User login
Chronic obstructive pulmonary disease (COPD) affects as many as 24 million individuals in the United States, is responsible for more than 700,000 annual hospital admissions, and is currently the nation's third leading cause of death, accounting for nearly $49.9 billion in medical spending in 2010.[1, 2] Reported in‐hospital mortality rates for patients hospitalized for exacerbations of COPD range from 2% to 5%.[3, 4, 5, 6, 7] Information about 30‐day mortality rates following hospitalization for COPD is more limited; however, international studies suggest that rates range from 3% to 9%,[8, 9] and 90‐day mortality rates exceed 15%.[10]
Despite this significant clinical and economic impact, there have been no large‐scale, sustained efforts to measure the quality or outcomes of hospital care for patients with COPD in the United States. What little is known about the treatment of patients with COPD suggests widespread opportunities to increase adherence to guideline‐recommended therapies, to reduce the use of ineffective treatments and tests, and to address variation in care across institutions.[5, 11, 12]
Public reporting of hospital performance is a key strategy for improving the quality and safety of hospital care, both in the United States and internationally.[13] Since 2007, the Centers for Medicare and Medicaid Services (CMS) has reported hospital mortality rates on the Hospital Compare Web site, and COPD is 1 of the conditions highlighted in the Affordable Care Act for future consideration.[14] Such initiatives rely on validated, risk‐adjusted performance measures for comparisons across institutions and to enable outcomes to be tracked over time. We present the development, validation, and results of a model intended for public reporting of risk‐standardized mortality rates for patients hospitalized with exacerbations of COPD that has been endorsed by the National Quality Forum.[15]
METHODS
Approach to Measure Development
We developed this measure in accordance with guidelines described by the National Quality Forum,[16] CMS' Measure Management System,[17] and the American Heart Association scientific statement, Standards for Statistical Models Used for Public Reporting of Health Outcomes.[18] Throughout the process we obtained expert clinical and stakeholder input through meetings with a clinical advisory group and a national technical expert panel (see Acknowledgments). Last, we presented the proposed measure specifications and a summary of the technical expert panel discussions online and made a widely distributed call for public comments. We took the comments into consideration during the final stages of measure development (available at
Data Sources
We used claims data from Medicare inpatient, outpatient, and carrier (physician) Standard Analytic Files from 2008 to develop and validate the model, and examined model reliability using data from 2007 and 2009. The Medicare enrollment database was used to determine Medicare Fee‐for‐Service enrollment and mortality.
Study Cohort
Admissions were considered eligible for inclusion if the patient was 65 years or older, was admitted to a nonfederal acute care hospital in the United States, and had a principal diagnosis of COPD or a principal diagnosis of acute respiratory failure or respiratory arrest when paired with a secondary diagnosis of COPD with exacerbation (Table 1).
ICD‐9‐CM | Description |
---|---|
| |
491.21 | Obstructive chronic bronchitis; with (acute) exacerbation; acute exacerbation of COPD, decompensated COPD, decompensated COPD with exacerbation |
491.22 | Obstructive chronic bronchitis; with acute bronchitis |
491.8 | Other chronic bronchitis; chronic: tracheitis, tracheobronchitis |
491.9 | Unspecified chronic bronchitis |
492.8 | Other emphysema; emphysema (lung or pulmonary): NOS, centriacinar, centrilobular, obstructive, panacinar, panlobular, unilateral, vesicular; MacLeod's syndrome; Swyer‐James syndrome; unilateral hyperlucent lung |
493.20 | Chronic obstructive asthma; asthma with COPD, chronic asthmatic bronchitis, unspecified |
493.21 | Chronic obstructive asthma; asthma with COPD, chronic asthmatic bronchitis, with status asthmaticus |
493.22 | Chronic obstructive asthma; asthma with COPD, chronic asthmatic bronchitis, with (acute) exacerbation |
496 | Chronic: nonspecific lung disease, obstructive lung disease, obstructive pulmonary disease (COPD) NOS. (Note: This code is not to be used with any code from categories 491493.) |
518.81a | Other diseases of lung; acute respiratory failure; respiratory failure NOS |
518.82a | Other diseases of lung; acute respiratory failure; other pulmonary insufficiency, acute respiratory distress |
518.84a | Other diseases of lung; acute respiratory failure; acute and chronic respiratory failure |
799.1a | Other ill‐defined and unknown causes of morbidity and mortality; respiratory arrest, cardiorespiratory failure |
If a patient was discharged and readmitted to a second hospital on the same or the next day, we combined the 2 acute care admissions into a single episode of care and assigned the mortality outcome to the first admitting hospital. We excluded admissions for patients who were enrolled in Medicare Hospice in the 12 months prior to or on the first day of the index hospitalization. An index admission was any eligible admission assessed in the measure for the outcome. We also excluded admissions for patients who were discharged against medical advice, those for whom vital status at 30 days was unknown or recorded inconsistently, and patients with unreliable data (eg, age >115 years). For patients with multiple hospitalizations during a single year, we randomly selected 1 admission per patient to avoid survival bias. Finally, to assure adequate risk adjustment we limited the analysis to patients who had continuous enrollment in Medicare Fee‐for‐Service Parts A and B for the 12 months prior to their index admission so that we could identify comorbid conditions coded during all prior encounters.
Outcomes
The outcome of 30‐day mortality was defined as death from any cause within 30 days of the admission date for the index hospitalization. Mortality was assessed at 30 days to standardize the period of outcome ascertainment,[19] and because 30 days is a clinically meaningful time frame, during which differences in the quality of hospital care may be revealed.
Risk‐Adjustment Variables
We randomly selected half of all COPD admissions in 2008 that met the inclusion and exclusion criteria to create a model development sample. Candidate variables for inclusion in the risk‐standardized model were selected by a clinician team from diagnostic groups included in the Hierarchical Condition Category clinical classification system[20] and included age and comorbid conditions. Sleep apnea (International Classification of Diseases, 9th Revision, Clinical Modification [ICD‐9‐CM] condition codes 327.20, 327.21, 327.23, 327.27, 327.29, 780.51, 780.53, and 780.57) and mechanical ventilation (ICD‐9‐CM procedure codes 93.90, 96.70, 96.71, and 96.72) were also included as candidate variables.
We defined a condition as present for a given patient if it was coded in the inpatient, outpatient, or physician claims data sources in the preceding 12 months, including the index admission. Because a subset of the condition category variables can represent a complication of care, we did not consider them to be risk factors if they appeared only as secondary diagnosis codes for the index admission and not in claims submitted during the prior year.
We selected final variables for inclusion in the risk‐standardized model based on clinical considerations and a modified approach to stepwise logistic regression. The final patient‐level risk‐adjustment model included 42 variables (Table 2).
Variable | Development Sample (150,035 Admissions at 4537 Hospitals) | Validation Sample (149,646 Admissions at 4535 Hospitals) | ||||
---|---|---|---|---|---|---|
Frequency, % | OR | 95% CI | Frequency, % | OR | 95% CI | |
| ||||||
Demographics | ||||||
Age 65 years (continuous) | 1.03 | 1.03‐1.04 | 1.03 | 1.03‐1.04 | ||
Cardiovascular/respiratory | ||||||
Sleep apnea (ICD‐9‐CM: 327.20, 327.21, 327.23, 327.27, 327.29, 780.51, 780.53, 780.57)a | 9.57 | 0.87 | 0.81‐0.94 | 9.72 | 0.84 | 0.78‐0.90 |
History of mechanical ventilation (ICD‐9‐CM: 93.90, 96.70, 96.71, 96.72)a | 6.00 | 1.19 | 1.11‐1.27 | 6.00 | 1.15 | 1.08‐1.24 |
Respirator dependence/respiratory failure (CC 7778)a | 1.15 | 0.89 | 0.77‐1.02 | 1.20 | 0.78 | 0.68‐0.91 |
Cardiorespiratory failure and shock (CC 79) | 26.35 | 1.60 | 1.53‐1.68 | 26.34 | 1.59 | 1.52‐1.66 |
Congestive heart failure (CC 80) | 41.50 | 1.34 | 1.28‐1.39 | 41.39 | 1.31 | 1.25‐1.36 |
Chronic atherosclerosis (CC 8384)a | 50.44 | 0.87 | 0.83‐0.90 | 50.12 | 0.91 | 0.87‐0.94 |
Arrhythmias (CC 9293) | 37.15 | 1.17 | 1.12‐1.22 | 37.06 | 1.15 | 1.10‐1.20 |
Vascular or circulatory disease (CC 104106) | 38.20 | 1.09 | 1.05‐1.14 | 38.09 | 1.02 | 0.98‐1.06 |
Fibrosis of lung and other chronic lung disorder (CC 109) | 16.96 | 1.08 | 1.03‐1.13 | 17.08 | 1.11 | 1.06‐1.17 |
Asthma (CC 110) | 17.05 | 0.67 | 0.63‐0.70 | 16.90 | 0.67 | 0.63‐0.70 |
Pneumonia (CC 111113) | 49.46 | 1.29 | 1.24‐1.35 | 49.41 | 1.27 | 1.22‐1.33 |
Pleural effusion/pneumothorax (CC 114) | 11.78 | 1.17 | 1.11‐1.23 | 11.54 | 1.18 | 1.12‐1.25 |
Other lung disorders (CC 115) | 53.07 | 0.80 | 0.77‐0.83 | 53.17 | 0.83 | 0.80‐0.87 |
Other comorbid conditions | ||||||
Metastatic cancer and acute leukemia (CC 7) | 2.76 | 2.34 | 2.14‐2.56 | 2.79 | 2.15 | 1.97‐2.35 |
Lung, upper digestive tract, and other severe cancers (CC 8)a | 5.98 | 1.80 | 1.68‐1.92 | 6.02 | 1.98 | 1.85‐2.11 |
Lymphatic, head and neck, brain, and other major cancers; breast, prostate, colorectal and other cancers and tumors; other respiratory and heart neoplasms (CC 911) | 14.13 | 1.03 | 0.97‐1.08 | 14.19 | 1.01 | 0.95‐1.06 |
Other digestive and urinary neoplasms (CC 12) | 6.91 | 0.91 | 0.84‐0.98 | 7.05 | 0.85 | 0.79‐0.92 |
Diabetes and DM complications (CC 1520, 119120) | 38.31 | 0.91 | 0.87‐0.94 | 38.29 | 0.91 | 0.87‐0.94 |
Protein‐calorie malnutrition (CC 21) | 7.40 | 2.18 | 2.07‐2.30 | 7.44 | 2.09 | 1.98‐2.20 |
Disorders of fluid/electrolyte/acid‐base (CC 2223) | 32.05 | 1.13 | 1.08‐1.18 | 32.16 | 1.24 | 1.19‐1.30 |
Other endocrine/metabolic/nutritional disorders (CC 24) | 67.99 | 0.75 | 0.72‐0.78 | 67.88 | 0.76 | 0.73‐0.79 |
Other gastrointestinal disorders (CC 36) | 56.21 | 0.81 | 0.78‐0.84 | 56.18 | 0.78 | 0.75‐0.81 |
Osteoarthritis of hip or knee (CC 40) | 9.32 | 0.74 | 0.69‐0.79 | 9.33 | 0.80 | 0.74‐0.85 |
Other musculoskeletal and connective tissue disorders (CC 43) | 64.14 | 0.83 | 0.80‐0.86 | 64.20 | 0.83 | 0.80‐0.87 |
Iron deficiency and other/unspecified anemias and blood disease (CC 47) | 40.80 | 1.08 | 1.04‐1.12 | 40.72 | 1.08 | 1.04‐1.13 |
Dementia and senility (CC 4950) | 17.06 | 1.09 | 1.04‐1.14 | 16.97 | 1.09 | 1.04‐1.15 |
Drug/alcohol abuse, without dependence (CC 53)a | 23.51 | 0.78 | 0.75‐0.82 | 23.38 | 0.76 | 0.72‐0.80 |
Other psychiatric disorders (CC 60)a | 16.49 | 1.12 | 1.07‐1.18 | 16.43 | 1.12 | 1.06‐1.17 |
Quadriplegia, paraplegia, functional disability (CC 6769, 100102, 177178) | 4.92 | 1.03 | 0.95‐1.12 | 4.92 | 1.08 | 0.99‐1.17 |
Mononeuropathy, other neurological conditions/emnjuries (CC 76) | 11.35 | 0.85 | 0.80‐0.91 | 11.28 | 0.88 | 0.83‐0.93 |
Hypertension and hypertensive disease (CC 9091) | 80.40 | 0.78 | 0.75‐0.82 | 80.35 | 0.79 | 0.75‐0.83 |
Stroke (CC 9596)a | 6.77 | 1.00 | 0.93‐1.08 | 6.73 | 0.98 | 0.91‐1.05 |
Retinal disorders, except detachment and vascular retinopathies (CC 121) | 10.79 | 0.87 | 0.82‐0.93 | 10.69 | 0.90 | 0.85‐0.96 |
Other eye disorders (CC 124)a | 19.05 | 0.90 | 0.86‐0.95 | 19.13 | 0.98 | 0.85‐0.93 |
Other ear, nose, throat, and mouth disorders (CC 127) | 35.21 | 0.83 | 0.80‐0.87 | 35.02 | 0.80 | 0.77‐0.83 |
Renal failure (CC 131)a | 17.92 | 1.12 | 1.07‐1.18 | 18.16 | 1.13 | 1.08‐1.19 |
Decubitus ulcer or chronic skin ulcer (CC 148149) | 7.42 | 1.27 | 1.19‐1.35 | 7.42 | 1.33 | 1.25‐1.42 |
Other dermatological disorders (CC 153) | 28.46 | 0.90 | 0.87‐0.94 | 28.32 | 0.89 | 0.86‐0.93 |
Trauma (CC 154156, 158161) | 9.04 | 1.09 | 1.03‐1.16 | 8.99 | 1.15 | 1.08‐1.22 |
Vertebral fractures (CC 157) | 5.01 | 1.33 | 1.24‐1.44 | 4.97 | 1.29 | 1.20‐1.39 |
Major complications of medical care and trauma (CC 164) | 5.47 | 0.81 | 0.75‐0.88 | 5.55 | 0.82 | 0.76‐0.89 |
Model Derivation
We used hierarchical logistic regression models to model the log‐odds of mortality as a function of patient‐level clinical characteristics and a random hospital‐level intercept. At the patient level, each model adjusts the log‐odds of mortality for age and the selected clinical covariates. The second level models the hospital‐specific intercepts as arising from a normal distribution. The hospital intercept represents the underlying risk of mortality, after accounting for patient risk. If there were no differences among hospitals, then after adjusting for patient risk, the hospital intercepts should be identical across all hospitals.
Estimation of Hospital Risk‐Standardized Mortality Rate
We calculated a risk‐standardized mortality rate, defined as the ratio of predicted to expected deaths (similar to observed‐to‐expected), multiplied by the national unadjusted mortality rate.[21] The expected number of deaths for each hospital was estimated by applying the estimated regression coefficients to the characteristics of each hospital's patients, adding the average of the hospital‐specific intercepts, transforming the data by using an inverse logit function, and summing the data from all patients in the hospital to obtain the count. The predicted number of deaths was calculated in the same way, substituting the hospital‐specific intercept for the average hospital‐specific intercept.
