User login
Hospital Readmissions in End of Life
The need to improve end‐of‐life care is well recognized. Its quality is often poor, and its cost is enormous, with 30% of the Medicare expenditures used for medical treatments of the 6% of beneficiaries who die each year.[1, 2] Repeated hospitalizations are frequent toward the end of life,[3] where each admission should be viewed as an opportunity to initiate advance care planning to improve end‐of‐life care and possibly reduce future unnecessary readmissions.[4, 5] Identified problems include undertreatment of pain, lack of awareness of patient wishes or advance directives, and unwanted overtreatment.
To improve quality and reduce unnecessary hospital use near the end of life, there is an urgent need to help healthcare providers to better identify the most vulnerable and at‐risk patients to provide them with care coordination and supportive care services. We aimed to identify the risk factors for having a 30‐day potentially avoidable readmission (PAR) due to end‐of‐life care issues.
METHODS
Study Design and Population
A nested case‐control study was designed where potentially avoidable end‐of‐life readmissions were compared to nonreadmitted controls. We collected data on all consecutive adult patient admissions to any medical services of the Brigham and Women's Hospital with a discharge date between July 1, 2009 and June 30, 2010. Brigham and Women's Hospital is a 780‐bed academic medical center in Boston, Massachusetts. To avoid observation stays, only admissions with a length of stay of more than 1 day were included. We excluded patients who died before discharge, were transferred to another acute care hospital, and those who left against medical advice. We also excluded patients with no available data on medication treatment at discharge. The protocol was approved by the institutional review board of Brigham and Women's Hospital/Partners Healthcare.
Study Outcome
The study outcome was any 30‐day PAR due to end‐of‐life issues. To determine this outcome, first we identified all 30‐day readmissions to any service of 3 hospitals within the Partners network in Boston that followed the index hospitalization (prior studies have shown that these hospitals capture approximately 80% of readmissions after a Brigham and Women's Hospital medical hospitalization).[6, 7] These readmissions were subsequently differentiated as potentially avoidable or not using a validated algorithm (SQLape; SQLape, Corseaux, Switzerland).[8, 9] This algorithm uses administrative data and International Classification of Diseases, 9th Revision, Clinical Modification codes from the index and repeat hospitalization. Readmissions were considered potentially avoidable if they were: (1) readmissions related to previously known conditions during the index hospitalization, or (2) complications of treatment (eg, deep vein thrombosis, drug‐induced disorders). Conversely, readmissions were considered unavoidable if they were: (1) foreseen (such as readmissions for transplantation, delivery, chemo‐ or radiotherapy, and other specific surgical procedures), (2) follow‐up and rehabilitation treatments, or (3) readmissions for a new condition unknown during the preceding hospitalization. The algorithm has both a sensitivity and specificity of 96% compared with medical record review using the same criteria. Finally, a random sample of the 30‐day PARs was reviewed independently by 9 trained senior resident physicians to identify those due to end‐of‐life issues, defined by the following 2 criteria: (1) patient has a terminal clinical condition, such as malignancy, end stage renal disease, end stage congestive heart failure, or other condition with a life expectancy of 6 months or less; and (2) the readmission is part of the terminal disease process that was not adequately addressed during the index hospitalization. Examples of factors that were used when identifying cases included lack of healthcare proxy and lack of documentation of why end‐of‐life discussions did not take place during the index hospitalization. Training of adjudicators included a didactic session and review of standardized cases.
Risk Factors
We collected candidate risk factors based on a priori knowledge and according to the medical literature,[10, 11, 12] including demographic information, previous healthcare utilization, and index hospitalization characteristics from administrative data sources; procedures and chronic medical conditions from billing data; last laboratory values and medication information prior to discharge from the electronic medical record (Table 1). When laboratory values were missing (<1%), values were considered as normal.
Characteristics | No 30‐Day Readmission, n=7,974 | 30‐Day PAR due to End of Life, n=80 | P Value |
---|---|---|---|
| |||
Age, y, mean (SD) | 61.5 (16.6) | 60.8 (11.9) | 0.69 |
Male sex, n (%) | 3875 (48.6) | 37 (46.3) | 0.69 |
Ethnicity, n (%) | 0.05 | ||
Non‐Hispanic white* | 5772 (72.4) | 69 (86.3) | |
Non‐Hispanic black | 1281 (16.1) | 4 (5.0) | |
Hispanic | 666 (8.4) | 5 (6.3) | |
Other | 255 (3.2) | 2 (2.5) | |
Language, n (%) | 0.99 | ||
English* | 7254 (91.0) | 73 (91.3) | |
Spanish | 415 (5.2) | 4 (5.0) | |
Other | 305 (3.8) | 3 (3.8) | |
Marital status, n (%) | 0.37 | ||
Currently married or partner* | 4107 (51.35) | 46 (57.5) | |
Single/never married | 1967 (24.7) | 14 (17.5) | |
Separated/divorced/widowed/no answer | 1900 (23.8) | 20 (25.0) | |
Source of index admission, n (%) | 0.10 | ||
Direct from home/outpatient clinic | 2456 (30.8) | 33 (41.3) | |
Emergency department* | 4222 (53.0) | 34 (42.5) | |
Nursing home/rehabilitation/other hospital | 1296 (16.3) | 13 (16.3) | |
Length of stay of the index admission, median (IQR) | 4 (27) | 5.5 (38] | 0.13 |
No. of hospital admissions in the past year, median (IQR) | 1 (02) | 2 (03) | <0.001 |
Any procedure during the hospital stay, n (%) | 4809 (60.3) | 57 (71.3) | 0.05 |
Identified caregiver at discharge | 7300 (91.6) | 76 (95.0) | 0.27 |
No. of medications at discharge, mean (SD) | 10.6 (5.1) | 13.0 (5.0) | <0.001 |
No. of opiate medication at discharge | <0.001 | ||
0 | 5297 (66.4) | 21 (26.3) | |
1 | 2677 (33.2) | 59 (73.8) | |
Elixhauser, median (IQR) | 8 (215) | 23 (1442) | <0.001 |
Selected comorbidities, n (%) | |||
Diabetes mellitus | 1971 (24.7) | 20 (25.0) | 0.96 |
Heart failure | 1756 (22.0) | 11 (13.8) | 0.10 |
Atrial fibrillation | 1439 (18.1) | 10 (12.5) | 0.20 |
COPD | 816 (10.2) | 7 (8.8) | 0.66 |
Neoplasm | 2705 (33.9) | 69 (86.3) | <0.001 |
Stroke | 294 (3.7) | 2 (2.5) | 0.57 |
ESRD | 1258 (15.8) | 6 (7.5) | 0.04 |
Liver disease | 328 (4.1) | 2 (2.5) | 0.47 |
Statistical Analysis
We first conducted a bivariate analysis on all collected potential risk factors, comparing admissions followed by a 30‐day PAR due to end‐of‐life care issues with admissions not followed by any 30‐day readmission, using the Pearson [2] test for categorical variables and Student t test for continuous variables. Then, we performed a multivariable logistic regression restricted to the variables that were found significantly associated with the outcome in the bivariate analysis. Age and Elixhauser comorbidity index were forced into the model as important potential confounders. Because a patient could have several outcomes over the study period, we used general estimating equations to cluster at the patient level. All tests were conducted as 2‐sided at a 0.05 level of significance. Analyses were performed using the SAS system for Windows, version 9.3 (SAS Institute Inc., Cary, NC).
