User login
Pharmacotherapy for Tobacco Use and COPD
Up to one‐third of the 700,000 patients admitted annually for an exacerbation of chronic obstructive pulmonary disease (COPD) continue to smoke tobacco.[1, 2] Smokers with COPD are at high risk for poor health outcomes directly attributable to tobacco‐related conditions, including progression of lung disease and cardiovascular diseases.[3, 4, 5] Treatment for tobacco addiction is the most essential intervention for these patients.
Hospital admission has been suggested as an opportune time for the initiation of smoking cessation.[6] Hospitalized patients are already in a smoke‐free environment, and have access to physicians, nurses, and pharmacists who can prescribe medications for support.[7] Documenting smoking status and offering smoking cessation treatment during and after discharge are quality metrics required by the Joint Commission, and recommended by the National Quality Forum.[8, 9] Hospitals have made significant efforts to comply with these requirements.[10]
Limited data exist regarding the effectiveness and utilization of treatments known to reduce cigarette use among COPD patients in nontrial environments. Prescribing patterns of medications for smoking cessation in the real world following admission for COPD are not well studied. We sought to examine the utilization of inpatient brief tobacco counseling and postdischarge pharmacotherapy following discharge for exacerbation of COPD, as well as to (1) examine the association of postdischarge pharmacotherapy with self‐reported smoking cessation at 6 to 12 months and (2) assess differences in effectiveness between cessation medications prescribed.
METHODS
We conducted a cohort study of current smokers discharged following a COPD exacerbation within the Veterans Affairs (VA) Veterans Integrated Service Network (VISN)‐20. This study was approved by the VA Puget Sound Health Care System Institutional Review Board (#00461).
We utilized clinical information from the VISN‐20 data warehouse that collects data using the VA electronic medical record, including demographics, prescription medications, hospital admissions, hospital and outpatient diagnoses, and dates of death, and is commonly used for research. In addition, we utilized health factors, coded electronic entries describing patient health behaviors that are entered by nursing staff at the time of a patient encounter, and the text of chart notes that were available for electronic query.
Study Cohort
We identified all smokers aged 40 years hospitalized between 2005 and 2012 with either a primary discharge diagnosis of COPD based on International Classification of Diseases, 9th Revision codes (491, 492, 493.2, and 496) or an admission diagnosis from the text of the admit notes indicating an exacerbation of COPD. We limited to patients aged 40 years to improve the specificity of the diagnosis of COPD, and we selected the first hospitalization that met inclusion criteria. We excluded subjects who died within 6 months of discharge (Figure 1).
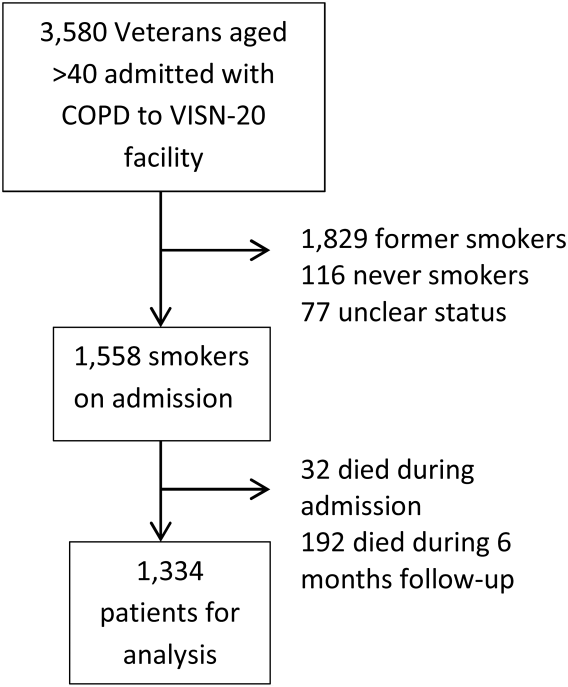
To establish tobacco status, we built on previously developed and validated methodology,[11] and performed truncated natural language processing using phrases in the medical record that reflected patients' tobacco status, querying all notes from the day of admission up to 6 months prior. If no tobacco status was indicated in the notes, we identified the status encoded by the most recent health factor. We manually examined the results of the natural language processing and the determination of health factors to confirm the tobacco status. Manual review was undertaken by 1 of 2 trained study personnel. In the case of an ambiguous or contradictory status, an additional team member reviewed the information to attempt to make a determination. If no determination could be made, the record was coded to unknown. This method allowed us to identify a baseline status for all but 77 of the 3580 patients admitted for COPD.
Outcome and Exposure
The outcome was tobacco status at 6 to 12 months after discharge. Using the same methods developed for identification of baseline smoking status, we obtained smoking status for each subject up to 12 months postdischarge. If multiple notes and encounters were available indicating smoking status, we chose the latest within 12 months of discharge. Subjects lacking a follow‐up status were presumed to be smokers, a common assumption.[12] The 6 to 12month time horizon was chosen as these are the most common time points used to examine a sustained change in tobacco status,[13, 14, 15] and allowed for adequate time for treatment and clinical follow‐up.
Our primary exposure was any smoking cessation medication or combination dispensed within 90 days of discharge. This time horizon for treatment was chosen due to recent studies indicating this is a meaningful period for postdischarge treatment.[14] We assessed the use of nicotine patch, short‐acting nicotine, varenicline, buproprion, or any combination. Accurate data on the prescription and dispensing of these medications were available from the VA pharmacy record. Secondary exposure was the choice of medication dispensed among treated patients. We assessed additional exposures including receipt of cessation medications within 48 hours of discharge, treatment in the year prior to admission, and predischarge counseling. Predischarge counseling was determined as having occurred if nurses documented that they completed a discharge process focused on smoking cessation. Referral to a quit line is part of this process; however, due to the confidential nature of these interactions, generally low use of this service, and lack of linkage to the VA electronic health record, it was not considered in the analysis.
Confounders
Potential confounders were assessed in the year prior to admission up to discharge from the index hospitalization, with the use of mechanical or noninvasive ventilation assessed during the hospitalization. We adjusted for variables chosen a priori for their known or expected association with smoking cessation including demographics, Charlson Comorbidity Index,[16] markers of COPD severity (need for invasive or noninvasive mechanical ventilation during index hospitalization, use of oral steroids, long‐acting inhaled bronchodilators, and/or canister count of short‐acting bronchodilators in the year prior to admission), history of drug or alcohol abuse, homelessness, depression, psychosis, post‐traumatic stress disorder, lung cancer, coronary artery disease, and under‐ or overweight status. Nurse‐based counseling prior to discharge was included as a variable for adjustment for our primary and secondary predictors to assess the influence of pharmacotherapy specifically. Due to 3.1% missingness in body mass index, multiple imputation with chained equations was used to impute missing values, with 10 imputations performed. The imputation was performed using a linear regression model containing all variables included in the final model, grouped by facility.
Statistical Analysis
All analyses were performed using Stata 13 (StataCorp, College Station, TX) software. 2 tests and t tests were used to assess for unadjusted bivariate associations. Using the pooled imputed datasets, we performed multivariable logistic regression to compare odds ratios for a change in smoking status, adjusting the estimates of coefficients and standard errors by applying combination rules to the 10 completed‐data estimates.[17] We analyzed our primary and secondary predictors, adjusting for the confounders chosen a priori, clustered by facility with robust standard errors. An level of <0.05 was considered significant.
Sensitivity Analysis
We assumed that subjects missing a follow‐up status were ongoing smokers. However, given the high mortality rate observed in our cohort, we were concerned that some subjects lacking a follow‐up status may have died, missing the opportunity to have a quit attempt recorded. Therefore, we performed sensitivity analysis excluding subjects who died during the 6 to 12 months of follow‐up, repeating the imputation and analysis as described above. In addition, due to concern for indication bias in the choice of medication used for our secondary analysis, we performed propensity score matching for treatment with each medication in comparison to nicotine patch, using the teffects command, with 3 nearest neighbor matches. We included additional comorbidities in the propensity score matching.[18]
RESULTS
Among these 1334 subjects at 6 to 12 months of follow‐up, 63.7% reported ongoing smoking, 19.8% of patients reported quitting, and 17.5% of patients had no reported status and were presumed to be smokers. Four hundred fifty (33.7%) patients were dispensed a smoking cessation medication within 90 days of discharge. Patients who were dispensed medications were younger and more likely to be female. Nearly all patients who received medications also received documented predischarge counseling (94.6%), as did the majority of patients who did not receive medications (83.8%) (Table 1).
Variable | No Medication Dispensed, n = 884, No. (%) | Medication Dispensed, n = 450, No. (%) | P Value |
---|---|---|---|
| |||
Not smoking at 612 months | 179 (20.2) | 85 (18.9) | 0.56 |
Brief counseling at discharge | 742 (83.8%) | 424 (94.6%) | <0.001* |
Age | 64.49.13 (4094) | 61.07.97 (4185) | <0.001* |
Male | 852 (96.3) | 423 (94.0) | 0.05* |
Race | 0.12 | ||
White | 744 (84.2) | 377 (83.8) | |
Black | 41 (4.6) | 12 (2.7) | |
Other/unknown | 99 (11.1) | 61 (13.6) | |
BMI | 28.09.5 (12.669.0) | 28.910.8 (14.860.0) | 0.15 |
Homeless | 68 (7.7) | 36 (8.0) | 0.84 |
Psychiatric conditions/substance abuse | |||
History of alcohol abuse | 205 (23.2) | 106 (23.6) | 0.88 |
History of drug abuse | 110 (12.4) | 72 (16.0) | 0.07 |
Depression | 39 (4.4) | 29 (6.4) | 0.11 |
Psychosis | 201 (22.7) | 88 (19.6) | 0.18 |
PTSD | 146 (16.5) | 88 (19.6) | 0.17 |
Comorbidities | |||
Coronary artery disease | 254 (28.7) | 110 (24.4) | 0.10 |
Cerebrovascular accident | 80 (9.0) | 28 (2.2) | 0.86 |
Obstructive sleep apnea | 42 (4.8) | 23 (5.1) | 0.77 |
Lung cancer | 21 (2.4) | 10 (2.2) | 0.86 |
Charlson Comorbidity Index | 2.251.93 (014) | 2.111.76 (010) | 0.49 |
Markers of COPD severity | |||
Mechanical ventilation during admission | 28 (3.2) | 14 (3.1) | 0.96 |
NIPPV during admission | 97 (11.0) | 51 (11.3) | 0.84 |
Oral steroids prescribed in the past year | 334 (37.8) | 154 (34.2) | 0.20 |
Treatment with tiotropium in the past year | 97 (11.0) | 55 (12.2) | 0.50 |
Treatment with LABA in the past year | 264 (29.9) | 155 (34.4) | 0.09 |
Canisters of SABA used in past year | 6.639.8, (084) | 7.469.63 (045) | 0.14 |
Canisters of ipratropium used in past year | 6.458.81 (054) | 6.869.08 (064) | 0.42 |
Died during 612 months of follow‐up | 78 (8.8) | 28 (6.6) | 0.10 |
Of patients dispensed a study medication, 246 (18.4% of patients, 54.7% of all medications dispensed) were dispensed medications within 48 hours of discharge (Table 2). Of the patients dispensed medication, the majority received nicotine patches alone (Table 3), and 18.9% of patients received combination therapy, with the majority receiving nicotine patch and short‐acting nicotine replacement therapy (NRT) or patch and buproprion. A significant number of patients were prescribed medications within 90 days of discharge, but did not have them dispensed within that timeframe (n = 224, 16.8%).
Medication Dispensed | No. (%) | % Quit (Unadjusted) | OR (95% CI) | P Value |
---|---|---|---|---|
| ||||
No medications dispensed | 884 (66.3) | 20.2 | Referent | |
Any medication from | ||||
Discharge to 90 days | 450 (33.7) | 18.9 | 0.88 (0.741.04) | 0.137 |
Within 48 hours of discharge | 246 (18.4) | 18.3 | 0.87 (0.661.14) | 0.317 |
Treated in the year prior to admission | 221 (16.6) | 19.6 | Referent | |
Treated in the year prior to admission + 090 days postdischarge | 152 (11.4) | 18.4 | 0.95 (0.791.13) | 0.534 |
No nurse‐provided counseling prior to discharge | 169 (12.7) | 20.5 | Referent | |
Nurse‐provided counseling prior to discharge | 1,165 (87.3) | 19.5 | 0.95 (0.661.36) | 0.774 |
Medication Dispensed | No. (%) | % Quit (Unadjusted) | OR (95% CI) | P Value |
---|---|---|---|---|
| ||||
Nicotine patch | 242 (53.8) | 18.6 | Referent | |
Monotherapy with | ||||
Varenicline | 36 (8.0) | 30.6 | 2.44 (1.484.05) | 0.001 |
Short‐acting NRT | 34 (7.6) | 11.8 | 0.66 (0.510.85) | 0.001 |
Buproprion | 55 (12.2) | 21.8 | 1.05 (0.671.62) | 0.843 |
Combination therapy | 85 (18.9) | 15.7 | 0.94 (0.711.24) | 0.645 |
Association of Treatment With Study Medications and Quitting Smoking
In adjusted analyses, the odds of quitting smoking at 6 to 12 months were not greater among patients who were dispensed a study medication within 90 days of discharge (odds ratio [OR]: 0.88, 95% confidence interval [CI]: 0.74‐1.04). We found no association between counseling provided at discharge and smoking cessation (OR: 0.95, 95% CI: 0.0.66‐1.), adjusted for the receipt of medications. There was no difference in quit rate between patients dispensed medication within 48 hours of discharge, or between patients treated in the year prior to admission and again postdischarge (Table 2).
We then assessed differences in effectiveness between specific medications among the 450 patients who were dispensed medications. Using nicotine patch alone as the referent group, patients treated with varenicline demonstrated greater odds of smoking cessation (OR: 2.44, 95% CI: 1.48‐4.05). Patients treated with short‐acting NRT alone were less likely to report smoking cessation (OR: 0.66, 95% CI: 0.51‐0.85). Patients treated with buproprion or combination therapy were no more likely to report cessation (Table 3). When sensitivity analysis was performed using propensity score matching with additional variables included, there were no significant differences in the observed associations.
Our overall mortality rate observed at 1 year was 19.5%, nearly identical to previous cohort studies of patients admitted for COPD.[19, 20] Because of the possibility of behavioral differences on the part of patients and physicians regarding subjects with a limited life expectancy, we performed sensitivity analysis limited to the patients who survived to at least 12 months of follow‐up. One hundred six patients (7.9%) died during 6 to 12 months of follow‐up. There was no change in inference for our primary exposure (OR: 0.95, 95% CI: 0.79‐1.14) or any of the secondary exposures examined.
DISCUSSION
In this observational study, postdischarge pharmacotherapy within 90 days of discharge was provided to a minority of high‐risk smokers admitted for COPD, and was not associated with smoking cessation at 6 to 12 months. In comparison to nicotine patch alone, varenicline was associated with a higher odds of cessation, with decreased odds of cessation among patients treated with short‐acting NRT alone. The overall quit rate was significant at 19.8%, and is consistent with annual quit rates observed among patients with COPD in other settings,[21, 22] but is far lower than quit rates observed after admission for acute myocardial infarction.[23, 24, 25] Although the proportion of patients treated at the time of discharge or within 90 days was low, our findings are in keeping with previous studies, which demonstrated low rates of pharmacologic treatment following hospitalization, averaging 14%.[26] Treatment for tobacco use is likely underutilized for this group of high‐risk smokers. However, a significant proportion of patients who were prescribed medications in the postdischarge period did not have medications filled. This likely reflects both the rapid changes in motivation that characterize quit attempts,[27] as well as efforts on the part of primary care physicians to make these medications available to facilitate future quit attempts.
There are several possible explanations for the findings in our study. Pharmaceutical therapies were not provided at random. The provision of pharmacotherapy and the ultimate success of a quit attempt reflects a complex interaction of patient beliefs concerning medications, level of addiction and motivation, physician behavior and knowledge, and organizational factors. Organizational factors such as the structure of electronic discharge orders and the availability of decision support materials may influence a physician's likelihood of prescribing medications, the choice of medication prescribed, and therefore the adequacy of control of withdrawal symptoms. NRT is often under dosed to control ongoing symptoms,[28] and needs to be adjusted until relief is obtained, providing an additional barrier to effectiveness during the transition out of the hospital. Because most smokers with COPD are highly addicted to nicotine,[29] high‐dose NRT, combination therapy, or varenicline would be necessary to adequately control symptoms.[30] However, a significant minority of patients received short‐acting NRT alone.
Despite a high observed efficacy in recent trials,[31, 32] few subjects in our study received varenicline. This may be related to both secular trends and administrative barriers to the use of varenicline in the VA system. Use of this medication was limited among patients with psychiatric disorders due to safety concerns. These concerns have since been largely disproven, but may have limited access to this medication.[33, 34, 35] Although we adjusted for a history of mental illness, patients who received varenicline may have had more past quit attempts and less active mental illness, which may be associated with improved cessation rates. Despite the high prevalence of mental illness we observed, this is typical of the population of smokers, with studies indicating nearly one‐third of smokers overall suffer from mental illness.[36]
Although the majority of our patients received a brief, nurse‐based counseling intervention, there is considerable concern about the overall effectiveness of a single predischarge interaction to produce sustained smoking cessation among highly addicted smokers.[37, 38, 39, 40] The Joint Commission has recently restructured the requirements for smoking cessation treatment for hospitalized patients, and it is now up to hospitals to implement treatment mechanisms that not only meet the national requirements, but also provide a meaningful clinical effect. Though the optimum treatment for hospitalized smokers with COPD is unknown, previous positive studies of smoking cessation among hospitalized patients underscore the need for a higher‐intensity counseling intervention that begins during hospitalization and continues after discharge.[13, 41] Cessation counseling services including tobacco cessation groups and quit lines are available through the VA; however, the use of these services is typically low and requires the patient to enroll independently after discharge, an additional barrier. The lack of association between medications and smoking cessation found in our study could reflect poor effectiveness of medications in the absence of a systematic counseling intervention. Alternatively, the association may be explained that patients who were more highly addicted and perhaps less motivated to quit received tobacco cessation medications more often, but were also less likely to stop tobacco use, a form of indication bias.
Our study has several limitations. We do not have addiction or motivation levels for a cessation attempt, a potential unmeasured confounder. Although predictive of quit attempts, motivation factors are less predictive of cessation maintenance, and may therefore have an unclear effect on our outcome.[42, 43] Our outcome was gathered as part of routine clinical care, which may have introduced bias if patients over‐reported cessation because of social desirability. In healthcare settings, however, this form of assessing smoking status is generally valid.[44] Exposure to counseling or medications obtained outside of the VA system would not have been captured. Given the financial incentive, we believe it is unlikely that many patients admitted to a VA medical center obtained medications elsewhere.[45] The diagnosis of COPD was made administratively. However, all subjects were admitted for an exacerbation, which is associated with more severe COPD by Global Initiative for Obstructive Lung Disease (GOLD) stage.[46] Patients with more severe COPD are often excluded from studies of smoking cessation due to concerns of high dropout and lower prevalence of smoking among patients with GOLD stage IV disease,[47, 48] making this a strength of our study. Subjects who died may have quit only in extremis, or failed to document their quit attempts. However, our sensitivity analysis limited to survivors did not change the study results. There may have been some misclassification in the use of buproprion, which may also be prescribed as an antidepressant. Finally, although representative of the veterans who seek care within the VISN‐20, our patients were primarily white and male, limiting the ability to generalize outside of this group.
Our study had several strengths. We examined a large cohort of patients admitted to a complete care organization, including patients from a diverse group of VA settings comprising academically and nonacademically affiliated centers. We performed an unbiased collection of patients, including all smokers discharged for COPD. We had access to excellent completeness of medications prescribed and filled as collected within the VA system, enabling us to observe medications dispensed and prescribed at several time points. We also had near complete ascertainment of outcomes including by using natural language processing with manual confirmation of smoking status.
In summary, we found that provision of medications to treat ongoing tobacco use among patients discharged for COPD was low, and receipt of medications was not associated with a reduction in smoking tobacco at 6 to 12 months postdischarge. However, among those treated, varenicline appears to be superior to the nicotine patch, with short‐acting nicotine replacement potentially less effective, a biologically plausible finding. The motivation to quit smoking changes rapidly over time. Providing these medications in the hospital and during the time after discharge is a potential means to improve quit rates, but medications need to be paired with counseling to be most effective. Collectively, these data suggest that systems‐based interventions are needed to increase the availability of intense counseling and the use of tailored pharmacotherapy to these patients.
Acknowledgements
The authors acknowledge Mr. Robert Plumley, who performed the data extraction and natural language processing necessary to complete this project.
Disclosures: Dr. Melzer conceived of the research question and performed background reading, analyses, primary drafting, and final revision of the manuscript. Drs. Collins and Feemster participated in finalizing the research question, developing the cohort, performing data collection, and revising the manuscript. Dr. Au provided the database for analysis, helped finalize the research question, and assisted in interpretation of the data and revision of the manuscript. Dr. Au has personally reviewed the data, understands the statistical methods employed, and confirms an understanding of this analysis, that the methods are clearly described, and that they are a fair way to report the results. This material is based upon work supported in part by the Department of Veterans Affairs, Veterans Health Administration, Office of Research and Development, who provided access to data, office space, and programming and data management. The views expressed in this article are those of the authors and do not necessarily reflect the position or policy of the Department of Veterans Affairs, the United States government, or the National Institutes of Health. Dr. Au is an unpaid research consultant for Analysis Group. None of the other authors have any conflicts of interest to disclose. Dr. Melzer is supported by an institutional F‐32 (HL007287‐36) through the University of Washington Department of Pulmonary and Critical Care. Dr. Feemster is supported by an National Institutes of Health, National Heart, Lung, and Blood Institute, K23 Mentored Career Development Award (HL111116). Partial support of this project was provided by Gilead Sciences with research funding to the Seattle Institute for Biomedical and Clinical Research. Additional support was received through the VA Health Services Research and Development. A portion of this work was presented in abstract form at the American Thoracic Society International Meeting, May 2015, in Denver, Colorado.
- Patients hospitalized for COPD have a high prevalence of modifiable risk factors for exacerbation (EFRAM study). Eur Respir J. 2000;16(6):1037–1042. , , , , , .
- Analysis of hospitalizations for COPD exacerbation: opportunities for improving care. COPD. 2010;7(2):85–92. , , , et al.
- Mortality in COPD: role of comorbidities. Eur Respir J. 2006;28(6):1245–1257. , , , .
- Cardiovascular comorbidity in COPD: systematic literature review. Chest. 2013;144(4):1163–1178. , , , .
- Engaging patients and clinicians in treating tobacco addiction. JAMA Intern Med. 2014;174(8):1299–1300. .
- Smokers who are hospitalized: a window of opportunity for cessation interventions. Prev Med. 1992;21(2):262–269. , .
- Interventions for smoking cessation in hospitalised patients. Cochrane Database Syst Rev. 2012;5:CD001837. , , , .
- Specifications Manual for National Hospital Inpatient Quality Measures. Available at: http://www.jointcommission.org/specifications_manual_for_national_hospital_inpatient_quality_measures.aspx. Accessed January 15, 2015.
- Treating Tobacco Use and Dependence. April 2013. Agency for Healthcare Research and Quality, Rockford, MD. Available at: http://www.ahrq.gov/professionals/clinicians‐providers/guidelines‐recommendations/tobacco/clinicians/update/index.html. Accessed January 15, 2015.
- Smoking cessation advice rates in US hospitals. Arch Intern Med. 2011;171(18):1682–1684. , , , .
- Validating smoking data from the Veteran's Affairs Health Factors dataset, an electronic data source. Nicotine Tob Res. 2011;13(12):1233–1239. , , , et al.
- Do u smoke after txt? Results of a randomised trial of smoking cessation using mobile phone text messaging. Tob Control. 2005;14(4):255–261. , , , et al.
- The effectiveness of smoking cessation groups offered to hospitalised patients with symptoms of exacerbations of chronic obstructive pulmonary disease (COPD). Clin Respir J. 2008;2(3):158–165. , , , .
- Sustained care intervention and postdischarge smoking cessation among hospitalized adults: a randomized clinical trial. JAMA. 2014;312(7):719–728. , , , et al.
- Bupropion for smokers hospitalized with acute cardiovascular disease. Am J Med. 2006;119(12):1080–1087. , , , et al.
- Adapting a clinical comorbidity index for use with ICD‐9‐CM administrative databases. J Clin Epidemiol. 1992;45(6):613–619. , , .
- Multiple Imputation for Nonresponse in Surveys. New York, NY: Wiley; 1987. .
- Methods for constructing and assessing propensity scores. Health Serv Res. 2014;49(5):1701–1720. , , , et al.
- Mortality and mortality‐related factors after hospitalization for acute exacerbation of COPD. Chest. 2003;124(2):459–467. , , .
- Mortality after hospitalization for COPD. Chest. 2002;121(5):1441–1448. , , , et al.
- State quitlines and cessation patterns among adults with selected chronic diseases in 15 states, 2005–2008. Prev Chronic Dis. 2012;9(10):120105. , , , , , .
- The effects of counseling on smoking cessation among patients hospitalized with chronic obstructive pulmonary disease: a randomized clinical trial. Int J Addict. 1991;26(1):107–119. , , .
- Predictors of smoking cessation after a myocardial infarction: the role of institutional smoking cessation programs in improving success. Arch Intern Med. 2008;168(18):1961–1967. , , , et al.
- Post‐myocardial infarction smoking cessation counseling: associations with immediate and late mortality in older Medicare patients. Am J Med. 2005;118(3):269–275. , , , , , .
- Smoking cessation after acute myocardial infarction: effects of a nurse‐‐managed intervention. Ann Intern Med. 1990;113(2):118–123. , , , .
- Smoking care provision in hospitals: a review of prevalence. Nicotine Tob Res. 2008;10(5):757–774. , , , et al.
- Intentions to quit smoking change over short periods of time. Addict Behav. 2005;30(4):653–662. , , , .
- Association of amount and duration of NRT use in smokers with cigarette consumption and motivation to stop smoking: a national survey of smokers in England. Addict Behav. 2015;40(0):33–38. , , , , .
- Smoking prevalence, behaviours, and cessation among individuals with COPD or asthma. Respir Med. 2011;105(3):477–484. , .
- American College of Chest Physicians. Tobacco Dependence Treatment ToolKit. 3rd ed. Available at: http://tobaccodependence.chestnet.org. Accessed January 29, 2015. , , , et al.
- Effects of varenicline on smoking cessation in patients with mild to moderate COPD: a randomized controlled trial. Chest. 2011;139(3):591–599. , , , , , .
- Varenicline versus transdermal nicotine patch for smoking cessation: results from a randomised open‐label trial. Thorax. 2008;63(8):717–724. , , , et al.
- Psychiatric adverse events in randomized, double‐blind, placebo‐controlled clinical trials of varenicline. Drug Saf. 2010;33(4):289–301. , , , , .
- Studies linking smoking‐cessation drug with suicide risk spark concerns. JAMA. 2009;301(10):1007–1008. .
- A randomized, double‐blind, placebo‐controlled study evaluating the safety and efficacy of varenicline for smoking cessation in patients with schizophrenia or schizoaffective disorder. J Clin Psychiatry. 2012;73(5):654–660. , , , et al.
- Smoking and mental illness: results from population surveys in Australia and the United States. BMC Public Health. 2009;9(1):285. , , .
- Implementation and effectiveness of a brief smoking‐cessation intervention for hospital patients. Med Care. 2000;38(5):451–459. , , , .
- Clinical trial comparing nicotine replacement therapy (NRT) plus brief counselling, brief counselling alone, and minimal intervention on smoking cessation in hospital inpatients. Thorax. 2003;58(6):484–488. , , , et al.
- Dissociation between hospital performance of the smoking cessation counseling quality metric and cessation outcomes after myocardial infarction. Arch Intern Med. 2008;168(19):2111–2117. , , , et al.