Model Performance, Validation, and Reliability Testing
We used the remaining admissions in 2008 as the model validation sample. We computed several summary statistics to assess the patient‐level model performance in both the development and validation samples,[22] including over‐fitting indices, predictive ability, area under the receiver operating characteristic (ROC) curve, distribution of residuals, and model 2. In addition, we assessed face validity through a survey of members of the technical expert panel. To assess reliability of the model across data years, we repeated the modeling process using qualifying COPD admissions in both 2007 and 2009. Finally, to assess generalizability we evaluated the model's performance in an all‐payer sample of data from patients admitted to California hospitals in 2006.
Analyses were conducted using SAS version 9.1.3 (SAS Institute Inc., Cary, NC). We estimated the hierarchical models using the GLIMMIX procedure in SAS.
The Human Investigation Committee at the Yale University School of Medicine/Yale New Haven Hospital approved an exemption (HIC#0903004927) for the authors to use CMS claims and enrollment data for research analyses and publication.
RESULTS
Model Derivation
After exclusions were applied, the development sample included 150,035 admissions in 2008 at 4537 US hospitals (Figure 1). Factors that were most strongly associated with the risk of mortality included metastatic cancer (odds ratio [OR] 2.34), protein calorie malnutrition (OR 2.18), nonmetastatic cancers of the lung and upper digestive tract, (OR 1.80) cardiorespiratory failure and shock (OR 1.60), and congestive heart failure (OR 1.34) (Table 2).
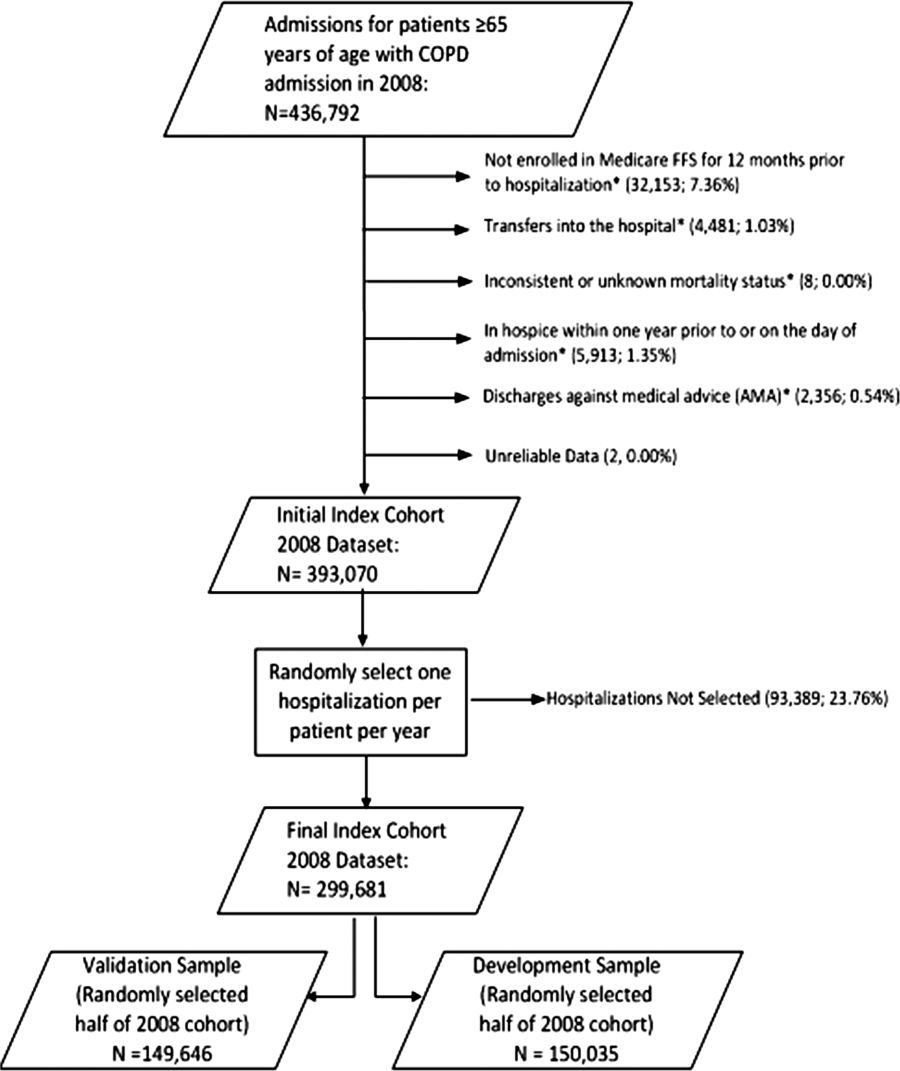
Model Performance, Validation, and Reliability
The model had a C statistic of 0.72, indicating good discrimination, and predicted mortality in the development sample ranged from 1.52% in the lowest decile to 23.74% in the highest. The model validation sample, using the remaining cases from 2008, included 149,646 admissions from 4535 hospitals. Variable frequencies and ORs were similar in both samples (Table 2). Model performance was also similar in the validation samples, with good model discrimination and fit (Table 3). Ten of 12 technical expert panel members responded to the survey, of whom 90% at least somewhat agreed with the statement, the COPD mortality measure provides an accurate reflection of quality. When the model was applied to patients age 18 years and older in the 2006 California Patient Discharge Data, overall discrimination was good (C statistic, 0.74), including in those age 18 to 64 years (C statistic, 0.75; 65 and above C statistic, 0.70).
Development | Validation | Data Years | ||
---|---|---|---|---|
Indices | Sample, 2008 | Sample, 2008 | 2007 | 2009 |
| ||||
Number of admissions | 150,035 | 149,646 | 259,911 | 279,377 |
Number of hospitals | 4537 | 4535 | 4636 | 4571 |
Mean risk‐standardized mortality rate, % (SD) | 8.62 (0.94) | 8.64 (1.07) | 8.97 (1.12) | 8.08 (1.09) |
Calibration, 0, 1 | 0.034, 0.985 | 0.009, 1.004 | 0.095, 1.022 | 0.120, 0.981 |
Discriminationpredictive ability, lowest decile %highest decile % | 1.5223.74 | 1.6023.78 | 1.5424.64 | 1.4222.36 |
Discriminationarea under the ROC curve, C statistic | 0.720 | 0.723 | 0.728 | 0.722 |
Residuals lack of fit, Pearson residual fall % | ||||
2 | 0 | 0 | 0 | 0 |
2, 0 | 91.14 | 91.4 | 91.08 | 91.93 |
0, 2 | 1.66 | 1.7 | 1.96 | 1.42 |
2+ | 6.93 | 6.91 | 6.96 | 6.65 |
Model Wald 2 (number of covariates) | 6982.11 (42) | 7051.50 (42) | 13042.35 (42) | 12542.15 (42) |
P value | <0.0001 | <0.0001 | <0.0001 | <0.0001 |
Between‐hospital variance, (standard error) | 0.067 (0.008) | 0.078 (0.009) | 0.067 (0.006) | 0.072 (0.006) |
Reliability testing demonstrated consistent performance over several years. The frequency and ORs of the variables included in the model showed only minor changes over time. The area under the ROC curve (C statistic) was 0.73 for the model in the 2007 sample and 0.72 for the model using 2009 data (Table 3).
Hospital Risk‐Standardized Mortality Rates
The mean unadjusted hospital 30‐day mortality rate was 8.6% and ranged from 0% to 100% (Figure 2a). Risk‐standardized mortality rates varied across hospitals (Figure 2b). The mean risk‐standardized mortality rate was 8.6% and ranged from 5.9% to 13.5%. The odds of mortality at a hospital 1 standard deviation above average was 1.20 times that of a hospital 1 standard deviation below average.
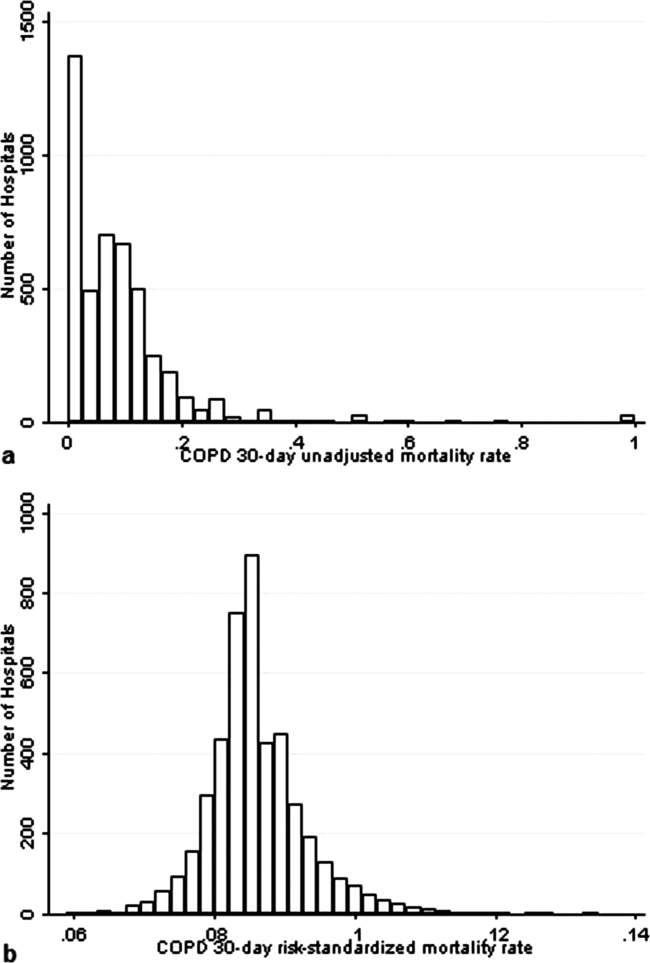
DISCUSSION
We present a hospital‐level risk‐standardized mortality measure for patients admitted with COPD based on administrative claims data that are intended for public reporting and that have achieved endorsement by the National Quality Forum, a voluntary consensus standards‐setting organization. Across more than 4500 US hospitals, the mean 30‐day risk‐standardized mortality rate in 2008 was 8.6%, and we observed considerable variation across institutions, despite adjustment for case mix, suggesting that improvement by lower‐performing institutions may be an achievable goal.
Although improving the delivery of evidence‐based care processes and outcomes of patients with acute myocardial infarction, heart failure, and pneumonia has been the focus of national quality improvement efforts for more than a decade, COPD has largely been overlooked.[23] Within this context, this analysis represents the first attempt to systematically measure, at the hospital level, 30‐day all‐cause mortality for patients admitted to US hospitals for exacerbation of COPD. The model we have developed and validated is intended to be used to compare the performance of hospitals while controlling for differences in the pretreatment risk of mortality of patients and accounting for the clustering of patients within hospitals, and will facilitate surveillance of hospital‐level risk‐adjusted outcomes over time.
In contrast to process‐based measures of quality, such as the percentage of patients with pneumonia who receive appropriate antibiotic therapy, performance measures based on patient outcomes provide a more comprehensive view of care and are more consistent with patients' goals.[24] Additionally, it is well established that hospital performance on individual and composite process measures explains only a small amount of the observed variation in patient outcomes between institutions.[25] In this regard, outcome measures incorporate important, but difficult to measure aspects of care, such as diagnostic accuracy and timing, communication and teamwork, the recognition and response to complications, care coordination at the time of transfers between levels of care, and care settings. Nevertheless, when used for making inferences about the quality of hospital care, individual measures such as the risk‐standardized hospital mortality rate should be interpreted in the context of other performance measures, including readmission, patient experience, and costs of care.
A number of prior investigators have described the outcomes of care for patients hospitalized with exacerbations of COPD, including identifying risk factors for mortality. Patil et al. carried out an analysis of the 1996 Nationwide Inpatient Sample and described an overall in‐hospital mortality rate of 2.5% among patients with COPD, and reported that a multivariable model containing sociodemographic characteristics about the patient and comorbidities had an area under the ROC curve of 0.70.[3] In contrast, this hospital‐level measure includes patients with a principal diagnosis of respiratory failure and focuses on 30‐day rather than inpatient mortality, accounting for the nearly 3‐fold higher mortality rate we observed. In a more recent study that used clinical from a large multistate database, Tabak et al. developed a prediction model for inpatient mortality for patients with COPD that contained only 4 factors: age, blood urea nitrogen, mental status, and pulse, and achieved an area under the ROC curve of 0.72.[4] The simplicity of such a model and its reliance on clinical measurements makes it particularly well suited for bedside application by clinicians, but less valuable for large‐scale public reporting programs that rely on administrative data. In the only other study identified that focused on the assessment of hospital mortality rates, Agabiti et al. analyzed the outcomes of 12,756 patients hospitalized for exacerbations of COPD, using similar ICD‐9‐CM diagnostic criteria as in this study, at 21 hospitals in Rome, Italy.[26] They reported an average crude 30‐day mortality rate of 3.8% among a group of 5 benchmark hospitals and an average mortality of 7.5% (range, 5.2%17.2%) among the remaining institutions.
To put the variation we observed in mortality rates into a broader context, the relative difference in the risk‐standardized hospital mortality rates across the 10th to 90th percentiles of hospital performance was 25% for acute myocardial infarction and 39% for heart failure, whereas rates varied 30% for COPD, from 7.6% to 9.9%.[27] Model discrimination in COPD (C statistic, 0.72) was also similar to that reported for models used for public reporting of hospital mortality in acute myocardial infarction (C statistic, 0.71) and pneumonia (C statistic, 0.72).
This study has a number of important strengths. First, the model was developed from a large sample of recent Medicare claims, achieved good discrimination, and was validated in samples not limited to Medicare beneficiaries. Second, by including patients with a principal diagnosis of COPD, as well as those with a principal diagnosis of acute respiratory failure when accompanied by a secondary diagnosis of COPD with acute exacerbation, this model can be used to assess hospital performance across the full spectrum of disease severity. This broad set of ICD‐9‐CM codes used to define the cohort also ensures that efforts to measure hospital performance will be less influenced by differences in documentation and coding practices across hospitals relating to the diagnosis or sequencing of acute respiratory failure diagnoses. Moreover, the inclusion of patients with respiratory failure is important because these patients have the greatest risk of mortality, and are those in whom efforts to improve the quality and safety of care may have the greatest impact. Third, rather than relying solely on information documented during the index admission, we used ambulatory and inpatient claims from the full year prior to the index admission to identify comorbidities and to distinguish them from potential complications of care. Finally, we did not include factors such as hospital characteristics (eg, number of beds, teaching status) in the model. Although they might have improved overall predictive ability, the goal of the hospital mortality measure is to enable comparisons of mortality rates among hospitals while controlling for differences in patient characteristics. To the extent that factors such as size or teaching status might be independently associated with hospital outcomes, it would be inappropriate to adjust away their effects, because mortality risk should not be influenced by hospital characteristics other than through their effects on quality.
