User login
Effect of Hospital Readmission Reduction on Patients at Low, Medium, and High Risk of Readmission in the Medicare Population
Given the high cost of readmissions to the healthcare system, there has been a substantial push to reduce readmissions by policymakers.1 Among these is the Hospital Readmissions Reduction Program (HRRP), in which hospitals with higher than expected readmission rates receive reduced payments from Medicare.2 Recent evidence has suggested the success of such policy changes, with multiple reports demonstrating a decrease in 30-day readmission rates in the Medicare population starting in 2010.3-8
Initiatives to reduce readmissions can also have an effect on total number of admissions.9,10 Indeed, along with the recent reduction in readmission, there has been a reduction in all admissions among Medicare beneficiaries.11,12 Some studies have found that as admissions have decreased, the burden of comorbidity has increased among hospitalized patients,3,11 suggesting that hospitals may be increasingly filled with patients at high risk of readmission. However, whether readmission risk among hospitalized patients has changed remains unknown, and understanding changes in risk profile could help inform which patients to target with future interventions to reduce readmissions.
Hospital efforts to reduce readmissions may have differential effects on types of patients by risk. For instance, low-intensity, system-wide interventions such as standardized discharge instructions or medicine reconciliation may have a stronger effect on patients at relatively low risk of readmission who may have a few important drivers of readmission that are easily overcome. Alternatively, the impact of intensive care transitions management might be greatest for high-risk patients, who have the most need for postdischarge medications, follow-up, and self-care.
The purpose of this study was therefore twofold: (1) to observe changes in average monthly risk of readmission among hospitalized Medicare patients and (2) to examine changes in readmission rates for Medicare patients at various risk of readmission. We hypothesized that readmission risk in the Medicare population would increase in recent years, as overall number of admissions and readmissions have fallen.7,11 Additionally, we hypothesized that standardized readmission rates would decline less in highest risk patients as compared with the lowest risk patients because transitional care interventions may not be able to mitigate the large burden of comorbidity and social issues present in many high-risk patients.13,14
METHODS
We performed a retrospective cohort study of hospitalizations to US nonfederal short-term acute care facilities by Medicare beneficiaries between January 2009 and June 2015. The design involved 4 steps. First, we estimated a predictive model for unplanned readmissions within 30 days of discharge. Second, we assigned each hospitalization a predicted risk of readmission based on the model. Third, we studied trends in mean predicted risk of readmission during the study period. Fourth, we examined trends in observed to expected (O/E) readmission for hospitalizations in the lowest, middle, and highest categories of predicted risk of readmission to determine whether reductions in readmissions were more substantial in certain risk groups than in others.
Data were obtained from the Centers for Medicare and Medicaid Services (CMS) Inpatient Standard Analytic File and the Medicare Enrollment Data Base. We included hospitalizations of fee-for-service Medicare beneficiaries age ≥65 with continuous enrollment in Part A Medicare fee-for-service for at least 1 year prior and 30 days after the hospitalization.15 Hospitalizations with a discharge disposition of death, transfer to another acute hospital, and left against medical advice (AMA) were excluded. We also excluded patients with enrollment in hospice care prior to hospitalization. We excluded hospitalizations in June 2012 because of an irregularity in data availability for that month.
Hospitalizations were categorized into 5 specialty cohorts according to service line. The 5 cohorts were those used for the CMS hospital-wide readmission measure and included surgery/gynecology, medicine, cardiovascular, cardiorespiratory, and neurology.15 Among the 3 clinical conditions tracked as part of HRRP, heart failure and pneumonia were a subset of the cardiorespiratory cohort, while acute myocardial infarction was a subset of the cardiovascular cohort. Our use of cohorts was threefold: first, the average risk of readmission differs substantially across these cohorts, so pooling them produces heterogeneous risk strata; second, risk variables perform differently in different cohorts, so one single model may not be as accurate for calculating risk; and, third, the use of disease cohorts makes our results comparable to the CMS model and similar to other readmission studies in Medicare.7,8,15
For development of the risk model, the outcome was 30-day unplanned hospital readmission. Planned readmissions were excluded; these were defined by the CMS algorithm as readmissions in which a typically planned procedure occurred in a hospitalization with a nonacute principal diagnosis.16 Independent variables included age and comorbidities in the final hospital-wide readmission models for each of the 5 specialty cohorts.15 In order to produce the best possible individual risk prediction for each patient, we added additional independent variables that CMS avoids for hospital quality measurement purposes but that contribute to risk of readmission: sex, race, dual eligibility status, number of prior AMA discharges, intensive care unit stay during current hospitalization, coronary care unit stay during current hospitalization, and hospitalization in the prior 30, 90, and 180 days. We also included an indicator variable for hospitalizations with more than 9 discharge diagnosis codes on or after January 2011, the time at which Medicare allowed an increase of the number of International Classification of Diseases, 9th Revision-Clinical Modification diagnosis billing codes from 9 to 25.17 This indicator adjusts for the increased availability of comorbidity codes, which might otherwise inflate the predicted risk relative to hospitalizations prior to that date.
Based on the risk models, each hospitalization was assigned a predicted risk of readmission. For each specialty cohort, we pooled all hospitalizations across all study years and divided them into risk quintiles. We categorized hospitalizations as high risk if in the highest quintile, medium risk if in the middle 3 quintiles, and low risk if in the lowest quintile of predicted risk for all study hospitalizations in a given specialty cohort.
For our time trend analyses, we studied 2 outcomes: monthly mean predicted risk and monthly ratio of observed readmissions to expected readmissions for patients in the lowest, middle, and highest categories of predicted risk of readmission. We studied monthly predicted risk to determine whether the average readmission risk of patients was changing over time as admission and readmission rates were declining. We studied the ratio of O/E readmissions to determine whether the decline in overall readmissions was more substantial in particular risk strata; we used the ratio of O/E readmissions, which measures number of readmissions divided by number of readmissions predicted by the model, rather than crude observed readmissions, as O/E readmissions account for any changes in risk profiles over time within each risk stratum. Independent variables in our trend analyses were year—entered as a continuous variable—and indicators for postintroduction of the Affordable Care Act (ACA, March 2010) and for postintroduction of HRRP (October 2012); these time indicators were included because of prior studies demonstrating that the introduction of ACA was associated with a decrease from baseline in readmission rates, which leveled off after introduction of HRRP.7 We also included an indicator for calendar quarter to account for seasonal effects.
Statistical Analysis
We developed generalized estimating equation models to predict 30-day unplanned readmission for each of the 5 specialty cohorts. The 5 models were fit using all patients in each cohort for the included time period and were adjusted for clustering by hospital. We assessed discrimination by calculating area under the receiver operating characteristic curve (AUC) for the 5 models; the AUCs measured the models’ ability to distinguish patients who were readmitted versus those who were not.18 We also calculated AUCs for each year to examine model performance over time.
Using these models, we calculated predicted risk for each hospitalization and averaged these to obtain mean predicted risk for each specialty cohort for each month. To test for trends in mean risk, we estimated 5 time series models, one for each cohort, with the dependent variable of monthly mean predicted risk. For each cohort, we first estimated a series of 12 empty autoregressive models, each with a different autoregressive term (1, 2...12). For each model, we calculated χ2 for the test that the autocorrelation was 0; based on a comparison of chi-squared values, we specified an autocorrelation of 1 month for all models. Accordingly, a 1-month lag was used to estimate one final model for each cohort. Independent variables included year and indicators for post-ACA and post-HRRP; these variables captured the effect of trends over time and the introduction of these policy changes, respectively.19
To determine whether changes in risk over time were a result of changes in particular risk groups, we categorized hospitalizations into risk strata based on quintiles of predicted risk for each specialty cohort for the entire study period. For each individual year, we calculated the proportion of hospitalizations in the highest, middle, and lowest readmission risk strata for each cohort.
We calculated the monthly ratio of O/E readmission for hospitalizations in the lowest 20%, middle 60%, and highest 20% of readmission risk by month; O/E reflects the excess or deficit observed events relative to the number predicted by the model. Using this monthly O/E as the dependent variable, we developed autoregressive time series models as above, again with a 1-month lag, for each of these 3 risk strata in each cohort. As before, independent variables were year as a continuous variable, indicator variables for post-ACA and post-HRRP, and a categorical variable for calendar quarter.
All analyses were done in SAS version 9.3 (SAS Institute Inc., Cary, NC) and Stata version 14.2 (StataCorp LLC, College Station, TX).
RESULTS
We included 47,288,961 hospitalizations in the study, of which 11,231,242 (23.8%) were in the surgery/gynecology cohort, 19,548,711 (41.3%) were in the medicine cohort, 5,433,125 (11.5%) were in the cardiovascular cohort, 8,179,691 (17.3%) were in the cardiorespiratory cohort, and 2,896,192 (6.1%) were in the neurology cohort. The readmission rate was 16.2% (n = 7,642,161) overall, with the highest rates observed in the cardiorespiratory (20.5%) and medicine (17.6%) cohorts and the lowest rates observed in the surgery/gynecology (11.8%) and neurology (13.8%) cohorts.
The final predictive models for each cohort ranged in number of parameters from 56 for the cardiorespiratory cohort to 264 for the surgery/gynecology cohort. The models had AUCs of 0.70, 0.65, 0.67, 0.65, and 0.63 for the surgery/gynecology, medicine, cardiovascular, cardiorespiratory, and neurology cohorts, respectively; AUCs remained fairly stable over time for all disease cohorts (Appendix Table 1).
DISCUSSION
A number of mechanisms may account for the across-the-board improvements in readmission reduction. Many hospitals have instituted system-wide interventions, including patient education, medicine reconciliation, and early postdischarge follow-up,20 which may have reduced readmissions across all patient risk strata. Alternatively, hospitals may have implemented interventions that disproportionally benefited low-risk patients while simultaneously utilizing interventions that only benefited high-risk patients. For instance, increasing threshold for admission7 may have the greatest effect on low-risk patients who could be most easily managed at home, while many intensive transitional care interventions have been developed to target only high-risk patients.21,22
With the introduction of HRRP, there have been a number of concerns about the readmission measure used to penalize hospitals for high readmission rates. One major concern has been that the readmission metric may be flawed in its ability to capture continued improvement related to readmission.23 Some have suggested that with better population health management, admissions will decrease, patient risk of the remaining patients will increase, and hospitals will be increasingly filled with patients who have high likelihood of readmission. This potential for increased risk with HRRP was suggested by a recent study that found that comorbidities increased in hospitalized Medicare beneficiaries between 2010 and 2013.11 Our results were mixed in supporting this potential phenomenon because we examined global risk of readmission and found that some of the cohorts had increased risk over time while others did not. Others have expressed concern that readmission measure does not account for socioeconomic status, which has been associated with readmission rates.24-27 Although we did not directly examine socioeconomic status in our study, we found that hospitals have been able to reduce readmission across all levels of risk, which includes markers of socioeconomic status, including race and Medicaid eligibility status.
Although we hypothesized that readmission risk would increase as number of hospitalizations decreased over time, we found no increase in readmission risk among the cohorts with HRRP diagnoses that had the largest decrease in readmission rates.7,8 Conversely, readmission risk did increase—with a concurrent increase in the proportion of high-risk hospitalizations—in the surgery/gynecology and neurology cohorts that were not subject to HRRP penalties. Nonetheless, rehospitalizations were reduced for all risk categories in these 2 cohorts. Notably, surgery/gynecology and neurology had the lowest readmission rates overall. These findings suggest that initiatives to prevent initial hospitalizations, such as increasing the threshold for postoperative admission, may have had a greater effect on low- versus high-risk patients in low-risk hospitalizations. However, once a patient is hospitalized, multidisciplinary strategies appear to be effective at reducing readmissions for all risk classes in these cohorts.
For the 3 cohorts in which we observed an increase in readmission risk among hospitalized patients, the risk appeared to increase in early 2011. This time was about 10 months after passage of ACA, the timing of which was previously associated with a drop in readmission rates,7,8 but well before HRRP went into effect in October 2012. The increase in readmission risk coincided with an increase in the number of diagnostic codes that could be included on a hospital claim to Medicare.17 This increase in allowable codes allowed us to capture more diagnoses for some patients, potentially resulting in an increase in apparent predicted risk of readmissions. While we adjusted for this in our predictive models, we may not have fully accounted for differences in risk related to coding change. As a result, some of the observed differences in risk in our study may be attributable to coding differences. More broadly, studies demonstrating the success of HRRP have typically examined risk-adjusted rates of readmission.3,7 It is possible that a small portion of the observed reduction in risk-adjusted readmission rates may be related to the increase in predicted risk of readmission observed in our study. Future assessment of trends in readmission during this period should consider accounting for change in the number of allowed billing codes.
Other limitations should be considered in the interpretation of this study. First, like many predictive models for readmission,14 ours had imperfect discrimination, which could affect our results. Second, our study was based on older Medicare patients, so findings may not be applicable to younger patients. Third, while we accounted for surrogates for socioeconomic status, including dual eligibility and race, our models lacked other socioeconomic and community factors that can influence readmission.24-26 Nonetheless, 1 study suggested that easily measured socioeconomic factors may not have a strong influence on the readmission metric used by Medicare.28 Fourth, while our study included over 47 million hospitalizations, our time trend analyses used calendar month as the primary independent variable. As our study included 77 months, we may not have had sufficient power to detect small changes in risk over time.
Medicare readmissions have declined steadily in recent years, presumably at least partly in response to policy changes including HRRP. We found that hospitals have been effective at reducing readmissions across a range of patient risk strata and clinical conditions. As a result, the overall risk of readmission for hospitalized patients has remained constant for some but not all conditions. Whether institutions can continue to reduce readmission rates for most types of patients remains to be seen.
Acknowledgments
This study was supported by the Agency for Healthcare Research and Quality (AHRQ) grant R01HS022882. Dr. Blecker was supported by the AHRQ grant K08HS23683. The authors would like to thank Shawn Hoke and Jane Padikkala for administrative support.
Disclosure
This study was supported by the Agency for Healthcare Research and Quality (AHRQ) grants R01HS022882 and K08HS23683. The authors have no conflicts to report.
1. Jha AK. Seeking Rational Approaches to Fixing Hospital Readmissions. JAMA. 2015;314(16):1681-1682. PubMed
2. Centers for Medicare & Medicaid Services. Readmissions Reduction Program. https://www.cms.gov/Medicare/Medicare-Fee-for-Service-Payment/AcuteInpatientPPS/Readmissions-Reduction-Program.html. Accessed on January 17, 2017.
3. Suter LG, Li SX, Grady JN, et al. National patterns of risk-standardized mortality and readmission after hospitalization for acute myocardial infarction, heart failure, and pneumonia: update on publicly reported outcomes measures based on the 2013 release. J Gen Intern Med. 2014;29(10):1333-1340. PubMed
4. Gerhardt G, Yemane A, Hickman P, Oelschlaeger A, Rollins E, Brennan N. Medicare readmission rates showed meaningful decline in 2012. Medicare Medicaid Res Rev. 2013;3(2):pii:mmrr.003.02.b01. PubMed
5. Centers for Medicare and Medicaid Services. New Data Shows Affordable Care Act Reforms Are Leading to Lower Hospital Readmission Rates for Medicare Beneficiaries. http://blog.cms.gov/2013/12/06/new-data-shows-affordable-care-act-reforms-are-leading-to-lower-hospital-readmission-rates-for-medicare-beneficiaries/. Accessed on January 17, 2017.
6. Krumholz HM, Normand SL, Wang Y. Trends in hospitalizations and outcomes for acute cardiovascular disease and stroke, 1999-2011. Circulation. 2014;130(12):966-975. PubMed
7. Zuckerman RB, Sheingold SH, Orav EJ, Ruhter J, Epstein AM. Readmissions, Observation, and the Hospital Readmissions Reduction Program. N Engl J Med. 2016;374(16):1543-1551. PubMed
8. Desai NR, Ross JS, Kwon JY, et al. Association Between Hospital Penalty Status Under the Hospital Readmission Reduction Program and Readmission Rates for Target and Nontarget Conditions. JAMA. 2016;316(24):2647-2656. PubMed
9. Brock J, Mitchell J, Irby K, et al. Association between quality improvement for care transitions in communities and rehospitalizations among Medicare beneficiaries. JAMA. 2013;309(4):381-391. PubMed
10. Jencks S. Protecting Hospitals That Improve Population Health. http://medicaring.org/2014/12/16/protecting-hospitals/. Accessed on January 5, 2017.
11. Dharmarajan K, Qin L, Lin Z, et al. Declining Admission Rates And Thirty-Day Readmission Rates Positively Associated Even Though Patients Grew Sicker Over Time. Health Aff (Millwood). 2016;35(7):1294-1302. PubMed
12. Krumholz HM, Nuti SV, Downing NS, Normand SL, Wang Y. Mortality, Hospitalizations, and Expenditures for the Medicare Population Aged 65 Years or Older, 1999-2013. JAMA. 2015;314(4):355-365. PubMed
13. Amarasingham R, Moore BJ, Tabak YP, et al. An automated model to identify heart failure patients at risk for 30-day readmission or death using electronic medical record data. Med Care. 2010;48(11):981-988. PubMed
14. Kansagara D, Englander H, Salanitro A, et al. Risk prediction models for hospital readmission: a systematic review. JAMA. 2011;306(15):1688-1698. PubMed
15. Horwitz LI, Partovian C, Lin Z, et al. Development and use of an administrative claims measure for profiling hospital-wide performance on 30-day unplanned readmission. Ann Intern Med. 2014;161(10 Suppl):S66-S75. PubMed
16. 2016 Condition-Specific Measures Updates and Specifications Report Hospital-Level 30-Day Risk-Standardized Readmission Measures. https://www.cms.gov/Medicare/Quality-Initiatives-Patient-Assessment-Instruments/HospitalQualityInits/Downloads/AMI-HF-PN-COPD-and-Stroke-Readmission-Updates.zip. Accessed on January 19, 2017.
17. Centers for Medicare & Medicaid Services. Pub 100-04 Medicare Claims Processing, Transmittal 2028. https://www.cms.gov/Regulations-and-Guidance/Guidance/Transmittals/downloads/R2028CP.pdf. Accessed on November 28, 2016.
18. Martens FK, Tonk EC, Kers JG, Janssens AC. Small improvement in the area under the receiver operating characteristic curve indicated small changes in predicted risks. J Clin Epidemiol. 2016;79:159-164. PubMed
19. Blecker S, Goldfeld K, Park H, et al. Impact of an Intervention to Improve Weekend Hospital Care at an Academic Medical Center: An Observational Study. J Gen Intern Med. 2015;30(11):1657-1664. PubMed
20. Hansen LO, Young RS, Hinami K, Leung A, Williams MV. Interventions to reduce 30-day rehospitalization: a systematic review. Ann Intern Med. 2011;155(8):520-528. PubMed
21. Cavanaugh JJ, Jones CD, Embree G, et al. Implementation Science Workshop: primary care-based multidisciplinary readmission prevention program. J Gen Intern Med. 2014;29(5):798-804. PubMed
22. Jenq GY, Doyle MM, Belton BM, Herrin J, Horwitz LI. Quasi-Experimental Evaluation of the Effectiveness of a Large-Scale Readmission Reduction Program. JAMA Intern Med. 2016;176(5):681-690. PubMed
23. Lynn J, Jencks S. A Dangerous Malfunction in the Measure of Readmission Reduction. http://medicaring.org/2014/08/26/malfunctioning-metrics/. Accessed on January 17, 2017.
24. Calvillo-King L, Arnold D, Eubank KJ, et al. Impact of social factors on risk of readmission or mortality in pneumonia and heart failure: systematic review. J Gen Intern Med. 2013;28(2):269-282. PubMed
25. Barnett ML, Hsu J, McWilliams JM. Patient Characteristics and Differences in Hospital Readmission Rates. JAMA Intern Med. 2015;175(11):1803-1812. PubMed
26. Singh S, Lin YL, Kuo YF, Nattinger AB, Goodwin JS. Variation in the risk of readmission among hospitals: the relative contribution of patient, hospital and inpatient provider characteristics. J Gen Intern Med. 2014;29(4):572-578. PubMed
27. American Hospital Association. American Hospital Association (AHA) Detailed Comments on the Inpatient Prospective Payment System (PPS) Proposed Rule for Fiscal Year (FY) 2016. http://www.aha.org/advocacy-issues/letter/2015/150616-cl-cms1632-p-ipps.pdf. Accessed on January 10, 2017.
28. Bernheim SM, Parzynski CS, Horwitz L, et al. Accounting For Patients’ Socioeconomic Status Does Not Change Hospital Readmission Rates. Health Aff (Millwood). 2016;35(8):1461-1470. PubMed
Given the high cost of readmissions to the healthcare system, there has been a substantial push to reduce readmissions by policymakers.1 Among these is the Hospital Readmissions Reduction Program (HRRP), in which hospitals with higher than expected readmission rates receive reduced payments from Medicare.2 Recent evidence has suggested the success of such policy changes, with multiple reports demonstrating a decrease in 30-day readmission rates in the Medicare population starting in 2010.3-8
Initiatives to reduce readmissions can also have an effect on total number of admissions.9,10 Indeed, along with the recent reduction in readmission, there has been a reduction in all admissions among Medicare beneficiaries.11,12 Some studies have found that as admissions have decreased, the burden of comorbidity has increased among hospitalized patients,3,11 suggesting that hospitals may be increasingly filled with patients at high risk of readmission. However, whether readmission risk among hospitalized patients has changed remains unknown, and understanding changes in risk profile could help inform which patients to target with future interventions to reduce readmissions.
Hospital efforts to reduce readmissions may have differential effects on types of patients by risk. For instance, low-intensity, system-wide interventions such as standardized discharge instructions or medicine reconciliation may have a stronger effect on patients at relatively low risk of readmission who may have a few important drivers of readmission that are easily overcome. Alternatively, the impact of intensive care transitions management might be greatest for high-risk patients, who have the most need for postdischarge medications, follow-up, and self-care.
The purpose of this study was therefore twofold: (1) to observe changes in average monthly risk of readmission among hospitalized Medicare patients and (2) to examine changes in readmission rates for Medicare patients at various risk of readmission. We hypothesized that readmission risk in the Medicare population would increase in recent years, as overall number of admissions and readmissions have fallen.7,11 Additionally, we hypothesized that standardized readmission rates would decline less in highest risk patients as compared with the lowest risk patients because transitional care interventions may not be able to mitigate the large burden of comorbidity and social issues present in many high-risk patients.13,14
METHODS
We performed a retrospective cohort study of hospitalizations to US nonfederal short-term acute care facilities by Medicare beneficiaries between January 2009 and June 2015. The design involved 4 steps. First, we estimated a predictive model for unplanned readmissions within 30 days of discharge. Second, we assigned each hospitalization a predicted risk of readmission based on the model. Third, we studied trends in mean predicted risk of readmission during the study period. Fourth, we examined trends in observed to expected (O/E) readmission for hospitalizations in the lowest, middle, and highest categories of predicted risk of readmission to determine whether reductions in readmissions were more substantial in certain risk groups than in others.
Data were obtained from the Centers for Medicare and Medicaid Services (CMS) Inpatient Standard Analytic File and the Medicare Enrollment Data Base. We included hospitalizations of fee-for-service Medicare beneficiaries age ≥65 with continuous enrollment in Part A Medicare fee-for-service for at least 1 year prior and 30 days after the hospitalization.15 Hospitalizations with a discharge disposition of death, transfer to another acute hospital, and left against medical advice (AMA) were excluded. We also excluded patients with enrollment in hospice care prior to hospitalization. We excluded hospitalizations in June 2012 because of an irregularity in data availability for that month.
Hospitalizations were categorized into 5 specialty cohorts according to service line. The 5 cohorts were those used for the CMS hospital-wide readmission measure and included surgery/gynecology, medicine, cardiovascular, cardiorespiratory, and neurology.15 Among the 3 clinical conditions tracked as part of HRRP, heart failure and pneumonia were a subset of the cardiorespiratory cohort, while acute myocardial infarction was a subset of the cardiovascular cohort. Our use of cohorts was threefold: first, the average risk of readmission differs substantially across these cohorts, so pooling them produces heterogeneous risk strata; second, risk variables perform differently in different cohorts, so one single model may not be as accurate for calculating risk; and, third, the use of disease cohorts makes our results comparable to the CMS model and similar to other readmission studies in Medicare.7,8,15
For development of the risk model, the outcome was 30-day unplanned hospital readmission. Planned readmissions were excluded; these were defined by the CMS algorithm as readmissions in which a typically planned procedure occurred in a hospitalization with a nonacute principal diagnosis.16 Independent variables included age and comorbidities in the final hospital-wide readmission models for each of the 5 specialty cohorts.15 In order to produce the best possible individual risk prediction for each patient, we added additional independent variables that CMS avoids for hospital quality measurement purposes but that contribute to risk of readmission: sex, race, dual eligibility status, number of prior AMA discharges, intensive care unit stay during current hospitalization, coronary care unit stay during current hospitalization, and hospitalization in the prior 30, 90, and 180 days. We also included an indicator variable for hospitalizations with more than 9 discharge diagnosis codes on or after January 2011, the time at which Medicare allowed an increase of the number of International Classification of Diseases, 9th Revision-Clinical Modification diagnosis billing codes from 9 to 25.17 This indicator adjusts for the increased availability of comorbidity codes, which might otherwise inflate the predicted risk relative to hospitalizations prior to that date.