- Smoking cessation in hospitalized patients: Results of a randomized trial. Arch Intern Med. 1997;157(4):409–415. , , , , .
- Intensive smoking cessation counseling versus minimal counseling among hospitalized smokers treated with transdermal nicotine replacement: a randomized trial. Am J Med. 2003;114(7):555–562. , , , , .
- Motivational factors predict quit attempts but not maintenance of smoking cessation: findings from the International Tobacco Control Four country project. Nicotine Tob Res. 2010;12(suppl):S4–S11. , , , et al.
- Predictors of attempts to stop smoking and their success in adult general population samples: a systematic review. Addiction. 2011;106(12):2110–2121. , , , , .
- Validity of self‐reported smoking status among participants in a lung cancer screening trial. Cancer Epidemiol Biomarkers Prev. 2006;15(10):1825–1828. , , , et al.
- VHA enrollees' health care coverage and use of care. Med Care Res Rev. 2003;60(2):253–67. , , , .
- Association between lung function and exacerbation frequency in patients with COPD. Int J Chron Obstruct Pulmon Dis. 2010;5:435–444. , , , , .
- Smoking cessation in patients with chronic obstructive pulmonary disease: a double‐blind, placebo‐controlled, randomised trial. Lancet. 2001;357(9268):1571–1575. , , , et al.
- Nurse‐conducted smoking cessation in patients with COPD using nicotine sublingual tablets and behavioral support. Chest. 2006;130(2):334–342. , , .
Up to one‐third of the 700,000 patients admitted annually for an exacerbation of chronic obstructive pulmonary disease (COPD) continue to smoke tobacco.[1, 2] Smokers with COPD are at high risk for poor health outcomes directly attributable to tobacco‐related conditions, including progression of lung disease and cardiovascular diseases.[3, 4, 5] Treatment for tobacco addiction is the most essential intervention for these patients.
Hospital admission has been suggested as an opportune time for the initiation of smoking cessation.[6] Hospitalized patients are already in a smoke‐free environment, and have access to physicians, nurses, and pharmacists who can prescribe medications for support.[7] Documenting smoking status and offering smoking cessation treatment during and after discharge are quality metrics required by the Joint Commission, and recommended by the National Quality Forum.[8, 9] Hospitals have made significant efforts to comply with these requirements.[10]
Limited data exist regarding the effectiveness and utilization of treatments known to reduce cigarette use among COPD patients in nontrial environments. Prescribing patterns of medications for smoking cessation in the real world following admission for COPD are not well studied. We sought to examine the utilization of inpatient brief tobacco counseling and postdischarge pharmacotherapy following discharge for exacerbation of COPD, as well as to (1) examine the association of postdischarge pharmacotherapy with self‐reported smoking cessation at 6 to 12 months and (2) assess differences in effectiveness between cessation medications prescribed.
METHODS
We conducted a cohort study of current smokers discharged following a COPD exacerbation within the Veterans Affairs (VA) Veterans Integrated Service Network (VISN)‐20. This study was approved by the VA Puget Sound Health Care System Institutional Review Board (#00461).
We utilized clinical information from the VISN‐20 data warehouse that collects data using the VA electronic medical record, including demographics, prescription medications, hospital admissions, hospital and outpatient diagnoses, and dates of death, and is commonly used for research. In addition, we utilized health factors, coded electronic entries describing patient health behaviors that are entered by nursing staff at the time of a patient encounter, and the text of chart notes that were available for electronic query.
Study Cohort
We identified all smokers aged 40 years hospitalized between 2005 and 2012 with either a primary discharge diagnosis of COPD based on International Classification of Diseases, 9th Revision codes (491, 492, 493.2, and 496) or an admission diagnosis from the text of the admit notes indicating an exacerbation of COPD. We limited to patients aged 40 years to improve the specificity of the diagnosis of COPD, and we selected the first hospitalization that met inclusion criteria. We excluded subjects who died within 6 months of discharge (Figure 1).
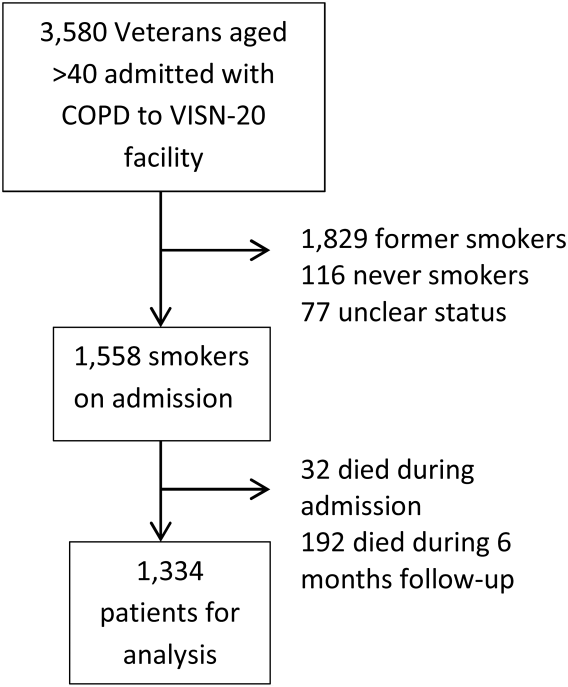
To establish tobacco status, we built on previously developed and validated methodology,[11] and performed truncated natural language processing using phrases in the medical record that reflected patients' tobacco status, querying all notes from the day of admission up to 6 months prior. If no tobacco status was indicated in the notes, we identified the status encoded by the most recent health factor. We manually examined the results of the natural language processing and the determination of health factors to confirm the tobacco status. Manual review was undertaken by 1 of 2 trained study personnel. In the case of an ambiguous or contradictory status, an additional team member reviewed the information to attempt to make a determination. If no determination could be made, the record was coded to unknown. This method allowed us to identify a baseline status for all but 77 of the 3580 patients admitted for COPD.
Outcome and Exposure
The outcome was tobacco status at 6 to 12 months after discharge. Using the same methods developed for identification of baseline smoking status, we obtained smoking status for each subject up to 12 months postdischarge. If multiple notes and encounters were available indicating smoking status, we chose the latest within 12 months of discharge. Subjects lacking a follow‐up status were presumed to be smokers, a common assumption.[12] The 6 to 12month time horizon was chosen as these are the most common time points used to examine a sustained change in tobacco status,[13, 14, 15] and allowed for adequate time for treatment and clinical follow‐up.
Our primary exposure was any smoking cessation medication or combination dispensed within 90 days of discharge. This time horizon for treatment was chosen due to recent studies indicating this is a meaningful period for postdischarge treatment.[14] We assessed the use of nicotine patch, short‐acting nicotine, varenicline, buproprion, or any combination. Accurate data on the prescription and dispensing of these medications were available from the VA pharmacy record. Secondary exposure was the choice of medication dispensed among treated patients. We assessed additional exposures including receipt of cessation medications within 48 hours of discharge, treatment in the year prior to admission, and predischarge counseling. Predischarge counseling was determined as having occurred if nurses documented that they completed a discharge process focused on smoking cessation. Referral to a quit line is part of this process; however, due to the confidential nature of these interactions, generally low use of this service, and lack of linkage to the VA electronic health record, it was not considered in the analysis.
Confounders
Potential confounders were assessed in the year prior to admission up to discharge from the index hospitalization, with the use of mechanical or noninvasive ventilation assessed during the hospitalization. We adjusted for variables chosen a priori for their known or expected association with smoking cessation including demographics, Charlson Comorbidity Index,[16] markers of COPD severity (need for invasive or noninvasive mechanical ventilation during index hospitalization, use of oral steroids, long‐acting inhaled bronchodilators, and/or canister count of short‐acting bronchodilators in the year prior to admission), history of drug or alcohol abuse, homelessness, depression, psychosis, post‐traumatic stress disorder, lung cancer, coronary artery disease, and under‐ or overweight status. Nurse‐based counseling prior to discharge was included as a variable for adjustment for our primary and secondary predictors to assess the influence of pharmacotherapy specifically. Due to 3.1% missingness in body mass index, multiple imputation with chained equations was used to impute missing values, with 10 imputations performed. The imputation was performed using a linear regression model containing all variables included in the final model, grouped by facility.
Statistical Analysis
All analyses were performed using Stata 13 (StataCorp, College Station, TX) software. 2 tests and t tests were used to assess for unadjusted bivariate associations. Using the pooled imputed datasets, we performed multivariable logistic regression to compare odds ratios for a change in smoking status, adjusting the estimates of coefficients and standard errors by applying combination rules to the 10 completed‐data estimates.[17] We analyzed our primary and secondary predictors, adjusting for the confounders chosen a priori, clustered by facility with robust standard errors. An level of <0.05 was considered significant.
Sensitivity Analysis
We assumed that subjects missing a follow‐up status were ongoing smokers. However, given the high mortality rate observed in our cohort, we were concerned that some subjects lacking a follow‐up status may have died, missing the opportunity to have a quit attempt recorded. Therefore, we performed sensitivity analysis excluding subjects who died during the 6 to 12 months of follow‐up, repeating the imputation and analysis as described above. In addition, due to concern for indication bias in the choice of medication used for our secondary analysis, we performed propensity score matching for treatment with each medication in comparison to nicotine patch, using the teffects command, with 3 nearest neighbor matches. We included additional comorbidities in the propensity score matching.[18]
RESULTS
Among these 1334 subjects at 6 to 12 months of follow‐up, 63.7% reported ongoing smoking, 19.8% of patients reported quitting, and 17.5% of patients had no reported status and were presumed to be smokers. Four hundred fifty (33.7%) patients were dispensed a smoking cessation medication within 90 days of discharge. Patients who were dispensed medications were younger and more likely to be female. Nearly all patients who received medications also received documented predischarge counseling (94.6%), as did the majority of patients who did not receive medications (83.8%) (Table 1).
Variable | No Medication Dispensed, n = 884, No. (%) | Medication Dispensed, n = 450, No. (%) | P Value |
---|---|---|---|
| |||
Not smoking at 612 months | 179 (20.2) | 85 (18.9) | 0.56 |
Brief counseling at discharge | 742 (83.8%) | 424 (94.6%) | <0.001* |
Age | 64.49.13 (4094) | 61.07.97 (4185) | <0.001* |
Male | 852 (96.3) | 423 (94.0) | 0.05* |
Race | 0.12 | ||
White | 744 (84.2) | 377 (83.8) | |
Black | 41 (4.6) | 12 (2.7) | |
Other/unknown | 99 (11.1) | 61 (13.6) | |
BMI | 28.09.5 (12.669.0) | 28.910.8 (14.860.0) | 0.15 |
Homeless | 68 (7.7) | 36 (8.0) | 0.84 |
Psychiatric conditions/substance abuse | |||
History of alcohol abuse | 205 (23.2) | 106 (23.6) | 0.88 |
History of drug abuse | 110 (12.4) | 72 (16.0) | 0.07 |
Depression | 39 (4.4) | 29 (6.4) | 0.11 |
Psychosis | 201 (22.7) | 88 (19.6) | 0.18 |
PTSD | 146 (16.5) | 88 (19.6) | 0.17 |
Comorbidities | |||
Coronary artery disease | 254 (28.7) | 110 (24.4) | 0.10 |
Cerebrovascular accident | 80 (9.0) | 28 (2.2) | 0.86 |
Obstructive sleep apnea | 42 (4.8) | 23 (5.1) | 0.77 |
Lung cancer | 21 (2.4) | 10 (2.2) | 0.86 |
Charlson Comorbidity Index | 2.251.93 (014) | 2.111.76 (010) | 0.49 |
Markers of COPD severity | |||
Mechanical ventilation during admission | 28 (3.2) | 14 (3.1) | 0.96 |
NIPPV during admission | 97 (11.0) | 51 (11.3) | 0.84 |
Oral steroids prescribed in the past year | 334 (37.8) | 154 (34.2) | 0.20 |
Treatment with tiotropium in the past year | 97 (11.0) | 55 (12.2) | 0.50 |
Treatment with LABA in the past year | 264 (29.9) | 155 (34.4) | 0.09 |
Canisters of SABA used in past year | 6.639.8, (084) | 7.469.63 (045) | 0.14 |
Canisters of ipratropium used in past year | 6.458.81 (054) | 6.869.08 (064) | 0.42 |
Died during 612 months of follow‐up | 78 (8.8) | 28 (6.6) | 0.10 |
Of patients dispensed a study medication, 246 (18.4% of patients, 54.7% of all medications dispensed) were dispensed medications within 48 hours of discharge (Table 2). Of the patients dispensed medication, the majority received nicotine patches alone (Table 3), and 18.9% of patients received combination therapy, with the majority receiving nicotine patch and short‐acting nicotine replacement therapy (NRT) or patch and buproprion. A significant number of patients were prescribed medications within 90 days of discharge, but did not have them dispensed within that timeframe (n = 224, 16.8%).
Medication Dispensed | No. (%) | % Quit (Unadjusted) | OR (95% CI) | P Value |
---|---|---|---|---|
| ||||
No medications dispensed | 884 (66.3) | 20.2 | Referent | |
Any medication from | ||||
Discharge to 90 days | 450 (33.7) | 18.9 | 0.88 (0.741.04) | 0.137 |
Within 48 hours of discharge | 246 (18.4) | 18.3 | 0.87 (0.661.14) | 0.317 |
Treated in the year prior to admission | 221 (16.6) | 19.6 | Referent | |
Treated in the year prior to admission + 090 days postdischarge | 152 (11.4) | 18.4 | 0.95 (0.791.13) | 0.534 |
No nurse‐provided counseling prior to discharge | 169 (12.7) | 20.5 | Referent | |
Nurse‐provided counseling prior to discharge | 1,165 (87.3) | 19.5 | 0.95 (0.661.36) | 0.774 |
Medication Dispensed | No. (%) | % Quit (Unadjusted) | OR (95% CI) | P Value |
---|---|---|---|---|
| ||||
Nicotine patch | 242 (53.8) | 18.6 | Referent | |
Monotherapy with | ||||
Varenicline | 36 (8.0) | 30.6 | 2.44 (1.484.05) | 0.001 |
Short‐acting NRT | 34 (7.6) | 11.8 | 0.66 (0.510.85) | 0.001 |
Buproprion | 55 (12.2) | 21.8 | 1.05 (0.671.62) | 0.843 |
Combination therapy | 85 (18.9) | 15.7 | 0.94 (0.711.24) | 0.645 |
Association of Treatment With Study Medications and Quitting Smoking
In adjusted analyses, the odds of quitting smoking at 6 to 12 months were not greater among patients who were dispensed a study medication within 90 days of discharge (odds ratio [OR]: 0.88, 95% confidence interval [CI]: 0.74‐1.04). We found no association between counseling provided at discharge and smoking cessation (OR: 0.95, 95% CI: 0.0.66‐1.), adjusted for the receipt of medications. There was no difference in quit rate between patients dispensed medication within 48 hours of discharge, or between patients treated in the year prior to admission and again postdischarge (Table 2).
We then assessed differences in effectiveness between specific medications among the 450 patients who were dispensed medications. Using nicotine patch alone as the referent group, patients treated with varenicline demonstrated greater odds of smoking cessation (OR: 2.44, 95% CI: 1.48‐4.05). Patients treated with short‐acting NRT alone were less likely to report smoking cessation (OR: 0.66, 95% CI: 0.51‐0.85). Patients treated with buproprion or combination therapy were no more likely to report cessation (Table 3). When sensitivity analysis was performed using propensity score matching with additional variables included, there were no significant differences in the observed associations.
Our overall mortality rate observed at 1 year was 19.5%, nearly identical to previous cohort studies of patients admitted for COPD.[19, 20] Because of the possibility of behavioral differences on the part of patients and physicians regarding subjects with a limited life expectancy, we performed sensitivity analysis limited to the patients who survived to at least 12 months of follow‐up. One hundred six patients (7.9%) died during 6 to 12 months of follow‐up. There was no change in inference for our primary exposure (OR: 0.95, 95% CI: 0.79‐1.14) or any of the secondary exposures examined.
DISCUSSION
In this observational study, postdischarge pharmacotherapy within 90 days of discharge was provided to a minority of high‐risk smokers admitted for COPD, and was not associated with smoking cessation at 6 to 12 months. In comparison to nicotine patch alone, varenicline was associated with a higher odds of cessation, with decreased odds of cessation among patients treated with short‐acting NRT alone. The overall quit rate was significant at 19.8%, and is consistent with annual quit rates observed among patients with COPD in other settings,[21, 22] but is far lower than quit rates observed after admission for acute myocardial infarction.[23, 24, 25] Although the proportion of patients treated at the time of discharge or within 90 days was low, our findings are in keeping with previous studies, which demonstrated low rates of pharmacologic treatment following hospitalization, averaging 14%.[26] Treatment for tobacco use is likely underutilized for this group of high‐risk smokers. However, a significant proportion of patients who were prescribed medications in the postdischarge period did not have medications filled. This likely reflects both the rapid changes in motivation that characterize quit attempts,[27] as well as efforts on the part of primary care physicians to make these medications available to facilitate future quit attempts.
There are several possible explanations for the findings in our study. Pharmaceutical therapies were not provided at random. The provision of pharmacotherapy and the ultimate success of a quit attempt reflects a complex interaction of patient beliefs concerning medications, level of addiction and motivation, physician behavior and knowledge, and organizational factors. Organizational factors such as the structure of electronic discharge orders and the availability of decision support materials may influence a physician's likelihood of prescribing medications, the choice of medication prescribed, and therefore the adequacy of control of withdrawal symptoms. NRT is often under dosed to control ongoing symptoms,[28] and needs to be adjusted until relief is obtained, providing an additional barrier to effectiveness during the transition out of the hospital. Because most smokers with COPD are highly addicted to nicotine,[29] high‐dose NRT, combination therapy, or varenicline would be necessary to adequately control symptoms.[30] However, a significant minority of patients received short‐acting NRT alone.
Despite a high observed efficacy in recent trials,[31, 32] few subjects in our study received varenicline. This may be related to both secular trends and administrative barriers to the use of varenicline in the VA system. Use of this medication was limited among patients with psychiatric disorders due to safety concerns. These concerns have since been largely disproven, but may have limited access to this medication.[33, 34, 35] Although we adjusted for a history of mental illness, patients who received varenicline may have had more past quit attempts and less active mental illness, which may be associated with improved cessation rates. Despite the high prevalence of mental illness we observed, this is typical of the population of smokers, with studies indicating nearly one‐third of smokers overall suffer from mental illness.[36]
Although the majority of our patients received a brief, nurse‐based counseling intervention, there is considerable concern about the overall effectiveness of a single predischarge interaction to produce sustained smoking cessation among highly addicted smokers.[37, 38, 39, 40] The Joint Commission has recently restructured the requirements for smoking cessation treatment for hospitalized patients, and it is now up to hospitals to implement treatment mechanisms that not only meet the national requirements, but also provide a meaningful clinical effect. Though the optimum treatment for hospitalized smokers with COPD is unknown, previous positive studies of smoking cessation among hospitalized patients underscore the need for a higher‐intensity counseling intervention that begins during hospitalization and continues after discharge.[13, 41] Cessation counseling services including tobacco cessation groups and quit lines are available through the VA; however, the use of these services is typically low and requires the patient to enroll independently after discharge, an additional barrier. The lack of association between medications and smoking cessation found in our study could reflect poor effectiveness of medications in the absence of a systematic counseling intervention. Alternatively, the association may be explained that patients who were more highly addicted and perhaps less motivated to quit received tobacco cessation medications more often, but were also less likely to stop tobacco use, a form of indication bias.
Our study has several limitations. We do not have addiction or motivation levels for a cessation attempt, a potential unmeasured confounder. Although predictive of quit attempts, motivation factors are less predictive of cessation maintenance, and may therefore have an unclear effect on our outcome.[42, 43] Our outcome was gathered as part of routine clinical care, which may have introduced bias if patients over‐reported cessation because of social desirability. In healthcare settings, however, this form of assessing smoking status is generally valid.[44] Exposure to counseling or medications obtained outside of the VA system would not have been captured. Given the financial incentive, we believe it is unlikely that many patients admitted to a VA medical center obtained medications elsewhere.[45] The diagnosis of COPD was made administratively. However, all subjects were admitted for an exacerbation, which is associated with more severe COPD by Global Initiative for Obstructive Lung Disease (GOLD) stage.[46] Patients with more severe COPD are often excluded from studies of smoking cessation due to concerns of high dropout and lower prevalence of smoking among patients with GOLD stage IV disease,[47, 48] making this a strength of our study. Subjects who died may have quit only in extremis, or failed to document their quit attempts. However, our sensitivity analysis limited to survivors did not change the study results. There may have been some misclassification in the use of buproprion, which may also be prescribed as an antidepressant. Finally, although representative of the veterans who seek care within the VISN‐20, our patients were primarily white and male, limiting the ability to generalize outside of this group.
Our study had several strengths. We examined a large cohort of patients admitted to a complete care organization, including patients from a diverse group of VA settings comprising academically and nonacademically affiliated centers. We performed an unbiased collection of patients, including all smokers discharged for COPD. We had access to excellent completeness of medications prescribed and filled as collected within the VA system, enabling us to observe medications dispensed and prescribed at several time points. We also had near complete ascertainment of outcomes including by using natural language processing with manual confirmation of smoking status.
In summary, we found that provision of medications to treat ongoing tobacco use among patients discharged for COPD was low, and receipt of medications was not associated with a reduction in smoking tobacco at 6 to 12 months postdischarge. However, among those treated, varenicline appears to be superior to the nicotine patch, with short‐acting nicotine replacement potentially less effective, a biologically plausible finding. The motivation to quit smoking changes rapidly over time. Providing these medications in the hospital and during the time after discharge is a potential means to improve quit rates, but medications need to be paired with counseling to be most effective. Collectively, these data suggest that systems‐based interventions are needed to increase the availability of intense counseling and the use of tailored pharmacotherapy to these patients.
Acknowledgements
The authors acknowledge Mr. Robert Plumley, who performed the data extraction and natural language processing necessary to complete this project.
Disclosures: Dr. Melzer conceived of the research question and performed background reading, analyses, primary drafting, and final revision of the manuscript. Drs. Collins and Feemster participated in finalizing the research question, developing the cohort, performing data collection, and revising the manuscript. Dr. Au provided the database for analysis, helped finalize the research question, and assisted in interpretation of the data and revision of the manuscript. Dr. Au has personally reviewed the data, understands the statistical methods employed, and confirms an understanding of this analysis, that the methods are clearly described, and that they are a fair way to report the results. This material is based upon work supported in part by the Department of Veterans Affairs, Veterans Health Administration, Office of Research and Development, who provided access to data, office space, and programming and data management. The views expressed in this article are those of the authors and do not necessarily reflect the position or policy of the Department of Veterans Affairs, the United States government, or the National Institutes of Health. Dr. Au is an unpaid research consultant for Analysis Group. None of the other authors have any conflicts of interest to disclose. Dr. Melzer is supported by an institutional F‐32 (HL007287‐36) through the University of Washington Department of Pulmonary and Critical Care. Dr. Feemster is supported by an National Institutes of Health, National Heart, Lung, and Blood Institute, K23 Mentored Career Development Award (HL111116). Partial support of this project was provided by Gilead Sciences with research funding to the Seattle Institute for Biomedical and Clinical Research. Additional support was received through the VA Health Services Research and Development. A portion of this work was presented in abstract form at the American Thoracic Society International Meeting, May 2015, in Denver, Colorado.
Up to one‐third of the 700,000 patients admitted annually for an exacerbation of chronic obstructive pulmonary disease (COPD) continue to smoke tobacco.[1, 2] Smokers with COPD are at high risk for poor health outcomes directly attributable to tobacco‐related conditions, including progression of lung disease and cardiovascular diseases.[3, 4, 5] Treatment for tobacco addiction is the most essential intervention for these patients.
Hospital admission has been suggested as an opportune time for the initiation of smoking cessation.[6] Hospitalized patients are already in a smoke‐free environment, and have access to physicians, nurses, and pharmacists who can prescribe medications for support.[7] Documenting smoking status and offering smoking cessation treatment during and after discharge are quality metrics required by the Joint Commission, and recommended by the National Quality Forum.[8, 9] Hospitals have made significant efforts to comply with these requirements.[10]
Limited data exist regarding the effectiveness and utilization of treatments known to reduce cigarette use among COPD patients in nontrial environments. Prescribing patterns of medications for smoking cessation in the real world following admission for COPD are not well studied. We sought to examine the utilization of inpatient brief tobacco counseling and postdischarge pharmacotherapy following discharge for exacerbation of COPD, as well as to (1) examine the association of postdischarge pharmacotherapy with self‐reported smoking cessation at 6 to 12 months and (2) assess differences in effectiveness between cessation medications prescribed.
METHODS
We conducted a cohort study of current smokers discharged following a COPD exacerbation within the Veterans Affairs (VA) Veterans Integrated Service Network (VISN)‐20. This study was approved by the VA Puget Sound Health Care System Institutional Review Board (#00461).
We utilized clinical information from the VISN‐20 data warehouse that collects data using the VA electronic medical record, including demographics, prescription medications, hospital admissions, hospital and outpatient diagnoses, and dates of death, and is commonly used for research. In addition, we utilized health factors, coded electronic entries describing patient health behaviors that are entered by nursing staff at the time of a patient encounter, and the text of chart notes that were available for electronic query.
Study Cohort
We identified all smokers aged 40 years hospitalized between 2005 and 2012 with either a primary discharge diagnosis of COPD based on International Classification of Diseases, 9th Revision codes (491, 492, 493.2, and 496) or an admission diagnosis from the text of the admit notes indicating an exacerbation of COPD. We limited to patients aged 40 years to improve the specificity of the diagnosis of COPD, and we selected the first hospitalization that met inclusion criteria. We excluded subjects who died within 6 months of discharge (Figure 1).
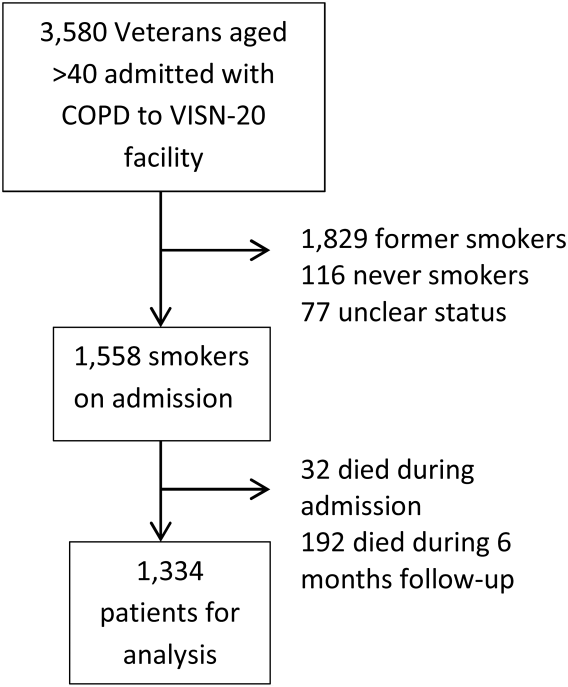
To establish tobacco status, we built on previously developed and validated methodology,[11] and performed truncated natural language processing using phrases in the medical record that reflected patients' tobacco status, querying all notes from the day of admission up to 6 months prior. If no tobacco status was indicated in the notes, we identified the status encoded by the most recent health factor. We manually examined the results of the natural language processing and the determination of health factors to confirm the tobacco status. Manual review was undertaken by 1 of 2 trained study personnel. In the case of an ambiguous or contradictory status, an additional team member reviewed the information to attempt to make a determination. If no determination could be made, the record was coded to unknown. This method allowed us to identify a baseline status for all but 77 of the 3580 patients admitted for COPD.
Outcome and Exposure
The outcome was tobacco status at 6 to 12 months after discharge. Using the same methods developed for identification of baseline smoking status, we obtained smoking status for each subject up to 12 months postdischarge. If multiple notes and encounters were available indicating smoking status, we chose the latest within 12 months of discharge. Subjects lacking a follow‐up status were presumed to be smokers, a common assumption.[12] The 6 to 12month time horizon was chosen as these are the most common time points used to examine a sustained change in tobacco status,[13, 14, 15] and allowed for adequate time for treatment and clinical follow‐up.
