | 84.9% | 75.0% |
Not available | 17.4% | 15.0% | 13.2% | 20.8% | 17.2% | 10.3% | 25.0% |
Language | |||||||
% English | 69.7% | 75.6% | 88.8% | 69.6% | 85.9% | 89.7% | 89.3% |
% Spanish | 3.8% | 1.9% | 0.6% | 4.2% | 1.0% | 0.5% | 0.0% |
% Chinese (Mandarin or Cantonese) | 8.0% | 6.7% | 1.2% | 4.8% | 2.1% | 0.0% | 0.0% |
% Russian | 1.7% | 0.6% | 0.1% | 0.4% | 1.0% | 0.0% | 0.0% |
% Vietnamese | 1.0% | 1.4% | 0.0% | 0.4% | 0.0% | 0.0% | 0.0% |
% Other | 11.7% | 9.3% | 5.6% | 18.0% | 6.9% | 8.1% | 4.8% |
Not available | 4.1% | 4.6% | 3.7% | 2.8% | 3.1% | 1.6% | 6.0% |
Insurance type | |||||||
% Private | 35.4% | 58.7% | 77.4% | 10.7% | 63.8% | 36.8% | 66.7% |
% Medicare | 22.0% | 17.2% | 12.0% | 67.8% | 14.8% | 8.7% | 33.3% |
% Medicaid | 15.5% | 12.5% | 3.1% | 1.4% | 12.8% | 24.3% | 0.0% |
% Dual eligible | 24.4% | 8.8% | 5.4% | 19.4% | 6.6% | 27.6% | 0.0% |
% Self‐pay/other | 2.6% | 2.9% | 2.2% | 0.7% | 2.1% | 2.7% | 0.0% |
All‐cause 30‐day readmission rates varied across practices, with HIV Primary Care being the highest at 14.9%, followed by Geriatrics at 13.7%, General Internal Medicine at 13.3%, Concierge Internal Medicine at 9.7%, combined IM/FP at 8.9%, Family Practice at 8.6%, and Women's Health at 7.7% (Figure 1). Despite HIV Primary Care having the highest readmission rate, the number of index discharges during the 3‐year period was relatively low (249) compared to General Internal Medicine (5388). For the index admission, the top 5 admitting services were medicine, obstetrics, cardiology, orthopedic surgery, and general surgery (Table 2). Medicine was the primary admitting service for patients in the following clinics: General Internal Medicine, Geriatrics, HIV Primary Care, and Concierge Internal Medicine. Obstetrics was the primary admitting service for patients in the Family Practice, Women's Health, and combined IM/FP clinics. Vaginal delivery was the top discharge diagnosis‐related group (DRG) for patients in the General Internal Medicine, Family Practice, Women's Health, and combined IM/FP clinics. The top discharge DRG was urinary tract infection for Geriatrics, HIV for the HIV clinic (13.6%), and chemotherapy (8.0%) for Concierge Internal Medicine. Average LOS varied from 4.7 days for patients in the HIV Primary Care clinic to 2.8 days for patients in the Concierge Internal Medicine clinic. Average LOS was 3.4, 3.1, and 3.2 days in the Family Practice, Women's Health, and Geriatrics clinics, respectively, and 3.8 days in the General Internal Medicine and combined IM/FP clinics. For all clinics except Geriatrics, the majority of patients were discharged home without home health. A larger proportion of patients in the Geriatrics clinic were discharged home with home health or discharged to a skilled nursing facility.
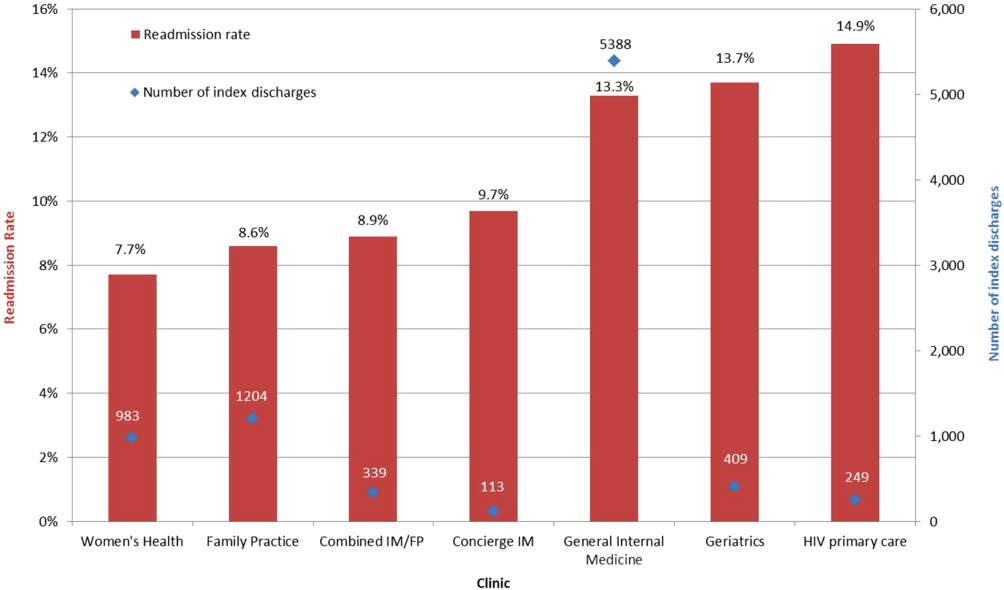
General Internal Medicine, N=5,388 | Family Practice, N=1,204 | Women's Health, N=983 | Geriatrics, N=409 | Combined IM/FP, N=339 | HIV Primary Care, N=249 | Concierge IM, N=113 | |
---|---|---|---|---|---|---|---|
| |||||||
Top 5 admitting services | |||||||
Medicine | 41.8% | 25.0% | 15.2% | 56.4% | 23.5% | 59.7% | 19.0% |
Obstetrics | 7.5% | 24.7% | 38.7% | 0.0% | 35.6% | 2.1% | 9.0% |
Cardiology | 15.1% | 10.9% | 6.2% | 16.5% | 10.3% | 6.5% | 17.0% |
Orthopedic surgery | 8.3% | 8.3% | 8.2% | 7.3% | 5.3% | 4.8% | 12.0% |
Adult general surgery | 7.2% | 9.8% | 10.0% | 6.0% | 5.0% | 5.8% | 7.0% |
Top 5 discharge diagnoses | |||||||
Vaginal delivery | 3.5% | 13.6% | 20.6% | 0.0% | 17.9% | 0.3% | 3.0% |
Major joint replacement | 3.5% | 3.4% | 4.8% | 4.3% | 3.8% | 0.7% | 5.0% |
Vaginal delivery with complications | 1.3% | 5.0% | 6.9% | 0.0% | 4.1% | 1.0% | 2.0% |
Simple pneumonia and pleurisy | 2.8% | 1.2% | 0.6% | 3.8% | 0.3% | 2.1% | 0.0% |
Urinary tract infection | 2.0% | 1.5% | 1.4% | 6.2% | 0.9% | 2.4% | 1.0% |
Discharge disposition | |||||||
% Home | 69.1% | 74.6% | 76.6% | 47.8% | 69.9% | 78.6% | 68.4% |
% Home with home health | 19.4% | 16.5% | 17.8% | 24.1% | 21.7% | 11.8% | 18.8% |
% Skilled nursing facility | 7.2% | 4.9% | 3.0% | 18.9% | 3.3% | 5.0% | 0.8% |
% Other | 4.3% | 4.0% | 2.6% | 9.2% | 5.1% | 4.6% | 12.0% |
Average length of stay (SD) | 3.8 (5.6) | 3.4 (7.5) | 3.1 (3.7) | 3.2 (3.7) | 3.8 (5.2) | 4.7 (6.5) | 2.8 (2.8) |
% Discharges with ICU stay | 11.0% | 10.6% | 5.9% | 8.5% | 9.2% | 11.1% | 15.8% |
Factors associated with variation in readmission rates included: male gender (OR: 1.21, 95% CI: 1.051.40), Medicare (OR: 1.31, 95% CI: 1.051.64; Ref=private) and dual‐eligible Medicare‐Medicaid insurance (OR: 1.26, 95% CI: 1.011.56), unknown primary language (OR: 0.06, 95% CI: 0.020.25; Ref=English), and the following comorbidities: peripheral vascular disease, renal failure, lymphoma, fluid and electrolyte disorders, and anemia. Multivariable logistic regression modeling results are listed in Table 3. Patients having a resident PCP showed no increased odds of readmission (OR: 1.13, 95% CI: 0.931.37; Ref=attending PCP). However, patients with a graduated resident PCP or departed faculty PCP awaiting transfer to a new PCP had an OR of 1.59 (95% CI: 1.162.17) compared with having a current faculty PCP. The C‐statistic for this model was 0.67.
Adjusted Odds Ratio (95% CI) | |
---|---|
| |
Age | |
<65 years | Ref |
>65 years | 0.91 (0.751.10) |
Clinic | |
General Internal Medicine | 1.24 (0.991.56) |
Family Practice | Ref |
Women's Health | 1.13 (0.821.56) |
Geriatrics | 1.20 (0.841.74) |
Combined Internal Medicine/Family Practice | 1.13 (0.731.74) |
HIV Primary Care | 1.24 (0.811.89) |
Concierge Internal Medicine | 1.06 (0.542.07) |
Sex | |
Female | Ref |
Male | 1.21 (1.051.40) |
Insurance | |
Private | Ref |
Medicaid | 1.09 (0.871.37) |
Medicare | 1.31 (1.051.64) |
Dual eligible MedicareMedicaid | 1.26 (1.011.56) |
Self/other | 1.20 (0.741.94) |
Language | |
English | Ref |
Spanish | 1.02 (0.701.47) |
Chinese | 1.13 (0.871.47) |
Other | 0.87 (0.701.08) |
Unknown | 0.06 (0.020.25) |
Comorbidities | |
Pulmonary circulation disease | 1.51 (0.982.32) |
Peripheral vascular disease | 1.50 (1.141.98) |
Renal failure | 1.31 (1.091.58) |
Lymphoma | 2.50 (1.434.36) |
Fluid and electrolyte disorders | 1.27 (1.071.52) |
Deficiency anemia | 1.25 (1.041.51) |
Physician | |
Attending | Ref |
Resident | 1.13 (0.931.37) |
Departed PCP (internal medicine only) | 1.59 (1.162.17) |
ICU stay | 1.03 (0.831.28) |
LOS (log) | 1.02 (0.981.06) |
Admitting service | |
Medicine | Ref |
Obstetrics | 0.41 (0.300.56) |
Cardiology | 1.12 (0.911.37) |
Orthopedic surgery | 0.36 (0.250.51) |
Adult general surgery | 0.64 (0.470.87) |
Other services | 0.76 (0.630.92) |
DISCUSSION
In this study we introduce a complementary way to view hospital readmissions, from the perspective of primary care practices. We found variation in readmission rates across primary care practices. After controlling for admitting service, clinic, provider, and patient factors, the specific characteristics of male gender, patients with Medicare or Medicare with Medicaid, and patients in our General Internal Medicine clinic with a departed PCP were independent risk factors for hospital readmission. Patients with specific comorbidities were also at increased risk for admissions, including those with peripheral vascular disease, renal failure, lymphoma, fluid and electrolyte disorders, and anemia. Because this is the first study viewing readmissions from the perspective of primary care practices, our findings are unique in the literature. However, hospital‐based studies have found similar relationships between readmission rates and these specific comorbidities.[11, 12, 15] Unlike other studies, our study cohort did not show CHF as an independent risk factor. We hypothesize this is because in 2008, UCSF Medical Center introduced an inpatient‐based intervention to reduce readmissions in patients with heart failure. By 2011, the readmission rates of patients with heart failure (primary or secondary diagnoses) had dropped by 30%. The success of this program, focused only on patients with heart failure, likely affected our analysis of comorbidities as risk factors for readmissions.
Models developed to predict hospital readmissions, overall and for specific disease conditions, have inconsistently identified predictive factors, and there is not a specific set of variables that dominate.[16, 17, 18, 19] A recent review of readmission risk prediction models suggested that models that take into account psychosocial factors such as social support, substance abuse, and functional status improved model performance.[16] We hypothesize that the reason why male gender was significant in our model may be related to lack of social support, especially among those who may be single or widowed. Other studies have also showed male gender as a predictor for hospital readmissions.[14, 20]
People with Medicare as the primary payor or Medicare with Medicaid also tended to have higher risk of readmission. We believe that this may be a proxy for the combined effect of age, multiple comorbidities, and psychosocial factors. In a multicenter study of general medicine inpatients, Medicare, but not age, was also found to be a predictive variable.[21] Unlike other studies, our study did not find Medicaid alone as a significant predictive variable for readmissions.[15, 21] One explanation may be that in hospital‐based studies, people with Medicaid who are at high risk for readmission may be high‐risk because they do not have a PCP or good access to outpatient care. In our study, all patients have a PCP in 1 of the UCSF clinics, and access to care is improved with this established relationship. These other studies did not examine Medicare‐Medicaid dual eligibility status. Our results are consistent with a national study on avoidable hospital admissions that showed the dual eligibility population experiencing 60% higher avoidable admission rates compared to the Medicaid‐only population.[22]
An interesting finding was that there were no statistically significant differences in readmissions among patients who report a language other than English as their primary language. Although language barriers and health literacy can affect a patient's ability to understand discharge instructions, the use of translators at UCSF Medical Center may have decreased the risk of readmissions. For a small number of patients whose primary language was not recorded (unknown language in our model), they appeared to have a lower risk of readmissions. Language preferences are recorded by our admitting staff during the process of admission. This step may have been skipped after hours or if the patient was not able to answer the question. However, we do not have a good hypothesis as to why these patients may have a reduced risk of readmissions.
Having a departed PCP in the General Internal Medicine clinic was an independent predictor of readmissions. These patients have access to primary care; they can schedule acute appointments with covering providers for new medical issues or follow‐up of chronic conditions. However, until they are transferred to a new PCP, they do not have a provider who is proactively managing their preventive and chronic disease care, including follow‐up and coordinating care after hospital discharge. Our study is not the first to suggest adverse outcomes for patients who are in transition from 1 primary care provider to the next.[23] Studies conducted in General Internal Medicine clinics have shown missed opportunities for cancer screening and overlooked test results during the transition period,[24] and as many as one‐fifth of patients whom residents identified as high‐risk were lost to follow‐up.[25] However, our study is the first to show the link between PCP transition in a teaching clinic and hospital readmissions. This finding underscores the importance of continuity of care in the optimal management of patients following hospital discharge.
There are several limitations to this study. First, our study only considers hospitalizations to UCSF Medical Center, potentially undercounting readmissions to area hospitals. Because our study population are patients with PCPs at UCSF, these patients tend to seek specialty and acute care at UCSF Medical Center as well. We obtained payor data from our medical group (Hill Physicians), which covers our largest private payors, to understand whether our results can be applied on a global basis. We found that in 87.4% of index admissions with readmissions from January 1, 2010 through May 30, 2012, the readmission occurred at UCSF Medical Center. We conducted a similar analysis with CMS data from October 1, 2008 to June 30, 2011, the latest data we have from CMS. For patients with UCSF PCPs and a diagnosis of AMI or CHF, 100% were readmitted back to UCSF Medical Center. For patients with UCSF PCPs and a diagnosis of pneumonia, 89% were readmitted back to UCSF Medical Center. Given that only a small percentage of patients with PCPs at UCSF present for readmission at other area hospitals, we believe that limiting our analysis to UCSF Medical Center is reasonable.
In our study, we did not remove vaginal deliveries or Caesarian sections prior to building the model. Primary care physicians and their clinic leadership are accustomed to taking a population‐health perspective. We anticipate they would be interested in designing interventions and addressing readmission for the entire primary care panel. Although readmissions after delivery are not frequent, they can still occur, and interventions should not necessarily exclude this population. We did run a sensitivity analysis by removing vaginal delivery and Caesarian sections from the analysis. As expected, readmission rates for all practices increased except for Geriatrics. However, the independent predictors of readmissions did not change.
Our study is based on a population of patients at 1 urban academic medical center and may not be generalizable across all delivery systems. Our population is racially and ethnically diverse, and many do not speak English as their primary language. The study also spans different types of primary care clinics, capturing a wide range of ages and case mix. As PCP assignments fluctuate over time, there may be errors with PCP attribution in the UCSF Medical Center data systems. We do not believe errors in PCP attribution would differ across primary care practices. Because the primary care practices' performance reports on quality measures are based on PCP assignment, each clinic regularly updates their clinic panels and has specific protocols to address errors with PCP attribution.
Finally, our study includes only the variables that we were able to extract from administrative claims. Other explanatory variables that have been suggested as important for evaluation, such as social support, functional assessment, access to care, hospital discharge process, and posthospitalization follow‐up, were not included. Each of these could be explored in future studies.
This study offers a unique perspective of hospital readmission by introducing a new methodology for primary care clinics to calculate and evaluate their all‐cause 30‐day readmission rates. Although this methodology is not intended to provide real‐time feedback to clinics on readmitted patients, it opens the door for benchmarking based on specific case‐mix indices. Another direction for future research is to design robust evaluations of the impact of interventions, both inpatient and outpatient, on primary care clinic readmission rates. Finally, future research should replicate this analysis across teaching clinics to identify whether provider turnover is a consistent independent predictor of hospital readmissions.
This study also has implications for inpatient interventions. For example, discharging physicians may consider proactively identifying whether the patient has a continuous primary care provider. Patients who are in between PCPs may need closer follow‐up after discharge, until they re‐establish with a new PCP. This can be accomplished through a postdischarge clinic visit, either run by inpatient providers or covering physicians in the primary care clinic. In addition, the discharging physician may work with the case manager to increase the level of care coordination. The case manager could contact the primary care clinic and proactively ask for immediate PCP re‐assignment. Once a new PCP has been identified, the discharging physician could consider a warm hand‐off. With a warm hand‐off, the new PCP may feel more comfortable managing problems that may arise after hospital discharge, and especially before the first outpatient visit with the new PCP. Future research can test whether these interventions could effectively reduce hospital readmissions across a broad primary care population.
CONCLUSION
Primary care providers and their clinics play an important role in managing population health, decreasing healthcare spending, and keeping patients out of the hospital. In this study, we introduce a tool in which primary care clinics can begin to understand their hospital readmission rates. This may be particularly valuable as primary care providers enter global payment arrangements such as accountable care organizations or bundled payments and are responsible for a population of patients across the continuum of care. We found significant variation in readmission rates between different primary care practices, but much of this variation appears to be due to differences between practices in patient demographics, comorbidities, and hospitalization factors. Our study is the first to show the association between provider transitions and higher hospital readmissions. Continuity of care is critical for the optimal management of patients following hospital discharge. More attention will need to be focused on providing good continuity outpatient care for patients at high risk for readmissions.
Acknowledgements
The authors acknowledge Janelle Lee, MBA, MHA, DrPH, for her assistance with extracting the hospital claims data at UCSF Medical Center.
Disclosure: Dr. Tang and Ms. Maselli were supported by a University of California, Center for Health Quality and Innovation grant. The authors report no conflicts of interest.
- Medicare's new hospital value‐based purchasing program is likely to have only a small impact on hospital payments. Health Aff. 2012;31:1932–1940. , .
- Contemporary evidence about hospital strategies for reducing 30‐day readmissions. J Am Coll Cardiol. 2012;60:607–614. , , , et al.
- Interventions to reduce 30‐day rehospitalization: a systematic review. Ann Intern Med. 2011;155:520–528. , , , , .
- Reducing hospital readmissions: lessons from top‐performing hospitals. The Commonwealth Fund Synthesis Report. Available at: http://www.commonwealthfund.org/publications/case‐studies/2011/apr/reducing‐hospital‐readmissions. Published April 6, 2011. Accessed February 8, 2013. , , .
- Effective Interventions to Reduce Rehospitalizations: A Survey of the Published Evidence. Cambridge, MA: Institute for Healthcare Improvement; 2009. , .
- Recasting readmissions by placing the hospital role in community context. JAMA. 2013;309:351–352. , , .
- Hospitalists and care transitions: the divorce of inpatient and outpatient care. Health Affairs. 2008;27:1315–1327. , , , .
- Center for Medicare and Medicaid Innovation. Community‐based Care Transitions Program. Available at: http://innovation.cms.gov/initiatives/CCTP/#collapse‐tableDetails. Accessed February 8, 2013.
- Medicare's transitional care payment—a step toward the medical home. N Engl J Med. 2013;368:692–694. , , .
- Volume thresholds and hospital characteristics in the United States. Health Aff (Millwood). 2003;22:167–177. , , .
- Thirty‐day rehospitalizations after acute myocardial infarction: a cohort study. Ann Intern Med. 2012;157:11–18. , , , , , .
- Rehospitalizations among patients in the Medicare fee‐for‐service program. N Engl J Med. 2009;360:1418–1428. , , .
- Associations between reduced hospital length of stay and 30‐day readmission rate and mortality: 14‐year experience in 129 Veterans Affairs hospitals. Ann Intern Med. 2012;157:837–845. , , , et al.
- Readmission after hospitalization for congestive heart failure among Medicare beneficiaries. Arch Intern Med. 1997;157:99–104. , , , et al.
- Redefining readmission risk factors for general medicine patients. J Hosp Med. 2011;6:54–60. , , , .
- Risk prediction models for hospital readmission: a systematic review. JAMA. 2011;206:1688–1698. , , , et al.
- Statistical models and patient predictors of readmission for heart failure: a systematic review. Arch Intern Med. 2008;168:1371–1386. , , , et al.
- Statistical models and patient predictors of readmission for acute myocardial infarction: a systematic review. Circ Cardiovasc Qual Outcomes. 2009;2:500–507. , , , .
- Predictors of hospital readmission after stroke: a systematic review. Stroke. 2010;41:2525–2533. , , , et al.
- Risk factors for 30‐day hospital readmission in patients ≥65 years of age. Proc (Bayl Univ Med Cent). 2008;21:363–372. , , , , .
- Hospital readmission in general medicine patients: a prediction model. J Gen Intern Med. 2010;25:211–219. , , , et al.
- Users of Medicaid home and community‐based services are especially vulnerable to costly avoidable hospital admissions. Health Affairs. 2012;31:1167–1175. , , .
- Academic year‐end transfers of outpatients from outgoing to incoming residents: an unaddressed patient safety issue. JAMA. 2009;302:1327–1329. , .
- The revolving door of resident continuity practice: identifying gaps in transitions of care. J Gen Intern Med. 2011;26:995–998. , , , , , .
- Outcomes for resident‐identified high‐risk patients and resident perspectives of year‐end continuity clinic handoffs. J Gen Intern Med. 2012;27:1438–1444. , , , et al.
- Medicare's new hospital value‐based purchasing program is likely to have only a small impact on hospital payments. Health Aff. 2012;31:1932–1940. , .
- Contemporary evidence about hospital strategies for reducing 30‐day readmissions. J Am Coll Cardiol. 2012;60:607–614. , , , et al.
- Interventions to reduce 30‐day rehospitalization: a systematic review. Ann Intern Med. 2011;155:520–528. , , , , .
- Reducing hospital readmissions: lessons from top‐performing hospitals. The Commonwealth Fund Synthesis Report. Available at: http://www.commonwealthfund.org/publications/case‐studies/2011/apr/reducing‐hospital‐readmissions. Published April 6, 2011. Accessed February 8, 2013. , , .
- Effective Interventions to Reduce Rehospitalizations: A Survey of the Published Evidence. Cambridge, MA: Institute for Healthcare Improvement; 2009. , .
- Recasting readmissions by placing the hospital role in community context. JAMA. 2013;309:351–352. , , .
- Hospitalists and care transitions: the divorce of inpatient and outpatient care. Health Affairs. 2008;27:1315–1327. , , , .
- Center for Medicare and Medicaid Innovation. Community‐based Care Transitions Program. Available at: http://innovation.cms.gov/initiatives/CCTP/#collapse‐tableDetails. Accessed February 8, 2013.
- Medicare's transitional care payment—a step toward the medical home. N Engl J Med. 2013;368:692–694. , , .
- Volume thresholds and hospital characteristics in the United States. Health Aff (Millwood). 2003;22:167–177. , , .
- Thirty‐day rehospitalizations after acute myocardial infarction: a cohort study. Ann Intern Med. 2012;157:11–18. , , , , , .
- Rehospitalizations among patients in the Medicare fee‐for‐service program. N Engl J Med. 2009;360:1418–1428. , , .
- Associations between reduced hospital length of stay and 30‐day readmission rate and mortality: 14‐year experience in 129 Veterans Affairs hospitals. Ann Intern Med. 2012;157:837–845. , , , et al.
- Readmission after hospitalization for congestive heart failure among Medicare beneficiaries. Arch Intern Med. 1997;157:99–104. , , , et al.
- Redefining readmission risk factors for general medicine patients. J Hosp Med. 2011;6:54–60. , , , .
- Risk prediction models for hospital readmission: a systematic review. JAMA. 2011;206:1688–1698. , , , et al.
- Statistical models and patient predictors of readmission for heart failure: a systematic review. Arch Intern Med. 2008;168:1371–1386. , , , et al.
- Statistical models and patient predictors of readmission for acute myocardial infarction: a systematic review. Circ Cardiovasc Qual Outcomes. 2009;2:500–507. , , , .
- Predictors of hospital readmission after stroke: a systematic review. Stroke. 2010;41:2525–2533. , , , et al.
- Risk factors for 30‐day hospital readmission in patients ≥65 years of age. Proc (Bayl Univ Med Cent). 2008;21:363–372. , , , , .
- Hospital readmission in general medicine patients: a prediction model. J Gen Intern Med. 2010;25:211–219. , , , et al.
- Users of Medicaid home and community‐based services are especially vulnerable to costly avoidable hospital admissions. Health Affairs. 2012;31:1167–1175. , , .
- Academic year‐end transfers of outpatients from outgoing to incoming residents: an unaddressed patient safety issue. JAMA. 2009;302:1327–1329. , .
- The revolving door of resident continuity practice: identifying gaps in transitions of care. J Gen Intern Med. 2011;26:995–998. , , , , , .
- Outcomes for resident‐identified high‐risk patients and resident perspectives of year‐end continuity clinic handoffs. J Gen Intern Med. 2012;27:1438–1444. , , , et al.
© 2014 Society of Hospital Medicine
High Users of Hospital Care
Despite signs of a slowing trend,[1, 2, 3] US healthcare costs continue to rise, and cost containment remains a major area of concern. Hospital costs are the largest single category of national healthcare expenditures,[4] and the burden of cost containment is increasingly being shifted to hospitals.[5] As such, hospitals are increasingly focusing on implementing interventions to reduce rates of hospitalizations and readmissions as a mechanism to reduce overall healthcare costs.[5, 6, 7, 8, 9]
Multiple factors potentially contribute to patients being high cost, including acute care utilization,[10, 11, 12] pharmaceuticals,[13, 14] procedures,[15] catastrophic illness,[16] and high‐risk chronic conditions.[11, 17, 18] However, many hospitals are implementing interventions focused on a single subset of these high cost patientshigh users of inpatient services. Care management interventions have received particular attention, due to their perceived potential to improve quality of care while reducing costs through the mechanism of reducing hospital admissions.[19, 20, 21] Despite their increasing prevalence, there is limited evidence demonstrating the effectiveness of these programs. Among interventions targeting high cost individuals, the Medicare Care Management for High Cost Beneficiaries showed no effect on hospital admissions.[19] Another high‐profile intervention, the Citywide Care Management System led by the Camden Coalition, showed promising preliminary results, but data from a systematic evaluation are lacking.[22] Conversely, interventions targeting individuals with frequent hospitalizations have similarly shown mixed results in reducing costs.[6, 7, 9, 23]
Taken together, these data suggest that the relationship between high costs and frequent hospital use is complicated, and the population of individuals with frequent hospitalizations may not represent the entire population of high cost individuals. Thus, focusing on reduction of hospitalizations alone may be inadequate to reduce costs. For these reasons, we sought to determine how many high cost individuals would be captured by focusing only on frequently hospitalized (high admit) individuals by examining the overlap between these populations. We also sought to describe the characteristics and distinctions between the resulting subgroups of high users to better inform the design of future interventions.