Based on the risk models, each hospitalization was assigned a predicted risk of readmission. For each specialty cohort, we pooled all hospitalizations across all study years and divided them into risk quintiles. We categorized hospitalizations as high risk if in the highest quintile, medium risk if in the middle 3 quintiles, and low risk if in the lowest quintile of predicted risk for all study hospitalizations in a given specialty cohort.
For our time trend analyses, we studied 2 outcomes: monthly mean predicted risk and monthly ratio of observed readmissions to expected readmissions for patients in the lowest, middle, and highest categories of predicted risk of readmission. We studied monthly predicted risk to determine whether the average readmission risk of patients was changing over time as admission and readmission rates were declining. We studied the ratio of O/E readmissions to determine whether the decline in overall readmissions was more substantial in particular risk strata; we used the ratio of O/E readmissions, which measures number of readmissions divided by number of readmissions predicted by the model, rather than crude observed readmissions, as O/E readmissions account for any changes in risk profiles over time within each risk stratum. Independent variables in our trend analyses were year—entered as a continuous variable—and indicators for postintroduction of the Affordable Care Act (ACA, March 2010) and for postintroduction of HRRP (October 2012); these time indicators were included because of prior studies demonstrating that the introduction of ACA was associated with a decrease from baseline in readmission rates, which leveled off after introduction of HRRP.7 We also included an indicator for calendar quarter to account for seasonal effects.
Statistical Analysis
We developed generalized estimating equation models to predict 30-day unplanned readmission for each of the 5 specialty cohorts. The 5 models were fit using all patients in each cohort for the included time period and were adjusted for clustering by hospital. We assessed discrimination by calculating area under the receiver operating characteristic curve (AUC) for the 5 models; the AUCs measured the models’ ability to distinguish patients who were readmitted versus those who were not.18 We also calculated AUCs for each year to examine model performance over time.
Using these models, we calculated predicted risk for each hospitalization and averaged these to obtain mean predicted risk for each specialty cohort for each month. To test for trends in mean risk, we estimated 5 time series models, one for each cohort, with the dependent variable of monthly mean predicted risk. For each cohort, we first estimated a series of 12 empty autoregressive models, each with a different autoregressive term (1, 2...12). For each model, we calculated χ2 for the test that the autocorrelation was 0; based on a comparison of chi-squared values, we specified an autocorrelation of 1 month for all models. Accordingly, a 1-month lag was used to estimate one final model for each cohort. Independent variables included year and indicators for post-ACA and post-HRRP; these variables captured the effect of trends over time and the introduction of these policy changes, respectively.19
To determine whether changes in risk over time were a result of changes in particular risk groups, we categorized hospitalizations into risk strata based on quintiles of predicted risk for each specialty cohort for the entire study period. For each individual year, we calculated the proportion of hospitalizations in the highest, middle, and lowest readmission risk strata for each cohort.
We calculated the monthly ratio of O/E readmission for hospitalizations in the lowest 20%, middle 60%, and highest 20% of readmission risk by month; O/E reflects the excess or deficit observed events relative to the number predicted by the model. Using this monthly O/E as the dependent variable, we developed autoregressive time series models as above, again with a 1-month lag, for each of these 3 risk strata in each cohort. As before, independent variables were year as a continuous variable, indicator variables for post-ACA and post-HRRP, and a categorical variable for calendar quarter.
All analyses were done in SAS version 9.3 (SAS Institute Inc., Cary, NC) and Stata version 14.2 (StataCorp LLC, College Station, TX).
RESULTS
We included 47,288,961 hospitalizations in the study, of which 11,231,242 (23.8%) were in the surgery/gynecology cohort, 19,548,711 (41.3%) were in the medicine cohort, 5,433,125 (11.5%) were in the cardiovascular cohort, 8,179,691 (17.3%) were in the cardiorespiratory cohort, and 2,896,192 (6.1%) were in the neurology cohort. The readmission rate was 16.2% (n = 7,642,161) overall, with the highest rates observed in the cardiorespiratory (20.5%) and medicine (17.6%) cohorts and the lowest rates observed in the surgery/gynecology (11.8%) and neurology (13.8%) cohorts.
The final predictive models for each cohort ranged in number of parameters from 56 for the cardiorespiratory cohort to 264 for the surgery/gynecology cohort. The models had AUCs of 0.70, 0.65, 0.67, 0.65, and 0.63 for the surgery/gynecology, medicine, cardiovascular, cardiorespiratory, and neurology cohorts, respectively; AUCs remained fairly stable over time for all disease cohorts (Appendix Table 1).
DISCUSSION
A number of mechanisms may account for the across-the-board improvements in readmission reduction. Many hospitals have instituted system-wide interventions, including patient education, medicine reconciliation, and early postdischarge follow-up,20 which may have reduced readmissions across all patient risk strata. Alternatively, hospitals may have implemented interventions that disproportionally benefited low-risk patients while simultaneously utilizing interventions that only benefited high-risk patients. For instance, increasing threshold for admission7 may have the greatest effect on low-risk patients who could be most easily managed at home, while many intensive transitional care interventions have been developed to target only high-risk patients.21,22
With the introduction of HRRP, there have been a number of concerns about the readmission measure used to penalize hospitals for high readmission rates. One major concern has been that the readmission metric may be flawed in its ability to capture continued improvement related to readmission.23 Some have suggested that with better population health management, admissions will decrease, patient risk of the remaining patients will increase, and hospitals will be increasingly filled with patients who have high likelihood of readmission. This potential for increased risk with HRRP was suggested by a recent study that found that comorbidities increased in hospitalized Medicare beneficiaries between 2010 and 2013.11 Our results were mixed in supporting this potential phenomenon because we examined global risk of readmission and found that some of the cohorts had increased risk over time while others did not. Others have expressed concern that readmission measure does not account for socioeconomic status, which has been associated with readmission rates.24-27 Although we did not directly examine socioeconomic status in our study, we found that hospitals have been able to reduce readmission across all levels of risk, which includes markers of socioeconomic status, including race and Medicaid eligibility status.
Although we hypothesized that readmission risk would increase as number of hospitalizations decreased over time, we found no increase in readmission risk among the cohorts with HRRP diagnoses that had the largest decrease in readmission rates.7,8 Conversely, readmission risk did increase—with a concurrent increase in the proportion of high-risk hospitalizations—in the surgery/gynecology and neurology cohorts that were not subject to HRRP penalties. Nonetheless, rehospitalizations were reduced for all risk categories in these 2 cohorts. Notably, surgery/gynecology and neurology had the lowest readmission rates overall. These findings suggest that initiatives to prevent initial hospitalizations, such as increasing the threshold for postoperative admission, may have had a greater effect on low- versus high-risk patients in low-risk hospitalizations. However, once a patient is hospitalized, multidisciplinary strategies appear to be effective at reducing readmissions for all risk classes in these cohorts.
For the 3 cohorts in which we observed an increase in readmission risk among hospitalized patients, the risk appeared to increase in early 2011. This time was about 10 months after passage of ACA, the timing of which was previously associated with a drop in readmission rates,7,8 but well before HRRP went into effect in October 2012. The increase in readmission risk coincided with an increase in the number of diagnostic codes that could be included on a hospital claim to Medicare.17 This increase in allowable codes allowed us to capture more diagnoses for some patients, potentially resulting in an increase in apparent predicted risk of readmissions. While we adjusted for this in our predictive models, we may not have fully accounted for differences in risk related to coding change. As a result, some of the observed differences in risk in our study may be attributable to coding differences. More broadly, studies demonstrating the success of HRRP have typically examined risk-adjusted rates of readmission.3,7 It is possible that a small portion of the observed reduction in risk-adjusted readmission rates may be related to the increase in predicted risk of readmission observed in our study. Future assessment of trends in readmission during this period should consider accounting for change in the number of allowed billing codes.
Other limitations should be considered in the interpretation of this study. First, like many predictive models for readmission,14 ours had imperfect discrimination, which could affect our results. Second, our study was based on older Medicare patients, so findings may not be applicable to younger patients. Third, while we accounted for surrogates for socioeconomic status, including dual eligibility and race, our models lacked other socioeconomic and community factors that can influence readmission.24-26 Nonetheless, 1 study suggested that easily measured socioeconomic factors may not have a strong influence on the readmission metric used by Medicare.28 Fourth, while our study included over 47 million hospitalizations, our time trend analyses used calendar month as the primary independent variable. As our study included 77 months, we may not have had sufficient power to detect small changes in risk over time.
Medicare readmissions have declined steadily in recent years, presumably at least partly in response to policy changes including HRRP. We found that hospitals have been effective at reducing readmissions across a range of patient risk strata and clinical conditions. As a result, the overall risk of readmission for hospitalized patients has remained constant for some but not all conditions. Whether institutions can continue to reduce readmission rates for most types of patients remains to be seen.
Acknowledgments
This study was supported by the Agency for Healthcare Research and Quality (AHRQ) grant R01HS022882. Dr. Blecker was supported by the AHRQ grant K08HS23683. The authors would like to thank Shawn Hoke and Jane Padikkala for administrative support.
Disclosure
This study was supported by the Agency for Healthcare Research and Quality (AHRQ) grants R01HS022882 and K08HS23683. The authors have no conflicts to report.
Given the high cost of readmissions to the healthcare system, there has been a substantial push to reduce readmissions by policymakers.1 Among these is the Hospital Readmissions Reduction Program (HRRP), in which hospitals with higher than expected readmission rates receive reduced payments from Medicare.2 Recent evidence has suggested the success of such policy changes, with multiple reports demonstrating a decrease in 30-day readmission rates in the Medicare population starting in 2010.3-8
Initiatives to reduce readmissions can also have an effect on total number of admissions.9,10 Indeed, along with the recent reduction in readmission, there has been a reduction in all admissions among Medicare beneficiaries.11,12 Some studies have found that as admissions have decreased, the burden of comorbidity has increased among hospitalized patients,3,11 suggesting that hospitals may be increasingly filled with patients at high risk of readmission. However, whether readmission risk among hospitalized patients has changed remains unknown, and understanding changes in risk profile could help inform which patients to target with future interventions to reduce readmissions.
Hospital efforts to reduce readmissions may have differential effects on types of patients by risk. For instance, low-intensity, system-wide interventions such as standardized discharge instructions or medicine reconciliation may have a stronger effect on patients at relatively low risk of readmission who may have a few important drivers of readmission that are easily overcome. Alternatively, the impact of intensive care transitions management might be greatest for high-risk patients, who have the most need for postdischarge medications, follow-up, and self-care.
The purpose of this study was therefore twofold: (1) to observe changes in average monthly risk of readmission among hospitalized Medicare patients and (2) to examine changes in readmission rates for Medicare patients at various risk of readmission. We hypothesized that readmission risk in the Medicare population would increase in recent years, as overall number of admissions and readmissions have fallen.7,11 Additionally, we hypothesized that standardized readmission rates would decline less in highest risk patients as compared with the lowest risk patients because transitional care interventions may not be able to mitigate the large burden of comorbidity and social issues present in many high-risk patients.13,14
METHODS
We performed a retrospective cohort study of hospitalizations to US nonfederal short-term acute care facilities by Medicare beneficiaries between January 2009 and June 2015. The design involved 4 steps. First, we estimated a predictive model for unplanned readmissions within 30 days of discharge. Second, we assigned each hospitalization a predicted risk of readmission based on the model. Third, we studied trends in mean predicted risk of readmission during the study period. Fourth, we examined trends in observed to expected (O/E) readmission for hospitalizations in the lowest, middle, and highest categories of predicted risk of readmission to determine whether reductions in readmissions were more substantial in certain risk groups than in others.
Data were obtained from the Centers for Medicare and Medicaid Services (CMS) Inpatient Standard Analytic File and the Medicare Enrollment Data Base. We included hospitalizations of fee-for-service Medicare beneficiaries age ≥65 with continuous enrollment in Part A Medicare fee-for-service for at least 1 year prior and 30 days after the hospitalization.15 Hospitalizations with a discharge disposition of death, transfer to another acute hospital, and left against medical advice (AMA) were excluded. We also excluded patients with enrollment in hospice care prior to hospitalization. We excluded hospitalizations in June 2012 because of an irregularity in data availability for that month.
Hospitalizations were categorized into 5 specialty cohorts according to service line. The 5 cohorts were those used for the CMS hospital-wide readmission measure and included surgery/gynecology, medicine, cardiovascular, cardiorespiratory, and neurology.15 Among the 3 clinical conditions tracked as part of HRRP, heart failure and pneumonia were a subset of the cardiorespiratory cohort, while acute myocardial infarction was a subset of the cardiovascular cohort. Our use of cohorts was threefold: first, the average risk of readmission differs substantially across these cohorts, so pooling them produces heterogeneous risk strata; second, risk variables perform differently in different cohorts, so one single model may not be as accurate for calculating risk; and, third, the use of disease cohorts makes our results comparable to the CMS model and similar to other readmission studies in Medicare.7,8,15
For development of the risk model, the outcome was 30-day unplanned hospital readmission. Planned readmissions were excluded; these were defined by the CMS algorithm as readmissions in which a typically planned procedure occurred in a hospitalization with a nonacute principal diagnosis.16 Independent variables included age and comorbidities in the final hospital-wide readmission models for each of the 5 specialty cohorts.15 In order to produce the best possible individual risk prediction for each patient, we added additional independent variables that CMS avoids for hospital quality measurement purposes but that contribute to risk of readmission: sex, race, dual eligibility status, number of prior AMA discharges, intensive care unit stay during current hospitalization, coronary care unit stay during current hospitalization, and hospitalization in the prior 30, 90, and 180 days. We also included an indicator variable for hospitalizations with more than 9 discharge diagnosis codes on or after January 2011, the time at which Medicare allowed an increase of the number of International Classification of Diseases, 9th Revision-Clinical Modification diagnosis billing codes from 9 to 25.17 This indicator adjusts for the increased availability of comorbidity codes, which might otherwise inflate the predicted risk relative to hospitalizations prior to that date.
Based on the risk models, each hospitalization was assigned a predicted risk of readmission. For each specialty cohort, we pooled all hospitalizations across all study years and divided them into risk quintiles. We categorized hospitalizations as high risk if in the highest quintile, medium risk if in the middle 3 quintiles, and low risk if in the lowest quintile of predicted risk for all study hospitalizations in a given specialty cohort.
For our time trend analyses, we studied 2 outcomes: monthly mean predicted risk and monthly ratio of observed readmissions to expected readmissions for patients in the lowest, middle, and highest categories of predicted risk of readmission. We studied monthly predicted risk to determine whether the average readmission risk of patients was changing over time as admission and readmission rates were declining. We studied the ratio of O/E readmissions to determine whether the decline in overall readmissions was more substantial in particular risk strata; we used the ratio of O/E readmissions, which measures number of readmissions divided by number of readmissions predicted by the model, rather than crude observed readmissions, as O/E readmissions account for any changes in risk profiles over time within each risk stratum. Independent variables in our trend analyses were year—entered as a continuous variable—and indicators for postintroduction of the Affordable Care Act (ACA, March 2010) and for postintroduction of HRRP (October 2012); these time indicators were included because of prior studies demonstrating that the introduction of ACA was associated with a decrease from baseline in readmission rates, which leveled off after introduction of HRRP.7 We also included an indicator for calendar quarter to account for seasonal effects.
Statistical Analysis
We developed generalized estimating equation models to predict 30-day unplanned readmission for each of the 5 specialty cohorts. The 5 models were fit using all patients in each cohort for the included time period and were adjusted for clustering by hospital. We assessed discrimination by calculating area under the receiver operating characteristic curve (AUC) for the 5 models; the AUCs measured the models’ ability to distinguish patients who were readmitted versus those who were not.18 We also calculated AUCs for each year to examine model performance over time.
Using these models, we calculated predicted risk for each hospitalization and averaged these to obtain mean predicted risk for each specialty cohort for each month. To test for trends in mean risk, we estimated 5 time series models, one for each cohort, with the dependent variable of monthly mean predicted risk. For each cohort, we first estimated a series of 12 empty autoregressive models, each with a different autoregressive term (1, 2...12). For each model, we calculated χ2 for the test that the autocorrelation was 0; based on a comparison of chi-squared values, we specified an autocorrelation of 1 month for all models. Accordingly, a 1-month lag was used to estimate one final model for each cohort. Independent variables included year and indicators for post-ACA and post-HRRP; these variables captured the effect of trends over time and the introduction of these policy changes, respectively.19
To determine whether changes in risk over time were a result of changes in particular risk groups, we categorized hospitalizations into risk strata based on quintiles of predicted risk for each specialty cohort for the entire study period. For each individual year, we calculated the proportion of hospitalizations in the highest, middle, and lowest readmission risk strata for each cohort.
We calculated the monthly ratio of O/E readmission for hospitalizations in the lowest 20%, middle 60%, and highest 20% of readmission risk by month; O/E reflects the excess or deficit observed events relative to the number predicted by the model. Using this monthly O/E as the dependent variable, we developed autoregressive time series models as above, again with a 1-month lag, for each of these 3 risk strata in each cohort. As before, independent variables were year as a continuous variable, indicator variables for post-ACA and post-HRRP, and a categorical variable for calendar quarter.
All analyses were done in SAS version 9.3 (SAS Institute Inc., Cary, NC) and Stata version 14.2 (StataCorp LLC, College Station, TX).
RESULTS
We included 47,288,961 hospitalizations in the study, of which 11,231,242 (23.8%) were in the surgery/gynecology cohort, 19,548,711 (41.3%) were in the medicine cohort, 5,433,125 (11.5%) were in the cardiovascular cohort, 8,179,691 (17.3%) were in the cardiorespiratory cohort, and 2,896,192 (6.1%) were in the neurology cohort. The readmission rate was 16.2% (n = 7,642,161) overall, with the highest rates observed in the cardiorespiratory (20.5%) and medicine (17.6%) cohorts and the lowest rates observed in the surgery/gynecology (11.8%) and neurology (13.8%) cohorts.
The final predictive models for each cohort ranged in number of parameters from 56 for the cardiorespiratory cohort to 264 for the surgery/gynecology cohort. The models had AUCs of 0.70, 0.65, 0.67, 0.65, and 0.63 for the surgery/gynecology, medicine, cardiovascular, cardiorespiratory, and neurology cohorts, respectively; AUCs remained fairly stable over time for all disease cohorts (Appendix Table 1).
DISCUSSION
A number of mechanisms may account for the across-the-board improvements in readmission reduction. Many hospitals have instituted system-wide interventions, including patient education, medicine reconciliation, and early postdischarge follow-up,20 which may have reduced readmissions across all patient risk strata. Alternatively, hospitals may have implemented interventions that disproportionally benefited low-risk patients while simultaneously utilizing interventions that only benefited high-risk patients. For instance, increasing threshold for admission7 may have the greatest effect on low-risk patients who could be most easily managed at home, while many intensive transitional care interventions have been developed to target only high-risk patients.21,22
With the introduction of HRRP, there have been a number of concerns about the readmission measure used to penalize hospitals for high readmission rates. One major concern has been that the readmission metric may be flawed in its ability to capture continued improvement related to readmission.23 Some have suggested that with better population health management, admissions will decrease, patient risk of the remaining patients will increase, and hospitals will be increasingly filled with patients who have high likelihood of readmission. This potential for increased risk with HRRP was suggested by a recent study that found that comorbidities increased in hospitalized Medicare beneficiaries between 2010 and 2013.11 Our results were mixed in supporting this potential phenomenon because we examined global risk of readmission and found that some of the cohorts had increased risk over time while others did not. Others have expressed concern that readmission measure does not account for socioeconomic status, which has been associated with readmission rates.24-27 Although we did not directly examine socioeconomic status in our study, we found that hospitals have been able to reduce readmission across all levels of risk, which includes markers of socioeconomic status, including race and Medicaid eligibility status.
Although we hypothesized that readmission risk would increase as number of hospitalizations decreased over time, we found no increase in readmission risk among the cohorts with HRRP diagnoses that had the largest decrease in readmission rates.7,8 Conversely, readmission risk did increase—with a concurrent increase in the proportion of high-risk hospitalizations—in the surgery/gynecology and neurology cohorts that were not subject to HRRP penalties. Nonetheless, rehospitalizations were reduced for all risk categories in these 2 cohorts. Notably, surgery/gynecology and neurology had the lowest readmission rates overall. These findings suggest that initiatives to prevent initial hospitalizations, such as increasing the threshold for postoperative admission, may have had a greater effect on low- versus high-risk patients in low-risk hospitalizations. However, once a patient is hospitalized, multidisciplinary strategies appear to be effective at reducing readmissions for all risk classes in these cohorts.
For the 3 cohorts in which we observed an increase in readmission risk among hospitalized patients, the risk appeared to increase in early 2011. This time was about 10 months after passage of ACA, the timing of which was previously associated with a drop in readmission rates,7,8 but well before HRRP went into effect in October 2012. The increase in readmission risk coincided with an increase in the number of diagnostic codes that could be included on a hospital claim to Medicare.17 This increase in allowable codes allowed us to capture more diagnoses for some patients, potentially resulting in an increase in apparent predicted risk of readmissions. While we adjusted for this in our predictive models, we may not have fully accounted for differences in risk related to coding change. As a result, some of the observed differences in risk in our study may be attributable to coding differences. More broadly, studies demonstrating the success of HRRP have typically examined risk-adjusted rates of readmission.3,7 It is possible that a small portion of the observed reduction in risk-adjusted readmission rates may be related to the increase in predicted risk of readmission observed in our study. Future assessment of trends in readmission during this period should consider accounting for change in the number of allowed billing codes.
Other limitations should be considered in the interpretation of this study. First, like many predictive models for readmission,14 ours had imperfect discrimination, which could affect our results. Second, our study was based on older Medicare patients, so findings may not be applicable to younger patients. Third, while we accounted for surrogates for socioeconomic status, including dual eligibility and race, our models lacked other socioeconomic and community factors that can influence readmission.24-26 Nonetheless, 1 study suggested that easily measured socioeconomic factors may not have a strong influence on the readmission metric used by Medicare.28 Fourth, while our study included over 47 million hospitalizations, our time trend analyses used calendar month as the primary independent variable. As our study included 77 months, we may not have had sufficient power to detect small changes in risk over time.
Medicare readmissions have declined steadily in recent years, presumably at least partly in response to policy changes including HRRP. We found that hospitals have been effective at reducing readmissions across a range of patient risk strata and clinical conditions. As a result, the overall risk of readmission for hospitalized patients has remained constant for some but not all conditions. Whether institutions can continue to reduce readmission rates for most types of patients remains to be seen.
Acknowledgments
This study was supported by the Agency for Healthcare Research and Quality (AHRQ) grant R01HS022882. Dr. Blecker was supported by the AHRQ grant K08HS23683. The authors would like to thank Shawn Hoke and Jane Padikkala for administrative support.
Disclosure
This study was supported by the Agency for Healthcare Research and Quality (AHRQ) grants R01HS022882 and K08HS23683. The authors have no conflicts to report.
1. Jha AK. Seeking Rational Approaches to Fixing Hospital Readmissions. JAMA. 2015;314(16):1681-1682. PubMed
2. Centers for Medicare & Medicaid Services. Readmissions Reduction Program. https://www.cms.gov/Medicare/Medicare-Fee-for-Service-Payment/AcuteInpatientPPS/Readmissions-Reduction-Program.html. Accessed on January 17, 2017.
3. Suter LG, Li SX, Grady JN, et al. National patterns of risk-standardized mortality and readmission after hospitalization for acute myocardial infarction, heart failure, and pneumonia: update on publicly reported outcomes measures based on the 2013 release. J Gen Intern Med. 2014;29(10):1333-1340. PubMed
4. Gerhardt G, Yemane A, Hickman P, Oelschlaeger A, Rollins E, Brennan N. Medicare readmission rates showed meaningful decline in 2012. Medicare Medicaid Res Rev. 2013;3(2):pii:mmrr.003.02.b01. PubMed
5. Centers for Medicare and Medicaid Services. New Data Shows Affordable Care Act Reforms Are Leading to Lower Hospital Readmission Rates for Medicare Beneficiaries. http://blog.cms.gov/2013/12/06/new-data-shows-affordable-care-act-reforms-are-leading-to-lower-hospital-readmission-rates-for-medicare-beneficiaries/. Accessed on January 17, 2017.