Our primary exposure was any smoking cessation medication or combination dispensed within 90 days of discharge. This time horizon for treatment was chosen due to recent studies indicating this is a meaningful period for postdischarge treatment.[14] We assessed the use of nicotine patch, short‐acting nicotine, varenicline, buproprion, or any combination. Accurate data on the prescription and dispensing of these medications were available from the VA pharmacy record. Secondary exposure was the choice of medication dispensed among treated patients. We assessed additional exposures including receipt of cessation medications within 48 hours of discharge, treatment in the year prior to admission, and predischarge counseling. Predischarge counseling was determined as having occurred if nurses documented that they completed a discharge process focused on smoking cessation. Referral to a quit line is part of this process; however, due to the confidential nature of these interactions, generally low use of this service, and lack of linkage to the VA electronic health record, it was not considered in the analysis.
Confounders
Potential confounders were assessed in the year prior to admission up to discharge from the index hospitalization, with the use of mechanical or noninvasive ventilation assessed during the hospitalization. We adjusted for variables chosen a priori for their known or expected association with smoking cessation including demographics, Charlson Comorbidity Index,[16] markers of COPD severity (need for invasive or noninvasive mechanical ventilation during index hospitalization, use of oral steroids, long‐acting inhaled bronchodilators, and/or canister count of short‐acting bronchodilators in the year prior to admission), history of drug or alcohol abuse, homelessness, depression, psychosis, post‐traumatic stress disorder, lung cancer, coronary artery disease, and under‐ or overweight status. Nurse‐based counseling prior to discharge was included as a variable for adjustment for our primary and secondary predictors to assess the influence of pharmacotherapy specifically. Due to 3.1% missingness in body mass index, multiple imputation with chained equations was used to impute missing values, with 10 imputations performed. The imputation was performed using a linear regression model containing all variables included in the final model, grouped by facility.
Statistical Analysis
All analyses were performed using Stata 13 (StataCorp, College Station, TX) software. 2 tests and t tests were used to assess for unadjusted bivariate associations. Using the pooled imputed datasets, we performed multivariable logistic regression to compare odds ratios for a change in smoking status, adjusting the estimates of coefficients and standard errors by applying combination rules to the 10 completed‐data estimates.[17] We analyzed our primary and secondary predictors, adjusting for the confounders chosen a priori, clustered by facility with robust standard errors. An level of <0.05 was considered significant.
Sensitivity Analysis
We assumed that subjects missing a follow‐up status were ongoing smokers. However, given the high mortality rate observed in our cohort, we were concerned that some subjects lacking a follow‐up status may have died, missing the opportunity to have a quit attempt recorded. Therefore, we performed sensitivity analysis excluding subjects who died during the 6 to 12 months of follow‐up, repeating the imputation and analysis as described above. In addition, due to concern for indication bias in the choice of medication used for our secondary analysis, we performed propensity score matching for treatment with each medication in comparison to nicotine patch, using the teffects command, with 3 nearest neighbor matches. We included additional comorbidities in the propensity score matching.[18]
RESULTS
Among these 1334 subjects at 6 to 12 months of follow‐up, 63.7% reported ongoing smoking, 19.8% of patients reported quitting, and 17.5% of patients had no reported status and were presumed to be smokers. Four hundred fifty (33.7%) patients were dispensed a smoking cessation medication within 90 days of discharge. Patients who were dispensed medications were younger and more likely to be female. Nearly all patients who received medications also received documented predischarge counseling (94.6%), as did the majority of patients who did not receive medications (83.8%) (Table 1).
Variable | No Medication Dispensed, n = 884, No. (%) | Medication Dispensed, n = 450, No. (%) | P Value |
---|---|---|---|
| |||
Not smoking at 612 months | 179 (20.2) | 85 (18.9) | 0.56 |
Brief counseling at discharge | 742 (83.8%) | 424 (94.6%) | <0.001* |
Age | 64.49.13 (4094) | 61.07.97 (4185) | <0.001* |
Male | 852 (96.3) | 423 (94.0) | 0.05* |
Race | 0.12 | ||
White | 744 (84.2) | 377 (83.8) | |
Black | 41 (4.6) | 12 (2.7) | |
Other/unknown | 99 (11.1) | 61 (13.6) | |
BMI | 28.09.5 (12.669.0) | 28.910.8 (14.860.0) | 0.15 |
Homeless | 68 (7.7) | 36 (8.0) | 0.84 |
Psychiatric conditions/substance abuse | |||
History of alcohol abuse | 205 (23.2) | 106 (23.6) | 0.88 |
History of drug abuse | 110 (12.4) | 72 (16.0) | 0.07 |
Depression | 39 (4.4) | 29 (6.4) | 0.11 |
Psychosis | 201 (22.7) | 88 (19.6) | 0.18 |
PTSD | 146 (16.5) | 88 (19.6) | 0.17 |
Comorbidities | |||
Coronary artery disease | 254 (28.7) | 110 (24.4) | 0.10 |
Cerebrovascular accident | 80 (9.0) | 28 (2.2) | 0.86 |
Obstructive sleep apnea | 42 (4.8) | 23 (5.1) | 0.77 |
Lung cancer | 21 (2.4) | 10 (2.2) | 0.86 |
Charlson Comorbidity Index | 2.251.93 (014) | 2.111.76 (010) | 0.49 |
Markers of COPD severity | |||
Mechanical ventilation during admission | 28 (3.2) | 14 (3.1) | 0.96 |
NIPPV during admission | 97 (11.0) | 51 (11.3) | 0.84 |
Oral steroids prescribed in the past year | 334 (37.8) | 154 (34.2) | 0.20 |
Treatment with tiotropium in the past year | 97 (11.0) | 55 (12.2) | 0.50 |
Treatment with LABA in the past year | 264 (29.9) | 155 (34.4) | 0.09 |
Canisters of SABA used in past year | 6.639.8, (084) | 7.469.63 (045) | 0.14 |
Canisters of ipratropium used in past year | 6.458.81 (054) | 6.869.08 (064) | 0.42 |
Died during 612 months of follow‐up | 78 (8.8) | 28 (6.6) | 0.10 |
Of patients dispensed a study medication, 246 (18.4% of patients, 54.7% of all medications dispensed) were dispensed medications within 48 hours of discharge (Table 2). Of the patients dispensed medication, the majority received nicotine patches alone (Table 3), and 18.9% of patients received combination therapy, with the majority receiving nicotine patch and short‐acting nicotine replacement therapy (NRT) or patch and buproprion. A significant number of patients were prescribed medications within 90 days of discharge, but did not have them dispensed within that timeframe (n = 224, 16.8%).
Medication Dispensed | No. (%) | % Quit (Unadjusted) | OR (95% CI) | P Value |
---|---|---|---|---|
| ||||
No medications dispensed | 884 (66.3) | 20.2 | Referent | |
Any medication from | ||||
Discharge to 90 days | 450 (33.7) | 18.9 | 0.88 (0.741.04) | 0.137 |
Within 48 hours of discharge | 246 (18.4) | 18.3 | 0.87 (0.661.14) | 0.317 |
Treated in the year prior to admission | 221 (16.6) | 19.6 | Referent | |
Treated in the year prior to admission + 090 days postdischarge | 152 (11.4) | 18.4 | 0.95 (0.791.13) | 0.534 |
No nurse‐provided counseling prior to discharge | 169 (12.7) | 20.5 | Referent | |
Nurse‐provided counseling prior to discharge | 1,165 (87.3) | 19.5 | 0.95 (0.661.36) | 0.774 |
Medication Dispensed | No. (%) | % Quit (Unadjusted) | OR (95% CI) | P Value |
---|---|---|---|---|
| ||||
Nicotine patch | 242 (53.8) | 18.6 | Referent | |
Monotherapy with | ||||
Varenicline | 36 (8.0) | 30.6 | 2.44 (1.484.05) | 0.001 |
Short‐acting NRT | 34 (7.6) | 11.8 | 0.66 (0.510.85) | 0.001 |
Buproprion | 55 (12.2) | 21.8 | 1.05 (0.671.62) | 0.843 |
Combination therapy | 85 (18.9) | 15.7 | 0.94 (0.711.24) | 0.645 |
Association of Treatment With Study Medications and Quitting Smoking
In adjusted analyses, the odds of quitting smoking at 6 to 12 months were not greater among patients who were dispensed a study medication within 90 days of discharge (odds ratio [OR]: 0.88, 95% confidence interval [CI]: 0.74‐1.04). We found no association between counseling provided at discharge and smoking cessation (OR: 0.95, 95% CI: 0.0.66‐1.), adjusted for the receipt of medications. There was no difference in quit rate between patients dispensed medication within 48 hours of discharge, or between patients treated in the year prior to admission and again postdischarge (Table 2).
We then assessed differences in effectiveness between specific medications among the 450 patients who were dispensed medications. Using nicotine patch alone as the referent group, patients treated with varenicline demonstrated greater odds of smoking cessation (OR: 2.44, 95% CI: 1.48‐4.05). Patients treated with short‐acting NRT alone were less likely to report smoking cessation (OR: 0.66, 95% CI: 0.51‐0.85). Patients treated with buproprion or combination therapy were no more likely to report cessation (Table 3). When sensitivity analysis was performed using propensity score matching with additional variables included, there were no significant differences in the observed associations.
Our overall mortality rate observed at 1 year was 19.5%, nearly identical to previous cohort studies of patients admitted for COPD.[19, 20] Because of the possibility of behavioral differences on the part of patients and physicians regarding subjects with a limited life expectancy, we performed sensitivity analysis limited to the patients who survived to at least 12 months of follow‐up. One hundred six patients (7.9%) died during 6 to 12 months of follow‐up. There was no change in inference for our primary exposure (OR: 0.95, 95% CI: 0.79‐1.14) or any of the secondary exposures examined.
DISCUSSION
In this observational study, postdischarge pharmacotherapy within 90 days of discharge was provided to a minority of high‐risk smokers admitted for COPD, and was not associated with smoking cessation at 6 to 12 months. In comparison to nicotine patch alone, varenicline was associated with a higher odds of cessation, with decreased odds of cessation among patients treated with short‐acting NRT alone. The overall quit rate was significant at 19.8%, and is consistent with annual quit rates observed among patients with COPD in other settings,[21, 22] but is far lower than quit rates observed after admission for acute myocardial infarction.[23, 24, 25] Although the proportion of patients treated at the time of discharge or within 90 days was low, our findings are in keeping with previous studies, which demonstrated low rates of pharmacologic treatment following hospitalization, averaging 14%.[26] Treatment for tobacco use is likely underutilized for this group of high‐risk smokers. However, a significant proportion of patients who were prescribed medications in the postdischarge period did not have medications filled. This likely reflects both the rapid changes in motivation that characterize quit attempts,[27] as well as efforts on the part of primary care physicians to make these medications available to facilitate future quit attempts.
There are several possible explanations for the findings in our study. Pharmaceutical therapies were not provided at random. The provision of pharmacotherapy and the ultimate success of a quit attempt reflects a complex interaction of patient beliefs concerning medications, level of addiction and motivation, physician behavior and knowledge, and organizational factors. Organizational factors such as the structure of electronic discharge orders and the availability of decision support materials may influence a physician's likelihood of prescribing medications, the choice of medication prescribed, and therefore the adequacy of control of withdrawal symptoms. NRT is often under dosed to control ongoing symptoms,[28] and needs to be adjusted until relief is obtained, providing an additional barrier to effectiveness during the transition out of the hospital. Because most smokers with COPD are highly addicted to nicotine,[29] high‐dose NRT, combination therapy, or varenicline would be necessary to adequately control symptoms.[30] However, a significant minority of patients received short‐acting NRT alone.
Despite a high observed efficacy in recent trials,[31, 32] few subjects in our study received varenicline. This may be related to both secular trends and administrative barriers to the use of varenicline in the VA system. Use of this medication was limited among patients with psychiatric disorders due to safety concerns. These concerns have since been largely disproven, but may have limited access to this medication.[33, 34, 35] Although we adjusted for a history of mental illness, patients who received varenicline may have had more past quit attempts and less active mental illness, which may be associated with improved cessation rates. Despite the high prevalence of mental illness we observed, this is typical of the population of smokers, with studies indicating nearly one‐third of smokers overall suffer from mental illness.[36]
Although the majority of our patients received a brief, nurse‐based counseling intervention, there is considerable concern about the overall effectiveness of a single predischarge interaction to produce sustained smoking cessation among highly addicted smokers.[37, 38, 39, 40] The Joint Commission has recently restructured the requirements for smoking cessation treatment for hospitalized patients, and it is now up to hospitals to implement treatment mechanisms that not only meet the national requirements, but also provide a meaningful clinical effect. Though the optimum treatment for hospitalized smokers with COPD is unknown, previous positive studies of smoking cessation among hospitalized patients underscore the need for a higher‐intensity counseling intervention that begins during hospitalization and continues after discharge.[13, 41] Cessation counseling services including tobacco cessation groups and quit lines are available through the VA; however, the use of these services is typically low and requires the patient to enroll independently after discharge, an additional barrier. The lack of association between medications and smoking cessation found in our study could reflect poor effectiveness of medications in the absence of a systematic counseling intervention. Alternatively, the association may be explained that patients who were more highly addicted and perhaps less motivated to quit received tobacco cessation medications more often, but were also less likely to stop tobacco use, a form of indication bias.
Our study has several limitations. We do not have addiction or motivation levels for a cessation attempt, a potential unmeasured confounder. Although predictive of quit attempts, motivation factors are less predictive of cessation maintenance, and may therefore have an unclear effect on our outcome.[42, 43] Our outcome was gathered as part of routine clinical care, which may have introduced bias if patients over‐reported cessation because of social desirability. In healthcare settings, however, this form of assessing smoking status is generally valid.[44] Exposure to counseling or medications obtained outside of the VA system would not have been captured. Given the financial incentive, we believe it is unlikely that many patients admitted to a VA medical center obtained medications elsewhere.[45] The diagnosis of COPD was made administratively. However, all subjects were admitted for an exacerbation, which is associated with more severe COPD by Global Initiative for Obstructive Lung Disease (GOLD) stage.[46] Patients with more severe COPD are often excluded from studies of smoking cessation due to concerns of high dropout and lower prevalence of smoking among patients with GOLD stage IV disease,[47, 48] making this a strength of our study. Subjects who died may have quit only in extremis, or failed to document their quit attempts. However, our sensitivity analysis limited to survivors did not change the study results. There may have been some misclassification in the use of buproprion, which may also be prescribed as an antidepressant. Finally, although representative of the veterans who seek care within the VISN‐20, our patients were primarily white and male, limiting the ability to generalize outside of this group.
Our study had several strengths. We examined a large cohort of patients admitted to a complete care organization, including patients from a diverse group of VA settings comprising academically and nonacademically affiliated centers. We performed an unbiased collection of patients, including all smokers discharged for COPD. We had access to excellent completeness of medications prescribed and filled as collected within the VA system, enabling us to observe medications dispensed and prescribed at several time points. We also had near complete ascertainment of outcomes including by using natural language processing with manual confirmation of smoking status.
In summary, we found that provision of medications to treat ongoing tobacco use among patients discharged for COPD was low, and receipt of medications was not associated with a reduction in smoking tobacco at 6 to 12 months postdischarge. However, among those treated, varenicline appears to be superior to the nicotine patch, with short‐acting nicotine replacement potentially less effective, a biologically plausible finding. The motivation to quit smoking changes rapidly over time. Providing these medications in the hospital and during the time after discharge is a potential means to improve quit rates, but medications need to be paired with counseling to be most effective. Collectively, these data suggest that systems‐based interventions are needed to increase the availability of intense counseling and the use of tailored pharmacotherapy to these patients.
Acknowledgements
The authors acknowledge Mr. Robert Plumley, who performed the data extraction and natural language processing necessary to complete this project.
Disclosures: Dr. Melzer conceived of the research question and performed background reading, analyses, primary drafting, and final revision of the manuscript. Drs. Collins and Feemster participated in finalizing the research question, developing the cohort, performing data collection, and revising the manuscript. Dr. Au provided the database for analysis, helped finalize the research question, and assisted in interpretation of the data and revision of the manuscript. Dr. Au has personally reviewed the data, understands the statistical methods employed, and confirms an understanding of this analysis, that the methods are clearly described, and that they are a fair way to report the results. This material is based upon work supported in part by the Department of Veterans Affairs, Veterans Health Administration, Office of Research and Development, who provided access to data, office space, and programming and data management. The views expressed in this article are those of the authors and do not necessarily reflect the position or policy of the Department of Veterans Affairs, the United States government, or the National Institutes of Health. Dr. Au is an unpaid research consultant for Analysis Group. None of the other authors have any conflicts of interest to disclose. Dr. Melzer is supported by an institutional F‐32 (HL007287‐36) through the University of Washington Department of Pulmonary and Critical Care. Dr. Feemster is supported by an National Institutes of Health, National Heart, Lung, and Blood Institute, K23 Mentored Career Development Award (HL111116). Partial support of this project was provided by Gilead Sciences with research funding to the Seattle Institute for Biomedical and Clinical Research. Additional support was received through the VA Health Services Research and Development. A portion of this work was presented in abstract form at the American Thoracic Society International Meeting, May 2015, in Denver, Colorado.
- Patients hospitalized for COPD have a high prevalence of modifiable risk factors for exacerbation (EFRAM study). Eur Respir J. 2000;16(6):1037–1042. , , , , , .
- Analysis of hospitalizations for COPD exacerbation: opportunities for improving care. COPD. 2010;7(2):85–92. , , , et al.
- Mortality in COPD: role of comorbidities. Eur Respir J. 2006;28(6):1245–1257. , , , .
- Cardiovascular comorbidity in COPD: systematic literature review. Chest. 2013;144(4):1163–1178. , , , .
- Engaging patients and clinicians in treating tobacco addiction. JAMA Intern Med. 2014;174(8):1299–1300. .
- Smokers who are hospitalized: a window of opportunity for cessation interventions. Prev Med. 1992;21(2):262–269. , .
- Interventions for smoking cessation in hospitalised patients. Cochrane Database Syst Rev. 2012;5:CD001837. , , , .
- Specifications Manual for National Hospital Inpatient Quality Measures. Available at: http://www.jointcommission.org/specifications_manual_for_national_hospital_inpatient_quality_measures.aspx. Accessed January 15, 2015.
- Treating Tobacco Use and Dependence. April 2013. Agency for Healthcare Research and Quality, Rockford, MD. Available at: http://www.ahrq.gov/professionals/clinicians‐providers/guidelines‐recommendations/tobacco/clinicians/update/index.html. Accessed January 15, 2015.
- Smoking cessation advice rates in US hospitals. Arch Intern Med. 2011;171(18):1682–1684. , , , .
- Validating smoking data from the Veteran's Affairs Health Factors dataset, an electronic data source. Nicotine Tob Res. 2011;13(12):1233–1239. , , , et al.
- Do u smoke after txt? Results of a randomised trial of smoking cessation using mobile phone text messaging. Tob Control. 2005;14(4):255–261. , , , et al.
- The effectiveness of smoking cessation groups offered to hospitalised patients with symptoms of exacerbations of chronic obstructive pulmonary disease (COPD). Clin Respir J. 2008;2(3):158–165. , , , .
- Sustained care intervention and postdischarge smoking cessation among hospitalized adults: a randomized clinical trial. JAMA. 2014;312(7):719–728. , , , et al.
- Bupropion for smokers hospitalized with acute cardiovascular disease. Am J Med. 2006;119(12):1080–1087. , , , et al.
- Adapting a clinical comorbidity index for use with ICD‐9‐CM administrative databases. J Clin Epidemiol. 1992;45(6):613–619. , , .
- Multiple Imputation for Nonresponse in Surveys. New York, NY: Wiley; 1987. .
- Methods for constructing and assessing propensity scores. Health Serv Res. 2014;49(5):1701–1720. , , , et al.
- Mortality and mortality‐related factors after hospitalization for acute exacerbation of COPD. Chest. 2003;124(2):459–467. , , .
- Mortality after hospitalization for COPD. Chest. 2002;121(5):1441–1448. , , , et al.
- State quitlines and cessation patterns among adults with selected chronic diseases in 15 states, 2005–2008. Prev Chronic Dis. 2012;9(10):120105. , , , , , .
- The effects of counseling on smoking cessation among patients hospitalized with chronic obstructive pulmonary disease: a randomized clinical trial. Int J Addict. 1991;26(1):107–119. , , .
- Predictors of smoking cessation after a myocardial infarction: the role of institutional smoking cessation programs in improving success. Arch Intern Med. 2008;168(18):1961–1967. , , , et al.
- Post‐myocardial infarction smoking cessation counseling: associations with immediate and late mortality in older Medicare patients. Am J Med. 2005;118(3):269–275. , , , , , .
- Smoking cessation after acute myocardial infarction: effects of a nurse‐‐managed intervention. Ann Intern Med. 1990;113(2):118–123. , , , .
- Smoking care provision in hospitals: a review of prevalence. Nicotine Tob Res. 2008;10(5):757–774. , , , et al.
- Intentions to quit smoking change over short periods of time. Addict Behav. 2005;30(4):653–662. , , , .
- Association of amount and duration of NRT use in smokers with cigarette consumption and motivation to stop smoking: a national survey of smokers in England. Addict Behav. 2015;40(0):33–38. , , , , .
- Smoking prevalence, behaviours, and cessation among individuals with COPD or asthma. Respir Med. 2011;105(3):477–484. , .
- American College of Chest Physicians. Tobacco Dependence Treatment ToolKit. 3rd ed. Available at: http://tobaccodependence.chestnet.org. Accessed January 29, 2015. , , , et al.
- Effects of varenicline on smoking cessation in patients with mild to moderate COPD: a randomized controlled trial. Chest. 2011;139(3):591–599. , , , , , .
- Varenicline versus transdermal nicotine patch for smoking cessation: results from a randomised open‐label trial. Thorax. 2008;63(8):717–724. , , , et al.
- Psychiatric adverse events in randomized, double‐blind, placebo‐controlled clinical trials of varenicline. Drug Saf. 2010;33(4):289–301. , , , , .
- Studies linking smoking‐cessation drug with suicide risk spark concerns. JAMA. 2009;301(10):1007–1008. .
- A randomized, double‐blind, placebo‐controlled study evaluating the safety and efficacy of varenicline for smoking cessation in patients with schizophrenia or schizoaffective disorder. J Clin Psychiatry. 2012;73(5):654–660. , , , et al.
- Smoking and mental illness: results from population surveys in Australia and the United States. BMC Public Health. 2009;9(1):285. , , .
- Implementation and effectiveness of a brief smoking‐cessation intervention for hospital patients. Med Care. 2000;38(5):451–459. , , , .
- Clinical trial comparing nicotine replacement therapy (NRT) plus brief counselling, brief counselling alone, and minimal intervention on smoking cessation in hospital inpatients. Thorax. 2003;58(6):484–488. , , , et al.
- Dissociation between hospital performance of the smoking cessation counseling quality metric and cessation outcomes after myocardial infarction. Arch Intern Med. 2008;168(19):2111–2117. , , , et al.
- Smoking cessation in hospitalized patients: Results of a randomized trial. Arch Intern Med. 1997;157(4):409–415. , , , , .
- Intensive smoking cessation counseling versus minimal counseling among hospitalized smokers treated with transdermal nicotine replacement: a randomized trial. Am J Med. 2003;114(7):555–562. , , , , .
- Motivational factors predict quit attempts but not maintenance of smoking cessation: findings from the International Tobacco Control Four country project. Nicotine Tob Res. 2010;12(suppl):S4–S11. , , , et al.
- Predictors of attempts to stop smoking and their success in adult general population samples: a systematic review. Addiction. 2011;106(12):2110–2121. , , , , .
- Validity of self‐reported smoking status among participants in a lung cancer screening trial. Cancer Epidemiol Biomarkers Prev. 2006;15(10):1825–1828. , , , et al.
- VHA enrollees' health care coverage and use of care. Med Care Res Rev. 2003;60(2):253–67. , , , .
- Association between lung function and exacerbation frequency in patients with COPD. Int J Chron Obstruct Pulmon Dis. 2010;5:435–444. , , , , .
- Smoking cessation in patients with chronic obstructive pulmonary disease: a double‐blind, placebo‐controlled, randomised trial. Lancet. 2001;357(9268):1571–1575. , , , et al.
- Nurse‐conducted smoking cessation in patients with COPD using nicotine sublingual tablets and behavioral support. Chest. 2006;130(2):334–342. , , .
- Patients hospitalized for COPD have a high prevalence of modifiable risk factors for exacerbation (EFRAM study). Eur Respir J. 2000;16(6):1037–1042. , , , , , .
- Analysis of hospitalizations for COPD exacerbation: opportunities for improving care. COPD. 2010;7(2):85–92. , , , et al.
- Mortality in COPD: role of comorbidities. Eur Respir J. 2006;28(6):1245–1257. , , , .
- Cardiovascular comorbidity in COPD: systematic literature review. Chest. 2013;144(4):1163–1178. , , , .
- Engaging patients and clinicians in treating tobacco addiction. JAMA Intern Med. 2014;174(8):1299–1300. .
- Smokers who are hospitalized: a window of opportunity for cessation interventions. Prev Med. 1992;21(2):262–269. , .
- Interventions for smoking cessation in hospitalised patients. Cochrane Database Syst Rev. 2012;5:CD001837. , , , .
- Specifications Manual for National Hospital Inpatient Quality Measures. Available at: http://www.jointcommission.org/specifications_manual_for_national_hospital_inpatient_quality_measures.aspx. Accessed January 15, 2015.
- Treating Tobacco Use and Dependence. April 2013. Agency for Healthcare Research and Quality, Rockford, MD. Available at: http://www.ahrq.gov/professionals/clinicians‐providers/guidelines‐recommendations/tobacco/clinicians/update/index.html. Accessed January 15, 2015.
- Smoking cessation advice rates in US hospitals. Arch Intern Med. 2011;171(18):1682–1684. , , , .
- Validating smoking data from the Veteran's Affairs Health Factors dataset, an electronic data source. Nicotine Tob Res. 2011;13(12):1233–1239. , , , et al.
- Do u smoke after txt? Results of a randomised trial of smoking cessation using mobile phone text messaging. Tob Control. 2005;14(4):255–261. , , , et al.
- The effectiveness of smoking cessation groups offered to hospitalised patients with symptoms of exacerbations of chronic obstructive pulmonary disease (COPD). Clin Respir J. 2008;2(3):158–165. , , , .
- Sustained care intervention and postdischarge smoking cessation among hospitalized adults: a randomized clinical trial. JAMA. 2014;312(7):719–728. , , , et al.
- Bupropion for smokers hospitalized with acute cardiovascular disease. Am J Med. 2006;119(12):1080–1087. , , , et al.
- Adapting a clinical comorbidity index for use with ICD‐9‐CM administrative databases. J Clin Epidemiol. 1992;45(6):613–619. , , .
- Multiple Imputation for Nonresponse in Surveys. New York, NY: Wiley; 1987. .
- Methods for constructing and assessing propensity scores. Health Serv Res. 2014;49(5):1701–1720. , , , et al.
- Mortality and mortality‐related factors after hospitalization for acute exacerbation of COPD. Chest. 2003;124(2):459–467. , , .
- Mortality after hospitalization for COPD. Chest. 2002;121(5):1441–1448. , , , et al.
- State quitlines and cessation patterns among adults with selected chronic diseases in 15 states, 2005–2008. Prev Chronic Dis. 2012;9(10):120105. , , , , , .
- The effects of counseling on smoking cessation among patients hospitalized with chronic obstructive pulmonary disease: a randomized clinical trial. Int J Addict. 1991;26(1):107–119. , , .
- Predictors of smoking cessation after a myocardial infarction: the role of institutional smoking cessation programs in improving success. Arch Intern Med. 2008;168(18):1961–1967. , , , et al.