These results should be viewed in light of several limitations. First, we used ICD‐9‐CM codes derived from claims files to define the patient populations included in the measure rather than collecting clinical or physiologic information prospectively or through manual review of medical records, such as the forced expiratory volume in 1 second or whether the patient required long‐term oxygen therapy. Nevertheless, we included a broad set of potential diagnosis codes to capture the full spectrum of COPD exacerbations and to minimize differences in coding across hospitals. Second, because the risk‐adjustment included diagnoses coded in the year prior to the index admission, it is potentially subject to bias due to regional differences in medical care utilization that are not driven by underlying differences in patient illness.[28] Third, using administrative claims data, we observed some paradoxical associations in the model that are difficult to explain on clinical grounds, such as a protective effect of substance and alcohol abuse or prior episodes of respiratory failure. Fourth, although we excluded patients from the analysis who were enrolled in hospice prior to, or on the day of, the index admission, we did not exclude those who choose to withdraw support, transition to comfort measures only, or enrolled in hospice care during a hospitalization. We do not seek to penalize hospitals for being sensitive to the preferences of patients at the end of life. At the same time, it is equally important that the measure is capable of detecting the outcomes of suboptimal care that may in some instances lead a patient or their family to withdraw support or choose hospice. Finally, we did not have the opportunity to validate the model against a clinical registry of patients with COPD, because such data do not currently exist. Nevertheless, the use of claims as a surrogate for chart data for risk adjustment has been validated for several conditions, including acute myocardial infarction, heart failure, and pneumonia.[29, 30]
CONCLUSIONS
Risk‐standardized 30‐day mortality rates for Medicare beneficiaries with COPD vary across hospitals in the US. Calculating and reporting hospital outcomes using validated performance measures may catalyze quality improvement activities and lead to better outcomes. Additional research would be helpful to confirm that hospitals with lower mortality rates achieve care that meets the goals of patients and their families better than at hospitals with higher mortality rates.
Acknowledgment
The authors thank the following members of the technical expert panel: Darlene Bainbridge, RN, MS, NHA, CPHQ, CPHRM, President/CEO, Darlene D. Bainbridge & Associates, Inc.; Robert A. Balk, MD, Director of Pulmonary and Critical Care Medicine, Rush University Medical Center; Dale Bratzler, DO, MPH, President and CEO, Oklahoma Foundation for Medical Quality; Scott Cerreta, RRT, Director of Education, COPD Foundation; Gerard J. Criner, MD, Director of Temple Lung Center and Divisions of Pulmonary and Critical Care Medicine, Temple University; Guy D'Andrea, MBA, President, Discern Consulting; Jonathan Fine, MD, Director of Pulmonary Fellowship, Research and Medical Education, Norwalk Hospital; David Hopkins, MS, PhD, Senior Advisor, Pacific Business Group on Health; Fred Martin Jacobs, MD, JD, FACP, FCCP, FCLM, Executive Vice President and Director, Saint Barnabas Quality Institute; Natalie Napolitano, MPH, RRT‐NPS, Respiratory Therapist, Inova Fairfax Hospital; Russell Robbins, MD, MBA, Principal and Senior Clinical Consultant, Mercer. In addition, the authors acknowledge and thank Angela Merrill, Sandi Nelson, Marian Wrobel, and Eric Schone from Mathematica Policy Research, Inc., Sharon‐Lise T. Normand from Harvard Medical School, and Lein Han and Michael Rapp at The Centers for Medicare & Medicaid Services for their contributions to this work.
Disclosures
Peter K. Lindenauer, MD, MSc, is the guarantor of this article, taking responsibility for the integrity of the work as a whole, from inception to published article, and takes responsibility for the content of the manuscript, including the data and data analysis. All authors have made substantial contributions to the conception and design, or acquisition of data, or analysis and interpretation of data; have drafted the submitted article or revised it critically for important intellectual content; and have provided final approval of the version to be published. Preparation of this manuscript was completed under Contract Number: HHSM‐5002008‐0025I/HHSM‐500‐T0001, Modification No. 000007, Option Year 2 Measure Instrument Development and Support (MIDS). Sponsors did not contribute to the development of the research or manuscript. Dr. Au reports being an unpaid research consultant for Bosch Inc. He receives research funding from the NIH, Department of Veterans Affairs, AHRQ, and Gilead Sciences. The views of the this manuscript represent the authors and do not necessarily represent those of the Department of Veterans Affairs. Drs. Drye and Bernheim report receiving contract funding from CMS to develop and maintain quality measures.
- FASTSTATS—chronic lower respiratory disease. Available at: http://www.cdc.gov/nchs/fastats/copd.htm. Accessed September 18, 2010.
- National Heart, Lung and Blood Institute. Morbidity and mortality chartbook. Available at: http://www.nhlbi.nih.gov/resources/docs/cht‐book.htm. Accessed April 27, 2010.
- In‐hospital mortality following acute exacerbations of chronic obstructive pulmonary disease. Arch Intern Med. 2003;163(10):1180–1186. , , , .
- Mortality and need for mechanical ventilation in acute exacerbations of chronic obstructive pulmonary disease: development and validation of a simple risk score. Arch Intern Med. 2009;169(17):1595–1602. , , , , .
- Quality of care for patients hospitalized for acute exacerbations of chronic obstructive pulmonary disease. Ann Intern Med. 2006;144(12):894–903. , , , , , .
- Use of beta blockers and the risk of death in hospitalised patients with acute exacerbations of COPD. Thorax. 2008;63(4):301–305. , , , , .
- HCUP facts and figures: statistics on hospital‐based care in the United States, 2007. 2009. Available at: http://www.hcup‐us.ahrq.gov/reports.jsp. Accessed August 6, 2012. , , , , .
- Predictors of long‐term survival in elderly patients hospitalized for acute exacerbations of chronic obstructive pulmonary disease. Respirology. 2008;13(6):851–855. , .
- The impact on risk‐factor analysis of different mortality outcomes in COPD patients. Eur Respir J 2008;32(3):629–636. , , , , , .
- Clinical audit indicators of outcome following admission to hospital with acute exacerbation of chronic obstructive pulmonary disease. Thorax. 2002;57(2):137–141. , , , , , .
- The quality of obstructive lung disease care for adults in the United States as measured by adherence to recommended processes. Chest. 2006;130(6):1844–1850. , , , et al.
- Management of acute exacerbations of chronic obstructive pulmonary disease in the elderly: physician practices in the community hospital setting. J Okla State Med Assoc. 2004;97(6):227–232. , , , , , .
- Leadership by Example: Coordinating Government Roles in Improving Health Care Quality. Washington, DC: National Academies Press; 2002. , , .
- Patient Protection and Affordable Care Act [H.R. 3590], Pub. L. No. 111–148, §2702, 124 Stat. 119, 318–319 (March 23, 2010). Available at: http://www.gpo.gov/fdsys/pkg/PLAW‐111publ148/html/PLAW‐111publ148.htm. Accessed July 15, 2012.
- National Quality Forum. NQF Endorses Additional Pulmonary Measure. 2013. Available at: http://www.qualityforum.org/News_And_Resources/Press_Releases/2013/NQF_Endorses_Additional_Pulmonary_Measure.aspx. Accessed January 11, 2013.
- National Quality Forum. National voluntary consensus standards for patient outcomes: a consensus report. Washington, DC: National Quality Forum; 2011.
- The Measures Management System. The Centers for Medicare and Medicaid Services. Available at: http://www.cms.gov/Medicare/Quality‐Initiatives‐Patient‐Assessment‐Instruments/MMS/index.html?redirect=/MMS/. Accessed August 6, 2012.
- Standards for statistical models used for public reporting of health outcomes: an American Heart Association Scientific Statement from the Quality of Care and Outcomes Research Interdisciplinary Writing Group: cosponsored by the Council on Epidemiology and Prevention and the Stroke Council. Endorsed by the American College of Cardiology Foundation. Circulation. 2006;113(3):456–462. , , , et al.
- Comparison of hospital risk‐standardized mortality rates calculated by using in‐hospital and 30‐day models: an observational study with implications for hospital profiling. Ann Intern Med. 2012;156(1 pt 1):19–26. , , , et al.
- Diagnostic cost group hierarchical condition category models for Medicare risk adjustment. Report prepared for the Health Care Financing Administration. Health Economics Research, Inc.; 2000. Available at: http://www.cms.gov/Research‐Statistics‐Data‐and‐Systems/Statistics‐Trends‐and‐Reports/Reports/downloads/pope_2000_2.pdf. Accessed November 7, 2009. , , , et al.
- Statistical and clinical aspects of hospital outcomes profiling. Stat Sci. 2007;22(2):206–226. , .
- Using full probability models to compute probabilities of actual interest to decision makers. Int J Technol Assess Health Care. 2001;17(1):17–26. , .
- COPD performance measures: missing opportunities for improving care. Chest. 2010;137(5):1181–1189. , , .
- Measuring Performance For Treating Heart Attacks And Heart Failure: The Case For Outcomes Measurement. Health Aff. 2007;26(1):75–85. , , , , .
- Hospital quality for acute myocardial infarction: correlation among process measures and relationship with short‐term mortality. JAMA. 2006;296(1):72–78. , , , et al.
- Profiling hospital performance to monitor the quality of care: the case of COPD. Eur Respir J. 2010;35(5):1031–1038. , , , et al.
- Patterns of hospital performance in acute myocardial infarction and heart failure 30‐day mortality and readmission. Circ Cardiovasc Qual Outcomes. 2009;2(5):407–413. , , , et al.
- Geographic variation in diagnosis frequency and risk of death among Medicare beneficiaries. JAMA. 2011;305(11):1113–1118. , , , , .
- An administrative claims model for profiling hospital 30‐day mortality rates for pneumonia patients. PLoS ONE. 2011;6(4):e17401. , , , et al.
- An Administrative Claims Model Suitable for Profiling Hospital Performance Based on 30‐Day Mortality Rates Among Patients With Heart Failure. Circulation. 2006;113(13):1693–1701. , , , et al.
Chronic obstructive pulmonary disease (COPD) affects as many as 24 million individuals in the United States, is responsible for more than 700,000 annual hospital admissions, and is currently the nation's third leading cause of death, accounting for nearly $49.9 billion in medical spending in 2010.[1, 2] Reported in‐hospital mortality rates for patients hospitalized for exacerbations of COPD range from 2% to 5%.[3, 4, 5, 6, 7] Information about 30‐day mortality rates following hospitalization for COPD is more limited; however, international studies suggest that rates range from 3% to 9%,[8, 9] and 90‐day mortality rates exceed 15%.[10]
Despite this significant clinical and economic impact, there have been no large‐scale, sustained efforts to measure the quality or outcomes of hospital care for patients with COPD in the United States. What little is known about the treatment of patients with COPD suggests widespread opportunities to increase adherence to guideline‐recommended therapies, to reduce the use of ineffective treatments and tests, and to address variation in care across institutions.[5, 11, 12]
Public reporting of hospital performance is a key strategy for improving the quality and safety of hospital care, both in the United States and internationally.[13] Since 2007, the Centers for Medicare and Medicaid Services (CMS) has reported hospital mortality rates on the Hospital Compare Web site, and COPD is 1 of the conditions highlighted in the Affordable Care Act for future consideration.[14] Such initiatives rely on validated, risk‐adjusted performance measures for comparisons across institutions and to enable outcomes to be tracked over time. We present the development, validation, and results of a model intended for public reporting of risk‐standardized mortality rates for patients hospitalized with exacerbations of COPD that has been endorsed by the National Quality Forum.[15]
METHODS
Approach to Measure Development
We developed this measure in accordance with guidelines described by the National Quality Forum,[16] CMS' Measure Management System,[17] and the American Heart Association scientific statement, Standards for Statistical Models Used for Public Reporting of Health Outcomes.[18] Throughout the process we obtained expert clinical and stakeholder input through meetings with a clinical advisory group and a national technical expert panel (see Acknowledgments). Last, we presented the proposed measure specifications and a summary of the technical expert panel discussions online and made a widely distributed call for public comments. We took the comments into consideration during the final stages of measure development (available at
Data Sources
We used claims data from Medicare inpatient, outpatient, and carrier (physician) Standard Analytic Files from 2008 to develop and validate the model, and examined model reliability using data from 2007 and 2009. The Medicare enrollment database was used to determine Medicare Fee‐for‐Service enrollment and mortality.
Study Cohort
Admissions were considered eligible for inclusion if the patient was 65 years or older, was admitted to a nonfederal acute care hospital in the United States, and had a principal diagnosis of COPD or a principal diagnosis of acute respiratory failure or respiratory arrest when paired with a secondary diagnosis of COPD with exacerbation (Table 1).
ICD‐9‐CM | Description |
---|---|
| |
491.21 | Obstructive chronic bronchitis; with (acute) exacerbation; acute exacerbation of COPD, decompensated COPD, decompensated COPD with exacerbation |
491.22 | Obstructive chronic bronchitis; with acute bronchitis |
491.8 | Other chronic bronchitis; chronic: tracheitis, tracheobronchitis |
491.9 | Unspecified chronic bronchitis |
492.8 | Other emphysema; emphysema (lung or pulmonary): NOS, centriacinar, centrilobular, obstructive, panacinar, panlobular, unilateral, vesicular; MacLeod's syndrome; Swyer‐James syndrome; unilateral hyperlucent lung |
493.20 | Chronic obstructive asthma; asthma with COPD, chronic asthmatic bronchitis, unspecified |
493.21 | Chronic obstructive asthma; asthma with COPD, chronic asthmatic bronchitis, with status asthmaticus |
493.22 | Chronic obstructive asthma; asthma with COPD, chronic asthmatic bronchitis, with (acute) exacerbation |
496 | Chronic: nonspecific lung disease, obstructive lung disease, obstructive pulmonary disease (COPD) NOS. (Note: This code is not to be used with any code from categories 491493.) |
518.81a | Other diseases of lung; acute respiratory failure; respiratory failure NOS |
518.82a | Other diseases of lung; acute respiratory failure; other pulmonary insufficiency, acute respiratory distress |
518.84a | Other diseases of lung; acute respiratory failure; acute and chronic respiratory failure |
799.1a | Other ill‐defined and unknown causes of morbidity and mortality; respiratory arrest, cardiorespiratory failure |
If a patient was discharged and readmitted to a second hospital on the same or the next day, we combined the 2 acute care admissions into a single episode of care and assigned the mortality outcome to the first admitting hospital. We excluded admissions for patients who were enrolled in Medicare Hospice in the 12 months prior to or on the first day of the index hospitalization. An index admission was any eligible admission assessed in the measure for the outcome. We also excluded admissions for patients who were discharged against medical advice, those for whom vital status at 30 days was unknown or recorded inconsistently, and patients with unreliable data (eg, age >115 years). For patients with multiple hospitalizations during a single year, we randomly selected 1 admission per patient to avoid survival bias. Finally, to assure adequate risk adjustment we limited the analysis to patients who had continuous enrollment in Medicare Fee‐for‐Service Parts A and B for the 12 months prior to their index admission so that we could identify comorbid conditions coded during all prior encounters.