6. Krumholz HM, Normand SL, Wang Y. Trends in hospitalizations and outcomes for acute cardiovascular disease and stroke, 1999-2011. Circulation. 2014;130(12):966-975. PubMed
7. Zuckerman RB, Sheingold SH, Orav EJ, Ruhter J, Epstein AM. Readmissions, Observation, and the Hospital Readmissions Reduction Program. N Engl J Med. 2016;374(16):1543-1551. PubMed
8. Desai NR, Ross JS, Kwon JY, et al. Association Between Hospital Penalty Status Under the Hospital Readmission Reduction Program and Readmission Rates for Target and Nontarget Conditions. JAMA. 2016;316(24):2647-2656. PubMed
9. Brock J, Mitchell J, Irby K, et al. Association between quality improvement for care transitions in communities and rehospitalizations among Medicare beneficiaries. JAMA. 2013;309(4):381-391. PubMed
10. Jencks S. Protecting Hospitals That Improve Population Health. http://medicaring.org/2014/12/16/protecting-hospitals/. Accessed on January 5, 2017.
11. Dharmarajan K, Qin L, Lin Z, et al. Declining Admission Rates And Thirty-Day Readmission Rates Positively Associated Even Though Patients Grew Sicker Over Time. Health Aff (Millwood). 2016;35(7):1294-1302. PubMed
12. Krumholz HM, Nuti SV, Downing NS, Normand SL, Wang Y. Mortality, Hospitalizations, and Expenditures for the Medicare Population Aged 65 Years or Older, 1999-2013. JAMA. 2015;314(4):355-365. PubMed
13. Amarasingham R, Moore BJ, Tabak YP, et al. An automated model to identify heart failure patients at risk for 30-day readmission or death using electronic medical record data. Med Care. 2010;48(11):981-988. PubMed
14. Kansagara D, Englander H, Salanitro A, et al. Risk prediction models for hospital readmission: a systematic review. JAMA. 2011;306(15):1688-1698. PubMed
15. Horwitz LI, Partovian C, Lin Z, et al. Development and use of an administrative claims measure for profiling hospital-wide performance on 30-day unplanned readmission. Ann Intern Med. 2014;161(10 Suppl):S66-S75. PubMed
16. 2016 Condition-Specific Measures Updates and Specifications Report Hospital-Level 30-Day Risk-Standardized Readmission Measures. https://www.cms.gov/Medicare/Quality-Initiatives-Patient-Assessment-Instruments/HospitalQualityInits/Downloads/AMI-HF-PN-COPD-and-Stroke-Readmission-Updates.zip. Accessed on January 19, 2017.
17. Centers for Medicare & Medicaid Services. Pub 100-04 Medicare Claims Processing, Transmittal 2028. https://www.cms.gov/Regulations-and-Guidance/Guidance/Transmittals/downloads/R2028CP.pdf. Accessed on November 28, 2016.
18. Martens FK, Tonk EC, Kers JG, Janssens AC. Small improvement in the area under the receiver operating characteristic curve indicated small changes in predicted risks. J Clin Epidemiol. 2016;79:159-164. PubMed
19. Blecker S, Goldfeld K, Park H, et al. Impact of an Intervention to Improve Weekend Hospital Care at an Academic Medical Center: An Observational Study. J Gen Intern Med. 2015;30(11):1657-1664. PubMed
20. Hansen LO, Young RS, Hinami K, Leung A, Williams MV. Interventions to reduce 30-day rehospitalization: a systematic review. Ann Intern Med. 2011;155(8):520-528. PubMed
21. Cavanaugh JJ, Jones CD, Embree G, et al. Implementation Science Workshop: primary care-based multidisciplinary readmission prevention program. J Gen Intern Med. 2014;29(5):798-804. PubMed
22. Jenq GY, Doyle MM, Belton BM, Herrin J, Horwitz LI. Quasi-Experimental Evaluation of the Effectiveness of a Large-Scale Readmission Reduction Program. JAMA Intern Med. 2016;176(5):681-690. PubMed
23. Lynn J, Jencks S. A Dangerous Malfunction in the Measure of Readmission Reduction. http://medicaring.org/2014/08/26/malfunctioning-metrics/. Accessed on January 17, 2017.
24. Calvillo-King L, Arnold D, Eubank KJ, et al. Impact of social factors on risk of readmission or mortality in pneumonia and heart failure: systematic review. J Gen Intern Med. 2013;28(2):269-282. PubMed
25. Barnett ML, Hsu J, McWilliams JM. Patient Characteristics and Differences in Hospital Readmission Rates. JAMA Intern Med. 2015;175(11):1803-1812. PubMed
26. Singh S, Lin YL, Kuo YF, Nattinger AB, Goodwin JS. Variation in the risk of readmission among hospitals: the relative contribution of patient, hospital and inpatient provider characteristics. J Gen Intern Med. 2014;29(4):572-578. PubMed
27. American Hospital Association. American Hospital Association (AHA) Detailed Comments on the Inpatient Prospective Payment System (PPS) Proposed Rule for Fiscal Year (FY) 2016. http://www.aha.org/advocacy-issues/letter/2015/150616-cl-cms1632-p-ipps.pdf. Accessed on January 10, 2017.
28. Bernheim SM, Parzynski CS, Horwitz L, et al. Accounting For Patients’ Socioeconomic Status Does Not Change Hospital Readmission Rates. Health Aff (Millwood). 2016;35(8):1461-1470. PubMed
1. Jha AK. Seeking Rational Approaches to Fixing Hospital Readmissions. JAMA. 2015;314(16):1681-1682. PubMed
2. Centers for Medicare & Medicaid Services. Readmissions Reduction Program. https://www.cms.gov/Medicare/Medicare-Fee-for-Service-Payment/AcuteInpatientPPS/Readmissions-Reduction-Program.html. Accessed on January 17, 2017.
3. Suter LG, Li SX, Grady JN, et al. National patterns of risk-standardized mortality and readmission after hospitalization for acute myocardial infarction, heart failure, and pneumonia: update on publicly reported outcomes measures based on the 2013 release. J Gen Intern Med. 2014;29(10):1333-1340. PubMed
4. Gerhardt G, Yemane A, Hickman P, Oelschlaeger A, Rollins E, Brennan N. Medicare readmission rates showed meaningful decline in 2012. Medicare Medicaid Res Rev. 2013;3(2):pii:mmrr.003.02.b01. PubMed
5. Centers for Medicare and Medicaid Services. New Data Shows Affordable Care Act Reforms Are Leading to Lower Hospital Readmission Rates for Medicare Beneficiaries. http://blog.cms.gov/2013/12/06/new-data-shows-affordable-care-act-reforms-are-leading-to-lower-hospital-readmission-rates-for-medicare-beneficiaries/. Accessed on January 17, 2017.
6. Krumholz HM, Normand SL, Wang Y. Trends in hospitalizations and outcomes for acute cardiovascular disease and stroke, 1999-2011. Circulation. 2014;130(12):966-975. PubMed
7. Zuckerman RB, Sheingold SH, Orav EJ, Ruhter J, Epstein AM. Readmissions, Observation, and the Hospital Readmissions Reduction Program. N Engl J Med. 2016;374(16):1543-1551. PubMed
8. Desai NR, Ross JS, Kwon JY, et al. Association Between Hospital Penalty Status Under the Hospital Readmission Reduction Program and Readmission Rates for Target and Nontarget Conditions. JAMA. 2016;316(24):2647-2656. PubMed
9. Brock J, Mitchell J, Irby K, et al. Association between quality improvement for care transitions in communities and rehospitalizations among Medicare beneficiaries. JAMA. 2013;309(4):381-391. PubMed
10. Jencks S. Protecting Hospitals That Improve Population Health. http://medicaring.org/2014/12/16/protecting-hospitals/. Accessed on January 5, 2017.
11. Dharmarajan K, Qin L, Lin Z, et al. Declining Admission Rates And Thirty-Day Readmission Rates Positively Associated Even Though Patients Grew Sicker Over Time. Health Aff (Millwood). 2016;35(7):1294-1302. PubMed
12. Krumholz HM, Nuti SV, Downing NS, Normand SL, Wang Y. Mortality, Hospitalizations, and Expenditures for the Medicare Population Aged 65 Years or Older, 1999-2013. JAMA. 2015;314(4):355-365. PubMed
13. Amarasingham R, Moore BJ, Tabak YP, et al. An automated model to identify heart failure patients at risk for 30-day readmission or death using electronic medical record data. Med Care. 2010;48(11):981-988. PubMed
14. Kansagara D, Englander H, Salanitro A, et al. Risk prediction models for hospital readmission: a systematic review. JAMA. 2011;306(15):1688-1698. PubMed
15. Horwitz LI, Partovian C, Lin Z, et al. Development and use of an administrative claims measure for profiling hospital-wide performance on 30-day unplanned readmission. Ann Intern Med. 2014;161(10 Suppl):S66-S75. PubMed
16. 2016 Condition-Specific Measures Updates and Specifications Report Hospital-Level 30-Day Risk-Standardized Readmission Measures. https://www.cms.gov/Medicare/Quality-Initiatives-Patient-Assessment-Instruments/HospitalQualityInits/Downloads/AMI-HF-PN-COPD-and-Stroke-Readmission-Updates.zip. Accessed on January 19, 2017.
17. Centers for Medicare & Medicaid Services. Pub 100-04 Medicare Claims Processing, Transmittal 2028. https://www.cms.gov/Regulations-and-Guidance/Guidance/Transmittals/downloads/R2028CP.pdf. Accessed on November 28, 2016.
18. Martens FK, Tonk EC, Kers JG, Janssens AC. Small improvement in the area under the receiver operating characteristic curve indicated small changes in predicted risks. J Clin Epidemiol. 2016;79:159-164. PubMed
19. Blecker S, Goldfeld K, Park H, et al. Impact of an Intervention to Improve Weekend Hospital Care at an Academic Medical Center: An Observational Study. J Gen Intern Med. 2015;30(11):1657-1664. PubMed
20. Hansen LO, Young RS, Hinami K, Leung A, Williams MV. Interventions to reduce 30-day rehospitalization: a systematic review. Ann Intern Med. 2011;155(8):520-528. PubMed
21. Cavanaugh JJ, Jones CD, Embree G, et al. Implementation Science Workshop: primary care-based multidisciplinary readmission prevention program. J Gen Intern Med. 2014;29(5):798-804. PubMed
22. Jenq GY, Doyle MM, Belton BM, Herrin J, Horwitz LI. Quasi-Experimental Evaluation of the Effectiveness of a Large-Scale Readmission Reduction Program. JAMA Intern Med. 2016;176(5):681-690. PubMed
23. Lynn J, Jencks S. A Dangerous Malfunction in the Measure of Readmission Reduction. http://medicaring.org/2014/08/26/malfunctioning-metrics/. Accessed on January 17, 2017.
24. Calvillo-King L, Arnold D, Eubank KJ, et al. Impact of social factors on risk of readmission or mortality in pneumonia and heart failure: systematic review. J Gen Intern Med. 2013;28(2):269-282. PubMed
25. Barnett ML, Hsu J, McWilliams JM. Patient Characteristics and Differences in Hospital Readmission Rates. JAMA Intern Med. 2015;175(11):1803-1812. PubMed
26. Singh S, Lin YL, Kuo YF, Nattinger AB, Goodwin JS. Variation in the risk of readmission among hospitals: the relative contribution of patient, hospital and inpatient provider characteristics. J Gen Intern Med. 2014;29(4):572-578. PubMed
27. American Hospital Association. American Hospital Association (AHA) Detailed Comments on the Inpatient Prospective Payment System (PPS) Proposed Rule for Fiscal Year (FY) 2016. http://www.aha.org/advocacy-issues/letter/2015/150616-cl-cms1632-p-ipps.pdf. Accessed on January 10, 2017.
28. Bernheim SM, Parzynski CS, Horwitz L, et al. Accounting For Patients’ Socioeconomic Status Does Not Change Hospital Readmission Rates. Health Aff (Millwood). 2016;35(8):1461-1470. PubMed
© 2018 Society of Hospital Medicine
“We’re almost guests in their clinical care”: Inpatient provider attitudes toward chronic disease management
Millions of individuals with chronic diseases are hospitalized annually in the United States. More than 90% of hospitalized adults have at least 1 chronic disease,1 and almost half of Medicare beneficiaries in the hospital have 4 or more chronic conditions.2 While many patients are admitted for worsening of a single chronic disease, patients are hospitalized more commonly for other causes. For instance, although acute heart failure is among the most frequent causes of hospitalizations among older adults, three-fourths of hospitalizations of patients with heart failure are for reasons other than acute heart failure.3
When a patient with a chronic disease is hospitalized, the inpatient provider must consider whether to actively or passively manage the chronic disease. Studies have suggested that intervening in chronic diseases during hospitalizations can lead to long-term improvement in treatment;4-6 for instance, stroke patients who were started on antihypertensive therapy at discharge were more likely to have their blood pressure controlled in the next year.5 However, some authors have argued that aggressive hypertension management by inpatient providers may result in patient harm.7 One case-based survey suggested that hospitalists were mixed in their interest in participating in chronic disease management in the hospital.8 This study found that providers were less likely to participate in chronic disease management if it was unrelated to the reason for hospitalization.8 However, to our knowledge, no studies have broadly evaluated inpatient provider attitudes, motivating factors, or barriers to participation in chronic disease management.
The purpose of this study was to understand provider attitudes towards chronic disease management for patients who are hospitalized for other causes. We were particularly interested in perceptions of barriers and facilitators to delivery of inpatient chronic disease management. Ultimately, such findings can inform future interventions to improve inpatient care of chronic disease.
METHODS
In this qualitative study, we conducted in-depth interviews with providers to understand attitudes, barriers, and facilitators towards inpatient management of chronic disease; this study was part of a larger study to implement an electronic health record-based clinical decision-support system intervention to improve quality of care for hospitalized patients with heart failure.
We included providers who care for and can write medication orders for hospitalized adult patients at New York University (NYU) Langone Medical Center, an urban academic medical center. As patients with chronic conditions are commonly hospitalized for many reasons, we sought to interview providers from a range of clinical services without consideration of factors such as frequency of caring for patients with heart failure. We used a purposive sampling framework: we invited participants to ensure a range of services, including medicine, surgery, and neurology, and provider types, including attending physicians, resident physicians, nurse practitioners, and physician assistants. Potential participants, therefore, included all providers for adult hospitalized patients.
We identified potential participants through study team members, referrals from department heads and prior interviewees, and e-mails to department list serves. We did not formally track declinations to being interviewed, although we estimate them as fewer than 20% of providers directly approached. While we focused on inpatient providers at New York University Langone Medical Center, many of the attending physicians and residents spend a portion of their time at the Manhattan Veterans Affairs Hospital and Bellevue Hospital, a safety-net city hospital; providers could have outpatient responsibilities as well.
All participants provided verbal consent to participate. The study was approved by the New York University Institutional Review Board, which granted a waiver of documentation of consent. Participants received a $25 gift card following the interview.
We used a semi-structured interview guide (Appendix) to elicit in-depth accounts of provider attitudes, experiences with, and barriers and facilitators towards chronic disease management in the hospital. The interview began by asking about chronic disease in general and then asked more specific questions about heart failure; we included responses to both groups of questions in the current study. The interview also included questions related to the clinical decision-support system being developed as part of the larger implementation study, although we do not report on these results in the current study. The semi-structured interview guide was informed by the consolidated framework for advancing implementation science (CFIR), which offers an overarching typology for delineating factors that influence guideline implementation;9 we also used CFIR constructs in theme development. We conducted in-depth interviews with providers.
A priori, we estimated 25 interviews would be sufficient to include the purposive sample and achieve data saturation,10 which was reached after 31 interviews. Interviews were held in person or by telephone, at the convenience of the subject. All interviews were transcribed by a professional service. Transcriptions were reviewed against recordings with any mistakes corrected. Prior to each interview, we conducted a brief demographic survey.
Qualitative data were analyzed using a constant comparative analytic technique.11 The investigative team met after reviewing the first 10 interviews and discussed emergent themes from these early transcripts, which led to the initial code list. Two investigators coded the transcripts. Reliability was evaluated by independent coding of a 20% subset of interviews. Differences were reviewed and discussed until consensus was reached. Final intercoder reliability was determined to be greater than 95%.12 All investigators reviewed and refined the code list during the analysis phase. Codes were clustered into themes based on CFIR constructs.9 Analyses were performed using Atlas.ti v. 7 (ATLAS.ti Scientific Software Development GmbH, Berlin, Germany).
RESULTS
We conducted interviews with 31 providers. Of these, 12 were on the medicine service, 12 were on the surgery or a surgical subspecialty service, and 7 were on other services; 11 were attending physicians, 12 were resident physicians, 5 were NPs, and 3 were PAs. Only 2 providers—an attending in medicine and a resident in surgery—had a specialty focus that was cardiac-related. Median time in current position was 4 years (Table 1). Seventeen of the interviews were in person, and 14 were conducted by telephone. The mean interview time was 20 minutes and ranged from 11 to 41 minutes.
We identified 5 main themes with 29 supporting codes (Table 2) describing provider attitudes towards the management of chronic disease for hospitalized patients. These themes, with related CFIR constructs, were: 1) perceived impact on patient outcomes (CFIR construct: intervention characteristics, relative advantage); 2) hospital structural characteristics (inner setting, structural characteristics); 3) provider knowledge and self-efficacy (characteristic of individual, knowledge and beliefs about the intervention and self-efficacy); 4) hospital priorities (inner setting, implementation climate, relative priority); and 5) continuity and communication (inner setting, networks and communications). For most themes, subjects described both positive and negative aspects of chronic disease management, as well as related facilitators and barriers to delivery of chronic disease care for hospitalized patients. Illustrative quotes for each theme are shown in Table 3.
Perceived Impact on Patient Outcomes
Perceived impact on patient outcomes was mixed. Most providers believed the management of chronic diseases could lead to improvement in important patient outcomes, including decreased length of stay (LOS), prevention of hospital complication, and decreased readmissions. Surgical providers focused particularly on the benefits of preventing surgical complications and noted that they were more likely to manage chronic conditions—primarily through use of specialist consultation—when they perceived a benefit to prevention of surgical outcomes or a fear that surgery may worsen a stable chronic condition:
“Most of the surgery I do is pretty stressful on the body and is very likely to induce acute on chronic exacerbations of heart failure. For someone with Class II or higher heart failure, I’m definitely gonna have cardiology on board or at least internal medicine on board right from the beginning.”
However, some providers acknowledged that there were potential risks to such management, including “prolonging hospital stays for nonemergent indications” and treatment with therapies that had previously led to an “adverse reaction that wasn’t clearly documented.” Providers were also concerned that treating chronic conditions may take focus away from acute conditions, which could lead to worse patient-centered outcomes. One attending in medicine described it:
“If you do potentially focus on those chronic issues, and there’s already a lot of other stuff going on with the patient, you might not be prioritizing the patient’s active issues appropriately. The patient’s saying, ‘I’m in pain. I’m in pain. I’m in pain,’ and you’re saying, ‘Thank you very much. Look, your heart failure, you didn’t get your beta-blocker.’ There could be a disconnect between patient’s goals, expectations, and your goals and expectations.”
Hospital Structural Characteristics
For many providers, the hospital setting provides a unique opportunity for care of patients with chronic disease. First, a hospitalization is a time for a patient’s management to be reviewed by a new care team. The hospital team reviews the management plan for patients at admission, which is a time to reevaluate whether patients are on evidence-based therapies: “It’s helpful to have a new set of eyes on somebody, like fresh information.” According to providers, this reevaluation can overcome instances of therapeutic inertia by the outpatient physician. Second, the hospital has many resources, including readily available specialist services and diagnostic tests, which can allow a patient-centered approach that coordinates care in 1 place, as a surgery NP described: “I think the advantage for the patient is that they wind up stopping in for 1 thing but we wind up taking care of a few without requiring the need for him or her to go to all these different specialists on the outside. They’re mostly elderly and not able to get around.” Third, the high availability of services and frequent monitoring allows rapid titration of evidence-based medicines, as discussed by a medicine resident: “It’s easier and faster to titrate medication—they’re in a monitored setting; you can ensure compliance.”
Patients may also differ from their usual state while hospitalized, creating both risks and benefits. The hospital setting can provide an opportunity to educate patients on their chronic disease(s) because they are motivated: “They’re in an office visit and their sugars are out of whack or something, they may take it a little bit more seriously if they were just in the hospital even though it was on an unrelated issue. I think it probably just changes their perspective on their disease.” However, in the hospital, patients are in an unusual environment with a restricted diet and forced medication compliance. Furthermore, the acute condition can lead to changes in their chronic disease, as described by 1 medicine attending: “their sugar is high because they’re acutely ill.” Providers expressed concern that changing medications in this setting may lead to adverse events (AEs) when patients return to their usual environment.
Provider Knowledge and Self-Efficacy
Insufficient knowledge of treatments for chronic conditions was cited as a barrier to some providers’ ability to actively manage chronic disease for hospitalized patients. Some providers described management of conditions outside their area as less satisfying than their primary focus. For example, an orthopedic surgeon explained: “…it’s very simple. You see your bone is broken, you fix it, that’s it…it’s intellectually satisfying…managing chronic diseases is less like that.” Reliance on consultants was 1 approach to deal with knowledge gaps in areas outside a provider’s expertise.
For a number of providers, management of stable chronic disease is the responsibility of the outpatient provider. Providers expressed concern that inpatient management was a reach into the domain of the primary care provider (PCP) and might take “away from the primary focus” of the hospitalization. Nonetheless, some providers noted an “ethical responsibility to manage [a] patient correctly,” and some providers believed that engaging in chronic disease management in the hospital would present an opportunity to expand their own expertise.
A few providers were worried about legal risk related to chronic disease management: “we don’t typically deal too much with managing some of these other medical issues for medical and legal reasons.” Providers again suggested that consults can help overcome this concern for risk, as discussed by 1 surgical attending: “We’re all not wanting to be sued, and we want to do the right thing. It costs me nothing to have a cardiologist on board, so like—why not.”
Hospital Priorities
Providers explained that the hospital has strong interests in early discharge and minimizing LOS. These priorities are based on goals of improving patient outcomes, increasing bed availability and hospital volume, and reducing costs. Providers perceive these hospital priorities as potential barriers to chronic disease management, which can increase LOS and costs through additional testing and treatment. As a medicine resident described: “The DBN philosophy, ‘discharge before noon’ philosophy, which is part of the hospital efficiency to get people in and out of the hospital as quickly as [is] safe, or maybe faster. And I think that there’s a culture where you’re encouraged to only focus on the acute issue and tend to defer everything else.”
Continuity and Communication
According to many providers, care continuity between the outpatient setting and the hospital played a major role in management of chronic disease. One barrier to starting a new evidence-based medication was lack of knowledge of patient history. As noted, providers expressed concern that a patient may not be on a given therapy because of an adverse reaction that was not documented in the hospital chart. This is particularly true because, as discussed by a surgery resident, patients with “PCPs outside the system [in which providers] don’t have access to the electronic medical record.” To overcome this barrier, providers attempt to communicate with the outpatient provider to confirm a lack of contraindications to therapies prior to any changes; notably, communication is easier if the inpatient provider has a relationship with the outpatient PCP.
Some providers were more likely to start chronic disease therapies if the patient had no prior outpatient care, because the provider was reassured that there was no rationale for missing therapies. One neurology attending noted that if a patient had newly documented “hypertension even if they were in for something else, I might start them on an antihypertensive, but then arrange for a close follow-up with a new PCP.”
Following hospitalization, providers wanted assurance that any changes to chronic disease management would be followed up by an outpatient physician. Any changes are relayed to the outpatient provider and the “level of communication…with the outpatient provider who’s gonna inherit” these changes can influence how aggressively the inpatient provider manages chronic diseases. Providers may be reluctant to start therapy for patients if they are concerned about outpatient follow up: “they have diabetes and they should really technically be on an ACE [angiotensin converting enzyme]inhibitor and aspirin, but they’re not. I might send them out on the aspirin but I might either start ACE inhibitor and have them follow up with their PCP in 2 weeks if I’m confident that they’ll do it or if I’m really confident that they’ll not follow up, I will help them get the appointment and then the discharge instruction is to the PCP is ‘Please start this patient on ACE inhibitor if they show up.’”
DISCUSSION
Providers frequently perceive benefit to chronic disease management in the hospital, including improvements in clinical outcomes. Notably, providers see opportunities to improve compliance with evidence-based care to overcome potential barriers to managing chronic disease in the outpatient setting, which can be limited by pressure for brief encounters,13 clinical inertia,14 difficulty with close monitoring of patients,15 and care fragmentation.16 Concurrently, inpatient providers are concerned about potential for patient harm related to chronic disease management, primarily related to AEs from medications. Similar to a case study about a patient with outpatient hypotension following aggressive inpatient hypertension management,7 providers fear that changing a patient’s chronic disease management in a hospital setting may cause harm when the patient returns home.