- Post‐myocardial infarction smoking cessation counseling: associations with immediate and late mortality in older Medicare patients. Am J Med. 2005;118(3):269–275. , , , , , .
- Smoking cessation after acute myocardial infarction: effects of a nurse‐‐managed intervention. Ann Intern Med. 1990;113(2):118–123. , , , .
- Smoking care provision in hospitals: a review of prevalence. Nicotine Tob Res. 2008;10(5):757–774. , , , et al.
- Intentions to quit smoking change over short periods of time. Addict Behav. 2005;30(4):653–662. , , , .
- Association of amount and duration of NRT use in smokers with cigarette consumption and motivation to stop smoking: a national survey of smokers in England. Addict Behav. 2015;40(0):33–38. , , , , .
- Smoking prevalence, behaviours, and cessation among individuals with COPD or asthma. Respir Med. 2011;105(3):477–484. , .
- American College of Chest Physicians. Tobacco Dependence Treatment ToolKit. 3rd ed. Available at: http://tobaccodependence.chestnet.org. Accessed January 29, 2015. , , , et al.
- Effects of varenicline on smoking cessation in patients with mild to moderate COPD: a randomized controlled trial. Chest. 2011;139(3):591–599. , , , , , .
- Varenicline versus transdermal nicotine patch for smoking cessation: results from a randomised open‐label trial. Thorax. 2008;63(8):717–724. , , , et al.
- Psychiatric adverse events in randomized, double‐blind, placebo‐controlled clinical trials of varenicline. Drug Saf. 2010;33(4):289–301. , , , , .
- Studies linking smoking‐cessation drug with suicide risk spark concerns. JAMA. 2009;301(10):1007–1008. .
- A randomized, double‐blind, placebo‐controlled study evaluating the safety and efficacy of varenicline for smoking cessation in patients with schizophrenia or schizoaffective disorder. J Clin Psychiatry. 2012;73(5):654–660. , , , et al.
- Smoking and mental illness: results from population surveys in Australia and the United States. BMC Public Health. 2009;9(1):285. , , .
- Implementation and effectiveness of a brief smoking‐cessation intervention for hospital patients. Med Care. 2000;38(5):451–459. , , , .
- Clinical trial comparing nicotine replacement therapy (NRT) plus brief counselling, brief counselling alone, and minimal intervention on smoking cessation in hospital inpatients. Thorax. 2003;58(6):484–488. , , , et al.
- Dissociation between hospital performance of the smoking cessation counseling quality metric and cessation outcomes after myocardial infarction. Arch Intern Med. 2008;168(19):2111–2117. , , , et al.
- Smoking cessation in hospitalized patients: Results of a randomized trial. Arch Intern Med. 1997;157(4):409–415. , , , , .
- Intensive smoking cessation counseling versus minimal counseling among hospitalized smokers treated with transdermal nicotine replacement: a randomized trial. Am J Med. 2003;114(7):555–562. , , , , .
- Motivational factors predict quit attempts but not maintenance of smoking cessation: findings from the International Tobacco Control Four country project. Nicotine Tob Res. 2010;12(suppl):S4–S11. , , , et al.
- Predictors of attempts to stop smoking and their success in adult general population samples: a systematic review. Addiction. 2011;106(12):2110–2121. , , , , .
- Validity of self‐reported smoking status among participants in a lung cancer screening trial. Cancer Epidemiol Biomarkers Prev. 2006;15(10):1825–1828. , , , et al.
- VHA enrollees' health care coverage and use of care. Med Care Res Rev. 2003;60(2):253–67. , , , .
- Association between lung function and exacerbation frequency in patients with COPD. Int J Chron Obstruct Pulmon Dis. 2010;5:435–444. , , , , .
- Smoking cessation in patients with chronic obstructive pulmonary disease: a double‐blind, placebo‐controlled, randomised trial. Lancet. 2001;357(9268):1571–1575. , , , et al.
- Nurse‐conducted smoking cessation in patients with COPD using nicotine sublingual tablets and behavioral support. Chest. 2006;130(2):334–342. , , .
© 2015 Society of Hospital Medicine
Dyspnea Assessment and Management Survey
Dyspnea, defined as a subjective experience of breathing discomfort,[1] is the seventh most frequent reason adult patients present to the emergency room and the most frequent cause for emergency room visits in patients 65 years or older.[2] Moreover, dyspnea is experienced by 49% of patients hospitalized with a medical condition[3, 4, 5] and by 70% of patients who are seriously ill.[6]
Based on evidence that patients are not treated consistently and effectively for relief of their shortness of breath, the American College of Chest Physicians (ACCP) statement on dyspnea management in patients with advanced lung or heart disease recommended that patients should be asked to rate their dyspnea, and the rating should be routinely documented in the medical record to guide management.[7] Although clinicians may question the utility of routine assessment of dyspnea using a standardized scale, studies have found that the prevalence of dyspnea reported from chart review is much lower than when patients are directly interviewed.[8] This may be the result of underrecognition of dyspnea or poor documentation by physicians, or that patients may not communicate their symptoms unless the physician specifically asks. As is the case with pain, routine assessment of dyspnea severity could lead to improved clinical management and greater patient‐centered care. However, unlike in the case of pain, regulatory bodies, such as the Joint Commission for Accreditation of Healthcare Organization, do not require routine dyspnea assessment.[9]
Currently, there are more than 40,000 hospitalists in the United States, and the vast majority of hospitals with >200 beds have a hospitalist group.[10] Hospitalists care for over 60% of inpatients[11] and play a major role in the management of patients with acute cardiopulmonary diseases. If standardized approaches for the assessment and documentation of dyspnea are to be implemented, hospitalists would be a key stakeholder group for utilizing enhanced clinical information about dyspnea. Therefore, we evaluated attitudes and practices of hospitalists in regard to the assessment and management of dyspnea, including the potential benefits and challenges related to the implementation of standardized assessment. We hypothesized that hospitalists would believe that a dyspnea scale for assessment of severity could improve their management of patients with cardiovascular diseases. Further, we hypothesized that physicians who agreed with the general statement that dyspnea is an important clinical problem would be more likely to believe that routine dyspnea assessment would be valuable.
METHODS
Study Sample
We invited 255 attending hospitalists from 9 geographically and structurally diverse hospitals to complete a survey about the assessment and management of dyspnea. The 9 hospitals represent range of practice environments including 4 academic medical centers, 2 community teaching and 3 nonteaching hospitals, 1 Veterans Administration hospital, and 2 staff‐model HMOs (see Supporting Table 1 in the online version of this article). The survey was distributed online using REDCap (Research Electronic Data Capture), a secure web‐based interface application.[12] A coinvestigator who was a pulmonary critical‐care physician at each site sent an initial email to their hospitalist groups that alerted them to expect a survey from the principal investigator. This notification was subsequently followed by an email invitation containing an informational cover letter and a link to the online survey. The cover letter stated that the completed surveys would not be stored at the local sites and that all the analyzed data would be deidentified. Nonrespondents were sent reminders at 2 and 4 weeks after the initial mailing. A $25 electronic gift card was provided as a gesture of appreciation for their time. The survey was conducted between September 2013 and December 2013.
The study was approved by the Baystate Health Institutional Review Board, Springfield, Massachusetts, with a waiver for written informed consent.
Questionnaire
We developed a 17‐item instrument based on a review of the dyspnea literature and a prior ACCP survey.[12] Questions were piloted with 4 hospitalists at a single institution and modified to improve face validity and clarity (see Supporting Information in the online version of this article for the full survey).
Hospitalists were asked to consider the care of patients admitted for acute cardiopulmonary disease, including heart failure, chronic obstructive pulmonary disease, and pneumonia. A series of 5‐point Likert scales were used to assess the respondents level of agreement with statements related to the following domains: the importance of dyspnea in clinical care, the potential benefits and challenges of routine dyspnea assessment (statements such as: Having a standardized assessment of dyspnea severity would be helpful in management of patients with cardiopulmonary diseases. Dyspnea assessment by a scale should be part of the vital signs for patients with cardiopulmonary diseases.), and management of dyspnea (questions regarding the use of opioids and other nonpharmacological therapies). Additional questions were asked about current assessment practices (questions such as: How often do you assess severity of dyspnea? What is your approach in assessing dyspnea? with options of choosing a categorical or numerical scale), if dyspnea is assessed in their institution by nurses and how often, and the influence of dyspnea severity assessment on their management. The survey had 1 question that solicited comments from the participants: If you don't think that it would be useful to have a standardized dyspnea assessment, please tell us why.
Data Analysis
Responses to survey questions were summarized via counts and percentages in each response category. Adopting the methodology used in the ACCP consensus statement, strongly agree and somewhat agree were combined into a single category of agreement. We also presented percentage of responses in the 2 levels of agreement (strongly agree and somewhat agree) for each question in a bar graph.
Associations between tertiles of physicians' time in practice and attitude toward dyspnea were evaluated via 2 or Fisher exact test.
To examine how answers to the first 2 questions, which assessed attitude toward importance of dyspnea in clinical care, affect answers to the remaining questions, we grouped respondents in 3 categories (strongly agree, agree to these questions, do not agree) and tested the associations using 2 or Fisher exact test.
All analyses were performed using SAS version 9.3 (SAS institute, Inc., Cary, NC) and Stata release 13.1 (StataCorp, College Station, TX).
RESULTS
Overall, 178 (69.8%) of 255 identified hospitalists completed the survey, and all 9 participating hospitals had a response rate greater than 50%. The median number of years in practice was 6 (range, 038 years). A majority (77.5%) of respondents agreed with the statement that dyspnea is 1 of the major symptoms of patients with cardiopulmonary disease, and that its treatment is central to the management of these patients (77.0%) (Figure 1).
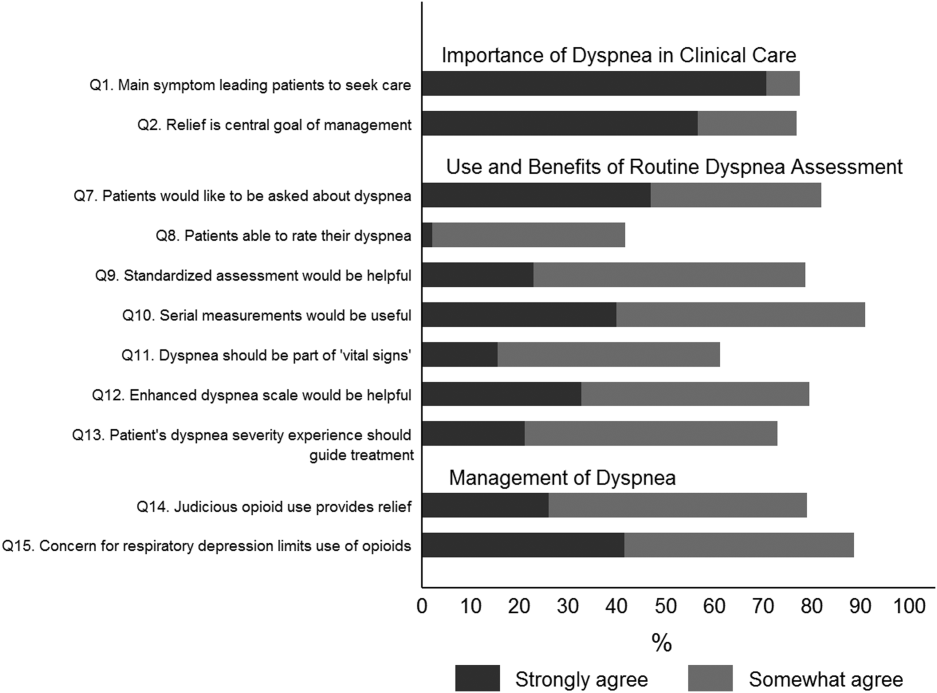
Attitude and Practices Surrounding Dyspnea Assessment
When asked about their current assessment of dyspnea, a majority (84.3%) of the hospitalists stated that they assess dyspnea on a daily basis; two‐thirds indicated that they use a categorical scale (ie, no shortness of breath, improved or worsened compared with a prior date), and one‐third indicated that they ask whether the patient is dyspneic or not. Fifty‐six percent of hospitalists stated that dyspnea is regularly assessed by nurses in their hospital.
The majority of respondents agreed (78.6%, 23.0% strongly and 55.6% somewhat agree) that standardized assessment of dyspnea severity, using a numeric scale and serial measurements as part of the vital signs, would benefit the management of patients with cardiopulmonary diseases. Furthermore, 79.6% (33.0% strongly and 46.6% somewhat agree) reported that using a dyspnea scale that included information to further characterize the patient‐reported experience, such as the level of distress associated with dyspnea, would be helpful in management.
Approximately 90% of the hospitalists indicated that awareness of dyspnea severity has an influence on clinical decision making, including whether to intensify treatment of underlying conditions, to pursue additional diagnostic testing, or to modify discharge timing. Additionally, two‐thirds of hospitalists agreed that awareness of dyspnea severity influences their decision to add opioids, whereas only one‐third prescribed nonpharmacologic symptom‐oriented treatment (Table 1).
Frequency (%) | |
---|---|
| |
When caring for patients with acute cardiopulmonary diseases, how often do you assess severity of dyspnea?* | |
At admission | 66 (37.1) |
At discharge | 59 (33.2) |
Daily until discharge | 150 (84.3) |
More often than daily | 58 (32.6) |
Which description best characterizes your approach to assessing dyspnea severity? | |
I don't regularly ask about dyspnea severity | 3 (1.7) |
I ask the patient whether or not they are having shortness of breath | 50 (28.3) |
I ask the patient to rate the severity of shortness of breath using a numeric scale | 4 (2.3) |
I ask the patient to rate the severity of shortness of breath using a categorical scale (eg, somewhat SOB, no SOB, improved or worsened compared with a prior date) | 120 (67.8) |
When is dyspnea severity assessed and documented by nursing at your hospital?* | |
Dyspnea is not routinely assessed | 60 (33.7) |
At admission | 30 (16.9) |
Daily | 43 (24.2) |
Each shift | 64 (36.0) |
Awareness of dyspnea severity affects my management by:* | |
Influencing my decision to intensify treatment of the patient's underlying condition | 170 (95.5) |
Influencing my decision to pursue additional diagnostic testing | 160 (89.9) |
Influencing my decision to add pharmacologic‐based, symptom‐oriented treatment for dyspnea, such as opioids | 115 (64.6) |
Influencing my decision to add nonpharmacologic‐based, symptom‐oriented treatment for dyspnea, such as fans or pursed lip breathing technique | 58 (32.6) |
Influencing my decision regarding timing of discharge | 162 (91.0) |
Which of the following nonpharmacologic therapies are effective for the relief of dyspnea?* | |
Pursed lip breathing | 113 (63.5) |
Relaxation techniques | 137 (77.0) |
Noninvasive ventilation | 143 (80.3) |
O2 for nonhypoxemic patients | 89 (50.0) |
Cool air/fan | 125 (70.2) |
Cognitive behavioral strategies | 101 (56.7) |
Forty‐two percent of the respondents agreed that patients are able to rate their dyspnea on a scale (2.3% strongly agree and 40.0% agree), and 73.0% indicated that patient experience of dyspnea should guide management independent of physiologic measures such as respiratory rate and oxygen saturation (Figure 1).
Several potential barriers were identified among the 18 participants who did not think that a standardized assessment of dyspnea would be beneficial, including concerns that (1) a dyspnea severity scale is too subjective and numerical scales are not useful for a subjective symptom (19.0%), (2) patients may overrate their symptom or will not be able to rate their dyspnea using a scale (31.0%), or (3) categorical description is sufficient (31.0%).
Practices in Dyspnea Management
Seventy‐nine percent of respondents agreed with the statement that judicious use of opioids can provide relief of dyspnea (26.1% strongly and 52.8% agreed), and 88.7% hospitalists identified the risk of respiratory depression as 1 of the barriers for the limited use of opioids. The majority of physicians (60%80%) considered nonpharmacologic therapies effective for symptomatic treatment of dyspnea, including in the order of agreement: noninvasive ventilation, relaxation techniques, cool air/fan, use of pursed lip breathing, and oxygen for nonhypoxemic patients (Table 1).
Physician Experience and Attitudes Toward Dyspnea Management
When we stratified hospitalists in tertiles of median years of time in practice (median [range]: 2 [04], 6 [58] and 15 [938]), we did not find an association with any of the responses to the questions.
Attitude Regarding the Importance of Dyspnea in Clinical Care and Responses to Subsequent Questions
Respondents who strongly agree or agree that dyspnea is the primary presenting symptom in patients with cardiovascular condition and that dyspnea relief is central to the management of these patients were more likely to believe that patients would like to be asked about their dyspnea (61.2% vs 30.2% vs 29.7%). They also had a more positive attitude about the usefulness of a standardized assessment of dyspnea and the inclusion of the assessment of dyspnea by a scale in the vital signs (Table 2).
Description | Do Not Agree, n (%) | Somewhat Agree, n (%) | Strongly Agree, n (%) | P Value* |
---|---|---|---|---|
| ||||
37 (20.9) | 43 (24.3) | 97 (54.8) | ||
Which description best characterizes your approach to assessing dyspnea severity? | 0.552 | |||
I don't regularly ask about dyspnea severity | 0 (0) | 0 (0) | 3 (3.1) | |
I ask the patient whether or not they are having shortness of breath | 11 (29.7) | 14 (32.6) | 25 (25.8) | |
I ask the patient to rate the severity of shortness of breath using a numeric scale | 2 (5.4) | 1 (2.3) | 1 (1.0) | |
I ask the patient to rate the severity of shortness of breath using a categorical scale (e.g., somewhat shortness of breath, no shortness of breath, improved or worsened compared with a prior date) | 24 (64.9) | 28 (65.1) | 68 (70.1) | |
Patients would like me to ask them about their dyspnea. | <0.0001 | |||
Somewhat agree | 9 (24.3) | 21 (48.8) | 32 (32.7) | |
Strongly agree | 11 (29.7) | 13 (30.2) | 60 (61.2) | |
Patients are able to rate their own dyspnea intensity on a scale of 0‐10. | 0.432 | |||
Somewhat agree | 12 (32.4) | 16 (37.2) | 42 (43.3) | |
Strongly agree | 2 (5.4) | 0 (0) | 2 (2.1) | |
Having a standardized assessment of dyspnea severity would be helpful to me in management of patients with cardiopulmonary diseases. | 0.026 | |||
Somewhat agree | 17 (46.0) | 25 (58.1) | 57 (58.2) | |
Strongly agree | 7 (18.9) | 6 (14.0) | 28 (28.6) | |
Serial measurements of dyspnea would be useful for assessing response to therapy. | 0.042 | |||
Somewhat agree | 14 (37.8) | 28 (65.1) | 48 (49.5) | |
Strongly agree | 16 (43.2) | 12 (27.9) | 43 (44.3) | |
Dyspnea assessment by a scale should be part of the vital signs for patients with cardiopulmonary diseases. | 0.042 | |||
Somewhat agree | 13 (35.1) | 17 (39.5) | 51 (52.0) | |
Strongly agree | 4 (10.8) | 5 (11.6) | 19 (19.4) | |
Using an enhanced dyspnea scale that includes information about the following 4 features 1) Current dyspnea severity, 2) Worst dyspnea ever, 3) Improvement of dyspnea since admission, 4) Acceptability of current level of dyspnea, would be more helpful for my management than a single question focused on dyspnea severity. | 0.03 | |||
Somewhat agree | 14 (40.0) | 24 (55.8) | 44 (44.9) | |
Strongly agree | 9 (25.7) | 9 (20.9) | 40 (40.8) | |
The patients experience of dyspnea should be used to guide treatment decisions independent of objective measures such as respiratory rate and oxygen saturation. | 0.10 | |||
Somewhat agree | 20 (54.0) | 21 (48.8) | 51 (52.0) | |
Strongly agree | 5 (13.5) | 6 (14.0) | 27 (27.6) | |
Judicious use of oral and/or parenteral opioids can provide relief of dyspnea. | 0.21 | |||
Somewhat agree | 20 (54.0) | 23 (54.8) | 50 (51.6) | |
Strongly agree | 10 (27.0) | 6 (14.3) | 30 (30.9) | |
Limited use of opioids for relief of dyspnea in patients with advanced cardiopulmonary disorders is often due to concerns of respiratory depression. | 0.71 | |||
Somewhat agree | 17 (46.0) | 23 (54.8) | 43 (43.9) | |
Strongly agree | 15 (40.5) | 14 (33.3) | 45 (45.9) |
DISCUSSION
In this survey of 178 most hospitalists from a diverse group of 9 US hospitals, we found that most indicate that severity of dyspnea has a profound influence on their clinical practice (including their decision whether to intensify treatments such as diuretics or bronchodilators, to pursue additional diagnostic testing, add opioids or other nonpharmacological treatments) and ultimately their decision regarding the timing of hospital discharge. More importantly, whereas less than half reported experience with standardized assessment of dyspnea severity, most stated that such data would be very useful in their practice.
Despite being a highly prevalent symptom in diverse patient populations, several studies have shown that documentation of dyspnea is sporadic and evaluation of dyspnea quality of care is not routinely performed.[13, 14, 15] Statements from a number of professional societies, including the ACCP, the American Thoracic Society and the Canadian Respiratory Society, recommend that dyspnea management should rely on patient reporting, and that dyspnea severity should be recorded.[1, 4, 7] Assessment is an essential step to guide interventions; however, simply asking about the presence or absence of dyspnea is insufficient.
Several rating scales have been validated and might be implementable in the acute care setting, including the Numerical Rating Scale and the Visual Assessment Scale.[16, 17, 18, 19, 20] Our survey shows that standardized documentation of dyspnea severity in clinical practice is uncommon. However, most hospitalists in our study believed that assessment of dyspnea, using a standardized scale, would positively impact their management of patients with cardiopulmonary disease.
There are a number of potential benefits of routine assessment of dyspnea in hospitalized patients. Implementation of a standardized approach to dyspnea measurement would result in more uniform assessment and documentation practices, and in turn greater awareness among members of the patient‐care team. Though not sufficient to improve care, measurement is necessary because physicians do not always recognize the severity of patients' dyspnea or may not recognize its presence. A retrospective study that assessed the prevalence of symptoms in 410 ambulatory patients showed that one‐quarter of patients had dyspnea, but only half of them told their doctor about it.[21] Two other studies of patients with cancer diagnoses found that 30%70% of patients had dyspnea, but the symptom was recognized in only half of them; even when recognized, dyspnea severity was frequently underrated by physicians.[21, 22] Importantly, underestimation appears to correlate with underutilization of symptomatic management of dyspnea.[8]
Although the results of our survey are encouraging, they highlight a number of potential barriers and misconceptions among hospitalists. For example, although dyspnea can be characterized only by the person experiencing it, only 42% of our survey respondents believed that patients are able to rate their dyspnea intensity on a scale. Some of these responses may be influenced by the fact that dyspnea scales are not currently available to patients under their care. Another explanation is that similar to the case for pain, some hospitalists may believe that patients will exaggerate dyspnea severity. Almost one‐third of the respondents stated that objective measures, such as respiratory rate or oxygen saturation, are more important than a patient's experience of dyspnea in guiding the treatment, and that dyspnea is a subjective symptom and not a vital sign itself. Hospitalists who appreciated the importance of dyspnea in clinical practice were more likely to support the implementation of a standardized dyspnea scale for dyspnea assessment.
Although the potential benefits of including routine measurement of dyspnea in standard hospital practice may seem obvious, evidence that implementing routine assessment improves patient care or outcomes is lacking. Even if hospitalists see the value of dyspnea assessment, asking nurses to collect and document additional information would represent a substantial change in hospital workflow. Finally, without specific protocols to guide care, it is unclear whether physicians will be able to use new information about dyspnea severity effectively. Future studies need to evaluate the impact of implementing routine dyspnea assessment on the management of patients with cardiopulmonary diseases including the use of evidence‐based interventions and reducing the use of less valuable care.
Most hospitalists agreed with the basic principles of dyspnea treatment in patients with advanced cardiopulmonary disease after the primary disease had been stabilized. Effective measures are available, and several guidelines endorse opioids in dyspnea management.[1, 4, 7] However, many clinicians are uncomfortable with this approach for dyspnea, and opioids remain underused. In our study, almost 90% of physicians recognized that concerns about respiratory depression limits opioids use as a treatment. A qualitative study that explored the physicians' perspective toward opioids showed that most physicians were reluctant to prescribe opioids for refractory dyspnea, describing a lack of related knowledge and experience, and fears related to the potential adverse effects. The findings of our study also outline the need to better educate residents and hospitalists on the assessment and management of dyspnea, including prescribing opioids for refractory dyspnea.[23]
Study Strengths and Limitations
This study has several strengths. To our knowledge, it is the first to explore hospitalists' perspectives on incorporating dyspnea assessment in their clinical practice. Hospitalists are the attending physicians for a large majority of inpatients and would be the main users of a dyspnea severity scale. Our questionnaire survey included a large number of hospitalists, from 9 geographically and structurally diverse hospitals, which increased the generalizability of the findings to other hospitals around the country.
The study also has several limitations that need be kept in mind in interpreting the study results. First, desirability bias may have exaggerated some of the positive views expressed by hospitalists toward implementation of routine assessment of dyspnea. Second, because this was a survey, the estimates of dyspnea assessment and documentation practices of both physicians and nurses were based on the respondents' perception and not an objective review of medical records, and the results may be different from actual practice. Third, this was not a population‐based random sample of hospitalists, and it may not be entirely representative; however, those surveyed were from a diverse set of sites with different geographical location, size, academic affiliation, and practice environment, and their time in practice varied widely. Last, we do not have information on nonrespondents, and there is a possibility of nonresponse bias, although the high response rate lessens the risk.
CONCLUSIONS
The results of this survey suggest that most hospitalists believe that routine assessment of dyspnea severity would enhance their clinical decision making and improve patient care. Standardized assessment of dyspnea might result in better awareness of this symptom among providers, reduce undertreatment and mistreatment, and ultimately result in better outcomes for patients. However, implementation of the routine assessment of dyspnea would change current clinical practices and may have a significant effect on existing nursing and physician workflows. Additional research is needed to determine the feasibility and impact on outcomes of routine dyspnea assessment.
Acknowledgements
The authors wish to acknowledge Ms. Anu Joshi for her help with editing the manuscript and assisting with table preparations.
Disclosures
Dr. Stefan is supported by grant K01HL114631‐01A1 from the National Heart, Lung, and Blood Institute of the National Institutes of Health, and by the National Center for Research Resources and the National Center for Advancing Translational Sciences, National Institutes of Health, through grant UL1RR025752. The funder had no role in the design and conduct of the study; collection, management, analysis, and interpretation of the data; preparation, review, or approval of the manuscript; and decision to submit the manuscript for publication. M.S.S. and P.K.L. conceived of the study. M.S.S. acquired the data with the help of all collaborators. M.S.S., P.K.L., P.S.P., and A.P. analyzed and interpreted the data. M.S.S. drafted the manuscript. All authors critically reviewed the manuscript for intellectual content. M.S.S., P.K.L., and A.P. had full access to all of the data in the study and take responsibility for the integrity of the data and the accuracy of the data analysis. M.S.S. is the guarantor for this article, and is responsible for the content of the article, including data and analysis. The authors report no conflicts of interest.
- An Official American Thoracic Society Statement: Update on the Mechanisms, Assessment, and Management of Dyspnea. Am J Respir Crit Care Med. 2012;185(4):435–452. , , , et al.
- CDC/ National Center for Health Statistics. National Hospital Amulatory Medical Care Survey: 2011 Emergency Department Summary Tables. http://www.cdc.gov/nchs/data/ahcd/nhamcs_emergency/2011_ed_web_tables.pdf. Accessed May 15, 2015.
- Signs and symptoms of heart failure: are you asking the right questions? Am J Crit Care. 2010;19(5):443–452. , , , .