Outcomes
The outcome of 30‐day mortality was defined as death from any cause within 30 days of the admission date for the index hospitalization. Mortality was assessed at 30 days to standardize the period of outcome ascertainment,[19] and because 30 days is a clinically meaningful time frame, during which differences in the quality of hospital care may be revealed.
Risk‐Adjustment Variables
We randomly selected half of all COPD admissions in 2008 that met the inclusion and exclusion criteria to create a model development sample. Candidate variables for inclusion in the risk‐standardized model were selected by a clinician team from diagnostic groups included in the Hierarchical Condition Category clinical classification system[20] and included age and comorbid conditions. Sleep apnea (International Classification of Diseases, 9th Revision, Clinical Modification [ICD‐9‐CM] condition codes 327.20, 327.21, 327.23, 327.27, 327.29, 780.51, 780.53, and 780.57) and mechanical ventilation (ICD‐9‐CM procedure codes 93.90, 96.70, 96.71, and 96.72) were also included as candidate variables.
We defined a condition as present for a given patient if it was coded in the inpatient, outpatient, or physician claims data sources in the preceding 12 months, including the index admission. Because a subset of the condition category variables can represent a complication of care, we did not consider them to be risk factors if they appeared only as secondary diagnosis codes for the index admission and not in claims submitted during the prior year.
We selected final variables for inclusion in the risk‐standardized model based on clinical considerations and a modified approach to stepwise logistic regression. The final patient‐level risk‐adjustment model included 42 variables (Table 2).
Variable | Development Sample (150,035 Admissions at 4537 Hospitals) | Validation Sample (149,646 Admissions at 4535 Hospitals) | ||||
---|---|---|---|---|---|---|
Frequency, % | OR | 95% CI | Frequency, % | OR | 95% CI | |
| ||||||
Demographics | ||||||
Age 65 years (continuous) | 1.03 | 1.03‐1.04 | 1.03 | 1.03‐1.04 | ||
Cardiovascular/respiratory | ||||||
Sleep apnea (ICD‐9‐CM: 327.20, 327.21, 327.23, 327.27, 327.29, 780.51, 780.53, 780.57)a | 9.57 | 0.87 | 0.81‐0.94 | 9.72 | 0.84 | 0.78‐0.90 |
History of mechanical ventilation (ICD‐9‐CM: 93.90, 96.70, 96.71, 96.72)a | 6.00 | 1.19 | 1.11‐1.27 | 6.00 | 1.15 | 1.08‐1.24 |
Respirator dependence/respiratory failure (CC 7778)a | 1.15 | 0.89 | 0.77‐1.02 | 1.20 | 0.78 | 0.68‐0.91 |
Cardiorespiratory failure and shock (CC 79) | 26.35 | 1.60 | 1.53‐1.68 | 26.34 | 1.59 | 1.52‐1.66 |
Congestive heart failure (CC 80) | 41.50 | 1.34 | 1.28‐1.39 | 41.39 | 1.31 | 1.25‐1.36 |
Chronic atherosclerosis (CC 8384)a | 50.44 | 0.87 | 0.83‐0.90 | 50.12 | 0.91 | 0.87‐0.94 |
Arrhythmias (CC 9293) | 37.15 | 1.17 | 1.12‐1.22 | 37.06 | 1.15 | 1.10‐1.20 |
Vascular or circulatory disease (CC 104106) | 38.20 | 1.09 | 1.05‐1.14 | 38.09 | 1.02 | 0.98‐1.06 |
Fibrosis of lung and other chronic lung disorder (CC 109) | 16.96 | 1.08 | 1.03‐1.13 | 17.08 | 1.11 | 1.06‐1.17 |
Asthma (CC 110) | 17.05 | 0.67 | 0.63‐0.70 | 16.90 | 0.67 | 0.63‐0.70 |
Pneumonia (CC 111113) | 49.46 | 1.29 | 1.24‐1.35 | 49.41 | 1.27 | 1.22‐1.33 |
Pleural effusion/pneumothorax (CC 114) | 11.78 | 1.17 | 1.11‐1.23 | 11.54 | 1.18 | 1.12‐1.25 |
Other lung disorders (CC 115) | 53.07 | 0.80 | 0.77‐0.83 | 53.17 | 0.83 | 0.80‐0.87 |
Other comorbid conditions | ||||||
Metastatic cancer and acute leukemia (CC 7) | 2.76 | 2.34 | 2.14‐2.56 | 2.79 | 2.15 | 1.97‐2.35 |
Lung, upper digestive tract, and other severe cancers (CC 8)a | 5.98 | 1.80 | 1.68‐1.92 | 6.02 | 1.98 | 1.85‐2.11 |
Lymphatic, head and neck, brain, and other major cancers; breast, prostate, colorectal and other cancers and tumors; other respiratory and heart neoplasms (CC 911) | 14.13 | 1.03 | 0.97‐1.08 | 14.19 | 1.01 | 0.95‐1.06 |
Other digestive and urinary neoplasms (CC 12) | 6.91 | 0.91 | 0.84‐0.98 | 7.05 | 0.85 | 0.79‐0.92 |
Diabetes and DM complications (CC 1520, 119120) | 38.31 | 0.91 | 0.87‐0.94 | 38.29 | 0.91 | 0.87‐0.94 |
Protein‐calorie malnutrition (CC 21) | 7.40 | 2.18 | 2.07‐2.30 | 7.44 | 2.09 | 1.98‐2.20 |
Disorders of fluid/electrolyte/acid‐base (CC 2223) | 32.05 | 1.13 | 1.08‐1.18 | 32.16 | 1.24 | 1.19‐1.30 |
Other endocrine/metabolic/nutritional disorders (CC 24) | 67.99 | 0.75 | 0.72‐0.78 | 67.88 | 0.76 | 0.73‐0.79 |
Other gastrointestinal disorders (CC 36) | 56.21 | 0.81 | 0.78‐0.84 | 56.18 | 0.78 | 0.75‐0.81 |
Osteoarthritis of hip or knee (CC 40) | 9.32 | 0.74 | 0.69‐0.79 | 9.33 | 0.80 | 0.74‐0.85 |
Other musculoskeletal and connective tissue disorders (CC 43) | 64.14 | 0.83 | 0.80‐0.86 | 64.20 | 0.83 | 0.80‐0.87 |
Iron deficiency and other/unspecified anemias and blood disease (CC 47) | 40.80 | 1.08 | 1.04‐1.12 | 40.72 | 1.08 | 1.04‐1.13 |
Dementia and senility (CC 4950) | 17.06 | 1.09 | 1.04‐1.14 | 16.97 | 1.09 | 1.04‐1.15 |
Drug/alcohol abuse, without dependence (CC 53)a | 23.51 | 0.78 | 0.75‐0.82 | 23.38 | 0.76 | 0.72‐0.80 |
Other psychiatric disorders (CC 60)a | 16.49 | 1.12 | 1.07‐1.18 | 16.43 | 1.12 | 1.06‐1.17 |
Quadriplegia, paraplegia, functional disability (CC 6769, 100102, 177178) | 4.92 | 1.03 | 0.95‐1.12 | 4.92 | 1.08 | 0.99‐1.17 |
Mononeuropathy, other neurological conditions/emnjuries (CC 76) | 11.35 | 0.85 | 0.80‐0.91 | 11.28 | 0.88 | 0.83‐0.93 |
Hypertension and hypertensive disease (CC 9091) | 80.40 | 0.78 | 0.75‐0.82 | 80.35 | 0.79 | 0.75‐0.83 |
Stroke (CC 9596)a | 6.77 | 1.00 | 0.93‐1.08 | 6.73 | 0.98 | 0.91‐1.05 |
Retinal disorders, except detachment and vascular retinopathies (CC 121) | 10.79 | 0.87 | 0.82‐0.93 | 10.69 | 0.90 | 0.85‐0.96 |
Other eye disorders (CC 124)a | 19.05 | 0.90 | 0.86‐0.95 | 19.13 | 0.98 | 0.85‐0.93 |
Other ear, nose, throat, and mouth disorders (CC 127) | 35.21 | 0.83 | 0.80‐0.87 | 35.02 | 0.80 | 0.77‐0.83 |
Renal failure (CC 131)a | 17.92 | 1.12 | 1.07‐1.18 | 18.16 | 1.13 | 1.08‐1.19 |
Decubitus ulcer or chronic skin ulcer (CC 148149) | 7.42 | 1.27 | 1.19‐1.35 | 7.42 | 1.33 | 1.25‐1.42 |
Other dermatological disorders (CC 153) | 28.46 | 0.90 | 0.87‐0.94 | 28.32 | 0.89 | 0.86‐0.93 |
Trauma (CC 154156, 158161) | 9.04 | 1.09 | 1.03‐1.16 | 8.99 | 1.15 | 1.08‐1.22 |
Vertebral fractures (CC 157) | 5.01 | 1.33 | 1.24‐1.44 | 4.97 | 1.29 | 1.20‐1.39 |
Major complications of medical care and trauma (CC 164) | 5.47 | 0.81 | 0.75‐0.88 | 5.55 | 0.82 | 0.76‐0.89 |
Model Derivation
We used hierarchical logistic regression models to model the log‐odds of mortality as a function of patient‐level clinical characteristics and a random hospital‐level intercept. At the patient level, each model adjusts the log‐odds of mortality for age and the selected clinical covariates. The second level models the hospital‐specific intercepts as arising from a normal distribution. The hospital intercept represents the underlying risk of mortality, after accounting for patient risk. If there were no differences among hospitals, then after adjusting for patient risk, the hospital intercepts should be identical across all hospitals.
Estimation of Hospital Risk‐Standardized Mortality Rate
We calculated a risk‐standardized mortality rate, defined as the ratio of predicted to expected deaths (similar to observed‐to‐expected), multiplied by the national unadjusted mortality rate.[21] The expected number of deaths for each hospital was estimated by applying the estimated regression coefficients to the characteristics of each hospital's patients, adding the average of the hospital‐specific intercepts, transforming the data by using an inverse logit function, and summing the data from all patients in the hospital to obtain the count. The predicted number of deaths was calculated in the same way, substituting the hospital‐specific intercept for the average hospital‐specific intercept.
Model Performance, Validation, and Reliability Testing
We used the remaining admissions in 2008 as the model validation sample. We computed several summary statistics to assess the patient‐level model performance in both the development and validation samples,[22] including over‐fitting indices, predictive ability, area under the receiver operating characteristic (ROC) curve, distribution of residuals, and model 2. In addition, we assessed face validity through a survey of members of the technical expert panel. To assess reliability of the model across data years, we repeated the modeling process using qualifying COPD admissions in both 2007 and 2009. Finally, to assess generalizability we evaluated the model's performance in an all‐payer sample of data from patients admitted to California hospitals in 2006.
Analyses were conducted using SAS version 9.1.3 (SAS Institute Inc., Cary, NC). We estimated the hierarchical models using the GLIMMIX procedure in SAS.
The Human Investigation Committee at the Yale University School of Medicine/Yale New Haven Hospital approved an exemption (HIC#0903004927) for the authors to use CMS claims and enrollment data for research analyses and publication.
RESULTS
Model Derivation
After exclusions were applied, the development sample included 150,035 admissions in 2008 at 4537 US hospitals (Figure 1). Factors that were most strongly associated with the risk of mortality included metastatic cancer (odds ratio [OR] 2.34), protein calorie malnutrition (OR 2.18), nonmetastatic cancers of the lung and upper digestive tract, (OR 1.80) cardiorespiratory failure and shock (OR 1.60), and congestive heart failure (OR 1.34) (Table 2).
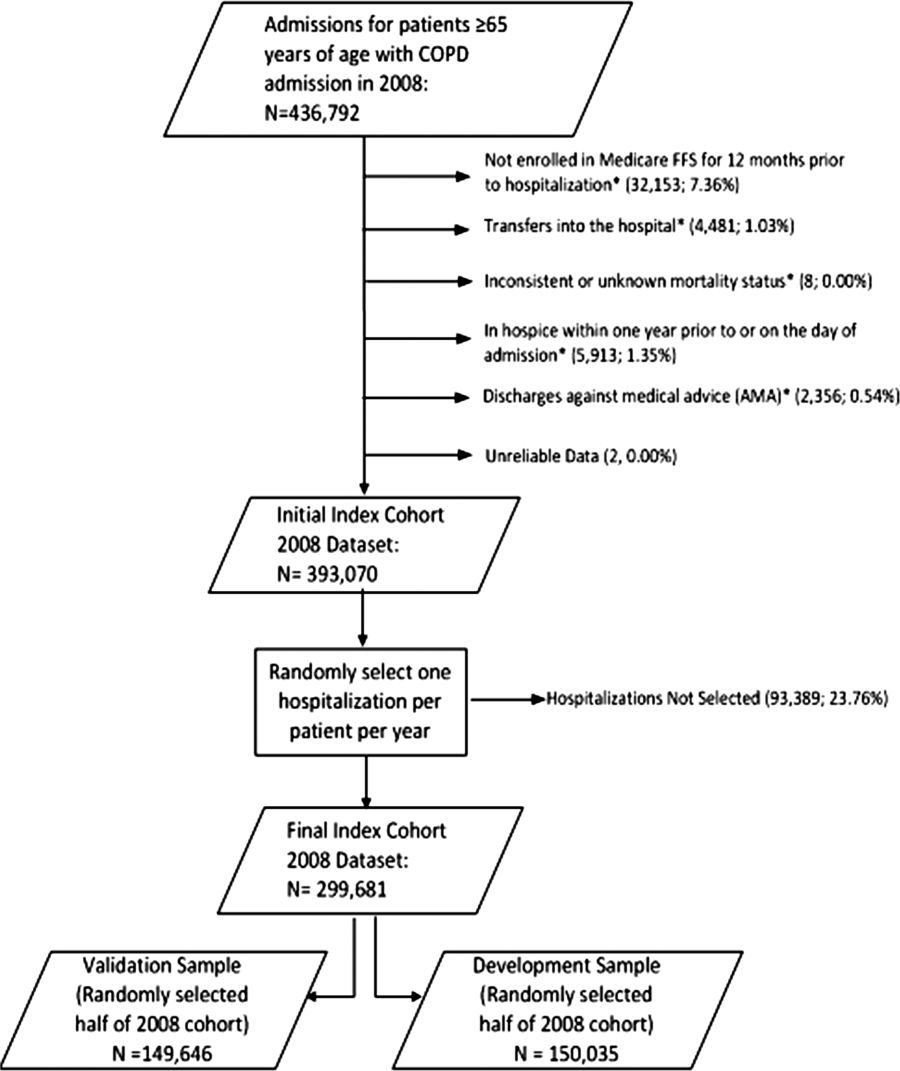
Model Performance, Validation, and Reliability
The model had a C statistic of 0.72, indicating good discrimination, and predicted mortality in the development sample ranged from 1.52% in the lowest decile to 23.74% in the highest. The model validation sample, using the remaining cases from 2008, included 149,646 admissions from 4535 hospitals. Variable frequencies and ORs were similar in both samples (Table 2). Model performance was also similar in the validation samples, with good model discrimination and fit (Table 3). Ten of 12 technical expert panel members responded to the survey, of whom 90% at least somewhat agreed with the statement, the COPD mortality measure provides an accurate reflection of quality. When the model was applied to patients age 18 years and older in the 2006 California Patient Discharge Data, overall discrimination was good (C statistic, 0.74), including in those age 18 to 64 years (C statistic, 0.75; 65 and above C statistic, 0.70).