METHODS
We examined the cross‐sectional relationship between high cost and high admit populations among adult patients 18 years of age hospitalized at the University of California, San Francisco (UCSF) Medical Center, a 660‐bed urban academic medical center from July 1, 2010 to June 30, 2011.
This study was conducted as part of a quality improvement project to identify high user primary care patients for complex care management. Individuals were included in the study if: (1) they had an assigned UCSF primary care provider (PCP), and (2) they had at least 1 hospitalization at UCSF during the study period. PCP assignments were ascertained from panel lists maintained by clinics; lists included individuals with at least 1 visit at any of the 8 primary care clinics at UCSF in the 2 years prior to the end of the study period. Because individuals are dropped from PCP panels at death, we were unable to ascertain or include individuals who died during the study period.
From the initial study population, we defined the high cost group as those who were in the top decile of total hospital costs, and the high admit group as those who were in the top decile of total hospitalizations during the study period. We elected to use the top decile as a cutoff given that it is a common operational definition used to identify high users to target for intervention.[24]
To examine the relationship between high cost and high admits we defined 3 further subgroups: high costlow admits, high costhigh admits, and low costhigh admits (Figure 1). To explore the face validity of these descriptors and classification scheme, we subsequently examined the proportion of total hospital costs and total hospitalizations each subgroup accounted for with respect to the study population.
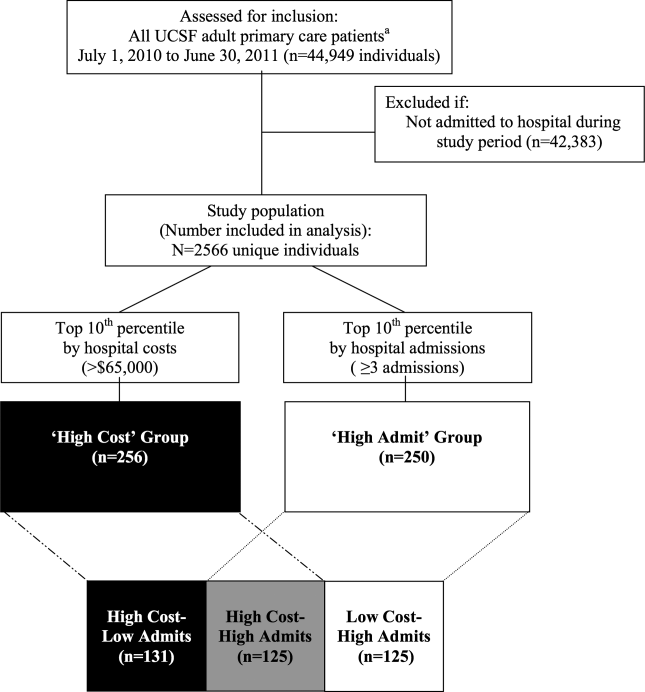
Data Sources
Hospital costs, demographic data, and encounter diagnoses were obtained from the hospital's Transition Systems Incorporated system (TSI, also known as Eclipsys or Allscripts), a commercially available automated cost accounting system that integrates multiple data sources to calculate total hospital costs on a per patient basis. Several studies have previously used the TSI system to estimate the costs of healthcare services at individual hospitals, and this approach is generally considered the most accurate method to estimate cost.[25, 26] Hospital costs included the sum of actual total costs (not billed charges) for all hospital episodes including lab costs, drug costs, surgical supplies, nurse salaries and benefits, utilities, housekeeping, and allocated administrative overhead. This cost total does not capture the cost of physician labor (pro‐fees), preadmission costs (e.g., outpatient care), or postadmission costs (e.g., home health, nursing home, or other postdischarge care). Preadmission lab, diagnostic tests, and imaging were included in hospital costs if these were ordered within 72 hours of hospital admission. Emergency department (ED) costs were included if an individual was admitted to the hospital via the ED. Hospitalizations were defined as inpatient admissions only to UCSF because we were unable to reliably ascertain hospitalizations outside of UCSF. PCP assignments were ascertained from administrative panel lists maintained by clinics.
Study Variables
We analyzed factors previously shown[13, 27, 28, 29] to be associated with high healthcare cost and utilization. We examined demographic characteristics and hospitalization characteristics, including admission source, length of stay (LOS), cost per hospitalization, whether the episode was a 30‐day readmission, days in the intensive care unit (ICU), and encounter diagnoses.
To ascertain whether a hospitalization was for a medical versus surgical condition, we used discharge diagnosis codes and designations as per the Medicare Severity Diagnosis‐Related Groups (MS‐DRG) versions 27 and 28 definitions manuals. We subsequently grouped medical and surgical conditions by Major Diagnostic Categories as per the MS‐DRG definitions manuals.
Using MS‐DRG codes, we also classified whether hospitalizations were for pneumonia, acute myocardial infarction (AMI), and congestive heart failure (CHF), as these 3 conditions have specific payment penalties under the Centers for Medicare & Medicaid Services (CMS) reimbursement policies. For these CMS core conditions, we included hospitalizations with MS‐DRG codes 193195, 280282, and 291293 (codes 283285 were not included for AMI because individuals who died during the study period were excluded.)
Analysis
We used descriptive statistics to compare patient and hospitalization characteristics between subgroups. Non‐normally distributed variables including LOS and cost per hospitalization were log transformed. Because a single individual could account for multiple hospitalizations, we performed a companion analysis of hospitalization characteristics using generalized estimating equations with an independent correlation structure to account for clustering of hospitalizations within individuals. Our findings were robust using either approach. For ease of interpretation, P values from the former analysis are presented.
To determine whether the overall distribution and characteristics we observed for high user subgroups were a single‐year anomaly or representative of temporally stable trends, we compared non‐high users and high user subgroup characteristics over the 3 years preceding the study period using linear regression for trend.
The institutional review board at UCSF approved this study protocol.
RESULTS
Of the 2566 unique individuals included in the analysis (Figure 1), 256 individuals were identified as high cost (top decile, $65,000). This group accounted for 45% of all costs and 22% of all hospitalizations (Figure 2). Two hundred fifty individuals were identified as high admits (top decile, 3 hospitalizations). This group accounted for 32% of all costs and 28% of all hospitalizations.
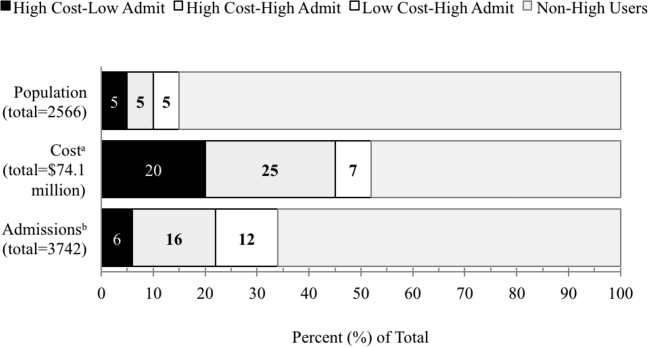
Only 48% of high cost individuals were also high admit ($65,000 and 3 hospitalizations; n=125, Figure 1). Among high users, we subsequently defined 3 subgroups based on the relationship between cost and hospitalizations (high costlow admits, high costhigh admits, and low costhigh admits). Each subgroup accounted for approximately 5% of the overall study population (Figure 2). The high costlow admits subgroup incurred a proportionate share of hospitalizations (6%) but a disproportionate share of costs (20%). The high costhigh admits subgroup had a disproportionate share of both costs (25%) and hospitalizations (16%). The low costhigh admits subgroup had a proportionate share of costs (7%) but a disproportionate share of hospitalizations (12%).
Patient and Hospitalization Characteristics
Compared to non‐high users, all high user subgroups were more likely to have public insurance (Medicare or Medicaid) or have dualeligible status, and the two high cost subgroups were more likely to be male and African American (P<0.05 for all). Compared to each other, subgroups were similar with respect to race/ethnicity, payer, and age (Table 1).
Non‐High Users,n=2145 | High User Subgroups | ||||||
---|---|---|---|---|---|---|---|
1 | 2 | 3 | P Value for Comparison | ||||
High CostLow Admit, n=131 | High CostHigh Admit, n=125 | Low CostHigh Admit, n=125 | 1 vs 2 | 2 vs 3 | 1 vs 3 | ||
| |||||||
Male, % | 34 | 57 | 42 | 38 | 0.02 | 0.52 | 0.003 |
Race/ethnicity, % | 0.15 | 0.76 | 0.18 | ||||
White | 45 | 46 | 36 | 38 | |||
Black | 14 | 21 | 26 | 19 | |||
Hispanic | 9 | 5 | 10 | 10 | |||
Asian | 22 | 18 | 22 | 26 | |||
Other | 10 | 10 | 6 | 6 | |||
Primary payer, % | 0.23 | 0.44 | 0.51 | ||||
Commercial | 42 | 24 | 20 | 17 | |||
Medicare | 42 | 58 | 55 | 61 | |||
Medicaid | 15 | 17 | 25 | 21 | |||
Other | <1 | 2 | 2 | ||||
Dual eligible, %* | 18 | 27 | 26 | 31 | 0.85 | 0.38 | 0.48 |
Age, mean yearsSD | 5720 | 6017 | 5817 | 6320 | 0.40 | 0.04 | 0.19 |
No. of hospitalizations per individual, median (interquartile range) | 1 (11) | 2 (12) | 4 (36) | 3 (34) | <0.001 | <0.001 | <0.001 |
Hospital costs per individual, median $1000 (interquartile range) | 12 (722) | 93 (75122) | 113 (85174) | 37 (3051) | <0.001 | <0.001 | <0.001 |
Regarding hospitalization characteristics, each high user subgroup was distinct and significantly different from each of the other subgroups with respect to admission source, proportion of 30‐day readmissions, LOS, and cost per hospitalization (Table 2, P<0.001 for all). The low costhigh admit subgroup had the highest proportion of admissions from the ED (73%), a moderate proportion of 30‐day readmissions (32%), the shortest LOS (median, 3 days; interquartile range [IQR], 24 days) and the lowest cost per hospitalization (median, $12,000; IQR, $8,000$15,000). In contrast, the high costlow admit subgroup had the highest proportion of admissions from clinic or physician referrals (45%), lowest proportion of 30‐day readmissions (17%), the longest LOS (median, 10; IQR, 417), the most ICU days per hospitalization (median, 1; range, 049) and the highest cost per hospitalization (median, $68,000; IQR, $43,000$95,000). High costhigh admit individuals had the highest proportion of 30‐day readmissions (47%) and a moderate cost per hospitalization (median, $28,000; IQR, $23,000$38,000), but the highest median cost per individual over 1 year ($113,000; IQR, $85,000$174,000, Table 1). Hospitalizations classified as 30‐day readmissions accounted for 42% of costs incurred by this subgroup; 30‐day readmissions specifically associated with CMS core conditions accounted for <1% of costs.
Non‐High Users | High User Subgroups | ||||||
---|---|---|---|---|---|---|---|
1 | 2 | 3 | P Value for Comparison | ||||
High CostLow Admit | High CostHigh Admit | Low CostHigh Admit | 1 vs 2 | 2 vs 3 | 1 vs 3 | ||
| |||||||
No. of admissions | 2500 | 206 | 605 | 431 | |||
Admission source, % | <0.001 | <0.001 | <0.001 | ||||
Emergency department | 53 | 50 | 65 | 73 | |||
Clinic or physician referral | 44 | 45 | 30 | 20 | |||
Transfer from outside facility | 2 | 5 | 4 | 4 | |||
Self‐referral | 1 | <1 | 1 | 3 | |||
Other | <1 | ||||||
30‐day readmission, % | 5 | 17 | 47 | 32 | <0.001 | <0.001 | <0.001 |
LOS, median days (IQR) | 3 (24) | 10 (417) | 5 (310) | 3 (24) | <0.001 | <0.001 | <0.001 |
ICU days, median (range)* | 0 (08) | 1 (049) | 0 (021) | 0 (03) | <0.001 | <0.001 | <0.001 |
Cost per hospitalization, median $1,000 (IQR) | 11 (719) | 68 (4395) | 28 (2338) | 12 (815) | <0.001 | <0.001 | <0.001 |
Encounter diagnoses | <0.001 | 0.002 | <0.001 | ||||
Surgical MS‐DRGs, % | 30 | 58 | 22 | 13 | |||
Most common MDCs | |||||||
Cardiovascular | 4 | 15 | 8 | 6 | |||
Orthopedic | 10 | 13 | 6 | 4 | |||
Transplant | <1 | 7 | 1 | 1 | |||
Medical MS‐DRGs, % | 70 | 42 | 78 | 87 | |||
Most common MDCs | |||||||
Pregnancy related | 17 | 2 | 2 | 2 | |||
Cardiovascular | 10 | 10 | 7 | 13 | |||
Respiratory | 9 | 4 | 14 | 17 | |||
Gastrointestinal | 7 | 3 | 10 | 14 | |||
Hematologic | 1 | 2 | 9 | 6 | |||
Myeloproliferative | <1 | 4 | 9 | 6 | |||
CMS core condition | 7 | 3 | 6 | 12 | 0.174 | 0.01 | 0.004 |
Encounter diagnoses associated with hospitalizations were also significantly different between each of the high user subgroups (Table 2, P<0.001 for all). The high costlow admit subgroup was predominantly hospitalized for surgical conditions (58% vs 42% for medical MS‐DRGs) and had the lowest proportion of hospitalizations for CMS core conditions (3%). The most common types of surgical hospitalizations in this subgroup were for cardiovascular procedures (15%; including coronary artery bypass grafting and cardiac valve replacement) and orthopedic procedures (13%; including hip, knee, and other joint replacements). Most surgical hospitalizations were from referrals (67%) rather than admissions through the ED. In contrast, the low costhigh admit group was predominantly hospitalized for medical conditions (87% vs 13% for surgical MS‐DRGs) and had the highest proportion of hospitalizations for CMS core conditions (12%). The most common types of medical hospitalizations in this subgroup were for respiratory conditions (17%; including chronic obstructive pulmonary disease and pneumonia), gastrointestinal conditions (14%), and cardiovascular conditions (13%; including CHF, AMI, arrhythmia, and chest pain). High costhigh admit individuals were also hospitalized primarily for medical rather than surgical conditions (78% vs 22% medical vs surgical MS‐DRGs). Only 6% of hospitalizations in this subgroup were for CMS core conditions, and only 2% of hospitalizations were 30‐day readmissions for CMS core conditions.
The overlap between the high cost and high admit groups was persistently 48% or less for the 3 years prior to the study period (Table 3). Although the extent of overlap was similar across years, the absolute dollar value for the cutoff to define the top decile by hospital costs gradually increased over time from $47,000 in 2008 to $65,000 in 2011 (P<0.001 for trend). Among the high costlow admit subgroup, there was a trend toward a decrease in the proportion of surgical hospitalizations from 67% in 2008 to 58% in 2011 (P=0.09).
2008 | 2009 | 2010 | 2011 | P Value (For Linear Trend) | |
---|---|---|---|---|---|
| |||||
Study population | 2408 | 2518 | 2647 | 2566 | |
Characteristics, n | |||||
Cutoff for high cost (top decile), nearest $1000 | >47 | >51 | >54 | >65 | <0.001 |
Proportion of total hospital costs incurred by high cost group, % | 46 | 47 | 47 | 48 | |
Cutoff for high admit (top decile), no. of admissions | 3 | 3 | 3 | 3 | |
High cost who are also high admit, % | 42 | 48 | 48 | 48 | |
Discharge diagnoses by subgroup* | |||||
Non‐high user population | |||||
Surgical MS‐DRG | 32 (751) | 33 (842) | 36 (932) | 30 (751) | 0.51 |
Medical MS‐DRG | 68 (1598) | 67 (1676) | 64 (1673) | 70 (1749) | |
High costlow admit | |||||
Surgical MS‐DRG | 67 (138) | 68 (132) | 61 (120) | 58 (119) | 0.09 |
Medical MS‐DRG | 33(67) | 32 (63) | 39 (78) | 42 (87) | |
High costhigh admit | |||||
Surgical MS‐DRG | 23 (104) | 25 (133) | 24 (150) | 22 (134) | 0.60 |
Medical MS‐DRG | 77 (341) | 75 (392) | 76 (464) | 78 (471) | |
Low costhigh admit | |||||
Surgical MS‐DRG | 11 (35) | 17 (44) | 13 (40) | 13 (54) | 0.90 |
Medical MS‐DRG | 89 (277) | 83 (219) | 87 (269) | 87 (377) |
DISCUSSION
In this study, we found that only half of high cost individuals were also high admit. Further categorizing high users into high costlow admit versus high costhigh admit versus low costhigh admit identified distinct patterns between each group. High costhigh admit individuals were more likely to be hospitalized for medical conditions, whereas high costlow admit individuals were more likely to be hospitalized for surgical conditions. CMS core conditions accounted for a low proportion of overall hospitalizations across all groups.
Our findings suggest several distinct types of high users with different clinical characteristics, utilization, and cost patterns. From a hospital perspective, one implication is that a multifaceted approach to cost containment, rather than the one‐size‐fits‐all strategy of reducing hospitalizations, may be more effective in reducing costs. For example, our findings show that high costlow admit individuals have a disproportionate number of hospitalizations for surgical conditions, longer LOS, and more ICU days. Costs incurred by this subgroup may be more responsive to in‐hospital interventions aimed at reducing procedural costs, LOS, unnecessary use of the ICU, and minimizing postoperative infections and complications rather than to a care management approach.
In contrast, care management strategies such as improving postdischarge care and chronic disease management, which aim to achieve cost savings through reducing hospitalizations, may be more effective in reducing costs among high costhigh admit individuals, who have a high proportion of hospitalizations for medical conditions and the highest proportion of 30‐day readmissions. Such strategies may also be important in optimizing the quality of care for low costhigh admit individuals, who have the highest proportion of medical hospitalizations among all high users, though the potential for cost savings may be more limited in this subgroup.
Our results suggest that current hospital‐based approachesdriven by readmissions penalties for CMS core conditionsmay have less than the expected impact on costs. For example, although high costhigh admit individuals had the highest proportion of 30‐day readmissions, readmissions specifically for CMS core conditions accounted for <1% of costs in this subgroup. Thus, the potential return on an expensive investment in a care management intervention is unclear, given the small number of readmissions for these select conditions. From a broader perspective, the focus on readmissions for CMS core conditions, which overall contribute relatively little to high hospital costs, may not be a comprehensive enough strategy for cost containment. To date, there have been limited policies targeting factors contributing to high hospital costs outside of frequent medical hospitalizations. Medicare's nonpayment policy for treatment of preventable hospital conditions, including surgical site infections, translates prevention of these conditions into cost savings for hospitals.[30] However, this rule has been criticized for not going far enough to drive substantial savings.[31] A new CMS rule authorizes states to identify other provider‐preventable conditions for which Medicaid payment will be prohibited.[32] Future policy efforts should further emphasize a comprehensive, multipronged approach beyond readmissions penalties for select conditions if healthcare cost containment remains a policy priority.
Our results should be interpreted in light of several limitations. First, this was a single‐site study at an academic medical center; the generalizability of our results to other settings is unclear. Our cost data likely reflect local market factors, including the highest wage rates for skilled healthcare labor in the United States.[33] Although the explicit distribution of high user subgroups may be institution‐specific due to variations in our cost structure, we anticipate that the general classification paradigm will be similar in other health systems. Second, we captured utilization and costs only at a single hospital. However, our study population includes only individuals with PCPs at UCSF, and internal data from both Medicare and UCSF's largest private payer show that over 85% of hospitalizations among this population are to UCSF Medical Center. Third, we were able to capture only hospital costs rather than overall healthcare costs. Given that hospital costs account for the single largest category of total national health costs,[4] we expect that future studies examining total health costs will show similar findings. Fourth, our data did not include measures of health status, socioeconomic status, housing, or mental health comorbidities to permit an analysis of these factors, which have been previously related to frequent hospitalizations and high costs.[34, 35, 36, 37, 38, 39] Fifth, due to resource constraints, we were unable to conduct a longitudinal analysis to examine the extent to which individuals are consistently high users over time. Previous studies have described that 20% to 30% of individuals are consistently high users; the remainder have discrete periods of high utilization.[34, 40] This may be an important consideration in the design of future interventions.
Finally, our analysis was limited to individuals with a PCP to allow identification of an accessible cohort for care management. Thus, we did not capture individuals without a PCP and individuals who died during the study period, because these individuals no longer had an assigned PCP following death. Although this approach is consistent with that of many care management programs,[19] these populations are likely to incur higher than average utilization and healthcare costs, and represent important areas for future investigation.
In summary, our study identifies three types of high‐user populations that differ in the proportion of costs attributable to frequent hospitalizations, clinical conditions associated with hospital use, and frequency of 30‐day readmissions. Stratifying high users by both costs and hospitalizations may help identify tailored strategies to more effectively reduce costs and utilization.
Acknowledgments
The authors acknowledge Diana Patterson, Leanna Zaporozhets, and Andre Devito for their assistance in data collection.
Disclosures: Dr. Nguyen had full access to all of the data in the study and takes responsibility for the integrity of the data and the accuracy of the data analysis. Dr. Nguyen's work on this project was completed as a primary care research fellow at the University of California, San Francisco funded by a federal training grant from the National Research Service Award (NRSA T32HP19025‐07‐00).
- National Health Expenditure Accounts Team. Recession contributes to slowest annual rate of increase in health spending in five decades. Health Aff (Millwood). 2011;30(1):11–22. , , , ;
- Growth in US health spending remained slow in 2010; health share of gross domestic product was unchanged from 2009. Health Aff (Millwood). 2012;31(1):208–219. , , , .
- the National Health Expenditure Accounts Team. National health spending in 2011: overall growth remains low, but some payers and services show signs of acceleration. Health Aff (Millwood). 2013;32(1):87–99. , , , ;
- Centers for Medicare and Medicaid Services; Office of the Actuary; National Health Statistics Group. National healthcare expenditures data. 2012. Available at: https://www.cms.gov/Research‐Statistics‐Data‐and‐Systems/Statistics‐Trends‐and‐Reports/NationalHealthExpendData/downloads/tables.pdf.
- Medicare Payment Advisory Commission. Report to the Congress: Promoting Greater Efficiency in Medicare. Washington, DC: Medicare Payment Advisory Commission; 2007.
- The care transitions intervention: results of a randomized controlled trial. Arch Intern Med. 2006;166(17):1822–1828. , , , .
- A reengineered hospital discharge program to decrease rehospitalization: a randomized trial. Ann Intern Med. 2009;150(3):178–187. , , , et al.
- How‐to Guide: Improving Transitions from the Hospital to the Clinical Office Practice to Reduce Avoidable Rehospitalizations. Cambridge, MA: Institute for Healthcare Improvement; 2012. , , , .
- Comprehensive discharge planning and home follow‐up of hospitalized elders: a randomized clinical trial. JAMA. 1999;281(7):613–620. , , , et al.
- High‐cost users of medical care. N Engl J Med. 1980;302(18):996–1002. , .
- Frequency and clinical description of high‐cost patients in 17 acute‐care hospitals. N Engl J Med. 1979;300(23):1306–1309. , , .
- Effectiveness of interventions targeting frequent users of emergency departments: a systematic review. Ann Emerg Med. 2011;58(1):41–52.e42. , , , et al.
- Medication, diagnostic, and cost information as predictors of high‐risk patients in need of care management. Am J Manag Care. 2009;15(1):41–48. , , , .
- Tracking health care costs: trends stabilize but remain high in 2002. Health Aff (Millwood). 2003;Suppl Web Exclusives:W3–266–274. , .
- An iconoclastic view of health cost containment. Health Aff (Millwood). 1993;12(suppl):152–171. .
- Comparisons of medical utilizations and categorical diagnoses of emergency visits between the elderly with catastrophic illness certificates and those without. BMC Health Serv Res. 2013;13:152. , , , et al.
- Finding future high‐cost cases: comparing prior cost versus diagnosis‐based methods. Health Serv Res. 2001;36(6 pt 2):194–206. , , , .
- Spending and service use among people with the fifteen most costly medical conditions, 1997. Health Aff (Millwood). 2003;22(2):129–138. , .
- Lessons from Medicare's Demonstration Projects on Disease Management and Care Coordination. Washington, D.C.: Congressional Budget Office; 2012. .
- The hot spotters. The New Yorker. January 24, 2011. .
- Collaboration Across the Disciplines in Health Care. Burlington, MA: Jones and Bartlett; 2010. , , .
- Hope for New Jersey's city hospitals: the Camden Initiative. Perspect Health Inf Manag. 2010:7:1d. , , .
- Hospital‐initiated transitional care interventions as a patient safety strategy: a systematic review. Ann Intern Med. 2013;158(5 pt 2):433–440. , , , , , .
- Agency for Healthcare Research and Quality. The concentration and persistence in the level of health expenditures over time: estimates for the U.S. population, 2008–2009. 2012. Available at: http://meps.ahrq.gov/mepsweb/data_files/publications/st354/stat354.shtml.
- The use of the transition cost accounting system in health services research. Cost Eff Resour Alloc. 2007;5:11. , , , , , .
- Cost reduction and quality improvement: it takes two to tango. Crit Care Med. 2000;28(2):581–583. , .
- Case finding for patients at risk of readmission to hospital: development of algorithm to identify high risk patients. BMJ. 2006;333(7563):327. , , , .
- Risk prediction models for hospital readmission: a systematic review. JAMA. 2011;306(15):1688–1698. , , , et al.
- Chronic disease score as a predictor of hospitalization. Epidemiology. 2002;13(3):340–346. , , , et al.
- Centers for Medicare and Medicaid Services. Hospital‐acquired conditions (HAC) in acute inpatient prospective payment system (IPPS) hospitals fact sheet. 2011. Available at: https://www.cms.gov/Medicare/Medicare‐Fee‐for‐Service‐Payment/HospitalAcqCond/downloads/HACFactsheet.pdf. Accessed August 31, 2013.
- Medicare's policy not to pay for treating hospital‐acquired conditions: the impact. Health Aff (Millwood). 2009;28(5):1485–1493. , , .
- Department of Health and Human Services, Centers for Medicare 2013.
- Consistently high users of medical care among the elderly. Med Care. 1990;28(6):527–540. , , , .
- Utilization patterns among long‐term enrollees in a prepaid group practice health maintenance organization. Med Care. 1985;23(11):1221–1233. , , , .
- Depressive symptoms and health service utilization among the community elderly. J Am Geriatr Soc. 1983;31(7):417–420. , , .
- The influence of socio‐economic status and multimorbidity patterns on healthcare costs: a six‐year follow‐up under a universal healthcare system. Int J Equity Health. 2013;12(1):69. , .
- Association between the level of housing instability, economic standing and health care access: a meta‐regression. J Health Care Poor Underserved. 2008;19(4):1212–1228. , , .
- High health care utilization and costs associated with lower socio‐economic status: results from a linked dataset. Can J Public Health. 2009;100(3):180–183. , , , .
- An analysis of the use of Medicare services by the continuously enrolled aged. Med Care. 1983;21(6):567–585. , .