- Managing dyspnea in patients with advanced chronic obstructive pulmonary disease: a Canadian Thoracic Society clinical practice guideline. Can Respir J. 2011;18(2):69–78. , , , et al.
- Prevalence of distressing symptoms in hospitalised patients on medical wards: A cross‐sectional study. BMC Palliat Care. 2008;7:16. , .
- Dyspnea in terminally ill cancer patients. Chest. 1986;89(2):234–236. , .
- American College of Chest Physicians consensus statement on the management of dyspnea in patients with advanced lung or heart disease. Chest. 2010;137(3):674–691. , , , et al.
- Common symptoms in ambulatory care: incidence, evaluation, therapy, and outcome. Am J Med. 1989;86(3):262–266. , .
- The Joint Commission. Facts about Pain Management. http://www.jointcommission.org/pain_management/. Accessed May, 15, 2015.
- Hospitalist programs in the age of healthcare reform. J Healthc Manag. 2010;55(6):378–380. .
- The Use of Hospitalists by Small Rural Hospitals: Results of a National Survey. Med Care Res Rev. 2014;71(4):356–366. , , , .
- Tufts CTSI. REDCap [Internet]. Tufts Clinical and Translational Science Institute. http://www.tuftsctsi.org/Services-and-Consultation/REDCap.aspx. Accessed May, 15, 2015.
- Multi‐dimensional Assessment of Dyspnea. Dyspnoea in Advanced Disease: A guide to clinical management; 2005. , .
- Cancer care quality measures: symptoms and end‐of‐life care. Evid Rep Technol Assess (Full Rep). 2006(137):1–77. , , , et al.
- Defining and measuring quality palliative and end‐of‐life care in the intensive care unit. Crit Care Med. 2006;34(11 Suppl):S309–316. .
- Validation of a vertical visual analogue scale as a measure of clinical dyspnea. Rehabil Nurs. 1989;14(6):323–325. .
- Can a self‐rating 0‐10 scale for dyspnea yield a common language that is understood by ED nurses, patients, and their families? J Emerg Nurs. 2000;26(3):233–234. .
- Measurement of dyspnea: word labeled visual analog scale vs. verbal ordinal scale. Respir Physiol Neurobiol. 2003;134(2):77–83. , , .
- Verbal numerical scales are as reliable and sensitive as visual analog scales for rating dyspnea in young and older subjects. Respir Physiol Neurobiol. 2007;157(2‐3):360–365. , , , , , .
- Validation of a three‐factor measurement model of dyspnea in hospitalized adults with heart failure. Heart Lung. 2011;41(1):44–56. , , , , .
- Patient reporting and doctor recognition of dyspnoea in a comprehensive cancer centre. Intern Med J. 2006;36(6):381–384. , , .
- Lung cancer and dyspnea: the patient's perception. Oncol Nurs Forum. 1986;13(5):19–24. , , , .
- Opioids, respiratory function, and dyspnea. Am J Hosp Palliat Care. 2003;20(1):57–61. , , , .
Dyspnea, defined as a subjective experience of breathing discomfort,[1] is the seventh most frequent reason adult patients present to the emergency room and the most frequent cause for emergency room visits in patients 65 years or older.[2] Moreover, dyspnea is experienced by 49% of patients hospitalized with a medical condition[3, 4, 5] and by 70% of patients who are seriously ill.[6]
Based on evidence that patients are not treated consistently and effectively for relief of their shortness of breath, the American College of Chest Physicians (ACCP) statement on dyspnea management in patients with advanced lung or heart disease recommended that patients should be asked to rate their dyspnea, and the rating should be routinely documented in the medical record to guide management.[7] Although clinicians may question the utility of routine assessment of dyspnea using a standardized scale, studies have found that the prevalence of dyspnea reported from chart review is much lower than when patients are directly interviewed.[8] This may be the result of underrecognition of dyspnea or poor documentation by physicians, or that patients may not communicate their symptoms unless the physician specifically asks. As is the case with pain, routine assessment of dyspnea severity could lead to improved clinical management and greater patient‐centered care. However, unlike in the case of pain, regulatory bodies, such as the Joint Commission for Accreditation of Healthcare Organization, do not require routine dyspnea assessment.[9]
Currently, there are more than 40,000 hospitalists in the United States, and the vast majority of hospitals with >200 beds have a hospitalist group.[10] Hospitalists care for over 60% of inpatients[11] and play a major role in the management of patients with acute cardiopulmonary diseases. If standardized approaches for the assessment and documentation of dyspnea are to be implemented, hospitalists would be a key stakeholder group for utilizing enhanced clinical information about dyspnea. Therefore, we evaluated attitudes and practices of hospitalists in regard to the assessment and management of dyspnea, including the potential benefits and challenges related to the implementation of standardized assessment. We hypothesized that hospitalists would believe that a dyspnea scale for assessment of severity could improve their management of patients with cardiovascular diseases. Further, we hypothesized that physicians who agreed with the general statement that dyspnea is an important clinical problem would be more likely to believe that routine dyspnea assessment would be valuable.
METHODS
Study Sample
We invited 255 attending hospitalists from 9 geographically and structurally diverse hospitals to complete a survey about the assessment and management of dyspnea. The 9 hospitals represent range of practice environments including 4 academic medical centers, 2 community teaching and 3 nonteaching hospitals, 1 Veterans Administration hospital, and 2 staff‐model HMOs (see Supporting Table 1 in the online version of this article). The survey was distributed online using REDCap (Research Electronic Data Capture), a secure web‐based interface application.[12] A coinvestigator who was a pulmonary critical‐care physician at each site sent an initial email to their hospitalist groups that alerted them to expect a survey from the principal investigator. This notification was subsequently followed by an email invitation containing an informational cover letter and a link to the online survey. The cover letter stated that the completed surveys would not be stored at the local sites and that all the analyzed data would be deidentified. Nonrespondents were sent reminders at 2 and 4 weeks after the initial mailing. A $25 electronic gift card was provided as a gesture of appreciation for their time. The survey was conducted between September 2013 and December 2013.
The study was approved by the Baystate Health Institutional Review Board, Springfield, Massachusetts, with a waiver for written informed consent.
Questionnaire
We developed a 17‐item instrument based on a review of the dyspnea literature and a prior ACCP survey.[12] Questions were piloted with 4 hospitalists at a single institution and modified to improve face validity and clarity (see Supporting Information in the online version of this article for the full survey).
Hospitalists were asked to consider the care of patients admitted for acute cardiopulmonary disease, including heart failure, chronic obstructive pulmonary disease, and pneumonia. A series of 5‐point Likert scales were used to assess the respondents level of agreement with statements related to the following domains: the importance of dyspnea in clinical care, the potential benefits and challenges of routine dyspnea assessment (statements such as: Having a standardized assessment of dyspnea severity would be helpful in management of patients with cardiopulmonary diseases. Dyspnea assessment by a scale should be part of the vital signs for patients with cardiopulmonary diseases.), and management of dyspnea (questions regarding the use of opioids and other nonpharmacological therapies). Additional questions were asked about current assessment practices (questions such as: How often do you assess severity of dyspnea? What is your approach in assessing dyspnea? with options of choosing a categorical or numerical scale), if dyspnea is assessed in their institution by nurses and how often, and the influence of dyspnea severity assessment on their management. The survey had 1 question that solicited comments from the participants: If you don't think that it would be useful to have a standardized dyspnea assessment, please tell us why.
Data Analysis
Responses to survey questions were summarized via counts and percentages in each response category. Adopting the methodology used in the ACCP consensus statement, strongly agree and somewhat agree were combined into a single category of agreement. We also presented percentage of responses in the 2 levels of agreement (strongly agree and somewhat agree) for each question in a bar graph.
Associations between tertiles of physicians' time in practice and attitude toward dyspnea were evaluated via 2 or Fisher exact test.
To examine how answers to the first 2 questions, which assessed attitude toward importance of dyspnea in clinical care, affect answers to the remaining questions, we grouped respondents in 3 categories (strongly agree, agree to these questions, do not agree) and tested the associations using 2 or Fisher exact test.
All analyses were performed using SAS version 9.3 (SAS institute, Inc., Cary, NC) and Stata release 13.1 (StataCorp, College Station, TX).
RESULTS
Overall, 178 (69.8%) of 255 identified hospitalists completed the survey, and all 9 participating hospitals had a response rate greater than 50%. The median number of years in practice was 6 (range, 038 years). A majority (77.5%) of respondents agreed with the statement that dyspnea is 1 of the major symptoms of patients with cardiopulmonary disease, and that its treatment is central to the management of these patients (77.0%) (Figure 1).
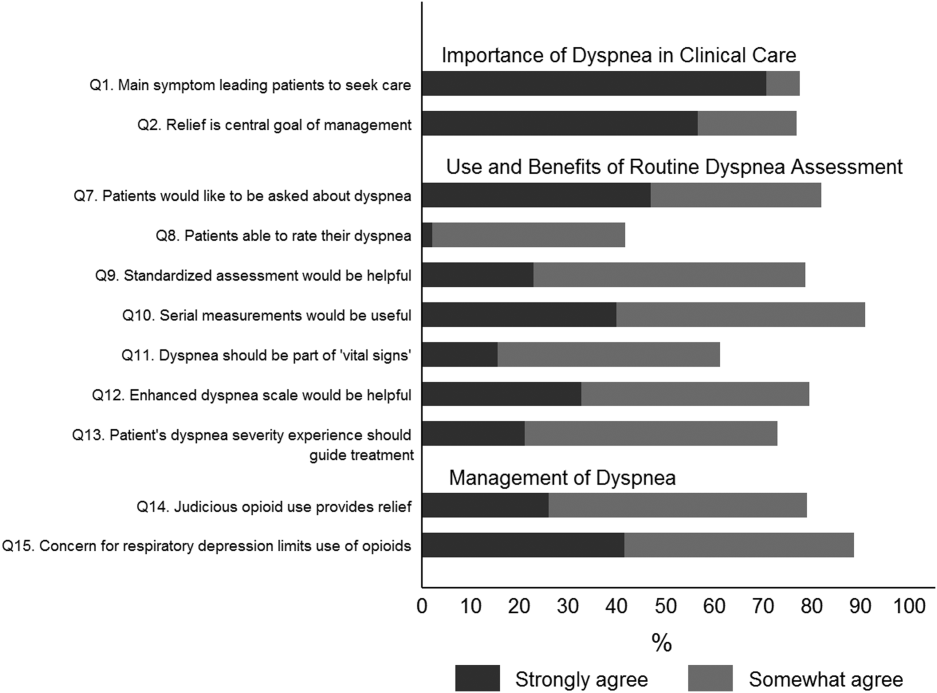
Attitude and Practices Surrounding Dyspnea Assessment
When asked about their current assessment of dyspnea, a majority (84.3%) of the hospitalists stated that they assess dyspnea on a daily basis; two‐thirds indicated that they use a categorical scale (ie, no shortness of breath, improved or worsened compared with a prior date), and one‐third indicated that they ask whether the patient is dyspneic or not. Fifty‐six percent of hospitalists stated that dyspnea is regularly assessed by nurses in their hospital.
The majority of respondents agreed (78.6%, 23.0% strongly and 55.6% somewhat agree) that standardized assessment of dyspnea severity, using a numeric scale and serial measurements as part of the vital signs, would benefit the management of patients with cardiopulmonary diseases. Furthermore, 79.6% (33.0% strongly and 46.6% somewhat agree) reported that using a dyspnea scale that included information to further characterize the patient‐reported experience, such as the level of distress associated with dyspnea, would be helpful in management.
Approximately 90% of the hospitalists indicated that awareness of dyspnea severity has an influence on clinical decision making, including whether to intensify treatment of underlying conditions, to pursue additional diagnostic testing, or to modify discharge timing. Additionally, two‐thirds of hospitalists agreed that awareness of dyspnea severity influences their decision to add opioids, whereas only one‐third prescribed nonpharmacologic symptom‐oriented treatment (Table 1).
Frequency (%) | |
---|---|
| |
When caring for patients with acute cardiopulmonary diseases, how often do you assess severity of dyspnea?* | |
At admission | 66 (37.1) |
At discharge | 59 (33.2) |
Daily until discharge | 150 (84.3) |
More often than daily | 58 (32.6) |
Which description best characterizes your approach to assessing dyspnea severity? | |
I don't regularly ask about dyspnea severity | 3 (1.7) |
I ask the patient whether or not they are having shortness of breath | 50 (28.3) |
I ask the patient to rate the severity of shortness of breath using a numeric scale | 4 (2.3) |
I ask the patient to rate the severity of shortness of breath using a categorical scale (eg, somewhat SOB, no SOB, improved or worsened compared with a prior date) | 120 (67.8) |
When is dyspnea severity assessed and documented by nursing at your hospital?* | |
Dyspnea is not routinely assessed | 60 (33.7) |
At admission | 30 (16.9) |
Daily | 43 (24.2) |
Each shift | 64 (36.0) |
Awareness of dyspnea severity affects my management by:* | |
Influencing my decision to intensify treatment of the patient's underlying condition | 170 (95.5) |
Influencing my decision to pursue additional diagnostic testing | 160 (89.9) |
Influencing my decision to add pharmacologic‐based, symptom‐oriented treatment for dyspnea, such as opioids | 115 (64.6) |
Influencing my decision to add nonpharmacologic‐based, symptom‐oriented treatment for dyspnea, such as fans or pursed lip breathing technique | 58 (32.6) |
Influencing my decision regarding timing of discharge | 162 (91.0) |
Which of the following nonpharmacologic therapies are effective for the relief of dyspnea?* | |
Pursed lip breathing | 113 (63.5) |
Relaxation techniques | 137 (77.0) |
Noninvasive ventilation | 143 (80.3) |
O2 for nonhypoxemic patients | 89 (50.0) |
Cool air/fan | 125 (70.2) |
Cognitive behavioral strategies | 101 (56.7) |
Forty‐two percent of the respondents agreed that patients are able to rate their dyspnea on a scale (2.3% strongly agree and 40.0% agree), and 73.0% indicated that patient experience of dyspnea should guide management independent of physiologic measures such as respiratory rate and oxygen saturation (Figure 1).
Several potential barriers were identified among the 18 participants who did not think that a standardized assessment of dyspnea would be beneficial, including concerns that (1) a dyspnea severity scale is too subjective and numerical scales are not useful for a subjective symptom (19.0%), (2) patients may overrate their symptom or will not be able to rate their dyspnea using a scale (31.0%), or (3) categorical description is sufficient (31.0%).
Practices in Dyspnea Management
Seventy‐nine percent of respondents agreed with the statement that judicious use of opioids can provide relief of dyspnea (26.1% strongly and 52.8% agreed), and 88.7% hospitalists identified the risk of respiratory depression as 1 of the barriers for the limited use of opioids. The majority of physicians (60%80%) considered nonpharmacologic therapies effective for symptomatic treatment of dyspnea, including in the order of agreement: noninvasive ventilation, relaxation techniques, cool air/fan, use of pursed lip breathing, and oxygen for nonhypoxemic patients (Table 1).
Physician Experience and Attitudes Toward Dyspnea Management
When we stratified hospitalists in tertiles of median years of time in practice (median [range]: 2 [04], 6 [58] and 15 [938]), we did not find an association with any of the responses to the questions.
Attitude Regarding the Importance of Dyspnea in Clinical Care and Responses to Subsequent Questions
Respondents who strongly agree or agree that dyspnea is the primary presenting symptom in patients with cardiovascular condition and that dyspnea relief is central to the management of these patients were more likely to believe that patients would like to be asked about their dyspnea (61.2% vs 30.2% vs 29.7%). They also had a more positive attitude about the usefulness of a standardized assessment of dyspnea and the inclusion of the assessment of dyspnea by a scale in the vital signs (Table 2).
Description | Do Not Agree, n (%) | Somewhat Agree, n (%) | Strongly Agree, n (%) | P Value* |
---|---|---|---|---|
| ||||
37 (20.9) | 43 (24.3) | 97 (54.8) | ||
Which description best characterizes your approach to assessing dyspnea severity? | 0.552 | |||
I don't regularly ask about dyspnea severity | 0 (0) | 0 (0) | 3 (3.1) | |
I ask the patient whether or not they are having shortness of breath | 11 (29.7) | 14 (32.6) | 25 (25.8) | |
I ask the patient to rate the severity of shortness of breath using a numeric scale | 2 (5.4) | 1 (2.3) | 1 (1.0) | |
I ask the patient to rate the severity of shortness of breath using a categorical scale (e.g., somewhat shortness of breath, no shortness of breath, improved or worsened compared with a prior date) | 24 (64.9) | 28 (65.1) | 68 (70.1) | |
Patients would like me to ask them about their dyspnea. | <0.0001 | |||
Somewhat agree | 9 (24.3) | 21 (48.8) | 32 (32.7) | |
Strongly agree | 11 (29.7) | 13 (30.2) | 60 (61.2) | |
Patients are able to rate their own dyspnea intensity on a scale of 0‐10. | 0.432 | |||
Somewhat agree | 12 (32.4) | 16 (37.2) | 42 (43.3) | |
Strongly agree | 2 (5.4) | 0 (0) | 2 (2.1) | |
Having a standardized assessment of dyspnea severity would be helpful to me in management of patients with cardiopulmonary diseases. | 0.026 | |||
Somewhat agree | 17 (46.0) | 25 (58.1) | 57 (58.2) | |
Strongly agree | 7 (18.9) | 6 (14.0) | 28 (28.6) | |
Serial measurements of dyspnea would be useful for assessing response to therapy. | 0.042 | |||
Somewhat agree | 14 (37.8) | 28 (65.1) | 48 (49.5) | |
Strongly agree | 16 (43.2) | 12 (27.9) | 43 (44.3) | |
Dyspnea assessment by a scale should be part of the vital signs for patients with cardiopulmonary diseases. | 0.042 | |||
Somewhat agree | 13 (35.1) | 17 (39.5) | 51 (52.0) | |
Strongly agree | 4 (10.8) | 5 (11.6) | 19 (19.4) | |
Using an enhanced dyspnea scale that includes information about the following 4 features 1) Current dyspnea severity, 2) Worst dyspnea ever, 3) Improvement of dyspnea since admission, 4) Acceptability of current level of dyspnea, would be more helpful for my management than a single question focused on dyspnea severity. | 0.03 | |||
Somewhat agree | 14 (40.0) | 24 (55.8) | 44 (44.9) | |
Strongly agree | 9 (25.7) | 9 (20.9) | 40 (40.8) | |
The patients experience of dyspnea should be used to guide treatment decisions independent of objective measures such as respiratory rate and oxygen saturation. | 0.10 | |||
Somewhat agree | 20 (54.0) | 21 (48.8) | 51 (52.0) | |
Strongly agree | 5 (13.5) | 6 (14.0) | 27 (27.6) | |
Judicious use of oral and/or parenteral opioids can provide relief of dyspnea. | 0.21 | |||
Somewhat agree | 20 (54.0) | 23 (54.8) | 50 (51.6) | |
Strongly agree | 10 (27.0) | 6 (14.3) | 30 (30.9) | |
Limited use of opioids for relief of dyspnea in patients with advanced cardiopulmonary disorders is often due to concerns of respiratory depression. | 0.71 | |||
Somewhat agree | 17 (46.0) | 23 (54.8) | 43 (43.9) | |
Strongly agree | 15 (40.5) | 14 (33.3) | 45 (45.9) |
DISCUSSION
In this survey of 178 most hospitalists from a diverse group of 9 US hospitals, we found that most indicate that severity of dyspnea has a profound influence on their clinical practice (including their decision whether to intensify treatments such as diuretics or bronchodilators, to pursue additional diagnostic testing, add opioids or other nonpharmacological treatments) and ultimately their decision regarding the timing of hospital discharge. More importantly, whereas less than half reported experience with standardized assessment of dyspnea severity, most stated that such data would be very useful in their practice.
Despite being a highly prevalent symptom in diverse patient populations, several studies have shown that documentation of dyspnea is sporadic and evaluation of dyspnea quality of care is not routinely performed.[13, 14, 15] Statements from a number of professional societies, including the ACCP, the American Thoracic Society and the Canadian Respiratory Society, recommend that dyspnea management should rely on patient reporting, and that dyspnea severity should be recorded.[1, 4, 7] Assessment is an essential step to guide interventions; however, simply asking about the presence or absence of dyspnea is insufficient.
Several rating scales have been validated and might be implementable in the acute care setting, including the Numerical Rating Scale and the Visual Assessment Scale.[16, 17, 18, 19, 20] Our survey shows that standardized documentation of dyspnea severity in clinical practice is uncommon. However, most hospitalists in our study believed that assessment of dyspnea, using a standardized scale, would positively impact their management of patients with cardiopulmonary disease.
There are a number of potential benefits of routine assessment of dyspnea in hospitalized patients. Implementation of a standardized approach to dyspnea measurement would result in more uniform assessment and documentation practices, and in turn greater awareness among members of the patient‐care team. Though not sufficient to improve care, measurement is necessary because physicians do not always recognize the severity of patients' dyspnea or may not recognize its presence. A retrospective study that assessed the prevalence of symptoms in 410 ambulatory patients showed that one‐quarter of patients had dyspnea, but only half of them told their doctor about it.[21] Two other studies of patients with cancer diagnoses found that 30%70% of patients had dyspnea, but the symptom was recognized in only half of them; even when recognized, dyspnea severity was frequently underrated by physicians.[21, 22] Importantly, underestimation appears to correlate with underutilization of symptomatic management of dyspnea.[8]
Although the results of our survey are encouraging, they highlight a number of potential barriers and misconceptions among hospitalists. For example, although dyspnea can be characterized only by the person experiencing it, only 42% of our survey respondents believed that patients are able to rate their dyspnea intensity on a scale. Some of these responses may be influenced by the fact that dyspnea scales are not currently available to patients under their care. Another explanation is that similar to the case for pain, some hospitalists may believe that patients will exaggerate dyspnea severity. Almost one‐third of the respondents stated that objective measures, such as respiratory rate or oxygen saturation, are more important than a patient's experience of dyspnea in guiding the treatment, and that dyspnea is a subjective symptom and not a vital sign itself. Hospitalists who appreciated the importance of dyspnea in clinical practice were more likely to support the implementation of a standardized dyspnea scale for dyspnea assessment.
Although the potential benefits of including routine measurement of dyspnea in standard hospital practice may seem obvious, evidence that implementing routine assessment improves patient care or outcomes is lacking. Even if hospitalists see the value of dyspnea assessment, asking nurses to collect and document additional information would represent a substantial change in hospital workflow. Finally, without specific protocols to guide care, it is unclear whether physicians will be able to use new information about dyspnea severity effectively. Future studies need to evaluate the impact of implementing routine dyspnea assessment on the management of patients with cardiopulmonary diseases including the use of evidence‐based interventions and reducing the use of less valuable care.
Most hospitalists agreed with the basic principles of dyspnea treatment in patients with advanced cardiopulmonary disease after the primary disease had been stabilized. Effective measures are available, and several guidelines endorse opioids in dyspnea management.[1, 4, 7] However, many clinicians are uncomfortable with this approach for dyspnea, and opioids remain underused. In our study, almost 90% of physicians recognized that concerns about respiratory depression limits opioids use as a treatment. A qualitative study that explored the physicians' perspective toward opioids showed that most physicians were reluctant to prescribe opioids for refractory dyspnea, describing a lack of related knowledge and experience, and fears related to the potential adverse effects. The findings of our study also outline the need to better educate residents and hospitalists on the assessment and management of dyspnea, including prescribing opioids for refractory dyspnea.[23]
Study Strengths and Limitations
This study has several strengths. To our knowledge, it is the first to explore hospitalists' perspectives on incorporating dyspnea assessment in their clinical practice. Hospitalists are the attending physicians for a large majority of inpatients and would be the main users of a dyspnea severity scale. Our questionnaire survey included a large number of hospitalists, from 9 geographically and structurally diverse hospitals, which increased the generalizability of the findings to other hospitals around the country.
The study also has several limitations that need be kept in mind in interpreting the study results. First, desirability bias may have exaggerated some of the positive views expressed by hospitalists toward implementation of routine assessment of dyspnea. Second, because this was a survey, the estimates of dyspnea assessment and documentation practices of both physicians and nurses were based on the respondents' perception and not an objective review of medical records, and the results may be different from actual practice. Third, this was not a population‐based random sample of hospitalists, and it may not be entirely representative; however, those surveyed were from a diverse set of sites with different geographical location, size, academic affiliation, and practice environment, and their time in practice varied widely. Last, we do not have information on nonrespondents, and there is a possibility of nonresponse bias, although the high response rate lessens the risk.
CONCLUSIONS
The results of this survey suggest that most hospitalists believe that routine assessment of dyspnea severity would enhance their clinical decision making and improve patient care. Standardized assessment of dyspnea might result in better awareness of this symptom among providers, reduce undertreatment and mistreatment, and ultimately result in better outcomes for patients. However, implementation of the routine assessment of dyspnea would change current clinical practices and may have a significant effect on existing nursing and physician workflows. Additional research is needed to determine the feasibility and impact on outcomes of routine dyspnea assessment.
Acknowledgements
The authors wish to acknowledge Ms. Anu Joshi for her help with editing the manuscript and assisting with table preparations.
Disclosures
Dr. Stefan is supported by grant K01HL114631‐01A1 from the National Heart, Lung, and Blood Institute of the National Institutes of Health, and by the National Center for Research Resources and the National Center for Advancing Translational Sciences, National Institutes of Health, through grant UL1RR025752. The funder had no role in the design and conduct of the study; collection, management, analysis, and interpretation of the data; preparation, review, or approval of the manuscript; and decision to submit the manuscript for publication. M.S.S. and P.K.L. conceived of the study. M.S.S. acquired the data with the help of all collaborators. M.S.S., P.K.L., P.S.P., and A.P. analyzed and interpreted the data. M.S.S. drafted the manuscript. All authors critically reviewed the manuscript for intellectual content. M.S.S., P.K.L., and A.P. had full access to all of the data in the study and take responsibility for the integrity of the data and the accuracy of the data analysis. M.S.S. is the guarantor for this article, and is responsible for the content of the article, including data and analysis. The authors report no conflicts of interest.
Dyspnea, defined as a subjective experience of breathing discomfort,[1] is the seventh most frequent reason adult patients present to the emergency room and the most frequent cause for emergency room visits in patients 65 years or older.[2] Moreover, dyspnea is experienced by 49% of patients hospitalized with a medical condition[3, 4, 5] and by 70% of patients who are seriously ill.[6]
Based on evidence that patients are not treated consistently and effectively for relief of their shortness of breath, the American College of Chest Physicians (ACCP) statement on dyspnea management in patients with advanced lung or heart disease recommended that patients should be asked to rate their dyspnea, and the rating should be routinely documented in the medical record to guide management.[7] Although clinicians may question the utility of routine assessment of dyspnea using a standardized scale, studies have found that the prevalence of dyspnea reported from chart review is much lower than when patients are directly interviewed.[8] This may be the result of underrecognition of dyspnea or poor documentation by physicians, or that patients may not communicate their symptoms unless the physician specifically asks. As is the case with pain, routine assessment of dyspnea severity could lead to improved clinical management and greater patient‐centered care. However, unlike in the case of pain, regulatory bodies, such as the Joint Commission for Accreditation of Healthcare Organization, do not require routine dyspnea assessment.[9]
Currently, there are more than 40,000 hospitalists in the United States, and the vast majority of hospitals with >200 beds have a hospitalist group.[10] Hospitalists care for over 60% of inpatients[11] and play a major role in the management of patients with acute cardiopulmonary diseases. If standardized approaches for the assessment and documentation of dyspnea are to be implemented, hospitalists would be a key stakeholder group for utilizing enhanced clinical information about dyspnea. Therefore, we evaluated attitudes and practices of hospitalists in regard to the assessment and management of dyspnea, including the potential benefits and challenges related to the implementation of standardized assessment. We hypothesized that hospitalists would believe that a dyspnea scale for assessment of severity could improve their management of patients with cardiovascular diseases. Further, we hypothesized that physicians who agreed with the general statement that dyspnea is an important clinical problem would be more likely to believe that routine dyspnea assessment would be valuable.