RESULTS
From the total of 12,383 patients who were discharged from the medical services of the Brigham and Women's Hospital during the study period, 2108 (17.0%) were excluded because of: (1) death before discharge, (2) transfer to another acute care hospital, (3) discharge against medical advice, or (4) missing data (Figure 1). Among the 10,275 eligible admissions, 22.3% (n=2301) were followed by a 30‐day readmission. Of these, 826 (8.0% of all admissions) were identified as potentially avoidable. Among a random sample of 534 PARs, 80 (15.0%) were related to end‐of‐life care issues (cases). Of note, only 16 (20%) of these patients received palliative care consultation during the index hospitalization. A total of 7974 discharges were not followed by any 30‐day readmission (controls).
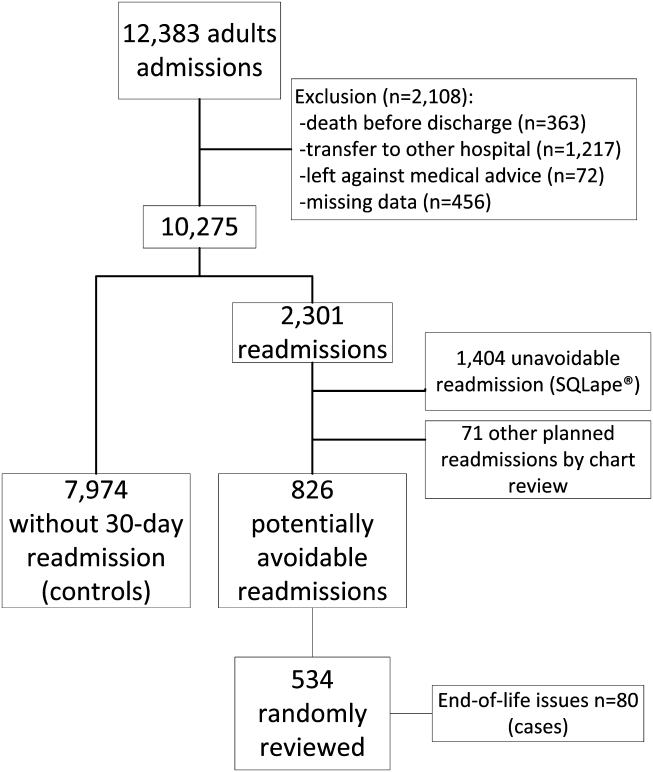
Baseline characteristics are presented in Table 1. Among the combined cohort of cases plus controls, the patient's mean age at inclusion was 61.3 years, and about half were male. In bivariate analysis, demographics such as age and sex were similar between cases and controls. Cases had more hospitalizations in the previous year, a higher number of medications at discharge, and a higher Elixhauser comorbidity index. When looking at diseases more specifically, neoplasm was significantly associated with potentially avoidable 30‐day readmission due to end‐of‐life care issues. In contrast, end‐stage renal disease was associated with a significantly lower risk of 30‐day PAR due to end‐of‐life care issues.
In multivariate analysis, 4 factors remained significantly associated with 30‐day PAR due to end‐of‐life care issues (Table 2). Neoplasm was the strongest risk factor, with an odds ratio of 5.6 (95% confidence interval: 2.8511.0), followed by opiate medication use, Elixhauser score, and number of admissions in the previous 12 months.
Variable | Odds Ratio (95% CI) |
---|---|
| |
Age, per 10 years | 1.04 (0.911.19) |
No. of admissions in the previous 12 months, per admission | 1.10 (1.021.20)a |
Total no. of medications at discharge, per medication | 1.04 (1.001.10) |
Neoplasm | 5.60 (2.8511.0)a |
Endstage renal disease | 0.60 (0.251.42) |
Opiate medication at discharge | 2.29 (1.294.07)a |
Elixhauser, per 5 unit increase | 1.16 (1.101.22)a |
The model, including all 4 variables, had an excellent discrimination power, with a C statistic of 0.85. Without the Elixhauser score, the C statistic remained very high, with a value of 0.82.
DISCUSSION
In a large medical population, potentially avoidable readmissions due to end‐of‐life care issues were not uncommon: 15% of all potentially avoidable readmissions (1.2% of all discharges). We identified 4 main risk factors for having a 30‐day potentially avoidable readmission due to end‐of‐life care issues: neoplasm, opiate use, Elixhauser comorbidity index, and number of admissions in the previous year. In a model that includes these 4 variables, the discrimination was very high with a C statistic of 0.85.
This study extends prior work indicating some risk factors for the need for palliative care. Neoplasm has been logically identified as a criterion for palliative care assessment at the time of admission.[13] Patients with neoplasm are not only at overall high risk for readmission,[14, 15, 16] but they obviously represent a fragile population whose condition is often terminal. Our results suggest that still more attention may be necessary to reduce the risk of readmission due to end‐of‐life care issues in this population (for example, only 20% of cases in our study received palliative care consultation during the index hospitalization). The overall comorbidity measured by the Elixhauser index was not surprisingly a significant risk factor. It probably accounts for the burden of comorbidities, but also for other advanced diseases besides neoplasm, like heart failure, chronic obstructive pulmonary disease, and others that may also be terminal. The number of previous hospital admissions in the past year is also an important risk factor, not only for the general population,[10, 11, 14, 17, 18] but also for patients with more advanced conditions,[19, 20, 21, 22] where admissions become more frequent as the disease progresses toward end stage. Opioid use was the final statistically significant risk factor, specific for this population, likely as a proxy for disease severity and progression toward terminal illness, especially in combination with the other risk factors such as cancer. Age was not a significant factor in either bivariate or multivariate analysis. Previous studies on the risk factors for readmission among patients receiving palliative care also failed to show age as a significant factor.[23, 24] Both of these studies looked at readmissions among patients who were already receiving palliative care. Our study asks a fundamentally different (and in many ways a more practical) question: who among a large population of medical patients might benefit from receiving input from palliative care in the first place. The number of medications at discharge was no longer significant in the multivariate analysis, likely due to its collinearity with the Elixhauser comorbidity index. An increased number of medications might be associated with a higher risk of adverse drug events and readmission, but they would not be necessarily considered to be end‐of‐life readmissions. Taken together, the 4 variables provide a very promising prediction model with high discrimination. To our knowledge, there is no previous existing list of risk factors for 30‐day potentially avoidable readmission due to end‐of‐life care issues, and no existing model to help prioritize palliative care to the most high‐risk patients. It is worth noting that the Elixhauser score might be difficult to calculate before the discharge of the patient (although hospitals with electronic capture of medical problem lists might be able to approximate it). However, even without the Elixhauser score, the C statistic remained very high at 0.82.
Our study has several limitations. Although we looked at readmissions at 2 other affiliated hospitals, some patients might have been readmitted to other acute care facilities outside our network. However, we would not expect the risk factors in these patients to be so different. The identification of end‐of‐life care issues by medical record review is based on a subjective judgment, although strict criteria were used. Furthermore, differentiation between potentially avoidable readmission and unavoidable readmission cannot be perfect. We used clear and logical criteria that were previously validated and allow large database management. Also, we did not analyze a comprehensive list of potential risk factors. It is probable that functional or cognitive status, for example, could also be important risk factors. We purposely chose a set of variables that could be easily obtained from administrative data sources. The small number of cases may have led to limited statistical power to identify less strongly associated risk factors. Last, the results may not be completely generalizable to small or community hospitals, in particular those that may care for less severely ill cancer patients.