Despite signs of a slowing trend,[1, 2, 3] US healthcare costs continue to rise, and cost containment remains a major area of concern. Hospital costs are the largest single category of national healthcare expenditures,[4] and the burden of cost containment is increasingly being shifted to hospitals.[5] As such, hospitals are increasingly focusing on implementing interventions to reduce rates of hospitalizations and readmissions as a mechanism to reduce overall healthcare costs.[5, 6, 7, 8, 9]
Multiple factors potentially contribute to patients being high cost, including acute care utilization,[10, 11, 12] pharmaceuticals,[13, 14] procedures,[15] catastrophic illness,[16] and high‐risk chronic conditions.[11, 17, 18] However, many hospitals are implementing interventions focused on a single subset of these high cost patientshigh users of inpatient services. Care management interventions have received particular attention, due to their perceived potential to improve quality of care while reducing costs through the mechanism of reducing hospital admissions.[19, 20, 21] Despite their increasing prevalence, there is limited evidence demonstrating the effectiveness of these programs. Among interventions targeting high cost individuals, the Medicare Care Management for High Cost Beneficiaries showed no effect on hospital admissions.[19] Another high‐profile intervention, the Citywide Care Management System led by the Camden Coalition, showed promising preliminary results, but data from a systematic evaluation are lacking.[22] Conversely, interventions targeting individuals with frequent hospitalizations have similarly shown mixed results in reducing costs.[6, 7, 9, 23]
Taken together, these data suggest that the relationship between high costs and frequent hospital use is complicated, and the population of individuals with frequent hospitalizations may not represent the entire population of high cost individuals. Thus, focusing on reduction of hospitalizations alone may be inadequate to reduce costs. For these reasons, we sought to determine how many high cost individuals would be captured by focusing only on frequently hospitalized (high admit) individuals by examining the overlap between these populations. We also sought to describe the characteristics and distinctions between the resulting subgroups of high users to better inform the design of future interventions.
METHODS
We examined the cross‐sectional relationship between high cost and high admit populations among adult patients 18 years of age hospitalized at the University of California, San Francisco (UCSF) Medical Center, a 660‐bed urban academic medical center from July 1, 2010 to June 30, 2011.
This study was conducted as part of a quality improvement project to identify high user primary care patients for complex care management. Individuals were included in the study if: (1) they had an assigned UCSF primary care provider (PCP), and (2) they had at least 1 hospitalization at UCSF during the study period. PCP assignments were ascertained from panel lists maintained by clinics; lists included individuals with at least 1 visit at any of the 8 primary care clinics at UCSF in the 2 years prior to the end of the study period. Because individuals are dropped from PCP panels at death, we were unable to ascertain or include individuals who died during the study period.
From the initial study population, we defined the high cost group as those who were in the top decile of total hospital costs, and the high admit group as those who were in the top decile of total hospitalizations during the study period. We elected to use the top decile as a cutoff given that it is a common operational definition used to identify high users to target for intervention.[24]
To examine the relationship between high cost and high admits we defined 3 further subgroups: high costlow admits, high costhigh admits, and low costhigh admits (Figure 1). To explore the face validity of these descriptors and classification scheme, we subsequently examined the proportion of total hospital costs and total hospitalizations each subgroup accounted for with respect to the study population.
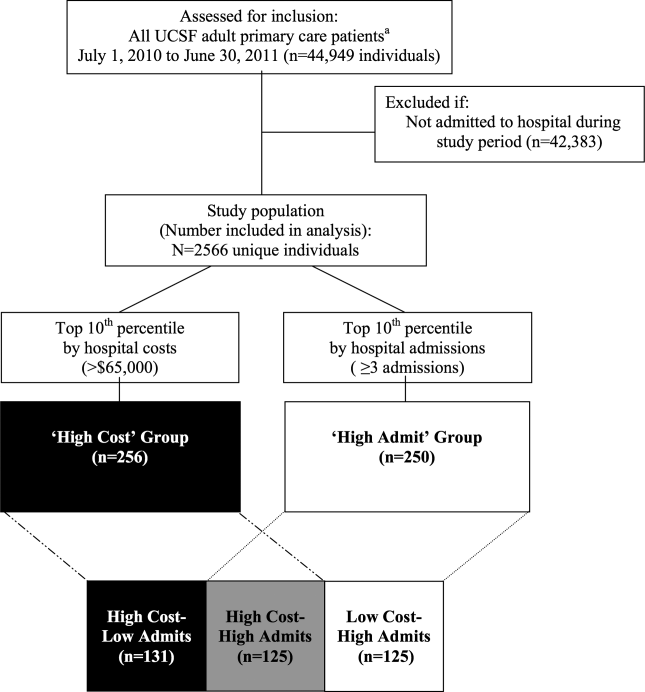
Data Sources
Hospital costs, demographic data, and encounter diagnoses were obtained from the hospital's Transition Systems Incorporated system (TSI, also known as Eclipsys or Allscripts), a commercially available automated cost accounting system that integrates multiple data sources to calculate total hospital costs on a per patient basis. Several studies have previously used the TSI system to estimate the costs of healthcare services at individual hospitals, and this approach is generally considered the most accurate method to estimate cost.[25, 26] Hospital costs included the sum of actual total costs (not billed charges) for all hospital episodes including lab costs, drug costs, surgical supplies, nurse salaries and benefits, utilities, housekeeping, and allocated administrative overhead. This cost total does not capture the cost of physician labor (pro‐fees), preadmission costs (e.g., outpatient care), or postadmission costs (e.g., home health, nursing home, or other postdischarge care). Preadmission lab, diagnostic tests, and imaging were included in hospital costs if these were ordered within 72 hours of hospital admission. Emergency department (ED) costs were included if an individual was admitted to the hospital via the ED. Hospitalizations were defined as inpatient admissions only to UCSF because we were unable to reliably ascertain hospitalizations outside of UCSF. PCP assignments were ascertained from administrative panel lists maintained by clinics.
Study Variables
We analyzed factors previously shown[13, 27, 28, 29] to be associated with high healthcare cost and utilization. We examined demographic characteristics and hospitalization characteristics, including admission source, length of stay (LOS), cost per hospitalization, whether the episode was a 30‐day readmission, days in the intensive care unit (ICU), and encounter diagnoses.
To ascertain whether a hospitalization was for a medical versus surgical condition, we used discharge diagnosis codes and designations as per the Medicare Severity Diagnosis‐Related Groups (MS‐DRG) versions 27 and 28 definitions manuals. We subsequently grouped medical and surgical conditions by Major Diagnostic Categories as per the MS‐DRG definitions manuals.
Using MS‐DRG codes, we also classified whether hospitalizations were for pneumonia, acute myocardial infarction (AMI), and congestive heart failure (CHF), as these 3 conditions have specific payment penalties under the Centers for Medicare & Medicaid Services (CMS) reimbursement policies. For these CMS core conditions, we included hospitalizations with MS‐DRG codes 193195, 280282, and 291293 (codes 283285 were not included for AMI because individuals who died during the study period were excluded.)
Analysis
We used descriptive statistics to compare patient and hospitalization characteristics between subgroups. Non‐normally distributed variables including LOS and cost per hospitalization were log transformed. Because a single individual could account for multiple hospitalizations, we performed a companion analysis of hospitalization characteristics using generalized estimating equations with an independent correlation structure to account for clustering of hospitalizations within individuals. Our findings were robust using either approach. For ease of interpretation, P values from the former analysis are presented.
To determine whether the overall distribution and characteristics we observed for high user subgroups were a single‐year anomaly or representative of temporally stable trends, we compared non‐high users and high user subgroup characteristics over the 3 years preceding the study period using linear regression for trend.
The institutional review board at UCSF approved this study protocol.
RESULTS
Of the 2566 unique individuals included in the analysis (Figure 1), 256 individuals were identified as high cost (top decile, $65,000). This group accounted for 45% of all costs and 22% of all hospitalizations (Figure 2). Two hundred fifty individuals were identified as high admits (top decile, 3 hospitalizations). This group accounted for 32% of all costs and 28% of all hospitalizations.
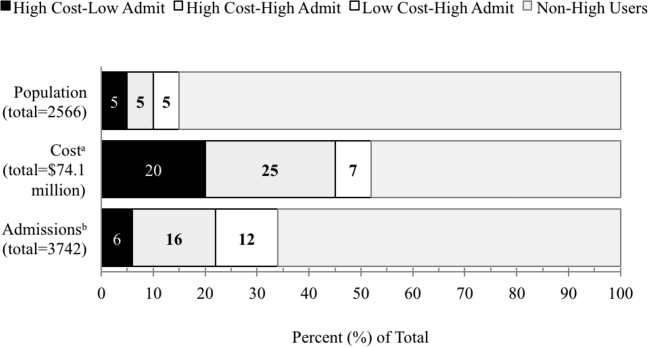
Only 48% of high cost individuals were also high admit ($65,000 and 3 hospitalizations; n=125, Figure 1). Among high users, we subsequently defined 3 subgroups based on the relationship between cost and hospitalizations (high costlow admits, high costhigh admits, and low costhigh admits). Each subgroup accounted for approximately 5% of the overall study population (Figure 2). The high costlow admits subgroup incurred a proportionate share of hospitalizations (6%) but a disproportionate share of costs (20%). The high costhigh admits subgroup had a disproportionate share of both costs (25%) and hospitalizations (16%). The low costhigh admits subgroup had a proportionate share of costs (7%) but a disproportionate share of hospitalizations (12%).
Patient and Hospitalization Characteristics
Compared to non‐high users, all high user subgroups were more likely to have public insurance (Medicare or Medicaid) or have dualeligible status, and the two high cost subgroups were more likely to be male and African American (P<0.05 for all). Compared to each other, subgroups were similar with respect to race/ethnicity, payer, and age (Table 1).
Non‐High Users,n=2145 | High User Subgroups | ||||||
---|---|---|---|---|---|---|---|
1 | 2 | 3 | P Value for Comparison | ||||
High CostLow Admit, n=131 | High CostHigh Admit, n=125 | Low CostHigh Admit, n=125 | 1 vs 2 | 2 vs 3 | 1 vs 3 | ||
| |||||||
Male, % | 34 | 57 | 42 | 38 | 0.02 | 0.52 | 0.003 |
Race/ethnicity, % | 0.15 | 0.76 | 0.18 | ||||
White | 45 | 46 | 36 | 38 | |||
Black | 14 | 21 | 26 | 19 | |||
Hispanic | 9 | 5 | 10 | 10 | |||
Asian | 22 | 18 | 22 | 26 | |||
Other | 10 | 10 | 6 | 6 | |||
Primary payer, % | 0.23 | 0.44 | 0.51 | ||||
Commercial | 42 | 24 | 20 | 17 | |||
Medicare | 42 | 58 | 55 | 61 | |||
Medicaid | 15 | 17 | 25 | 21 | |||
Other | <1 | 2 | 2 | ||||
Dual eligible, %* | 18 | 27 | 26 | 31 | 0.85 | 0.38 | 0.48 |
Age, mean yearsSD | 5720 | 6017 | 5817 | 6320 | 0.40 | 0.04 | 0.19 |
No. of hospitalizations per individual, median (interquartile range) | 1 (11) | 2 (12) | 4 (36) | 3 (34) | <0.001 | <0.001 | <0.001 |
Hospital costs per individual, median $1000 (interquartile range) | 12 (722) | 93 (75122) | 113 (85174) | 37 (3051) | <0.001 | <0.001 | <0.001 |
Regarding hospitalization characteristics, each high user subgroup was distinct and significantly different from each of the other subgroups with respect to admission source, proportion of 30‐day readmissions, LOS, and cost per hospitalization (Table 2, P<0.001 for all). The low costhigh admit subgroup had the highest proportion of admissions from the ED (73%), a moderate proportion of 30‐day readmissions (32%), the shortest LOS (median, 3 days; interquartile range [IQR], 24 days) and the lowest cost per hospitalization (median, $12,000; IQR, $8,000$15,000). In contrast, the high costlow admit subgroup had the highest proportion of admissions from clinic or physician referrals (45%), lowest proportion of 30‐day readmissions (17%), the longest LOS (median, 10; IQR, 417), the most ICU days per hospitalization (median, 1; range, 049) and the highest cost per hospitalization (median, $68,000; IQR, $43,000$95,000). High costhigh admit individuals had the highest proportion of 30‐day readmissions (47%) and a moderate cost per hospitalization (median, $28,000; IQR, $23,000$38,000), but the highest median cost per individual over 1 year ($113,000; IQR, $85,000$174,000, Table 1). Hospitalizations classified as 30‐day readmissions accounted for 42% of costs incurred by this subgroup; 30‐day readmissions specifically associated with CMS core conditions accounted for <1% of costs.
Non‐High Users | High User Subgroups | ||||||
---|---|---|---|---|---|---|---|
1 | 2 | 3 | P Value for Comparison | ||||
High CostLow Admit | High CostHigh Admit | Low CostHigh Admit | 1 vs 2 | 2 vs 3 | 1 vs 3 | ||
| |||||||
No. of admissions | 2500 | 206 | 605 | 431 | |||
Admission source, % | <0.001 | <0.001 | <0.001 | ||||
Emergency department | 53 | 50 | 65 | 73 | |||
Clinic or physician referral | 44 | 45 | 30 | 20 | |||
Transfer from outside facility | 2 | 5 | 4 | 4 | |||
Self‐referral | 1 | <1 | 1 | 3 | |||
Other | <1 | ||||||
30‐day readmission, % | 5 | 17 | 47 | 32 | <0.001 | <0.001 | <0.001 |
LOS, median days (IQR) | 3 (24) | 10 (417) | 5 (310) | 3 (24) | <0.001 | <0.001 | <0.001 |
ICU days, median (range)* | 0 (08) | 1 (049) | 0 (021) | 0 (03) | <0.001 | <0.001 | <0.001 |
Cost per hospitalization, median $1,000 (IQR) | 11 (719) | 68 (4395) | 28 (2338) | 12 (815) | <0.001 | <0.001 | <0.001 |
Encounter diagnoses | <0.001 | 0.002 | <0.001 | ||||
Surgical MS‐DRGs, % | 30 | 58 | 22 | 13 | |||
Most common MDCs | |||||||
Cardiovascular | 4 | 15 | 8 | 6 | |||
Orthopedic | 10 | 13 | 6 | 4 | |||
Transplant | <1 | 7 | 1 | 1 | |||
Medical MS‐DRGs, % | 70 | 42 | 78 | 87 | |||
Most common MDCs | |||||||
Pregnancy related | 17 | 2 | 2 | 2 | |||
Cardiovascular | 10 | 10 | 7 | 13 | |||
Respiratory | 9 | 4 | 14 | 17 | |||
Gastrointestinal | 7 | 3 | 10 | 14 | |||
Hematologic | 1 | 2 | 9 | 6 | |||
Myeloproliferative | <1 | 4 | 9 | 6 | |||
CMS core condition | 7 | 3 | 6 | 12 | 0.174 | 0.01 | 0.004 |
Encounter diagnoses associated with hospitalizations were also significantly different between each of the high user subgroups (Table 2, P<0.001 for all). The high costlow admit subgroup was predominantly hospitalized for surgical conditions (58% vs 42% for medical MS‐DRGs) and had the lowest proportion of hospitalizations for CMS core conditions (3%). The most common types of surgical hospitalizations in this subgroup were for cardiovascular procedures (15%; including coronary artery bypass grafting and cardiac valve replacement) and orthopedic procedures (13%; including hip, knee, and other joint replacements). Most surgical hospitalizations were from referrals (67%) rather than admissions through the ED. In contrast, the low costhigh admit group was predominantly hospitalized for medical conditions (87% vs 13% for surgical MS‐DRGs) and had the highest proportion of hospitalizations for CMS core conditions (12%). The most common types of medical hospitalizations in this subgroup were for respiratory conditions (17%; including chronic obstructive pulmonary disease and pneumonia), gastrointestinal conditions (14%), and cardiovascular conditions (13%; including CHF, AMI, arrhythmia, and chest pain). High costhigh admit individuals were also hospitalized primarily for medical rather than surgical conditions (78% vs 22% medical vs surgical MS‐DRGs). Only 6% of hospitalizations in this subgroup were for CMS core conditions, and only 2% of hospitalizations were 30‐day readmissions for CMS core conditions.
The overlap between the high cost and high admit groups was persistently 48% or less for the 3 years prior to the study period (Table 3). Although the extent of overlap was similar across years, the absolute dollar value for the cutoff to define the top decile by hospital costs gradually increased over time from $47,000 in 2008 to $65,000 in 2011 (P<0.001 for trend). Among the high costlow admit subgroup, there was a trend toward a decrease in the proportion of surgical hospitalizations from 67% in 2008 to 58% in 2011 (P=0.09).
2008 | 2009 | 2010 | 2011 | P Value (For Linear Trend) | |
---|---|---|---|---|---|
| |||||
Study population | 2408 | 2518 | 2647 | 2566 | |
Characteristics, n | |||||
Cutoff for high cost (top decile), nearest $1000 | >47 | >51 | >54 | >65 | <0.001 |
Proportion of total hospital costs incurred by high cost group, % | 46 | 47 | 47 | 48 | |
Cutoff for high admit (top decile), no. of admissions | 3 | 3 | 3 | 3 | |
High cost who are also high admit, % | 42 | 48 | 48 | 48 | |
Discharge diagnoses by subgroup* | |||||
Non‐high user population | |||||
Surgical MS‐DRG | 32 (751) | 33 (842) | 36 (932) | 30 (751) | 0.51 |
Medical MS‐DRG | 68 (1598) | 67 (1676) | 64 (1673) | 70 (1749) | |
High costlow admit | |||||
Surgical MS‐DRG | 67 (138) | 68 (132) | 61 (120) | 58 (119) | 0.09 |
Medical MS‐DRG | 33(67) | 32 (63) | 39 (78) | 42 (87) | |
High costhigh admit | |||||
Surgical MS‐DRG | 23 (104) | 25 (133) | 24 (150) | 22 (134) | 0.60 |
Medical MS‐DRG | 77 (341) | 75 (392) | 76 (464) | 78 (471) | |
Low costhigh admit | |||||
Surgical MS‐DRG | 11 (35) | 17 (44) | 13 (40) | 13 (54) | 0.90 |
Medical MS‐DRG | 89 (277) | 83 (219) | 87 (269) | 87 (377) |
DISCUSSION
In this study, we found that only half of high cost individuals were also high admit. Further categorizing high users into high costlow admit versus high costhigh admit versus low costhigh admit identified distinct patterns between each group. High costhigh admit individuals were more likely to be hospitalized for medical conditions, whereas high costlow admit individuals were more likely to be hospitalized for surgical conditions. CMS core conditions accounted for a low proportion of overall hospitalizations across all groups.
Our findings suggest several distinct types of high users with different clinical characteristics, utilization, and cost patterns. From a hospital perspective, one implication is that a multifaceted approach to cost containment, rather than the one‐size‐fits‐all strategy of reducing hospitalizations, may be more effective in reducing costs. For example, our findings show that high costlow admit individuals have a disproportionate number of hospitalizations for surgical conditions, longer LOS, and more ICU days. Costs incurred by this subgroup may be more responsive to in‐hospital interventions aimed at reducing procedural costs, LOS, unnecessary use of the ICU, and minimizing postoperative infections and complications rather than to a care management approach.
In contrast, care management strategies such as improving postdischarge care and chronic disease management, which aim to achieve cost savings through reducing hospitalizations, may be more effective in reducing costs among high costhigh admit individuals, who have a high proportion of hospitalizations for medical conditions and the highest proportion of 30‐day readmissions. Such strategies may also be important in optimizing the quality of care for low costhigh admit individuals, who have the highest proportion of medical hospitalizations among all high users, though the potential for cost savings may be more limited in this subgroup.
Our results suggest that current hospital‐based approachesdriven by readmissions penalties for CMS core conditionsmay have less than the expected impact on costs. For example, although high costhigh admit individuals had the highest proportion of 30‐day readmissions, readmissions specifically for CMS core conditions accounted for <1% of costs in this subgroup. Thus, the potential return on an expensive investment in a care management intervention is unclear, given the small number of readmissions for these select conditions. From a broader perspective, the focus on readmissions for CMS core conditions, which overall contribute relatively little to high hospital costs, may not be a comprehensive enough strategy for cost containment. To date, there have been limited policies targeting factors contributing to high hospital costs outside of frequent medical hospitalizations. Medicare's nonpayment policy for treatment of preventable hospital conditions, including surgical site infections, translates prevention of these conditions into cost savings for hospitals.[30] However, this rule has been criticized for not going far enough to drive substantial savings.[31] A new CMS rule authorizes states to identify other provider‐preventable conditions for which Medicaid payment will be prohibited.[32] Future policy efforts should further emphasize a comprehensive, multipronged approach beyond readmissions penalties for select conditions if healthcare cost containment remains a policy priority.
Our results should be interpreted in light of several limitations. First, this was a single‐site study at an academic medical center; the generalizability of our results to other settings is unclear. Our cost data likely reflect local market factors, including the highest wage rates for skilled healthcare labor in the United States.[33] Although the explicit distribution of high user subgroups may be institution‐specific due to variations in our cost structure, we anticipate that the general classification paradigm will be similar in other health systems. Second, we captured utilization and costs only at a single hospital. However, our study population includes only individuals with PCPs at UCSF, and internal data from both Medicare and UCSF's largest private payer show that over 85% of hospitalizations among this population are to UCSF Medical Center. Third, we were able to capture only hospital costs rather than overall healthcare costs. Given that hospital costs account for the single largest category of total national health costs,[4] we expect that future studies examining total health costs will show similar findings. Fourth, our data did not include measures of health status, socioeconomic status, housing, or mental health comorbidities to permit an analysis of these factors, which have been previously related to frequent hospitalizations and high costs.[34, 35, 36, 37, 38, 39] Fifth, due to resource constraints, we were unable to conduct a longitudinal analysis to examine the extent to which individuals are consistently high users over time. Previous studies have described that 20% to 30% of individuals are consistently high users; the remainder have discrete periods of high utilization.[34, 40] This may be an important consideration in the design of future interventions.
Finally, our analysis was limited to individuals with a PCP to allow identification of an accessible cohort for care management. Thus, we did not capture individuals without a PCP and individuals who died during the study period, because these individuals no longer had an assigned PCP following death. Although this approach is consistent with that of many care management programs,[19] these populations are likely to incur higher than average utilization and healthcare costs, and represent important areas for future investigation.
In summary, our study identifies three types of high‐user populations that differ in the proportion of costs attributable to frequent hospitalizations, clinical conditions associated with hospital use, and frequency of 30‐day readmissions. Stratifying high users by both costs and hospitalizations may help identify tailored strategies to more effectively reduce costs and utilization.
Acknowledgments
The authors acknowledge Diana Patterson, Leanna Zaporozhets, and Andre Devito for their assistance in data collection.
Disclosures: Dr. Nguyen had full access to all of the data in the study and takes responsibility for the integrity of the data and the accuracy of the data analysis. Dr. Nguyen's work on this project was completed as a primary care research fellow at the University of California, San Francisco funded by a federal training grant from the National Research Service Award (NRSA T32HP19025‐07‐00).
Despite signs of a slowing trend,[1, 2, 3] US healthcare costs continue to rise, and cost containment remains a major area of concern. Hospital costs are the largest single category of national healthcare expenditures,[4] and the burden of cost containment is increasingly being shifted to hospitals.[5] As such, hospitals are increasingly focusing on implementing interventions to reduce rates of hospitalizations and readmissions as a mechanism to reduce overall healthcare costs.[5, 6, 7, 8, 9]
Multiple factors potentially contribute to patients being high cost, including acute care utilization,[10, 11, 12] pharmaceuticals,[13, 14] procedures,[15] catastrophic illness,[16] and high‐risk chronic conditions.[11, 17, 18] However, many hospitals are implementing interventions focused on a single subset of these high cost patientshigh users of inpatient services. Care management interventions have received particular attention, due to their perceived potential to improve quality of care while reducing costs through the mechanism of reducing hospital admissions.[19, 20, 21] Despite their increasing prevalence, there is limited evidence demonstrating the effectiveness of these programs. Among interventions targeting high cost individuals, the Medicare Care Management for High Cost Beneficiaries showed no effect on hospital admissions.[19] Another high‐profile intervention, the Citywide Care Management System led by the Camden Coalition, showed promising preliminary results, but data from a systematic evaluation are lacking.[22] Conversely, interventions targeting individuals with frequent hospitalizations have similarly shown mixed results in reducing costs.[6, 7, 9, 23]
Taken together, these data suggest that the relationship between high costs and frequent hospital use is complicated, and the population of individuals with frequent hospitalizations may not represent the entire population of high cost individuals. Thus, focusing on reduction of hospitalizations alone may be inadequate to reduce costs. For these reasons, we sought to determine how many high cost individuals would be captured by focusing only on frequently hospitalized (high admit) individuals by examining the overlap between these populations. We also sought to describe the characteristics and distinctions between the resulting subgroups of high users to better inform the design of future interventions.
METHODS
We examined the cross‐sectional relationship between high cost and high admit populations among adult patients 18 years of age hospitalized at the University of California, San Francisco (UCSF) Medical Center, a 660‐bed urban academic medical center from July 1, 2010 to June 30, 2011.
This study was conducted as part of a quality improvement project to identify high user primary care patients for complex care management. Individuals were included in the study if: (1) they had an assigned UCSF primary care provider (PCP), and (2) they had at least 1 hospitalization at UCSF during the study period. PCP assignments were ascertained from panel lists maintained by clinics; lists included individuals with at least 1 visit at any of the 8 primary care clinics at UCSF in the 2 years prior to the end of the study period. Because individuals are dropped from PCP panels at death, we were unable to ascertain or include individuals who died during the study period.
From the initial study population, we defined the high cost group as those who were in the top decile of total hospital costs, and the high admit group as those who were in the top decile of total hospitalizations during the study period. We elected to use the top decile as a cutoff given that it is a common operational definition used to identify high users to target for intervention.[24]
To examine the relationship between high cost and high admits we defined 3 further subgroups: high costlow admits, high costhigh admits, and low costhigh admits (Figure 1). To explore the face validity of these descriptors and classification scheme, we subsequently examined the proportion of total hospital costs and total hospitalizations each subgroup accounted for with respect to the study population.
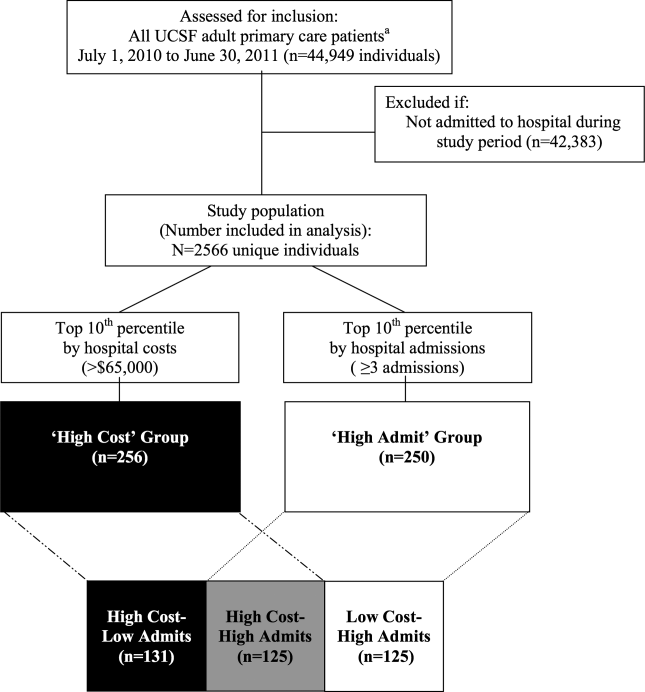
Data Sources
Hospital costs, demographic data, and encounter diagnoses were obtained from the hospital's Transition Systems Incorporated system (TSI, also known as Eclipsys or Allscripts), a commercially available automated cost accounting system that integrates multiple data sources to calculate total hospital costs on a per patient basis. Several studies have previously used the TSI system to estimate the costs of healthcare services at individual hospitals, and this approach is generally considered the most accurate method to estimate cost.[25, 26] Hospital costs included the sum of actual total costs (not billed charges) for all hospital episodes including lab costs, drug costs, surgical supplies, nurse salaries and benefits, utilities, housekeeping, and allocated administrative overhead. This cost total does not capture the cost of physician labor (pro‐fees), preadmission costs (e.g., outpatient care), or postadmission costs (e.g., home health, nursing home, or other postdischarge care). Preadmission lab, diagnostic tests, and imaging were included in hospital costs if these were ordered within 72 hours of hospital admission. Emergency department (ED) costs were included if an individual was admitted to the hospital via the ED. Hospitalizations were defined as inpatient admissions only to UCSF because we were unable to reliably ascertain hospitalizations outside of UCSF. PCP assignments were ascertained from administrative panel lists maintained by clinics.