METHODS
Study Sample
We invited 255 attending hospitalists from 9 geographically and structurally diverse hospitals to complete a survey about the assessment and management of dyspnea. The 9 hospitals represent range of practice environments including 4 academic medical centers, 2 community teaching and 3 nonteaching hospitals, 1 Veterans Administration hospital, and 2 staff‐model HMOs (see Supporting Table 1 in the online version of this article). The survey was distributed online using REDCap (Research Electronic Data Capture), a secure web‐based interface application.[12] A coinvestigator who was a pulmonary critical‐care physician at each site sent an initial email to their hospitalist groups that alerted them to expect a survey from the principal investigator. This notification was subsequently followed by an email invitation containing an informational cover letter and a link to the online survey. The cover letter stated that the completed surveys would not be stored at the local sites and that all the analyzed data would be deidentified. Nonrespondents were sent reminders at 2 and 4 weeks after the initial mailing. A $25 electronic gift card was provided as a gesture of appreciation for their time. The survey was conducted between September 2013 and December 2013.
The study was approved by the Baystate Health Institutional Review Board, Springfield, Massachusetts, with a waiver for written informed consent.
Questionnaire
We developed a 17‐item instrument based on a review of the dyspnea literature and a prior ACCP survey.[12] Questions were piloted with 4 hospitalists at a single institution and modified to improve face validity and clarity (see Supporting Information in the online version of this article for the full survey).
Hospitalists were asked to consider the care of patients admitted for acute cardiopulmonary disease, including heart failure, chronic obstructive pulmonary disease, and pneumonia. A series of 5‐point Likert scales were used to assess the respondents level of agreement with statements related to the following domains: the importance of dyspnea in clinical care, the potential benefits and challenges of routine dyspnea assessment (statements such as: Having a standardized assessment of dyspnea severity would be helpful in management of patients with cardiopulmonary diseases. Dyspnea assessment by a scale should be part of the vital signs for patients with cardiopulmonary diseases.), and management of dyspnea (questions regarding the use of opioids and other nonpharmacological therapies). Additional questions were asked about current assessment practices (questions such as: How often do you assess severity of dyspnea? What is your approach in assessing dyspnea? with options of choosing a categorical or numerical scale), if dyspnea is assessed in their institution by nurses and how often, and the influence of dyspnea severity assessment on their management. The survey had 1 question that solicited comments from the participants: If you don't think that it would be useful to have a standardized dyspnea assessment, please tell us why.
Data Analysis
Responses to survey questions were summarized via counts and percentages in each response category. Adopting the methodology used in the ACCP consensus statement, strongly agree and somewhat agree were combined into a single category of agreement. We also presented percentage of responses in the 2 levels of agreement (strongly agree and somewhat agree) for each question in a bar graph.
Associations between tertiles of physicians' time in practice and attitude toward dyspnea were evaluated via 2 or Fisher exact test.
To examine how answers to the first 2 questions, which assessed attitude toward importance of dyspnea in clinical care, affect answers to the remaining questions, we grouped respondents in 3 categories (strongly agree, agree to these questions, do not agree) and tested the associations using 2 or Fisher exact test.
All analyses were performed using SAS version 9.3 (SAS institute, Inc., Cary, NC) and Stata release 13.1 (StataCorp, College Station, TX).
RESULTS
Overall, 178 (69.8%) of 255 identified hospitalists completed the survey, and all 9 participating hospitals had a response rate greater than 50%. The median number of years in practice was 6 (range, 038 years). A majority (77.5%) of respondents agreed with the statement that dyspnea is 1 of the major symptoms of patients with cardiopulmonary disease, and that its treatment is central to the management of these patients (77.0%) (Figure 1).
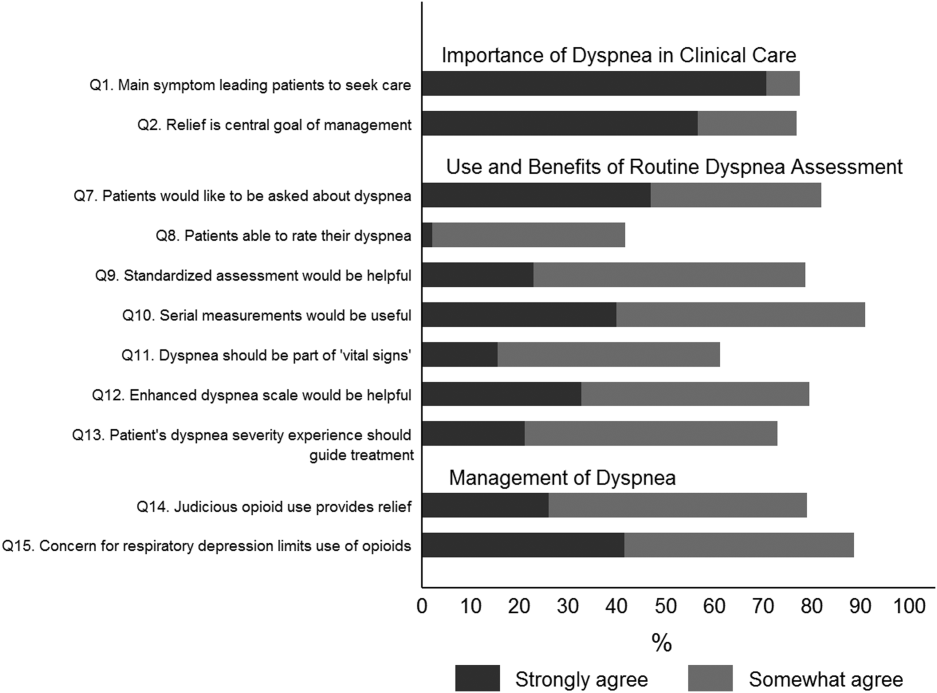
Attitude and Practices Surrounding Dyspnea Assessment
When asked about their current assessment of dyspnea, a majority (84.3%) of the hospitalists stated that they assess dyspnea on a daily basis; two‐thirds indicated that they use a categorical scale (ie, no shortness of breath, improved or worsened compared with a prior date), and one‐third indicated that they ask whether the patient is dyspneic or not. Fifty‐six percent of hospitalists stated that dyspnea is regularly assessed by nurses in their hospital.
The majority of respondents agreed (78.6%, 23.0% strongly and 55.6% somewhat agree) that standardized assessment of dyspnea severity, using a numeric scale and serial measurements as part of the vital signs, would benefit the management of patients with cardiopulmonary diseases. Furthermore, 79.6% (33.0% strongly and 46.6% somewhat agree) reported that using a dyspnea scale that included information to further characterize the patient‐reported experience, such as the level of distress associated with dyspnea, would be helpful in management.
Approximately 90% of the hospitalists indicated that awareness of dyspnea severity has an influence on clinical decision making, including whether to intensify treatment of underlying conditions, to pursue additional diagnostic testing, or to modify discharge timing. Additionally, two‐thirds of hospitalists agreed that awareness of dyspnea severity influences their decision to add opioids, whereas only one‐third prescribed nonpharmacologic symptom‐oriented treatment (Table 1).
Frequency (%) | |
---|---|
| |
When caring for patients with acute cardiopulmonary diseases, how often do you assess severity of dyspnea?* | |
At admission | 66 (37.1) |
At discharge | 59 (33.2) |
Daily until discharge | 150 (84.3) |
More often than daily | 58 (32.6) |
Which description best characterizes your approach to assessing dyspnea severity? | |
I don't regularly ask about dyspnea severity | 3 (1.7) |
I ask the patient whether or not they are having shortness of breath | 50 (28.3) |
I ask the patient to rate the severity of shortness of breath using a numeric scale | 4 (2.3) |
I ask the patient to rate the severity of shortness of breath using a categorical scale (eg, somewhat SOB, no SOB, improved or worsened compared with a prior date) | 120 (67.8) |
When is dyspnea severity assessed and documented by nursing at your hospital?* | |
Dyspnea is not routinely assessed | 60 (33.7) |
At admission | 30 (16.9) |
Daily | 43 (24.2) |
Each shift | 64 (36.0) |
Awareness of dyspnea severity affects my management by:* | |
Influencing my decision to intensify treatment of the patient's underlying condition | 170 (95.5) |
Influencing my decision to pursue additional diagnostic testing | 160 (89.9) |
Influencing my decision to add pharmacologic‐based, symptom‐oriented treatment for dyspnea, such as opioids | 115 (64.6) |
Influencing my decision to add nonpharmacologic‐based, symptom‐oriented treatment for dyspnea, such as fans or pursed lip breathing technique | 58 (32.6) |
Influencing my decision regarding timing of discharge | 162 (91.0) |
Which of the following nonpharmacologic therapies are effective for the relief of dyspnea?* | |
Pursed lip breathing | 113 (63.5) |
Relaxation techniques | 137 (77.0) |
Noninvasive ventilation | 143 (80.3) |
O2 for nonhypoxemic patients | 89 (50.0) |
Cool air/fan | 125 (70.2) |
Cognitive behavioral strategies | 101 (56.7) |
Forty‐two percent of the respondents agreed that patients are able to rate their dyspnea on a scale (2.3% strongly agree and 40.0% agree), and 73.0% indicated that patient experience of dyspnea should guide management independent of physiologic measures such as respiratory rate and oxygen saturation (Figure 1).
Several potential barriers were identified among the 18 participants who did not think that a standardized assessment of dyspnea would be beneficial, including concerns that (1) a dyspnea severity scale is too subjective and numerical scales are not useful for a subjective symptom (19.0%), (2) patients may overrate their symptom or will not be able to rate their dyspnea using a scale (31.0%), or (3) categorical description is sufficient (31.0%).
Practices in Dyspnea Management
Seventy‐nine percent of respondents agreed with the statement that judicious use of opioids can provide relief of dyspnea (26.1% strongly and 52.8% agreed), and 88.7% hospitalists identified the risk of respiratory depression as 1 of the barriers for the limited use of opioids. The majority of physicians (60%80%) considered nonpharmacologic therapies effective for symptomatic treatment of dyspnea, including in the order of agreement: noninvasive ventilation, relaxation techniques, cool air/fan, use of pursed lip breathing, and oxygen for nonhypoxemic patients (Table 1).
Physician Experience and Attitudes Toward Dyspnea Management
When we stratified hospitalists in tertiles of median years of time in practice (median [range]: 2 [04], 6 [58] and 15 [938]), we did not find an association with any of the responses to the questions.
Attitude Regarding the Importance of Dyspnea in Clinical Care and Responses to Subsequent Questions
Respondents who strongly agree or agree that dyspnea is the primary presenting symptom in patients with cardiovascular condition and that dyspnea relief is central to the management of these patients were more likely to believe that patients would like to be asked about their dyspnea (61.2% vs 30.2% vs 29.7%). They also had a more positive attitude about the usefulness of a standardized assessment of dyspnea and the inclusion of the assessment of dyspnea by a scale in the vital signs (Table 2).
Description | Do Not Agree, n (%) | Somewhat Agree, n (%) | Strongly Agree, n (%) | P Value* |
---|---|---|---|---|
| ||||
37 (20.9) | 43 (24.3) | 97 (54.8) | ||
Which description best characterizes your approach to assessing dyspnea severity? | 0.552 | |||
I don't regularly ask about dyspnea severity | 0 (0) | 0 (0) | 3 (3.1) | |
I ask the patient whether or not they are having shortness of breath | 11 (29.7) | 14 (32.6) | 25 (25.8) | |
I ask the patient to rate the severity of shortness of breath using a numeric scale | 2 (5.4) | 1 (2.3) | 1 (1.0) | |
I ask the patient to rate the severity of shortness of breath using a categorical scale (e.g., somewhat shortness of breath, no shortness of breath, improved or worsened compared with a prior date) | 24 (64.9) | 28 (65.1) | 68 (70.1) | |
Patients would like me to ask them about their dyspnea. | <0.0001 | |||
Somewhat agree | 9 (24.3) | 21 (48.8) | 32 (32.7) | |
Strongly agree | 11 (29.7) | 13 (30.2) | 60 (61.2) | |
Patients are able to rate their own dyspnea intensity on a scale of 0‐10. | 0.432 | |||
Somewhat agree | 12 (32.4) | 16 (37.2) | 42 (43.3) | |
Strongly agree | 2 (5.4) | 0 (0) | 2 (2.1) | |
Having a standardized assessment of dyspnea severity would be helpful to me in management of patients with cardiopulmonary diseases. | 0.026 | |||
Somewhat agree | 17 (46.0) | 25 (58.1) | 57 (58.2) | |
Strongly agree | 7 (18.9) | 6 (14.0) | 28 (28.6) | |
Serial measurements of dyspnea would be useful for assessing response to therapy. | 0.042 | |||
Somewhat agree | 14 (37.8) | 28 (65.1) | 48 (49.5) | |
Strongly agree | 16 (43.2) | 12 (27.9) | 43 (44.3) | |
Dyspnea assessment by a scale should be part of the vital signs for patients with cardiopulmonary diseases. | 0.042 | |||
Somewhat agree | 13 (35.1) | 17 (39.5) | 51 (52.0) | |
Strongly agree | 4 (10.8) | 5 (11.6) | 19 (19.4) | |
Using an enhanced dyspnea scale that includes information about the following 4 features 1) Current dyspnea severity, 2) Worst dyspnea ever, 3) Improvement of dyspnea since admission, 4) Acceptability of current level of dyspnea, would be more helpful for my management than a single question focused on dyspnea severity. | 0.03 | |||
Somewhat agree | 14 (40.0) | 24 (55.8) | 44 (44.9) | |
Strongly agree | 9 (25.7) | 9 (20.9) | 40 (40.8) | |
The patients experience of dyspnea should be used to guide treatment decisions independent of objective measures such as respiratory rate and oxygen saturation. | 0.10 | |||
Somewhat agree | 20 (54.0) | 21 (48.8) | 51 (52.0) | |
Strongly agree | 5 (13.5) | 6 (14.0) | 27 (27.6) | |
Judicious use of oral and/or parenteral opioids can provide relief of dyspnea. | 0.21 | |||
Somewhat agree | 20 (54.0) | 23 (54.8) | 50 (51.6) | |
Strongly agree | 10 (27.0) | 6 (14.3) | 30 (30.9) | |
Limited use of opioids for relief of dyspnea in patients with advanced cardiopulmonary disorders is often due to concerns of respiratory depression. | 0.71 | |||
Somewhat agree | 17 (46.0) | 23 (54.8) | 43 (43.9) | |
Strongly agree | 15 (40.5) | 14 (33.3) | 45 (45.9) |
DISCUSSION
In this survey of 178 most hospitalists from a diverse group of 9 US hospitals, we found that most indicate that severity of dyspnea has a profound influence on their clinical practice (including their decision whether to intensify treatments such as diuretics or bronchodilators, to pursue additional diagnostic testing, add opioids or other nonpharmacological treatments) and ultimately their decision regarding the timing of hospital discharge. More importantly, whereas less than half reported experience with standardized assessment of dyspnea severity, most stated that such data would be very useful in their practice.
Despite being a highly prevalent symptom in diverse patient populations, several studies have shown that documentation of dyspnea is sporadic and evaluation of dyspnea quality of care is not routinely performed.[13, 14, 15] Statements from a number of professional societies, including the ACCP, the American Thoracic Society and the Canadian Respiratory Society, recommend that dyspnea management should rely on patient reporting, and that dyspnea severity should be recorded.[1, 4, 7] Assessment is an essential step to guide interventions; however, simply asking about the presence or absence of dyspnea is insufficient.
Several rating scales have been validated and might be implementable in the acute care setting, including the Numerical Rating Scale and the Visual Assessment Scale.[16, 17, 18, 19, 20] Our survey shows that standardized documentation of dyspnea severity in clinical practice is uncommon. However, most hospitalists in our study believed that assessment of dyspnea, using a standardized scale, would positively impact their management of patients with cardiopulmonary disease.
There are a number of potential benefits of routine assessment of dyspnea in hospitalized patients. Implementation of a standardized approach to dyspnea measurement would result in more uniform assessment and documentation practices, and in turn greater awareness among members of the patient‐care team. Though not sufficient to improve care, measurement is necessary because physicians do not always recognize the severity of patients' dyspnea or may not recognize its presence. A retrospective study that assessed the prevalence of symptoms in 410 ambulatory patients showed that one‐quarter of patients had dyspnea, but only half of them told their doctor about it.[21] Two other studies of patients with cancer diagnoses found that 30%70% of patients had dyspnea, but the symptom was recognized in only half of them; even when recognized, dyspnea severity was frequently underrated by physicians.[21, 22] Importantly, underestimation appears to correlate with underutilization of symptomatic management of dyspnea.[8]
Although the results of our survey are encouraging, they highlight a number of potential barriers and misconceptions among hospitalists. For example, although dyspnea can be characterized only by the person experiencing it, only 42% of our survey respondents believed that patients are able to rate their dyspnea intensity on a scale. Some of these responses may be influenced by the fact that dyspnea scales are not currently available to patients under their care. Another explanation is that similar to the case for pain, some hospitalists may believe that patients will exaggerate dyspnea severity. Almost one‐third of the respondents stated that objective measures, such as respiratory rate or oxygen saturation, are more important than a patient's experience of dyspnea in guiding the treatment, and that dyspnea is a subjective symptom and not a vital sign itself. Hospitalists who appreciated the importance of dyspnea in clinical practice were more likely to support the implementation of a standardized dyspnea scale for dyspnea assessment.
Although the potential benefits of including routine measurement of dyspnea in standard hospital practice may seem obvious, evidence that implementing routine assessment improves patient care or outcomes is lacking. Even if hospitalists see the value of dyspnea assessment, asking nurses to collect and document additional information would represent a substantial change in hospital workflow. Finally, without specific protocols to guide care, it is unclear whether physicians will be able to use new information about dyspnea severity effectively. Future studies need to evaluate the impact of implementing routine dyspnea assessment on the management of patients with cardiopulmonary diseases including the use of evidence‐based interventions and reducing the use of less valuable care.
Most hospitalists agreed with the basic principles of dyspnea treatment in patients with advanced cardiopulmonary disease after the primary disease had been stabilized. Effective measures are available, and several guidelines endorse opioids in dyspnea management.[1, 4, 7] However, many clinicians are uncomfortable with this approach for dyspnea, and opioids remain underused. In our study, almost 90% of physicians recognized that concerns about respiratory depression limits opioids use as a treatment. A qualitative study that explored the physicians' perspective toward opioids showed that most physicians were reluctant to prescribe opioids for refractory dyspnea, describing a lack of related knowledge and experience, and fears related to the potential adverse effects. The findings of our study also outline the need to better educate residents and hospitalists on the assessment and management of dyspnea, including prescribing opioids for refractory dyspnea.[23]
Study Strengths and Limitations
This study has several strengths. To our knowledge, it is the first to explore hospitalists' perspectives on incorporating dyspnea assessment in their clinical practice. Hospitalists are the attending physicians for a large majority of inpatients and would be the main users of a dyspnea severity scale. Our questionnaire survey included a large number of hospitalists, from 9 geographically and structurally diverse hospitals, which increased the generalizability of the findings to other hospitals around the country.
The study also has several limitations that need be kept in mind in interpreting the study results. First, desirability bias may have exaggerated some of the positive views expressed by hospitalists toward implementation of routine assessment of dyspnea. Second, because this was a survey, the estimates of dyspnea assessment and documentation practices of both physicians and nurses were based on the respondents' perception and not an objective review of medical records, and the results may be different from actual practice. Third, this was not a population‐based random sample of hospitalists, and it may not be entirely representative; however, those surveyed were from a diverse set of sites with different geographical location, size, academic affiliation, and practice environment, and their time in practice varied widely. Last, we do not have information on nonrespondents, and there is a possibility of nonresponse bias, although the high response rate lessens the risk.
CONCLUSIONS
The results of this survey suggest that most hospitalists believe that routine assessment of dyspnea severity would enhance their clinical decision making and improve patient care. Standardized assessment of dyspnea might result in better awareness of this symptom among providers, reduce undertreatment and mistreatment, and ultimately result in better outcomes for patients. However, implementation of the routine assessment of dyspnea would change current clinical practices and may have a significant effect on existing nursing and physician workflows. Additional research is needed to determine the feasibility and impact on outcomes of routine dyspnea assessment.
Acknowledgements
The authors wish to acknowledge Ms. Anu Joshi for her help with editing the manuscript and assisting with table preparations.
Disclosures
Dr. Stefan is supported by grant K01HL114631‐01A1 from the National Heart, Lung, and Blood Institute of the National Institutes of Health, and by the National Center for Research Resources and the National Center for Advancing Translational Sciences, National Institutes of Health, through grant UL1RR025752. The funder had no role in the design and conduct of the study; collection, management, analysis, and interpretation of the data; preparation, review, or approval of the manuscript; and decision to submit the manuscript for publication. M.S.S. and P.K.L. conceived of the study. M.S.S. acquired the data with the help of all collaborators. M.S.S., P.K.L., P.S.P., and A.P. analyzed and interpreted the data. M.S.S. drafted the manuscript. All authors critically reviewed the manuscript for intellectual content. M.S.S., P.K.L., and A.P. had full access to all of the data in the study and take responsibility for the integrity of the data and the accuracy of the data analysis. M.S.S. is the guarantor for this article, and is responsible for the content of the article, including data and analysis. The authors report no conflicts of interest.
- An Official American Thoracic Society Statement: Update on the Mechanisms, Assessment, and Management of Dyspnea. Am J Respir Crit Care Med. 2012;185(4):435–452. , , , et al.
- CDC/ National Center for Health Statistics. National Hospital Amulatory Medical Care Survey: 2011 Emergency Department Summary Tables. http://www.cdc.gov/nchs/data/ahcd/nhamcs_emergency/2011_ed_web_tables.pdf. Accessed May 15, 2015.
- Signs and symptoms of heart failure: are you asking the right questions? Am J Crit Care. 2010;19(5):443–452. , , , .
- Managing dyspnea in patients with advanced chronic obstructive pulmonary disease: a Canadian Thoracic Society clinical practice guideline. Can Respir J. 2011;18(2):69–78. , , , et al.
- Prevalence of distressing symptoms in hospitalised patients on medical wards: A cross‐sectional study. BMC Palliat Care. 2008;7:16. , .
- Dyspnea in terminally ill cancer patients. Chest. 1986;89(2):234–236. , .
- American College of Chest Physicians consensus statement on the management of dyspnea in patients with advanced lung or heart disease. Chest. 2010;137(3):674–691. , , , et al.
- Common symptoms in ambulatory care: incidence, evaluation, therapy, and outcome. Am J Med. 1989;86(3):262–266. , .
- The Joint Commission. Facts about Pain Management. http://www.jointcommission.org/pain_management/. Accessed May, 15, 2015.
- Hospitalist programs in the age of healthcare reform. J Healthc Manag. 2010;55(6):378–380. .
- The Use of Hospitalists by Small Rural Hospitals: Results of a National Survey. Med Care Res Rev. 2014;71(4):356–366. , , , .
- Tufts CTSI. REDCap [Internet]. Tufts Clinical and Translational Science Institute. http://www.tuftsctsi.org/Services-and-Consultation/REDCap.aspx. Accessed May, 15, 2015.
- Multi‐dimensional Assessment of Dyspnea. Dyspnoea in Advanced Disease: A guide to clinical management; 2005. , .
- Cancer care quality measures: symptoms and end‐of‐life care. Evid Rep Technol Assess (Full Rep). 2006(137):1–77. , , , et al.
- Defining and measuring quality palliative and end‐of‐life care in the intensive care unit. Crit Care Med. 2006;34(11 Suppl):S309–316. .
- Validation of a vertical visual analogue scale as a measure of clinical dyspnea. Rehabil Nurs. 1989;14(6):323–325. .
- Can a self‐rating 0‐10 scale for dyspnea yield a common language that is understood by ED nurses, patients, and their families? J Emerg Nurs. 2000;26(3):233–234. .
- Measurement of dyspnea: word labeled visual analog scale vs. verbal ordinal scale. Respir Physiol Neurobiol. 2003;134(2):77–83. , , .
- Verbal numerical scales are as reliable and sensitive as visual analog scales for rating dyspnea in young and older subjects. Respir Physiol Neurobiol. 2007;157(2‐3):360–365. , , , , , .
- Validation of a three‐factor measurement model of dyspnea in hospitalized adults with heart failure. Heart Lung. 2011;41(1):44–56. , , , , .
- Patient reporting and doctor recognition of dyspnoea in a comprehensive cancer centre. Intern Med J. 2006;36(6):381–384. , , .
- Lung cancer and dyspnea: the patient's perception. Oncol Nurs Forum. 1986;13(5):19–24. , , , .
- Opioids, respiratory function, and dyspnea. Am J Hosp Palliat Care. 2003;20(1):57–61. , , , .
- An Official American Thoracic Society Statement: Update on the Mechanisms, Assessment, and Management of Dyspnea. Am J Respir Crit Care Med. 2012;185(4):435–452. , , , et al.
- CDC/ National Center for Health Statistics. National Hospital Amulatory Medical Care Survey: 2011 Emergency Department Summary Tables. http://www.cdc.gov/nchs/data/ahcd/nhamcs_emergency/2011_ed_web_tables.pdf. Accessed May 15, 2015.
- Signs and symptoms of heart failure: are you asking the right questions? Am J Crit Care. 2010;19(5):443–452. , , , .
- Managing dyspnea in patients with advanced chronic obstructive pulmonary disease: a Canadian Thoracic Society clinical practice guideline. Can Respir J. 2011;18(2):69–78. , , , et al.
- Prevalence of distressing symptoms in hospitalised patients on medical wards: A cross‐sectional study. BMC Palliat Care. 2008;7:16. , .
- Dyspnea in terminally ill cancer patients. Chest. 1986;89(2):234–236. , .
- American College of Chest Physicians consensus statement on the management of dyspnea in patients with advanced lung or heart disease. Chest. 2010;137(3):674–691. , , , et al.
- Common symptoms in ambulatory care: incidence, evaluation, therapy, and outcome. Am J Med. 1989;86(3):262–266. , .
- The Joint Commission. Facts about Pain Management. http://www.jointcommission.org/pain_management/. Accessed May, 15, 2015.
- Hospitalist programs in the age of healthcare reform. J Healthc Manag. 2010;55(6):378–380. .
- The Use of Hospitalists by Small Rural Hospitals: Results of a National Survey. Med Care Res Rev. 2014;71(4):356–366. , , , .
- Tufts CTSI. REDCap [Internet]. Tufts Clinical and Translational Science Institute. http://www.tuftsctsi.org/Services-and-Consultation/REDCap.aspx. Accessed May, 15, 2015.
- Multi‐dimensional Assessment of Dyspnea. Dyspnoea in Advanced Disease: A guide to clinical management; 2005. , .
- Cancer care quality measures: symptoms and end‐of‐life care. Evid Rep Technol Assess (Full Rep). 2006(137):1–77. , , , et al.
- Defining and measuring quality palliative and end‐of‐life care in the intensive care unit. Crit Care Med. 2006;34(11 Suppl):S309–316. .
- Validation of a vertical visual analogue scale as a measure of clinical dyspnea. Rehabil Nurs. 1989;14(6):323–325. .
- Can a self‐rating 0‐10 scale for dyspnea yield a common language that is understood by ED nurses, patients, and their families? J Emerg Nurs. 2000;26(3):233–234. .
- Measurement of dyspnea: word labeled visual analog scale vs. verbal ordinal scale. Respir Physiol Neurobiol. 2003;134(2):77–83. , , .
- Verbal numerical scales are as reliable and sensitive as visual analog scales for rating dyspnea in young and older subjects. Respir Physiol Neurobiol. 2007;157(2‐3):360–365. , , , , , .
- Validation of a three‐factor measurement model of dyspnea in hospitalized adults with heart failure. Heart Lung. 2011;41(1):44–56. , , , , .
- Patient reporting and doctor recognition of dyspnoea in a comprehensive cancer centre. Intern Med J. 2006;36(6):381–384. , , .