Although some clinicians have argued against aggressive in-hospital chronic disease management because of concerns for risk of AEs,7 our study and others8 have suggested that many clinicians perceive benefit. In some cases, such as smoking cessation counseling for all current smokers and prescribing an angiotensin converting enzyme inhibitor for patients with systolic heart failure, the perceived importance is so great that chronic disease management has been used as a national quality metric for hospitals. While these hospital metrics may be justified for short-term benefits after hospitalization, studies have demonstrated only weak improvement in short-term postdischarge outcomes related to chronic disease management.17 The true benefit is likely from improved processes of care in the short term that lead to long-term improvement in outcomes.4,5,18 Thus, the advantage of starting a patient hospitalized for a stroke on blood pressure medication is the increased likelihood that the patient will continue the medication as an outpatient, which may reduce long-term mortality.
For hospital delivery systems that are concerned with such care process improvement through in-hospital chronic disease management, we identified a number of barriers and facilitators to delivering this care. One significant barrier was poor transitions between the inpatient and the outpatient settings. When a patient transitions into the hospital, providers need to understand prior management choices. Facilitators to help inpatient providers understand prior management included either knowing the outpatient provider, or understanding that there was a lack of regular outpatient care; in both these cases, inpatient providers felt more comfortable managing chronic diseases because they had insight into the outpatient plan, or lack thereof. However, these facilitators may not be practical to incorporate in interventions to improve chronic disease care, which should consider overcoming these communication barriers. Use of shared electronic health records or standardized telephone calls with well-documented care plans obtained through health information exchanges may facilitate an inpatient provider to manage appropriately chronic disease. Similarly, discontinuity between the inpatient provider and the outpatient provider is a barrier that must be overcome to ease concerns that any chronic disease management changes do not result in harm in the postdischarge period. These findings again point to the need for improved documentation and communication between inpatient and outpatient providers. Of course, the transitional care period is one of high risk, and improving communication between providers has been an area of ongoing work.19
Lack of comfort among inpatient providers with managing chronic diseases is another important barrier, which appears to be largely overcome through the use of consultation services. Ready availability of specialists, common in academic medical centers, can facilitate delivery of chronic disease management. Inpatient interventions designed to improve evidence-based care for a chronic disease may benefit from involvement or at least availability of specialists in the effort. Another major barrier relates to hospital priorities, which in our study were closely aligned with external factors such as payment models. As hospitalizations are typically paid based on the discharge diagnosis, hospitals have incentives to discharge quickly and not order extra diagnostic tests. As a result, there are disincentives for chronic disease management that may require additional testing or monitoring in the hospital. Conversely, as hospitals accept postdischarge financial risks through readmission penalties or postdischarge cost savings, hospitals may perceive that long-term benefits of chronic disease management may outweigh short-term costs.
The study findings should be interpreted in the context of its limitations. Findings of our study of providers from a single academic medical center may not be generalizable. Nearly half of our interviews were conducted by telephone, which limits our ability to capture nonverbal cues in communication. Providers may have had social desirability bias towards positive aspects of chronic disease management. We did not have the power to determine differences in response by provider characteristic because this was an exploratory qualitative study. Future studies with representative sampling, a larger sample size, and measures for constructs such as provider self-efficacy are needed to examine differences by specialty, provider type, and experience level.
In conclusion, inpatient providers believe that hospital chronic disease management has the potential to be beneficial for both process of care and clinical outcomes; providers also express concern about potential adverse consequences of managing chronic disease during acute hospitalizations. To maximize both quality of care and patient safety, overcoming communication barriers between inpatient and outpatient providers is needed. Both a supportive hospital environment and availability of specialty support can facilitate in-hospital chronic disease management. Interventions that incorporate these factors may be well-suited to improve chronic disease care and long-term outcomes.
Disclosures
This work was supported by the Agency for Healthcare Research and Quality (AHRQ) grant K08HS23683. The authors report no financial conflicts of interest.
1. Friedman B, Jiang HJ, Elixhauser A, Segal A. Hospital inpatient costs for adults with multiple chronic conditions. Med Care Res Rev. 2006;63(3):327-346. PubMed
2. Steiner CA, Friedman B. Hospital utilization, costs, and mortality for adults with multiple chronic conditions, Nationwide Inpatient Sample, 2009. Prev Chronic Dis. 2013;10:E62. PubMed
3. Blecker S, Paul M, Taksler G, Ogedegbe G, Katz S. Heart failure-associated hospitalizations in the United States. J Am Coll Cardiol. 2013;61(12):1259-1267. PubMed
4. Fonarow GC. Role of in-hospital initiation of carvedilol to improve treatment rates and clinical outcomes. Am J Cardiol. 2004;93(9A):77B-81B. PubMed
5. Touze E, Coste J, Voicu M, et al. Importance of in-hospital initiation of therapies and therapeutic inertia in secondary stroke prevention: IMplementation of Prevention After a Cerebrovascular evenT (IMPACT) Study. Stroke. 2008;39(6):1834-1843. PubMed
6. Ovbiagele B, Saver JL, Fredieu A, et al. In-hospital initiation of secondary stroke prevention therapies yields high rates of adherence at follow-up. Stroke. 2004;35(12):2879-2883. PubMed
7. Steinman MA, Auerbach AD. Managing chronic disease in hospitalized patients. JAMA Intern Med. 2013;173(20):1857-1858. PubMed
8. Breu AC, Allen-Dicker J, Mueller S, Palamara K, Hinami K, Herzig SJ. Hospitalist and primary care physician perspectives on medication management of chronic conditions for hospitalized patients. J Hosp Med. 2014;9(5):303-309. PubMed
9. Damschroder LJ, Aron DC, Keith RE, Kirsh SR, Alexander JA, Lowery JC. Fostering implementation of health services research findings into practice: a consolidated framework for advancing implementation science. Implement Sci. 2009;4:50. PubMed
10. Morse JM. The significance of saturation. Qualitative Health Research. 1995;5(2):147-149.
11. Bradley EH, Curry LA, Devers KJ. Qualitative data analysis for health services research: developing taxonomy, themes, and theory. Health Services Research. 2007;42(4):1758-1772. PubMed
12. Riegel B, Dickson VV, Topaz M. Qualitative analysis of naturalistic decision making in adults with chronic heart failure. Nurs Res. 2013;62(2):91-98. PubMed
13. Linzer M, Konrad TR, Douglas J, et al. Managed care, time pressure, and physician job satisfaction: results from the physician worklife study. J Gen Intern Med. 2000;15(7):441-450. PubMed
14. Phillips LS, Branch WT, Cook CB, et al. Clinical inertia. Ann Intern Med. 2001;135(9):825-834.
15. Dev S, Hoffman TK, Kavalieratos D, et al. Barriers to adoption of mineralocorticoid receptor antagonists in patients with heart failure: A mixed-methods study. J Am Heart Assoc. 2016;4(3):e002493. PubMed
16. Stange KC. The problem of fragmentation and the need for integrative solutions. Ann Fam Med. 2009;7(2):100-103. PubMed
17. Fonarow GC, Abraham WT, Albert NM, et al. Association between performance measures and clinical outcomes for patients hospitalized with heart failure. JAMA. 2007;297(1):61-70. PubMed
18. Shah M, Norwood CA, Farias S, Ibrahim S, Chong PH, Fogelfeld L. Diabetes transitional care from inpatient to outpatient setting: pharmacist discharge counseling. J Pharm Pract. 2013;26(2):120-124. PubMed
19. Kripalani S, LeFevre F, Phillips CO, Williams MV, Basaviah P, Baker DW. Deficits in communication and information transfer between hospital-based and primary care physicians: implications for patient safety and continuity of care. JAMA. 2007;297(8):831-841. PubMed
Millions of individuals with chronic diseases are hospitalized annually in the United States. More than 90% of hospitalized adults have at least 1 chronic disease,1 and almost half of Medicare beneficiaries in the hospital have 4 or more chronic conditions.2 While many patients are admitted for worsening of a single chronic disease, patients are hospitalized more commonly for other causes. For instance, although acute heart failure is among the most frequent causes of hospitalizations among older adults, three-fourths of hospitalizations of patients with heart failure are for reasons other than acute heart failure.3
When a patient with a chronic disease is hospitalized, the inpatient provider must consider whether to actively or passively manage the chronic disease. Studies have suggested that intervening in chronic diseases during hospitalizations can lead to long-term improvement in treatment;4-6 for instance, stroke patients who were started on antihypertensive therapy at discharge were more likely to have their blood pressure controlled in the next year.5 However, some authors have argued that aggressive hypertension management by inpatient providers may result in patient harm.7 One case-based survey suggested that hospitalists were mixed in their interest in participating in chronic disease management in the hospital.8 This study found that providers were less likely to participate in chronic disease management if it was unrelated to the reason for hospitalization.8 However, to our knowledge, no studies have broadly evaluated inpatient provider attitudes, motivating factors, or barriers to participation in chronic disease management.
The purpose of this study was to understand provider attitudes towards chronic disease management for patients who are hospitalized for other causes. We were particularly interested in perceptions of barriers and facilitators to delivery of inpatient chronic disease management. Ultimately, such findings can inform future interventions to improve inpatient care of chronic disease.
METHODS
In this qualitative study, we conducted in-depth interviews with providers to understand attitudes, barriers, and facilitators towards inpatient management of chronic disease; this study was part of a larger study to implement an electronic health record-based clinical decision-support system intervention to improve quality of care for hospitalized patients with heart failure.
We included providers who care for and can write medication orders for hospitalized adult patients at New York University (NYU) Langone Medical Center, an urban academic medical center. As patients with chronic conditions are commonly hospitalized for many reasons, we sought to interview providers from a range of clinical services without consideration of factors such as frequency of caring for patients with heart failure. We used a purposive sampling framework: we invited participants to ensure a range of services, including medicine, surgery, and neurology, and provider types, including attending physicians, resident physicians, nurse practitioners, and physician assistants. Potential participants, therefore, included all providers for adult hospitalized patients.
We identified potential participants through study team members, referrals from department heads and prior interviewees, and e-mails to department list serves. We did not formally track declinations to being interviewed, although we estimate them as fewer than 20% of providers directly approached. While we focused on inpatient providers at New York University Langone Medical Center, many of the attending physicians and residents spend a portion of their time at the Manhattan Veterans Affairs Hospital and Bellevue Hospital, a safety-net city hospital; providers could have outpatient responsibilities as well.
All participants provided verbal consent to participate. The study was approved by the New York University Institutional Review Board, which granted a waiver of documentation of consent. Participants received a $25 gift card following the interview.
We used a semi-structured interview guide (Appendix) to elicit in-depth accounts of provider attitudes, experiences with, and barriers and facilitators towards chronic disease management in the hospital. The interview began by asking about chronic disease in general and then asked more specific questions about heart failure; we included responses to both groups of questions in the current study. The interview also included questions related to the clinical decision-support system being developed as part of the larger implementation study, although we do not report on these results in the current study. The semi-structured interview guide was informed by the consolidated framework for advancing implementation science (CFIR), which offers an overarching typology for delineating factors that influence guideline implementation;9 we also used CFIR constructs in theme development. We conducted in-depth interviews with providers.
A priori, we estimated 25 interviews would be sufficient to include the purposive sample and achieve data saturation,10 which was reached after 31 interviews. Interviews were held in person or by telephone, at the convenience of the subject. All interviews were transcribed by a professional service. Transcriptions were reviewed against recordings with any mistakes corrected. Prior to each interview, we conducted a brief demographic survey.
Qualitative data were analyzed using a constant comparative analytic technique.11 The investigative team met after reviewing the first 10 interviews and discussed emergent themes from these early transcripts, which led to the initial code list. Two investigators coded the transcripts. Reliability was evaluated by independent coding of a 20% subset of interviews. Differences were reviewed and discussed until consensus was reached. Final intercoder reliability was determined to be greater than 95%.12 All investigators reviewed and refined the code list during the analysis phase. Codes were clustered into themes based on CFIR constructs.9 Analyses were performed using Atlas.ti v. 7 (ATLAS.ti Scientific Software Development GmbH, Berlin, Germany).
RESULTS
We conducted interviews with 31 providers. Of these, 12 were on the medicine service, 12 were on the surgery or a surgical subspecialty service, and 7 were on other services; 11 were attending physicians, 12 were resident physicians, 5 were NPs, and 3 were PAs. Only 2 providers—an attending in medicine and a resident in surgery—had a specialty focus that was cardiac-related. Median time in current position was 4 years (Table 1). Seventeen of the interviews were in person, and 14 were conducted by telephone. The mean interview time was 20 minutes and ranged from 11 to 41 minutes.
We identified 5 main themes with 29 supporting codes (Table 2) describing provider attitudes towards the management of chronic disease for hospitalized patients. These themes, with related CFIR constructs, were: 1) perceived impact on patient outcomes (CFIR construct: intervention characteristics, relative advantage); 2) hospital structural characteristics (inner setting, structural characteristics); 3) provider knowledge and self-efficacy (characteristic of individual, knowledge and beliefs about the intervention and self-efficacy); 4) hospital priorities (inner setting, implementation climate, relative priority); and 5) continuity and communication (inner setting, networks and communications). For most themes, subjects described both positive and negative aspects of chronic disease management, as well as related facilitators and barriers to delivery of chronic disease care for hospitalized patients. Illustrative quotes for each theme are shown in Table 3.
Perceived Impact on Patient Outcomes
Perceived impact on patient outcomes was mixed. Most providers believed the management of chronic diseases could lead to improvement in important patient outcomes, including decreased length of stay (LOS), prevention of hospital complication, and decreased readmissions. Surgical providers focused particularly on the benefits of preventing surgical complications and noted that they were more likely to manage chronic conditions—primarily through use of specialist consultation—when they perceived a benefit to prevention of surgical outcomes or a fear that surgery may worsen a stable chronic condition:
“Most of the surgery I do is pretty stressful on the body and is very likely to induce acute on chronic exacerbations of heart failure. For someone with Class II or higher heart failure, I’m definitely gonna have cardiology on board or at least internal medicine on board right from the beginning.”
However, some providers acknowledged that there were potential risks to such management, including “prolonging hospital stays for nonemergent indications” and treatment with therapies that had previously led to an “adverse reaction that wasn’t clearly documented.” Providers were also concerned that treating chronic conditions may take focus away from acute conditions, which could lead to worse patient-centered outcomes. One attending in medicine described it:
“If you do potentially focus on those chronic issues, and there’s already a lot of other stuff going on with the patient, you might not be prioritizing the patient’s active issues appropriately. The patient’s saying, ‘I’m in pain. I’m in pain. I’m in pain,’ and you’re saying, ‘Thank you very much. Look, your heart failure, you didn’t get your beta-blocker.’ There could be a disconnect between patient’s goals, expectations, and your goals and expectations.”
Hospital Structural Characteristics
For many providers, the hospital setting provides a unique opportunity for care of patients with chronic disease. First, a hospitalization is a time for a patient’s management to be reviewed by a new care team. The hospital team reviews the management plan for patients at admission, which is a time to reevaluate whether patients are on evidence-based therapies: “It’s helpful to have a new set of eyes on somebody, like fresh information.” According to providers, this reevaluation can overcome instances of therapeutic inertia by the outpatient physician. Second, the hospital has many resources, including readily available specialist services and diagnostic tests, which can allow a patient-centered approach that coordinates care in 1 place, as a surgery NP described: “I think the advantage for the patient is that they wind up stopping in for 1 thing but we wind up taking care of a few without requiring the need for him or her to go to all these different specialists on the outside. They’re mostly elderly and not able to get around.” Third, the high availability of services and frequent monitoring allows rapid titration of evidence-based medicines, as discussed by a medicine resident: “It’s easier and faster to titrate medication—they’re in a monitored setting; you can ensure compliance.”
Patients may also differ from their usual state while hospitalized, creating both risks and benefits. The hospital setting can provide an opportunity to educate patients on their chronic disease(s) because they are motivated: “They’re in an office visit and their sugars are out of whack or something, they may take it a little bit more seriously if they were just in the hospital even though it was on an unrelated issue. I think it probably just changes their perspective on their disease.” However, in the hospital, patients are in an unusual environment with a restricted diet and forced medication compliance. Furthermore, the acute condition can lead to changes in their chronic disease, as described by 1 medicine attending: “their sugar is high because they’re acutely ill.” Providers expressed concern that changing medications in this setting may lead to adverse events (AEs) when patients return to their usual environment.
Provider Knowledge and Self-Efficacy
Insufficient knowledge of treatments for chronic conditions was cited as a barrier to some providers’ ability to actively manage chronic disease for hospitalized patients. Some providers described management of conditions outside their area as less satisfying than their primary focus. For example, an orthopedic surgeon explained: “…it’s very simple. You see your bone is broken, you fix it, that’s it…it’s intellectually satisfying…managing chronic diseases is less like that.” Reliance on consultants was 1 approach to deal with knowledge gaps in areas outside a provider’s expertise.
For a number of providers, management of stable chronic disease is the responsibility of the outpatient provider. Providers expressed concern that inpatient management was a reach into the domain of the primary care provider (PCP) and might take “away from the primary focus” of the hospitalization. Nonetheless, some providers noted an “ethical responsibility to manage [a] patient correctly,” and some providers believed that engaging in chronic disease management in the hospital would present an opportunity to expand their own expertise.
A few providers were worried about legal risk related to chronic disease management: “we don’t typically deal too much with managing some of these other medical issues for medical and legal reasons.” Providers again suggested that consults can help overcome this concern for risk, as discussed by 1 surgical attending: “We’re all not wanting to be sued, and we want to do the right thing. It costs me nothing to have a cardiologist on board, so like—why not.”
Hospital Priorities
Providers explained that the hospital has strong interests in early discharge and minimizing LOS. These priorities are based on goals of improving patient outcomes, increasing bed availability and hospital volume, and reducing costs. Providers perceive these hospital priorities as potential barriers to chronic disease management, which can increase LOS and costs through additional testing and treatment. As a medicine resident described: “The DBN philosophy, ‘discharge before noon’ philosophy, which is part of the hospital efficiency to get people in and out of the hospital as quickly as [is] safe, or maybe faster. And I think that there’s a culture where you’re encouraged to only focus on the acute issue and tend to defer everything else.”
Continuity and Communication
According to many providers, care continuity between the outpatient setting and the hospital played a major role in management of chronic disease. One barrier to starting a new evidence-based medication was lack of knowledge of patient history. As noted, providers expressed concern that a patient may not be on a given therapy because of an adverse reaction that was not documented in the hospital chart. This is particularly true because, as discussed by a surgery resident, patients with “PCPs outside the system [in which providers] don’t have access to the electronic medical record.” To overcome this barrier, providers attempt to communicate with the outpatient provider to confirm a lack of contraindications to therapies prior to any changes; notably, communication is easier if the inpatient provider has a relationship with the outpatient PCP.
Some providers were more likely to start chronic disease therapies if the patient had no prior outpatient care, because the provider was reassured that there was no rationale for missing therapies. One neurology attending noted that if a patient had newly documented “hypertension even if they were in for something else, I might start them on an antihypertensive, but then arrange for a close follow-up with a new PCP.”
Following hospitalization, providers wanted assurance that any changes to chronic disease management would be followed up by an outpatient physician. Any changes are relayed to the outpatient provider and the “level of communication…with the outpatient provider who’s gonna inherit” these changes can influence how aggressively the inpatient provider manages chronic diseases. Providers may be reluctant to start therapy for patients if they are concerned about outpatient follow up: “they have diabetes and they should really technically be on an ACE [angiotensin converting enzyme]inhibitor and aspirin, but they’re not. I might send them out on the aspirin but I might either start ACE inhibitor and have them follow up with their PCP in 2 weeks if I’m confident that they’ll do it or if I’m really confident that they’ll not follow up, I will help them get the appointment and then the discharge instruction is to the PCP is ‘Please start this patient on ACE inhibitor if they show up.’”
DISCUSSION
Providers frequently perceive benefit to chronic disease management in the hospital, including improvements in clinical outcomes. Notably, providers see opportunities to improve compliance with evidence-based care to overcome potential barriers to managing chronic disease in the outpatient setting, which can be limited by pressure for brief encounters,13 clinical inertia,14 difficulty with close monitoring of patients,15 and care fragmentation.16 Concurrently, inpatient providers are concerned about potential for patient harm related to chronic disease management, primarily related to AEs from medications. Similar to a case study about a patient with outpatient hypotension following aggressive inpatient hypertension management,7 providers fear that changing a patient’s chronic disease management in a hospital setting may cause harm when the patient returns home.
Although some clinicians have argued against aggressive in-hospital chronic disease management because of concerns for risk of AEs,7 our study and others8 have suggested that many clinicians perceive benefit. In some cases, such as smoking cessation counseling for all current smokers and prescribing an angiotensin converting enzyme inhibitor for patients with systolic heart failure, the perceived importance is so great that chronic disease management has been used as a national quality metric for hospitals. While these hospital metrics may be justified for short-term benefits after hospitalization, studies have demonstrated only weak improvement in short-term postdischarge outcomes related to chronic disease management.17 The true benefit is likely from improved processes of care in the short term that lead to long-term improvement in outcomes.4,5,18 Thus, the advantage of starting a patient hospitalized for a stroke on blood pressure medication is the increased likelihood that the patient will continue the medication as an outpatient, which may reduce long-term mortality.
For hospital delivery systems that are concerned with such care process improvement through in-hospital chronic disease management, we identified a number of barriers and facilitators to delivering this care. One significant barrier was poor transitions between the inpatient and the outpatient settings. When a patient transitions into the hospital, providers need to understand prior management choices. Facilitators to help inpatient providers understand prior management included either knowing the outpatient provider, or understanding that there was a lack of regular outpatient care; in both these cases, inpatient providers felt more comfortable managing chronic diseases because they had insight into the outpatient plan, or lack thereof. However, these facilitators may not be practical to incorporate in interventions to improve chronic disease care, which should consider overcoming these communication barriers. Use of shared electronic health records or standardized telephone calls with well-documented care plans obtained through health information exchanges may facilitate an inpatient provider to manage appropriately chronic disease. Similarly, discontinuity between the inpatient provider and the outpatient provider is a barrier that must be overcome to ease concerns that any chronic disease management changes do not result in harm in the postdischarge period. These findings again point to the need for improved documentation and communication between inpatient and outpatient providers. Of course, the transitional care period is one of high risk, and improving communication between providers has been an area of ongoing work.19
Lack of comfort among inpatient providers with managing chronic diseases is another important barrier, which appears to be largely overcome through the use of consultation services. Ready availability of specialists, common in academic medical centers, can facilitate delivery of chronic disease management. Inpatient interventions designed to improve evidence-based care for a chronic disease may benefit from involvement or at least availability of specialists in the effort. Another major barrier relates to hospital priorities, which in our study were closely aligned with external factors such as payment models. As hospitalizations are typically paid based on the discharge diagnosis, hospitals have incentives to discharge quickly and not order extra diagnostic tests. As a result, there are disincentives for chronic disease management that may require additional testing or monitoring in the hospital. Conversely, as hospitals accept postdischarge financial risks through readmission penalties or postdischarge cost savings, hospitals may perceive that long-term benefits of chronic disease management may outweigh short-term costs.
The study findings should be interpreted in the context of its limitations. Findings of our study of providers from a single academic medical center may not be generalizable. Nearly half of our interviews were conducted by telephone, which limits our ability to capture nonverbal cues in communication. Providers may have had social desirability bias towards positive aspects of chronic disease management. We did not have the power to determine differences in response by provider characteristic because this was an exploratory qualitative study. Future studies with representative sampling, a larger sample size, and measures for constructs such as provider self-efficacy are needed to examine differences by specialty, provider type, and experience level.
In conclusion, inpatient providers believe that hospital chronic disease management has the potential to be beneficial for both process of care and clinical outcomes; providers also express concern about potential adverse consequences of managing chronic disease during acute hospitalizations. To maximize both quality of care and patient safety, overcoming communication barriers between inpatient and outpatient providers is needed. Both a supportive hospital environment and availability of specialty support can facilitate in-hospital chronic disease management. Interventions that incorporate these factors may be well-suited to improve chronic disease care and long-term outcomes.
Disclosures
This work was supported by the Agency for Healthcare Research and Quality (AHRQ) grant K08HS23683. The authors report no financial conflicts of interest.