Our findings have important implications. End‐of‐life care issues are not infrequent causes of readmission. Our study's findings could help prioritize palliative care resources to those patients at higher risk to improve the quality of end‐of‐life care. The risk factors identified in this study could be used informally by physicians at the bedside to identify such patients. In addition, a hospital could use these factors to provide a second‐level screen, beyond clinician recognition, to assist palliative care teams to identify patients who may not have otherwise been referred. This screen could be automated, for example, by using a list of medical problems from an electronic medical record to approximate an Elixhauser comorbidity score, or even leaving comorbidities out and simply relying on the other 3 easily identifiable risk factors. Such efforts could have a substantial effect on improving care near the end of life and potentially reducing unnecessary hospitalizations.
Acknowledgements
The authors thank Yves Eggli for having screened the database for potentially avoidable readmission using the algorithm SQLape.
Disclosures: Dr. Donz was supported by the Swiss National Science Foundation and the Swiss Foundation for MedicalBiological Scholarships. The Swiss Science National Foundation and the Swiss Foundation for MedicalBiological Scholarships had no role in the design and conduct of this study, the analysis or interpretation of the data, or the preparation of this manuscript. Dr. Schnipper is a consultant to QuantiaMD, for which he has helped create online educational materials for both providers and patients regarding patient safety, including medication safety during transitions in care. The findings of this study are not a part of those materials. Dr. Schnipper has received grant funding from Sanofi‐Aventis for an investigator‐initiated study to design and evaluate an intensive discharge and follow‐up intervention in patients with diabetes. The funder had had no role in the design of the study.
- Medicare beneficiaries' costs of care in the last year of life. Health Aff (Millwood). 2001;20(4):188–195. , , , .
- Quality of End‐of‐Life Cancer Care for Medicare Beneficiaries: Regional and Hospital‐Specific Analyses. Lebanon, NH: The Dartmouth Institute for Health Policy and Clinical Practice; 2010. , , .
- Repeated hospitalizations predict mortality in the community population with heart failure. Am Heart J. 2007;154(2):260–266. , , .
- Perspectives on care at the close of life. Initiating end‐of‐life discussions with seriously ill patients: addressing the “elephant in the room.” JAMA. 2000;284(19):2502–2507. .
- Advance care planning as a process: structuring the discussions in practice. J Am Geriatr Soc. 1995;43(4):440–446. , , , .
- Rationale and design of the Pharmacist Intervention for Low Literacy in Cardiovascular Disease (PILL‐CVD) study. Circ Cardiovasc Qual Outcomes. 2010;3(2):212–219. , , , et al.
- Effect of a pharmacist intervention on clinically important medication errors after hospital discharge: a randomized trial. Ann Intern Med. 2012;157(1):1–10. , , , et al.
- Measuring potentially avoidable hospital readmissions. J Clin Epidemiol. 2002;55(6):573–587. , , , , , .
- Validation of the potentially avoidable hospital readmission rate as a routine indicator of the quality of hospital care. Med Care. 2006;44(11):972–981. , , , , , .
- Hospital readmission in general medicine patients: a prediction model. J Gen Intern Med. 2010;25(3):211–219. , , , et al.
- Screening elders for risk of hospital admission. J Am Geriatr Soc. 1993;41(8):811–817. , , , , , .
- Risk factors for 30‐day hospital readmission in patients ≥65 years of age. Proc (Bayl Univ Med Cent). 2008;21(4):363–372. , , , , .
- Identifying patients in need of a palliative care assessment in the hospital setting: a consensus report from the Center to Advance Palliative Care. J Palliat Med. 2011;14(1):17–23. , .
- Potentially avoidable 30‐day hospital readmissions in medical patients: derivation and validation of a prediction model. JAMA Intern Med. 2013;173(8):632–638. , , , .
- Redefining readmission risk factors for general medicine patients. J Hosp Med. 2011;6(2):54–60. , , , .
- Patient and disease profile of emergency medical readmissions to an Irish teaching hospital. Postgrad Med J. 2004;80(946):470–474. , , .
- Posthospital care transitions: patterns, complications, and risk identification. Health Serv Res. 2004;39(5):1449–1465. , , , .
- Factors predicting readmission of older general medicine patients. J Gen Intern Med. 1991;6(5):389–393. , .
- Differences in health care utilization at the end of life among patients with chronic obstructive pulmonary disease and patients with lung cancer. Arch Intern Med. 2006;166(3):326–331. , , , , .
- Frequent hospital readmissions for acute exacerbation of COPD and their associated factors. Respirology. 2006;11(2):188–195. , , , , .
- Consensus statement: Palliative and supportive care in advanced heart failure. J Card Fail. 2004;10(3):200–209. , , , et al.
- Unplanned discharges from a surgical intensive care unit: readmissions and mortality. J Crit Care. 2010;25(3):375–381. , , , , .
- Evaluating causes for unplanned hospital readmissions of palliative care patients. Am J Hosp Palliat Care. 2010;27(8):526–531. , , , , .
- 30‐day readmissions among seriously ill older adults. J Palliat Med. 2012;15(12):1356–1361. , , .
The need to improve end‐of‐life care is well recognized. Its quality is often poor, and its cost is enormous, with 30% of the Medicare expenditures used for medical treatments of the 6% of beneficiaries who die each year.[1, 2] Repeated hospitalizations are frequent toward the end of life,[3] where each admission should be viewed as an opportunity to initiate advance care planning to improve end‐of‐life care and possibly reduce future unnecessary readmissions.[4, 5] Identified problems include undertreatment of pain, lack of awareness of patient wishes or advance directives, and unwanted overtreatment.
To improve quality and reduce unnecessary hospital use near the end of life, there is an urgent need to help healthcare providers to better identify the most vulnerable and at‐risk patients to provide them with care coordination and supportive care services. We aimed to identify the risk factors for having a 30‐day potentially avoidable readmission (PAR) due to end‐of‐life care issues.
METHODS
Study Design and Population
A nested case‐control study was designed where potentially avoidable end‐of‐life readmissions were compared to nonreadmitted controls. We collected data on all consecutive adult patient admissions to any medical services of the Brigham and Women's Hospital with a discharge date between July 1, 2009 and June 30, 2010. Brigham and Women's Hospital is a 780‐bed academic medical center in Boston, Massachusetts. To avoid observation stays, only admissions with a length of stay of more than 1 day were included. We excluded patients who died before discharge, were transferred to another acute care hospital, and those who left against medical advice. We also excluded patients with no available data on medication treatment at discharge. The protocol was approved by the institutional review board of Brigham and Women's Hospital/Partners Healthcare.
Study Outcome
The study outcome was any 30‐day PAR due to end‐of‐life issues. To determine this outcome, first we identified all 30‐day readmissions to any service of 3 hospitals within the Partners network in Boston that followed the index hospitalization (prior studies have shown that these hospitals capture approximately 80% of readmissions after a Brigham and Women's Hospital medical hospitalization).[6, 7] These readmissions were subsequently differentiated as potentially avoidable or not using a validated algorithm (SQLape; SQLape, Corseaux, Switzerland).[8, 9] This algorithm uses administrative data and International Classification of Diseases, 9th Revision, Clinical Modification codes from the index and repeat hospitalization. Readmissions were considered potentially avoidable if they were: (1) readmissions related to previously known conditions during the index hospitalization, or (2) complications of treatment (eg, deep vein thrombosis, drug‐induced disorders). Conversely, readmissions were considered unavoidable if they were: (1) foreseen (such as readmissions for transplantation, delivery, chemo‐ or radiotherapy, and other specific surgical procedures), (2) follow‐up and rehabilitation treatments, or (3) readmissions for a new condition unknown during the preceding hospitalization. The algorithm has both a sensitivity and specificity of 96% compared with medical record review using the same criteria. Finally, a random sample of the 30‐day PARs was reviewed independently by 9 trained senior resident physicians to identify those due to end‐of‐life issues, defined by the following 2 criteria: (1) patient has a terminal clinical condition, such as malignancy, end stage renal disease, end stage congestive heart failure, or other condition with a life expectancy of 6 months or less; and (2) the readmission is part of the terminal disease process that was not adequately addressed during the index hospitalization. Examples of factors that were used when identifying cases included lack of healthcare proxy and lack of documentation of why end‐of‐life discussions did not take place during the index hospitalization. Training of adjudicators included a didactic session and review of standardized cases.