- Lung cancer and dyspnea: the patient's perception. Oncol Nurs Forum. 1986;13(5):19–24. , , , .
- Opioids, respiratory function, and dyspnea. Am J Hosp Palliat Care. 2003;20(1):57–61. , , , .
© 2015 Society of Hospital Medicine
Hospital Mortality Measure for COPD
Chronic obstructive pulmonary disease (COPD) affects as many as 24 million individuals in the United States, is responsible for more than 700,000 annual hospital admissions, and is currently the nation's third leading cause of death, accounting for nearly $49.9 billion in medical spending in 2010.[1, 2] Reported in‐hospital mortality rates for patients hospitalized for exacerbations of COPD range from 2% to 5%.[3, 4, 5, 6, 7] Information about 30‐day mortality rates following hospitalization for COPD is more limited; however, international studies suggest that rates range from 3% to 9%,[8, 9] and 90‐day mortality rates exceed 15%.[10]
Despite this significant clinical and economic impact, there have been no large‐scale, sustained efforts to measure the quality or outcomes of hospital care for patients with COPD in the United States. What little is known about the treatment of patients with COPD suggests widespread opportunities to increase adherence to guideline‐recommended therapies, to reduce the use of ineffective treatments and tests, and to address variation in care across institutions.[5, 11, 12]
Public reporting of hospital performance is a key strategy for improving the quality and safety of hospital care, both in the United States and internationally.[13] Since 2007, the Centers for Medicare and Medicaid Services (CMS) has reported hospital mortality rates on the Hospital Compare Web site, and COPD is 1 of the conditions highlighted in the Affordable Care Act for future consideration.[14] Such initiatives rely on validated, risk‐adjusted performance measures for comparisons across institutions and to enable outcomes to be tracked over time. We present the development, validation, and results of a model intended for public reporting of risk‐standardized mortality rates for patients hospitalized with exacerbations of COPD that has been endorsed by the National Quality Forum.[15]
METHODS
Approach to Measure Development
We developed this measure in accordance with guidelines described by the National Quality Forum,[16] CMS' Measure Management System,[17] and the American Heart Association scientific statement, Standards for Statistical Models Used for Public Reporting of Health Outcomes.[18] Throughout the process we obtained expert clinical and stakeholder input through meetings with a clinical advisory group and a national technical expert panel (see Acknowledgments). Last, we presented the proposed measure specifications and a summary of the technical expert panel discussions online and made a widely distributed call for public comments. We took the comments into consideration during the final stages of measure development (available at
Data Sources
We used claims data from Medicare inpatient, outpatient, and carrier (physician) Standard Analytic Files from 2008 to develop and validate the model, and examined model reliability using data from 2007 and 2009. The Medicare enrollment database was used to determine Medicare Fee‐for‐Service enrollment and mortality.
Study Cohort
Admissions were considered eligible for inclusion if the patient was 65 years or older, was admitted to a nonfederal acute care hospital in the United States, and had a principal diagnosis of COPD or a principal diagnosis of acute respiratory failure or respiratory arrest when paired with a secondary diagnosis of COPD with exacerbation (Table 1).
ICD‐9‐CM | Description |
---|---|
| |
491.21 | Obstructive chronic bronchitis; with (acute) exacerbation; acute exacerbation of COPD, decompensated COPD, decompensated COPD with exacerbation |
491.22 | Obstructive chronic bronchitis; with acute bronchitis |
491.8 | Other chronic bronchitis; chronic: tracheitis, tracheobronchitis |
491.9 | Unspecified chronic bronchitis |
492.8 | Other emphysema; emphysema (lung or pulmonary): NOS, centriacinar, centrilobular, obstructive, panacinar, panlobular, unilateral, vesicular; MacLeod's syndrome; Swyer‐James syndrome; unilateral hyperlucent lung |
493.20 | Chronic obstructive asthma; asthma with COPD, chronic asthmatic bronchitis, unspecified |
493.21 | Chronic obstructive asthma; asthma with COPD, chronic asthmatic bronchitis, with status asthmaticus |
493.22 | Chronic obstructive asthma; asthma with COPD, chronic asthmatic bronchitis, with (acute) exacerbation |
496 | Chronic: nonspecific lung disease, obstructive lung disease, obstructive pulmonary disease (COPD) NOS. (Note: This code is not to be used with any code from categories 491493.) |
518.81a | Other diseases of lung; acute respiratory failure; respiratory failure NOS |
518.82a | Other diseases of lung; acute respiratory failure; other pulmonary insufficiency, acute respiratory distress |
518.84a | Other diseases of lung; acute respiratory failure; acute and chronic respiratory failure |
799.1a | Other ill‐defined and unknown causes of morbidity and mortality; respiratory arrest, cardiorespiratory failure |
If a patient was discharged and readmitted to a second hospital on the same or the next day, we combined the 2 acute care admissions into a single episode of care and assigned the mortality outcome to the first admitting hospital. We excluded admissions for patients who were enrolled in Medicare Hospice in the 12 months prior to or on the first day of the index hospitalization. An index admission was any eligible admission assessed in the measure for the outcome. We also excluded admissions for patients who were discharged against medical advice, those for whom vital status at 30 days was unknown or recorded inconsistently, and patients with unreliable data (eg, age >115 years). For patients with multiple hospitalizations during a single year, we randomly selected 1 admission per patient to avoid survival bias. Finally, to assure adequate risk adjustment we limited the analysis to patients who had continuous enrollment in Medicare Fee‐for‐Service Parts A and B for the 12 months prior to their index admission so that we could identify comorbid conditions coded during all prior encounters.
Outcomes
The outcome of 30‐day mortality was defined as death from any cause within 30 days of the admission date for the index hospitalization. Mortality was assessed at 30 days to standardize the period of outcome ascertainment,[19] and because 30 days is a clinically meaningful time frame, during which differences in the quality of hospital care may be revealed.
Risk‐Adjustment Variables
We randomly selected half of all COPD admissions in 2008 that met the inclusion and exclusion criteria to create a model development sample. Candidate variables for inclusion in the risk‐standardized model were selected by a clinician team from diagnostic groups included in the Hierarchical Condition Category clinical classification system[20] and included age and comorbid conditions. Sleep apnea (International Classification of Diseases, 9th Revision, Clinical Modification [ICD‐9‐CM] condition codes 327.20, 327.21, 327.23, 327.27, 327.29, 780.51, 780.53, and 780.57) and mechanical ventilation (ICD‐9‐CM procedure codes 93.90, 96.70, 96.71, and 96.72) were also included as candidate variables.
We defined a condition as present for a given patient if it was coded in the inpatient, outpatient, or physician claims data sources in the preceding 12 months, including the index admission. Because a subset of the condition category variables can represent a complication of care, we did not consider them to be risk factors if they appeared only as secondary diagnosis codes for the index admission and not in claims submitted during the prior year.
We selected final variables for inclusion in the risk‐standardized model based on clinical considerations and a modified approach to stepwise logistic regression. The final patient‐level risk‐adjustment model included 42 variables (Table 2).
Variable | Development Sample (150,035 Admissions at 4537 Hospitals) | Validation Sample (149,646 Admissions at 4535 Hospitals) | ||||
---|---|---|---|---|---|---|
Frequency, % | OR | 95% CI | Frequency, % | OR | 95% CI | |
| ||||||
Demographics | ||||||
Age 65 years (continuous) | 1.03 | 1.03‐1.04 | 1.03 | 1.03‐1.04 | ||
Cardiovascular/respiratory | ||||||
Sleep apnea (ICD‐9‐CM: 327.20, 327.21, 327.23, 327.27, 327.29, 780.51, 780.53, 780.57)a | 9.57 | 0.87 | 0.81‐0.94 | 9.72 | 0.84 | 0.78‐0.90 |
History of mechanical ventilation (ICD‐9‐CM: 93.90, 96.70, 96.71, 96.72)a | 6.00 | 1.19 | 1.11‐1.27 | 6.00 | 1.15 | 1.08‐1.24 |
Respirator dependence/respiratory failure (CC 7778)a | 1.15 | 0.89 | 0.77‐1.02 | 1.20 | 0.78 | 0.68‐0.91 |
Cardiorespiratory failure and shock (CC 79) | 26.35 | 1.60 | 1.53‐1.68 | 26.34 | 1.59 | 1.52‐1.66 |
Congestive heart failure (CC 80) | 41.50 | 1.34 | 1.28‐1.39 | 41.39 | 1.31 | 1.25‐1.36 |
Chronic atherosclerosis (CC 8384)a | 50.44 | 0.87 | 0.83‐0.90 | 50.12 | 0.91 | 0.87‐0.94 |
Arrhythmias (CC 9293) | 37.15 | 1.17 | 1.12‐1.22 | 37.06 | 1.15 | 1.10‐1.20 |
Vascular or circulatory disease (CC 104106) | 38.20 | 1.09 | 1.05‐1.14 | 38.09 | 1.02 | 0.98‐1.06 |
Fibrosis of lung and other chronic lung disorder (CC 109) | 16.96 | 1.08 | 1.03‐1.13 | 17.08 | 1.11 | 1.06‐1.17 |
Asthma (CC 110) | 17.05 | 0.67 | 0.63‐0.70 | 16.90 | 0.67 | 0.63‐0.70 |
Pneumonia (CC 111113) | 49.46 | 1.29 | 1.24‐1.35 | 49.41 | 1.27 | 1.22‐1.33 |
Pleural effusion/pneumothorax (CC 114) | 11.78 | 1.17 | 1.11‐1.23 | 11.54 | 1.18 | 1.12‐1.25 |
Other lung disorders (CC 115) | 53.07 | 0.80 | 0.77‐0.83 | 53.17 | 0.83 | 0.80‐0.87 |
Other comorbid conditions | ||||||
Metastatic cancer and acute leukemia (CC 7) | 2.76 | 2.34 | 2.14‐2.56 | 2.79 | 2.15 | 1.97‐2.35 |
Lung, upper digestive tract, and other severe cancers (CC 8)a | 5.98 | 1.80 | 1.68‐1.92 | 6.02 | 1.98 | 1.85‐2.11 |
Lymphatic, head and neck, brain, and other major cancers; breast, prostate, colorectal and other cancers and tumors; other respiratory and heart neoplasms (CC 911) | 14.13 | 1.03 | 0.97‐1.08 | 14.19 | 1.01 | 0.95‐1.06 |
Other digestive and urinary neoplasms (CC 12) | 6.91 | 0.91 | 0.84‐0.98 | 7.05 | 0.85 | 0.79‐0.92 |
Diabetes and DM complications (CC 1520, 119120) | 38.31 | 0.91 | 0.87‐0.94 | 38.29 | 0.91 | 0.87‐0.94 |
Protein‐calorie malnutrition (CC 21) | 7.40 | 2.18 | 2.07‐2.30 | 7.44 | 2.09 | 1.98‐2.20 |
Disorders of fluid/electrolyte/acid‐base (CC 2223) | 32.05 | 1.13 | 1.08‐1.18 | 32.16 | 1.24 | 1.19‐1.30 |
Other endocrine/metabolic/nutritional disorders (CC 24) | 67.99 | 0.75 | 0.72‐0.78 | 67.88 | 0.76 | 0.73‐0.79 |
Other gastrointestinal disorders (CC 36) | 56.21 | 0.81 | 0.78‐0.84 | 56.18 | 0.78 | 0.75‐0.81 |
Osteoarthritis of hip or knee (CC 40) | 9.32 | 0.74 | 0.69‐0.79 | 9.33 | 0.80 | 0.74‐0.85 |
Other musculoskeletal and connective tissue disorders (CC 43) | 64.14 | 0.83 | 0.80‐0.86 | 64.20 | 0.83 | 0.80‐0.87 |
Iron deficiency and other/unspecified anemias and blood disease (CC 47) | 40.80 | 1.08 | 1.04‐1.12 | 40.72 | 1.08 | 1.04‐1.13 |
Dementia and senility (CC 4950) | 17.06 | 1.09 | 1.04‐1.14 | 16.97 | 1.09 | 1.04‐1.15 |
Drug/alcohol abuse, without dependence (CC 53)a | 23.51 | 0.78 | 0.75‐0.82 | 23.38 | 0.76 | 0.72‐0.80 |
Other psychiatric disorders (CC 60)a | 16.49 | 1.12 | 1.07‐1.18 | 16.43 | 1.12 | 1.06‐1.17 |
Quadriplegia, paraplegia, functional disability (CC 6769, 100102, 177178) | 4.92 | 1.03 | 0.95‐1.12 | 4.92 | 1.08 | 0.99‐1.17 |
Mononeuropathy, other neurological conditions/emnjuries (CC 76) | 11.35 | 0.85 | 0.80‐0.91 | 11.28 | 0.88 | 0.83‐0.93 |
Hypertension and hypertensive disease (CC 9091) | 80.40 | 0.78 | 0.75‐0.82 | 80.35 | 0.79 | 0.75‐0.83 |
Stroke (CC 9596)a | 6.77 | 1.00 | 0.93‐1.08 | 6.73 | 0.98 | 0.91‐1.05 |
Retinal disorders, except detachment and vascular retinopathies (CC 121) | 10.79 | 0.87 | 0.82‐0.93 | 10.69 | 0.90 | 0.85‐0.96 |
Other eye disorders (CC 124)a | 19.05 | 0.90 | 0.86‐0.95 | 19.13 | 0.98 | 0.85‐0.93 |
Other ear, nose, throat, and mouth disorders (CC 127) | 35.21 | 0.83 | 0.80‐0.87 | 35.02 | 0.80 | 0.77‐0.83 |
Renal failure (CC 131)a | 17.92 | 1.12 | 1.07‐1.18 | 18.16 | 1.13 | 1.08‐1.19 |
Decubitus ulcer or chronic skin ulcer (CC 148149) | 7.42 | 1.27 | 1.19‐1.35 | 7.42 | 1.33 | 1.25‐1.42 |
Other dermatological disorders (CC 153) | 28.46 | 0.90 | 0.87‐0.94 | 28.32 | 0.89 | 0.86‐0.93 |
Trauma (CC 154156, 158161) | 9.04 | 1.09 | 1.03‐1.16 | 8.99 | 1.15 | 1.08‐1.22 |
Vertebral fractures (CC 157) | 5.01 | 1.33 | 1.24‐1.44 | 4.97 | 1.29 | 1.20‐1.39 |
Major complications of medical care and trauma (CC 164) | 5.47 | 0.81 | 0.75‐0.88 | 5.55 | 0.82 | 0.76‐0.89 |
Model Derivation
We used hierarchical logistic regression models to model the log‐odds of mortality as a function of patient‐level clinical characteristics and a random hospital‐level intercept. At the patient level, each model adjusts the log‐odds of mortality for age and the selected clinical covariates. The second level models the hospital‐specific intercepts as arising from a normal distribution. The hospital intercept represents the underlying risk of mortality, after accounting for patient risk. If there were no differences among hospitals, then after adjusting for patient risk, the hospital intercepts should be identical across all hospitals.
Estimation of Hospital Risk‐Standardized Mortality Rate
We calculated a risk‐standardized mortality rate, defined as the ratio of predicted to expected deaths (similar to observed‐to‐expected), multiplied by the national unadjusted mortality rate.[21] The expected number of deaths for each hospital was estimated by applying the estimated regression coefficients to the characteristics of each hospital's patients, adding the average of the hospital‐specific intercepts, transforming the data by using an inverse logit function, and summing the data from all patients in the hospital to obtain the count. The predicted number of deaths was calculated in the same way, substituting the hospital‐specific intercept for the average hospital‐specific intercept.
Model Performance, Validation, and Reliability Testing
We used the remaining admissions in 2008 as the model validation sample. We computed several summary statistics to assess the patient‐level model performance in both the development and validation samples,[22] including over‐fitting indices, predictive ability, area under the receiver operating characteristic (ROC) curve, distribution of residuals, and model 2. In addition, we assessed face validity through a survey of members of the technical expert panel. To assess reliability of the model across data years, we repeated the modeling process using qualifying COPD admissions in both 2007 and 2009. Finally, to assess generalizability we evaluated the model's performance in an all‐payer sample of data from patients admitted to California hospitals in 2006.
Analyses were conducted using SAS version 9.1.3 (SAS Institute Inc., Cary, NC). We estimated the hierarchical models using the GLIMMIX procedure in SAS.
The Human Investigation Committee at the Yale University School of Medicine/Yale New Haven Hospital approved an exemption (HIC#0903004927) for the authors to use CMS claims and enrollment data for research analyses and publication.
RESULTS
Model Derivation
After exclusions were applied, the development sample included 150,035 admissions in 2008 at 4537 US hospitals (Figure 1). Factors that were most strongly associated with the risk of mortality included metastatic cancer (odds ratio [OR] 2.34), protein calorie malnutrition (OR 2.18), nonmetastatic cancers of the lung and upper digestive tract, (OR 1.80) cardiorespiratory failure and shock (OR 1.60), and congestive heart failure (OR 1.34) (Table 2).
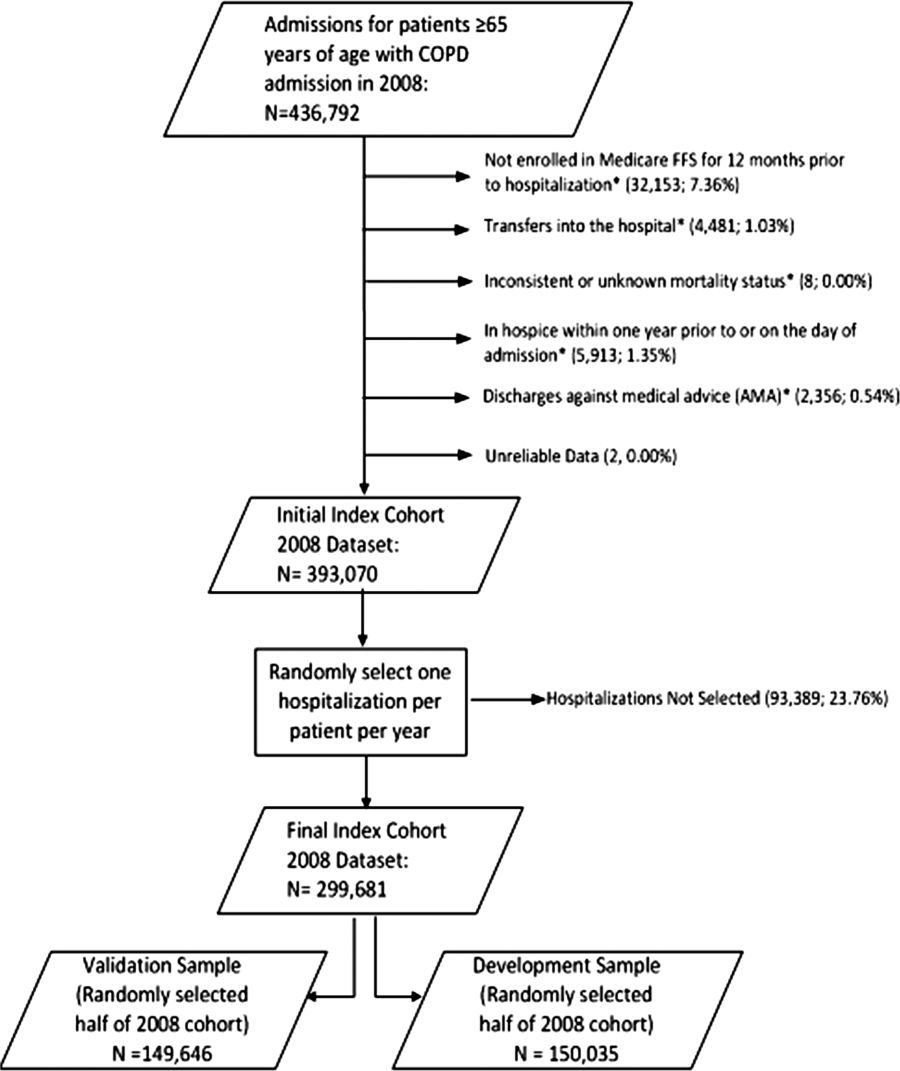
Model Performance, Validation, and Reliability
The model had a C statistic of 0.72, indicating good discrimination, and predicted mortality in the development sample ranged from 1.52% in the lowest decile to 23.74% in the highest. The model validation sample, using the remaining cases from 2008, included 149,646 admissions from 4535 hospitals. Variable frequencies and ORs were similar in both samples (Table 2). Model performance was also similar in the validation samples, with good model discrimination and fit (Table 3). Ten of 12 technical expert panel members responded to the survey, of whom 90% at least somewhat agreed with the statement, the COPD mortality measure provides an accurate reflection of quality. When the model was applied to patients age 18 years and older in the 2006 California Patient Discharge Data, overall discrimination was good (C statistic, 0.74), including in those age 18 to 64 years (C statistic, 0.75; 65 and above C statistic, 0.70).
Development | Validation | Data Years | ||
---|---|---|---|---|
Indices | Sample, 2008 | Sample, 2008 | 2007 | 2009 |
| ||||
Number of admissions | 150,035 | 149,646 | 259,911 | 279,377 |
Number of hospitals | 4537 | 4535 | 4636 | 4571 |
Mean risk‐standardized mortality rate, % (SD) | 8.62 (0.94) | 8.64 (1.07) | 8.97 (1.12) | 8.08 (1.09) |
Calibration, 0, 1 | 0.034, 0.985 | 0.009, 1.004 | 0.095, 1.022 | 0.120, 0.981 |
Discriminationpredictive ability, lowest decile %highest decile % | 1.5223.74 | 1.6023.78 | 1.5424.64 | 1.4222.36 |
Discriminationarea under the ROC curve, C statistic | 0.720 | 0.723 | 0.728 | 0.722 |
Residuals lack of fit, Pearson residual fall % | ||||
2 | 0 | 0 | 0 | 0 |
2, 0 | 91.14 | 91.4 | 91.08 | 91.93 |
0, 2 | 1.66 | 1.7 | 1.96 | 1.42 |
2+ | 6.93 | 6.91 | 6.96 | 6.65 |
Model Wald 2 (number of covariates) | 6982.11 (42) | 7051.50 (42) | 13042.35 (42) | 12542.15 (42) |
P value | <0.0001 | <0.0001 | <0.0001 | <0.0001 |
Between‐hospital variance, (standard error) | 0.067 (0.008) | 0.078 (0.009) | 0.067 (0.006) | 0.072 (0.006) |
Reliability testing demonstrated consistent performance over several years. The frequency and ORs of the variables included in the model showed only minor changes over time. The area under the ROC curve (C statistic) was 0.73 for the model in the 2007 sample and 0.72 for the model using 2009 data (Table 3).
Hospital Risk‐Standardized Mortality Rates
The mean unadjusted hospital 30‐day mortality rate was 8.6% and ranged from 0% to 100% (Figure 2a). Risk‐standardized mortality rates varied across hospitals (Figure 2b). The mean risk‐standardized mortality rate was 8.6% and ranged from 5.9% to 13.5%. The odds of mortality at a hospital 1 standard deviation above average was 1.20 times that of a hospital 1 standard deviation below average.
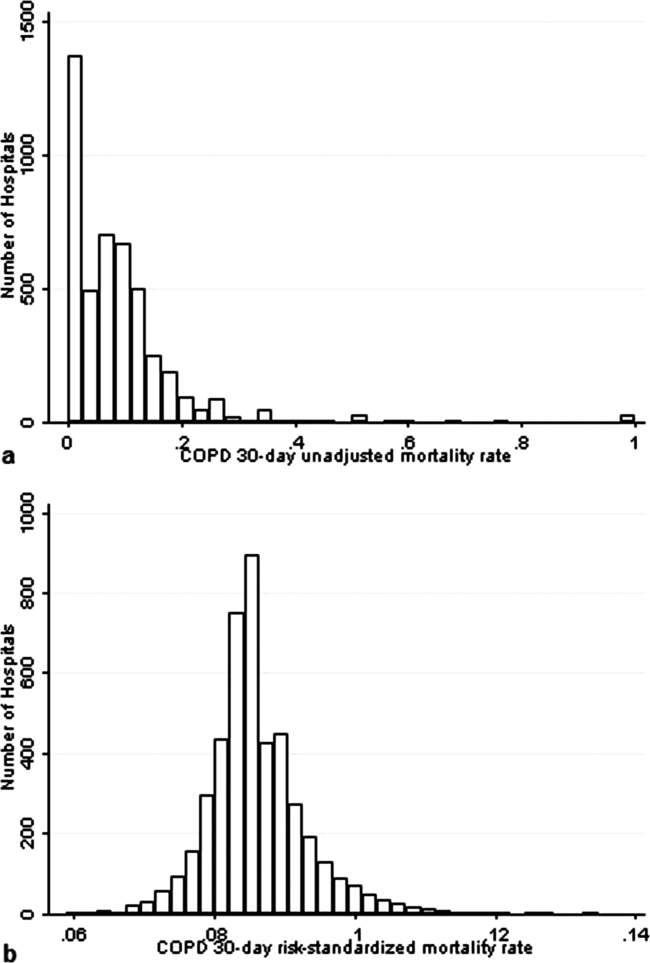
DISCUSSION
We present a hospital‐level risk‐standardized mortality measure for patients admitted with COPD based on administrative claims data that are intended for public reporting and that have achieved endorsement by the National Quality Forum, a voluntary consensus standards‐setting organization. Across more than 4500 US hospitals, the mean 30‐day risk‐standardized mortality rate in 2008 was 8.6%, and we observed considerable variation across institutions, despite adjustment for case mix, suggesting that improvement by lower‐performing institutions may be an achievable goal.
Although improving the delivery of evidence‐based care processes and outcomes of patients with acute myocardial infarction, heart failure, and pneumonia has been the focus of national quality improvement efforts for more than a decade, COPD has largely been overlooked.[23] Within this context, this analysis represents the first attempt to systematically measure, at the hospital level, 30‐day all‐cause mortality for patients admitted to US hospitals for exacerbation of COPD. The model we have developed and validated is intended to be used to compare the performance of hospitals while controlling for differences in the pretreatment risk of mortality of patients and accounting for the clustering of patients within hospitals, and will facilitate surveillance of hospital‐level risk‐adjusted outcomes over time.
In contrast to process‐based measures of quality, such as the percentage of patients with pneumonia who receive appropriate antibiotic therapy, performance measures based on patient outcomes provide a more comprehensive view of care and are more consistent with patients' goals.[24] Additionally, it is well established that hospital performance on individual and composite process measures explains only a small amount of the observed variation in patient outcomes between institutions.[25] In this regard, outcome measures incorporate important, but difficult to measure aspects of care, such as diagnostic accuracy and timing, communication and teamwork, the recognition and response to complications, care coordination at the time of transfers between levels of care, and care settings. Nevertheless, when used for making inferences about the quality of hospital care, individual measures such as the risk‐standardized hospital mortality rate should be interpreted in the context of other performance measures, including readmission, patient experience, and costs of care.
A number of prior investigators have described the outcomes of care for patients hospitalized with exacerbations of COPD, including identifying risk factors for mortality. Patil et al. carried out an analysis of the 1996 Nationwide Inpatient Sample and described an overall in‐hospital mortality rate of 2.5% among patients with COPD, and reported that a multivariable model containing sociodemographic characteristics about the patient and comorbidities had an area under the ROC curve of 0.70.[3] In contrast, this hospital‐level measure includes patients with a principal diagnosis of respiratory failure and focuses on 30‐day rather than inpatient mortality, accounting for the nearly 3‐fold higher mortality rate we observed. In a more recent study that used clinical from a large multistate database, Tabak et al. developed a prediction model for inpatient mortality for patients with COPD that contained only 4 factors: age, blood urea nitrogen, mental status, and pulse, and achieved an area under the ROC curve of 0.72.[4] The simplicity of such a model and its reliance on clinical measurements makes it particularly well suited for bedside application by clinicians, but less valuable for large‐scale public reporting programs that rely on administrative data. In the only other study identified that focused on the assessment of hospital mortality rates, Agabiti et al. analyzed the outcomes of 12,756 patients hospitalized for exacerbations of COPD, using similar ICD‐9‐CM diagnostic criteria as in this study, at 21 hospitals in Rome, Italy.[26] They reported an average crude 30‐day mortality rate of 3.8% among a group of 5 benchmark hospitals and an average mortality of 7.5% (range, 5.2%17.2%) among the remaining institutions.