Millions of individuals with chronic diseases are hospitalized annually in the United States. More than 90% of hospitalized adults have at least 1 chronic disease,1 and almost half of Medicare beneficiaries in the hospital have 4 or more chronic conditions.2 While many patients are admitted for worsening of a single chronic disease, patients are hospitalized more commonly for other causes. For instance, although acute heart failure is among the most frequent causes of hospitalizations among older adults, three-fourths of hospitalizations of patients with heart failure are for reasons other than acute heart failure.3
When a patient with a chronic disease is hospitalized, the inpatient provider must consider whether to actively or passively manage the chronic disease. Studies have suggested that intervening in chronic diseases during hospitalizations can lead to long-term improvement in treatment;4-6 for instance, stroke patients who were started on antihypertensive therapy at discharge were more likely to have their blood pressure controlled in the next year.5 However, some authors have argued that aggressive hypertension management by inpatient providers may result in patient harm.7 One case-based survey suggested that hospitalists were mixed in their interest in participating in chronic disease management in the hospital.8 This study found that providers were less likely to participate in chronic disease management if it was unrelated to the reason for hospitalization.8 However, to our knowledge, no studies have broadly evaluated inpatient provider attitudes, motivating factors, or barriers to participation in chronic disease management.
The purpose of this study was to understand provider attitudes towards chronic disease management for patients who are hospitalized for other causes. We were particularly interested in perceptions of barriers and facilitators to delivery of inpatient chronic disease management. Ultimately, such findings can inform future interventions to improve inpatient care of chronic disease.
METHODS
In this qualitative study, we conducted in-depth interviews with providers to understand attitudes, barriers, and facilitators towards inpatient management of chronic disease; this study was part of a larger study to implement an electronic health record-based clinical decision-support system intervention to improve quality of care for hospitalized patients with heart failure.
We included providers who care for and can write medication orders for hospitalized adult patients at New York University (NYU) Langone Medical Center, an urban academic medical center. As patients with chronic conditions are commonly hospitalized for many reasons, we sought to interview providers from a range of clinical services without consideration of factors such as frequency of caring for patients with heart failure. We used a purposive sampling framework: we invited participants to ensure a range of services, including medicine, surgery, and neurology, and provider types, including attending physicians, resident physicians, nurse practitioners, and physician assistants. Potential participants, therefore, included all providers for adult hospitalized patients.
We identified potential participants through study team members, referrals from department heads and prior interviewees, and e-mails to department list serves. We did not formally track declinations to being interviewed, although we estimate them as fewer than 20% of providers directly approached. While we focused on inpatient providers at New York University Langone Medical Center, many of the attending physicians and residents spend a portion of their time at the Manhattan Veterans Affairs Hospital and Bellevue Hospital, a safety-net city hospital; providers could have outpatient responsibilities as well.
All participants provided verbal consent to participate. The study was approved by the New York University Institutional Review Board, which granted a waiver of documentation of consent. Participants received a $25 gift card following the interview.
We used a semi-structured interview guide (Appendix) to elicit in-depth accounts of provider attitudes, experiences with, and barriers and facilitators towards chronic disease management in the hospital. The interview began by asking about chronic disease in general and then asked more specific questions about heart failure; we included responses to both groups of questions in the current study. The interview also included questions related to the clinical decision-support system being developed as part of the larger implementation study, although we do not report on these results in the current study. The semi-structured interview guide was informed by the consolidated framework for advancing implementation science (CFIR), which offers an overarching typology for delineating factors that influence guideline implementation;9 we also used CFIR constructs in theme development. We conducted in-depth interviews with providers.
A priori, we estimated 25 interviews would be sufficient to include the purposive sample and achieve data saturation,10 which was reached after 31 interviews. Interviews were held in person or by telephone, at the convenience of the subject. All interviews were transcribed by a professional service. Transcriptions were reviewed against recordings with any mistakes corrected. Prior to each interview, we conducted a brief demographic survey.
Qualitative data were analyzed using a constant comparative analytic technique.11 The investigative team met after reviewing the first 10 interviews and discussed emergent themes from these early transcripts, which led to the initial code list. Two investigators coded the transcripts. Reliability was evaluated by independent coding of a 20% subset of interviews. Differences were reviewed and discussed until consensus was reached. Final intercoder reliability was determined to be greater than 95%.12 All investigators reviewed and refined the code list during the analysis phase. Codes were clustered into themes based on CFIR constructs.9 Analyses were performed using Atlas.ti v. 7 (ATLAS.ti Scientific Software Development GmbH, Berlin, Germany).
RESULTS
We conducted interviews with 31 providers. Of these, 12 were on the medicine service, 12 were on the surgery or a surgical subspecialty service, and 7 were on other services; 11 were attending physicians, 12 were resident physicians, 5 were NPs, and 3 were PAs. Only 2 providers—an attending in medicine and a resident in surgery—had a specialty focus that was cardiac-related. Median time in current position was 4 years (Table 1). Seventeen of the interviews were in person, and 14 were conducted by telephone. The mean interview time was 20 minutes and ranged from 11 to 41 minutes.
We identified 5 main themes with 29 supporting codes (Table 2) describing provider attitudes towards the management of chronic disease for hospitalized patients. These themes, with related CFIR constructs, were: 1) perceived impact on patient outcomes (CFIR construct: intervention characteristics, relative advantage); 2) hospital structural characteristics (inner setting, structural characteristics); 3) provider knowledge and self-efficacy (characteristic of individual, knowledge and beliefs about the intervention and self-efficacy); 4) hospital priorities (inner setting, implementation climate, relative priority); and 5) continuity and communication (inner setting, networks and communications). For most themes, subjects described both positive and negative aspects of chronic disease management, as well as related facilitators and barriers to delivery of chronic disease care for hospitalized patients. Illustrative quotes for each theme are shown in Table 3.
Perceived Impact on Patient Outcomes
Perceived impact on patient outcomes was mixed. Most providers believed the management of chronic diseases could lead to improvement in important patient outcomes, including decreased length of stay (LOS), prevention of hospital complication, and decreased readmissions. Surgical providers focused particularly on the benefits of preventing surgical complications and noted that they were more likely to manage chronic conditions—primarily through use of specialist consultation—when they perceived a benefit to prevention of surgical outcomes or a fear that surgery may worsen a stable chronic condition:
“Most of the surgery I do is pretty stressful on the body and is very likely to induce acute on chronic exacerbations of heart failure. For someone with Class II or higher heart failure, I’m definitely gonna have cardiology on board or at least internal medicine on board right from the beginning.”
However, some providers acknowledged that there were potential risks to such management, including “prolonging hospital stays for nonemergent indications” and treatment with therapies that had previously led to an “adverse reaction that wasn’t clearly documented.” Providers were also concerned that treating chronic conditions may take focus away from acute conditions, which could lead to worse patient-centered outcomes. One attending in medicine described it:
“If you do potentially focus on those chronic issues, and there’s already a lot of other stuff going on with the patient, you might not be prioritizing the patient’s active issues appropriately. The patient’s saying, ‘I’m in pain. I’m in pain. I’m in pain,’ and you’re saying, ‘Thank you very much. Look, your heart failure, you didn’t get your beta-blocker.’ There could be a disconnect between patient’s goals, expectations, and your goals and expectations.”
Hospital Structural Characteristics
For many providers, the hospital setting provides a unique opportunity for care of patients with chronic disease. First, a hospitalization is a time for a patient’s management to be reviewed by a new care team. The hospital team reviews the management plan for patients at admission, which is a time to reevaluate whether patients are on evidence-based therapies: “It’s helpful to have a new set of eyes on somebody, like fresh information.” According to providers, this reevaluation can overcome instances of therapeutic inertia by the outpatient physician. Second, the hospital has many resources, including readily available specialist services and diagnostic tests, which can allow a patient-centered approach that coordinates care in 1 place, as a surgery NP described: “I think the advantage for the patient is that they wind up stopping in for 1 thing but we wind up taking care of a few without requiring the need for him or her to go to all these different specialists on the outside. They’re mostly elderly and not able to get around.” Third, the high availability of services and frequent monitoring allows rapid titration of evidence-based medicines, as discussed by a medicine resident: “It’s easier and faster to titrate medication—they’re in a monitored setting; you can ensure compliance.”
Patients may also differ from their usual state while hospitalized, creating both risks and benefits. The hospital setting can provide an opportunity to educate patients on their chronic disease(s) because they are motivated: “They’re in an office visit and their sugars are out of whack or something, they may take it a little bit more seriously if they were just in the hospital even though it was on an unrelated issue. I think it probably just changes their perspective on their disease.” However, in the hospital, patients are in an unusual environment with a restricted diet and forced medication compliance. Furthermore, the acute condition can lead to changes in their chronic disease, as described by 1 medicine attending: “their sugar is high because they’re acutely ill.” Providers expressed concern that changing medications in this setting may lead to adverse events (AEs) when patients return to their usual environment.
Provider Knowledge and Self-Efficacy
Insufficient knowledge of treatments for chronic conditions was cited as a barrier to some providers’ ability to actively manage chronic disease for hospitalized patients. Some providers described management of conditions outside their area as less satisfying than their primary focus. For example, an orthopedic surgeon explained: “…it’s very simple. You see your bone is broken, you fix it, that’s it…it’s intellectually satisfying…managing chronic diseases is less like that.” Reliance on consultants was 1 approach to deal with knowledge gaps in areas outside a provider’s expertise.
For a number of providers, management of stable chronic disease is the responsibility of the outpatient provider. Providers expressed concern that inpatient management was a reach into the domain of the primary care provider (PCP) and might take “away from the primary focus” of the hospitalization. Nonetheless, some providers noted an “ethical responsibility to manage [a] patient correctly,” and some providers believed that engaging in chronic disease management in the hospital would present an opportunity to expand their own expertise.
A few providers were worried about legal risk related to chronic disease management: “we don’t typically deal too much with managing some of these other medical issues for medical and legal reasons.” Providers again suggested that consults can help overcome this concern for risk, as discussed by 1 surgical attending: “We’re all not wanting to be sued, and we want to do the right thing. It costs me nothing to have a cardiologist on board, so like—why not.”
Hospital Priorities
Providers explained that the hospital has strong interests in early discharge and minimizing LOS. These priorities are based on goals of improving patient outcomes, increasing bed availability and hospital volume, and reducing costs. Providers perceive these hospital priorities as potential barriers to chronic disease management, which can increase LOS and costs through additional testing and treatment. As a medicine resident described: “The DBN philosophy, ‘discharge before noon’ philosophy, which is part of the hospital efficiency to get people in and out of the hospital as quickly as [is] safe, or maybe faster. And I think that there’s a culture where you’re encouraged to only focus on the acute issue and tend to defer everything else.”
Continuity and Communication
According to many providers, care continuity between the outpatient setting and the hospital played a major role in management of chronic disease. One barrier to starting a new evidence-based medication was lack of knowledge of patient history. As noted, providers expressed concern that a patient may not be on a given therapy because of an adverse reaction that was not documented in the hospital chart. This is particularly true because, as discussed by a surgery resident, patients with “PCPs outside the system [in which providers] don’t have access to the electronic medical record.” To overcome this barrier, providers attempt to communicate with the outpatient provider to confirm a lack of contraindications to therapies prior to any changes; notably, communication is easier if the inpatient provider has a relationship with the outpatient PCP.
Some providers were more likely to start chronic disease therapies if the patient had no prior outpatient care, because the provider was reassured that there was no rationale for missing therapies. One neurology attending noted that if a patient had newly documented “hypertension even if they were in for something else, I might start them on an antihypertensive, but then arrange for a close follow-up with a new PCP.”
Following hospitalization, providers wanted assurance that any changes to chronic disease management would be followed up by an outpatient physician. Any changes are relayed to the outpatient provider and the “level of communication…with the outpatient provider who’s gonna inherit” these changes can influence how aggressively the inpatient provider manages chronic diseases. Providers may be reluctant to start therapy for patients if they are concerned about outpatient follow up: “they have diabetes and they should really technically be on an ACE [angiotensin converting enzyme]inhibitor and aspirin, but they’re not. I might send them out on the aspirin but I might either start ACE inhibitor and have them follow up with their PCP in 2 weeks if I’m confident that they’ll do it or if I’m really confident that they’ll not follow up, I will help them get the appointment and then the discharge instruction is to the PCP is ‘Please start this patient on ACE inhibitor if they show up.’”
DISCUSSION
Providers frequently perceive benefit to chronic disease management in the hospital, including improvements in clinical outcomes. Notably, providers see opportunities to improve compliance with evidence-based care to overcome potential barriers to managing chronic disease in the outpatient setting, which can be limited by pressure for brief encounters,13 clinical inertia,14 difficulty with close monitoring of patients,15 and care fragmentation.16 Concurrently, inpatient providers are concerned about potential for patient harm related to chronic disease management, primarily related to AEs from medications. Similar to a case study about a patient with outpatient hypotension following aggressive inpatient hypertension management,7 providers fear that changing a patient’s chronic disease management in a hospital setting may cause harm when the patient returns home.
Although some clinicians have argued against aggressive in-hospital chronic disease management because of concerns for risk of AEs,7 our study and others8 have suggested that many clinicians perceive benefit. In some cases, such as smoking cessation counseling for all current smokers and prescribing an angiotensin converting enzyme inhibitor for patients with systolic heart failure, the perceived importance is so great that chronic disease management has been used as a national quality metric for hospitals. While these hospital metrics may be justified for short-term benefits after hospitalization, studies have demonstrated only weak improvement in short-term postdischarge outcomes related to chronic disease management.17 The true benefit is likely from improved processes of care in the short term that lead to long-term improvement in outcomes.4,5,18 Thus, the advantage of starting a patient hospitalized for a stroke on blood pressure medication is the increased likelihood that the patient will continue the medication as an outpatient, which may reduce long-term mortality.
For hospital delivery systems that are concerned with such care process improvement through in-hospital chronic disease management, we identified a number of barriers and facilitators to delivering this care. One significant barrier was poor transitions between the inpatient and the outpatient settings. When a patient transitions into the hospital, providers need to understand prior management choices. Facilitators to help inpatient providers understand prior management included either knowing the outpatient provider, or understanding that there was a lack of regular outpatient care; in both these cases, inpatient providers felt more comfortable managing chronic diseases because they had insight into the outpatient plan, or lack thereof. However, these facilitators may not be practical to incorporate in interventions to improve chronic disease care, which should consider overcoming these communication barriers. Use of shared electronic health records or standardized telephone calls with well-documented care plans obtained through health information exchanges may facilitate an inpatient provider to manage appropriately chronic disease. Similarly, discontinuity between the inpatient provider and the outpatient provider is a barrier that must be overcome to ease concerns that any chronic disease management changes do not result in harm in the postdischarge period. These findings again point to the need for improved documentation and communication between inpatient and outpatient providers. Of course, the transitional care period is one of high risk, and improving communication between providers has been an area of ongoing work.19
Lack of comfort among inpatient providers with managing chronic diseases is another important barrier, which appears to be largely overcome through the use of consultation services. Ready availability of specialists, common in academic medical centers, can facilitate delivery of chronic disease management. Inpatient interventions designed to improve evidence-based care for a chronic disease may benefit from involvement or at least availability of specialists in the effort. Another major barrier relates to hospital priorities, which in our study were closely aligned with external factors such as payment models. As hospitalizations are typically paid based on the discharge diagnosis, hospitals have incentives to discharge quickly and not order extra diagnostic tests. As a result, there are disincentives for chronic disease management that may require additional testing or monitoring in the hospital. Conversely, as hospitals accept postdischarge financial risks through readmission penalties or postdischarge cost savings, hospitals may perceive that long-term benefits of chronic disease management may outweigh short-term costs.
The study findings should be interpreted in the context of its limitations. Findings of our study of providers from a single academic medical center may not be generalizable. Nearly half of our interviews were conducted by telephone, which limits our ability to capture nonverbal cues in communication. Providers may have had social desirability bias towards positive aspects of chronic disease management. We did not have the power to determine differences in response by provider characteristic because this was an exploratory qualitative study. Future studies with representative sampling, a larger sample size, and measures for constructs such as provider self-efficacy are needed to examine differences by specialty, provider type, and experience level.
In conclusion, inpatient providers believe that hospital chronic disease management has the potential to be beneficial for both process of care and clinical outcomes; providers also express concern about potential adverse consequences of managing chronic disease during acute hospitalizations. To maximize both quality of care and patient safety, overcoming communication barriers between inpatient and outpatient providers is needed. Both a supportive hospital environment and availability of specialty support can facilitate in-hospital chronic disease management. Interventions that incorporate these factors may be well-suited to improve chronic disease care and long-term outcomes.
Disclosures
This work was supported by the Agency for Healthcare Research and Quality (AHRQ) grant K08HS23683. The authors report no financial conflicts of interest.
1. Friedman B, Jiang HJ, Elixhauser A, Segal A. Hospital inpatient costs for adults with multiple chronic conditions. Med Care Res Rev. 2006;63(3):327-346. PubMed
2. Steiner CA, Friedman B. Hospital utilization, costs, and mortality for adults with multiple chronic conditions, Nationwide Inpatient Sample, 2009. Prev Chronic Dis. 2013;10:E62. PubMed
3. Blecker S, Paul M, Taksler G, Ogedegbe G, Katz S. Heart failure-associated hospitalizations in the United States. J Am Coll Cardiol. 2013;61(12):1259-1267. PubMed
4. Fonarow GC. Role of in-hospital initiation of carvedilol to improve treatment rates and clinical outcomes. Am J Cardiol. 2004;93(9A):77B-81B. PubMed
5. Touze E, Coste J, Voicu M, et al. Importance of in-hospital initiation of therapies and therapeutic inertia in secondary stroke prevention: IMplementation of Prevention After a Cerebrovascular evenT (IMPACT) Study. Stroke. 2008;39(6):1834-1843. PubMed
6. Ovbiagele B, Saver JL, Fredieu A, et al. In-hospital initiation of secondary stroke prevention therapies yields high rates of adherence at follow-up. Stroke. 2004;35(12):2879-2883. PubMed
7. Steinman MA, Auerbach AD. Managing chronic disease in hospitalized patients. JAMA Intern Med. 2013;173(20):1857-1858. PubMed
8. Breu AC, Allen-Dicker J, Mueller S, Palamara K, Hinami K, Herzig SJ. Hospitalist and primary care physician perspectives on medication management of chronic conditions for hospitalized patients. J Hosp Med. 2014;9(5):303-309. PubMed
9. Damschroder LJ, Aron DC, Keith RE, Kirsh SR, Alexander JA, Lowery JC. Fostering implementation of health services research findings into practice: a consolidated framework for advancing implementation science. Implement Sci. 2009;4:50. PubMed
10. Morse JM. The significance of saturation. Qualitative Health Research. 1995;5(2):147-149.
11. Bradley EH, Curry LA, Devers KJ. Qualitative data analysis for health services research: developing taxonomy, themes, and theory. Health Services Research. 2007;42(4):1758-1772. PubMed
12. Riegel B, Dickson VV, Topaz M. Qualitative analysis of naturalistic decision making in adults with chronic heart failure. Nurs Res. 2013;62(2):91-98. PubMed
13. Linzer M, Konrad TR, Douglas J, et al. Managed care, time pressure, and physician job satisfaction: results from the physician worklife study. J Gen Intern Med. 2000;15(7):441-450. PubMed
14. Phillips LS, Branch WT, Cook CB, et al. Clinical inertia. Ann Intern Med. 2001;135(9):825-834.
15. Dev S, Hoffman TK, Kavalieratos D, et al. Barriers to adoption of mineralocorticoid receptor antagonists in patients with heart failure: A mixed-methods study. J Am Heart Assoc. 2016;4(3):e002493. PubMed
16. Stange KC. The problem of fragmentation and the need for integrative solutions. Ann Fam Med. 2009;7(2):100-103. PubMed
17. Fonarow GC, Abraham WT, Albert NM, et al. Association between performance measures and clinical outcomes for patients hospitalized with heart failure. JAMA. 2007;297(1):61-70. PubMed
18. Shah M, Norwood CA, Farias S, Ibrahim S, Chong PH, Fogelfeld L. Diabetes transitional care from inpatient to outpatient setting: pharmacist discharge counseling. J Pharm Pract. 2013;26(2):120-124. PubMed
19. Kripalani S, LeFevre F, Phillips CO, Williams MV, Basaviah P, Baker DW. Deficits in communication and information transfer between hospital-based and primary care physicians: implications for patient safety and continuity of care. JAMA. 2007;297(8):831-841. PubMed
1. Friedman B, Jiang HJ, Elixhauser A, Segal A. Hospital inpatient costs for adults with multiple chronic conditions. Med Care Res Rev. 2006;63(3):327-346. PubMed
2. Steiner CA, Friedman B. Hospital utilization, costs, and mortality for adults with multiple chronic conditions, Nationwide Inpatient Sample, 2009. Prev Chronic Dis. 2013;10:E62. PubMed
3. Blecker S, Paul M, Taksler G, Ogedegbe G, Katz S. Heart failure-associated hospitalizations in the United States. J Am Coll Cardiol. 2013;61(12):1259-1267. PubMed
4. Fonarow GC. Role of in-hospital initiation of carvedilol to improve treatment rates and clinical outcomes. Am J Cardiol. 2004;93(9A):77B-81B. PubMed
5. Touze E, Coste J, Voicu M, et al. Importance of in-hospital initiation of therapies and therapeutic inertia in secondary stroke prevention: IMplementation of Prevention After a Cerebrovascular evenT (IMPACT) Study. Stroke. 2008;39(6):1834-1843. PubMed
6. Ovbiagele B, Saver JL, Fredieu A, et al. In-hospital initiation of secondary stroke prevention therapies yields high rates of adherence at follow-up. Stroke. 2004;35(12):2879-2883. PubMed
7. Steinman MA, Auerbach AD. Managing chronic disease in hospitalized patients. JAMA Intern Med. 2013;173(20):1857-1858. PubMed
8. Breu AC, Allen-Dicker J, Mueller S, Palamara K, Hinami K, Herzig SJ. Hospitalist and primary care physician perspectives on medication management of chronic conditions for hospitalized patients. J Hosp Med. 2014;9(5):303-309. PubMed
9. Damschroder LJ, Aron DC, Keith RE, Kirsh SR, Alexander JA, Lowery JC. Fostering implementation of health services research findings into practice: a consolidated framework for advancing implementation science. Implement Sci. 2009;4:50. PubMed
10. Morse JM. The significance of saturation. Qualitative Health Research. 1995;5(2):147-149.
11. Bradley EH, Curry LA, Devers KJ. Qualitative data analysis for health services research: developing taxonomy, themes, and theory. Health Services Research. 2007;42(4):1758-1772. PubMed
12. Riegel B, Dickson VV, Topaz M. Qualitative analysis of naturalistic decision making in adults with chronic heart failure. Nurs Res. 2013;62(2):91-98. PubMed
13. Linzer M, Konrad TR, Douglas J, et al. Managed care, time pressure, and physician job satisfaction: results from the physician worklife study. J Gen Intern Med. 2000;15(7):441-450. PubMed
14. Phillips LS, Branch WT, Cook CB, et al. Clinical inertia. Ann Intern Med. 2001;135(9):825-834.
15. Dev S, Hoffman TK, Kavalieratos D, et al. Barriers to adoption of mineralocorticoid receptor antagonists in patients with heart failure: A mixed-methods study. J Am Heart Assoc. 2016;4(3):e002493. PubMed
16. Stange KC. The problem of fragmentation and the need for integrative solutions. Ann Fam Med. 2009;7(2):100-103. PubMed
17. Fonarow GC, Abraham WT, Albert NM, et al. Association between performance measures and clinical outcomes for patients hospitalized with heart failure. JAMA. 2007;297(1):61-70. PubMed
18. Shah M, Norwood CA, Farias S, Ibrahim S, Chong PH, Fogelfeld L. Diabetes transitional care from inpatient to outpatient setting: pharmacist discharge counseling. J Pharm Pract. 2013;26(2):120-124. PubMed
19. Kripalani S, LeFevre F, Phillips CO, Williams MV, Basaviah P, Baker DW. Deficits in communication and information transfer between hospital-based and primary care physicians: implications for patient safety and continuity of care. JAMA. 2007;297(8):831-841. PubMed
© 2017 Society of Hospital Medicine
EHR Use as a Measure of Care Intensity
Hospitals provide acute inpatient care all day and every day. Nonetheless, a number of studies have shown that weekend care may have lower quality than care delivered on weekdays. [1, 2, 3, 4, 5, 6, 7] Weekend care has been associated with increased mortality among patients suffering from a number of conditions [4, 5, 6, 7, 8, 9, 10] and in a number of clinical settings. [11, 12, 13, 14] Findings of poor outcomes on weekends are not universal, though exceptions occur typically in settings where services and clinical staffing are fairly constant throughout the week, such as intensive care units with on‐site intensivists. [15, 16, 17]
These differences in quality and outcomes suggest that there is a difference in intensity of care over the course of the week. Initiatives to address this important safety issue would be strengthened if a shared metric existed to describe the intensity of care provided at a given time of day or day of the week. However, few measures of global hospital intensity of care exist. The metrics that have been developed are limited by measuring only 1 aspect of care delivery, such as weekend nurse staffing, [18] by requiring extensive chart abstraction, [19] or through reliance on hospital expenses in an older payment model. [20] These measures notably predate contemporary hospital care delivery, which is increasingly dependent on the electronic health record (EHR). To our knowledge, no prior measure of hospital care intensity has been described using the EHR.