Risk Factors
We collected candidate risk factors based on a priori knowledge and according to the medical literature,[10, 11, 12] including demographic information, previous healthcare utilization, and index hospitalization characteristics from administrative data sources; procedures and chronic medical conditions from billing data; last laboratory values and medication information prior to discharge from the electronic medical record (Table 1). When laboratory values were missing (<1%), values were considered as normal.
Characteristics | No 30‐Day Readmission, n=7,974 | 30‐Day PAR due to End of Life, n=80 | P Value |
---|---|---|---|
| |||
Age, y, mean (SD) | 61.5 (16.6) | 60.8 (11.9) | 0.69 |
Male sex, n (%) | 3875 (48.6) | 37 (46.3) | 0.69 |
Ethnicity, n (%) | 0.05 | ||
Non‐Hispanic white* | 5772 (72.4) | 69 (86.3) | |
Non‐Hispanic black | 1281 (16.1) | 4 (5.0) | |
Hispanic | 666 (8.4) | 5 (6.3) | |
Other | 255 (3.2) | 2 (2.5) | |
Language, n (%) | 0.99 | ||
English* | 7254 (91.0) | 73 (91.3) | |
Spanish | 415 (5.2) | 4 (5.0) | |
Other | 305 (3.8) | 3 (3.8) | |
Marital status, n (%) | 0.37 | ||
Currently married or partner* | 4107 (51.35) | 46 (57.5) | |
Single/never married | 1967 (24.7) | 14 (17.5) | |
Separated/divorced/widowed/no answer | 1900 (23.8) | 20 (25.0) | |
Source of index admission, n (%) | 0.10 | ||
Direct from home/outpatient clinic | 2456 (30.8) | 33 (41.3) | |
Emergency department* | 4222 (53.0) | 34 (42.5) | |
Nursing home/rehabilitation/other hospital | 1296 (16.3) | 13 (16.3) | |
Length of stay of the index admission, median (IQR) | 4 (27) | 5.5 (38] | 0.13 |
No. of hospital admissions in the past year, median (IQR) | 1 (02) | 2 (03) | <0.001 |
Any procedure during the hospital stay, n (%) | 4809 (60.3) | 57 (71.3) | 0.05 |
Identified caregiver at discharge | 7300 (91.6) | 76 (95.0) | 0.27 |
No. of medications at discharge, mean (SD) | 10.6 (5.1) | 13.0 (5.0) | <0.001 |
No. of opiate medication at discharge | <0.001 | ||
0 | 5297 (66.4) | 21 (26.3) | |
1 | 2677 (33.2) | 59 (73.8) | |
Elixhauser, median (IQR) | 8 (215) | 23 (1442) | <0.001 |
Selected comorbidities, n (%) | |||
Diabetes mellitus | 1971 (24.7) | 20 (25.0) | 0.96 |
Heart failure | 1756 (22.0) | 11 (13.8) | 0.10 |
Atrial fibrillation | 1439 (18.1) | 10 (12.5) | 0.20 |
COPD | 816 (10.2) | 7 (8.8) | 0.66 |
Neoplasm | 2705 (33.9) | 69 (86.3) | <0.001 |
Stroke | 294 (3.7) | 2 (2.5) | 0.57 |
ESRD | 1258 (15.8) | 6 (7.5) | 0.04 |
Liver disease | 328 (4.1) | 2 (2.5) | 0.47 |
Statistical Analysis
We first conducted a bivariate analysis on all collected potential risk factors, comparing admissions followed by a 30‐day PAR due to end‐of‐life care issues with admissions not followed by any 30‐day readmission, using the Pearson [2] test for categorical variables and Student t test for continuous variables. Then, we performed a multivariable logistic regression restricted to the variables that were found significantly associated with the outcome in the bivariate analysis. Age and Elixhauser comorbidity index were forced into the model as important potential confounders. Because a patient could have several outcomes over the study period, we used general estimating equations to cluster at the patient level. All tests were conducted as 2‐sided at a 0.05 level of significance. Analyses were performed using the SAS system for Windows, version 9.3 (SAS Institute Inc., Cary, NC).
RESULTS
From the total of 12,383 patients who were discharged from the medical services of the Brigham and Women's Hospital during the study period, 2108 (17.0%) were excluded because of: (1) death before discharge, (2) transfer to another acute care hospital, (3) discharge against medical advice, or (4) missing data (Figure 1). Among the 10,275 eligible admissions, 22.3% (n=2301) were followed by a 30‐day readmission. Of these, 826 (8.0% of all admissions) were identified as potentially avoidable. Among a random sample of 534 PARs, 80 (15.0%) were related to end‐of‐life care issues (cases). Of note, only 16 (20%) of these patients received palliative care consultation during the index hospitalization. A total of 7974 discharges were not followed by any 30‐day readmission (controls).
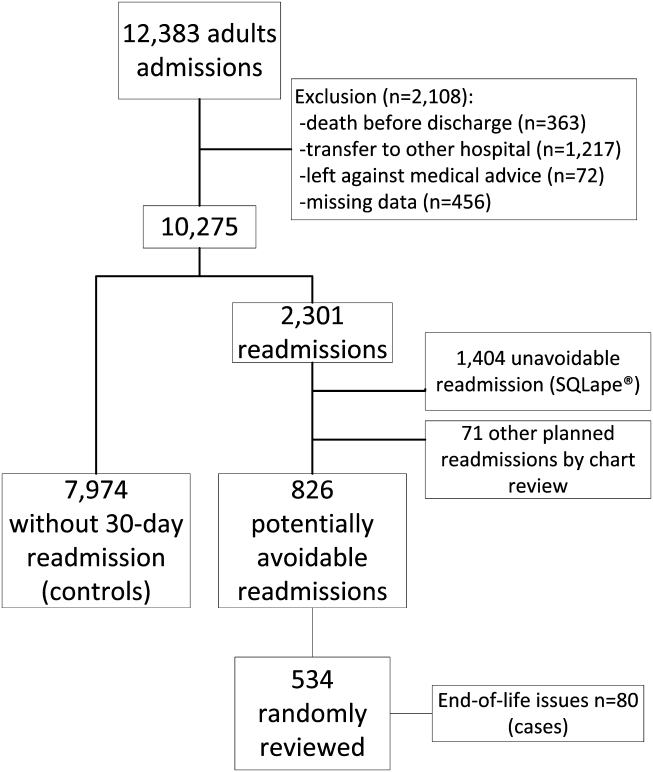
Baseline characteristics are presented in Table 1. Among the combined cohort of cases plus controls, the patient's mean age at inclusion was 61.3 years, and about half were male. In bivariate analysis, demographics such as age and sex were similar between cases and controls. Cases had more hospitalizations in the previous year, a higher number of medications at discharge, and a higher Elixhauser comorbidity index. When looking at diseases more specifically, neoplasm was significantly associated with potentially avoidable 30‐day readmission due to end‐of‐life care issues. In contrast, end‐stage renal disease was associated with a significantly lower risk of 30‐day PAR due to end‐of‐life care issues.