Study Variables
We analyzed factors previously shown[13, 27, 28, 29] to be associated with high healthcare cost and utilization. We examined demographic characteristics and hospitalization characteristics, including admission source, length of stay (LOS), cost per hospitalization, whether the episode was a 30‐day readmission, days in the intensive care unit (ICU), and encounter diagnoses.
To ascertain whether a hospitalization was for a medical versus surgical condition, we used discharge diagnosis codes and designations as per the Medicare Severity Diagnosis‐Related Groups (MS‐DRG) versions 27 and 28 definitions manuals. We subsequently grouped medical and surgical conditions by Major Diagnostic Categories as per the MS‐DRG definitions manuals.
Using MS‐DRG codes, we also classified whether hospitalizations were for pneumonia, acute myocardial infarction (AMI), and congestive heart failure (CHF), as these 3 conditions have specific payment penalties under the Centers for Medicare & Medicaid Services (CMS) reimbursement policies. For these CMS core conditions, we included hospitalizations with MS‐DRG codes 193195, 280282, and 291293 (codes 283285 were not included for AMI because individuals who died during the study period were excluded.)
Analysis
We used descriptive statistics to compare patient and hospitalization characteristics between subgroups. Non‐normally distributed variables including LOS and cost per hospitalization were log transformed. Because a single individual could account for multiple hospitalizations, we performed a companion analysis of hospitalization characteristics using generalized estimating equations with an independent correlation structure to account for clustering of hospitalizations within individuals. Our findings were robust using either approach. For ease of interpretation, P values from the former analysis are presented.
To determine whether the overall distribution and characteristics we observed for high user subgroups were a single‐year anomaly or representative of temporally stable trends, we compared non‐high users and high user subgroup characteristics over the 3 years preceding the study period using linear regression for trend.
The institutional review board at UCSF approved this study protocol.
RESULTS
Of the 2566 unique individuals included in the analysis (Figure 1), 256 individuals were identified as high cost (top decile, $65,000). This group accounted for 45% of all costs and 22% of all hospitalizations (Figure 2). Two hundred fifty individuals were identified as high admits (top decile, 3 hospitalizations). This group accounted for 32% of all costs and 28% of all hospitalizations.
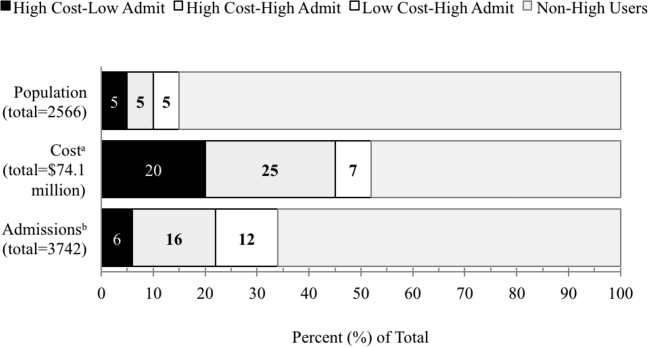
Only 48% of high cost individuals were also high admit ($65,000 and 3 hospitalizations; n=125, Figure 1). Among high users, we subsequently defined 3 subgroups based on the relationship between cost and hospitalizations (high costlow admits, high costhigh admits, and low costhigh admits). Each subgroup accounted for approximately 5% of the overall study population (Figure 2). The high costlow admits subgroup incurred a proportionate share of hospitalizations (6%) but a disproportionate share of costs (20%). The high costhigh admits subgroup had a disproportionate share of both costs (25%) and hospitalizations (16%). The low costhigh admits subgroup had a proportionate share of costs (7%) but a disproportionate share of hospitalizations (12%).
Patient and Hospitalization Characteristics
Compared to non‐high users, all high user subgroups were more likely to have public insurance (Medicare or Medicaid) or have dualeligible status, and the two high cost subgroups were more likely to be male and African American (P<0.05 for all). Compared to each other, subgroups were similar with respect to race/ethnicity, payer, and age (Table 1).
Non‐High Users,n=2145 | High User Subgroups | ||||||
---|---|---|---|---|---|---|---|
1 | 2 | 3 | P Value for Comparison | ||||
High CostLow Admit, n=131 | High CostHigh Admit, n=125 | Low CostHigh Admit, n=125 | 1 vs 2 | 2 vs 3 | 1 vs 3 | ||
| |||||||
Male, % | 34 | 57 | 42 | 38 | 0.02 | 0.52 | 0.003 |
Race/ethnicity, % | 0.15 | 0.76 | 0.18 | ||||
White | 45 | 46 | 36 | 38 | |||
Black | 14 | 21 | 26 | 19 | |||
Hispanic | 9 | 5 | 10 | 10 | |||
Asian | 22 | 18 | 22 | 26 | |||
Other | 10 | 10 | 6 | 6 | |||
Primary payer, % | 0.23 | 0.44 | 0.51 | ||||
Commercial | 42 | 24 | 20 | 17 | |||
Medicare | 42 | 58 | 55 | 61 | |||
Medicaid | 15 | 17 | 25 | 21 | |||
Other | <1 | 2 | 2 | ||||
Dual eligible, %* | 18 | 27 | 26 | 31 | 0.85 | 0.38 | 0.48 |
Age, mean yearsSD | 5720 | 6017 | 5817 | 6320 | 0.40 | 0.04 | 0.19 |
No. of hospitalizations per individual, median (interquartile range) | 1 (11) | 2 (12) | 4 (36) | 3 (34) | <0.001 | <0.001 | <0.001 |
Hospital costs per individual, median $1000 (interquartile range) | 12 (722) | 93 (75122) | 113 (85174) | 37 (3051) | <0.001 | <0.001 | <0.001 |
Regarding hospitalization characteristics, each high user subgroup was distinct and significantly different from each of the other subgroups with respect to admission source, proportion of 30‐day readmissions, LOS, and cost per hospitalization (Table 2, P<0.001 for all). The low costhigh admit subgroup had the highest proportion of admissions from the ED (73%), a moderate proportion of 30‐day readmissions (32%), the shortest LOS (median, 3 days; interquartile range [IQR], 24 days) and the lowest cost per hospitalization (median, $12,000; IQR, $8,000$15,000). In contrast, the high costlow admit subgroup had the highest proportion of admissions from clinic or physician referrals (45%), lowest proportion of 30‐day readmissions (17%), the longest LOS (median, 10; IQR, 417), the most ICU days per hospitalization (median, 1; range, 049) and the highest cost per hospitalization (median, $68,000; IQR, $43,000$95,000). High costhigh admit individuals had the highest proportion of 30‐day readmissions (47%) and a moderate cost per hospitalization (median, $28,000; IQR, $23,000$38,000), but the highest median cost per individual over 1 year ($113,000; IQR, $85,000$174,000, Table 1). Hospitalizations classified as 30‐day readmissions accounted for 42% of costs incurred by this subgroup; 30‐day readmissions specifically associated with CMS core conditions accounted for <1% of costs.
Non‐High Users | High User Subgroups | ||||||
---|---|---|---|---|---|---|---|
1 | 2 | 3 | P Value for Comparison | ||||
High CostLow Admit | High CostHigh Admit | Low CostHigh Admit | 1 vs 2 | 2 vs 3 | 1 vs 3 | ||
| |||||||
No. of admissions | 2500 | 206 | 605 | 431 | |||
Admission source, % | <0.001 | <0.001 | <0.001 | ||||
Emergency department | 53 | 50 | 65 | 73 | |||
Clinic or physician referral | 44 | 45 | 30 | 20 | |||
Transfer from outside facility | 2 | 5 | 4 | 4 | |||
Self‐referral | 1 | <1 | 1 | 3 | |||
Other | <1 | ||||||
30‐day readmission, % | 5 | 17 | 47 | 32 | <0.001 | <0.001 | <0.001 |
LOS, median days (IQR) | 3 (24) | 10 (417) | 5 (310) | 3 (24) | <0.001 | <0.001 | <0.001 |
ICU days, median (range)* | 0 (08) | 1 (049) | 0 (021) | 0 (03) | <0.001 | <0.001 | <0.001 |
Cost per hospitalization, median $1,000 (IQR) | 11 (719) | 68 (4395) | 28 (2338) | 12 (815) | <0.001 | <0.001 | <0.001 |
Encounter diagnoses | <0.001 | 0.002 | <0.001 | ||||
Surgical MS‐DRGs, % | 30 | 58 | 22 | 13 | |||
Most common MDCs | |||||||
Cardiovascular | 4 | 15 | 8 | 6 | |||
Orthopedic | 10 | 13 | 6 | 4 | |||
Transplant | <1 | 7 | 1 | 1 | |||
Medical MS‐DRGs, % | 70 | 42 | 78 | 87 | |||
Most common MDCs | |||||||
Pregnancy related | 17 | 2 | 2 | 2 | |||
Cardiovascular | 10 | 10 | 7 | 13 | |||
Respiratory | 9 | 4 | 14 | 17 | |||
Gastrointestinal | 7 | 3 | 10 | 14 | |||
Hematologic | 1 | 2 | 9 | 6 | |||
Myeloproliferative | <1 | 4 | 9 | 6 | |||
CMS core condition | 7 | 3 | 6 | 12 | 0.174 | 0.01 | 0.004 |
Encounter diagnoses associated with hospitalizations were also significantly different between each of the high user subgroups (Table 2, P<0.001 for all). The high costlow admit subgroup was predominantly hospitalized for surgical conditions (58% vs 42% for medical MS‐DRGs) and had the lowest proportion of hospitalizations for CMS core conditions (3%). The most common types of surgical hospitalizations in this subgroup were for cardiovascular procedures (15%; including coronary artery bypass grafting and cardiac valve replacement) and orthopedic procedures (13%; including hip, knee, and other joint replacements). Most surgical hospitalizations were from referrals (67%) rather than admissions through the ED. In contrast, the low costhigh admit group was predominantly hospitalized for medical conditions (87% vs 13% for surgical MS‐DRGs) and had the highest proportion of hospitalizations for CMS core conditions (12%). The most common types of medical hospitalizations in this subgroup were for respiratory conditions (17%; including chronic obstructive pulmonary disease and pneumonia), gastrointestinal conditions (14%), and cardiovascular conditions (13%; including CHF, AMI, arrhythmia, and chest pain). High costhigh admit individuals were also hospitalized primarily for medical rather than surgical conditions (78% vs 22% medical vs surgical MS‐DRGs). Only 6% of hospitalizations in this subgroup were for CMS core conditions, and only 2% of hospitalizations were 30‐day readmissions for CMS core conditions.
The overlap between the high cost and high admit groups was persistently 48% or less for the 3 years prior to the study period (Table 3). Although the extent of overlap was similar across years, the absolute dollar value for the cutoff to define the top decile by hospital costs gradually increased over time from $47,000 in 2008 to $65,000 in 2011 (P<0.001 for trend). Among the high costlow admit subgroup, there was a trend toward a decrease in the proportion of surgical hospitalizations from 67% in 2008 to 58% in 2011 (P=0.09).
2008 | 2009 | 2010 | 2011 | P Value (For Linear Trend) | |
---|---|---|---|---|---|
| |||||
Study population | 2408 | 2518 | 2647 | 2566 | |
Characteristics, n | |||||
Cutoff for high cost (top decile), nearest $1000 | >47 | >51 | >54 | >65 | <0.001 |
Proportion of total hospital costs incurred by high cost group, % | 46 | 47 | 47 | 48 | |
Cutoff for high admit (top decile), no. of admissions | 3 | 3 | 3 | 3 | |
High cost who are also high admit, % | 42 | 48 | 48 | 48 | |
Discharge diagnoses by subgroup* | |||||
Non‐high user population | |||||
Surgical MS‐DRG | 32 (751) | 33 (842) | 36 (932) | 30 (751) | 0.51 |
Medical MS‐DRG | 68 (1598) | 67 (1676) | 64 (1673) | 70 (1749) | |
High costlow admit | |||||
Surgical MS‐DRG | 67 (138) | 68 (132) | 61 (120) | 58 (119) | 0.09 |
Medical MS‐DRG | 33(67) | 32 (63) | 39 (78) | 42 (87) | |
High costhigh admit | |||||
Surgical MS‐DRG | 23 (104) | 25 (133) | 24 (150) | 22 (134) | 0.60 |
Medical MS‐DRG | 77 (341) | 75 (392) | 76 (464) | 78 (471) | |
Low costhigh admit | |||||
Surgical MS‐DRG | 11 (35) | 17 (44) | 13 (40) | 13 (54) | 0.90 |
Medical MS‐DRG | 89 (277) | 83 (219) | 87 (269) | 87 (377) |
DISCUSSION
In this study, we found that only half of high cost individuals were also high admit. Further categorizing high users into high costlow admit versus high costhigh admit versus low costhigh admit identified distinct patterns between each group. High costhigh admit individuals were more likely to be hospitalized for medical conditions, whereas high costlow admit individuals were more likely to be hospitalized for surgical conditions. CMS core conditions accounted for a low proportion of overall hospitalizations across all groups.
Our findings suggest several distinct types of high users with different clinical characteristics, utilization, and cost patterns. From a hospital perspective, one implication is that a multifaceted approach to cost containment, rather than the one‐size‐fits‐all strategy of reducing hospitalizations, may be more effective in reducing costs. For example, our findings show that high costlow admit individuals have a disproportionate number of hospitalizations for surgical conditions, longer LOS, and more ICU days. Costs incurred by this subgroup may be more responsive to in‐hospital interventions aimed at reducing procedural costs, LOS, unnecessary use of the ICU, and minimizing postoperative infections and complications rather than to a care management approach.
In contrast, care management strategies such as improving postdischarge care and chronic disease management, which aim to achieve cost savings through reducing hospitalizations, may be more effective in reducing costs among high costhigh admit individuals, who have a high proportion of hospitalizations for medical conditions and the highest proportion of 30‐day readmissions. Such strategies may also be important in optimizing the quality of care for low costhigh admit individuals, who have the highest proportion of medical hospitalizations among all high users, though the potential for cost savings may be more limited in this subgroup.
Our results suggest that current hospital‐based approachesdriven by readmissions penalties for CMS core conditionsmay have less than the expected impact on costs. For example, although high costhigh admit individuals had the highest proportion of 30‐day readmissions, readmissions specifically for CMS core conditions accounted for <1% of costs in this subgroup. Thus, the potential return on an expensive investment in a care management intervention is unclear, given the small number of readmissions for these select conditions. From a broader perspective, the focus on readmissions for CMS core conditions, which overall contribute relatively little to high hospital costs, may not be a comprehensive enough strategy for cost containment. To date, there have been limited policies targeting factors contributing to high hospital costs outside of frequent medical hospitalizations. Medicare's nonpayment policy for treatment of preventable hospital conditions, including surgical site infections, translates prevention of these conditions into cost savings for hospitals.[30] However, this rule has been criticized for not going far enough to drive substantial savings.[31] A new CMS rule authorizes states to identify other provider‐preventable conditions for which Medicaid payment will be prohibited.[32] Future policy efforts should further emphasize a comprehensive, multipronged approach beyond readmissions penalties for select conditions if healthcare cost containment remains a policy priority.
Our results should be interpreted in light of several limitations. First, this was a single‐site study at an academic medical center; the generalizability of our results to other settings is unclear. Our cost data likely reflect local market factors, including the highest wage rates for skilled healthcare labor in the United States.[33] Although the explicit distribution of high user subgroups may be institution‐specific due to variations in our cost structure, we anticipate that the general classification paradigm will be similar in other health systems. Second, we captured utilization and costs only at a single hospital. However, our study population includes only individuals with PCPs at UCSF, and internal data from both Medicare and UCSF's largest private payer show that over 85% of hospitalizations among this population are to UCSF Medical Center. Third, we were able to capture only hospital costs rather than overall healthcare costs. Given that hospital costs account for the single largest category of total national health costs,[4] we expect that future studies examining total health costs will show similar findings. Fourth, our data did not include measures of health status, socioeconomic status, housing, or mental health comorbidities to permit an analysis of these factors, which have been previously related to frequent hospitalizations and high costs.[34, 35, 36, 37, 38, 39] Fifth, due to resource constraints, we were unable to conduct a longitudinal analysis to examine the extent to which individuals are consistently high users over time. Previous studies have described that 20% to 30% of individuals are consistently high users; the remainder have discrete periods of high utilization.[34, 40] This may be an important consideration in the design of future interventions.
Finally, our analysis was limited to individuals with a PCP to allow identification of an accessible cohort for care management. Thus, we did not capture individuals without a PCP and individuals who died during the study period, because these individuals no longer had an assigned PCP following death. Although this approach is consistent with that of many care management programs,[19] these populations are likely to incur higher than average utilization and healthcare costs, and represent important areas for future investigation.
In summary, our study identifies three types of high‐user populations that differ in the proportion of costs attributable to frequent hospitalizations, clinical conditions associated with hospital use, and frequency of 30‐day readmissions. Stratifying high users by both costs and hospitalizations may help identify tailored strategies to more effectively reduce costs and utilization.
Acknowledgments
The authors acknowledge Diana Patterson, Leanna Zaporozhets, and Andre Devito for their assistance in data collection.
Disclosures: Dr. Nguyen had full access to all of the data in the study and takes responsibility for the integrity of the data and the accuracy of the data analysis. Dr. Nguyen's work on this project was completed as a primary care research fellow at the University of California, San Francisco funded by a federal training grant from the National Research Service Award (NRSA T32HP19025‐07‐00).
- National Health Expenditure Accounts Team. Recession contributes to slowest annual rate of increase in health spending in five decades. Health Aff (Millwood). 2011;30(1):11–22. , , , ;
- Growth in US health spending remained slow in 2010; health share of gross domestic product was unchanged from 2009. Health Aff (Millwood). 2012;31(1):208–219. , , , .
- the National Health Expenditure Accounts Team. National health spending in 2011: overall growth remains low, but some payers and services show signs of acceleration. Health Aff (Millwood). 2013;32(1):87–99. , , , ;
- Centers for Medicare and Medicaid Services; Office of the Actuary; National Health Statistics Group. National healthcare expenditures data. 2012. Available at: https://www.cms.gov/Research‐Statistics‐Data‐and‐Systems/Statistics‐Trends‐and‐Reports/NationalHealthExpendData/downloads/tables.pdf.
- Medicare Payment Advisory Commission. Report to the Congress: Promoting Greater Efficiency in Medicare. Washington, DC: Medicare Payment Advisory Commission; 2007.
- The care transitions intervention: results of a randomized controlled trial. Arch Intern Med. 2006;166(17):1822–1828. , , , .
- A reengineered hospital discharge program to decrease rehospitalization: a randomized trial. Ann Intern Med. 2009;150(3):178–187. , , , et al.
- How‐to Guide: Improving Transitions from the Hospital to the Clinical Office Practice to Reduce Avoidable Rehospitalizations. Cambridge, MA: Institute for Healthcare Improvement; 2012. , , , .
- Comprehensive discharge planning and home follow‐up of hospitalized elders: a randomized clinical trial. JAMA. 1999;281(7):613–620. , , , et al.
- High‐cost users of medical care. N Engl J Med. 1980;302(18):996–1002. , .
- Frequency and clinical description of high‐cost patients in 17 acute‐care hospitals. N Engl J Med. 1979;300(23):1306–1309. , , .
- Effectiveness of interventions targeting frequent users of emergency departments: a systematic review. Ann Emerg Med. 2011;58(1):41–52.e42. , , , et al.
- Medication, diagnostic, and cost information as predictors of high‐risk patients in need of care management. Am J Manag Care. 2009;15(1):41–48. , , , .
- Tracking health care costs: trends stabilize but remain high in 2002. Health Aff (Millwood). 2003;Suppl Web Exclusives:W3–266–274. , .
- An iconoclastic view of health cost containment. Health Aff (Millwood). 1993;12(suppl):152–171. .
- Comparisons of medical utilizations and categorical diagnoses of emergency visits between the elderly with catastrophic illness certificates and those without. BMC Health Serv Res. 2013;13:152. , , , et al.
- Finding future high‐cost cases: comparing prior cost versus diagnosis‐based methods. Health Serv Res. 2001;36(6 pt 2):194–206. , , , .
- Spending and service use among people with the fifteen most costly medical conditions, 1997. Health Aff (Millwood). 2003;22(2):129–138. , .
- Lessons from Medicare's Demonstration Projects on Disease Management and Care Coordination. Washington, D.C.: Congressional Budget Office; 2012. .
- The hot spotters. The New Yorker. January 24, 2011. .
- Collaboration Across the Disciplines in Health Care. Burlington, MA: Jones and Bartlett; 2010. , , .
- Hope for New Jersey's city hospitals: the Camden Initiative. Perspect Health Inf Manag. 2010:7:1d. , , .
- Hospital‐initiated transitional care interventions as a patient safety strategy: a systematic review. Ann Intern Med. 2013;158(5 pt 2):433–440. , , , , , .
- Agency for Healthcare Research and Quality. The concentration and persistence in the level of health expenditures over time: estimates for the U.S. population, 2008–2009. 2012. Available at: http://meps.ahrq.gov/mepsweb/data_files/publications/st354/stat354.shtml.
- The use of the transition cost accounting system in health services research. Cost Eff Resour Alloc. 2007;5:11. , , , , , .
- Cost reduction and quality improvement: it takes two to tango. Crit Care Med. 2000;28(2):581–583. , .
- Case finding for patients at risk of readmission to hospital: development of algorithm to identify high risk patients. BMJ. 2006;333(7563):327. , , , .
- Risk prediction models for hospital readmission: a systematic review. JAMA. 2011;306(15):1688–1698. , , , et al.
- Chronic disease score as a predictor of hospitalization. Epidemiology. 2002;13(3):340–346. , , , et al.
- Centers for Medicare and Medicaid Services. Hospital‐acquired conditions (HAC) in acute inpatient prospective payment system (IPPS) hospitals fact sheet. 2011. Available at: https://www.cms.gov/Medicare/Medicare‐Fee‐for‐Service‐Payment/HospitalAcqCond/downloads/HACFactsheet.pdf. Accessed August 31, 2013.
- Medicare's policy not to pay for treating hospital‐acquired conditions: the impact. Health Aff (Millwood). 2009;28(5):1485–1493. , , .
- Department of Health and Human Services, Centers for Medicare 2013.
- Consistently high users of medical care among the elderly. Med Care. 1990;28(6):527–540. , , , .
- Utilization patterns among long‐term enrollees in a prepaid group practice health maintenance organization. Med Care. 1985;23(11):1221–1233. , , , .
- Depressive symptoms and health service utilization among the community elderly. J Am Geriatr Soc. 1983;31(7):417–420. , , .
- The influence of socio‐economic status and multimorbidity patterns on healthcare costs: a six‐year follow‐up under a universal healthcare system. Int J Equity Health. 2013;12(1):69. , .
- Association between the level of housing instability, economic standing and health care access: a meta‐regression. J Health Care Poor Underserved. 2008;19(4):1212–1228. , , .
- High health care utilization and costs associated with lower socio‐economic status: results from a linked dataset. Can J Public Health. 2009;100(3):180–183. , , , .
- An analysis of the use of Medicare services by the continuously enrolled aged. Med Care. 1983;21(6):567–585. , .
- National Health Expenditure Accounts Team. Recession contributes to slowest annual rate of increase in health spending in five decades. Health Aff (Millwood). 2011;30(1):11–22. , , , ;
- Growth in US health spending remained slow in 2010; health share of gross domestic product was unchanged from 2009. Health Aff (Millwood). 2012;31(1):208–219. , , , .
- the National Health Expenditure Accounts Team. National health spending in 2011: overall growth remains low, but some payers and services show signs of acceleration. Health Aff (Millwood). 2013;32(1):87–99. , , , ;
- Centers for Medicare and Medicaid Services; Office of the Actuary; National Health Statistics Group. National healthcare expenditures data. 2012. Available at: https://www.cms.gov/Research‐Statistics‐Data‐and‐Systems/Statistics‐Trends‐and‐Reports/NationalHealthExpendData/downloads/tables.pdf.
- Medicare Payment Advisory Commission. Report to the Congress: Promoting Greater Efficiency in Medicare. Washington, DC: Medicare Payment Advisory Commission; 2007.
- The care transitions intervention: results of a randomized controlled trial. Arch Intern Med. 2006;166(17):1822–1828. , , , .
- A reengineered hospital discharge program to decrease rehospitalization: a randomized trial. Ann Intern Med. 2009;150(3):178–187. , , , et al.
- How‐to Guide: Improving Transitions from the Hospital to the Clinical Office Practice to Reduce Avoidable Rehospitalizations. Cambridge, MA: Institute for Healthcare Improvement; 2012. , , , .
- Comprehensive discharge planning and home follow‐up of hospitalized elders: a randomized clinical trial. JAMA. 1999;281(7):613–620. , , , et al.
- High‐cost users of medical care. N Engl J Med. 1980;302(18):996–1002. , .
- Frequency and clinical description of high‐cost patients in 17 acute‐care hospitals. N Engl J Med. 1979;300(23):1306–1309. , , .
- Effectiveness of interventions targeting frequent users of emergency departments: a systematic review. Ann Emerg Med. 2011;58(1):41–52.e42. , , , et al.
- Medication, diagnostic, and cost information as predictors of high‐risk patients in need of care management. Am J Manag Care. 2009;15(1):41–48. , , , .
- Tracking health care costs: trends stabilize but remain high in 2002. Health Aff (Millwood). 2003;Suppl Web Exclusives:W3–266–274. , .
- An iconoclastic view of health cost containment. Health Aff (Millwood). 1993;12(suppl):152–171. .
- Comparisons of medical utilizations and categorical diagnoses of emergency visits between the elderly with catastrophic illness certificates and those without. BMC Health Serv Res. 2013;13:152. , , , et al.
- Finding future high‐cost cases: comparing prior cost versus diagnosis‐based methods. Health Serv Res. 2001;36(6 pt 2):194–206. , , , .
- Spending and service use among people with the fifteen most costly medical conditions, 1997. Health Aff (Millwood). 2003;22(2):129–138. , .
- Lessons from Medicare's Demonstration Projects on Disease Management and Care Coordination. Washington, D.C.: Congressional Budget Office; 2012. .
- The hot spotters. The New Yorker. January 24, 2011. .
- Collaboration Across the Disciplines in Health Care. Burlington, MA: Jones and Bartlett; 2010. , , .
- Hope for New Jersey's city hospitals: the Camden Initiative. Perspect Health Inf Manag. 2010:7:1d. , , .
- Hospital‐initiated transitional care interventions as a patient safety strategy: a systematic review. Ann Intern Med. 2013;158(5 pt 2):433–440. , , , , , .