Recently, our medical center began an initiative to increase weekend services and staffing to create more uniform availability of care throughout the week. The purpose of this study was to develop a global measure of intensity of care using data derived from the EHR as part of the evaluation of this initiative. [21]
METHODS
We conducted a retrospective analysis of EHR activity for hospital inpatients between January 1, 2011 and December 31, 2011 at New York University Langone Medical Center (NYULMC). During that time period, nearly all inpatient clinical activities at NYULMC were performed and documented through the EHR, Sunrise Clinical Manager (Allscripts, Chicago, IL). These activities include clinical documentation, orders, medication administration, and results of diagnostic tests. Access to the EHR is through Citrix Xenapp Servers (Citrix Systems, Santa Clara, CA), which securely deliver applications from central servers to providers' local terminals.
The primary measure of EHR activity, which we refer to as EHR interactions, was defined as the accessing of a patient's electronic record by a clinician. A provider initiates an interaction by opening a patient's electronic record and concludes it by either opening the record of a different patient or logging out of the EHR. An EHR interaction thus captures a unit of direct patient care, such as documenting a clinical encounter, recording medication administration, or reviewing patient data. Most EHR systems routinely log such interactions to enable compliance departments to audit which users have accessed a patient chart.
The secondary measure of EHR activity was percent central processing unit (CPU), which represents the percent of total available server processing power being used by the Citrix servers at a given time. CPU utilization was averaged over an hour to determine the mean percentage of use for any given hour. As CPU data are not retained for more than 30 days at our institution, we examined CPU usage for the period July 1, 2012 to August 31, 2012.
We evaluated subgroups of EHR interactions by provider type: nurses, resident physicians, attending physicians, pharmacy staff, and all others. We also examined EHR interactions for 2 subgroups of patients: those admitted to the medicine service from the emergency room (ER) and those electively admitted to the surgical service. An elective hospitalization was defined as 1 in which the patient was neither admitted from the ER nor transferred from another hospital. These 2 subgroups were further refined to evaluate EHR interactions among groups of patients admitted during the day, night, weekday, and weeknight. Finally, we examined specific EHR orders that we considered reflective of advancing or altering care, including orders to increase the level of mobilization, to insert or remove a urinary catheter, or to initiate or discontinue antibiotics. As antibiotics are frequently initiated on the day of admission and stopped on the day of discharge, we excluded antibiotic orders that occurred on days of admission or discharge.
Statistical Analysis
EHR interactions were presented as hourly rates by dividing the number of patient chart accessions by the inpatient census for each hour. Hourly census was determined by summing the number of patients who were admitted prior to and discharged after the hour of interest. We calculated the arithmetic means of EHR interactions for each hour and day of the week in 2011. Analysis of variance was used to test differences in EHR interactions among days of the week, and t tests were used to test differences in EHR interactions between Saturday and Sunday, Tuesday and Wednesday, and weekend and weekdays. As a result of multiple comparisons in these analyses, we applied a Bonferroni correction.
EHR interaction rates were assigned to 1 of 3 periods based on a priori suspected peak and trough intensity: day (9:00 am to 4:59 pm ), morning/evening (7:00 am to 8:59 am and 5:00 pm to 7:59 pm ), and night (8:00 pm to 6:59 am ). Negative binomial regression models were used to determine the relative rate of weekday to weekend EHR interactions per patient for the 3 daily time periods. Similar models were developed for EHR interactions by provider types and for specific orders.
We calculated the correlation coefficient between total EHR interactions and occurrence of specific orders, EHR interactions by provider type, EHR interactions by patient subgroups, and CPU. A sensitivity analysis was performed in which EHR interactions were calculated as the number of patient chart accessions per number of daily discharges; this analysis considered the fact that discharges were likely to have high associated intensity but may be less common on weekends as compared to weekdays.
All analyses were performed using Stata 12 (StataCorp, College Station, TX). This study was approved by the institutional review board at NYU School of Medicine.
RESULTS
During the study period, the mean (standard deviation) number of EHR interactions per patient per hour was 2.49 (1.30). EHR interactions differed by hour and day of the week; the lowest number occurred in early morning on weekends, and the highest occurred around 11:00 am on weekdays (Figure 1 ). EHR interactions differed among days of the week at all times ( P <0.001), whereas EHR interactions were similar on most hours of Saturday and Sunday as well as Tuesday and Wednesday. At every hour, weekends had a lower number of EHR interactions in comparison to weekdays ( P <0.001). Weekdays showed a substantial increase in the number of EHR interactions between 8:00 am and 12:00 pm , followed by a slight decrease in activity between 12:00 pm and 2:00 pm , and another increase in activity from 2:00 pm to 5:00 pm (Figure 1 ). Weekend days demonstrated marked blunting of this midday peak in intensity. The tracking of an entire month of hospital EHR interactions produced a detailed graphic picture of hospital activity, clearly demarcating rounding hours, lunch hours, weekend days, hospital holidays, and other landmarks (Figure 2 ). The relative rates of census‐adjusted EHR interactions on weekdays versus weekends were 1.76 (95% confidence interval [CI]: 1.74‐1.77) for day, 1.52 (95% CI: 1.50‐1.55) for morning/evening, and 1.14 (95% CI: 1.12‐1.17) for night hours.
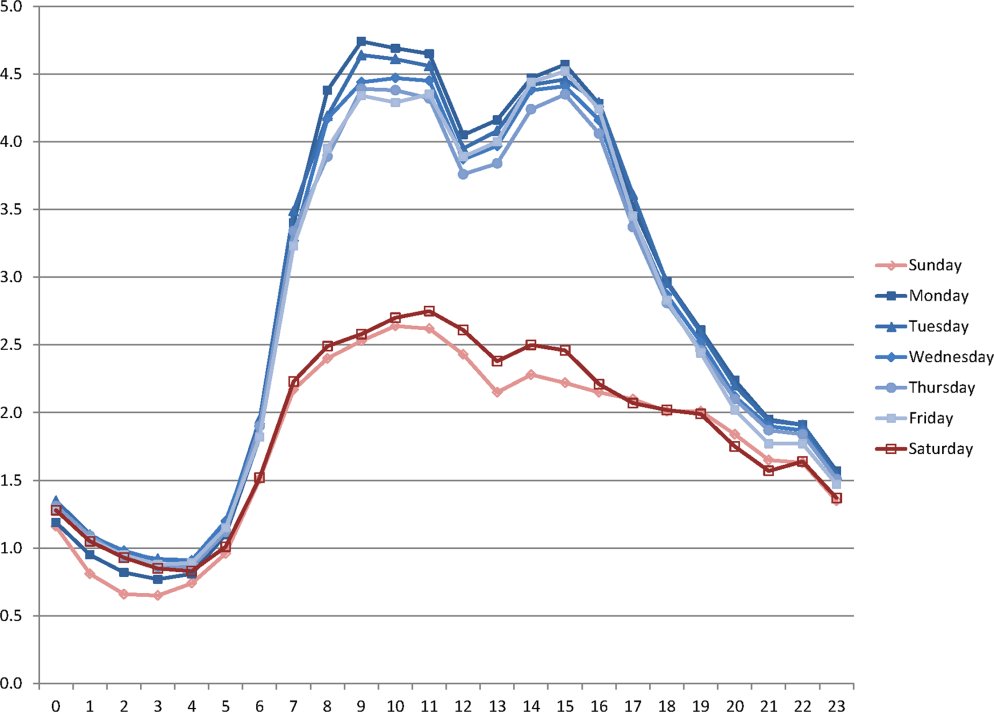
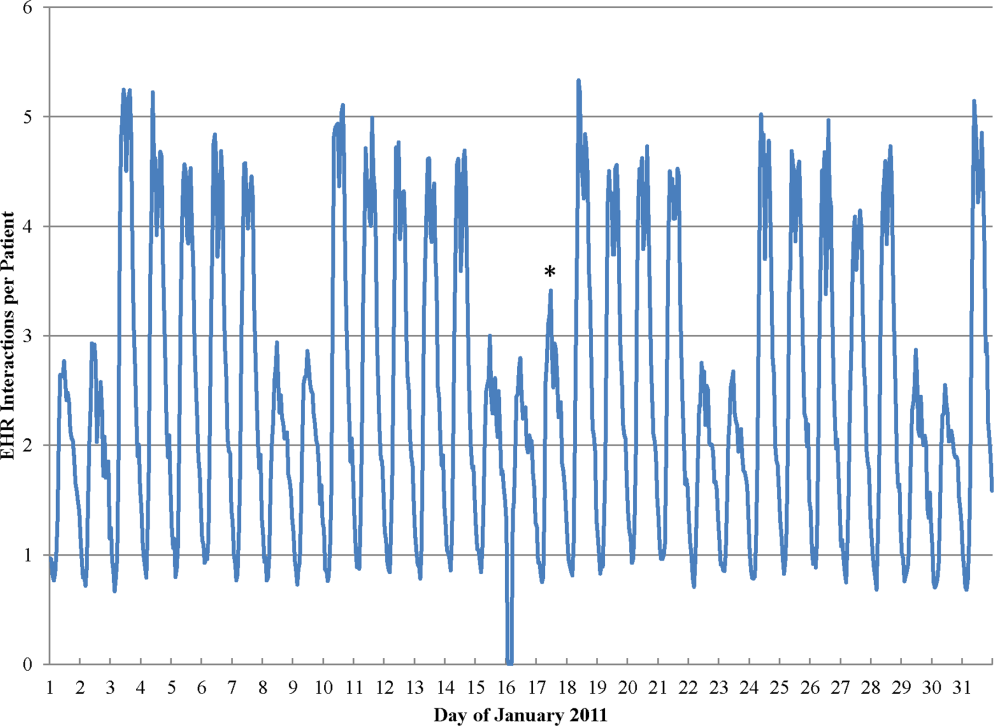
Nurses performed the largest number of EHR interactions (39.7%), followed by resident physicians (15.2%), attending physicians (10.2%), and pharmacists (7.6%). The remainder of EHR interactions were performed by other providers (27.4%), whose role in the majority of cases was undefined in the EHR (see Supporting Table 1 in the online version of this article). Daily variation in EHR interactions differed by provider type (Table 1 ). Nurses and resident physicians showed smaller differences in their EHR interactions between weekend and weekdays and among times of day when compared to attending physicians, pharmacy staff, and other staff. EHR interactions showed similar variations to the overall cohort for both medicine patients admitted from the ER and elective surgery patients (Table 1 ). Specific clinical orders designed to sample particularly meaningful interactions, including urinary catheter insertion and removal, patient mobilization orders, and new antibiotic orders, were moderately correlated with total EHR interactions (Table 2 ). In a sensitivity analysis, the comparison of weekday to weekend EHR interactions per daily discharge was similar to the primary analysis, with relative rates of 1.75 (95% CI: 1.73‐1.77), 1.54 (95% CI: 1.50‐1.57), and 1.15 (95% CI: 1.11‐1.18) for day, morning/evening, and night hours, respectively.
Hour Group a | |||||||
---|---|---|---|---|---|---|---|
Daytime Hours | Morning/Evening Hours | Nighttime Hours | Correlation Coefficient to | ||||
Rate Ratio | 95% CI | Rate Ratio | 95% CI | Rate Ratio | 95% CI | EHR Interactions | |
| |||||||
All EHR interactions | 1.76 | 1.74‐1.77 | 1.52 | 1.50‐1.55 | 1.14 | 1.12‐1.17 | 1.00 |
By provider subgroup | |||||||
Nurses | 1.35 | 1.34‐1.37 | 1.21 | 1.20‐1.22 | 1.10 | 1.08‐1.12 | 0.92 |
Residents | 1.38 | 1.36‐1.40 | 1.46 | 1.43‐1.49 | 1.14 | 1.11‐1.18 | 0.90 |
Attending physicians | 1.70 | 1.67‐1.73 | 2.04 | 1.97‐2.10 | 1.32 | 1.26‐1.39 | 0.96 |
Pharmacy staff | 1.64 | 1.61‐1.67 | 1.68 | 1.61‐1.75 | 1.19 | 1.15‐1.23 | 0.90 |
All others | 2.75 | 2.70‐2.79 | 2.08 | 2.002.17 | 1.20 | 1.15‐1.25 | 0.97 |
By admission subgroup | |||||||
Medicine ER admissions | |||||||
All | 1.64 | 1.62‐1.67 | 1.41 | 1.38‐1.44 | 1.07 | 1.04‐1.09 | 0.92 |
Day admissions | 1.64 | 1.62‐1.67 | 1.41 | 1.38‐1.44 | 1.08 | 1.05‐1.11 | 0.92 |
Night admissions | 1.65 | 1.62‐1.68 | 1.41 | 1.37‐1.45 | 1.03 | 1.001.05 | 0.88 |
Weekday admissions | 1.76 | 1.73‐1.79 | 1.56 | 1.52‐1.59 | 1.28 | 1.23‐1.30 | 0.93 |
Weekend admissions | 1.40 | 1.38‐1.42 | 1.14 | 1.11‐1.17 | 0.70 | 0.68‐0.72 | 0.88 |
Surgery elective admissions | |||||||
All | 1.63 | 1.61‐1.65 | 1.53 | 1.50‐1.56 | 1.25 | 1.21‐1.28 | 0.97 |
Day admissions | 1.62 | 1.60‐1.64 | 1.54 | 1.51‐1.57 | 1.26 | 1.23‐1.30 | 0.96 |
Night admissions | 1.65 | 1.62‐1.67 | 1.50 | 1.46‐1.54 | 1.22 | 1.18‐1.25 | 0.94 |
Weekday admissions | 1.64 | 1.62‐1.66 | 1.55 | 1.52‐1.59 | 1.27 | 1.24‐1.31 | 0.96 |
Weekend admissions | 1.60 | 1.56‐1.65 | 1.26 | 1.21‐1.31 | 0.89 | 0.85‐0.93 | 0.73 |
Hour Group a | |||||||
---|---|---|---|---|---|---|---|
Daytime Hours | Morning/Evening Hours | Nighttime Hours | Correlation Coefficient to | ||||
Rate Ratio | 95% CI | Rate Ratio | 95% CI | Rate Ratio | 95% CI | EHR Interactions | |
| |||||||
Urinary catheter | 2.81 | 2.61‐3.02 | 3.17 | 2.85‐3.54 | 1.42 | 1.27‐1.58 | 0.66 |
Mobilization | 2.51 | 2.39‐2.63 | 2.91 | 2.71‐3.12 | 1.42 | 1.34‐1.52 | 0.70 |
Antibiotic initiation b | 1.33 | 1.24‐1.42 | 1.65 | 1.47‐1.85 | 1.13 | 1.01‐1.25 | 0.41 |
Antibiotic discontinue c | 1.74 | 1.63‐1.85 | 1.68 | 1.50‐1.87 | 1.28 | 1.13‐1.45 | 0.61 |
As seen in Figure 3 , CPU usage was well correlated with EHR interactions ( r =0.90) and both metrics were lower on weekends as compared to weekdays. A few outliers of increased CPU usage on weekends were observed; these outliers all occurred on Sundays from 12:00 am to 2:00 am . The information technology (IT) department at our institution confirmed that these outliers coincided with a weekly virus scan performed at that time.
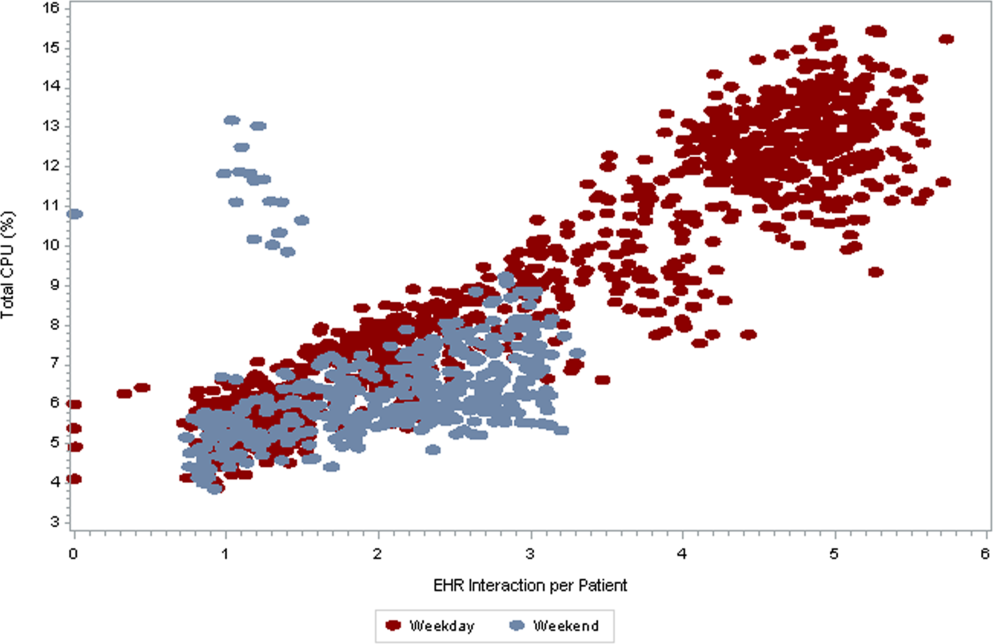
am
and 1:00
am
, which were determined to be outliers related to a weekly virus scan. The correlation coefficient is 0.90.
DISCUSSION
EHR interactions represents a new and accessible measure of hospital care intensity that may be used to track temporal variations in care that are likely to exist in all hospital systems. As the number of hospitals that process and document all clinical activities through the EHR continues to increase, [22, 23] such a measure has the potential to serve as a useful metric in efforts to raise nighttime and weekend care to the same quality as that during weekdays.
We found that EHR interactions differed markedly between days and nights and between weekdays and weekends. A number of prior studies have found temporal variations in clinical outcomes and care. [1, 2, 3, 4, 5, 6, 7, 8, 9, 10, 11, 12, 13, 14] Our study adds to this literature by defining a new measure of the overall intensity of the process of inpatient care. Using this measure, we demonstrate that the increase in midday clinical activity seen during the week is substantially blunted on weekends. This finding adds validity to the designation of both nights and weekends as off hours when examining temporal variations in clinical care. [2, 7]
Other subtle patterns of care delivery emerged throughout the day. For instance, we found a decrease in EHR interactions between 12:00 pm and 2:00 pm , likely representing a small lull in clinical activity during the lunchtime hour, whereas EHR interactions were higher in the night and evening hours than in the early morning. The clinical significance of such fluctuations remains undetermined.
Variation throughout the week differed by provider type. Nurses and resident physicians, who are scheduled around the clock, demonstrated the least fluctuation in EHR interaction intensity over time. Attending physicians and other staff showed the greatest variation in weekday versus weekend care.
We defined a second measure of care intensity based on EHR utilization, which we named CPU usage. The advantage of using CPU as a metric is that it easy to measure and track as it is routinely monitored by hospital IT departments as part of regular maintenance. CPU usage can therefore be easily implemented in any institution with an EHR, whereas measuring EHR interactions requires EHR data abstraction and manipulation. The disadvantage of CPU usage as a measure is that it includes nonclinical reporting and other functions and therefore represents a less specific measure of clinical activity. Nonetheless, at our institution, nearly all clinical activities are processed or documented through the EHR, which is maintained on central servers, and CPU usage was well correlated with EHR interactions. We therefore believe that CPU usage represents a useful indicator of hospital clinical activity at a given time.
Several limitations deserve mention. First, EHR interactions may not always reflect actual patient care or clinical documentation. Nonetheless, we also examined specific patient orders and found that variations in these process measures were similar to those in the EHR interaction measure. Second, our methods were developed and evaluated at a single institution and may not be generalizable. Third, increased intensity of care does not necessarily increase quality of care. For instance, laboratory tests are commonly obtained on a daily basis in the hospital, a practice that is costly and often unnecessary. [24] Weekends have been associated with fewer laboratory tests, which may be appropriate as compared to the more frequent testing observed on weekdays. [25] Finally, we do not ascertain whether variations in EHR interactions correlate with clinical outcomes, and believe this to be an area for further research.
In conclusion, EHR interactions represent a new global process measure of care intensity, which was demonstrated to vary over the course of a week. We intend to use this measure at our institution to track progress of an initiative to ensure a high standard of care throughout the course of the week. The extent to which temporal variations in EHR interactions or reductions in these variations are correlated with clinical outcomes deserves further study. We believe this measure, which can be adapted to other institutions, may have a valuable role to play in hospitals' efforts to eliminate excess morbidity and mortality associated with care delivered during nights and weekends.
- Impact of weekend admissions on quality of care and outcomes in patients with acute myeloid leukemia . Cancer. 2010 ; 116 : 3614 – 3620 . , , , et al.
- Impact of time of presentation on the care and outcomes of acute myocardial infarction . Circulation. 2008 ; 117 : 2502 – 2509 . , , , et al.
- Weekend hospital admission and discharge for heart failure: association with quality of care and clinical outcomes . Am Heart J. 2009 ; 158 : 451 – 458 . , , , et al.
- Hospital care for patients experiencing weekend vs weekday stroke: a comparison of quality and aggressiveness of care . Arch Neurol. 2010 ; 67 : 39 – 44 . , , , .
- Weekend versus weekday admission and mortality from myocardial infarction . N Engl J Med. 2007 ; 356 : 1099 – 1109 . , , , , , .
- Do hospitals provide lower quality care on weekends? Health Serv Res. 2007 ; 42 : 1589 – 1612 . .
- Relationship between time of day, day of week, timeliness of reperfusion, and in‐hospital mortality for patients with acute ST‐segment elevation myocardial infarction . JAMA. 2005 ; 294 : 803 – 812 . , , , et al.
- Weekend versus weekday admission and mortality after acute pulmonary embolism . Circulation. 2009 ; 119 : 962 – 968 . , , , , , .
- Influence of weekend hospital admission on short‐term mortality after intracerebral hemorrhage . Stroke. 2009 ; 40 : 2387 – 2392 . , , , , , .
- Weekend hospital admission, acute kidney injury, and mortality . J Am Soc Nephrol. 2010 ; 21 : 845 – 851 . , , , et al.
- Mortality among patients admitted to hospitals on weekends as compared with weekdays . N Engl J Med. 2001 ; 345 : 663 – 668 . , .
- Mortality after noncardiac surgery: prediction from administrative versus clinical data . Med Care. 2005 ; 43 : 159 – 167 . , , , et al.
- Weekend birth and higher neonatal mortality: a problem of patient acuity or quality of care? J Obstet Gynecol Neonatal Nurs. 2003 ; 32 : 724 – 733 . , .
- Analysis of the mortality of patients admitted to internal medicine wards over the weekend . Am J Med Qual. 2010 ; 25 : 312 – 318 . , , , , , .
- Do weekends or evenings matter in a pediatric intensive care unit? Pediatr Crit Care Med. 2005 ; 6 : 523 – 530 . , , , .
- Hospital mortality associated with day and time of admission to intensive care units . Intensive Care Medicine. 2004 ; 30 : 895 – 901 . , , , , .
- Weekend and weeknight admissions have the same outcome of weekday admissions to an intensive care unit with onsite intensivist coverage . Crit Care Med. 2006 ; 34 : 605 – 611 . , , .
- Measuring the intensity of nursing care: making use of the Belgian Nursing Minimum Data Set . Int J Nurs Stud. 2008 ; 45 : 1011 – 1021 . , , , , .
- The relationship between intensity and duration of medical services and outcomes for hospitalized patients . Med Care. 1979 ; 17 : 1088 – 1102 . , , , , .
- Differences in length of hospital stay for Medicaid and Blue Cross patients and the effect of intensity of services . Public Health Rep. 1979 ; 94 : 438 – 445 . .
- Health care's lost weekend. New York Times . October 3, 2010 . Section A, p. 27 . .
- A progress report on electronic health records in U.S. hospitals . Health Aff (Millwood). 2010 ; 29 : 1951 – 1957 . , , , .
- US Department of Health 146 : 524 – 527 .
- Temporal approach to hematological test usage in a major teaching hospital . Lab Hematol. 2003 ; 9 : 207 – 213 . , , .
Hospitals provide acute inpatient care all day and every day. Nonetheless, a number of studies have shown that weekend care may have lower quality than care delivered on weekdays. [1, 2, 3, 4, 5, 6, 7] Weekend care has been associated with increased mortality among patients suffering from a number of conditions [4, 5, 6, 7, 8, 9, 10] and in a number of clinical settings. [11, 12, 13, 14] Findings of poor outcomes on weekends are not universal, though exceptions occur typically in settings where services and clinical staffing are fairly constant throughout the week, such as intensive care units with on‐site intensivists. [15, 16, 17]
These differences in quality and outcomes suggest that there is a difference in intensity of care over the course of the week. Initiatives to address this important safety issue would be strengthened if a shared metric existed to describe the intensity of care provided at a given time of day or day of the week. However, few measures of global hospital intensity of care exist. The metrics that have been developed are limited by measuring only 1 aspect of care delivery, such as weekend nurse staffing, [18] by requiring extensive chart abstraction, [19] or through reliance on hospital expenses in an older payment model. [20] These measures notably predate contemporary hospital care delivery, which is increasingly dependent on the electronic health record (EHR). To our knowledge, no prior measure of hospital care intensity has been described using the EHR.