Development | Validation | Data Years | ||
---|---|---|---|---|
Indices | Sample, 2008 | Sample, 2008 | 2007 | 2009 |
| ||||
Number of admissions | 150,035 | 149,646 | 259,911 | 279,377 |
Number of hospitals | 4537 | 4535 | 4636 | 4571 |
Mean risk‐standardized mortality rate, % (SD) | 8.62 (0.94) | 8.64 (1.07) | 8.97 (1.12) | 8.08 (1.09) |
Calibration, 0, 1 | 0.034, 0.985 | 0.009, 1.004 | 0.095, 1.022 | 0.120, 0.981 |
Discriminationpredictive ability, lowest decile %highest decile % | 1.5223.74 | 1.6023.78 | 1.5424.64 | 1.4222.36 |
Discriminationarea under the ROC curve, C statistic | 0.720 | 0.723 | 0.728 | 0.722 |
Residuals lack of fit, Pearson residual fall % | ||||
2 | 0 | 0 | 0 | 0 |
2, 0 | 91.14 | 91.4 | 91.08 | 91.93 |
0, 2 | 1.66 | 1.7 | 1.96 | 1.42 |
2+ | 6.93 | 6.91 | 6.96 | 6.65 |
Model Wald 2 (number of covariates) | 6982.11 (42) | 7051.50 (42) | 13042.35 (42) | 12542.15 (42) |
P value | <0.0001 | <0.0001 | <0.0001 | <0.0001 |
Between‐hospital variance, (standard error) | 0.067 (0.008) | 0.078 (0.009) | 0.067 (0.006) | 0.072 (0.006) |
Reliability testing demonstrated consistent performance over several years. The frequency and ORs of the variables included in the model showed only minor changes over time. The area under the ROC curve (C statistic) was 0.73 for the model in the 2007 sample and 0.72 for the model using 2009 data (Table 3).
Hospital Risk‐Standardized Mortality Rates
The mean unadjusted hospital 30‐day mortality rate was 8.6% and ranged from 0% to 100% (Figure 2a). Risk‐standardized mortality rates varied across hospitals (Figure 2b). The mean risk‐standardized mortality rate was 8.6% and ranged from 5.9% to 13.5%. The odds of mortality at a hospital 1 standard deviation above average was 1.20 times that of a hospital 1 standard deviation below average.
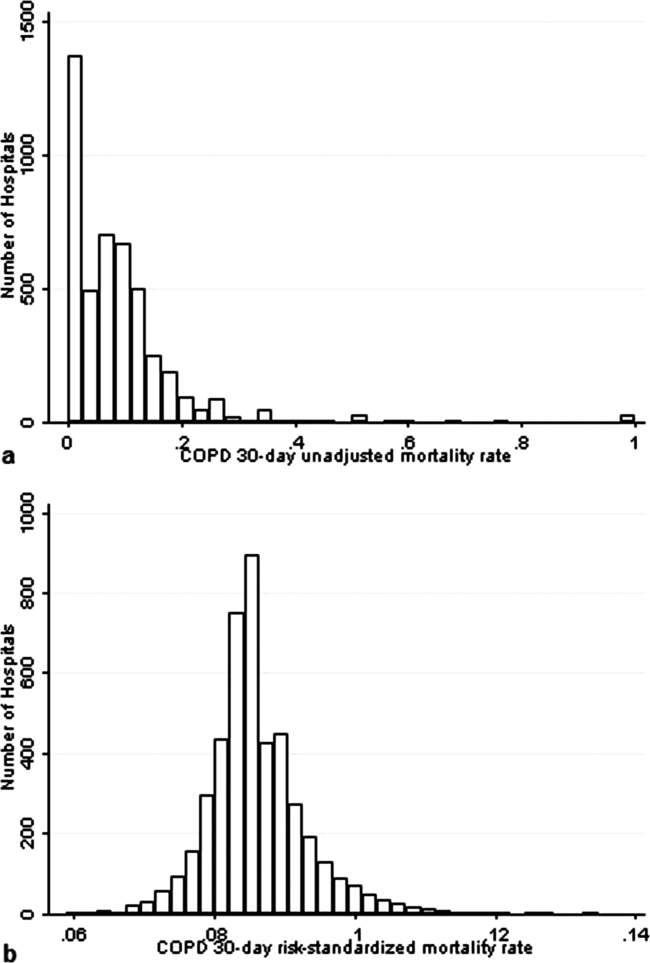
DISCUSSION
We present a hospital‐level risk‐standardized mortality measure for patients admitted with COPD based on administrative claims data that are intended for public reporting and that have achieved endorsement by the National Quality Forum, a voluntary consensus standards‐setting organization. Across more than 4500 US hospitals, the mean 30‐day risk‐standardized mortality rate in 2008 was 8.6%, and we observed considerable variation across institutions, despite adjustment for case mix, suggesting that improvement by lower‐performing institutions may be an achievable goal.
Although improving the delivery of evidence‐based care processes and outcomes of patients with acute myocardial infarction, heart failure, and pneumonia has been the focus of national quality improvement efforts for more than a decade, COPD has largely been overlooked.[23] Within this context, this analysis represents the first attempt to systematically measure, at the hospital level, 30‐day all‐cause mortality for patients admitted to US hospitals for exacerbation of COPD. The model we have developed and validated is intended to be used to compare the performance of hospitals while controlling for differences in the pretreatment risk of mortality of patients and accounting for the clustering of patients within hospitals, and will facilitate surveillance of hospital‐level risk‐adjusted outcomes over time.
In contrast to process‐based measures of quality, such as the percentage of patients with pneumonia who receive appropriate antibiotic therapy, performance measures based on patient outcomes provide a more comprehensive view of care and are more consistent with patients' goals.[24] Additionally, it is well established that hospital performance on individual and composite process measures explains only a small amount of the observed variation in patient outcomes between institutions.[25] In this regard, outcome measures incorporate important, but difficult to measure aspects of care, such as diagnostic accuracy and timing, communication and teamwork, the recognition and response to complications, care coordination at the time of transfers between levels of care, and care settings. Nevertheless, when used for making inferences about the quality of hospital care, individual measures such as the risk‐standardized hospital mortality rate should be interpreted in the context of other performance measures, including readmission, patient experience, and costs of care.
A number of prior investigators have described the outcomes of care for patients hospitalized with exacerbations of COPD, including identifying risk factors for mortality. Patil et al. carried out an analysis of the 1996 Nationwide Inpatient Sample and described an overall in‐hospital mortality rate of 2.5% among patients with COPD, and reported that a multivariable model containing sociodemographic characteristics about the patient and comorbidities had an area under the ROC curve of 0.70.[3] In contrast, this hospital‐level measure includes patients with a principal diagnosis of respiratory failure and focuses on 30‐day rather than inpatient mortality, accounting for the nearly 3‐fold higher mortality rate we observed. In a more recent study that used clinical from a large multistate database, Tabak et al. developed a prediction model for inpatient mortality for patients with COPD that contained only 4 factors: age, blood urea nitrogen, mental status, and pulse, and achieved an area under the ROC curve of 0.72.[4] The simplicity of such a model and its reliance on clinical measurements makes it particularly well suited for bedside application by clinicians, but less valuable for large‐scale public reporting programs that rely on administrative data. In the only other study identified that focused on the assessment of hospital mortality rates, Agabiti et al. analyzed the outcomes of 12,756 patients hospitalized for exacerbations of COPD, using similar ICD‐9‐CM diagnostic criteria as in this study, at 21 hospitals in Rome, Italy.[26] They reported an average crude 30‐day mortality rate of 3.8% among a group of 5 benchmark hospitals and an average mortality of 7.5% (range, 5.2%17.2%) among the remaining institutions.
To put the variation we observed in mortality rates into a broader context, the relative difference in the risk‐standardized hospital mortality rates across the 10th to 90th percentiles of hospital performance was 25% for acute myocardial infarction and 39% for heart failure, whereas rates varied 30% for COPD, from 7.6% to 9.9%.[27] Model discrimination in COPD (C statistic, 0.72) was also similar to that reported for models used for public reporting of hospital mortality in acute myocardial infarction (C statistic, 0.71) and pneumonia (C statistic, 0.72).
This study has a number of important strengths. First, the model was developed from a large sample of recent Medicare claims, achieved good discrimination, and was validated in samples not limited to Medicare beneficiaries. Second, by including patients with a principal diagnosis of COPD, as well as those with a principal diagnosis of acute respiratory failure when accompanied by a secondary diagnosis of COPD with acute exacerbation, this model can be used to assess hospital performance across the full spectrum of disease severity. This broad set of ICD‐9‐CM codes used to define the cohort also ensures that efforts to measure hospital performance will be less influenced by differences in documentation and coding practices across hospitals relating to the diagnosis or sequencing of acute respiratory failure diagnoses. Moreover, the inclusion of patients with respiratory failure is important because these patients have the greatest risk of mortality, and are those in whom efforts to improve the quality and safety of care may have the greatest impact. Third, rather than relying solely on information documented during the index admission, we used ambulatory and inpatient claims from the full year prior to the index admission to identify comorbidities and to distinguish them from potential complications of care. Finally, we did not include factors such as hospital characteristics (eg, number of beds, teaching status) in the model. Although they might have improved overall predictive ability, the goal of the hospital mortality measure is to enable comparisons of mortality rates among hospitals while controlling for differences in patient characteristics. To the extent that factors such as size or teaching status might be independently associated with hospital outcomes, it would be inappropriate to adjust away their effects, because mortality risk should not be influenced by hospital characteristics other than through their effects on quality.
These results should be viewed in light of several limitations. First, we used ICD‐9‐CM codes derived from claims files to define the patient populations included in the measure rather than collecting clinical or physiologic information prospectively or through manual review of medical records, such as the forced expiratory volume in 1 second or whether the patient required long‐term oxygen therapy. Nevertheless, we included a broad set of potential diagnosis codes to capture the full spectrum of COPD exacerbations and to minimize differences in coding across hospitals. Second, because the risk‐adjustment included diagnoses coded in the year prior to the index admission, it is potentially subject to bias due to regional differences in medical care utilization that are not driven by underlying differences in patient illness.[28] Third, using administrative claims data, we observed some paradoxical associations in the model that are difficult to explain on clinical grounds, such as a protective effect of substance and alcohol abuse or prior episodes of respiratory failure. Fourth, although we excluded patients from the analysis who were enrolled in hospice prior to, or on the day of, the index admission, we did not exclude those who choose to withdraw support, transition to comfort measures only, or enrolled in hospice care during a hospitalization. We do not seek to penalize hospitals for being sensitive to the preferences of patients at the end of life. At the same time, it is equally important that the measure is capable of detecting the outcomes of suboptimal care that may in some instances lead a patient or their family to withdraw support or choose hospice. Finally, we did not have the opportunity to validate the model against a clinical registry of patients with COPD, because such data do not currently exist. Nevertheless, the use of claims as a surrogate for chart data for risk adjustment has been validated for several conditions, including acute myocardial infarction, heart failure, and pneumonia.[29, 30]
CONCLUSIONS
Risk‐standardized 30‐day mortality rates for Medicare beneficiaries with COPD vary across hospitals in the US. Calculating and reporting hospital outcomes using validated performance measures may catalyze quality improvement activities and lead to better outcomes. Additional research would be helpful to confirm that hospitals with lower mortality rates achieve care that meets the goals of patients and their families better than at hospitals with higher mortality rates.
Acknowledgment
The authors thank the following members of the technical expert panel: Darlene Bainbridge, RN, MS, NHA, CPHQ, CPHRM, President/CEO, Darlene D. Bainbridge & Associates, Inc.; Robert A. Balk, MD, Director of Pulmonary and Critical Care Medicine, Rush University Medical Center; Dale Bratzler, DO, MPH, President and CEO, Oklahoma Foundation for Medical Quality; Scott Cerreta, RRT, Director of Education, COPD Foundation; Gerard J. Criner, MD, Director of Temple Lung Center and Divisions of Pulmonary and Critical Care Medicine, Temple University; Guy D'Andrea, MBA, President, Discern Consulting; Jonathan Fine, MD, Director of Pulmonary Fellowship, Research and Medical Education, Norwalk Hospital; David Hopkins, MS, PhD, Senior Advisor, Pacific Business Group on Health; Fred Martin Jacobs, MD, JD, FACP, FCCP, FCLM, Executive Vice President and Director, Saint Barnabas Quality Institute; Natalie Napolitano, MPH, RRT‐NPS, Respiratory Therapist, Inova Fairfax Hospital; Russell Robbins, MD, MBA, Principal and Senior Clinical Consultant, Mercer. In addition, the authors acknowledge and thank Angela Merrill, Sandi Nelson, Marian Wrobel, and Eric Schone from Mathematica Policy Research, Inc., Sharon‐Lise T. Normand from Harvard Medical School, and Lein Han and Michael Rapp at The Centers for Medicare & Medicaid Services for their contributions to this work.
Disclosures
Peter K. Lindenauer, MD, MSc, is the guarantor of this article, taking responsibility for the integrity of the work as a whole, from inception to published article, and takes responsibility for the content of the manuscript, including the data and data analysis. All authors have made substantial contributions to the conception and design, or acquisition of data, or analysis and interpretation of data; have drafted the submitted article or revised it critically for important intellectual content; and have provided final approval of the version to be published. Preparation of this manuscript was completed under Contract Number: HHSM‐5002008‐0025I/HHSM‐500‐T0001, Modification No. 000007, Option Year 2 Measure Instrument Development and Support (MIDS). Sponsors did not contribute to the development of the research or manuscript. Dr. Au reports being an unpaid research consultant for Bosch Inc. He receives research funding from the NIH, Department of Veterans Affairs, AHRQ, and Gilead Sciences. The views of the this manuscript represent the authors and do not necessarily represent those of the Department of Veterans Affairs. Drs. Drye and Bernheim report receiving contract funding from CMS to develop and maintain quality measures.