- Agency for Healthcare Research and Quality. The concentration and persistence in the level of health expenditures over time: estimates for the U.S. population, 2008–2009. 2012. Available at: http://meps.ahrq.gov/mepsweb/data_files/publications/st354/stat354.shtml.
- The use of the transition cost accounting system in health services research. Cost Eff Resour Alloc. 2007;5:11. , , , , , .
- Cost reduction and quality improvement: it takes two to tango. Crit Care Med. 2000;28(2):581–583. , .
- Case finding for patients at risk of readmission to hospital: development of algorithm to identify high risk patients. BMJ. 2006;333(7563):327. , , , .
- Risk prediction models for hospital readmission: a systematic review. JAMA. 2011;306(15):1688–1698. , , , et al.
- Chronic disease score as a predictor of hospitalization. Epidemiology. 2002;13(3):340–346. , , , et al.
- Centers for Medicare and Medicaid Services. Hospital‐acquired conditions (HAC) in acute inpatient prospective payment system (IPPS) hospitals fact sheet. 2011. Available at: https://www.cms.gov/Medicare/Medicare‐Fee‐for‐Service‐Payment/HospitalAcqCond/downloads/HACFactsheet.pdf. Accessed August 31, 2013.
- Medicare's policy not to pay for treating hospital‐acquired conditions: the impact. Health Aff (Millwood). 2009;28(5):1485–1493. , , .
- Department of Health and Human Services, Centers for Medicare 2013.
- Consistently high users of medical care among the elderly. Med Care. 1990;28(6):527–540. , , , .
- Utilization patterns among long‐term enrollees in a prepaid group practice health maintenance organization. Med Care. 1985;23(11):1221–1233. , , , .
- Depressive symptoms and health service utilization among the community elderly. J Am Geriatr Soc. 1983;31(7):417–420. , , .
- The influence of socio‐economic status and multimorbidity patterns on healthcare costs: a six‐year follow‐up under a universal healthcare system. Int J Equity Health. 2013;12(1):69. , .
- Association between the level of housing instability, economic standing and health care access: a meta‐regression. J Health Care Poor Underserved. 2008;19(4):1212–1228. , , .
- High health care utilization and costs associated with lower socio‐economic status: results from a linked dataset. Can J Public Health. 2009;100(3):180–183. , , , .
- An analysis of the use of Medicare services by the continuously enrolled aged. Med Care. 1983;21(6):567–585. , .
© 2013 Society of Hospital Medicine
Balancing acts: Deciding for or against antibiotics in acute respiratory infections
- Keep in mind that patients may not distinguish antibiotics from other forms of prescription cold remedies.
- When patients ask for antibiotics, they really may be seeking reassurance and effective symptom management.
- Consider using negotiation strategies to delay antibiotic prescribing.
Patient, clinician, and system factors influence unnecessary antibiotic prescribing for viral acute respiratory infections (ARIs). When patients seek care for ARIs, they use a variety of tactics to obtain antibiotic prescriptions.1 Both clinicians and patients tend to overemphasize the importance of purulent secretions, whether from the nose or lung, in deciding whether an antibiotic is needed.2-4
Antibiotic prescribing increases with clinician age5 and number of years in practice,6 and antibiotics are more likely to be prescribed for ARIs in urban5 and nonteaching7,8 practice settings.
In addition, clinicians’ perceptions that patients or parents expect antibiotics are often inaccurate. They frequently assume that patients are dissatisfied when they do not receive antibiotic prescriptions for ARIs; however, accurate explanations and reassurance, not antibiotic prescriptions, have been shown to increase patient satisfaction.9,10
These factors, though known to be associated with excessive antibiotic prescribing, do not explain how clinicians actually make decisions to give antibiotics for ARIs. Thus, we applied grounded theory—a qualitative research method used to examine social processes—to provide insight into the antibiotic prescribing process.11
Methods
Setting
Our study was conducted in a rural Western community with a population of 32,014, served by 24 primary care clinicians.
Sample
We aimed to interview all of the community’s 24 primary care clinicians. Each clinician was mailed information about the study and then received a follow-up phone call to arrange an interview. Three clinicians were not interviewed due to scheduling conflicts.
The median age of the participants was 43 years with a range of 32 to 58. Nine subjects (43%) were women. Four subjects were nurse practitioners (NPs) and 17 were MDs. Areas of practice included internal medicine (n=5), college health (n=5), family medicine (n=4), pediatrics (n=4), and emergency medicine (n=3). No clinicians were representative of minority ethnic groups.
Interviews
We gathered data in audiotaped, semi-structured interviews. Hart, a practicing NP in the study community who received doctoral training in qualitative research, interviewed the 21 clinicians. The interviews ranged from 30 to 120 minutes with an average of 1 hour.
The clinician was asked to describe how he or she decided when patients presenting with ARIs should receive antibiotics. They were also asked to describe situations in which they would “most definitely” or “definitely not” prescribe antibiotics and situations that caused uncertainty. They were also asked to describe challenges associated with ARI management.
Data analysis
Hart analyzed the interviews using the constant comparative method, a form of qualitative analysis whereby each unit of data is compared with previously identified units.11 Each line of transcribed interview data was analyzed chronologically and coded for patterns, themes, and processes. The resulting codes were then compared for similarities and differences. Categories and “families” of categories were developed. This process continued until the basic social process, or one phenomenon central to each interview, was discovered. The findings were then discussed and refined by members of the research team, consisting of Pepper and Gonzales, as well as 3 PhD-prepared researchers with expertise in qualitative research.
Credibility
A recognized method for establishing credibility in qualitative research is through the use of “member checks,” wherein findings are presented to the original study participants for confirmation and clarification.12 A group member check was obtained during a “Grand Rounds” presentation at the community hospital. Thirty-one people attended, including 9 of the original participants. During a forum following the presentation, study participants confirmed that the findings accurately described their antibiotic prescribing processes.
Results
Two main concepts emerged from the interviews: individual best practice and perceived patient/parent satisfaction. These concepts are discussed using illustrative quotes from actual interviews.
Individual best practice describes how each clinician was attempting to do what he or she believed to be clinically “best” for the patient presenting with ARI symptoms. Clinicians differed in their approaches to patients presenting with ARIs. Some prescribed antibiotics for non-specific upper respiratory infection symptoms. Others were reluctant to prescribe antibiotics unless strict diagnostic criteria were met. Ultimately, each clinician made a decision based on what he or she believed was best for the patient. What defined “best” was clinician-specific. Though “best practice” always derived from evidence that informed the individual’s practice, it should not be confused with the term “best research evidence,” as defined by Straus et al.13 Five categories influenced the concept of individual best practice (TABLE).
Types of evidence used/valued to inform practice was the most influential of the 5 categories. Twelve clinicians indicated that their main source of clinical evidence was current research-based findings and related practice guidelines. The other 9 based their clinical practice on other forms of evidence, including personal and professional observations or experiences; discussions with pharmaceutical representatives; discussions with colleagues; and experiences reported by patients. Regarding personal experiences, one clinician said, “We’ll talk about my personal experiences…. Just like you have yours, I have mine and they don’t just include patients that I see. They include family members and people that I live with who’ve had an infection for 5 days—I give them an antibiotic. Two days later, they are up and about…so my empiric evidence over and over again reinforces that antibiotics are great. They work.”
Perceived patient/parent satisfaction, the second concept, describes how clinicians attempted to satisfy patients or parents according to their own perceptions of the patients’ potential to be satisfied. The 4 categories influencing the concept of perceived patient satisfaction are shown at the bottom of the TABLE.
Business concerns was the most interesting category. All 21 clinicians described the impact of patient satisfaction on the financial aspects of their practices. The 16 clinicians who collected on a fee-for-service basis described patient satisfaction as an important aspect of their livelihood as illustrated in the following comment: “You shouldn’t be treating all respiratory infections with antibiotics? Certainly. Is it practical? Probably not. I probably wouldn’t have as good of a collection rate. I truly think that part of what you’re doing is consumer-based medicine.”
Conversely, the 5 clinicians who were salaried and who did not charge on a fee-for-service basis indicated that, from a business standpoint, they were not as concerned about patient satisfaction. One clinician stated, “We can practice the better standards because of the cost elements…. You know I would feel very put upon if I had to charge every time I saw someone.”
Balancing acts. Further analysis revealed that the basic social process common to all participants was balancing acts—a process whereby clinicians weigh individual best practice against perceived patient satisfaction. Each clinician had ideas about what constituted best practice; however, each was also concerned about maintaining good patient relationships and often saw these 2 concepts at odds. Every decision that included individual best practice information was adjusted for perceived patient satisfaction. As one clinician aptly commented, “I always debate. I do a lot of mental hand wringing.”
Four types of balancing acts were identified: (a) education, (b) negotiation, (c) giving in, and (d) holding firm.
- By educating patients about the data informing their decision to not prescribe antibiotics, clinicians offered that they could often increase perceived patient satisfaction and successfully refrain from prescribing antibiotics: “Eighty percent of the time, if I explain to them about the study where they actually punctured the maxillary sinuses and cultured what they brought out and you know 90% of them did not grow out—they’re shocked, and lots of time, they’ll say, “Why didn’t my doctor ever tell me this?”
- Negotiation was another strategy identified for increasing perceived patient satisfaction. Every clinician described discussions and prescriptions regarding symptom management as a negotiation strategy for increasing patient satisfaction. Eleven clinicians described how they would negotiate with the patient about what to do if symptoms did not improve: “So I give them a specific date…and I say, “Well, let’s give it a week—if it’s not any better then call and if there are no new symptoms, then I’ll call something (an antibiotic) in for it.”
- All of the clinicians reported situations where education and negotiation failed to work, or where the patient seemed so unconvincible that attempt seemed futile. In these situations, clinicians found themselves giving in—ie, abandoning individual best practice to salvage perceived patient satisfaction. One example: “Well in some cases, when someone seems so persistent, I will say, ‘Look, I’ve been able to tell you how I feel and what I think about what’s going on with you. If you are still adamant that you need an antibiotic, then fine, if you’re going to go somewhere else to get an antibiotic, I will prescribe an antibiotic for you.’”
- The last balancing act described by all but 1 of the clinicians was holding firm to their ideas regarding individual best practice, regardless of perceived patient satisfaction: “Once in awhile, I just kind of have to say, ‘You know, I don’t think antibiotics are necessary. I just don’t want to do that.’”
TABLE
The balancing act: Individual best practice vs patient satisfaction
FACTORS INFLUENCING INDIVIDUAL BEST PRACTICE |
Initial training |
Types of evidence used/valued to inform practice (eg, current research-based evidence, clinical experience) |
Familiarity with evidence-based practice |
Interaction with peers |
Clinical uncertainty |
FACTORS INFLUENCING PERCEIVED PATIENT SATISFACTION |
Patient/parent concerns |
Day care and school concerns |
Unique patient situations |
Business concerns |
Discussion
Though individual best practice has not been referred to as such, it is by no means a novel idea and is at the core of the evidence-based practice debate, wherein evidence obtained from research is often viewed as being at odds with clinician experience.14-16 However, our study also revealed that some clinicians were unfamiliar with the research-based evidence they claimed to use. For example, 2 clinicians who claimed familiarity with research-based evidence indicated they would strongly consider prescribing antibiotics for a productive cough regardless of other historical or exam findings.
Perceived patient satisfaction has received much attention in and out of the “antibiotic” literature for its powerful influence on clinical decision-making.1,17-19 Our findings further this concept by identifying several of the factors that influence it, including patient-driven (eg, unique patient situations) and clinician-driven (eg, business concerns).
Though balancing acts is a new term, 3 of the 4 types of balancing acts have been alluded to in the antibiotic literature. Several studies have demonstrated that patient education can decrease antibiotic prescribing and use.10,20,21 Similarly patient negotiation, through the use of delayed antibiotic prescribing or a contingency plan for delayed prescribing, has also decreased antibiotic prescribing22,23 and increased patient satisfaction.24 Furthermore, it is well documented that the act of giving in to perceived patient desires for antibiotics occurs frequently.1,10,25
Holding firm was the only balancing act not paralleled in the literature. This likely stems from the fact that most research efforts have focused on situations where antibiotics were inappropriately prescribed, as opposed to situations where they were appropriately withheld. However, now that there is evidence of a decrease in antibiotic use for ARIs,26,27 it is likely that holding firm is occurring more often.
Study strengths and limitations
Interviewing both physicians and nurse practitioners increases our study’s overall applicability. Moreover, the fact that our participants practiced in the same close-knit community increased the likelihood that they were dealing with a consistent patient population.
One facet that serves as both a strength and limitation is that the main investigator (Hart) worked as a nurse practitioner in the community of study. Her experience treating patients with ARIs and her role as a community clinician undoubtedly helped her gain access to the participants, but being an “inside” investigator might have caused participants to be less candid than they would have been with an unknown investigator.
Our study relied solely on information gleaned from participant interviews; thus it is possible that some of the participants described their antibiotic prescribing processes differently than how the processes actually work during clinical encounters.
Finally, our findings represent actual descriptions of clinicians’ practices. Balancing acts and related concepts should not be confused with our beliefs about “ideal” clinical decision-making.
Implications
The balancing acts process and related concepts have several implications for clinician education and practice. Regarding individual best practice, we need to recognize that clinicians make decisions based on many different forms of evidence, including but not limited to research-based evidence, outdated or incorrect sources of literature, and personal and professional experiences. They also may be most comfortable practicing in the manner and style they were exposed to in their initial training experiences. Thus, in addition to exposing clinicians to research-based evidence, we need to teach them how to integrate research-based evidence into their practices, as well as how to deal with research findings that seem to conflict with their own observations or primary training experiences. Recognizing and using local peer influences is one way to support this concept and has been shown to be an effective strategy.28
Furthermore, we should not underestimate the impact of perceived patient satisfaction on clinician decision-making. Clinicians need to understand patient satisfaction and how it influences their practices. They need to appreciate that their own perceptions of patient desires may be inaccurate.29,30 They also need to be aware that studies have shown no relationship between antibiotic prescribing and patient satisfaction,30-32 and that patients are most satisfied when clinicians spend time with them, respect their symptoms, and honestly address their concerns.33-35
Clinicians should also appreciate that patients may not distinguish antibiotics from other forms of prescription cold remedies and that when they ask for antibiotics, they really may be seeking reassurance and effective symptom management.36 Furthermore, they need to be aware of and comfortable using various patient education, comfort, and negotiation strategies, such as symptom management and delayed antibiotic prescribing. Professional education that includes examples of realistic clinician/patient scenarios might be helpful with this.
Acknowledgments
This study was funded by the National Institutes of Health, National Institute for Nursing Research grant # NR07531-02.
CORRESPONDENCE
Ann Marie Hart, PhD, FNP, Assistant Professor, University of Wyoming, Fay W. Whitney School of Nursing, Department 3065, 1000 E. University Ave, Laramie, WY 82071. E-mail: [email protected]
1. Scott JG, Cohen D, DiCicco-Boom B, Orzano AJ, Jaén CR, Crabtree BF. Antibiotic use in acute respiratory infections and the ways patients pressure physicians for a prescription. J Fam Pract 2001;50:853-858.
2. Dosh SA, Hickner JM, Mainous AG, Ebell MH. Predictors of antibiotic prescribing for nonspecific upper respiratory infections, acute bronchitis, and acute sinusitis. J Fam Pract 2000;49:407-414.
3. Watson RL, Dowell SF, Jayaraman M, Keyserling H, Kolczak M, Schwartz B. Antimicrobial use for pediatric upper respiratory infections: reported practice, actual practice, and parent beliefs. Pediatrics 1999;104:1251-1257.
4. Gonzales R, Steiner JR, Lum A, Barrett PH. Decreasing antibiotic use in ambulatory practice: impact of a multidimensional intervention on the treatment of uncomplicated acute bronchitis in adults. JAMA 1999;281:1512-1519.
5. Huang N, Chou YJ, Chang HJ, Ho M, Morlock L. Antibiotic prescribing by ambulatory care physicians for adults with nasopharyngitis, URIs, and acute bronchitis in Taiwan; a multi-level modeling approach. Fam Practice 2005;22:160-167.
6. Akkerman AE, Kuyvenhoven MM, van der Wouden JC, Verheij TJ. Prescribing antibiotics for respiratory tract infections by GPs: management and prescriber characteristics. Br J Gen Pract 2005;55:114-118.
7. Wilson RP, Hatcher J, Barton S, Walley T. The association of some practice characteristics with antibiotic prescribing. Pharmacoepidemiol Drug Saf 1999;8:15-21.
8. Gaur AH, Hare ME, Shorr RI. Provider and practice characteristics associated with antibiotic use in children with presumed viral respiratory tract infections. Pediatrics 2005;115:635-641.
9. Barden LS, Dowell SF, Schwartz B, Lackey C. Current attitudes regarding use of antimicrobial agents: results form physicians’ and parents’ focus group discussions. Clin Pediatr 1998;37:665-672.
10. Bauchner H, Pelton SI, Klein JO. Parents, physicians, and antibiotic use. Pediatrics 1999;103:395-401.
11. Streubert HJ, Carpenter DR. Qualitative Research in Nursing: Advancing the Humanistic Imperative. 2nd ed. Philadelphia, Pa: Lippincott, 1999.
12. Lincoln YS, Guba EG. Naturalistic Inquiry. Beverly Hills, Calif: Sage, 1985.
13. Straus SE, Richardson WS, Paul Glasziou, Haynes RB. Evidence-Based Medicine: How to Practice and Teach EBM. 3rd ed. Edinburgh: Churchill Livingstone, 2005.
14. Driever MJ. Issues in clinical nursing research: are evidenced-base practice and best practice the same? West J Nurs Res 2002;24:591-597.
15. Sinclair S. Evidence-based medicine: a new ritual in medical teaching. Br Med Bull 2004;69:179-196.
16. Zitter M. Defining the great debate. Am J Manag Care 2004;10(8 Suppl):S231-S239.
17. Cockburn J, Pit S. Prescribing behavior in clinical practice: Patients’ expectations and doctors’ perceptions of patients’ expectations-a questionnaire study. BMJ 1997;315:520-523.
18. Macfarlane J, Holmes W, Macfarlane R, Britten N. Influence of patients’ expectations on antibiotic management of acute lower respiratory tract illness in general practice. BMJ 1997;315:1211-1214.
19. Little P, Martina D, Warner G, Stephens K, Senior J, Moore M. Importance of patient pressure and perceived pressure and perceived medical need for investigations, referral, and prescribing in primary care: nested observational study. BMJ 2004;328:444-446.
20. Belongia EA, Sullivan BJ, Chyou P, Madagame E, Reed KD, Schwartz B. A community trial to promote judicious antibiotic use and reduce penicillin-resistant streptococcus pneumoniae carriage in children. Pediatrics 2001;108:575-583.
21. Macfarlane J, Gard P. Reducing antibiotic use for acute bronchitis in primary care: blinded, randomized controlled trial of patient information leaflet. BMJ 2002;324:91-94.
22. Arroll B, Kenealy T, Kerse N. Do delayed prescriptions reduce the use of antibiotics for the common cold? J Fam Pract 2002;51:324-328.
23. Dowell J, Pitkethly M, Bain J, Martin S. A randomized controlled trial of delayed antibiotic prescribing as a strategy for managing uncomplicated respiratory tract infection in primary care. Br J Gen Pract 2001;51:200-205.
24. Mangione-Smith R, McGlynn EA, Elliott MN, McDonald L, Franz CE, Kravitz RL. Parent expectations for antibiotics, physician-parent communication, and satisfaction. Arch Pediatr Adolesc Med 2001;155:800-806.
25. Butler CB, Rollnick S, Pill R, Maggs-Rapport F, Stott N. Understanding the culture of prescribing. BMJ 1998;317:637-642.
26. McCaig LF, Besser RE, Hughes JM. Trends in antimicrobial prescribing rates for children and adolescents. JAMA 2002;287:3096-3102.
27. Steinman MA, Gonzales R, Linder JA, Landefeld CS. Changing use of antibiotics in community-based out-patient practice, 1991-1999. Ann Intern Med 2003;138:525-533.
28. Perz JF, Craig AS, Coffey CS, et al. Changes in antibiotic prescribing for children after a community-wide campaign. JAMA 2002;287:3103-3109.
29. Laine C, Davidoff F, Lewis CE, et al. Important elements of outpatient care: a comparison of patients’ and physicians’ opinions. Ann Intern Med 1996;125:640-645
30. Cockburn J, Pit S. Prescribing behaviour in clinical practice: patients’ expectations and doctors’ perceptions of patients’ expectations-a questionnaire study. BMJ 1997;315:520-523.
31. Gonzales R, Steiner JF, Maselli J, Lum A, Barrett PH. Impact of reducing antibiotic prescribing for acute bronchitis on patient satisfaction. Effective Clinical Practice 2001;4:105-111.
32. Hamm RM, Hicks RJ, Bemben DA. Antibiotics and respiratory infections: are patients more satisfied when expectations are met? J Fam Pract 1996;43:56-62.
33. Ray DA, Rohren CH. Characteristics of patients with upper respiratory tract infection presenting to a walk-in clinic. Mayo Clinic Proceedings 2001;76:169-173.
34. Froehlich GW, Welch HG. Meeting walk-in patients’ expectations for testing: effects on satisfaction. J Gen Intern Med 1996;11:470-474.
35. Probst JC, Greenhouse DL, Selassie AW. Patient and physician satisfaction with an outpatient care visit. J Fam Pract 1997;45:418-425.
36. Hong JS, Philbrick JT, Schorling JB. Treatment of upper respiratory infections: do patients really want antibiotics. Am J Med 1999;107:511-514.
- Keep in mind that patients may not distinguish antibiotics from other forms of prescription cold remedies.
- When patients ask for antibiotics, they really may be seeking reassurance and effective symptom management.
- Consider using negotiation strategies to delay antibiotic prescribing.
Patient, clinician, and system factors influence unnecessary antibiotic prescribing for viral acute respiratory infections (ARIs). When patients seek care for ARIs, they use a variety of tactics to obtain antibiotic prescriptions.1 Both clinicians and patients tend to overemphasize the importance of purulent secretions, whether from the nose or lung, in deciding whether an antibiotic is needed.2-4
Antibiotic prescribing increases with clinician age5 and number of years in practice,6 and antibiotics are more likely to be prescribed for ARIs in urban5 and nonteaching7,8 practice settings.
In addition, clinicians’ perceptions that patients or parents expect antibiotics are often inaccurate. They frequently assume that patients are dissatisfied when they do not receive antibiotic prescriptions for ARIs; however, accurate explanations and reassurance, not antibiotic prescriptions, have been shown to increase patient satisfaction.9,10
These factors, though known to be associated with excessive antibiotic prescribing, do not explain how clinicians actually make decisions to give antibiotics for ARIs. Thus, we applied grounded theory—a qualitative research method used to examine social processes—to provide insight into the antibiotic prescribing process.11
Methods
Setting
Our study was conducted in a rural Western community with a population of 32,014, served by 24 primary care clinicians.
Sample
We aimed to interview all of the community’s 24 primary care clinicians. Each clinician was mailed information about the study and then received a follow-up phone call to arrange an interview. Three clinicians were not interviewed due to scheduling conflicts.
The median age of the participants was 43 years with a range of 32 to 58. Nine subjects (43%) were women. Four subjects were nurse practitioners (NPs) and 17 were MDs. Areas of practice included internal medicine (n=5), college health (n=5), family medicine (n=4), pediatrics (n=4), and emergency medicine (n=3). No clinicians were representative of minority ethnic groups.
Interviews
We gathered data in audiotaped, semi-structured interviews. Hart, a practicing NP in the study community who received doctoral training in qualitative research, interviewed the 21 clinicians. The interviews ranged from 30 to 120 minutes with an average of 1 hour.
The clinician was asked to describe how he or she decided when patients presenting with ARIs should receive antibiotics. They were also asked to describe situations in which they would “most definitely” or “definitely not” prescribe antibiotics and situations that caused uncertainty. They were also asked to describe challenges associated with ARI management.
Data analysis
Hart analyzed the interviews using the constant comparative method, a form of qualitative analysis whereby each unit of data is compared with previously identified units.11 Each line of transcribed interview data was analyzed chronologically and coded for patterns, themes, and processes. The resulting codes were then compared for similarities and differences. Categories and “families” of categories were developed. This process continued until the basic social process, or one phenomenon central to each interview, was discovered. The findings were then discussed and refined by members of the research team, consisting of Pepper and Gonzales, as well as 3 PhD-prepared researchers with expertise in qualitative research.
Credibility
A recognized method for establishing credibility in qualitative research is through the use of “member checks,” wherein findings are presented to the original study participants for confirmation and clarification.12 A group member check was obtained during a “Grand Rounds” presentation at the community hospital. Thirty-one people attended, including 9 of the original participants. During a forum following the presentation, study participants confirmed that the findings accurately described their antibiotic prescribing processes.
Results
Two main concepts emerged from the interviews: individual best practice and perceived patient/parent satisfaction. These concepts are discussed using illustrative quotes from actual interviews.
Individual best practice describes how each clinician was attempting to do what he or she believed to be clinically “best” for the patient presenting with ARI symptoms. Clinicians differed in their approaches to patients presenting with ARIs. Some prescribed antibiotics for non-specific upper respiratory infection symptoms. Others were reluctant to prescribe antibiotics unless strict diagnostic criteria were met. Ultimately, each clinician made a decision based on what he or she believed was best for the patient. What defined “best” was clinician-specific. Though “best practice” always derived from evidence that informed the individual’s practice, it should not be confused with the term “best research evidence,” as defined by Straus et al.13 Five categories influenced the concept of individual best practice (TABLE).
Types of evidence used/valued to inform practice was the most influential of the 5 categories. Twelve clinicians indicated that their main source of clinical evidence was current research-based findings and related practice guidelines. The other 9 based their clinical practice on other forms of evidence, including personal and professional observations or experiences; discussions with pharmaceutical representatives; discussions with colleagues; and experiences reported by patients. Regarding personal experiences, one clinician said, “We’ll talk about my personal experiences…. Just like you have yours, I have mine and they don’t just include patients that I see. They include family members and people that I live with who’ve had an infection for 5 days—I give them an antibiotic. Two days later, they are up and about…so my empiric evidence over and over again reinforces that antibiotics are great. They work.”
Perceived patient/parent satisfaction, the second concept, describes how clinicians attempted to satisfy patients or parents according to their own perceptions of the patients’ potential to be satisfied. The 4 categories influencing the concept of perceived patient satisfaction are shown at the bottom of the TABLE.
Business concerns was the most interesting category. All 21 clinicians described the impact of patient satisfaction on the financial aspects of their practices. The 16 clinicians who collected on a fee-for-service basis described patient satisfaction as an important aspect of their livelihood as illustrated in the following comment: “You shouldn’t be treating all respiratory infections with antibiotics? Certainly. Is it practical? Probably not. I probably wouldn’t have as good of a collection rate. I truly think that part of what you’re doing is consumer-based medicine.”
Conversely, the 5 clinicians who were salaried and who did not charge on a fee-for-service basis indicated that, from a business standpoint, they were not as concerned about patient satisfaction. One clinician stated, “We can practice the better standards because of the cost elements…. You know I would feel very put upon if I had to charge every time I saw someone.”
Balancing acts. Further analysis revealed that the basic social process common to all participants was balancing acts—a process whereby clinicians weigh individual best practice against perceived patient satisfaction. Each clinician had ideas about what constituted best practice; however, each was also concerned about maintaining good patient relationships and often saw these 2 concepts at odds. Every decision that included individual best practice information was adjusted for perceived patient satisfaction. As one clinician aptly commented, “I always debate. I do a lot of mental hand wringing.”
Four types of balancing acts were identified: (a) education, (b) negotiation, (c) giving in, and (d) holding firm.