In multivariate analysis, 4 factors remained significantly associated with 30‐day PAR due to end‐of‐life care issues (Table 2). Neoplasm was the strongest risk factor, with an odds ratio of 5.6 (95% confidence interval: 2.8511.0), followed by opiate medication use, Elixhauser score, and number of admissions in the previous 12 months.
Variable | Odds Ratio (95% CI) |
---|---|
| |
Age, per 10 years | 1.04 (0.911.19) |
No. of admissions in the previous 12 months, per admission | 1.10 (1.021.20)a |
Total no. of medications at discharge, per medication | 1.04 (1.001.10) |
Neoplasm | 5.60 (2.8511.0)a |
Endstage renal disease | 0.60 (0.251.42) |
Opiate medication at discharge | 2.29 (1.294.07)a |
Elixhauser, per 5 unit increase | 1.16 (1.101.22)a |
The model, including all 4 variables, had an excellent discrimination power, with a C statistic of 0.85. Without the Elixhauser score, the C statistic remained very high, with a value of 0.82.
DISCUSSION
In a large medical population, potentially avoidable readmissions due to end‐of‐life care issues were not uncommon: 15% of all potentially avoidable readmissions (1.2% of all discharges). We identified 4 main risk factors for having a 30‐day potentially avoidable readmission due to end‐of‐life care issues: neoplasm, opiate use, Elixhauser comorbidity index, and number of admissions in the previous year. In a model that includes these 4 variables, the discrimination was very high with a C statistic of 0.85.
This study extends prior work indicating some risk factors for the need for palliative care. Neoplasm has been logically identified as a criterion for palliative care assessment at the time of admission.[13] Patients with neoplasm are not only at overall high risk for readmission,[14, 15, 16] but they obviously represent a fragile population whose condition is often terminal. Our results suggest that still more attention may be necessary to reduce the risk of readmission due to end‐of‐life care issues in this population (for example, only 20% of cases in our study received palliative care consultation during the index hospitalization). The overall comorbidity measured by the Elixhauser index was not surprisingly a significant risk factor. It probably accounts for the burden of comorbidities, but also for other advanced diseases besides neoplasm, like heart failure, chronic obstructive pulmonary disease, and others that may also be terminal. The number of previous hospital admissions in the past year is also an important risk factor, not only for the general population,[10, 11, 14, 17, 18] but also for patients with more advanced conditions,[19, 20, 21, 22] where admissions become more frequent as the disease progresses toward end stage. Opioid use was the final statistically significant risk factor, specific for this population, likely as a proxy for disease severity and progression toward terminal illness, especially in combination with the other risk factors such as cancer. Age was not a significant factor in either bivariate or multivariate analysis. Previous studies on the risk factors for readmission among patients receiving palliative care also failed to show age as a significant factor.[23, 24] Both of these studies looked at readmissions among patients who were already receiving palliative care. Our study asks a fundamentally different (and in many ways a more practical) question: who among a large population of medical patients might benefit from receiving input from palliative care in the first place. The number of medications at discharge was no longer significant in the multivariate analysis, likely due to its collinearity with the Elixhauser comorbidity index. An increased number of medications might be associated with a higher risk of adverse drug events and readmission, but they would not be necessarily considered to be end‐of‐life readmissions. Taken together, the 4 variables provide a very promising prediction model with high discrimination. To our knowledge, there is no previous existing list of risk factors for 30‐day potentially avoidable readmission due to end‐of‐life care issues, and no existing model to help prioritize palliative care to the most high‐risk patients. It is worth noting that the Elixhauser score might be difficult to calculate before the discharge of the patient (although hospitals with electronic capture of medical problem lists might be able to approximate it). However, even without the Elixhauser score, the C statistic remained very high at 0.82.
Our study has several limitations. Although we looked at readmissions at 2 other affiliated hospitals, some patients might have been readmitted to other acute care facilities outside our network. However, we would not expect the risk factors in these patients to be so different. The identification of end‐of‐life care issues by medical record review is based on a subjective judgment, although strict criteria were used. Furthermore, differentiation between potentially avoidable readmission and unavoidable readmission cannot be perfect. We used clear and logical criteria that were previously validated and allow large database management. Also, we did not analyze a comprehensive list of potential risk factors. It is probable that functional or cognitive status, for example, could also be important risk factors. We purposely chose a set of variables that could be easily obtained from administrative data sources. The small number of cases may have led to limited statistical power to identify less strongly associated risk factors. Last, the results may not be completely generalizable to small or community hospitals, in particular those that may care for less severely ill cancer patients.
Our findings have important implications. End‐of‐life care issues are not infrequent causes of readmission. Our study's findings could help prioritize palliative care resources to those patients at higher risk to improve the quality of end‐of‐life care. The risk factors identified in this study could be used informally by physicians at the bedside to identify such patients. In addition, a hospital could use these factors to provide a second‐level screen, beyond clinician recognition, to assist palliative care teams to identify patients who may not have otherwise been referred. This screen could be automated, for example, by using a list of medical problems from an electronic medical record to approximate an Elixhauser comorbidity score, or even leaving comorbidities out and simply relying on the other 3 easily identifiable risk factors. Such efforts could have a substantial effect on improving care near the end of life and potentially reducing unnecessary hospitalizations.
Acknowledgements
The authors thank Yves Eggli for having screened the database for potentially avoidable readmission using the algorithm SQLape.
Disclosures: Dr. Donz was supported by the Swiss National Science Foundation and the Swiss Foundation for MedicalBiological Scholarships. The Swiss Science National Foundation and the Swiss Foundation for MedicalBiological Scholarships had no role in the design and conduct of this study, the analysis or interpretation of the data, or the preparation of this manuscript. Dr. Schnipper is a consultant to QuantiaMD, for which he has helped create online educational materials for both providers and patients regarding patient safety, including medication safety during transitions in care. The findings of this study are not a part of those materials. Dr. Schnipper has received grant funding from Sanofi‐Aventis for an investigator‐initiated study to design and evaluate an intensive discharge and follow‐up intervention in patients with diabetes. The funder had had no role in the design of the study.
The need to improve end‐of‐life care is well recognized. Its quality is often poor, and its cost is enormous, with 30% of the Medicare expenditures used for medical treatments of the 6% of beneficiaries who die each year.[1, 2] Repeated hospitalizations are frequent toward the end of life,[3] where each admission should be viewed as an opportunity to initiate advance care planning to improve end‐of‐life care and possibly reduce future unnecessary readmissions.[4, 5] Identified problems include undertreatment of pain, lack of awareness of patient wishes or advance directives, and unwanted overtreatment.
To improve quality and reduce unnecessary hospital use near the end of life, there is an urgent need to help healthcare providers to better identify the most vulnerable and at‐risk patients to provide them with care coordination and supportive care services. We aimed to identify the risk factors for having a 30‐day potentially avoidable readmission (PAR) due to end‐of‐life care issues.