Recently, our medical center began an initiative to increase weekend services and staffing to create more uniform availability of care throughout the week. The purpose of this study was to develop a global measure of intensity of care using data derived from the EHR as part of the evaluation of this initiative. [21]
METHODS
We conducted a retrospective analysis of EHR activity for hospital inpatients between January 1, 2011 and December 31, 2011 at New York University Langone Medical Center (NYULMC). During that time period, nearly all inpatient clinical activities at NYULMC were performed and documented through the EHR, Sunrise Clinical Manager (Allscripts, Chicago, IL). These activities include clinical documentation, orders, medication administration, and results of diagnostic tests. Access to the EHR is through Citrix Xenapp Servers (Citrix Systems, Santa Clara, CA), which securely deliver applications from central servers to providers' local terminals.
The primary measure of EHR activity, which we refer to as EHR interactions, was defined as the accessing of a patient's electronic record by a clinician. A provider initiates an interaction by opening a patient's electronic record and concludes it by either opening the record of a different patient or logging out of the EHR. An EHR interaction thus captures a unit of direct patient care, such as documenting a clinical encounter, recording medication administration, or reviewing patient data. Most EHR systems routinely log such interactions to enable compliance departments to audit which users have accessed a patient chart.
The secondary measure of EHR activity was percent central processing unit (CPU), which represents the percent of total available server processing power being used by the Citrix servers at a given time. CPU utilization was averaged over an hour to determine the mean percentage of use for any given hour. As CPU data are not retained for more than 30 days at our institution, we examined CPU usage for the period July 1, 2012 to August 31, 2012.
We evaluated subgroups of EHR interactions by provider type: nurses, resident physicians, attending physicians, pharmacy staff, and all others. We also examined EHR interactions for 2 subgroups of patients: those admitted to the medicine service from the emergency room (ER) and those electively admitted to the surgical service. An elective hospitalization was defined as 1 in which the patient was neither admitted from the ER nor transferred from another hospital. These 2 subgroups were further refined to evaluate EHR interactions among groups of patients admitted during the day, night, weekday, and weeknight. Finally, we examined specific EHR orders that we considered reflective of advancing or altering care, including orders to increase the level of mobilization, to insert or remove a urinary catheter, or to initiate or discontinue antibiotics. As antibiotics are frequently initiated on the day of admission and stopped on the day of discharge, we excluded antibiotic orders that occurred on days of admission or discharge.
Statistical Analysis
EHR interactions were presented as hourly rates by dividing the number of patient chart accessions by the inpatient census for each hour. Hourly census was determined by summing the number of patients who were admitted prior to and discharged after the hour of interest. We calculated the arithmetic means of EHR interactions for each hour and day of the week in 2011. Analysis of variance was used to test differences in EHR interactions among days of the week, and t tests were used to test differences in EHR interactions between Saturday and Sunday, Tuesday and Wednesday, and weekend and weekdays. As a result of multiple comparisons in these analyses, we applied a Bonferroni correction.
EHR interaction rates were assigned to 1 of 3 periods based on a priori suspected peak and trough intensity: day (9:00 am to 4:59 pm ), morning/evening (7:00 am to 8:59 am and 5:00 pm to 7:59 pm ), and night (8:00 pm to 6:59 am ). Negative binomial regression models were used to determine the relative rate of weekday to weekend EHR interactions per patient for the 3 daily time periods. Similar models were developed for EHR interactions by provider types and for specific orders.
We calculated the correlation coefficient between total EHR interactions and occurrence of specific orders, EHR interactions by provider type, EHR interactions by patient subgroups, and CPU. A sensitivity analysis was performed in which EHR interactions were calculated as the number of patient chart accessions per number of daily discharges; this analysis considered the fact that discharges were likely to have high associated intensity but may be less common on weekends as compared to weekdays.
All analyses were performed using Stata 12 (StataCorp, College Station, TX). This study was approved by the institutional review board at NYU School of Medicine.
RESULTS
During the study period, the mean (standard deviation) number of EHR interactions per patient per hour was 2.49 (1.30). EHR interactions differed by hour and day of the week; the lowest number occurred in early morning on weekends, and the highest occurred around 11:00 am on weekdays (Figure 1 ). EHR interactions differed among days of the week at all times ( P <0.001), whereas EHR interactions were similar on most hours of Saturday and Sunday as well as Tuesday and Wednesday. At every hour, weekends had a lower number of EHR interactions in comparison to weekdays ( P <0.001). Weekdays showed a substantial increase in the number of EHR interactions between 8:00 am and 12:00 pm , followed by a slight decrease in activity between 12:00 pm and 2:00 pm , and another increase in activity from 2:00 pm to 5:00 pm (Figure 1 ). Weekend days demonstrated marked blunting of this midday peak in intensity. The tracking of an entire month of hospital EHR interactions produced a detailed graphic picture of hospital activity, clearly demarcating rounding hours, lunch hours, weekend days, hospital holidays, and other landmarks (Figure 2 ). The relative rates of census‐adjusted EHR interactions on weekdays versus weekends were 1.76 (95% confidence interval [CI]: 1.74‐1.77) for day, 1.52 (95% CI: 1.50‐1.55) for morning/evening, and 1.14 (95% CI: 1.12‐1.17) for night hours.
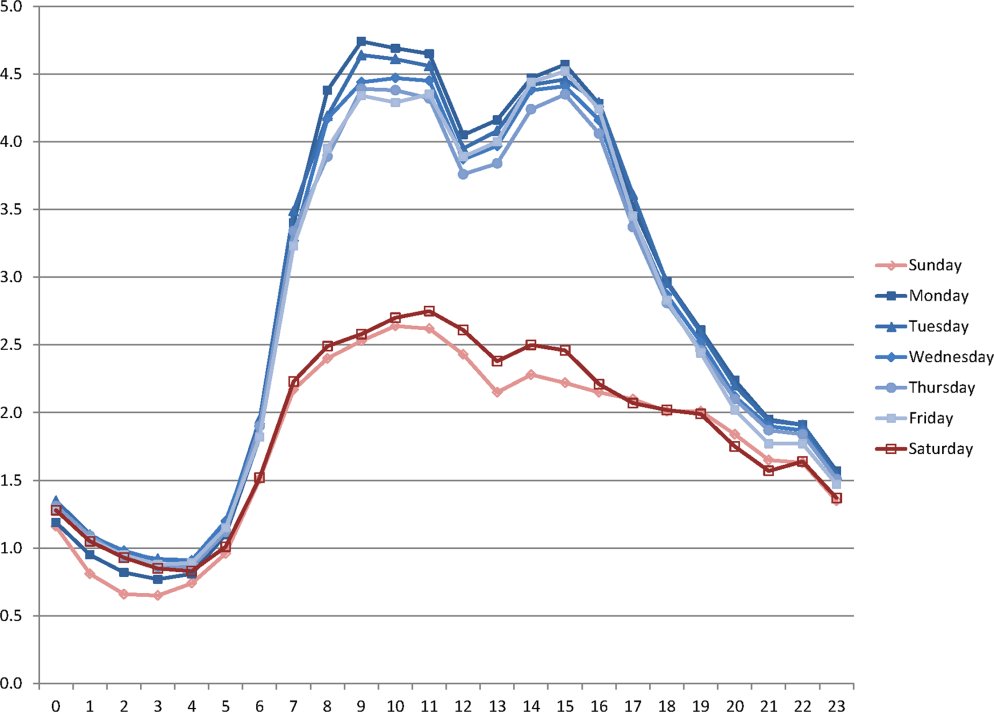
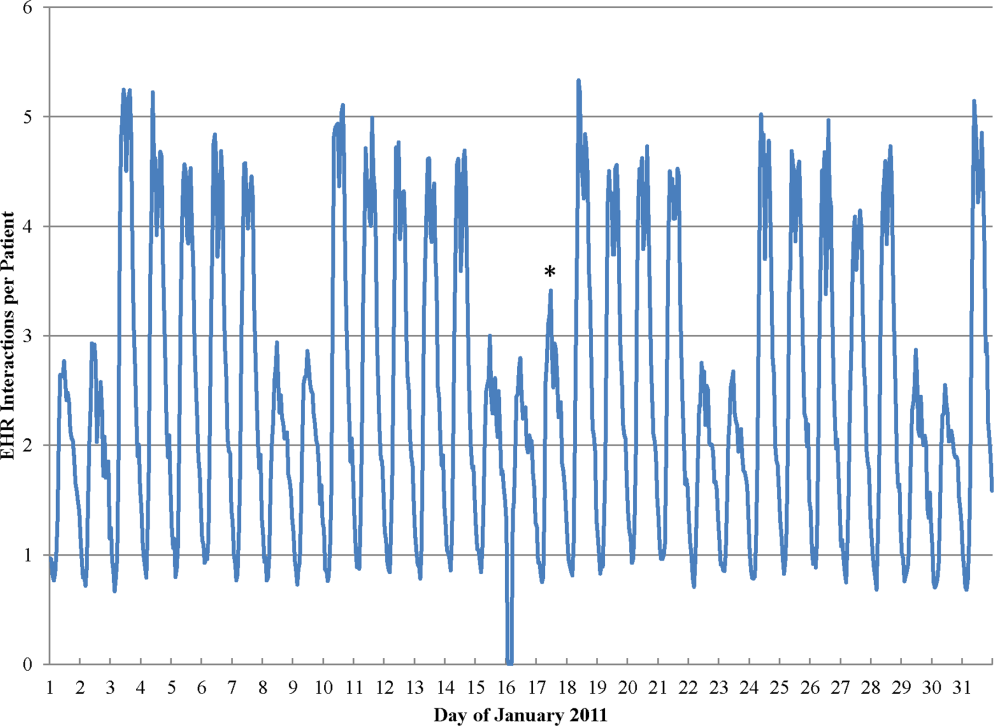
Nurses performed the largest number of EHR interactions (39.7%), followed by resident physicians (15.2%), attending physicians (10.2%), and pharmacists (7.6%). The remainder of EHR interactions were performed by other providers (27.4%), whose role in the majority of cases was undefined in the EHR (see Supporting Table 1 in the online version of this article). Daily variation in EHR interactions differed by provider type (Table 1 ). Nurses and resident physicians showed smaller differences in their EHR interactions between weekend and weekdays and among times of day when compared to attending physicians, pharmacy staff, and other staff. EHR interactions showed similar variations to the overall cohort for both medicine patients admitted from the ER and elective surgery patients (Table 1 ). Specific clinical orders designed to sample particularly meaningful interactions, including urinary catheter insertion and removal, patient mobilization orders, and new antibiotic orders, were moderately correlated with total EHR interactions (Table 2 ). In a sensitivity analysis, the comparison of weekday to weekend EHR interactions per daily discharge was similar to the primary analysis, with relative rates of 1.75 (95% CI: 1.73‐1.77), 1.54 (95% CI: 1.50‐1.57), and 1.15 (95% CI: 1.11‐1.18) for day, morning/evening, and night hours, respectively.
Hour Group a | |||||||
---|---|---|---|---|---|---|---|
Daytime Hours | Morning/Evening Hours | Nighttime Hours | Correlation Coefficient to | ||||
Rate Ratio | 95% CI | Rate Ratio | 95% CI | Rate Ratio | 95% CI | EHR Interactions | |
| |||||||
All EHR interactions | 1.76 | 1.74‐1.77 | 1.52 | 1.50‐1.55 | 1.14 | 1.12‐1.17 | 1.00 |
By provider subgroup | |||||||
Nurses | 1.35 | 1.34‐1.37 | 1.21 | 1.20‐1.22 | 1.10 | 1.08‐1.12 | 0.92 |
Residents | 1.38 | 1.36‐1.40 | 1.46 | 1.43‐1.49 | 1.14 | 1.11‐1.18 | 0.90 |
Attending physicians | 1.70 | 1.67‐1.73 | 2.04 | 1.97‐2.10 | 1.32 | 1.26‐1.39 | 0.96 |
Pharmacy staff | 1.64 | 1.61‐1.67 | 1.68 | 1.61‐1.75 | 1.19 | 1.15‐1.23 | 0.90 |
All others | 2.75 | 2.70‐2.79 | 2.08 | 2.002.17 | 1.20 | 1.15‐1.25 | 0.97 |
By admission subgroup | |||||||
Medicine ER admissions | |||||||
All | 1.64 | 1.62‐1.67 | 1.41 | 1.38‐1.44 | 1.07 | 1.04‐1.09 | 0.92 |
Day admissions | 1.64 | 1.62‐1.67 | 1.41 | 1.38‐1.44 | 1.08 | 1.05‐1.11 | 0.92 |
Night admissions | 1.65 | 1.62‐1.68 | 1.41 | 1.37‐1.45 | 1.03 | 1.001.05 | 0.88 |
Weekday admissions | 1.76 | 1.73‐1.79 | 1.56 | 1.52‐1.59 | 1.28 | 1.23‐1.30 | 0.93 |
Weekend admissions | 1.40 | 1.38‐1.42 | 1.14 | 1.11‐1.17 | 0.70 | 0.68‐0.72 | 0.88 |
Surgery elective admissions | |||||||
All | 1.63 | 1.61‐1.65 | 1.53 | 1.50‐1.56 | 1.25 | 1.21‐1.28 | 0.97 |
Day admissions | 1.62 | 1.60‐1.64 | 1.54 | 1.51‐1.57 | 1.26 | 1.23‐1.30 | 0.96 |
Night admissions | 1.65 | 1.62‐1.67 | 1.50 | 1.46‐1.54 | 1.22 | 1.18‐1.25 | 0.94 |
Weekday admissions | 1.64 | 1.62‐1.66 | 1.55 | 1.52‐1.59 | 1.27 | 1.24‐1.31 | 0.96 |
Weekend admissions | 1.60 | 1.56‐1.65 | 1.26 | 1.21‐1.31 | 0.89 | 0.85‐0.93 | 0.73 |
Hour Group a | |||||||
---|---|---|---|---|---|---|---|
Daytime Hours | Morning/Evening Hours | Nighttime Hours | Correlation Coefficient to | ||||
Rate Ratio | 95% CI | Rate Ratio | 95% CI | Rate Ratio | 95% CI | EHR Interactions | |
| |||||||
Urinary catheter | 2.81 | 2.61‐3.02 | 3.17 | 2.85‐3.54 | 1.42 | 1.27‐1.58 | 0.66 |
Mobilization | 2.51 | 2.39‐2.63 | 2.91 | 2.71‐3.12 | 1.42 | 1.34‐1.52 | 0.70 |
Antibiotic initiation b | 1.33 | 1.24‐1.42 | 1.65 | 1.47‐1.85 | 1.13 | 1.01‐1.25 | 0.41 |
Antibiotic discontinue c | 1.74 | 1.63‐1.85 | 1.68 | 1.50‐1.87 | 1.28 | 1.13‐1.45 | 0.61 |
As seen in Figure 3 , CPU usage was well correlated with EHR interactions ( r =0.90) and both metrics were lower on weekends as compared to weekdays. A few outliers of increased CPU usage on weekends were observed; these outliers all occurred on Sundays from 12:00 am to 2:00 am . The information technology (IT) department at our institution confirmed that these outliers coincided with a weekly virus scan performed at that time.
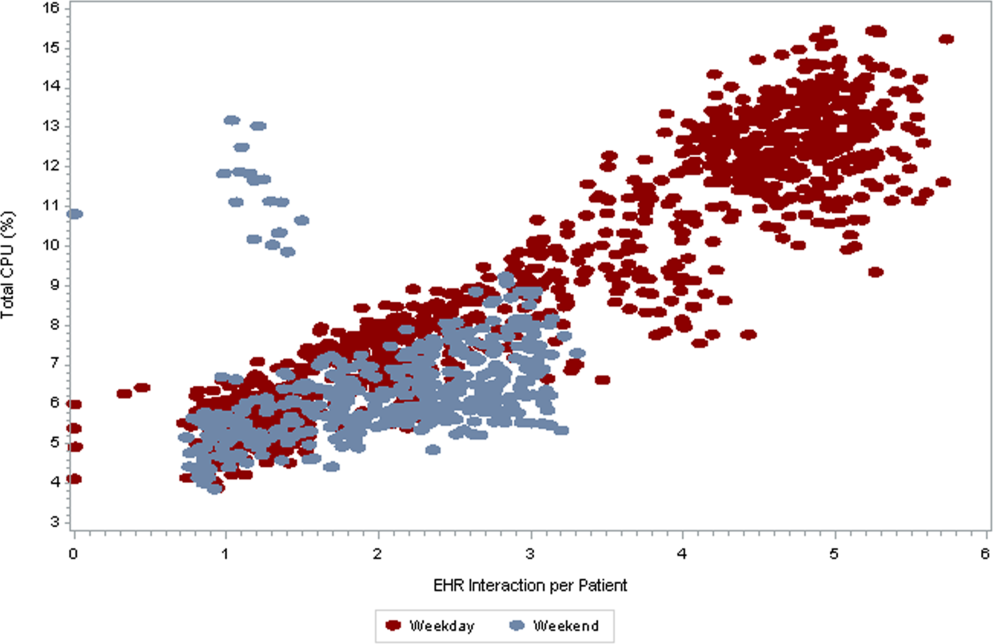
am
and 1:00
am
, which were determined to be outliers related to a weekly virus scan. The correlation coefficient is 0.90.
DISCUSSION
EHR interactions represents a new and accessible measure of hospital care intensity that may be used to track temporal variations in care that are likely to exist in all hospital systems. As the number of hospitals that process and document all clinical activities through the EHR continues to increase, [22, 23] such a measure has the potential to serve as a useful metric in efforts to raise nighttime and weekend care to the same quality as that during weekdays.
We found that EHR interactions differed markedly between days and nights and between weekdays and weekends. A number of prior studies have found temporal variations in clinical outcomes and care. [1, 2, 3, 4, 5, 6, 7, 8, 9, 10, 11, 12, 13, 14] Our study adds to this literature by defining a new measure of the overall intensity of the process of inpatient care. Using this measure, we demonstrate that the increase in midday clinical activity seen during the week is substantially blunted on weekends. This finding adds validity to the designation of both nights and weekends as off hours when examining temporal variations in clinical care. [2, 7]
Other subtle patterns of care delivery emerged throughout the day. For instance, we found a decrease in EHR interactions between 12:00 pm and 2:00 pm , likely representing a small lull in clinical activity during the lunchtime hour, whereas EHR interactions were higher in the night and evening hours than in the early morning. The clinical significance of such fluctuations remains undetermined.
Variation throughout the week differed by provider type. Nurses and resident physicians, who are scheduled around the clock, demonstrated the least fluctuation in EHR interaction intensity over time. Attending physicians and other staff showed the greatest variation in weekday versus weekend care.
We defined a second measure of care intensity based on EHR utilization, which we named CPU usage. The advantage of using CPU as a metric is that it easy to measure and track as it is routinely monitored by hospital IT departments as part of regular maintenance. CPU usage can therefore be easily implemented in any institution with an EHR, whereas measuring EHR interactions requires EHR data abstraction and manipulation. The disadvantage of CPU usage as a measure is that it includes nonclinical reporting and other functions and therefore represents a less specific measure of clinical activity. Nonetheless, at our institution, nearly all clinical activities are processed or documented through the EHR, which is maintained on central servers, and CPU usage was well correlated with EHR interactions. We therefore believe that CPU usage represents a useful indicator of hospital clinical activity at a given time.
Several limitations deserve mention. First, EHR interactions may not always reflect actual patient care or clinical documentation. Nonetheless, we also examined specific patient orders and found that variations in these process measures were similar to those in the EHR interaction measure. Second, our methods were developed and evaluated at a single institution and may not be generalizable. Third, increased intensity of care does not necessarily increase quality of care. For instance, laboratory tests are commonly obtained on a daily basis in the hospital, a practice that is costly and often unnecessary. [24] Weekends have been associated with fewer laboratory tests, which may be appropriate as compared to the more frequent testing observed on weekdays. [25] Finally, we do not ascertain whether variations in EHR interactions correlate with clinical outcomes, and believe this to be an area for further research.
In conclusion, EHR interactions represent a new global process measure of care intensity, which was demonstrated to vary over the course of a week. We intend to use this measure at our institution to track progress of an initiative to ensure a high standard of care throughout the course of the week. The extent to which temporal variations in EHR interactions or reductions in these variations are correlated with clinical outcomes deserves further study. We believe this measure, which can be adapted to other institutions, may have a valuable role to play in hospitals' efforts to eliminate excess morbidity and mortality associated with care delivered during nights and weekends.
Hospitals provide acute inpatient care all day and every day. Nonetheless, a number of studies have shown that weekend care may have lower quality than care delivered on weekdays. [1, 2, 3, 4, 5, 6, 7] Weekend care has been associated with increased mortality among patients suffering from a number of conditions [4, 5, 6, 7, 8, 9, 10] and in a number of clinical settings. [11, 12, 13, 14] Findings of poor outcomes on weekends are not universal, though exceptions occur typically in settings where services and clinical staffing are fairly constant throughout the week, such as intensive care units with on‐site intensivists. [15, 16, 17]
These differences in quality and outcomes suggest that there is a difference in intensity of care over the course of the week. Initiatives to address this important safety issue would be strengthened if a shared metric existed to describe the intensity of care provided at a given time of day or day of the week. However, few measures of global hospital intensity of care exist. The metrics that have been developed are limited by measuring only 1 aspect of care delivery, such as weekend nurse staffing, [18] by requiring extensive chart abstraction, [19] or through reliance on hospital expenses in an older payment model. [20] These measures notably predate contemporary hospital care delivery, which is increasingly dependent on the electronic health record (EHR). To our knowledge, no prior measure of hospital care intensity has been described using the EHR.
Recently, our medical center began an initiative to increase weekend services and staffing to create more uniform availability of care throughout the week. The purpose of this study was to develop a global measure of intensity of care using data derived from the EHR as part of the evaluation of this initiative. [21]
METHODS
We conducted a retrospective analysis of EHR activity for hospital inpatients between January 1, 2011 and December 31, 2011 at New York University Langone Medical Center (NYULMC). During that time period, nearly all inpatient clinical activities at NYULMC were performed and documented through the EHR, Sunrise Clinical Manager (Allscripts, Chicago, IL). These activities include clinical documentation, orders, medication administration, and results of diagnostic tests. Access to the EHR is through Citrix Xenapp Servers (Citrix Systems, Santa Clara, CA), which securely deliver applications from central servers to providers' local terminals.
The primary measure of EHR activity, which we refer to as EHR interactions, was defined as the accessing of a patient's electronic record by a clinician. A provider initiates an interaction by opening a patient's electronic record and concludes it by either opening the record of a different patient or logging out of the EHR. An EHR interaction thus captures a unit of direct patient care, such as documenting a clinical encounter, recording medication administration, or reviewing patient data. Most EHR systems routinely log such interactions to enable compliance departments to audit which users have accessed a patient chart.
The secondary measure of EHR activity was percent central processing unit (CPU), which represents the percent of total available server processing power being used by the Citrix servers at a given time. CPU utilization was averaged over an hour to determine the mean percentage of use for any given hour. As CPU data are not retained for more than 30 days at our institution, we examined CPU usage for the period July 1, 2012 to August 31, 2012.
We evaluated subgroups of EHR interactions by provider type: nurses, resident physicians, attending physicians, pharmacy staff, and all others. We also examined EHR interactions for 2 subgroups of patients: those admitted to the medicine service from the emergency room (ER) and those electively admitted to the surgical service. An elective hospitalization was defined as 1 in which the patient was neither admitted from the ER nor transferred from another hospital. These 2 subgroups were further refined to evaluate EHR interactions among groups of patients admitted during the day, night, weekday, and weeknight. Finally, we examined specific EHR orders that we considered reflective of advancing or altering care, including orders to increase the level of mobilization, to insert or remove a urinary catheter, or to initiate or discontinue antibiotics. As antibiotics are frequently initiated on the day of admission and stopped on the day of discharge, we excluded antibiotic orders that occurred on days of admission or discharge.
Statistical Analysis
EHR interactions were presented as hourly rates by dividing the number of patient chart accessions by the inpatient census for each hour. Hourly census was determined by summing the number of patients who were admitted prior to and discharged after the hour of interest. We calculated the arithmetic means of EHR interactions for each hour and day of the week in 2011. Analysis of variance was used to test differences in EHR interactions among days of the week, and t tests were used to test differences in EHR interactions between Saturday and Sunday, Tuesday and Wednesday, and weekend and weekdays. As a result of multiple comparisons in these analyses, we applied a Bonferroni correction.