Chronic obstructive pulmonary disease (COPD) affects as many as 24 million individuals in the United States, is responsible for more than 700,000 annual hospital admissions, and is currently the nation's third leading cause of death, accounting for nearly $49.9 billion in medical spending in 2010.[1, 2] Reported in‐hospital mortality rates for patients hospitalized for exacerbations of COPD range from 2% to 5%.[3, 4, 5, 6, 7] Information about 30‐day mortality rates following hospitalization for COPD is more limited; however, international studies suggest that rates range from 3% to 9%,[8, 9] and 90‐day mortality rates exceed 15%.[10]
Despite this significant clinical and economic impact, there have been no large‐scale, sustained efforts to measure the quality or outcomes of hospital care for patients with COPD in the United States. What little is known about the treatment of patients with COPD suggests widespread opportunities to increase adherence to guideline‐recommended therapies, to reduce the use of ineffective treatments and tests, and to address variation in care across institutions.[5, 11, 12]
Public reporting of hospital performance is a key strategy for improving the quality and safety of hospital care, both in the United States and internationally.[13] Since 2007, the Centers for Medicare and Medicaid Services (CMS) has reported hospital mortality rates on the Hospital Compare Web site, and COPD is 1 of the conditions highlighted in the Affordable Care Act for future consideration.[14] Such initiatives rely on validated, risk‐adjusted performance measures for comparisons across institutions and to enable outcomes to be tracked over time. We present the development, validation, and results of a model intended for public reporting of risk‐standardized mortality rates for patients hospitalized with exacerbations of COPD that has been endorsed by the National Quality Forum.[15]
METHODS
Approach to Measure Development
We developed this measure in accordance with guidelines described by the National Quality Forum,[16] CMS' Measure Management System,[17] and the American Heart Association scientific statement, Standards for Statistical Models Used for Public Reporting of Health Outcomes.[18] Throughout the process we obtained expert clinical and stakeholder input through meetings with a clinical advisory group and a national technical expert panel (see Acknowledgments). Last, we presented the proposed measure specifications and a summary of the technical expert panel discussions online and made a widely distributed call for public comments. We took the comments into consideration during the final stages of measure development (available at
Data Sources
We used claims data from Medicare inpatient, outpatient, and carrier (physician) Standard Analytic Files from 2008 to develop and validate the model, and examined model reliability using data from 2007 and 2009. The Medicare enrollment database was used to determine Medicare Fee‐for‐Service enrollment and mortality.
Study Cohort
Admissions were considered eligible for inclusion if the patient was 65 years or older, was admitted to a nonfederal acute care hospital in the United States, and had a principal diagnosis of COPD or a principal diagnosis of acute respiratory failure or respiratory arrest when paired with a secondary diagnosis of COPD with exacerbation (Table 1).
ICD‐9‐CM | Description |
---|---|
| |
491.21 | Obstructive chronic bronchitis; with (acute) exacerbation; acute exacerbation of COPD, decompensated COPD, decompensated COPD with exacerbation |
491.22 | Obstructive chronic bronchitis; with acute bronchitis |
491.8 | Other chronic bronchitis; chronic: tracheitis, tracheobronchitis |
491.9 | Unspecified chronic bronchitis |
492.8 | Other emphysema; emphysema (lung or pulmonary): NOS, centriacinar, centrilobular, obstructive, panacinar, panlobular, unilateral, vesicular; MacLeod's syndrome; Swyer‐James syndrome; unilateral hyperlucent lung |
493.20 | Chronic obstructive asthma; asthma with COPD, chronic asthmatic bronchitis, unspecified |
493.21 | Chronic obstructive asthma; asthma with COPD, chronic asthmatic bronchitis, with status asthmaticus |
493.22 | Chronic obstructive asthma; asthma with COPD, chronic asthmatic bronchitis, with (acute) exacerbation |
496 | Chronic: nonspecific lung disease, obstructive lung disease, obstructive pulmonary disease (COPD) NOS. (Note: This code is not to be used with any code from categories 491493.) |
518.81a | Other diseases of lung; acute respiratory failure; respiratory failure NOS |
518.82a | Other diseases of lung; acute respiratory failure; other pulmonary insufficiency, acute respiratory distress |
518.84a | Other diseases of lung; acute respiratory failure; acute and chronic respiratory failure |
799.1a | Other ill‐defined and unknown causes of morbidity and mortality; respiratory arrest, cardiorespiratory failure |
If a patient was discharged and readmitted to a second hospital on the same or the next day, we combined the 2 acute care admissions into a single episode of care and assigned the mortality outcome to the first admitting hospital. We excluded admissions for patients who were enrolled in Medicare Hospice in the 12 months prior to or on the first day of the index hospitalization. An index admission was any eligible admission assessed in the measure for the outcome. We also excluded admissions for patients who were discharged against medical advice, those for whom vital status at 30 days was unknown or recorded inconsistently, and patients with unreliable data (eg, age >115 years). For patients with multiple hospitalizations during a single year, we randomly selected 1 admission per patient to avoid survival bias. Finally, to assure adequate risk adjustment we limited the analysis to patients who had continuous enrollment in Medicare Fee‐for‐Service Parts A and B for the 12 months prior to their index admission so that we could identify comorbid conditions coded during all prior encounters.
Outcomes
The outcome of 30‐day mortality was defined as death from any cause within 30 days of the admission date for the index hospitalization. Mortality was assessed at 30 days to standardize the period of outcome ascertainment,[19] and because 30 days is a clinically meaningful time frame, during which differences in the quality of hospital care may be revealed.
Risk‐Adjustment Variables
We randomly selected half of all COPD admissions in 2008 that met the inclusion and exclusion criteria to create a model development sample. Candidate variables for inclusion in the risk‐standardized model were selected by a clinician team from diagnostic groups included in the Hierarchical Condition Category clinical classification system[20] and included age and comorbid conditions. Sleep apnea (International Classification of Diseases, 9th Revision, Clinical Modification [ICD‐9‐CM] condition codes 327.20, 327.21, 327.23, 327.27, 327.29, 780.51, 780.53, and 780.57) and mechanical ventilation (ICD‐9‐CM procedure codes 93.90, 96.70, 96.71, and 96.72) were also included as candidate variables.
We defined a condition as present for a given patient if it was coded in the inpatient, outpatient, or physician claims data sources in the preceding 12 months, including the index admission. Because a subset of the condition category variables can represent a complication of care, we did not consider them to be risk factors if they appeared only as secondary diagnosis codes for the index admission and not in claims submitted during the prior year.
We selected final variables for inclusion in the risk‐standardized model based on clinical considerations and a modified approach to stepwise logistic regression. The final patient‐level risk‐adjustment model included 42 variables (Table 2).
Variable | Development Sample (150,035 Admissions at 4537 Hospitals) | Validation Sample (149,646 Admissions at 4535 Hospitals) | ||||
---|---|---|---|---|---|---|
Frequency, % | OR | 95% CI | Frequency, % | OR | 95% CI | |
| ||||||
Demographics | ||||||
Age 65 years (continuous) | 1.03 | 1.03‐1.04 | 1.03 | 1.03‐1.04 | ||
Cardiovascular/respiratory | ||||||
Sleep apnea (ICD‐9‐CM: 327.20, 327.21, 327.23, 327.27, 327.29, 780.51, 780.53, 780.57)a | 9.57 | 0.87 | 0.81‐0.94 | 9.72 | 0.84 | 0.78‐0.90 |
History of mechanical ventilation (ICD‐9‐CM: 93.90, 96.70, 96.71, 96.72)a | 6.00 | 1.19 | 1.11‐1.27 | 6.00 | 1.15 | 1.08‐1.24 |
Respirator dependence/respiratory failure (CC 7778)a | 1.15 | 0.89 | 0.77‐1.02 | 1.20 | 0.78 | 0.68‐0.91 |
Cardiorespiratory failure and shock (CC 79) | 26.35 | 1.60 | 1.53‐1.68 | 26.34 | 1.59 | 1.52‐1.66 |
Congestive heart failure (CC 80) | 41.50 | 1.34 | 1.28‐1.39 | 41.39 | 1.31 | 1.25‐1.36 |
Chronic atherosclerosis (CC 8384)a | 50.44 | 0.87 | 0.83‐0.90 | 50.12 | 0.91 | 0.87‐0.94 |
Arrhythmias (CC 9293) | 37.15 | 1.17 | 1.12‐1.22 | 37.06 | 1.15 | 1.10‐1.20 |
Vascular or circulatory disease (CC 104106) | 38.20 | 1.09 | 1.05‐1.14 | 38.09 | 1.02 | 0.98‐1.06 |
Fibrosis of lung and other chronic lung disorder (CC 109) | 16.96 | 1.08 | 1.03‐1.13 | 17.08 | 1.11 | 1.06‐1.17 |
Asthma (CC 110) | 17.05 | 0.67 | 0.63‐0.70 | 16.90 | 0.67 | 0.63‐0.70 |
Pneumonia (CC 111113) | 49.46 | 1.29 | 1.24‐1.35 | 49.41 | 1.27 | 1.22‐1.33 |
Pleural effusion/pneumothorax (CC 114) | 11.78 | 1.17 | 1.11‐1.23 | 11.54 | 1.18 | 1.12‐1.25 |
Other lung disorders (CC 115) | 53.07 | 0.80 | 0.77‐0.83 | 53.17 | 0.83 | 0.80‐0.87 |
Other comorbid conditions | ||||||
Metastatic cancer and acute leukemia (CC 7) | 2.76 | 2.34 | 2.14‐2.56 | 2.79 | 2.15 | 1.97‐2.35 |
Lung, upper digestive tract, and other severe cancers (CC 8)a | 5.98 | 1.80 | 1.68‐1.92 | 6.02 | 1.98 | 1.85‐2.11 |
Lymphatic, head and neck, brain, and other major cancers; breast, prostate, colorectal and other cancers and tumors; other respiratory and heart neoplasms (CC 911) | 14.13 | 1.03 | 0.97‐1.08 | 14.19 | 1.01 | 0.95‐1.06 |
Other digestive and urinary neoplasms (CC 12) | 6.91 | 0.91 | 0.84‐0.98 | 7.05 | 0.85 | 0.79‐0.92 |
Diabetes and DM complications (CC 1520, 119120) | 38.31 | 0.91 | 0.87‐0.94 | 38.29 | 0.91 | 0.87‐0.94 |
Protein‐calorie malnutrition (CC 21) | 7.40 | 2.18 | 2.07‐2.30 | 7.44 | 2.09 | 1.98‐2.20 |
Disorders of fluid/electrolyte/acid‐base (CC 2223) | 32.05 | 1.13 | 1.08‐1.18 | 32.16 | 1.24 | 1.19‐1.30 |
Other endocrine/metabolic/nutritional disorders (CC 24) | 67.99 | 0.75 | 0.72‐0.78 | 67.88 | 0.76 | 0.73‐0.79 |
Other gastrointestinal disorders (CC 36) | 56.21 | 0.81 | 0.78‐0.84 | 56.18 | 0.78 | 0.75‐0.81 |
Osteoarthritis of hip or knee (CC 40) | 9.32 | 0.74 | 0.69‐0.79 | 9.33 | 0.80 | 0.74‐0.85 |
Other musculoskeletal and connective tissue disorders (CC 43) | 64.14 | 0.83 | 0.80‐0.86 | 64.20 | 0.83 | 0.80‐0.87 |
Iron deficiency and other/unspecified anemias and blood disease (CC 47) | 40.80 | 1.08 | 1.04‐1.12 | 40.72 | 1.08 | 1.04‐1.13 |
Dementia and senility (CC 4950) | 17.06 | 1.09 | 1.04‐1.14 | 16.97 | 1.09 | 1.04‐1.15 |
Drug/alcohol abuse, without dependence (CC 53)a | 23.51 | 0.78 | 0.75‐0.82 | 23.38 | 0.76 | 0.72‐0.80 |
Other psychiatric disorders (CC 60)a | 16.49 | 1.12 | 1.07‐1.18 | 16.43 | 1.12 | 1.06‐1.17 |
Quadriplegia, paraplegia, functional disability (CC 6769, 100102, 177178) | 4.92 | 1.03 | 0.95‐1.12 | 4.92 | 1.08 | 0.99‐1.17 |
Mononeuropathy, other neurological conditions/emnjuries (CC 76) | 11.35 | 0.85 | 0.80‐0.91 | 11.28 | 0.88 | 0.83‐0.93 |
Hypertension and hypertensive disease (CC 9091) | 80.40 | 0.78 | 0.75‐0.82 | 80.35 | 0.79 | 0.75‐0.83 |
Stroke (CC 9596)a | 6.77 | 1.00 | 0.93‐1.08 | 6.73 | 0.98 | 0.91‐1.05 |
Retinal disorders, except detachment and vascular retinopathies (CC 121) | 10.79 | 0.87 | 0.82‐0.93 | 10.69 | 0.90 | 0.85‐0.96 |
Other eye disorders (CC 124)a | 19.05 | 0.90 | 0.86‐0.95 | 19.13 | 0.98 | 0.85‐0.93 |
Other ear, nose, throat, and mouth disorders (CC 127) | 35.21 | 0.83 | 0.80‐0.87 | 35.02 | 0.80 | 0.77‐0.83 |
Renal failure (CC 131)a | 17.92 | 1.12 | 1.07‐1.18 | 18.16 | 1.13 | 1.08‐1.19 |
Decubitus ulcer or chronic skin ulcer (CC 148149) | 7.42 | 1.27 | 1.19‐1.35 | 7.42 | 1.33 | 1.25‐1.42 |
Other dermatological disorders (CC 153) | 28.46 | 0.90 | 0.87‐0.94 | 28.32 | 0.89 | 0.86‐0.93 |
Trauma (CC 154156, 158161) | 9.04 | 1.09 | 1.03‐1.16 | 8.99 | 1.15 | 1.08‐1.22 |
Vertebral fractures (CC 157) | 5.01 | 1.33 | 1.24‐1.44 | 4.97 | 1.29 | 1.20‐1.39 |
Major complications of medical care and trauma (CC 164) | 5.47 | 0.81 | 0.75‐0.88 | 5.55 | 0.82 | 0.76‐0.89 |
Model Derivation
We used hierarchical logistic regression models to model the log‐odds of mortality as a function of patient‐level clinical characteristics and a random hospital‐level intercept. At the patient level, each model adjusts the log‐odds of mortality for age and the selected clinical covariates. The second level models the hospital‐specific intercepts as arising from a normal distribution. The hospital intercept represents the underlying risk of mortality, after accounting for patient risk. If there were no differences among hospitals, then after adjusting for patient risk, the hospital intercepts should be identical across all hospitals.
Estimation of Hospital Risk‐Standardized Mortality Rate
We calculated a risk‐standardized mortality rate, defined as the ratio of predicted to expected deaths (similar to observed‐to‐expected), multiplied by the national unadjusted mortality rate.[21] The expected number of deaths for each hospital was estimated by applying the estimated regression coefficients to the characteristics of each hospital's patients, adding the average of the hospital‐specific intercepts, transforming the data by using an inverse logit function, and summing the data from all patients in the hospital to obtain the count. The predicted number of deaths was calculated in the same way, substituting the hospital‐specific intercept for the average hospital‐specific intercept.
Model Performance, Validation, and Reliability Testing
We used the remaining admissions in 2008 as the model validation sample. We computed several summary statistics to assess the patient‐level model performance in both the development and validation samples,[22] including over‐fitting indices, predictive ability, area under the receiver operating characteristic (ROC) curve, distribution of residuals, and model 2. In addition, we assessed face validity through a survey of members of the technical expert panel. To assess reliability of the model across data years, we repeated the modeling process using qualifying COPD admissions in both 2007 and 2009. Finally, to assess generalizability we evaluated the model's performance in an all‐payer sample of data from patients admitted to California hospitals in 2006.