METHODS
Study Design and Population
A nested case‐control study was designed where potentially avoidable end‐of‐life readmissions were compared to nonreadmitted controls. We collected data on all consecutive adult patient admissions to any medical services of the Brigham and Women's Hospital with a discharge date between July 1, 2009 and June 30, 2010. Brigham and Women's Hospital is a 780‐bed academic medical center in Boston, Massachusetts. To avoid observation stays, only admissions with a length of stay of more than 1 day were included. We excluded patients who died before discharge, were transferred to another acute care hospital, and those who left against medical advice. We also excluded patients with no available data on medication treatment at discharge. The protocol was approved by the institutional review board of Brigham and Women's Hospital/Partners Healthcare.
Study Outcome
The study outcome was any 30‐day PAR due to end‐of‐life issues. To determine this outcome, first we identified all 30‐day readmissions to any service of 3 hospitals within the Partners network in Boston that followed the index hospitalization (prior studies have shown that these hospitals capture approximately 80% of readmissions after a Brigham and Women's Hospital medical hospitalization).[6, 7] These readmissions were subsequently differentiated as potentially avoidable or not using a validated algorithm (SQLape; SQLape, Corseaux, Switzerland).[8, 9] This algorithm uses administrative data and International Classification of Diseases, 9th Revision, Clinical Modification codes from the index and repeat hospitalization. Readmissions were considered potentially avoidable if they were: (1) readmissions related to previously known conditions during the index hospitalization, or (2) complications of treatment (eg, deep vein thrombosis, drug‐induced disorders). Conversely, readmissions were considered unavoidable if they were: (1) foreseen (such as readmissions for transplantation, delivery, chemo‐ or radiotherapy, and other specific surgical procedures), (2) follow‐up and rehabilitation treatments, or (3) readmissions for a new condition unknown during the preceding hospitalization. The algorithm has both a sensitivity and specificity of 96% compared with medical record review using the same criteria. Finally, a random sample of the 30‐day PARs was reviewed independently by 9 trained senior resident physicians to identify those due to end‐of‐life issues, defined by the following 2 criteria: (1) patient has a terminal clinical condition, such as malignancy, end stage renal disease, end stage congestive heart failure, or other condition with a life expectancy of 6 months or less; and (2) the readmission is part of the terminal disease process that was not adequately addressed during the index hospitalization. Examples of factors that were used when identifying cases included lack of healthcare proxy and lack of documentation of why end‐of‐life discussions did not take place during the index hospitalization. Training of adjudicators included a didactic session and review of standardized cases.
Risk Factors
We collected candidate risk factors based on a priori knowledge and according to the medical literature,[10, 11, 12] including demographic information, previous healthcare utilization, and index hospitalization characteristics from administrative data sources; procedures and chronic medical conditions from billing data; last laboratory values and medication information prior to discharge from the electronic medical record (Table 1). When laboratory values were missing (<1%), values were considered as normal.
Characteristics | No 30‐Day Readmission, n=7,974 | 30‐Day PAR due to End of Life, n=80 | P Value |
---|---|---|---|
| |||
Age, y, mean (SD) | 61.5 (16.6) | 60.8 (11.9) | 0.69 |
Male sex, n (%) | 3875 (48.6) | 37 (46.3) | 0.69 |
Ethnicity, n (%) | 0.05 | ||
Non‐Hispanic white* | 5772 (72.4) | 69 (86.3) | |
Non‐Hispanic black | 1281 (16.1) | 4 (5.0) | |
Hispanic | 666 (8.4) | 5 (6.3) | |
Other | 255 (3.2) | 2 (2.5) | |
Language, n (%) | 0.99 | ||
English* | 7254 (91.0) | 73 (91.3) | |
Spanish | 415 (5.2) | 4 (5.0) | |
Other | 305 (3.8) | 3 (3.8) | |
Marital status, n (%) | 0.37 | ||
Currently married or partner* | 4107 (51.35) | 46 (57.5) | |
Single/never married | 1967 (24.7) | 14 (17.5) | |
Separated/divorced/widowed/no answer | 1900 (23.8) | 20 (25.0) | |
Source of index admission, n (%) | 0.10 | ||
Direct from home/outpatient clinic | 2456 (30.8) | 33 (41.3) | |
Emergency department* | 4222 (53.0) | 34 (42.5) | |
Nursing home/rehabilitation/other hospital | 1296 (16.3) | 13 (16.3) | |
Length of stay of the index admission, median (IQR) | 4 (27) | 5.5 (38] | 0.13 |
No. of hospital admissions in the past year, median (IQR) | 1 (02) | 2 (03) | <0.001 |
Any procedure during the hospital stay, n (%) | 4809 (60.3) | 57 (71.3) | 0.05 |
Identified caregiver at discharge | 7300 (91.6) | 76 (95.0) | 0.27 |
No. of medications at discharge, mean (SD) | 10.6 (5.1) | 13.0 (5.0) | <0.001 |
No. of opiate medication at discharge | <0.001 | ||
0 | 5297 (66.4) | 21 (26.3) | |
1 | 2677 (33.2) | 59 (73.8) | |
Elixhauser, median (IQR) | 8 (215) | 23 (1442) | <0.001 |
Selected comorbidities, n (%) | |||
Diabetes mellitus | 1971 (24.7) | 20 (25.0) | 0.96 |
Heart failure | 1756 (22.0) | 11 (13.8) | 0.10 |
Atrial fibrillation | 1439 (18.1) | 10 (12.5) | 0.20 |
COPD | 816 (10.2) | 7 (8.8) | 0.66 |
Neoplasm | 2705 (33.9) | 69 (86.3) | <0.001 |
Stroke | 294 (3.7) | 2 (2.5) | 0.57 |
ESRD | 1258 (15.8) | 6 (7.5) | 0.04 |
Liver disease | 328 (4.1) | 2 (2.5) | 0.47 |
Statistical Analysis
We first conducted a bivariate analysis on all collected potential risk factors, comparing admissions followed by a 30‐day PAR due to end‐of‐life care issues with admissions not followed by any 30‐day readmission, using the Pearson [2] test for categorical variables and Student t test for continuous variables. Then, we performed a multivariable logistic regression restricted to the variables that were found significantly associated with the outcome in the bivariate analysis. Age and Elixhauser comorbidity index were forced into the model as important potential confounders. Because a patient could have several outcomes over the study period, we used general estimating equations to cluster at the patient level. All tests were conducted as 2‐sided at a 0.05 level of significance. Analyses were performed using the SAS system for Windows, version 9.3 (SAS Institute Inc., Cary, NC).
RESULTS
From the total of 12,383 patients who were discharged from the medical services of the Brigham and Women's Hospital during the study period, 2108 (17.0%) were excluded because of: (1) death before discharge, (2) transfer to another acute care hospital, (3) discharge against medical advice, or (4) missing data (Figure 1). Among the 10,275 eligible admissions, 22.3% (n=2301) were followed by a 30‐day readmission. Of these, 826 (8.0% of all admissions) were identified as potentially avoidable. Among a random sample of 534 PARs, 80 (15.0%) were related to end‐of‐life care issues (cases). Of note, only 16 (20%) of these patients received palliative care consultation during the index hospitalization. A total of 7974 discharges were not followed by any 30‐day readmission (controls).