- By educating patients about the data informing their decision to not prescribe antibiotics, clinicians offered that they could often increase perceived patient satisfaction and successfully refrain from prescribing antibiotics: “Eighty percent of the time, if I explain to them about the study where they actually punctured the maxillary sinuses and cultured what they brought out and you know 90% of them did not grow out—they’re shocked, and lots of time, they’ll say, “Why didn’t my doctor ever tell me this?”
- Negotiation was another strategy identified for increasing perceived patient satisfaction. Every clinician described discussions and prescriptions regarding symptom management as a negotiation strategy for increasing patient satisfaction. Eleven clinicians described how they would negotiate with the patient about what to do if symptoms did not improve: “So I give them a specific date…and I say, “Well, let’s give it a week—if it’s not any better then call and if there are no new symptoms, then I’ll call something (an antibiotic) in for it.”
- All of the clinicians reported situations where education and negotiation failed to work, or where the patient seemed so unconvincible that attempt seemed futile. In these situations, clinicians found themselves giving in—ie, abandoning individual best practice to salvage perceived patient satisfaction. One example: “Well in some cases, when someone seems so persistent, I will say, ‘Look, I’ve been able to tell you how I feel and what I think about what’s going on with you. If you are still adamant that you need an antibiotic, then fine, if you’re going to go somewhere else to get an antibiotic, I will prescribe an antibiotic for you.’”
- The last balancing act described by all but 1 of the clinicians was holding firm to their ideas regarding individual best practice, regardless of perceived patient satisfaction: “Once in awhile, I just kind of have to say, ‘You know, I don’t think antibiotics are necessary. I just don’t want to do that.’”
TABLE
The balancing act: Individual best practice vs patient satisfaction
FACTORS INFLUENCING INDIVIDUAL BEST PRACTICE |
Initial training |
Types of evidence used/valued to inform practice (eg, current research-based evidence, clinical experience) |
Familiarity with evidence-based practice |
Interaction with peers |
Clinical uncertainty |
FACTORS INFLUENCING PERCEIVED PATIENT SATISFACTION |
Patient/parent concerns |
Day care and school concerns |
Unique patient situations |
Business concerns |
Discussion
Though individual best practice has not been referred to as such, it is by no means a novel idea and is at the core of the evidence-based practice debate, wherein evidence obtained from research is often viewed as being at odds with clinician experience.14-16 However, our study also revealed that some clinicians were unfamiliar with the research-based evidence they claimed to use. For example, 2 clinicians who claimed familiarity with research-based evidence indicated they would strongly consider prescribing antibiotics for a productive cough regardless of other historical or exam findings.
Perceived patient satisfaction has received much attention in and out of the “antibiotic” literature for its powerful influence on clinical decision-making.1,17-19 Our findings further this concept by identifying several of the factors that influence it, including patient-driven (eg, unique patient situations) and clinician-driven (eg, business concerns).
Though balancing acts is a new term, 3 of the 4 types of balancing acts have been alluded to in the antibiotic literature. Several studies have demonstrated that patient education can decrease antibiotic prescribing and use.10,20,21 Similarly patient negotiation, through the use of delayed antibiotic prescribing or a contingency plan for delayed prescribing, has also decreased antibiotic prescribing22,23 and increased patient satisfaction.24 Furthermore, it is well documented that the act of giving in to perceived patient desires for antibiotics occurs frequently.1,10,25
Holding firm was the only balancing act not paralleled in the literature. This likely stems from the fact that most research efforts have focused on situations where antibiotics were inappropriately prescribed, as opposed to situations where they were appropriately withheld. However, now that there is evidence of a decrease in antibiotic use for ARIs,26,27 it is likely that holding firm is occurring more often.
Study strengths and limitations
Interviewing both physicians and nurse practitioners increases our study’s overall applicability. Moreover, the fact that our participants practiced in the same close-knit community increased the likelihood that they were dealing with a consistent patient population.
One facet that serves as both a strength and limitation is that the main investigator (Hart) worked as a nurse practitioner in the community of study. Her experience treating patients with ARIs and her role as a community clinician undoubtedly helped her gain access to the participants, but being an “inside” investigator might have caused participants to be less candid than they would have been with an unknown investigator.
Our study relied solely on information gleaned from participant interviews; thus it is possible that some of the participants described their antibiotic prescribing processes differently than how the processes actually work during clinical encounters.
Finally, our findings represent actual descriptions of clinicians’ practices. Balancing acts and related concepts should not be confused with our beliefs about “ideal” clinical decision-making.
Implications
The balancing acts process and related concepts have several implications for clinician education and practice. Regarding individual best practice, we need to recognize that clinicians make decisions based on many different forms of evidence, including but not limited to research-based evidence, outdated or incorrect sources of literature, and personal and professional experiences. They also may be most comfortable practicing in the manner and style they were exposed to in their initial training experiences. Thus, in addition to exposing clinicians to research-based evidence, we need to teach them how to integrate research-based evidence into their practices, as well as how to deal with research findings that seem to conflict with their own observations or primary training experiences. Recognizing and using local peer influences is one way to support this concept and has been shown to be an effective strategy.28
Furthermore, we should not underestimate the impact of perceived patient satisfaction on clinician decision-making. Clinicians need to understand patient satisfaction and how it influences their practices. They need to appreciate that their own perceptions of patient desires may be inaccurate.29,30 They also need to be aware that studies have shown no relationship between antibiotic prescribing and patient satisfaction,30-32 and that patients are most satisfied when clinicians spend time with them, respect their symptoms, and honestly address their concerns.33-35
Clinicians should also appreciate that patients may not distinguish antibiotics from other forms of prescription cold remedies and that when they ask for antibiotics, they really may be seeking reassurance and effective symptom management.36 Furthermore, they need to be aware of and comfortable using various patient education, comfort, and negotiation strategies, such as symptom management and delayed antibiotic prescribing. Professional education that includes examples of realistic clinician/patient scenarios might be helpful with this.
Acknowledgments
This study was funded by the National Institutes of Health, National Institute for Nursing Research grant # NR07531-02.
CORRESPONDENCE
Ann Marie Hart, PhD, FNP, Assistant Professor, University of Wyoming, Fay W. Whitney School of Nursing, Department 3065, 1000 E. University Ave, Laramie, WY 82071. E-mail: [email protected]
- Keep in mind that patients may not distinguish antibiotics from other forms of prescription cold remedies.
- When patients ask for antibiotics, they really may be seeking reassurance and effective symptom management.
- Consider using negotiation strategies to delay antibiotic prescribing.
Patient, clinician, and system factors influence unnecessary antibiotic prescribing for viral acute respiratory infections (ARIs). When patients seek care for ARIs, they use a variety of tactics to obtain antibiotic prescriptions.1 Both clinicians and patients tend to overemphasize the importance of purulent secretions, whether from the nose or lung, in deciding whether an antibiotic is needed.2-4
Antibiotic prescribing increases with clinician age5 and number of years in practice,6 and antibiotics are more likely to be prescribed for ARIs in urban5 and nonteaching7,8 practice settings.
In addition, clinicians’ perceptions that patients or parents expect antibiotics are often inaccurate. They frequently assume that patients are dissatisfied when they do not receive antibiotic prescriptions for ARIs; however, accurate explanations and reassurance, not antibiotic prescriptions, have been shown to increase patient satisfaction.9,10
These factors, though known to be associated with excessive antibiotic prescribing, do not explain how clinicians actually make decisions to give antibiotics for ARIs. Thus, we applied grounded theory—a qualitative research method used to examine social processes—to provide insight into the antibiotic prescribing process.11
Methods
Setting
Our study was conducted in a rural Western community with a population of 32,014, served by 24 primary care clinicians.
Sample
We aimed to interview all of the community’s 24 primary care clinicians. Each clinician was mailed information about the study and then received a follow-up phone call to arrange an interview. Three clinicians were not interviewed due to scheduling conflicts.
The median age of the participants was 43 years with a range of 32 to 58. Nine subjects (43%) were women. Four subjects were nurse practitioners (NPs) and 17 were MDs. Areas of practice included internal medicine (n=5), college health (n=5), family medicine (n=4), pediatrics (n=4), and emergency medicine (n=3). No clinicians were representative of minority ethnic groups.
Interviews
We gathered data in audiotaped, semi-structured interviews. Hart, a practicing NP in the study community who received doctoral training in qualitative research, interviewed the 21 clinicians. The interviews ranged from 30 to 120 minutes with an average of 1 hour.
The clinician was asked to describe how he or she decided when patients presenting with ARIs should receive antibiotics. They were also asked to describe situations in which they would “most definitely” or “definitely not” prescribe antibiotics and situations that caused uncertainty. They were also asked to describe challenges associated with ARI management.
Data analysis
Hart analyzed the interviews using the constant comparative method, a form of qualitative analysis whereby each unit of data is compared with previously identified units.11 Each line of transcribed interview data was analyzed chronologically and coded for patterns, themes, and processes. The resulting codes were then compared for similarities and differences. Categories and “families” of categories were developed. This process continued until the basic social process, or one phenomenon central to each interview, was discovered. The findings were then discussed and refined by members of the research team, consisting of Pepper and Gonzales, as well as 3 PhD-prepared researchers with expertise in qualitative research.
Credibility
A recognized method for establishing credibility in qualitative research is through the use of “member checks,” wherein findings are presented to the original study participants for confirmation and clarification.12 A group member check was obtained during a “Grand Rounds” presentation at the community hospital. Thirty-one people attended, including 9 of the original participants. During a forum following the presentation, study participants confirmed that the findings accurately described their antibiotic prescribing processes.
Results
Two main concepts emerged from the interviews: individual best practice and perceived patient/parent satisfaction. These concepts are discussed using illustrative quotes from actual interviews.
Individual best practice describes how each clinician was attempting to do what he or she believed to be clinically “best” for the patient presenting with ARI symptoms. Clinicians differed in their approaches to patients presenting with ARIs. Some prescribed antibiotics for non-specific upper respiratory infection symptoms. Others were reluctant to prescribe antibiotics unless strict diagnostic criteria were met. Ultimately, each clinician made a decision based on what he or she believed was best for the patient. What defined “best” was clinician-specific. Though “best practice” always derived from evidence that informed the individual’s practice, it should not be confused with the term “best research evidence,” as defined by Straus et al.13 Five categories influenced the concept of individual best practice (TABLE).
Types of evidence used/valued to inform practice was the most influential of the 5 categories. Twelve clinicians indicated that their main source of clinical evidence was current research-based findings and related practice guidelines. The other 9 based their clinical practice on other forms of evidence, including personal and professional observations or experiences; discussions with pharmaceutical representatives; discussions with colleagues; and experiences reported by patients. Regarding personal experiences, one clinician said, “We’ll talk about my personal experiences…. Just like you have yours, I have mine and they don’t just include patients that I see. They include family members and people that I live with who’ve had an infection for 5 days—I give them an antibiotic. Two days later, they are up and about…so my empiric evidence over and over again reinforces that antibiotics are great. They work.”
Perceived patient/parent satisfaction, the second concept, describes how clinicians attempted to satisfy patients or parents according to their own perceptions of the patients’ potential to be satisfied. The 4 categories influencing the concept of perceived patient satisfaction are shown at the bottom of the TABLE.
Business concerns was the most interesting category. All 21 clinicians described the impact of patient satisfaction on the financial aspects of their practices. The 16 clinicians who collected on a fee-for-service basis described patient satisfaction as an important aspect of their livelihood as illustrated in the following comment: “You shouldn’t be treating all respiratory infections with antibiotics? Certainly. Is it practical? Probably not. I probably wouldn’t have as good of a collection rate. I truly think that part of what you’re doing is consumer-based medicine.”
Conversely, the 5 clinicians who were salaried and who did not charge on a fee-for-service basis indicated that, from a business standpoint, they were not as concerned about patient satisfaction. One clinician stated, “We can practice the better standards because of the cost elements…. You know I would feel very put upon if I had to charge every time I saw someone.”
Balancing acts. Further analysis revealed that the basic social process common to all participants was balancing acts—a process whereby clinicians weigh individual best practice against perceived patient satisfaction. Each clinician had ideas about what constituted best practice; however, each was also concerned about maintaining good patient relationships and often saw these 2 concepts at odds. Every decision that included individual best practice information was adjusted for perceived patient satisfaction. As one clinician aptly commented, “I always debate. I do a lot of mental hand wringing.”
Four types of balancing acts were identified: (a) education, (b) negotiation, (c) giving in, and (d) holding firm.
- By educating patients about the data informing their decision to not prescribe antibiotics, clinicians offered that they could often increase perceived patient satisfaction and successfully refrain from prescribing antibiotics: “Eighty percent of the time, if I explain to them about the study where they actually punctured the maxillary sinuses and cultured what they brought out and you know 90% of them did not grow out—they’re shocked, and lots of time, they’ll say, “Why didn’t my doctor ever tell me this?”
- Negotiation was another strategy identified for increasing perceived patient satisfaction. Every clinician described discussions and prescriptions regarding symptom management as a negotiation strategy for increasing patient satisfaction. Eleven clinicians described how they would negotiate with the patient about what to do if symptoms did not improve: “So I give them a specific date…and I say, “Well, let’s give it a week—if it’s not any better then call and if there are no new symptoms, then I’ll call something (an antibiotic) in for it.”
- All of the clinicians reported situations where education and negotiation failed to work, or where the patient seemed so unconvincible that attempt seemed futile. In these situations, clinicians found themselves giving in—ie, abandoning individual best practice to salvage perceived patient satisfaction. One example: “Well in some cases, when someone seems so persistent, I will say, ‘Look, I’ve been able to tell you how I feel and what I think about what’s going on with you. If you are still adamant that you need an antibiotic, then fine, if you’re going to go somewhere else to get an antibiotic, I will prescribe an antibiotic for you.’”
- The last balancing act described by all but 1 of the clinicians was holding firm to their ideas regarding individual best practice, regardless of perceived patient satisfaction: “Once in awhile, I just kind of have to say, ‘You know, I don’t think antibiotics are necessary. I just don’t want to do that.’”
TABLE
The balancing act: Individual best practice vs patient satisfaction
FACTORS INFLUENCING INDIVIDUAL BEST PRACTICE |
Initial training |
Types of evidence used/valued to inform practice (eg, current research-based evidence, clinical experience) |
Familiarity with evidence-based practice |
Interaction with peers |
Clinical uncertainty |
FACTORS INFLUENCING PERCEIVED PATIENT SATISFACTION |
Patient/parent concerns |
Day care and school concerns |
Unique patient situations |
Business concerns |
Discussion
Though individual best practice has not been referred to as such, it is by no means a novel idea and is at the core of the evidence-based practice debate, wherein evidence obtained from research is often viewed as being at odds with clinician experience.14-16 However, our study also revealed that some clinicians were unfamiliar with the research-based evidence they claimed to use. For example, 2 clinicians who claimed familiarity with research-based evidence indicated they would strongly consider prescribing antibiotics for a productive cough regardless of other historical or exam findings.
Perceived patient satisfaction has received much attention in and out of the “antibiotic” literature for its powerful influence on clinical decision-making.1,17-19 Our findings further this concept by identifying several of the factors that influence it, including patient-driven (eg, unique patient situations) and clinician-driven (eg, business concerns).
Though balancing acts is a new term, 3 of the 4 types of balancing acts have been alluded to in the antibiotic literature. Several studies have demonstrated that patient education can decrease antibiotic prescribing and use.10,20,21 Similarly patient negotiation, through the use of delayed antibiotic prescribing or a contingency plan for delayed prescribing, has also decreased antibiotic prescribing22,23 and increased patient satisfaction.24 Furthermore, it is well documented that the act of giving in to perceived patient desires for antibiotics occurs frequently.1,10,25
Holding firm was the only balancing act not paralleled in the literature. This likely stems from the fact that most research efforts have focused on situations where antibiotics were inappropriately prescribed, as opposed to situations where they were appropriately withheld. However, now that there is evidence of a decrease in antibiotic use for ARIs,26,27 it is likely that holding firm is occurring more often.
Study strengths and limitations
Interviewing both physicians and nurse practitioners increases our study’s overall applicability. Moreover, the fact that our participants practiced in the same close-knit community increased the likelihood that they were dealing with a consistent patient population.
One facet that serves as both a strength and limitation is that the main investigator (Hart) worked as a nurse practitioner in the community of study. Her experience treating patients with ARIs and her role as a community clinician undoubtedly helped her gain access to the participants, but being an “inside” investigator might have caused participants to be less candid than they would have been with an unknown investigator.
Our study relied solely on information gleaned from participant interviews; thus it is possible that some of the participants described their antibiotic prescribing processes differently than how the processes actually work during clinical encounters.
Finally, our findings represent actual descriptions of clinicians’ practices. Balancing acts and related concepts should not be confused with our beliefs about “ideal” clinical decision-making.
Implications
The balancing acts process and related concepts have several implications for clinician education and practice. Regarding individual best practice, we need to recognize that clinicians make decisions based on many different forms of evidence, including but not limited to research-based evidence, outdated or incorrect sources of literature, and personal and professional experiences. They also may be most comfortable practicing in the manner and style they were exposed to in their initial training experiences. Thus, in addition to exposing clinicians to research-based evidence, we need to teach them how to integrate research-based evidence into their practices, as well as how to deal with research findings that seem to conflict with their own observations or primary training experiences. Recognizing and using local peer influences is one way to support this concept and has been shown to be an effective strategy.28
Furthermore, we should not underestimate the impact of perceived patient satisfaction on clinician decision-making. Clinicians need to understand patient satisfaction and how it influences their practices. They need to appreciate that their own perceptions of patient desires may be inaccurate.29,30 They also need to be aware that studies have shown no relationship between antibiotic prescribing and patient satisfaction,30-32 and that patients are most satisfied when clinicians spend time with them, respect their symptoms, and honestly address their concerns.33-35
Clinicians should also appreciate that patients may not distinguish antibiotics from other forms of prescription cold remedies and that when they ask for antibiotics, they really may be seeking reassurance and effective symptom management.36 Furthermore, they need to be aware of and comfortable using various patient education, comfort, and negotiation strategies, such as symptom management and delayed antibiotic prescribing. Professional education that includes examples of realistic clinician/patient scenarios might be helpful with this.
Acknowledgments
This study was funded by the National Institutes of Health, National Institute for Nursing Research grant # NR07531-02.
CORRESPONDENCE
Ann Marie Hart, PhD, FNP, Assistant Professor, University of Wyoming, Fay W. Whitney School of Nursing, Department 3065, 1000 E. University Ave, Laramie, WY 82071. E-mail: [email protected]
1. Scott JG, Cohen D, DiCicco-Boom B, Orzano AJ, Jaén CR, Crabtree BF. Antibiotic use in acute respiratory infections and the ways patients pressure physicians for a prescription. J Fam Pract 2001;50:853-858.
2. Dosh SA, Hickner JM, Mainous AG, Ebell MH. Predictors of antibiotic prescribing for nonspecific upper respiratory infections, acute bronchitis, and acute sinusitis. J Fam Pract 2000;49:407-414.
3. Watson RL, Dowell SF, Jayaraman M, Keyserling H, Kolczak M, Schwartz B. Antimicrobial use for pediatric upper respiratory infections: reported practice, actual practice, and parent beliefs. Pediatrics 1999;104:1251-1257.
4. Gonzales R, Steiner JR, Lum A, Barrett PH. Decreasing antibiotic use in ambulatory practice: impact of a multidimensional intervention on the treatment of uncomplicated acute bronchitis in adults. JAMA 1999;281:1512-1519.
5. Huang N, Chou YJ, Chang HJ, Ho M, Morlock L. Antibiotic prescribing by ambulatory care physicians for adults with nasopharyngitis, URIs, and acute bronchitis in Taiwan; a multi-level modeling approach. Fam Practice 2005;22:160-167.
6. Akkerman AE, Kuyvenhoven MM, van der Wouden JC, Verheij TJ. Prescribing antibiotics for respiratory tract infections by GPs: management and prescriber characteristics. Br J Gen Pract 2005;55:114-118.
7. Wilson RP, Hatcher J, Barton S, Walley T. The association of some practice characteristics with antibiotic prescribing. Pharmacoepidemiol Drug Saf 1999;8:15-21.
8. Gaur AH, Hare ME, Shorr RI. Provider and practice characteristics associated with antibiotic use in children with presumed viral respiratory tract infections. Pediatrics 2005;115:635-641.
9. Barden LS, Dowell SF, Schwartz B, Lackey C. Current attitudes regarding use of antimicrobial agents: results form physicians’ and parents’ focus group discussions. Clin Pediatr 1998;37:665-672.
10. Bauchner H, Pelton SI, Klein JO. Parents, physicians, and antibiotic use. Pediatrics 1999;103:395-401.
11. Streubert HJ, Carpenter DR. Qualitative Research in Nursing: Advancing the Humanistic Imperative. 2nd ed. Philadelphia, Pa: Lippincott, 1999.
12. Lincoln YS, Guba EG. Naturalistic Inquiry. Beverly Hills, Calif: Sage, 1985.
13. Straus SE, Richardson WS, Paul Glasziou, Haynes RB. Evidence-Based Medicine: How to Practice and Teach EBM. 3rd ed. Edinburgh: Churchill Livingstone, 2005.
14. Driever MJ. Issues in clinical nursing research: are evidenced-base practice and best practice the same? West J Nurs Res 2002;24:591-597.
15. Sinclair S. Evidence-based medicine: a new ritual in medical teaching. Br Med Bull 2004;69:179-196.
16. Zitter M. Defining the great debate. Am J Manag Care 2004;10(8 Suppl):S231-S239.
17. Cockburn J, Pit S. Prescribing behavior in clinical practice: Patients’ expectations and doctors’ perceptions of patients’ expectations-a questionnaire study. BMJ 1997;315:520-523.
18. Macfarlane J, Holmes W, Macfarlane R, Britten N. Influence of patients’ expectations on antibiotic management of acute lower respiratory tract illness in general practice. BMJ 1997;315:1211-1214.
19. Little P, Martina D, Warner G, Stephens K, Senior J, Moore M. Importance of patient pressure and perceived pressure and perceived medical need for investigations, referral, and prescribing in primary care: nested observational study. BMJ 2004;328:444-446.
20. Belongia EA, Sullivan BJ, Chyou P, Madagame E, Reed KD, Schwartz B. A community trial to promote judicious antibiotic use and reduce penicillin-resistant streptococcus pneumoniae carriage in children. Pediatrics 2001;108:575-583.
21. Macfarlane J, Gard P. Reducing antibiotic use for acute bronchitis in primary care: blinded, randomized controlled trial of patient information leaflet. BMJ 2002;324:91-94.
22. Arroll B, Kenealy T, Kerse N. Do delayed prescriptions reduce the use of antibiotics for the common cold? J Fam Pract 2002;51:324-328.
23. Dowell J, Pitkethly M, Bain J, Martin S. A randomized controlled trial of delayed antibiotic prescribing as a strategy for managing uncomplicated respiratory tract infection in primary care. Br J Gen Pract 2001;51:200-205.
24. Mangione-Smith R, McGlynn EA, Elliott MN, McDonald L, Franz CE, Kravitz RL. Parent expectations for antibiotics, physician-parent communication, and satisfaction. Arch Pediatr Adolesc Med 2001;155:800-806.
25. Butler CB, Rollnick S, Pill R, Maggs-Rapport F, Stott N. Understanding the culture of prescribing. BMJ 1998;317:637-642.
26. McCaig LF, Besser RE, Hughes JM. Trends in antimicrobial prescribing rates for children and adolescents. JAMA 2002;287:3096-3102.
27. Steinman MA, Gonzales R, Linder JA, Landefeld CS. Changing use of antibiotics in community-based out-patient practice, 1991-1999. Ann Intern Med 2003;138:525-533.
28. Perz JF, Craig AS, Coffey CS, et al. Changes in antibiotic prescribing for children after a community-wide campaign. JAMA 2002;287:3103-3109.
29. Laine C, Davidoff F, Lewis CE, et al. Important elements of outpatient care: a comparison of patients’ and physicians’ opinions. Ann Intern Med 1996;125:640-645
30. Cockburn J, Pit S. Prescribing behaviour in clinical practice: patients’ expectations and doctors’ perceptions of patients’ expectations-a questionnaire study. BMJ 1997;315:520-523.
31. Gonzales R, Steiner JF, Maselli J, Lum A, Barrett PH. Impact of reducing antibiotic prescribing for acute bronchitis on patient satisfaction. Effective Clinical Practice 2001;4:105-111.
32. Hamm RM, Hicks RJ, Bemben DA. Antibiotics and respiratory infections: are patients more satisfied when expectations are met? J Fam Pract 1996;43:56-62.
33. Ray DA, Rohren CH. Characteristics of patients with upper respiratory tract infection presenting to a walk-in clinic. Mayo Clinic Proceedings 2001;76:169-173.
34. Froehlich GW, Welch HG. Meeting walk-in patients’ expectations for testing: effects on satisfaction. J Gen Intern Med 1996;11:470-474.
35. Probst JC, Greenhouse DL, Selassie AW. Patient and physician satisfaction with an outpatient care visit. J Fam Pract 1997;45:418-425.
36. Hong JS, Philbrick JT, Schorling JB. Treatment of upper respiratory infections: do patients really want antibiotics. Am J Med 1999;107:511-514.
1. Scott JG, Cohen D, DiCicco-Boom B, Orzano AJ, Jaén CR, Crabtree BF. Antibiotic use in acute respiratory infections and the ways patients pressure physicians for a prescription. J Fam Pract 2001;50:853-858.
2. Dosh SA, Hickner JM, Mainous AG, Ebell MH. Predictors of antibiotic prescribing for nonspecific upper respiratory infections, acute bronchitis, and acute sinusitis. J Fam Pract 2000;49:407-414.
3. Watson RL, Dowell SF, Jayaraman M, Keyserling H, Kolczak M, Schwartz B. Antimicrobial use for pediatric upper respiratory infections: reported practice, actual practice, and parent beliefs. Pediatrics 1999;104:1251-1257.
4. Gonzales R, Steiner JR, Lum A, Barrett PH. Decreasing antibiotic use in ambulatory practice: impact of a multidimensional intervention on the treatment of uncomplicated acute bronchitis in adults. JAMA 1999;281:1512-1519.
5. Huang N, Chou YJ, Chang HJ, Ho M, Morlock L. Antibiotic prescribing by ambulatory care physicians for adults with nasopharyngitis, URIs, and acute bronchitis in Taiwan; a multi-level modeling approach. Fam Practice 2005;22:160-167.
6. Akkerman AE, Kuyvenhoven MM, van der Wouden JC, Verheij TJ. Prescribing antibiotics for respiratory tract infections by GPs: management and prescriber characteristics. Br J Gen Pract 2005;55:114-118.
7. Wilson RP, Hatcher J, Barton S, Walley T. The association of some practice characteristics with antibiotic prescribing. Pharmacoepidemiol Drug Saf 1999;8:15-21.
8. Gaur AH, Hare ME, Shorr RI. Provider and practice characteristics associated with antibiotic use in children with presumed viral respiratory tract infections. Pediatrics 2005;115:635-641.
9. Barden LS, Dowell SF, Schwartz B, Lackey C. Current attitudes regarding use of antimicrobial agents: results form physicians’ and parents’ focus group discussions. Clin Pediatr 1998;37:665-672.
10. Bauchner H, Pelton SI, Klein JO. Parents, physicians, and antibiotic use. Pediatrics 1999;103:395-401.
11. Streubert HJ, Carpenter DR. Qualitative Research in Nursing: Advancing the Humanistic Imperative. 2nd ed. Philadelphia, Pa: Lippincott, 1999.