Analyses were conducted using SAS version 9.1.3 (SAS Institute Inc., Cary, NC). We estimated the hierarchical models using the GLIMMIX procedure in SAS.
The Human Investigation Committee at the Yale University School of Medicine/Yale New Haven Hospital approved an exemption (HIC#0903004927) for the authors to use CMS claims and enrollment data for research analyses and publication.
RESULTS
Model Derivation
After exclusions were applied, the development sample included 150,035 admissions in 2008 at 4537 US hospitals (Figure 1). Factors that were most strongly associated with the risk of mortality included metastatic cancer (odds ratio [OR] 2.34), protein calorie malnutrition (OR 2.18), nonmetastatic cancers of the lung and upper digestive tract, (OR 1.80) cardiorespiratory failure and shock (OR 1.60), and congestive heart failure (OR 1.34) (Table 2).
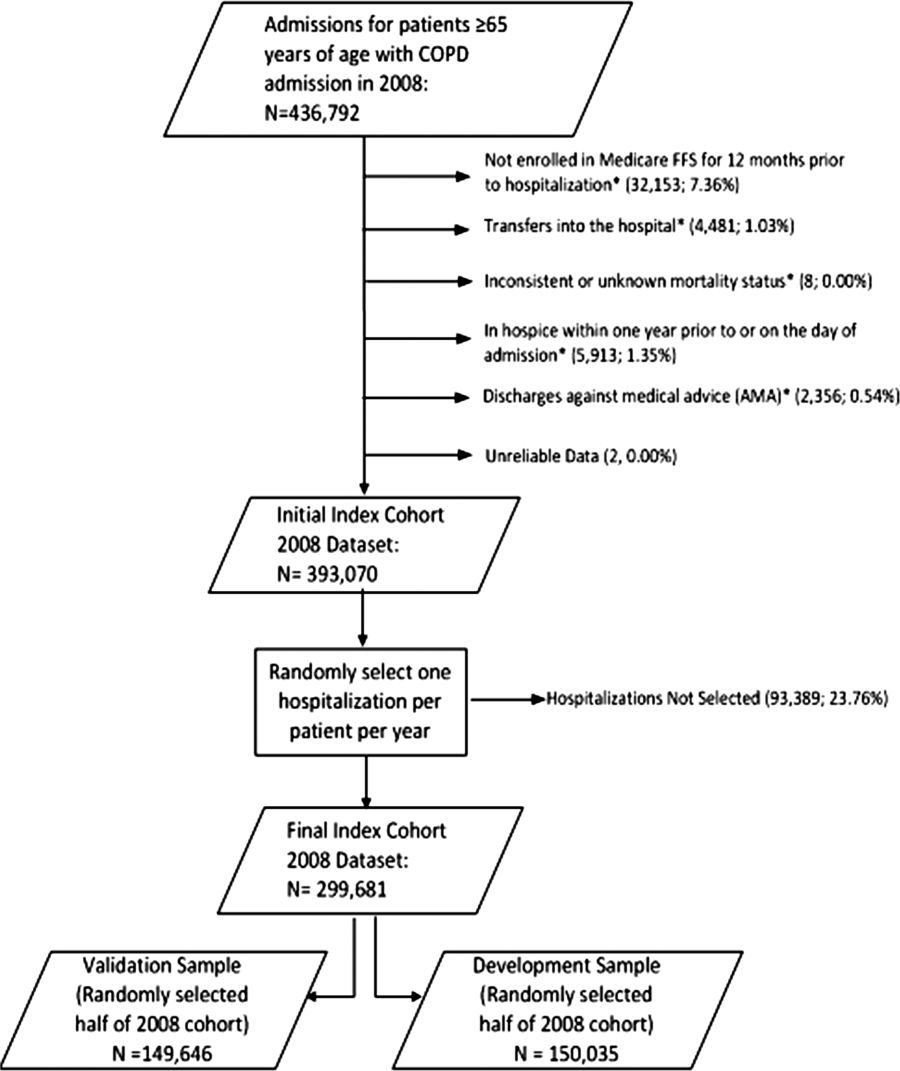
Model Performance, Validation, and Reliability
The model had a C statistic of 0.72, indicating good discrimination, and predicted mortality in the development sample ranged from 1.52% in the lowest decile to 23.74% in the highest. The model validation sample, using the remaining cases from 2008, included 149,646 admissions from 4535 hospitals. Variable frequencies and ORs were similar in both samples (Table 2). Model performance was also similar in the validation samples, with good model discrimination and fit (Table 3). Ten of 12 technical expert panel members responded to the survey, of whom 90% at least somewhat agreed with the statement, the COPD mortality measure provides an accurate reflection of quality. When the model was applied to patients age 18 years and older in the 2006 California Patient Discharge Data, overall discrimination was good (C statistic, 0.74), including in those age 18 to 64 years (C statistic, 0.75; 65 and above C statistic, 0.70).
Development | Validation | Data Years | ||
---|---|---|---|---|
Indices | Sample, 2008 | Sample, 2008 | 2007 | 2009 |
| ||||
Number of admissions | 150,035 | 149,646 | 259,911 | 279,377 |
Number of hospitals | 4537 | 4535 | 4636 | 4571 |
Mean risk‐standardized mortality rate, % (SD) | 8.62 (0.94) | 8.64 (1.07) | 8.97 (1.12) | 8.08 (1.09) |
Calibration, 0, 1 | 0.034, 0.985 | 0.009, 1.004 | 0.095, 1.022 | 0.120, 0.981 |
Discriminationpredictive ability, lowest decile %highest decile % | 1.5223.74 | 1.6023.78 | 1.5424.64 | 1.4222.36 |
Discriminationarea under the ROC curve, C statistic | 0.720 | 0.723 | 0.728 | 0.722 |
Residuals lack of fit, Pearson residual fall % | ||||
2 | 0 | 0 | 0 | 0 |
2, 0 | 91.14 | 91.4 | 91.08 | 91.93 |
0, 2 | 1.66 | 1.7 | 1.96 | 1.42 |
2+ | 6.93 | 6.91 | 6.96 | 6.65 |
Model Wald 2 (number of covariates) | 6982.11 (42) | 7051.50 (42) | 13042.35 (42) | 12542.15 (42) |
P value | <0.0001 | <0.0001 | <0.0001 | <0.0001 |
Between‐hospital variance, (standard error) | 0.067 (0.008) | 0.078 (0.009) | 0.067 (0.006) | 0.072 (0.006) |
Reliability testing demonstrated consistent performance over several years. The frequency and ORs of the variables included in the model showed only minor changes over time. The area under the ROC curve (C statistic) was 0.73 for the model in the 2007 sample and 0.72 for the model using 2009 data (Table 3).
Hospital Risk‐Standardized Mortality Rates
The mean unadjusted hospital 30‐day mortality rate was 8.6% and ranged from 0% to 100% (Figure 2a). Risk‐standardized mortality rates varied across hospitals (Figure 2b). The mean risk‐standardized mortality rate was 8.6% and ranged from 5.9% to 13.5%. The odds of mortality at a hospital 1 standard deviation above average was 1.20 times that of a hospital 1 standard deviation below average.
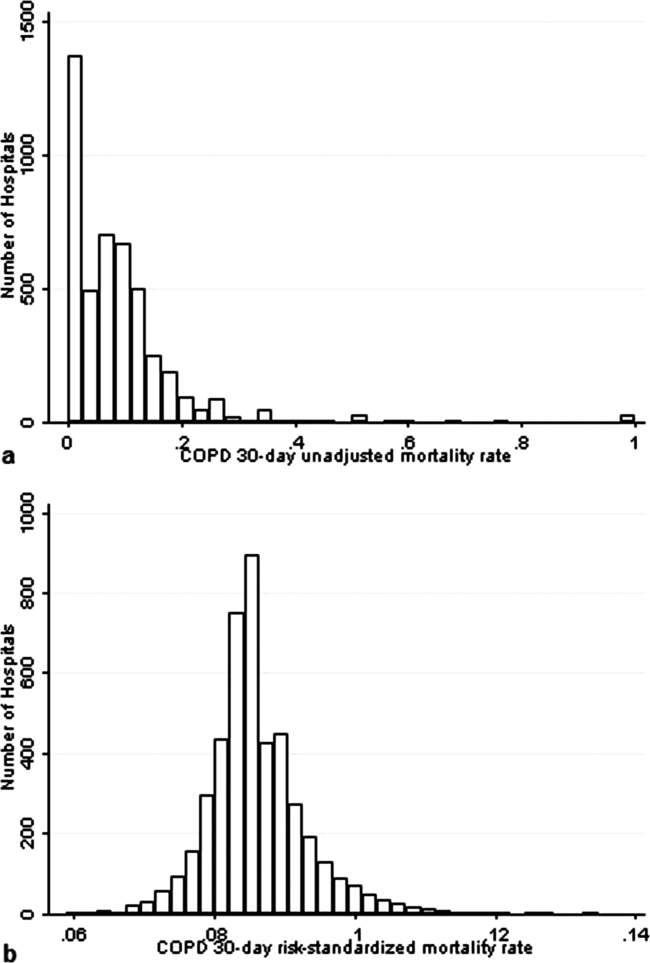
DISCUSSION
We present a hospital‐level risk‐standardized mortality measure for patients admitted with COPD based on administrative claims data that are intended for public reporting and that have achieved endorsement by the National Quality Forum, a voluntary consensus standards‐setting organization. Across more than 4500 US hospitals, the mean 30‐day risk‐standardized mortality rate in 2008 was 8.6%, and we observed considerable variation across institutions, despite adjustment for case mix, suggesting that improvement by lower‐performing institutions may be an achievable goal.
Although improving the delivery of evidence‐based care processes and outcomes of patients with acute myocardial infarction, heart failure, and pneumonia has been the focus of national quality improvement efforts for more than a decade, COPD has largely been overlooked.[23] Within this context, this analysis represents the first attempt to systematically measure, at the hospital level, 30‐day all‐cause mortality for patients admitted to US hospitals for exacerbation of COPD. The model we have developed and validated is intended to be used to compare the performance of hospitals while controlling for differences in the pretreatment risk of mortality of patients and accounting for the clustering of patients within hospitals, and will facilitate surveillance of hospital‐level risk‐adjusted outcomes over time.
In contrast to process‐based measures of quality, such as the percentage of patients with pneumonia who receive appropriate antibiotic therapy, performance measures based on patient outcomes provide a more comprehensive view of care and are more consistent with patients' goals.[24] Additionally, it is well established that hospital performance on individual and composite process measures explains only a small amount of the observed variation in patient outcomes between institutions.[25] In this regard, outcome measures incorporate important, but difficult to measure aspects of care, such as diagnostic accuracy and timing, communication and teamwork, the recognition and response to complications, care coordination at the time of transfers between levels of care, and care settings. Nevertheless, when used for making inferences about the quality of hospital care, individual measures such as the risk‐standardized hospital mortality rate should be interpreted in the context of other performance measures, including readmission, patient experience, and costs of care.
A number of prior investigators have described the outcomes of care for patients hospitalized with exacerbations of COPD, including identifying risk factors for mortality. Patil et al. carried out an analysis of the 1996 Nationwide Inpatient Sample and described an overall in‐hospital mortality rate of 2.5% among patients with COPD, and reported that a multivariable model containing sociodemographic characteristics about the patient and comorbidities had an area under the ROC curve of 0.70.[3] In contrast, this hospital‐level measure includes patients with a principal diagnosis of respiratory failure and focuses on 30‐day rather than inpatient mortality, accounting for the nearly 3‐fold higher mortality rate we observed. In a more recent study that used clinical from a large multistate database, Tabak et al. developed a prediction model for inpatient mortality for patients with COPD that contained only 4 factors: age, blood urea nitrogen, mental status, and pulse, and achieved an area under the ROC curve of 0.72.[4] The simplicity of such a model and its reliance on clinical measurements makes it particularly well suited for bedside application by clinicians, but less valuable for large‐scale public reporting programs that rely on administrative data. In the only other study identified that focused on the assessment of hospital mortality rates, Agabiti et al. analyzed the outcomes of 12,756 patients hospitalized for exacerbations of COPD, using similar ICD‐9‐CM diagnostic criteria as in this study, at 21 hospitals in Rome, Italy.[26] They reported an average crude 30‐day mortality rate of 3.8% among a group of 5 benchmark hospitals and an average mortality of 7.5% (range, 5.2%17.2%) among the remaining institutions.
To put the variation we observed in mortality rates into a broader context, the relative difference in the risk‐standardized hospital mortality rates across the 10th to 90th percentiles of hospital performance was 25% for acute myocardial infarction and 39% for heart failure, whereas rates varied 30% for COPD, from 7.6% to 9.9%.[27] Model discrimination in COPD (C statistic, 0.72) was also similar to that reported for models used for public reporting of hospital mortality in acute myocardial infarction (C statistic, 0.71) and pneumonia (C statistic, 0.72).
This study has a number of important strengths. First, the model was developed from a large sample of recent Medicare claims, achieved good discrimination, and was validated in samples not limited to Medicare beneficiaries. Second, by including patients with a principal diagnosis of COPD, as well as those with a principal diagnosis of acute respiratory failure when accompanied by a secondary diagnosis of COPD with acute exacerbation, this model can be used to assess hospital performance across the full spectrum of disease severity. This broad set of ICD‐9‐CM codes used to define the cohort also ensures that efforts to measure hospital performance will be less influenced by differences in documentation and coding practices across hospitals relating to the diagnosis or sequencing of acute respiratory failure diagnoses. Moreover, the inclusion of patients with respiratory failure is important because these patients have the greatest risk of mortality, and are those in whom efforts to improve the quality and safety of care may have the greatest impact. Third, rather than relying solely on information documented during the index admission, we used ambulatory and inpatient claims from the full year prior to the index admission to identify comorbidities and to distinguish them from potential complications of care. Finally, we did not include factors such as hospital characteristics (eg, number of beds, teaching status) in the model. Although they might have improved overall predictive ability, the goal of the hospital mortality measure is to enable comparisons of mortality rates among hospitals while controlling for differences in patient characteristics. To the extent that factors such as size or teaching status might be independently associated with hospital outcomes, it would be inappropriate to adjust away their effects, because mortality risk should not be influenced by hospital characteristics other than through their effects on quality.