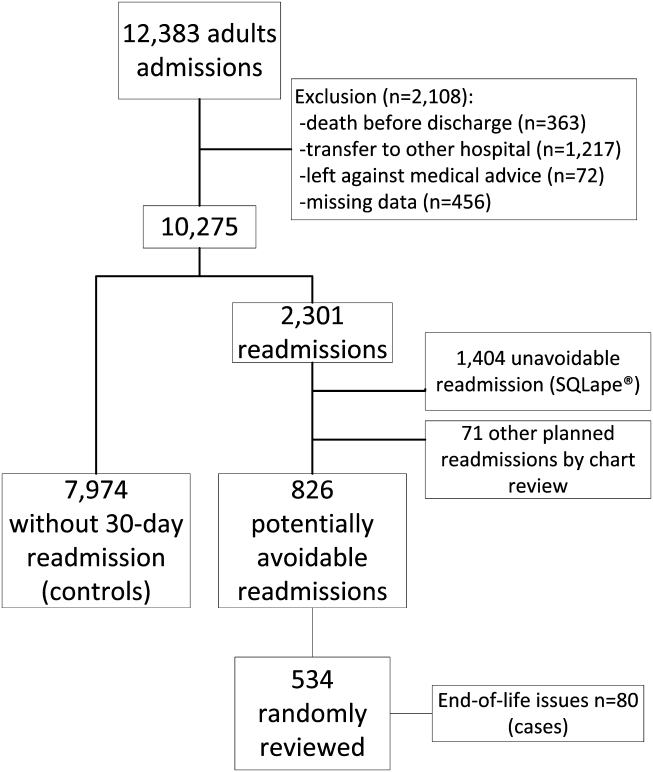
Baseline characteristics are presented in Table 1. Among the combined cohort of cases plus controls, the patient's mean age at inclusion was 61.3 years, and about half were male. In bivariate analysis, demographics such as age and sex were similar between cases and controls. Cases had more hospitalizations in the previous year, a higher number of medications at discharge, and a higher Elixhauser comorbidity index. When looking at diseases more specifically, neoplasm was significantly associated with potentially avoidable 30‐day readmission due to end‐of‐life care issues. In contrast, end‐stage renal disease was associated with a significantly lower risk of 30‐day PAR due to end‐of‐life care issues.
In multivariate analysis, 4 factors remained significantly associated with 30‐day PAR due to end‐of‐life care issues (Table 2). Neoplasm was the strongest risk factor, with an odds ratio of 5.6 (95% confidence interval: 2.8511.0), followed by opiate medication use, Elixhauser score, and number of admissions in the previous 12 months.
Variable | Odds Ratio (95% CI) |
---|---|
| |
Age, per 10 years | 1.04 (0.911.19) |
No. of admissions in the previous 12 months, per admission | 1.10 (1.021.20)a |
Total no. of medications at discharge, per medication | 1.04 (1.001.10) |
Neoplasm | 5.60 (2.8511.0)a |
Endstage renal disease | 0.60 (0.251.42) |
Opiate medication at discharge | 2.29 (1.294.07)a |
Elixhauser, per 5 unit increase | 1.16 (1.101.22)a |
The model, including all 4 variables, had an excellent discrimination power, with a C statistic of 0.85. Without the Elixhauser score, the C statistic remained very high, with a value of 0.82.
DISCUSSION
In a large medical population, potentially avoidable readmissions due to end‐of‐life care issues were not uncommon: 15% of all potentially avoidable readmissions (1.2% of all discharges). We identified 4 main risk factors for having a 30‐day potentially avoidable readmission due to end‐of‐life care issues: neoplasm, opiate use, Elixhauser comorbidity index, and number of admissions in the previous year. In a model that includes these 4 variables, the discrimination was very high with a C statistic of 0.85.
This study extends prior work indicating some risk factors for the need for palliative care. Neoplasm has been logically identified as a criterion for palliative care assessment at the time of admission.[13] Patients with neoplasm are not only at overall high risk for readmission,[14, 15, 16] but they obviously represent a fragile population whose condition is often terminal. Our results suggest that still more attention may be necessary to reduce the risk of readmission due to end‐of‐life care issues in this population (for example, only 20% of cases in our study received palliative care consultation during the index hospitalization). The overall comorbidity measured by the Elixhauser index was not surprisingly a significant risk factor. It probably accounts for the burden of comorbidities, but also for other advanced diseases besides neoplasm, like heart failure, chronic obstructive pulmonary disease, and others that may also be terminal. The number of previous hospital admissions in the past year is also an important risk factor, not only for the general population,[10, 11, 14, 17, 18] but also for patients with more advanced conditions,[19, 20, 21, 22] where admissions become more frequent as the disease progresses toward end stage. Opioid use was the final statistically significant risk factor, specific for this population, likely as a proxy for disease severity and progression toward terminal illness, especially in combination with the other risk factors such as cancer. Age was not a significant factor in either bivariate or multivariate analysis. Previous studies on the risk factors for readmission among patients receiving palliative care also failed to show age as a significant factor.[23, 24] Both of these studies looked at readmissions among patients who were already receiving palliative care. Our study asks a fundamentally different (and in many ways a more practical) question: who among a large population of medical patients might benefit from receiving input from palliative care in the first place. The number of medications at discharge was no longer significant in the multivariate analysis, likely due to its collinearity with the Elixhauser comorbidity index. An increased number of medications might be associated with a higher risk of adverse drug events and readmission, but they would not be necessarily considered to be end‐of‐life readmissions. Taken together, the 4 variables provide a very promising prediction model with high discrimination. To our knowledge, there is no previous existing list of risk factors for 30‐day potentially avoidable readmission due to end‐of‐life care issues, and no existing model to help prioritize palliative care to the most high‐risk patients. It is worth noting that the Elixhauser score might be difficult to calculate before the discharge of the patient (although hospitals with electronic capture of medical problem lists might be able to approximate it). However, even without the Elixhauser score, the C statistic remained very high at 0.82.
Our study has several limitations. Although we looked at readmissions at 2 other affiliated hospitals, some patients might have been readmitted to other acute care facilities outside our network. However, we would not expect the risk factors in these patients to be so different. The identification of end‐of‐life care issues by medical record review is based on a subjective judgment, although strict criteria were used. Furthermore, differentiation between potentially avoidable readmission and unavoidable readmission cannot be perfect. We used clear and logical criteria that were previously validated and allow large database management. Also, we did not analyze a comprehensive list of potential risk factors. It is probable that functional or cognitive status, for example, could also be important risk factors. We purposely chose a set of variables that could be easily obtained from administrative data sources. The small number of cases may have led to limited statistical power to identify less strongly associated risk factors. Last, the results may not be completely generalizable to small or community hospitals, in particular those that may care for less severely ill cancer patients.
Our findings have important implications. End‐of‐life care issues are not infrequent causes of readmission. Our study's findings could help prioritize palliative care resources to those patients at higher risk to improve the quality of end‐of‐life care. The risk factors identified in this study could be used informally by physicians at the bedside to identify such patients. In addition, a hospital could use these factors to provide a second‐level screen, beyond clinician recognition, to assist palliative care teams to identify patients who may not have otherwise been referred. This screen could be automated, for example, by using a list of medical problems from an electronic medical record to approximate an Elixhauser comorbidity score, or even leaving comorbidities out and simply relying on the other 3 easily identifiable risk factors. Such efforts could have a substantial effect on improving care near the end of life and potentially reducing unnecessary hospitalizations.
Acknowledgements
The authors thank Yves Eggli for having screened the database for potentially avoidable readmission using the algorithm SQLape.