12. Lincoln YS, Guba EG. Naturalistic Inquiry. Beverly Hills, Calif: Sage, 1985.
13. Straus SE, Richardson WS, Paul Glasziou, Haynes RB. Evidence-Based Medicine: How to Practice and Teach EBM. 3rd ed. Edinburgh: Churchill Livingstone, 2005.
14. Driever MJ. Issues in clinical nursing research: are evidenced-base practice and best practice the same? West J Nurs Res 2002;24:591-597.
15. Sinclair S. Evidence-based medicine: a new ritual in medical teaching. Br Med Bull 2004;69:179-196.
16. Zitter M. Defining the great debate. Am J Manag Care 2004;10(8 Suppl):S231-S239.
17. Cockburn J, Pit S. Prescribing behavior in clinical practice: Patients’ expectations and doctors’ perceptions of patients’ expectations-a questionnaire study. BMJ 1997;315:520-523.
18. Macfarlane J, Holmes W, Macfarlane R, Britten N. Influence of patients’ expectations on antibiotic management of acute lower respiratory tract illness in general practice. BMJ 1997;315:1211-1214.
19. Little P, Martina D, Warner G, Stephens K, Senior J, Moore M. Importance of patient pressure and perceived pressure and perceived medical need for investigations, referral, and prescribing in primary care: nested observational study. BMJ 2004;328:444-446.
20. Belongia EA, Sullivan BJ, Chyou P, Madagame E, Reed KD, Schwartz B. A community trial to promote judicious antibiotic use and reduce penicillin-resistant streptococcus pneumoniae carriage in children. Pediatrics 2001;108:575-583.
21. Macfarlane J, Gard P. Reducing antibiotic use for acute bronchitis in primary care: blinded, randomized controlled trial of patient information leaflet. BMJ 2002;324:91-94.
22. Arroll B, Kenealy T, Kerse N. Do delayed prescriptions reduce the use of antibiotics for the common cold? J Fam Pract 2002;51:324-328.
23. Dowell J, Pitkethly M, Bain J, Martin S. A randomized controlled trial of delayed antibiotic prescribing as a strategy for managing uncomplicated respiratory tract infection in primary care. Br J Gen Pract 2001;51:200-205.
24. Mangione-Smith R, McGlynn EA, Elliott MN, McDonald L, Franz CE, Kravitz RL. Parent expectations for antibiotics, physician-parent communication, and satisfaction. Arch Pediatr Adolesc Med 2001;155:800-806.
25. Butler CB, Rollnick S, Pill R, Maggs-Rapport F, Stott N. Understanding the culture of prescribing. BMJ 1998;317:637-642.
26. McCaig LF, Besser RE, Hughes JM. Trends in antimicrobial prescribing rates for children and adolescents. JAMA 2002;287:3096-3102.
27. Steinman MA, Gonzales R, Linder JA, Landefeld CS. Changing use of antibiotics in community-based out-patient practice, 1991-1999. Ann Intern Med 2003;138:525-533.
28. Perz JF, Craig AS, Coffey CS, et al. Changes in antibiotic prescribing for children after a community-wide campaign. JAMA 2002;287:3103-3109.
29. Laine C, Davidoff F, Lewis CE, et al. Important elements of outpatient care: a comparison of patients’ and physicians’ opinions. Ann Intern Med 1996;125:640-645
30. Cockburn J, Pit S. Prescribing behaviour in clinical practice: patients’ expectations and doctors’ perceptions of patients’ expectations-a questionnaire study. BMJ 1997;315:520-523.
31. Gonzales R, Steiner JF, Maselli J, Lum A, Barrett PH. Impact of reducing antibiotic prescribing for acute bronchitis on patient satisfaction. Effective Clinical Practice 2001;4:105-111.
32. Hamm RM, Hicks RJ, Bemben DA. Antibiotics and respiratory infections: are patients more satisfied when expectations are met? J Fam Pract 1996;43:56-62.
33. Ray DA, Rohren CH. Characteristics of patients with upper respiratory tract infection presenting to a walk-in clinic. Mayo Clinic Proceedings 2001;76:169-173.
34. Froehlich GW, Welch HG. Meeting walk-in patients’ expectations for testing: effects on satisfaction. J Gen Intern Med 1996;11:470-474.
35. Probst JC, Greenhouse DL, Selassie AW. Patient and physician satisfaction with an outpatient care visit. J Fam Pract 1997;45:418-425.
36. Hong JS, Philbrick JT, Schorling JB. Treatment of upper respiratory infections: do patients really want antibiotics. Am J Med 1999;107:511-514.
Treatment of Community‐Acquired Pneumonia
In the United States, community‐acquired pneumonia (CAP) leads to nearly 1 million hospitalizations annually, with aggregate costs of hospitalization approaching $9 billion.1, 2 In an effort to improve the appropriate, cost‐effective care for patients with CAP, several professional societies have developed clinical practice guidelines and pathways for pneumonia.37 Although the guidelines address all aspects of care, they devote substantial attention to antibiotic recommendations. Most U.S. guidelines recommend treatment of hospitalized patients with an intravenous beta‐lactam combined with a macrolide, or a fluoroquinolone with activity against Streptococcus pneumoniae. Of the major U.S. CAP practice guidelines, only one6 recommends doxycycline as an alternative to a macrolide for inpatients.
Doxycycline is an attractive alternative to macrolides. Similar to macrolides, doxycycline is active against a wide variety of organisms including atypical bacteria (Chlamydia pneumoniae, Legionella pneumophilus, and Mycoplasma pneumoniae) and is well tolerated.810 In addition, it is inexpensive (cost of $1.00/day [awp] for 100 mg p.o. bid), and rates of tetracycline/doxycycline resistance among S. pneumoniae isolates have remained low, in contrast to the increasing rates of resistance to macrolides and fluoroquinolones.11, 12 The most recent guidelines from the Infectious Diseases Society of America cited limited published clinical data on the effectiveness of doxycycline in CAP as a barrier to increased use.7 Only one study of hospitalized patients has been published in the era of penicillin‐resistant pneumococcus, and this study included only 43 low‐risk patients treated with doxycycline.13 At the university hospital affiliated with the University of California, San Francisco, ceftriaxone plus doxycycline is generally recommended as initial empiric antibiotic therapy for patients hospitalized with CAP, but significant variability in prescribing exists, allowing for comparisons between patients treated with different initial empiric antibiotic regimens. We compared outcomes of hospitalized patients with CAP treated with ceftriaxone plus doxycycline to those of patients treated with alternative initial empiric therapy at an academic medical center.
METHODS
Study Population
A retrospective cohort study of all adults (age 18 years) discharged from the inpatient general medicine service of Moffitt‐Long Hospital at the University of California, San Francisco, was conducted from January 1999 through July 2001. Eligibility criteria included a principal discharge diagnosis of CAP and a chest radiograph demonstrating an infiltrate within 48 hours of admission. Exclusion criteria included infection with the human immunodeficiency virus, history of organ transplantation or use of immunosuppressive therapy (including prednisone > 15 mg/day), cystic fibrosis, postobstructive pneumonia, active tuberculosis, recent hospitalization (within 10 days), or admission for comfort care. The study protocol and procedures were reviewed and approved by the UCSF Committee for Human Research.
Data Collection
Medical record review by trained research assistants blinded to the research question was used to gather demographic data, comorbid illnesses, physical examination findings on initial presentation, and laboratory or radiographic results on initial presentation. The pneumonia severity index (PSI) score was calculated for each patient using the above data.14 In addition, data were collected on antibiotic allergies, antibiotics used within the 30 days prior to admission, results of sputum or blood cultures, and admission location (intensive care unit [ICU] versus medical floor).
Data from TSI (Transition Systems Inc., Boston, MA), the hospital administrative database, were used to identify the initial empiric antibiotic regimen. All antibiotics prescribed within the first 48 hours of hospitalization were considered initial empiric therapy with few exceptions. Initial empiric therapy was classified as 1) ceftriaxone plus doxycycline (including patients treated with these agents alone in the first 48 hours, as well as patients treated with both agents in the first 24 hours who were switched to alternative therapy [broader coverage] on the second day), or 2) other appropriate therapy (treatment consistent with current national guideline recommendations including at least a beta‐lactam plus a macrolide or a beta‐lactam plus a fluoroquinolone, or fluoroquinolone monotherapy). Patients receiving therapy inconsistent with current national guideline recommendations were excluded.
Outcomes
TSI data were used to identify length of stay, death during the index hospitalization, and return to the emergency department or readmission within 30 days of discharge. The National Death Index was used to identify all deaths that occurred after hospital discharge. The 30‐day mortality data included deaths occurring during the index hospitalization and in the 30 days after the index hospitalization discharge.
Statistical Analysis
For the purposes of this analysis we compared patients treated with ceftriaxone plus doxycycline to patients treated with other appropriate therapy. To examine demographic and clinical differences between the two groups, statistical tests of comparison were performed using chi‐square tests for the dichotomous variables and t tests for the numeric variables, all of which were normally distributed (after log transformation in the case of length of stay).
To adjust for clinical variables that might contribute to differences in outcomes between the two groups, we used backward stepwise logistic regression analysis to construct a propensity score15 for the likelihood of ceftriaxone plus doxycycline use. The propensity score reflected the conditional probability of exposure to ceftriaxone plus doxycycline and allowed for stratification and, subsequently, comparisons by quintiles of propensity score. Propensity scores often have distinct advantages over direct adjustment for a large number of confounding variables and allow direct comparisons between groups with a similar propensity for receiving ceftriaxone plus doxycycline.15 Unlike random assignment of treatment, however, the propensity score cannot balance unmeasured variables that may affect treatment assignment. Thus, the possibility of bias remains. The variables used to build the score included age, presence of comorbid illness, admission from a nursing home or long‐term care facility, antibiotic allergy, prior antibiotic use, PSI score, PSI risk class, diagnosis of aspiration, admission to the ICU, and positive blood cultures. The propensity score was then stratified and used as an adjustment variable in comparisons between groups for in‐hospital mortality, 30‐day mortality, and 30‐day readmission rates. As expected, length of stay was highly skewed and was therefore log‐transformed and compared between groups with adjustment for the propensity score.
To further address issues related to potential selection bias, a separate analysis was performed on a subset of the original cohort that excluded patients for whom ceftriaxone plus doxycycline would not generally be recommended as first‐line therapy. For this analysis, patients admitted from a nursing home or long‐term care facility, patients admitted to the ICU, and patients with a principal diagnosis of aspiration pneumonia were excluded. A propensity score was rederived for this subset, which was used to adjust for differences in outcomes. All statistical procedures were performed using STATA (Ver. 7.0, Stata Corporation, College Station TX).
RESULTS
Patient Characteristics
A total of 341 patients were eligible for analysis. Of this group, 216 were treated with ceftriaxone plus doxycycline and 125 received other appropriate therapy. Both groups of patients were similar in age. Patients treated with ceftriaxone plus doxycycline had a lower median PSI score and fewer comorbid illnesses than did patients treated with other appropriate therapy (Table 1). Blood cultures were positive in 30 (8.8%) of the 341 patients included in the analysis, with S. pneumoniae the most commonly isolated organism (n = 17, 5.0%). Of S. pneumoniae isolates, 4 (24%) were resistant to penicillin (MIC 1 g/mL), and 2 (12%) were resistant to tetracycline (MIC 8 g/mL).
Ceftriaxone/doxycycline | Other appropriate therapy | |
---|---|---|
| ||
Patients (n) | 216 | 125 |
Age (median) | 76 | 74 |
PSI Score (median)a | 97 | 108 |
PSI Risk Class (%)a | ||
Class I | 9.3 | 5.6 |
Class II | 11.1 | 8.8 |
Class III | 21.8 | 13.6 |
Class IV | 40.7 | 40.0 |
Class V | 17.1 | 32.0 |
Comorbid Illness (%)a | 36.1 | 47.2 |
Nursing Home/LCF (%)a | 5.1 | 14.4 |
Aspiration (%)a | 3.2 | 20.0 |
Admission to ICU (%)a | 6.0 | 28.0 |
Common antibiotic choices in patients receiving other appropriate therapy included a beta‐lactam/beta‐lactamase inhibitor plus doxycycline or a macrolide (n = 36, 29%), fluoroquinolone monotherapy (n = 16, 13%), and a variety of other antibiotic combinations with activity against S. pneumoniae and atypical bacteria (n = 52, 42%).
Clinical Outcomes
Analyses of unadjusted outcomes showed that patients treated with ceftriaxone plus doxycycline had significantly lower inpatient (2% vs. 14%, P < .001) and 30‐day (6% vs. 20%, P < .001) mortality compared to patients treated with other regimens (Table 2). Multivariable logistic regression analysis identified three variables (diagnosis of congestive heart failure, admission to the ICU, and the presence of comorbid illness) associated with initial antibiotic selection, which were used to build a propensity score. After adjustment for the propensity score, use of ceftriaxone plus doxycycline remained significantly associated with lower inpatient mortality (OR = 0.26, 95% CI: 0.080.81) and 30‐day mortality (OR = 0.37, 95% CI: 0.170.81). Differences in length of stay and 30‐day readmission rates between the treatment groups were not significant (Table 2).
Ceftriaxone + doxycycline (n = 216) | Other appropriate therapy (n = 125) | Adjusted odds ratio (95% confidence interval) | |
---|---|---|---|
| |||
Inpatient Mortality | 2.3% | 14.4% | 0.26 (0.080.81) |
30‐day mortality | 6.0% | 20.0% | 0.37 (0.170.81) |
Length of stay (median days) | 3.0 | 4.0 | 0.09 (0.250.06)a |
30‐day readmission | 10.7% | 12.0% | 0.87 (0.421.81) |
Subset Analysis
To address issues related to selection bias, we performed an analysis of a subset of the patients after excluding those admitted from a nursing home, diagnosed with aspiration, or admitted to the ICU, for whom ceftriaxone plus doxycycline would not be considered recommended (or first‐line) therapy. The two resulting groups were similar, except there were fewer patients with comorbid illness in the ceftriaxone plus doxycycline group (34% vs. 50%, P = .015). The propensity score was rederived for this subset and used for adjustment. Unadjusted and adjusted outcomes are shown in Table 3. Use of ceftriaxone plus doxycycline in this subset also was associated with reduced odds of inpatient mortality (OR = 0.17, 95% CI: 0.040.77). The odds of 30‐day mortality also were reduced but not significantly, as the confidence interval included 1.0 (OR = 0.43, 95% CI: 0.141.31). There were no differences between groups in length of stay or in 30‐day readmission rate.
Ceftriaxone + doxycycline (n = 188) | Other appropriate therapy (n = 70) | Adjusted odds ratio (95% CI) | |
---|---|---|---|
| |||
Age (median years) | 75 | 71 | |
PSI score (mean) | 95 | 98 | |
Comorbid illness (%)a | 33.5 | 50.0 | |
Inpatient mortality | 1.6% | 7.1% | 0.17 (0.040.77) |
30‐day mortality | 4.8% | 8.6% | 0.43 (0.141.31) |
LOS (median days) | 3 | 3 | 0.06 (0.240.12)b |
30‐day readmission | 11.9% | 10.0% | 1.31 (0.523.28) |
DISCUSSION
In our hospital setting, the use of ceftriaxone plus doxycycline as the initial empiric antibiotic therapy for patients hospitalized with community‐acquired pneumonia was associated with significantly lower inpatient and 30‐day mortality, even after adjusting for clinical differences between groups. We did not find a difference between regimens in hospital length of stay or 30‐day readmission rate. In case the multivariable model was insufficient to account for the clinical differences (i.e., selection bias) between groups, we also performed an analysis of a subgroup of less severely ill patients by excluding those admitted from nursing homes, those admitted to the intensive care unit, and those with aspiration pneumonia. In this subset, use of ceftriaxone plus doxycycline remained associated with lower inpatient mortality but not with lower 30‐day mortality. Although, as an observational study, the results of our findings could still be a result of residual confounding, we believe the results provide valuable information regarding doxycycline.
Combination therapy with a macrolide, but not doxycycline, is advocated by the practice guidelines of several major U.S. professional societies,3, 4, 7 apparently because of a lack of data on the effectiveness of combination therapy with doxycycline.7 Only one randomized, unblinded study, in 87 low‐risk patients hospitalized with CAP, that compared monotherapy with IV doxycycline versus physician‐determined therapy has been conducted.13 This study found no differences between treatment groups in clinical outcomes but did find that use of doxycycline was associated with shorter hospital stays and reduced costs. Our results, achieved in a real‐world setting in relatively ill hospitalized patients (58% were in PSI risk class IV or V), provide further support for the use of combination therapy with doxycycline.
Hospitalized patients treated with a beta‐lactam in combination with a macrolide are often discharged on macrolide monotherapy. In our population most patients treated with ceftriaxone plus doxycycline were discharged on doxycycline if they required continued therapy (data not shown). In the current era of resistance of Streptococcus pneumoniae to antibiotics, there is good reason to believe doxycycline may perform as well, if not better, than macrolides when hospitalized patients with CAP are discharged on oral monotherapy. Macrolide resistance rates among invasive pneumococcal isolates in the United States doubled from 10% to 20% during a period in which prescriptions for macrolides increased by 13%.12 In addition, a large surveillance study of more than 1500 isolates collected in 1999 and 2000 found that 26% of the isolates were resistant to macrolides, whereas only 16% were resistant to tetracycline.16 In vitro testing against S. Pneumoniae has also suggested that tetracycline resistance overestimates doxycycline resistance.17, 18 More recently, Streptococcus pneumoniae susceptibility data from the SENTRY Antimicrobial Surveillance program reaffirmed doxycycline's in vitro superiority over macrolides.17
Our study had several limitations. The study design adopted precluded determining whether favorable results with the use of ceftriaxone plus doxycycline resulted from an effect unique to this combination of antibiotics, the possible anti‐inflammatory properties of doxycycline alone,19, 20 or unmeasured confounders. For example, processes of care that affect clinical outcomes for patients hospitalized with CAP, such as the timing of antibiotic delivery, the timing of blood cultures, and stability assessment on discharge were not measured in this study. To affect outcomes, these processes of care would need to be differentially distributed between our comparison groups. However, because this study was performed in a single institution during a single interval, it is likely that the performance of these processes of care would be similar for all patients.
In conclusion, ceftriaxone plus doxycycline appears to be an effective, and possibly superior, therapy for patients hospitalized with CAP. Randomized controlled trials of doxycycline‐containing regimens versus other regimens are warranted.
- Hospitalized pneumonia. Outcomes, treatment patterns, and costs in urban and rural areas.J Gen Intern Med.1996;11:415–421. , , , et al.
- The cost of treating community‐acquired pneumonia.Clin Ther.1998;20:820–837. , , , et al.
- Practice guidelines for the management of community‐acquired pneumonia in adults. Infectious Diseases Society of America.Clin Infect Dis.2000;31:347–382. , , , et al.
- Management of community‐acquired pneumonia in the era of pneumococcal resistance: a report from the Drug‐Resistant Streptococcus pneumoniae Therapeutic Working Group.Arch Intern Med.2000;160:1399–1408. , , , et al.
- Canadian guidelines for the initial management of community‐acquired pneumonia: an evidence‐based update by the Canadian Infectious Diseases Society and the Canadian Thoracic Society. The Canadian Community‐Acquired Pneumonia Working Group.Clin Infect Dis.2000;31:383–421. , , , et al.
- Guidelines for the management of adults with community‐acquired pneumonia. Diagnosis, assessment of severity, antimicrobial therapy, and prevention.Am J Respir Crit Care Med.2001;163:1730–1754. , , , et al.
- Update of practice guidelines for the management of community‐acquired pneumonia in immunocompetent adults.Clin Infect Dis.2003;37:1405–1433 , , , et al.
- Doxycycline.Ther Drug Monit1982;4:115–135 , , .
- Chloramphenicol and tetracyclines.Med Clin North Am.1987;71:1155–1168 , .
- Tetracyclines.Med Clin North Am.1995;79:789–801 , .
- Antibiotic resistance among gram‐negative bacilli in US intensive care units: implications for fluoroquinolone use.JAMA.2003;289:885–888 , , , et al.
- Macrolide resistance among invasive Streptococcus pneumoniae isolates.JAMA.2001;286:1857–1862. , , , et al.
- Doxycycline is a cost‐effective therapy for hospitalized patients with community‐acquired pneumonia.Arch Intern Med.1999;159:266–270. , , , et al.
- A prediction rule to identify low‐risk patients with community‐acquired pneumonia.N Engl J Med.1997;336:243–250. , , , et al.
- Reducing bias in observational studies using subclassification on the propensity score.J Am Stat Assoc.1984;79:516–524 .
- Antimicrobial resistance among clinical isolates of Streptococcus pneumoniae in the United States during 1999—2000, including a comparison of resistance rates since 1994—1995.Antimicrob Agents Chemother.2001;45:1721–1729 , , , et al.
- Doxycycline use for community‐acquired pneumonia: contemporary in vitro spectrum of activity against Streptococcus pneumoniae (1999–2002).Diagn Microbiol Infect Dis.2004;49:147–149 , , .
- Doxycycline activity against Streptococcus pneumoniae.Chest.1995;108:1775–1776. , , , et al.
- Inhibition of enzymatic activity of phospholipases A2 by minocycline and doxycycline.Biochem Pharmacol.1992;44:1165–1170. , , , et al.
- Doxycycline reduces mortality to lethal endotoxemia by reducing nitric oxide synthesis via an interleukin‐10‐independent mechanism.J Infect Dis.1998;177:489–492. , , , et al.
In the United States, community‐acquired pneumonia (CAP) leads to nearly 1 million hospitalizations annually, with aggregate costs of hospitalization approaching $9 billion.1, 2 In an effort to improve the appropriate, cost‐effective care for patients with CAP, several professional societies have developed clinical practice guidelines and pathways for pneumonia.37 Although the guidelines address all aspects of care, they devote substantial attention to antibiotic recommendations. Most U.S. guidelines recommend treatment of hospitalized patients with an intravenous beta‐lactam combined with a macrolide, or a fluoroquinolone with activity against Streptococcus pneumoniae. Of the major U.S. CAP practice guidelines, only one6 recommends doxycycline as an alternative to a macrolide for inpatients.
Doxycycline is an attractive alternative to macrolides. Similar to macrolides, doxycycline is active against a wide variety of organisms including atypical bacteria (Chlamydia pneumoniae, Legionella pneumophilus, and Mycoplasma pneumoniae) and is well tolerated.810 In addition, it is inexpensive (cost of $1.00/day [awp] for 100 mg p.o. bid), and rates of tetracycline/doxycycline resistance among S. pneumoniae isolates have remained low, in contrast to the increasing rates of resistance to macrolides and fluoroquinolones.11, 12 The most recent guidelines from the Infectious Diseases Society of America cited limited published clinical data on the effectiveness of doxycycline in CAP as a barrier to increased use.7 Only one study of hospitalized patients has been published in the era of penicillin‐resistant pneumococcus, and this study included only 43 low‐risk patients treated with doxycycline.13 At the university hospital affiliated with the University of California, San Francisco, ceftriaxone plus doxycycline is generally recommended as initial empiric antibiotic therapy for patients hospitalized with CAP, but significant variability in prescribing exists, allowing for comparisons between patients treated with different initial empiric antibiotic regimens. We compared outcomes of hospitalized patients with CAP treated with ceftriaxone plus doxycycline to those of patients treated with alternative initial empiric therapy at an academic medical center.
METHODS
Study Population
A retrospective cohort study of all adults (age 18 years) discharged from the inpatient general medicine service of Moffitt‐Long Hospital at the University of California, San Francisco, was conducted from January 1999 through July 2001. Eligibility criteria included a principal discharge diagnosis of CAP and a chest radiograph demonstrating an infiltrate within 48 hours of admission. Exclusion criteria included infection with the human immunodeficiency virus, history of organ transplantation or use of immunosuppressive therapy (including prednisone > 15 mg/day), cystic fibrosis, postobstructive pneumonia, active tuberculosis, recent hospitalization (within 10 days), or admission for comfort care. The study protocol and procedures were reviewed and approved by the UCSF Committee for Human Research.
Data Collection
Medical record review by trained research assistants blinded to the research question was used to gather demographic data, comorbid illnesses, physical examination findings on initial presentation, and laboratory or radiographic results on initial presentation. The pneumonia severity index (PSI) score was calculated for each patient using the above data.14 In addition, data were collected on antibiotic allergies, antibiotics used within the 30 days prior to admission, results of sputum or blood cultures, and admission location (intensive care unit [ICU] versus medical floor).
Data from TSI (Transition Systems Inc., Boston, MA), the hospital administrative database, were used to identify the initial empiric antibiotic regimen. All antibiotics prescribed within the first 48 hours of hospitalization were considered initial empiric therapy with few exceptions. Initial empiric therapy was classified as 1) ceftriaxone plus doxycycline (including patients treated with these agents alone in the first 48 hours, as well as patients treated with both agents in the first 24 hours who were switched to alternative therapy [broader coverage] on the second day), or 2) other appropriate therapy (treatment consistent with current national guideline recommendations including at least a beta‐lactam plus a macrolide or a beta‐lactam plus a fluoroquinolone, or fluoroquinolone monotherapy). Patients receiving therapy inconsistent with current national guideline recommendations were excluded.
Outcomes
TSI data were used to identify length of stay, death during the index hospitalization, and return to the emergency department or readmission within 30 days of discharge. The National Death Index was used to identify all deaths that occurred after hospital discharge. The 30‐day mortality data included deaths occurring during the index hospitalization and in the 30 days after the index hospitalization discharge.
Statistical Analysis
For the purposes of this analysis we compared patients treated with ceftriaxone plus doxycycline to patients treated with other appropriate therapy. To examine demographic and clinical differences between the two groups, statistical tests of comparison were performed using chi‐square tests for the dichotomous variables and t tests for the numeric variables, all of which were normally distributed (after log transformation in the case of length of stay).
To adjust for clinical variables that might contribute to differences in outcomes between the two groups, we used backward stepwise logistic regression analysis to construct a propensity score15 for the likelihood of ceftriaxone plus doxycycline use. The propensity score reflected the conditional probability of exposure to ceftriaxone plus doxycycline and allowed for stratification and, subsequently, comparisons by quintiles of propensity score. Propensity scores often have distinct advantages over direct adjustment for a large number of confounding variables and allow direct comparisons between groups with a similar propensity for receiving ceftriaxone plus doxycycline.15 Unlike random assignment of treatment, however, the propensity score cannot balance unmeasured variables that may affect treatment assignment. Thus, the possibility of bias remains. The variables used to build the score included age, presence of comorbid illness, admission from a nursing home or long‐term care facility, antibiotic allergy, prior antibiotic use, PSI score, PSI risk class, diagnosis of aspiration, admission to the ICU, and positive blood cultures. The propensity score was then stratified and used as an adjustment variable in comparisons between groups for in‐hospital mortality, 30‐day mortality, and 30‐day readmission rates. As expected, length of stay was highly skewed and was therefore log‐transformed and compared between groups with adjustment for the propensity score.
To further address issues related to potential selection bias, a separate analysis was performed on a subset of the original cohort that excluded patients for whom ceftriaxone plus doxycycline would not generally be recommended as first‐line therapy. For this analysis, patients admitted from a nursing home or long‐term care facility, patients admitted to the ICU, and patients with a principal diagnosis of aspiration pneumonia were excluded. A propensity score was rederived for this subset, which was used to adjust for differences in outcomes. All statistical procedures were performed using STATA (Ver. 7.0, Stata Corporation, College Station TX).