These results should be viewed in light of several limitations. First, we used ICD‐9‐CM codes derived from claims files to define the patient populations included in the measure rather than collecting clinical or physiologic information prospectively or through manual review of medical records, such as the forced expiratory volume in 1 second or whether the patient required long‐term oxygen therapy. Nevertheless, we included a broad set of potential diagnosis codes to capture the full spectrum of COPD exacerbations and to minimize differences in coding across hospitals. Second, because the risk‐adjustment included diagnoses coded in the year prior to the index admission, it is potentially subject to bias due to regional differences in medical care utilization that are not driven by underlying differences in patient illness.[28] Third, using administrative claims data, we observed some paradoxical associations in the model that are difficult to explain on clinical grounds, such as a protective effect of substance and alcohol abuse or prior episodes of respiratory failure. Fourth, although we excluded patients from the analysis who were enrolled in hospice prior to, or on the day of, the index admission, we did not exclude those who choose to withdraw support, transition to comfort measures only, or enrolled in hospice care during a hospitalization. We do not seek to penalize hospitals for being sensitive to the preferences of patients at the end of life. At the same time, it is equally important that the measure is capable of detecting the outcomes of suboptimal care that may in some instances lead a patient or their family to withdraw support or choose hospice. Finally, we did not have the opportunity to validate the model against a clinical registry of patients with COPD, because such data do not currently exist. Nevertheless, the use of claims as a surrogate for chart data for risk adjustment has been validated for several conditions, including acute myocardial infarction, heart failure, and pneumonia.[29, 30]
CONCLUSIONS
Risk‐standardized 30‐day mortality rates for Medicare beneficiaries with COPD vary across hospitals in the US. Calculating and reporting hospital outcomes using validated performance measures may catalyze quality improvement activities and lead to better outcomes. Additional research would be helpful to confirm that hospitals with lower mortality rates achieve care that meets the goals of patients and their families better than at hospitals with higher mortality rates.
Acknowledgment
The authors thank the following members of the technical expert panel: Darlene Bainbridge, RN, MS, NHA, CPHQ, CPHRM, President/CEO, Darlene D. Bainbridge & Associates, Inc.; Robert A. Balk, MD, Director of Pulmonary and Critical Care Medicine, Rush University Medical Center; Dale Bratzler, DO, MPH, President and CEO, Oklahoma Foundation for Medical Quality; Scott Cerreta, RRT, Director of Education, COPD Foundation; Gerard J. Criner, MD, Director of Temple Lung Center and Divisions of Pulmonary and Critical Care Medicine, Temple University; Guy D'Andrea, MBA, President, Discern Consulting; Jonathan Fine, MD, Director of Pulmonary Fellowship, Research and Medical Education, Norwalk Hospital; David Hopkins, MS, PhD, Senior Advisor, Pacific Business Group on Health; Fred Martin Jacobs, MD, JD, FACP, FCCP, FCLM, Executive Vice President and Director, Saint Barnabas Quality Institute; Natalie Napolitano, MPH, RRT‐NPS, Respiratory Therapist, Inova Fairfax Hospital; Russell Robbins, MD, MBA, Principal and Senior Clinical Consultant, Mercer. In addition, the authors acknowledge and thank Angela Merrill, Sandi Nelson, Marian Wrobel, and Eric Schone from Mathematica Policy Research, Inc., Sharon‐Lise T. Normand from Harvard Medical School, and Lein Han and Michael Rapp at The Centers for Medicare & Medicaid Services for their contributions to this work.
Disclosures
Peter K. Lindenauer, MD, MSc, is the guarantor of this article, taking responsibility for the integrity of the work as a whole, from inception to published article, and takes responsibility for the content of the manuscript, including the data and data analysis. All authors have made substantial contributions to the conception and design, or acquisition of data, or analysis and interpretation of data; have drafted the submitted article or revised it critically for important intellectual content; and have provided final approval of the version to be published. Preparation of this manuscript was completed under Contract Number: HHSM‐5002008‐0025I/HHSM‐500‐T0001, Modification No. 000007, Option Year 2 Measure Instrument Development and Support (MIDS). Sponsors did not contribute to the development of the research or manuscript. Dr. Au reports being an unpaid research consultant for Bosch Inc. He receives research funding from the NIH, Department of Veterans Affairs, AHRQ, and Gilead Sciences. The views of the this manuscript represent the authors and do not necessarily represent those of the Department of Veterans Affairs. Drs. Drye and Bernheim report receiving contract funding from CMS to develop and maintain quality measures.
- FASTSTATS—chronic lower respiratory disease. Available at: http://www.cdc.gov/nchs/fastats/copd.htm. Accessed September 18, 2010.
- National Heart, Lung and Blood Institute. Morbidity and mortality chartbook. Available at: http://www.nhlbi.nih.gov/resources/docs/cht‐book.htm. Accessed April 27, 2010.
- In‐hospital mortality following acute exacerbations of chronic obstructive pulmonary disease. Arch Intern Med. 2003;163(10):1180–1186. , , , .
- Mortality and need for mechanical ventilation in acute exacerbations of chronic obstructive pulmonary disease: development and validation of a simple risk score. Arch Intern Med. 2009;169(17):1595–1602. , , , , .
- Quality of care for patients hospitalized for acute exacerbations of chronic obstructive pulmonary disease. Ann Intern Med. 2006;144(12):894–903. , , , , , .
- Use of beta blockers and the risk of death in hospitalised patients with acute exacerbations of COPD. Thorax. 2008;63(4):301–305. , , , , .
- HCUP facts and figures: statistics on hospital‐based care in the United States, 2007. 2009. Available at: http://www.hcup‐us.ahrq.gov/reports.jsp. Accessed August 6, 2012. , , , , .
- Predictors of long‐term survival in elderly patients hospitalized for acute exacerbations of chronic obstructive pulmonary disease. Respirology. 2008;13(6):851–855. , .
- The impact on risk‐factor analysis of different mortality outcomes in COPD patients. Eur Respir J 2008;32(3):629–636. , , , , , .
- Clinical audit indicators of outcome following admission to hospital with acute exacerbation of chronic obstructive pulmonary disease. Thorax. 2002;57(2):137–141. , , , , , .
- The quality of obstructive lung disease care for adults in the United States as measured by adherence to recommended processes. Chest. 2006;130(6):1844–1850. , , , et al.
- Management of acute exacerbations of chronic obstructive pulmonary disease in the elderly: physician practices in the community hospital setting. J Okla State Med Assoc. 2004;97(6):227–232. , , , , , .
- Leadership by Example: Coordinating Government Roles in Improving Health Care Quality. Washington, DC: National Academies Press; 2002. , , .
- Patient Protection and Affordable Care Act [H.R. 3590], Pub. L. No. 111–148, §2702, 124 Stat. 119, 318–319 (March 23, 2010). Available at: http://www.gpo.gov/fdsys/pkg/PLAW‐111publ148/html/PLAW‐111publ148.htm. Accessed July 15, 2012.
- National Quality Forum. NQF Endorses Additional Pulmonary Measure. 2013. Available at: http://www.qualityforum.org/News_And_Resources/Press_Releases/2013/NQF_Endorses_Additional_Pulmonary_Measure.aspx. Accessed January 11, 2013.
- National Quality Forum. National voluntary consensus standards for patient outcomes: a consensus report. Washington, DC: National Quality Forum; 2011.
- The Measures Management System. The Centers for Medicare and Medicaid Services. Available at: http://www.cms.gov/Medicare/Quality‐Initiatives‐Patient‐Assessment‐Instruments/MMS/index.html?redirect=/MMS/. Accessed August 6, 2012.
- Standards for statistical models used for public reporting of health outcomes: an American Heart Association Scientific Statement from the Quality of Care and Outcomes Research Interdisciplinary Writing Group: cosponsored by the Council on Epidemiology and Prevention and the Stroke Council. Endorsed by the American College of Cardiology Foundation. Circulation. 2006;113(3):456–462. , , , et al.
- Comparison of hospital risk‐standardized mortality rates calculated by using in‐hospital and 30‐day models: an observational study with implications for hospital profiling. Ann Intern Med. 2012;156(1 pt 1):19–26. , , , et al.
- Diagnostic cost group hierarchical condition category models for Medicare risk adjustment. Report prepared for the Health Care Financing Administration. Health Economics Research, Inc.; 2000. Available at: http://www.cms.gov/Research‐Statistics‐Data‐and‐Systems/Statistics‐Trends‐and‐Reports/Reports/downloads/pope_2000_2.pdf. Accessed November 7, 2009. , , , et al.
- Statistical and clinical aspects of hospital outcomes profiling. Stat Sci. 2007;22(2):206–226. , .
- Using full probability models to compute probabilities of actual interest to decision makers. Int J Technol Assess Health Care. 2001;17(1):17–26. , .
- COPD performance measures: missing opportunities for improving care. Chest. 2010;137(5):1181–1189. , , .
- Measuring Performance For Treating Heart Attacks And Heart Failure: The Case For Outcomes Measurement. Health Aff. 2007;26(1):75–85. , , , , .
- Hospital quality for acute myocardial infarction: correlation among process measures and relationship with short‐term mortality. JAMA. 2006;296(1):72–78. , , , et al.
- Profiling hospital performance to monitor the quality of care: the case of COPD. Eur Respir J. 2010;35(5):1031–1038. , , , et al.
- Patterns of hospital performance in acute myocardial infarction and heart failure 30‐day mortality and readmission. Circ Cardiovasc Qual Outcomes. 2009;2(5):407–413. , , , et al.
- Geographic variation in diagnosis frequency and risk of death among Medicare beneficiaries. JAMA. 2011;305(11):1113–1118. , , , , .
- An administrative claims model for profiling hospital 30‐day mortality rates for pneumonia patients. PLoS ONE. 2011;6(4):e17401. , , , et al.
- An Administrative Claims Model Suitable for Profiling Hospital Performance Based on 30‐Day Mortality Rates Among Patients With Heart Failure. Circulation. 2006;113(13):1693–1701. , , , et al.
- FASTSTATS—chronic lower respiratory disease. Available at: http://www.cdc.gov/nchs/fastats/copd.htm. Accessed September 18, 2010.
- National Heart, Lung and Blood Institute. Morbidity and mortality chartbook. Available at: http://www.nhlbi.nih.gov/resources/docs/cht‐book.htm. Accessed April 27, 2010.
- In‐hospital mortality following acute exacerbations of chronic obstructive pulmonary disease. Arch Intern Med. 2003;163(10):1180–1186. , , , .
- Mortality and need for mechanical ventilation in acute exacerbations of chronic obstructive pulmonary disease: development and validation of a simple risk score. Arch Intern Med. 2009;169(17):1595–1602. , , , , .
- Quality of care for patients hospitalized for acute exacerbations of chronic obstructive pulmonary disease. Ann Intern Med. 2006;144(12):894–903. , , , , , .
- Use of beta blockers and the risk of death in hospitalised patients with acute exacerbations of COPD. Thorax. 2008;63(4):301–305. , , , , .
- HCUP facts and figures: statistics on hospital‐based care in the United States, 2007. 2009. Available at: http://www.hcup‐us.ahrq.gov/reports.jsp. Accessed August 6, 2012. , , , , .
- Predictors of long‐term survival in elderly patients hospitalized for acute exacerbations of chronic obstructive pulmonary disease. Respirology. 2008;13(6):851–855. , .
- The impact on risk‐factor analysis of different mortality outcomes in COPD patients. Eur Respir J 2008;32(3):629–636. , , , , , .
- Clinical audit indicators of outcome following admission to hospital with acute exacerbation of chronic obstructive pulmonary disease. Thorax. 2002;57(2):137–141. , , , , , .
- The quality of obstructive lung disease care for adults in the United States as measured by adherence to recommended processes. Chest. 2006;130(6):1844–1850. , , , et al.
- Management of acute exacerbations of chronic obstructive pulmonary disease in the elderly: physician practices in the community hospital setting. J Okla State Med Assoc. 2004;97(6):227–232. , , , , , .
- Leadership by Example: Coordinating Government Roles in Improving Health Care Quality. Washington, DC: National Academies Press; 2002. , , .
- Patient Protection and Affordable Care Act [H.R. 3590], Pub. L. No. 111–148, §2702, 124 Stat. 119, 318–319 (March 23, 2010). Available at: http://www.gpo.gov/fdsys/pkg/PLAW‐111publ148/html/PLAW‐111publ148.htm. Accessed July 15, 2012.
- National Quality Forum. NQF Endorses Additional Pulmonary Measure. 2013. Available at: http://www.qualityforum.org/News_And_Resources/Press_Releases/2013/NQF_Endorses_Additional_Pulmonary_Measure.aspx. Accessed January 11, 2013.
- National Quality Forum. National voluntary consensus standards for patient outcomes: a consensus report. Washington, DC: National Quality Forum; 2011.
- The Measures Management System. The Centers for Medicare and Medicaid Services. Available at: http://www.cms.gov/Medicare/Quality‐Initiatives‐Patient‐Assessment‐Instruments/MMS/index.html?redirect=/MMS/. Accessed August 6, 2012.
- Standards for statistical models used for public reporting of health outcomes: an American Heart Association Scientific Statement from the Quality of Care and Outcomes Research Interdisciplinary Writing Group: cosponsored by the Council on Epidemiology and Prevention and the Stroke Council. Endorsed by the American College of Cardiology Foundation. Circulation. 2006;113(3):456–462. , , , et al.
- Comparison of hospital risk‐standardized mortality rates calculated by using in‐hospital and 30‐day models: an observational study with implications for hospital profiling. Ann Intern Med. 2012;156(1 pt 1):19–26. , , , et al.
- Diagnostic cost group hierarchical condition category models for Medicare risk adjustment. Report prepared for the Health Care Financing Administration. Health Economics Research, Inc.; 2000. Available at: http://www.cms.gov/Research‐Statistics‐Data‐and‐Systems/Statistics‐Trends‐and‐Reports/Reports/downloads/pope_2000_2.pdf. Accessed November 7, 2009. , , , et al.
- Statistical and clinical aspects of hospital outcomes profiling. Stat Sci. 2007;22(2):206–226. , .
- Using full probability models to compute probabilities of actual interest to decision makers. Int J Technol Assess Health Care. 2001;17(1):17–26. , .
- COPD performance measures: missing opportunities for improving care. Chest. 2010;137(5):1181–1189. , , .
- Measuring Performance For Treating Heart Attacks And Heart Failure: The Case For Outcomes Measurement. Health Aff. 2007;26(1):75–85. , , , , .
- Hospital quality for acute myocardial infarction: correlation among process measures and relationship with short‐term mortality. JAMA. 2006;296(1):72–78. , , , et al.
- Profiling hospital performance to monitor the quality of care: the case of COPD. Eur Respir J. 2010;35(5):1031–1038. , , , et al.
- Patterns of hospital performance in acute myocardial infarction and heart failure 30‐day mortality and readmission. Circ Cardiovasc Qual Outcomes. 2009;2(5):407–413. , , , et al.
- Geographic variation in diagnosis frequency and risk of death among Medicare beneficiaries. JAMA. 2011;305(11):1113–1118. , , , , .
- An administrative claims model for profiling hospital 30‐day mortality rates for pneumonia patients. PLoS ONE. 2011;6(4):e17401. , , , et al.
- An Administrative Claims Model Suitable for Profiling Hospital Performance Based on 30‐Day Mortality Rates Among Patients With Heart Failure. Circulation. 2006;113(13):1693–1701. , , , et al.
Copyright © 2013 Society of Hospital Medicine