Disclosures: Dr. Donz was supported by the Swiss National Science Foundation and the Swiss Foundation for MedicalBiological Scholarships. The Swiss Science National Foundation and the Swiss Foundation for MedicalBiological Scholarships had no role in the design and conduct of this study, the analysis or interpretation of the data, or the preparation of this manuscript. Dr. Schnipper is a consultant to QuantiaMD, for which he has helped create online educational materials for both providers and patients regarding patient safety, including medication safety during transitions in care. The findings of this study are not a part of those materials. Dr. Schnipper has received grant funding from Sanofi‐Aventis for an investigator‐initiated study to design and evaluate an intensive discharge and follow‐up intervention in patients with diabetes. The funder had had no role in the design of the study.
- Medicare beneficiaries' costs of care in the last year of life. Health Aff (Millwood). 2001;20(4):188–195. , , , .
- Quality of End‐of‐Life Cancer Care for Medicare Beneficiaries: Regional and Hospital‐Specific Analyses. Lebanon, NH: The Dartmouth Institute for Health Policy and Clinical Practice; 2010. , , .
- Repeated hospitalizations predict mortality in the community population with heart failure. Am Heart J. 2007;154(2):260–266. , , .
- Perspectives on care at the close of life. Initiating end‐of‐life discussions with seriously ill patients: addressing the “elephant in the room.” JAMA. 2000;284(19):2502–2507. .
- Advance care planning as a process: structuring the discussions in practice. J Am Geriatr Soc. 1995;43(4):440–446. , , , .
- Rationale and design of the Pharmacist Intervention for Low Literacy in Cardiovascular Disease (PILL‐CVD) study. Circ Cardiovasc Qual Outcomes. 2010;3(2):212–219. , , , et al.
- Effect of a pharmacist intervention on clinically important medication errors after hospital discharge: a randomized trial. Ann Intern Med. 2012;157(1):1–10. , , , et al.
- Measuring potentially avoidable hospital readmissions. J Clin Epidemiol. 2002;55(6):573–587. , , , , , .
- Validation of the potentially avoidable hospital readmission rate as a routine indicator of the quality of hospital care. Med Care. 2006;44(11):972–981. , , , , , .
- Hospital readmission in general medicine patients: a prediction model. J Gen Intern Med. 2010;25(3):211–219. , , , et al.
- Screening elders for risk of hospital admission. J Am Geriatr Soc. 1993;41(8):811–817. , , , , , .
- Risk factors for 30‐day hospital readmission in patients ≥65 years of age. Proc (Bayl Univ Med Cent). 2008;21(4):363–372. , , , , .
- Identifying patients in need of a palliative care assessment in the hospital setting: a consensus report from the Center to Advance Palliative Care. J Palliat Med. 2011;14(1):17–23. , .
- Potentially avoidable 30‐day hospital readmissions in medical patients: derivation and validation of a prediction model. JAMA Intern Med. 2013;173(8):632–638. , , , .
- Redefining readmission risk factors for general medicine patients. J Hosp Med. 2011;6(2):54–60. , , , .
- Patient and disease profile of emergency medical readmissions to an Irish teaching hospital. Postgrad Med J. 2004;80(946):470–474. , , .
- Posthospital care transitions: patterns, complications, and risk identification. Health Serv Res. 2004;39(5):1449–1465. , , , .
- Factors predicting readmission of older general medicine patients. J Gen Intern Med. 1991;6(5):389–393. , .
- Differences in health care utilization at the end of life among patients with chronic obstructive pulmonary disease and patients with lung cancer. Arch Intern Med. 2006;166(3):326–331. , , , , .
- Frequent hospital readmissions for acute exacerbation of COPD and their associated factors. Respirology. 2006;11(2):188–195. , , , , .
- Consensus statement: Palliative and supportive care in advanced heart failure. J Card Fail. 2004;10(3):200–209. , , , et al.
- Unplanned discharges from a surgical intensive care unit: readmissions and mortality. J Crit Care. 2010;25(3):375–381. , , , , .
- Evaluating causes for unplanned hospital readmissions of palliative care patients. Am J Hosp Palliat Care. 2010;27(8):526–531. , , , , .
- 30‐day readmissions among seriously ill older adults. J Palliat Med. 2012;15(12):1356–1361. , , .
- Medicare beneficiaries' costs of care in the last year of life. Health Aff (Millwood). 2001;20(4):188–195. , , , .
- Quality of End‐of‐Life Cancer Care for Medicare Beneficiaries: Regional and Hospital‐Specific Analyses. Lebanon, NH: The Dartmouth Institute for Health Policy and Clinical Practice; 2010. , , .
- Repeated hospitalizations predict mortality in the community population with heart failure. Am Heart J. 2007;154(2):260–266. , , .
- Perspectives on care at the close of life. Initiating end‐of‐life discussions with seriously ill patients: addressing the “elephant in the room.” JAMA. 2000;284(19):2502–2507. .
- Advance care planning as a process: structuring the discussions in practice. J Am Geriatr Soc. 1995;43(4):440–446. , , , .
- Rationale and design of the Pharmacist Intervention for Low Literacy in Cardiovascular Disease (PILL‐CVD) study. Circ Cardiovasc Qual Outcomes. 2010;3(2):212–219. , , , et al.
- Effect of a pharmacist intervention on clinically important medication errors after hospital discharge: a randomized trial. Ann Intern Med. 2012;157(1):1–10. , , , et al.
- Measuring potentially avoidable hospital readmissions. J Clin Epidemiol. 2002;55(6):573–587. , , , , , .
- Validation of the potentially avoidable hospital readmission rate as a routine indicator of the quality of hospital care. Med Care. 2006;44(11):972–981. , , , , , .
- Hospital readmission in general medicine patients: a prediction model. J Gen Intern Med. 2010;25(3):211–219. , , , et al.
- Screening elders for risk of hospital admission. J Am Geriatr Soc. 1993;41(8):811–817. , , , , , .
- Risk factors for 30‐day hospital readmission in patients ≥65 years of age. Proc (Bayl Univ Med Cent). 2008;21(4):363–372. , , , , .
- Identifying patients in need of a palliative care assessment in the hospital setting: a consensus report from the Center to Advance Palliative Care. J Palliat Med. 2011;14(1):17–23. , .
- Potentially avoidable 30‐day hospital readmissions in medical patients: derivation and validation of a prediction model. JAMA Intern Med. 2013;173(8):632–638. , , , .
- Redefining readmission risk factors for general medicine patients. J Hosp Med. 2011;6(2):54–60. , , , .
- Patient and disease profile of emergency medical readmissions to an Irish teaching hospital. Postgrad Med J. 2004;80(946):470–474. , , .
- Posthospital care transitions: patterns, complications, and risk identification. Health Serv Res. 2004;39(5):1449–1465. , , , .
- Factors predicting readmission of older general medicine patients. J Gen Intern Med. 1991;6(5):389–393. , .
- Differences in health care utilization at the end of life among patients with chronic obstructive pulmonary disease and patients with lung cancer. Arch Intern Med. 2006;166(3):326–331. , , , , .
- Frequent hospital readmissions for acute exacerbation of COPD and their associated factors. Respirology. 2006;11(2):188–195. , , , , .
- Consensus statement: Palliative and supportive care in advanced heart failure. J Card Fail. 2004;10(3):200–209. , , , et al.
- Unplanned discharges from a surgical intensive care unit: readmissions and mortality. J Crit Care. 2010;25(3):375–381. , , , , .
- Evaluating causes for unplanned hospital readmissions of palliative care patients. Am J Hosp Palliat Care. 2010;27(8):526–531. , , , , .
- 30‐day readmissions among seriously ill older adults. J Palliat Med. 2012;15(12):1356–1361. , , .
© 2014 Society of Hospital Medicine