EHR interaction rates were assigned to 1 of 3 periods based on a priori suspected peak and trough intensity: day (9:00 am to 4:59 pm ), morning/evening (7:00 am to 8:59 am and 5:00 pm to 7:59 pm ), and night (8:00 pm to 6:59 am ). Negative binomial regression models were used to determine the relative rate of weekday to weekend EHR interactions per patient for the 3 daily time periods. Similar models were developed for EHR interactions by provider types and for specific orders.
We calculated the correlation coefficient between total EHR interactions and occurrence of specific orders, EHR interactions by provider type, EHR interactions by patient subgroups, and CPU. A sensitivity analysis was performed in which EHR interactions were calculated as the number of patient chart accessions per number of daily discharges; this analysis considered the fact that discharges were likely to have high associated intensity but may be less common on weekends as compared to weekdays.
All analyses were performed using Stata 12 (StataCorp, College Station, TX). This study was approved by the institutional review board at NYU School of Medicine.
RESULTS
During the study period, the mean (standard deviation) number of EHR interactions per patient per hour was 2.49 (1.30). EHR interactions differed by hour and day of the week; the lowest number occurred in early morning on weekends, and the highest occurred around 11:00 am on weekdays (Figure 1 ). EHR interactions differed among days of the week at all times ( P <0.001), whereas EHR interactions were similar on most hours of Saturday and Sunday as well as Tuesday and Wednesday. At every hour, weekends had a lower number of EHR interactions in comparison to weekdays ( P <0.001). Weekdays showed a substantial increase in the number of EHR interactions between 8:00 am and 12:00 pm , followed by a slight decrease in activity between 12:00 pm and 2:00 pm , and another increase in activity from 2:00 pm to 5:00 pm (Figure 1 ). Weekend days demonstrated marked blunting of this midday peak in intensity. The tracking of an entire month of hospital EHR interactions produced a detailed graphic picture of hospital activity, clearly demarcating rounding hours, lunch hours, weekend days, hospital holidays, and other landmarks (Figure 2 ). The relative rates of census‐adjusted EHR interactions on weekdays versus weekends were 1.76 (95% confidence interval [CI]: 1.74‐1.77) for day, 1.52 (95% CI: 1.50‐1.55) for morning/evening, and 1.14 (95% CI: 1.12‐1.17) for night hours.
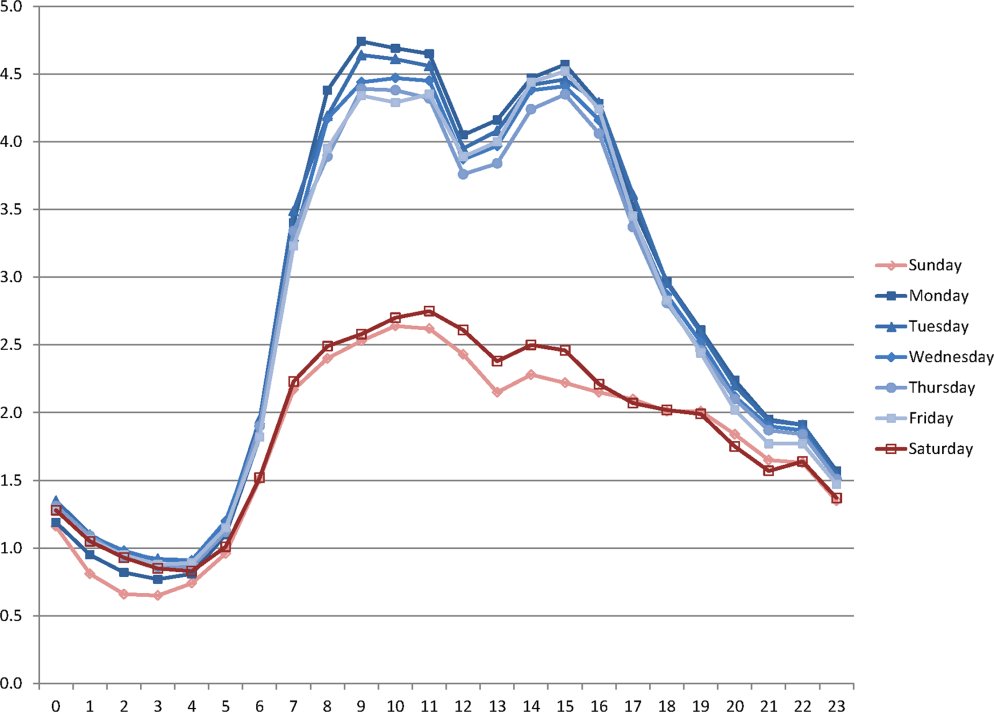
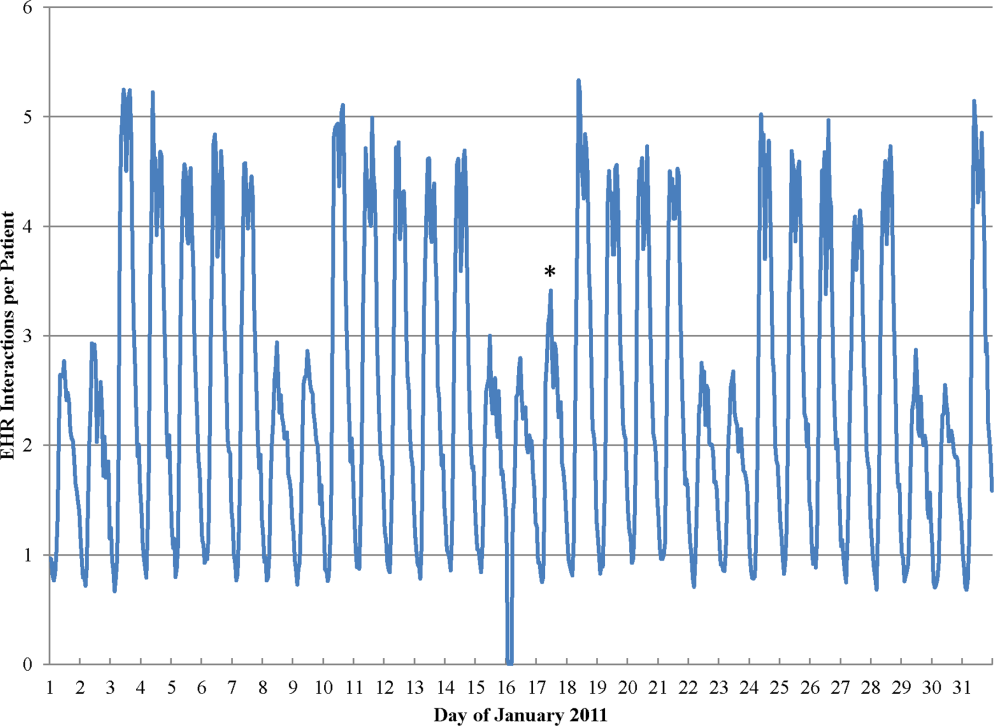
Nurses performed the largest number of EHR interactions (39.7%), followed by resident physicians (15.2%), attending physicians (10.2%), and pharmacists (7.6%). The remainder of EHR interactions were performed by other providers (27.4%), whose role in the majority of cases was undefined in the EHR (see Supporting Table 1 in the online version of this article). Daily variation in EHR interactions differed by provider type (Table 1 ). Nurses and resident physicians showed smaller differences in their EHR interactions between weekend and weekdays and among times of day when compared to attending physicians, pharmacy staff, and other staff. EHR interactions showed similar variations to the overall cohort for both medicine patients admitted from the ER and elective surgery patients (Table 1 ). Specific clinical orders designed to sample particularly meaningful interactions, including urinary catheter insertion and removal, patient mobilization orders, and new antibiotic orders, were moderately correlated with total EHR interactions (Table 2 ). In a sensitivity analysis, the comparison of weekday to weekend EHR interactions per daily discharge was similar to the primary analysis, with relative rates of 1.75 (95% CI: 1.73‐1.77), 1.54 (95% CI: 1.50‐1.57), and 1.15 (95% CI: 1.11‐1.18) for day, morning/evening, and night hours, respectively.
Hour Group a | |||||||
---|---|---|---|---|---|---|---|
Daytime Hours | Morning/Evening Hours | Nighttime Hours | Correlation Coefficient to | ||||
Rate Ratio | 95% CI | Rate Ratio | 95% CI | Rate Ratio | 95% CI | EHR Interactions | |
| |||||||
All EHR interactions | 1.76 | 1.74‐1.77 | 1.52 | 1.50‐1.55 | 1.14 | 1.12‐1.17 | 1.00 |
By provider subgroup | |||||||
Nurses | 1.35 | 1.34‐1.37 | 1.21 | 1.20‐1.22 | 1.10 | 1.08‐1.12 | 0.92 |
Residents | 1.38 | 1.36‐1.40 | 1.46 | 1.43‐1.49 | 1.14 | 1.11‐1.18 | 0.90 |
Attending physicians | 1.70 | 1.67‐1.73 | 2.04 | 1.97‐2.10 | 1.32 | 1.26‐1.39 | 0.96 |
Pharmacy staff | 1.64 | 1.61‐1.67 | 1.68 | 1.61‐1.75 | 1.19 | 1.15‐1.23 | 0.90 |
All others | 2.75 | 2.70‐2.79 | 2.08 | 2.002.17 | 1.20 | 1.15‐1.25 | 0.97 |
By admission subgroup | |||||||
Medicine ER admissions | |||||||
All | 1.64 | 1.62‐1.67 | 1.41 | 1.38‐1.44 | 1.07 | 1.04‐1.09 | 0.92 |
Day admissions | 1.64 | 1.62‐1.67 | 1.41 | 1.38‐1.44 | 1.08 | 1.05‐1.11 | 0.92 |
Night admissions | 1.65 | 1.62‐1.68 | 1.41 | 1.37‐1.45 | 1.03 | 1.001.05 | 0.88 |
Weekday admissions | 1.76 | 1.73‐1.79 | 1.56 | 1.52‐1.59 | 1.28 | 1.23‐1.30 | 0.93 |
Weekend admissions | 1.40 | 1.38‐1.42 | 1.14 | 1.11‐1.17 | 0.70 | 0.68‐0.72 | 0.88 |
Surgery elective admissions | |||||||
All | 1.63 | 1.61‐1.65 | 1.53 | 1.50‐1.56 | 1.25 | 1.21‐1.28 | 0.97 |
Day admissions | 1.62 | 1.60‐1.64 | 1.54 | 1.51‐1.57 | 1.26 | 1.23‐1.30 | 0.96 |
Night admissions | 1.65 | 1.62‐1.67 | 1.50 | 1.46‐1.54 | 1.22 | 1.18‐1.25 | 0.94 |
Weekday admissions | 1.64 | 1.62‐1.66 | 1.55 | 1.52‐1.59 | 1.27 | 1.24‐1.31 | 0.96 |
Weekend admissions | 1.60 | 1.56‐1.65 | 1.26 | 1.21‐1.31 | 0.89 | 0.85‐0.93 | 0.73 |
Hour Group a | |||||||
---|---|---|---|---|---|---|---|
Daytime Hours | Morning/Evening Hours | Nighttime Hours | Correlation Coefficient to | ||||
Rate Ratio | 95% CI | Rate Ratio | 95% CI | Rate Ratio | 95% CI | EHR Interactions | |
| |||||||
Urinary catheter | 2.81 | 2.61‐3.02 | 3.17 | 2.85‐3.54 | 1.42 | 1.27‐1.58 | 0.66 |
Mobilization | 2.51 | 2.39‐2.63 | 2.91 | 2.71‐3.12 | 1.42 | 1.34‐1.52 | 0.70 |
Antibiotic initiation b | 1.33 | 1.24‐1.42 | 1.65 | 1.47‐1.85 | 1.13 | 1.01‐1.25 | 0.41 |
Antibiotic discontinue c | 1.74 | 1.63‐1.85 | 1.68 | 1.50‐1.87 | 1.28 | 1.13‐1.45 | 0.61 |
As seen in Figure 3 , CPU usage was well correlated with EHR interactions ( r =0.90) and both metrics were lower on weekends as compared to weekdays. A few outliers of increased CPU usage on weekends were observed; these outliers all occurred on Sundays from 12:00 am to 2:00 am . The information technology (IT) department at our institution confirmed that these outliers coincided with a weekly virus scan performed at that time.
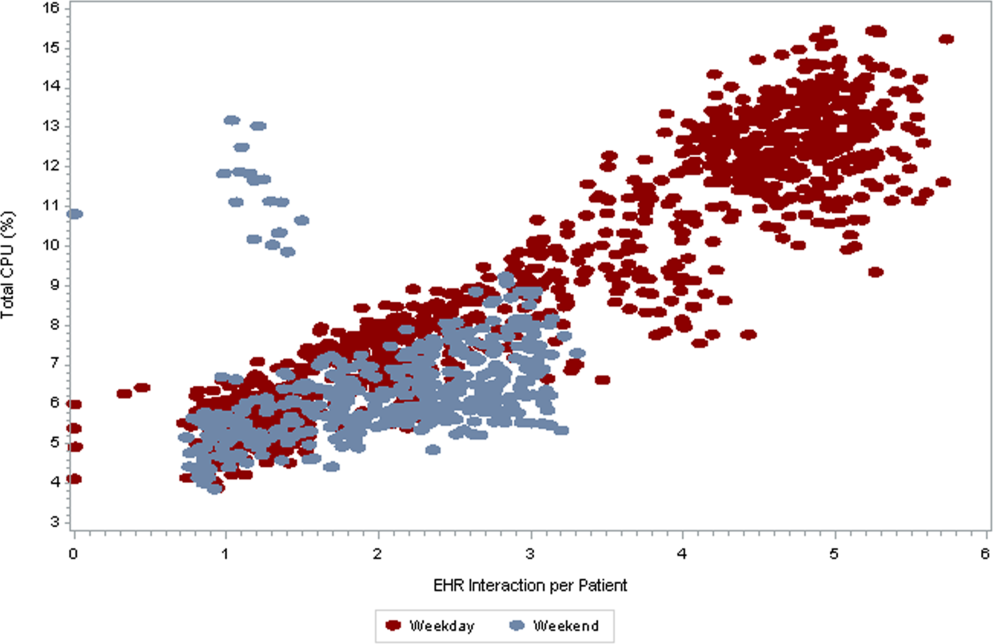
am
and 1:00
am
, which were determined to be outliers related to a weekly virus scan. The correlation coefficient is 0.90.
DISCUSSION
EHR interactions represents a new and accessible measure of hospital care intensity that may be used to track temporal variations in care that are likely to exist in all hospital systems. As the number of hospitals that process and document all clinical activities through the EHR continues to increase, [22, 23] such a measure has the potential to serve as a useful metric in efforts to raise nighttime and weekend care to the same quality as that during weekdays.
We found that EHR interactions differed markedly between days and nights and between weekdays and weekends. A number of prior studies have found temporal variations in clinical outcomes and care. [1, 2, 3, 4, 5, 6, 7, 8, 9, 10, 11, 12, 13, 14] Our study adds to this literature by defining a new measure of the overall intensity of the process of inpatient care. Using this measure, we demonstrate that the increase in midday clinical activity seen during the week is substantially blunted on weekends. This finding adds validity to the designation of both nights and weekends as off hours when examining temporal variations in clinical care. [2, 7]
Other subtle patterns of care delivery emerged throughout the day. For instance, we found a decrease in EHR interactions between 12:00 pm and 2:00 pm , likely representing a small lull in clinical activity during the lunchtime hour, whereas EHR interactions were higher in the night and evening hours than in the early morning. The clinical significance of such fluctuations remains undetermined.
Variation throughout the week differed by provider type. Nurses and resident physicians, who are scheduled around the clock, demonstrated the least fluctuation in EHR interaction intensity over time. Attending physicians and other staff showed the greatest variation in weekday versus weekend care.
We defined a second measure of care intensity based on EHR utilization, which we named CPU usage. The advantage of using CPU as a metric is that it easy to measure and track as it is routinely monitored by hospital IT departments as part of regular maintenance. CPU usage can therefore be easily implemented in any institution with an EHR, whereas measuring EHR interactions requires EHR data abstraction and manipulation. The disadvantage of CPU usage as a measure is that it includes nonclinical reporting and other functions and therefore represents a less specific measure of clinical activity. Nonetheless, at our institution, nearly all clinical activities are processed or documented through the EHR, which is maintained on central servers, and CPU usage was well correlated with EHR interactions. We therefore believe that CPU usage represents a useful indicator of hospital clinical activity at a given time.
Several limitations deserve mention. First, EHR interactions may not always reflect actual patient care or clinical documentation. Nonetheless, we also examined specific patient orders and found that variations in these process measures were similar to those in the EHR interaction measure. Second, our methods were developed and evaluated at a single institution and may not be generalizable. Third, increased intensity of care does not necessarily increase quality of care. For instance, laboratory tests are commonly obtained on a daily basis in the hospital, a practice that is costly and often unnecessary. [24] Weekends have been associated with fewer laboratory tests, which may be appropriate as compared to the more frequent testing observed on weekdays. [25] Finally, we do not ascertain whether variations in EHR interactions correlate with clinical outcomes, and believe this to be an area for further research.
In conclusion, EHR interactions represent a new global process measure of care intensity, which was demonstrated to vary over the course of a week. We intend to use this measure at our institution to track progress of an initiative to ensure a high standard of care throughout the course of the week. The extent to which temporal variations in EHR interactions or reductions in these variations are correlated with clinical outcomes deserves further study. We believe this measure, which can be adapted to other institutions, may have a valuable role to play in hospitals' efforts to eliminate excess morbidity and mortality associated with care delivered during nights and weekends.
- Impact of weekend admissions on quality of care and outcomes in patients with acute myeloid leukemia . Cancer. 2010 ; 116 : 3614 – 3620 . , , , et al.
- Impact of time of presentation on the care and outcomes of acute myocardial infarction . Circulation. 2008 ; 117 : 2502 – 2509 . , , , et al.
- Weekend hospital admission and discharge for heart failure: association with quality of care and clinical outcomes . Am Heart J. 2009 ; 158 : 451 – 458 . , , , et al.
- Hospital care for patients experiencing weekend vs weekday stroke: a comparison of quality and aggressiveness of care . Arch Neurol. 2010 ; 67 : 39 – 44 . , , , .
- Weekend versus weekday admission and mortality from myocardial infarction . N Engl J Med. 2007 ; 356 : 1099 – 1109 . , , , , , .
- Do hospitals provide lower quality care on weekends? Health Serv Res. 2007 ; 42 : 1589 – 1612 . .
- Relationship between time of day, day of week, timeliness of reperfusion, and in‐hospital mortality for patients with acute ST‐segment elevation myocardial infarction . JAMA. 2005 ; 294 : 803 – 812 . , , , et al.
- Weekend versus weekday admission and mortality after acute pulmonary embolism . Circulation. 2009 ; 119 : 962 – 968 . , , , , , .
- Influence of weekend hospital admission on short‐term mortality after intracerebral hemorrhage . Stroke. 2009 ; 40 : 2387 – 2392 . , , , , , .
- Weekend hospital admission, acute kidney injury, and mortality . J Am Soc Nephrol. 2010 ; 21 : 845 – 851 . , , , et al.
- Mortality among patients admitted to hospitals on weekends as compared with weekdays . N Engl J Med. 2001 ; 345 : 663 – 668 . , .
- Mortality after noncardiac surgery: prediction from administrative versus clinical data . Med Care. 2005 ; 43 : 159 – 167 . , , , et al.
- Weekend birth and higher neonatal mortality: a problem of patient acuity or quality of care? J Obstet Gynecol Neonatal Nurs. 2003 ; 32 : 724 – 733 . , .
- Analysis of the mortality of patients admitted to internal medicine wards over the weekend . Am J Med Qual. 2010 ; 25 : 312 – 318 . , , , , , .
- Do weekends or evenings matter in a pediatric intensive care unit? Pediatr Crit Care Med. 2005 ; 6 : 523 – 530 . , , , .
- Hospital mortality associated with day and time of admission to intensive care units . Intensive Care Medicine. 2004 ; 30 : 895 – 901 . , , , , .
- Weekend and weeknight admissions have the same outcome of weekday admissions to an intensive care unit with onsite intensivist coverage . Crit Care Med. 2006 ; 34 : 605 – 611 . , , .
- Measuring the intensity of nursing care: making use of the Belgian Nursing Minimum Data Set . Int J Nurs Stud. 2008 ; 45 : 1011 – 1021 . , , , , .
- The relationship between intensity and duration of medical services and outcomes for hospitalized patients . Med Care. 1979 ; 17 : 1088 – 1102 . , , , , .
- Differences in length of hospital stay for Medicaid and Blue Cross patients and the effect of intensity of services . Public Health Rep. 1979 ; 94 : 438 – 445 . .
- Health care's lost weekend. New York Times . October 3, 2010 . Section A, p. 27 . .
- A progress report on electronic health records in U.S. hospitals . Health Aff (Millwood). 2010 ; 29 : 1951 – 1957 . , , , .
- US Department of Health 146 : 524 – 527 .
- Temporal approach to hematological test usage in a major teaching hospital . Lab Hematol. 2003 ; 9 : 207 – 213 . , , .
- Impact of weekend admissions on quality of care and outcomes in patients with acute myeloid leukemia . Cancer. 2010 ; 116 : 3614 – 3620 . , , , et al.
- Impact of time of presentation on the care and outcomes of acute myocardial infarction . Circulation. 2008 ; 117 : 2502 – 2509 . , , , et al.
- Weekend hospital admission and discharge for heart failure: association with quality of care and clinical outcomes . Am Heart J. 2009 ; 158 : 451 – 458 . , , , et al.
- Hospital care for patients experiencing weekend vs weekday stroke: a comparison of quality and aggressiveness of care . Arch Neurol. 2010 ; 67 : 39 – 44 . , , , .
- Weekend versus weekday admission and mortality from myocardial infarction . N Engl J Med. 2007 ; 356 : 1099 – 1109 . , , , , , .
- Do hospitals provide lower quality care on weekends? Health Serv Res. 2007 ; 42 : 1589 – 1612 . .
- Relationship between time of day, day of week, timeliness of reperfusion, and in‐hospital mortality for patients with acute ST‐segment elevation myocardial infarction . JAMA. 2005 ; 294 : 803 – 812 . , , , et al.
- Weekend versus weekday admission and mortality after acute pulmonary embolism . Circulation. 2009 ; 119 : 962 – 968 . , , , , , .
- Influence of weekend hospital admission on short‐term mortality after intracerebral hemorrhage . Stroke. 2009 ; 40 : 2387 – 2392 . , , , , , .
- Weekend hospital admission, acute kidney injury, and mortality . J Am Soc Nephrol. 2010 ; 21 : 845 – 851 . , , , et al.
- Mortality among patients admitted to hospitals on weekends as compared with weekdays . N Engl J Med. 2001 ; 345 : 663 – 668 . , .
- Mortality after noncardiac surgery: prediction from administrative versus clinical data . Med Care. 2005 ; 43 : 159 – 167 . , , , et al.
- Weekend birth and higher neonatal mortality: a problem of patient acuity or quality of care? J Obstet Gynecol Neonatal Nurs. 2003 ; 32 : 724 – 733 . , .
- Analysis of the mortality of patients admitted to internal medicine wards over the weekend . Am J Med Qual. 2010 ; 25 : 312 – 318 . , , , , , .
- Do weekends or evenings matter in a pediatric intensive care unit? Pediatr Crit Care Med. 2005 ; 6 : 523 – 530 . , , , .
- Hospital mortality associated with day and time of admission to intensive care units . Intensive Care Medicine. 2004 ; 30 : 895 – 901 . , , , , .
- Weekend and weeknight admissions have the same outcome of weekday admissions to an intensive care unit with onsite intensivist coverage . Crit Care Med. 2006 ; 34 : 605 – 611 . , , .
- Measuring the intensity of nursing care: making use of the Belgian Nursing Minimum Data Set . Int J Nurs Stud. 2008 ; 45 : 1011 – 1021 . , , , , .
- The relationship between intensity and duration of medical services and outcomes for hospitalized patients . Med Care. 1979 ; 17 : 1088 – 1102 . , , , , .
- Differences in length of hospital stay for Medicaid and Blue Cross patients and the effect of intensity of services . Public Health Rep. 1979 ; 94 : 438 – 445 . .
- Health care's lost weekend. New York Times . October 3, 2010 . Section A, p. 27 . .
- A progress report on electronic health records in U.S. hospitals . Health Aff (Millwood). 2010 ; 29 : 1951 – 1957 . , , , .
- US Department of Health 146 : 524 – 527 .
- Temporal approach to hematological test usage in a major teaching hospital . Lab Hematol. 2003 ; 9 : 207 – 213 . , , .
Copyright © 2013 Society of Hospital Medicine
[email protected]