RESULTS
Patient Characteristics
A total of 341 patients were eligible for analysis. Of this group, 216 were treated with ceftriaxone plus doxycycline and 125 received other appropriate therapy. Both groups of patients were similar in age. Patients treated with ceftriaxone plus doxycycline had a lower median PSI score and fewer comorbid illnesses than did patients treated with other appropriate therapy (Table 1). Blood cultures were positive in 30 (8.8%) of the 341 patients included in the analysis, with S. pneumoniae the most commonly isolated organism (n = 17, 5.0%). Of S. pneumoniae isolates, 4 (24%) were resistant to penicillin (MIC 1 g/mL), and 2 (12%) were resistant to tetracycline (MIC 8 g/mL).
Ceftriaxone/doxycycline | Other appropriate therapy | |
---|---|---|
| ||
Patients (n) | 216 | 125 |
Age (median) | 76 | 74 |
PSI Score (median)a | 97 | 108 |
PSI Risk Class (%)a | ||
Class I | 9.3 | 5.6 |
Class II | 11.1 | 8.8 |
Class III | 21.8 | 13.6 |
Class IV | 40.7 | 40.0 |
Class V | 17.1 | 32.0 |
Comorbid Illness (%)a | 36.1 | 47.2 |
Nursing Home/LCF (%)a | 5.1 | 14.4 |
Aspiration (%)a | 3.2 | 20.0 |
Admission to ICU (%)a | 6.0 | 28.0 |
Common antibiotic choices in patients receiving other appropriate therapy included a beta‐lactam/beta‐lactamase inhibitor plus doxycycline or a macrolide (n = 36, 29%), fluoroquinolone monotherapy (n = 16, 13%), and a variety of other antibiotic combinations with activity against S. pneumoniae and atypical bacteria (n = 52, 42%).
Clinical Outcomes
Analyses of unadjusted outcomes showed that patients treated with ceftriaxone plus doxycycline had significantly lower inpatient (2% vs. 14%, P < .001) and 30‐day (6% vs. 20%, P < .001) mortality compared to patients treated with other regimens (Table 2). Multivariable logistic regression analysis identified three variables (diagnosis of congestive heart failure, admission to the ICU, and the presence of comorbid illness) associated with initial antibiotic selection, which were used to build a propensity score. After adjustment for the propensity score, use of ceftriaxone plus doxycycline remained significantly associated with lower inpatient mortality (OR = 0.26, 95% CI: 0.080.81) and 30‐day mortality (OR = 0.37, 95% CI: 0.170.81). Differences in length of stay and 30‐day readmission rates between the treatment groups were not significant (Table 2).
Ceftriaxone + doxycycline (n = 216) | Other appropriate therapy (n = 125) | Adjusted odds ratio (95% confidence interval) | |
---|---|---|---|
| |||
Inpatient Mortality | 2.3% | 14.4% | 0.26 (0.080.81) |
30‐day mortality | 6.0% | 20.0% | 0.37 (0.170.81) |
Length of stay (median days) | 3.0 | 4.0 | 0.09 (0.250.06)a |
30‐day readmission | 10.7% | 12.0% | 0.87 (0.421.81) |
Subset Analysis
To address issues related to selection bias, we performed an analysis of a subset of the patients after excluding those admitted from a nursing home, diagnosed with aspiration, or admitted to the ICU, for whom ceftriaxone plus doxycycline would not be considered recommended (or first‐line) therapy. The two resulting groups were similar, except there were fewer patients with comorbid illness in the ceftriaxone plus doxycycline group (34% vs. 50%, P = .015). The propensity score was rederived for this subset and used for adjustment. Unadjusted and adjusted outcomes are shown in Table 3. Use of ceftriaxone plus doxycycline in this subset also was associated with reduced odds of inpatient mortality (OR = 0.17, 95% CI: 0.040.77). The odds of 30‐day mortality also were reduced but not significantly, as the confidence interval included 1.0 (OR = 0.43, 95% CI: 0.141.31). There were no differences between groups in length of stay or in 30‐day readmission rate.
Ceftriaxone + doxycycline (n = 188) | Other appropriate therapy (n = 70) | Adjusted odds ratio (95% CI) | |
---|---|---|---|
| |||
Age (median years) | 75 | 71 | |
PSI score (mean) | 95 | 98 | |
Comorbid illness (%)a | 33.5 | 50.0 | |
Inpatient mortality | 1.6% | 7.1% | 0.17 (0.040.77) |
30‐day mortality | 4.8% | 8.6% | 0.43 (0.141.31) |
LOS (median days) | 3 | 3 | 0.06 (0.240.12)b |
30‐day readmission | 11.9% | 10.0% | 1.31 (0.523.28) |
DISCUSSION
In our hospital setting, the use of ceftriaxone plus doxycycline as the initial empiric antibiotic therapy for patients hospitalized with community‐acquired pneumonia was associated with significantly lower inpatient and 30‐day mortality, even after adjusting for clinical differences between groups. We did not find a difference between regimens in hospital length of stay or 30‐day readmission rate. In case the multivariable model was insufficient to account for the clinical differences (i.e., selection bias) between groups, we also performed an analysis of a subgroup of less severely ill patients by excluding those admitted from nursing homes, those admitted to the intensive care unit, and those with aspiration pneumonia. In this subset, use of ceftriaxone plus doxycycline remained associated with lower inpatient mortality but not with lower 30‐day mortality. Although, as an observational study, the results of our findings could still be a result of residual confounding, we believe the results provide valuable information regarding doxycycline.
Combination therapy with a macrolide, but not doxycycline, is advocated by the practice guidelines of several major U.S. professional societies,3, 4, 7 apparently because of a lack of data on the effectiveness of combination therapy with doxycycline.7 Only one randomized, unblinded study, in 87 low‐risk patients hospitalized with CAP, that compared monotherapy with IV doxycycline versus physician‐determined therapy has been conducted.13 This study found no differences between treatment groups in clinical outcomes but did find that use of doxycycline was associated with shorter hospital stays and reduced costs. Our results, achieved in a real‐world setting in relatively ill hospitalized patients (58% were in PSI risk class IV or V), provide further support for the use of combination therapy with doxycycline.
Hospitalized patients treated with a beta‐lactam in combination with a macrolide are often discharged on macrolide monotherapy. In our population most patients treated with ceftriaxone plus doxycycline were discharged on doxycycline if they required continued therapy (data not shown). In the current era of resistance of Streptococcus pneumoniae to antibiotics, there is good reason to believe doxycycline may perform as well, if not better, than macrolides when hospitalized patients with CAP are discharged on oral monotherapy. Macrolide resistance rates among invasive pneumococcal isolates in the United States doubled from 10% to 20% during a period in which prescriptions for macrolides increased by 13%.12 In addition, a large surveillance study of more than 1500 isolates collected in 1999 and 2000 found that 26% of the isolates were resistant to macrolides, whereas only 16% were resistant to tetracycline.16 In vitro testing against S. Pneumoniae has also suggested that tetracycline resistance overestimates doxycycline resistance.17, 18 More recently, Streptococcus pneumoniae susceptibility data from the SENTRY Antimicrobial Surveillance program reaffirmed doxycycline's in vitro superiority over macrolides.17
Our study had several limitations. The study design adopted precluded determining whether favorable results with the use of ceftriaxone plus doxycycline resulted from an effect unique to this combination of antibiotics, the possible anti‐inflammatory properties of doxycycline alone,19, 20 or unmeasured confounders. For example, processes of care that affect clinical outcomes for patients hospitalized with CAP, such as the timing of antibiotic delivery, the timing of blood cultures, and stability assessment on discharge were not measured in this study. To affect outcomes, these processes of care would need to be differentially distributed between our comparison groups. However, because this study was performed in a single institution during a single interval, it is likely that the performance of these processes of care would be similar for all patients.
In conclusion, ceftriaxone plus doxycycline appears to be an effective, and possibly superior, therapy for patients hospitalized with CAP. Randomized controlled trials of doxycycline‐containing regimens versus other regimens are warranted.
In the United States, community‐acquired pneumonia (CAP) leads to nearly 1 million hospitalizations annually, with aggregate costs of hospitalization approaching $9 billion.1, 2 In an effort to improve the appropriate, cost‐effective care for patients with CAP, several professional societies have developed clinical practice guidelines and pathways for pneumonia.37 Although the guidelines address all aspects of care, they devote substantial attention to antibiotic recommendations. Most U.S. guidelines recommend treatment of hospitalized patients with an intravenous beta‐lactam combined with a macrolide, or a fluoroquinolone with activity against Streptococcus pneumoniae. Of the major U.S. CAP practice guidelines, only one6 recommends doxycycline as an alternative to a macrolide for inpatients.
Doxycycline is an attractive alternative to macrolides. Similar to macrolides, doxycycline is active against a wide variety of organisms including atypical bacteria (Chlamydia pneumoniae, Legionella pneumophilus, and Mycoplasma pneumoniae) and is well tolerated.810 In addition, it is inexpensive (cost of $1.00/day [awp] for 100 mg p.o. bid), and rates of tetracycline/doxycycline resistance among S. pneumoniae isolates have remained low, in contrast to the increasing rates of resistance to macrolides and fluoroquinolones.11, 12 The most recent guidelines from the Infectious Diseases Society of America cited limited published clinical data on the effectiveness of doxycycline in CAP as a barrier to increased use.7 Only one study of hospitalized patients has been published in the era of penicillin‐resistant pneumococcus, and this study included only 43 low‐risk patients treated with doxycycline.13 At the university hospital affiliated with the University of California, San Francisco, ceftriaxone plus doxycycline is generally recommended as initial empiric antibiotic therapy for patients hospitalized with CAP, but significant variability in prescribing exists, allowing for comparisons between patients treated with different initial empiric antibiotic regimens. We compared outcomes of hospitalized patients with CAP treated with ceftriaxone plus doxycycline to those of patients treated with alternative initial empiric therapy at an academic medical center.
METHODS
Study Population
A retrospective cohort study of all adults (age 18 years) discharged from the inpatient general medicine service of Moffitt‐Long Hospital at the University of California, San Francisco, was conducted from January 1999 through July 2001. Eligibility criteria included a principal discharge diagnosis of CAP and a chest radiograph demonstrating an infiltrate within 48 hours of admission. Exclusion criteria included infection with the human immunodeficiency virus, history of organ transplantation or use of immunosuppressive therapy (including prednisone > 15 mg/day), cystic fibrosis, postobstructive pneumonia, active tuberculosis, recent hospitalization (within 10 days), or admission for comfort care. The study protocol and procedures were reviewed and approved by the UCSF Committee for Human Research.
Data Collection
Medical record review by trained research assistants blinded to the research question was used to gather demographic data, comorbid illnesses, physical examination findings on initial presentation, and laboratory or radiographic results on initial presentation. The pneumonia severity index (PSI) score was calculated for each patient using the above data.14 In addition, data were collected on antibiotic allergies, antibiotics used within the 30 days prior to admission, results of sputum or blood cultures, and admission location (intensive care unit [ICU] versus medical floor).
Data from TSI (Transition Systems Inc., Boston, MA), the hospital administrative database, were used to identify the initial empiric antibiotic regimen. All antibiotics prescribed within the first 48 hours of hospitalization were considered initial empiric therapy with few exceptions. Initial empiric therapy was classified as 1) ceftriaxone plus doxycycline (including patients treated with these agents alone in the first 48 hours, as well as patients treated with both agents in the first 24 hours who were switched to alternative therapy [broader coverage] on the second day), or 2) other appropriate therapy (treatment consistent with current national guideline recommendations including at least a beta‐lactam plus a macrolide or a beta‐lactam plus a fluoroquinolone, or fluoroquinolone monotherapy). Patients receiving therapy inconsistent with current national guideline recommendations were excluded.
Outcomes
TSI data were used to identify length of stay, death during the index hospitalization, and return to the emergency department or readmission within 30 days of discharge. The National Death Index was used to identify all deaths that occurred after hospital discharge. The 30‐day mortality data included deaths occurring during the index hospitalization and in the 30 days after the index hospitalization discharge.
Statistical Analysis
For the purposes of this analysis we compared patients treated with ceftriaxone plus doxycycline to patients treated with other appropriate therapy. To examine demographic and clinical differences between the two groups, statistical tests of comparison were performed using chi‐square tests for the dichotomous variables and t tests for the numeric variables, all of which were normally distributed (after log transformation in the case of length of stay).
To adjust for clinical variables that might contribute to differences in outcomes between the two groups, we used backward stepwise logistic regression analysis to construct a propensity score15 for the likelihood of ceftriaxone plus doxycycline use. The propensity score reflected the conditional probability of exposure to ceftriaxone plus doxycycline and allowed for stratification and, subsequently, comparisons by quintiles of propensity score. Propensity scores often have distinct advantages over direct adjustment for a large number of confounding variables and allow direct comparisons between groups with a similar propensity for receiving ceftriaxone plus doxycycline.15 Unlike random assignment of treatment, however, the propensity score cannot balance unmeasured variables that may affect treatment assignment. Thus, the possibility of bias remains. The variables used to build the score included age, presence of comorbid illness, admission from a nursing home or long‐term care facility, antibiotic allergy, prior antibiotic use, PSI score, PSI risk class, diagnosis of aspiration, admission to the ICU, and positive blood cultures. The propensity score was then stratified and used as an adjustment variable in comparisons between groups for in‐hospital mortality, 30‐day mortality, and 30‐day readmission rates. As expected, length of stay was highly skewed and was therefore log‐transformed and compared between groups with adjustment for the propensity score.
To further address issues related to potential selection bias, a separate analysis was performed on a subset of the original cohort that excluded patients for whom ceftriaxone plus doxycycline would not generally be recommended as first‐line therapy. For this analysis, patients admitted from a nursing home or long‐term care facility, patients admitted to the ICU, and patients with a principal diagnosis of aspiration pneumonia were excluded. A propensity score was rederived for this subset, which was used to adjust for differences in outcomes. All statistical procedures were performed using STATA (Ver. 7.0, Stata Corporation, College Station TX).
RESULTS
Patient Characteristics
A total of 341 patients were eligible for analysis. Of this group, 216 were treated with ceftriaxone plus doxycycline and 125 received other appropriate therapy. Both groups of patients were similar in age. Patients treated with ceftriaxone plus doxycycline had a lower median PSI score and fewer comorbid illnesses than did patients treated with other appropriate therapy (Table 1). Blood cultures were positive in 30 (8.8%) of the 341 patients included in the analysis, with S. pneumoniae the most commonly isolated organism (n = 17, 5.0%). Of S. pneumoniae isolates, 4 (24%) were resistant to penicillin (MIC 1 g/mL), and 2 (12%) were resistant to tetracycline (MIC 8 g/mL).
Ceftriaxone/doxycycline | Other appropriate therapy | |
---|---|---|
| ||
Patients (n) | 216 | 125 |
Age (median) | 76 | 74 |
PSI Score (median)a | 97 | 108 |
PSI Risk Class (%)a | ||
Class I | 9.3 | 5.6 |
Class II | 11.1 | 8.8 |
Class III | 21.8 | 13.6 |
Class IV | 40.7 | 40.0 |
Class V | 17.1 | 32.0 |
Comorbid Illness (%)a | 36.1 | 47.2 |
Nursing Home/LCF (%)a | 5.1 | 14.4 |
Aspiration (%)a | 3.2 | 20.0 |
Admission to ICU (%)a | 6.0 | 28.0 |
Common antibiotic choices in patients receiving other appropriate therapy included a beta‐lactam/beta‐lactamase inhibitor plus doxycycline or a macrolide (n = 36, 29%), fluoroquinolone monotherapy (n = 16, 13%), and a variety of other antibiotic combinations with activity against S. pneumoniae and atypical bacteria (n = 52, 42%).
Clinical Outcomes
Analyses of unadjusted outcomes showed that patients treated with ceftriaxone plus doxycycline had significantly lower inpatient (2% vs. 14%, P < .001) and 30‐day (6% vs. 20%, P < .001) mortality compared to patients treated with other regimens (Table 2). Multivariable logistic regression analysis identified three variables (diagnosis of congestive heart failure, admission to the ICU, and the presence of comorbid illness) associated with initial antibiotic selection, which were used to build a propensity score. After adjustment for the propensity score, use of ceftriaxone plus doxycycline remained significantly associated with lower inpatient mortality (OR = 0.26, 95% CI: 0.080.81) and 30‐day mortality (OR = 0.37, 95% CI: 0.170.81). Differences in length of stay and 30‐day readmission rates between the treatment groups were not significant (Table 2).
Ceftriaxone + doxycycline (n = 216) | Other appropriate therapy (n = 125) | Adjusted odds ratio (95% confidence interval) | |
---|---|---|---|
| |||
Inpatient Mortality | 2.3% | 14.4% | 0.26 (0.080.81) |
30‐day mortality | 6.0% | 20.0% | 0.37 (0.170.81) |
Length of stay (median days) | 3.0 | 4.0 | 0.09 (0.250.06)a |
30‐day readmission | 10.7% | 12.0% | 0.87 (0.421.81) |
Subset Analysis
To address issues related to selection bias, we performed an analysis of a subset of the patients after excluding those admitted from a nursing home, diagnosed with aspiration, or admitted to the ICU, for whom ceftriaxone plus doxycycline would not be considered recommended (or first‐line) therapy. The two resulting groups were similar, except there were fewer patients with comorbid illness in the ceftriaxone plus doxycycline group (34% vs. 50%, P = .015). The propensity score was rederived for this subset and used for adjustment. Unadjusted and adjusted outcomes are shown in Table 3. Use of ceftriaxone plus doxycycline in this subset also was associated with reduced odds of inpatient mortality (OR = 0.17, 95% CI: 0.040.77). The odds of 30‐day mortality also were reduced but not significantly, as the confidence interval included 1.0 (OR = 0.43, 95% CI: 0.141.31). There were no differences between groups in length of stay or in 30‐day readmission rate.
Ceftriaxone + doxycycline (n = 188) | Other appropriate therapy (n = 70) | Adjusted odds ratio (95% CI) | |
---|---|---|---|
| |||
Age (median years) | 75 | 71 | |
PSI score (mean) | 95 | 98 | |
Comorbid illness (%)a | 33.5 | 50.0 | |
Inpatient mortality | 1.6% | 7.1% | 0.17 (0.040.77) |
30‐day mortality | 4.8% | 8.6% | 0.43 (0.141.31) |
LOS (median days) | 3 | 3 | 0.06 (0.240.12)b |
30‐day readmission | 11.9% | 10.0% | 1.31 (0.523.28) |
DISCUSSION
In our hospital setting, the use of ceftriaxone plus doxycycline as the initial empiric antibiotic therapy for patients hospitalized with community‐acquired pneumonia was associated with significantly lower inpatient and 30‐day mortality, even after adjusting for clinical differences between groups. We did not find a difference between regimens in hospital length of stay or 30‐day readmission rate. In case the multivariable model was insufficient to account for the clinical differences (i.e., selection bias) between groups, we also performed an analysis of a subgroup of less severely ill patients by excluding those admitted from nursing homes, those admitted to the intensive care unit, and those with aspiration pneumonia. In this subset, use of ceftriaxone plus doxycycline remained associated with lower inpatient mortality but not with lower 30‐day mortality. Although, as an observational study, the results of our findings could still be a result of residual confounding, we believe the results provide valuable information regarding doxycycline.
Combination therapy with a macrolide, but not doxycycline, is advocated by the practice guidelines of several major U.S. professional societies,3, 4, 7 apparently because of a lack of data on the effectiveness of combination therapy with doxycycline.7 Only one randomized, unblinded study, in 87 low‐risk patients hospitalized with CAP, that compared monotherapy with IV doxycycline versus physician‐determined therapy has been conducted.13 This study found no differences between treatment groups in clinical outcomes but did find that use of doxycycline was associated with shorter hospital stays and reduced costs. Our results, achieved in a real‐world setting in relatively ill hospitalized patients (58% were in PSI risk class IV or V), provide further support for the use of combination therapy with doxycycline.
Hospitalized patients treated with a beta‐lactam in combination with a macrolide are often discharged on macrolide monotherapy. In our population most patients treated with ceftriaxone plus doxycycline were discharged on doxycycline if they required continued therapy (data not shown). In the current era of resistance of Streptococcus pneumoniae to antibiotics, there is good reason to believe doxycycline may perform as well, if not better, than macrolides when hospitalized patients with CAP are discharged on oral monotherapy. Macrolide resistance rates among invasive pneumococcal isolates in the United States doubled from 10% to 20% during a period in which prescriptions for macrolides increased by 13%.12 In addition, a large surveillance study of more than 1500 isolates collected in 1999 and 2000 found that 26% of the isolates were resistant to macrolides, whereas only 16% were resistant to tetracycline.16 In vitro testing against S. Pneumoniae has also suggested that tetracycline resistance overestimates doxycycline resistance.17, 18 More recently, Streptococcus pneumoniae susceptibility data from the SENTRY Antimicrobial Surveillance program reaffirmed doxycycline's in vitro superiority over macrolides.17
Our study had several limitations. The study design adopted precluded determining whether favorable results with the use of ceftriaxone plus doxycycline resulted from an effect unique to this combination of antibiotics, the possible anti‐inflammatory properties of doxycycline alone,19, 20 or unmeasured confounders. For example, processes of care that affect clinical outcomes for patients hospitalized with CAP, such as the timing of antibiotic delivery, the timing of blood cultures, and stability assessment on discharge were not measured in this study. To affect outcomes, these processes of care would need to be differentially distributed between our comparison groups. However, because this study was performed in a single institution during a single interval, it is likely that the performance of these processes of care would be similar for all patients.
In conclusion, ceftriaxone plus doxycycline appears to be an effective, and possibly superior, therapy for patients hospitalized with CAP. Randomized controlled trials of doxycycline‐containing regimens versus other regimens are warranted.
- Hospitalized pneumonia. Outcomes, treatment patterns, and costs in urban and rural areas.J Gen Intern Med.1996;11:415–421. , , , et al.
- The cost of treating community‐acquired pneumonia.Clin Ther.1998;20:820–837. , , , et al.
- Practice guidelines for the management of community‐acquired pneumonia in adults. Infectious Diseases Society of America.Clin Infect Dis.2000;31:347–382. , , , et al.
- Management of community‐acquired pneumonia in the era of pneumococcal resistance: a report from the Drug‐Resistant Streptococcus pneumoniae Therapeutic Working Group.Arch Intern Med.2000;160:1399–1408. , , , et al.
- Canadian guidelines for the initial management of community‐acquired pneumonia: an evidence‐based update by the Canadian Infectious Diseases Society and the Canadian Thoracic Society. The Canadian Community‐Acquired Pneumonia Working Group.Clin Infect Dis.2000;31:383–421. , , , et al.
- Guidelines for the management of adults with community‐acquired pneumonia. Diagnosis, assessment of severity, antimicrobial therapy, and prevention.Am J Respir Crit Care Med.2001;163:1730–1754. , , , et al.
- Update of practice guidelines for the management of community‐acquired pneumonia in immunocompetent adults.Clin Infect Dis.2003;37:1405–1433 , , , et al.
- Doxycycline.Ther Drug Monit1982;4:115–135 , , .
- Chloramphenicol and tetracyclines.Med Clin North Am.1987;71:1155–1168 , .
- Tetracyclines.Med Clin North Am.1995;79:789–801 , .
- Antibiotic resistance among gram‐negative bacilli in US intensive care units: implications for fluoroquinolone use.JAMA.2003;289:885–888 , , , et al.
- Macrolide resistance among invasive Streptococcus pneumoniae isolates.JAMA.2001;286:1857–1862. , , , et al.
- Doxycycline is a cost‐effective therapy for hospitalized patients with community‐acquired pneumonia.Arch Intern Med.1999;159:266–270. , , , et al.
- A prediction rule to identify low‐risk patients with community‐acquired pneumonia.N Engl J Med.1997;336:243–250. , , , et al.
- Reducing bias in observational studies using subclassification on the propensity score.J Am Stat Assoc.1984;79:516–524 .
- Antimicrobial resistance among clinical isolates of Streptococcus pneumoniae in the United States during 1999—2000, including a comparison of resistance rates since 1994—1995.Antimicrob Agents Chemother.2001;45:1721–1729 , , , et al.
- Doxycycline use for community‐acquired pneumonia: contemporary in vitro spectrum of activity against Streptococcus pneumoniae (1999–2002).Diagn Microbiol Infect Dis.2004;49:147–149 , , .
- Doxycycline activity against Streptococcus pneumoniae.Chest.1995;108:1775–1776. , , , et al.
- Inhibition of enzymatic activity of phospholipases A2 by minocycline and doxycycline.Biochem Pharmacol.1992;44:1165–1170. , , , et al.
- Doxycycline reduces mortality to lethal endotoxemia by reducing nitric oxide synthesis via an interleukin‐10‐independent mechanism.J Infect Dis.1998;177:489–492. , , , et al.
- Hospitalized pneumonia. Outcomes, treatment patterns, and costs in urban and rural areas.J Gen Intern Med.1996;11:415–421. , , , et al.
- The cost of treating community‐acquired pneumonia.Clin Ther.1998;20:820–837. , , , et al.
- Practice guidelines for the management of community‐acquired pneumonia in adults. Infectious Diseases Society of America.Clin Infect Dis.2000;31:347–382. , , , et al.
- Management of community‐acquired pneumonia in the era of pneumococcal resistance: a report from the Drug‐Resistant Streptococcus pneumoniae Therapeutic Working Group.Arch Intern Med.2000;160:1399–1408. , , , et al.
- Canadian guidelines for the initial management of community‐acquired pneumonia: an evidence‐based update by the Canadian Infectious Diseases Society and the Canadian Thoracic Society. The Canadian Community‐Acquired Pneumonia Working Group.Clin Infect Dis.2000;31:383–421. , , , et al.
- Guidelines for the management of adults with community‐acquired pneumonia. Diagnosis, assessment of severity, antimicrobial therapy, and prevention.Am J Respir Crit Care Med.2001;163:1730–1754. , , , et al.
- Update of practice guidelines for the management of community‐acquired pneumonia in immunocompetent adults.Clin Infect Dis.2003;37:1405–1433 , , , et al.
- Doxycycline.Ther Drug Monit1982;4:115–135 , , .
- Chloramphenicol and tetracyclines.Med Clin North Am.1987;71:1155–1168 , .
- Tetracyclines.Med Clin North Am.1995;79:789–801 , .
- Antibiotic resistance among gram‐negative bacilli in US intensive care units: implications for fluoroquinolone use.JAMA.2003;289:885–888 , , , et al.
- Macrolide resistance among invasive Streptococcus pneumoniae isolates.JAMA.2001;286:1857–1862. , , , et al.
- Doxycycline is a cost‐effective therapy for hospitalized patients with community‐acquired pneumonia.Arch Intern Med.1999;159:266–270. , , , et al.
- A prediction rule to identify low‐risk patients with community‐acquired pneumonia.N Engl J Med.1997;336:243–250. , , , et al.
- Reducing bias in observational studies using subclassification on the propensity score.J Am Stat Assoc.1984;79:516–524 .
- Antimicrobial resistance among clinical isolates of Streptococcus pneumoniae in the United States during 1999—2000, including a comparison of resistance rates since 1994—1995.Antimicrob Agents Chemother.2001;45:1721–1729 , , , et al.
- Doxycycline use for community‐acquired pneumonia: contemporary in vitro spectrum of activity against Streptococcus pneumoniae (1999–2002).Diagn Microbiol Infect Dis.2004;49:147–149 , , .
- Doxycycline activity against Streptococcus pneumoniae.Chest.1995;108:1775–1776. , , , et al.
- Inhibition of enzymatic activity of phospholipases A2 by minocycline and doxycycline.Biochem Pharmacol.1992;44:1165–1170. , , , et al.
- Doxycycline reduces mortality to lethal endotoxemia by reducing nitric oxide synthesis via an interleukin‐10‐independent mechanism.J Infect Dis.1998;177:489–492. , , , et al.
Copyright © 2006 Society of Hospital Medicine