To put the variation we observed in mortality rates into a broader context, the relative difference in the risk‐standardized hospital mortality rates across the 10th to 90th percentiles of hospital performance was 25% for acute myocardial infarction and 39% for heart failure, whereas rates varied 30% for COPD, from 7.6% to 9.9%.[27] Model discrimination in COPD (C statistic, 0.72) was also similar to that reported for models used for public reporting of hospital mortality in acute myocardial infarction (C statistic, 0.71) and pneumonia (C statistic, 0.72).
This study has a number of important strengths. First, the model was developed from a large sample of recent Medicare claims, achieved good discrimination, and was validated in samples not limited to Medicare beneficiaries. Second, by including patients with a principal diagnosis of COPD, as well as those with a principal diagnosis of acute respiratory failure when accompanied by a secondary diagnosis of COPD with acute exacerbation, this model can be used to assess hospital performance across the full spectrum of disease severity. This broad set of ICD‐9‐CM codes used to define the cohort also ensures that efforts to measure hospital performance will be less influenced by differences in documentation and coding practices across hospitals relating to the diagnosis or sequencing of acute respiratory failure diagnoses. Moreover, the inclusion of patients with respiratory failure is important because these patients have the greatest risk of mortality, and are those in whom efforts to improve the quality and safety of care may have the greatest impact. Third, rather than relying solely on information documented during the index admission, we used ambulatory and inpatient claims from the full year prior to the index admission to identify comorbidities and to distinguish them from potential complications of care. Finally, we did not include factors such as hospital characteristics (eg, number of beds, teaching status) in the model. Although they might have improved overall predictive ability, the goal of the hospital mortality measure is to enable comparisons of mortality rates among hospitals while controlling for differences in patient characteristics. To the extent that factors such as size or teaching status might be independently associated with hospital outcomes, it would be inappropriate to adjust away their effects, because mortality risk should not be influenced by hospital characteristics other than through their effects on quality.
These results should be viewed in light of several limitations. First, we used ICD‐9‐CM codes derived from claims files to define the patient populations included in the measure rather than collecting clinical or physiologic information prospectively or through manual review of medical records, such as the forced expiratory volume in 1 second or whether the patient required long‐term oxygen therapy. Nevertheless, we included a broad set of potential diagnosis codes to capture the full spectrum of COPD exacerbations and to minimize differences in coding across hospitals. Second, because the risk‐adjustment included diagnoses coded in the year prior to the index admission, it is potentially subject to bias due to regional differences in medical care utilization that are not driven by underlying differences in patient illness.[28] Third, using administrative claims data, we observed some paradoxical associations in the model that are difficult to explain on clinical grounds, such as a protective effect of substance and alcohol abuse or prior episodes of respiratory failure. Fourth, although we excluded patients from the analysis who were enrolled in hospice prior to, or on the day of, the index admission, we did not exclude those who choose to withdraw support, transition to comfort measures only, or enrolled in hospice care during a hospitalization. We do not seek to penalize hospitals for being sensitive to the preferences of patients at the end of life. At the same time, it is equally important that the measure is capable of detecting the outcomes of suboptimal care that may in some instances lead a patient or their family to withdraw support or choose hospice. Finally, we did not have the opportunity to validate the model against a clinical registry of patients with COPD, because such data do not currently exist. Nevertheless, the use of claims as a surrogate for chart data for risk adjustment has been validated for several conditions, including acute myocardial infarction, heart failure, and pneumonia.[29, 30]
CONCLUSIONS
Risk‐standardized 30‐day mortality rates for Medicare beneficiaries with COPD vary across hospitals in the US. Calculating and reporting hospital outcomes using validated performance measures may catalyze quality improvement activities and lead to better outcomes. Additional research would be helpful to confirm that hospitals with lower mortality rates achieve care that meets the goals of patients and their families better than at hospitals with higher mortality rates.
Acknowledgment
The authors thank the following members of the technical expert panel: Darlene Bainbridge, RN, MS, NHA, CPHQ, CPHRM, President/CEO, Darlene D. Bainbridge & Associates, Inc.; Robert A. Balk, MD, Director of Pulmonary and Critical Care Medicine, Rush University Medical Center; Dale Bratzler, DO, MPH, President and CEO, Oklahoma Foundation for Medical Quality; Scott Cerreta, RRT, Director of Education, COPD Foundation; Gerard J. Criner, MD, Director of Temple Lung Center and Divisions of Pulmonary and Critical Care Medicine, Temple University; Guy D'Andrea, MBA, President, Discern Consulting; Jonathan Fine, MD, Director of Pulmonary Fellowship, Research and Medical Education, Norwalk Hospital; David Hopkins, MS, PhD, Senior Advisor, Pacific Business Group on Health; Fred Martin Jacobs, MD, JD, FACP, FCCP, FCLM, Executive Vice President and Director, Saint Barnabas Quality Institute; Natalie Napolitano, MPH, RRT‐NPS, Respiratory Therapist, Inova Fairfax Hospital; Russell Robbins, MD, MBA, Principal and Senior Clinical Consultant, Mercer. In addition, the authors acknowledge and thank Angela Merrill, Sandi Nelson, Marian Wrobel, and Eric Schone from Mathematica Policy Research, Inc., Sharon‐Lise T. Normand from Harvard Medical School, and Lein Han and Michael Rapp at The Centers for Medicare & Medicaid Services for their contributions to this work.
Disclosures
Peter K. Lindenauer, MD, MSc, is the guarantor of this article, taking responsibility for the integrity of the work as a whole, from inception to published article, and takes responsibility for the content of the manuscript, including the data and data analysis. All authors have made substantial contributions to the conception and design, or acquisition of data, or analysis and interpretation of data; have drafted the submitted article or revised it critically for important intellectual content; and have provided final approval of the version to be published. Preparation of this manuscript was completed under Contract Number: HHSM‐5002008‐0025I/HHSM‐500‐T0001, Modification No. 000007, Option Year 2 Measure Instrument Development and Support (MIDS). Sponsors did not contribute to the development of the research or manuscript. Dr. Au reports being an unpaid research consultant for Bosch Inc. He receives research funding from the NIH, Department of Veterans Affairs, AHRQ, and Gilead Sciences. The views of the this manuscript represent the authors and do not necessarily represent those of the Department of Veterans Affairs. Drs. Drye and Bernheim report receiving contract funding from CMS to develop and maintain quality measures.
- FASTSTATS—chronic lower respiratory disease. Available at: http://www.cdc.gov/nchs/fastats/copd.htm. Accessed September 18, 2010.
- National Heart, Lung and Blood Institute. Morbidity and mortality chartbook. Available at: http://www.nhlbi.nih.gov/resources/docs/cht‐book.htm. Accessed April 27, 2010.
- In‐hospital mortality following acute exacerbations of chronic obstructive pulmonary disease. Arch Intern Med. 2003;163(10):1180–1186. , , , .
- Mortality and need for mechanical ventilation in acute exacerbations of chronic obstructive pulmonary disease: development and validation of a simple risk score. Arch Intern Med. 2009;169(17):1595–1602. , , , , .
- Quality of care for patients hospitalized for acute exacerbations of chronic obstructive pulmonary disease. Ann Intern Med. 2006;144(12):894–903. , , , , , .
- Use of beta blockers and the risk of death in hospitalised patients with acute exacerbations of COPD. Thorax. 2008;63(4):301–305. , , , , .
- HCUP facts and figures: statistics on hospital‐based care in the United States, 2007. 2009. Available at: http://www.hcup‐us.ahrq.gov/reports.jsp. Accessed August 6, 2012. , , , , .
- Predictors of long‐term survival in elderly patients hospitalized for acute exacerbations of chronic obstructive pulmonary disease. Respirology. 2008;13(6):851–855. , .
- The impact on risk‐factor analysis of different mortality outcomes in COPD patients. Eur Respir J 2008;32(3):629–636. , , , , , .
- Clinical audit indicators of outcome following admission to hospital with acute exacerbation of chronic obstructive pulmonary disease. Thorax. 2002;57(2):137–141. , , , , , .
- The quality of obstructive lung disease care for adults in the United States as measured by adherence to recommended processes. Chest. 2006;130(6):1844–1850. , , , et al.
- Management of acute exacerbations of chronic obstructive pulmonary disease in the elderly: physician practices in the community hospital setting. J Okla State Med Assoc. 2004;97(6):227–232. , , , , , .
- Leadership by Example: Coordinating Government Roles in Improving Health Care Quality. Washington, DC: National Academies Press; 2002. , , .
- Patient Protection and Affordable Care Act [H.R. 3590], Pub. L. No. 111–148, §2702, 124 Stat. 119, 318–319 (March 23, 2010). Available at: http://www.gpo.gov/fdsys/pkg/PLAW‐111publ148/html/PLAW‐111publ148.htm. Accessed July 15, 2012.
- National Quality Forum. NQF Endorses Additional Pulmonary Measure. 2013. Available at: http://www.qualityforum.org/News_And_Resources/Press_Releases/2013/NQF_Endorses_Additional_Pulmonary_Measure.aspx. Accessed January 11, 2013.
- National Quality Forum. National voluntary consensus standards for patient outcomes: a consensus report. Washington, DC: National Quality Forum; 2011.
- The Measures Management System. The Centers for Medicare and Medicaid Services. Available at: http://www.cms.gov/Medicare/Quality‐Initiatives‐Patient‐Assessment‐Instruments/MMS/index.html?redirect=/MMS/. Accessed August 6, 2012.
- Standards for statistical models used for public reporting of health outcomes: an American Heart Association Scientific Statement from the Quality of Care and Outcomes Research Interdisciplinary Writing Group: cosponsored by the Council on Epidemiology and Prevention and the Stroke Council. Endorsed by the American College of Cardiology Foundation. Circulation. 2006;113(3):456–462. , , , et al.
- Comparison of hospital risk‐standardized mortality rates calculated by using in‐hospital and 30‐day models: an observational study with implications for hospital profiling. Ann Intern Med. 2012;156(1 pt 1):19–26. , , , et al.
- Diagnostic cost group hierarchical condition category models for Medicare risk adjustment. Report prepared for the Health Care Financing Administration. Health Economics Research, Inc.; 2000. Available at: http://www.cms.gov/Research‐Statistics‐Data‐and‐Systems/Statistics‐Trends‐and‐Reports/Reports/downloads/pope_2000_2.pdf. Accessed November 7, 2009. , , , et al.
- Statistical and clinical aspects of hospital outcomes profiling. Stat Sci. 2007;22(2):206–226. , .
- Using full probability models to compute probabilities of actual interest to decision makers. Int J Technol Assess Health Care. 2001;17(1):17–26. , .
- COPD performance measures: missing opportunities for improving care. Chest. 2010;137(5):1181–1189. , , .
- Measuring Performance For Treating Heart Attacks And Heart Failure: The Case For Outcomes Measurement. Health Aff. 2007;26(1):75–85. , , , , .
- Hospital quality for acute myocardial infarction: correlation among process measures and relationship with short‐term mortality. JAMA. 2006;296(1):72–78. , , , et al.
- Profiling hospital performance to monitor the quality of care: the case of COPD. Eur Respir J. 2010;35(5):1031–1038. , , , et al.
- Patterns of hospital performance in acute myocardial infarction and heart failure 30‐day mortality and readmission. Circ Cardiovasc Qual Outcomes. 2009;2(5):407–413. , , , et al.
- Geographic variation in diagnosis frequency and risk of death among Medicare beneficiaries. JAMA. 2011;305(11):1113–1118. , , , , .
- An administrative claims model for profiling hospital 30‐day mortality rates for pneumonia patients. PLoS ONE. 2011;6(4):e17401. , , , et al.
- An Administrative Claims Model Suitable for Profiling Hospital Performance Based on 30‐Day Mortality Rates Among Patients With Heart Failure. Circulation. 2006;113(13):1693–1701. , , , et al.
Chronic obstructive pulmonary disease (COPD) affects as many as 24 million individuals in the United States, is responsible for more than 700,000 annual hospital admissions, and is currently the nation's third leading cause of death, accounting for nearly $49.9 billion in medical spending in 2010.[1, 2] Reported in‐hospital mortality rates for patients hospitalized for exacerbations of COPD range from 2% to 5%.[3, 4, 5, 6, 7] Information about 30‐day mortality rates following hospitalization for COPD is more limited; however, international studies suggest that rates range from 3% to 9%,[8, 9] and 90‐day mortality rates exceed 15%.[10]
Despite this significant clinical and economic impact, there have been no large‐scale, sustained efforts to measure the quality or outcomes of hospital care for patients with COPD in the United States. What little is known about the treatment of patients with COPD suggests widespread opportunities to increase adherence to guideline‐recommended therapies, to reduce the use of ineffective treatments and tests, and to address variation in care across institutions.[5, 11, 12]
Public reporting of hospital performance is a key strategy for improving the quality and safety of hospital care, both in the United States and internationally.[13] Since 2007, the Centers for Medicare and Medicaid Services (CMS) has reported hospital mortality rates on the Hospital Compare Web site, and COPD is 1 of the conditions highlighted in the Affordable Care Act for future consideration.[14] Such initiatives rely on validated, risk‐adjusted performance measures for comparisons across institutions and to enable outcomes to be tracked over time. We present the development, validation, and results of a model intended for public reporting of risk‐standardized mortality rates for patients hospitalized with exacerbations of COPD that has been endorsed by the National Quality Forum.[15]
METHODS
Approach to Measure Development
We developed this measure in accordance with guidelines described by the National Quality Forum,[16] CMS' Measure Management System,[17] and the American Heart Association scientific statement, Standards for Statistical Models Used for Public Reporting of Health Outcomes.[18] Throughout the process we obtained expert clinical and stakeholder input through meetings with a clinical advisory group and a national technical expert panel (see Acknowledgments). Last, we presented the proposed measure specifications and a summary of the technical expert panel discussions online and made a widely distributed call for public comments. We took the comments into consideration during the final stages of measure development (available at
Data Sources
We used claims data from Medicare inpatient, outpatient, and carrier (physician) Standard Analytic Files from 2008 to develop and validate the model, and examined model reliability using data from 2007 and 2009. The Medicare enrollment database was used to determine Medicare Fee‐for‐Service enrollment and mortality.
Study Cohort
Admissions were considered eligible for inclusion if the patient was 65 years or older, was admitted to a nonfederal acute care hospital in the United States, and had a principal diagnosis of COPD or a principal diagnosis of acute respiratory failure or respiratory arrest when paired with a secondary diagnosis of COPD with exacerbation (Table 1).
ICD‐9‐CM | Description |
---|---|
| |
491.21 | Obstructive chronic bronchitis; with (acute) exacerbation; acute exacerbation of COPD, decompensated COPD, decompensated COPD with exacerbation |
491.22 | Obstructive chronic bronchitis; with acute bronchitis |
491.8 | Other chronic bronchitis; chronic: tracheitis, tracheobronchitis |
491.9 | Unspecified chronic bronchitis |
492.8 | Other emphysema; emphysema (lung or pulmonary): NOS, centriacinar, centrilobular, obstructive, panacinar, panlobular, unilateral, vesicular; MacLeod's syndrome; Swyer‐James syndrome; unilateral hyperlucent lung |
493.20 | Chronic obstructive asthma; asthma with COPD, chronic asthmatic bronchitis, unspecified |
493.21 | Chronic obstructive asthma; asthma with COPD, chronic asthmatic bronchitis, with status asthmaticus |
493.22 | Chronic obstructive asthma; asthma with COPD, chronic asthmatic bronchitis, with (acute) exacerbation |
496 | Chronic: nonspecific lung disease, obstructive lung disease, obstructive pulmonary disease (COPD) NOS. (Note: This code is not to be used with any code from categories 491493.) |
518.81a | Other diseases of lung; acute respiratory failure; respiratory failure NOS |
518.82a | Other diseases of lung; acute respiratory failure; other pulmonary insufficiency, acute respiratory distress |
518.84a | Other diseases of lung; acute respiratory failure; acute and chronic respiratory failure |
799.1a | Other ill‐defined and unknown causes of morbidity and mortality; respiratory arrest, cardiorespiratory failure |
If a patient was discharged and readmitted to a second hospital on the same or the next day, we combined the 2 acute care admissions into a single episode of care and assigned the mortality outcome to the first admitting hospital. We excluded admissions for patients who were enrolled in Medicare Hospice in the 12 months prior to or on the first day of the index hospitalization. An index admission was any eligible admission assessed in the measure for the outcome. We also excluded admissions for patients who were discharged against medical advice, those for whom vital status at 30 days was unknown or recorded inconsistently, and patients with unreliable data (eg, age >115 years). For patients with multiple hospitalizations during a single year, we randomly selected 1 admission per patient to avoid survival bias. Finally, to assure adequate risk adjustment we limited the analysis to patients who had continuous enrollment in Medicare Fee‐for‐Service Parts A and B for the 12 months prior to their index admission so that we could identify comorbid conditions coded during all prior encounters.
Outcomes
The outcome of 30‐day mortality was defined as death from any cause within 30 days of the admission date for the index hospitalization. Mortality was assessed at 30 days to standardize the period of outcome ascertainment,[19] and because 30 days is a clinically meaningful time frame, during which differences in the quality of hospital care may be revealed.
Risk‐Adjustment Variables
We randomly selected half of all COPD admissions in 2008 that met the inclusion and exclusion criteria to create a model development sample. Candidate variables for inclusion in the risk‐standardized model were selected by a clinician team from diagnostic groups included in the Hierarchical Condition Category clinical classification system[20] and included age and comorbid conditions. Sleep apnea (International Classification of Diseases, 9th Revision, Clinical Modification [ICD‐9‐CM] condition codes 327.20, 327.21, 327.23, 327.27, 327.29, 780.51, 780.53, and 780.57) and mechanical ventilation (ICD‐9‐CM procedure codes 93.90, 96.70, 96.71, and 96.72) were also included as candidate variables.
We defined a condition as present for a given patient if it was coded in the inpatient, outpatient, or physician claims data sources in the preceding 12 months, including the index admission. Because a subset of the condition category variables can represent a complication of care, we did not consider them to be risk factors if they appeared only as secondary diagnosis codes for the index admission and not in claims submitted during the prior year.
We selected final variables for inclusion in the risk‐standardized model based on clinical considerations and a modified approach to stepwise logistic regression. The final patient‐level risk‐adjustment model included 42 variables (Table 2).
Variable | Development Sample (150,035 Admissions at 4537 Hospitals) | Validation Sample (149,646 Admissions at 4535 Hospitals) | ||||
---|---|---|---|---|---|---|
Frequency, % | OR | 95% CI | Frequency, % | OR | 95% CI | |
| ||||||
Demographics | ||||||
Age 65 years (continuous) | 1.03 | 1.03‐1.04 | 1.03 | 1.03‐1.04 | ||
Cardiovascular/respiratory | ||||||
Sleep apnea (ICD‐9‐CM: 327.20, 327.21, 327.23, 327.27, 327.29, 780.51, 780.53, 780.57)a | 9.57 | 0.87 | 0.81‐0.94 | 9.72 | 0.84 | 0.78‐0.90 |
History of mechanical ventilation (ICD‐9‐CM: 93.90, 96.70, 96.71, 96.72)a | 6.00 | 1.19 | 1.11‐1.27 | 6.00 | 1.15 | 1.08‐1.24 |
Respirator dependence/respiratory failure (CC 7778)a | 1.15 | 0.89 | 0.77‐1.02 | 1.20 | 0.78 | 0.68‐0.91 |
Cardiorespiratory failure and shock (CC 79) | 26.35 | 1.60 | 1.53‐1.68 | 26.34 | 1.59 | 1.52‐1.66 |
Congestive heart failure (CC 80) | 41.50 | 1.34 | 1.28‐1.39 | 41.39 | 1.31 | 1.25‐1.36 |
Chronic atherosclerosis (CC 8384)a | 50.44 | 0.87 | 0.83‐0.90 | 50.12 | 0.91 | 0.87‐0.94 |
Arrhythmias (CC 9293) | 37.15 | 1.17 | 1.12‐1.22 | 37.06 | 1.15 | 1.10‐1.20 |
Vascular or circulatory disease (CC 104106) | 38.20 | 1.09 | 1.05‐1.14 | 38.09 | 1.02 | 0.98‐1.06 |
Fibrosis of lung and other chronic lung disorder (CC 109) | 16.96 | 1.08 | 1.03‐1.13 | 17.08 | 1.11 | 1.06‐1.17 |
Asthma (CC 110) | 17.05 | 0.67 | 0.63‐0.70 | 16.90 | 0.67 | 0.63‐0.70 |
Pneumonia (CC 111113) | 49.46 | 1.29 | 1.24‐1.35 | 49.41 | 1.27 | 1.22‐1.33 |
Pleural effusion/pneumothorax (CC 114) | 11.78 | 1.17 | 1.11‐1.23 | 11.54 | 1.18 | 1.12‐1.25 |
Other lung disorders (CC 115) | 53.07 | 0.80 | 0.77‐0.83 | 53.17 | 0.83 | 0.80‐0.87 |
Other comorbid conditions | ||||||
Metastatic cancer and acute leukemia (CC 7) | 2.76 | 2.34 | 2.14‐2.56 | 2.79 | 2.15 | 1.97‐2.35 |
Lung, upper digestive tract, and other severe cancers (CC 8)a | 5.98 | 1.80 | 1.68‐1.92 | 6.02 | 1.98 | 1.85‐2.11 |
Lymphatic, head and neck, brain, and other major cancers; breast, prostate, colorectal and other cancers and tumors; other respiratory and heart neoplasms (CC 911) | 14.13 | 1.03 | 0.97‐1.08 | 14.19 | 1.01 | 0.95‐1.06 |
Other digestive and urinary neoplasms (CC 12) | 6.91 | 0.91 | 0.84‐0.98 | 7.05 | 0.85 | 0.79‐0.92 |
Diabetes and DM complications (CC 1520, 119120) | 38.31 | 0.91 | 0.87‐0.94 | 38.29 | 0.91 | 0.87‐0.94 |
Protein‐calorie malnutrition (CC 21) | 7.40 | 2.18 | 2.07‐2.30 | 7.44 | 2.09 | 1.98‐2.20 |
Disorders of fluid/electrolyte/acid‐base (CC 2223) | 32.05 | 1.13 | 1.08‐1.18 | 32.16 | 1.24 | 1.19‐1.30 |
Other endocrine/metabolic/nutritional disorders (CC 24) | 67.99 | 0.75 | 0.72‐0.78 | 67.88 | 0.76 | 0.73‐0.79 |
Other gastrointestinal disorders (CC 36) | 56.21 | 0.81 | 0.78‐0.84 | 56.18 | 0.78 | 0.75‐0.81 |
Osteoarthritis of hip or knee (CC 40) | 9.32 | 0.74 | 0.69‐0.79 | 9.33 | 0.80 | 0.74‐0.85 |
Other musculoskeletal and connective tissue disorders (CC 43) | 64.14 | 0.83 | 0.80‐0.86 | 64.20 | 0.83 | 0.80‐0.87 |
Iron deficiency and other/unspecified anemias and blood disease (CC 47) | 40.80 | 1.08 | 1.04‐1.12 | 40.72 | 1.08 | 1.04‐1.13 |
Dementia and senility (CC 4950) | 17.06 | 1.09 | 1.04‐1.14 | 16.97 | 1.09 | 1.04‐1.15 |
Drug/alcohol abuse, without dependence (CC 53)a | 23.51 | 0.78 | 0.75‐0.82 | 23.38 | 0.76 | 0.72‐0.80 |
Other psychiatric disorders (CC 60)a | 16.49 | 1.12 | 1.07‐1.18 | 16.43 | 1.12 | 1.06‐1.17 |
Quadriplegia, paraplegia, functional disability (CC 6769, 100102, 177178) | 4.92 | 1.03 | 0.95‐1.12 | 4.92 | 1.08 | 0.99‐1.17 |
Mononeuropathy, other neurological conditions/emnjuries (CC 76) | 11.35 | 0.85 | 0.80‐0.91 | 11.28 | 0.88 | 0.83‐0.93 |
Hypertension and hypertensive disease (CC 9091) | 80.40 | 0.78 | 0.75‐0.82 | 80.35 | 0.79 | 0.75‐0.83 |
Stroke (CC 9596)a | 6.77 | 1.00 | 0.93‐1.08 | 6.73 | 0.98 | 0.91‐1.05 |
Retinal disorders, except detachment and vascular retinopathies (CC 121) | 10.79 | 0.87 | 0.82‐0.93 | 10.69 | 0.90 | 0.85‐0.96 |
Other eye disorders (CC 124)a | 19.05 | 0.90 | 0.86‐0.95 | 19.13 | 0.98 | 0.85‐0.93 |
Other ear, nose, throat, and mouth disorders (CC 127) | 35.21 | 0.83 | 0.80‐0.87 | 35.02 | 0.80 | 0.77‐0.83 |
Renal failure (CC 131)a | 17.92 | 1.12 | 1.07‐1.18 | 18.16 | 1.13 | 1.08‐1.19 |
Decubitus ulcer or chronic skin ulcer (CC 148149) | 7.42 | 1.27 | 1.19‐1.35 | 7.42 | 1.33 | 1.25‐1.42 |
Other dermatological disorders (CC 153) | 28.46 | 0.90 | 0.87‐0.94 | 28.32 | 0.89 | 0.86‐0.93 |
Trauma (CC 154156, 158161) | 9.04 | 1.09 | 1.03‐1.16 | 8.99 | 1.15 | 1.08‐1.22 |
Vertebral fractures (CC 157) | 5.01 | 1.33 | 1.24‐1.44 | 4.97 | 1.29 | 1.20‐1.39 |
Major complications of medical care and trauma (CC 164) | 5.47 | 0.81 | 0.75‐0.88 | 5.55 | 0.82 | 0.76‐0.89 |
Model Derivation
We used hierarchical logistic regression models to model the log‐odds of mortality as a function of patient‐level clinical characteristics and a random hospital‐level intercept. At the patient level, each model adjusts the log‐odds of mortality for age and the selected clinical covariates. The second level models the hospital‐specific intercepts as arising from a normal distribution. The hospital intercept represents the underlying risk of mortality, after accounting for patient risk. If there were no differences among hospitals, then after adjusting for patient risk, the hospital intercepts should be identical across all hospitals.
Estimation of Hospital Risk‐Standardized Mortality Rate
We calculated a risk‐standardized mortality rate, defined as the ratio of predicted to expected deaths (similar to observed‐to‐expected), multiplied by the national unadjusted mortality rate.[21] The expected number of deaths for each hospital was estimated by applying the estimated regression coefficients to the characteristics of each hospital's patients, adding the average of the hospital‐specific intercepts, transforming the data by using an inverse logit function, and summing the data from all patients in the hospital to obtain the count. The predicted number of deaths was calculated in the same way, substituting the hospital‐specific intercept for the average hospital‐specific intercept.
Model Performance, Validation, and Reliability Testing
We used the remaining admissions in 2008 as the model validation sample. We computed several summary statistics to assess the patient‐level model performance in both the development and validation samples,[22] including over‐fitting indices, predictive ability, area under the receiver operating characteristic (ROC) curve, distribution of residuals, and model 2. In addition, we assessed face validity through a survey of members of the technical expert panel. To assess reliability of the model across data years, we repeated the modeling process using qualifying COPD admissions in both 2007 and 2009. Finally, to assess generalizability we evaluated the model's performance in an all‐payer sample of data from patients admitted to California hospitals in 2006.
Analyses were conducted using SAS version 9.1.3 (SAS Institute Inc., Cary, NC). We estimated the hierarchical models using the GLIMMIX procedure in SAS.
The Human Investigation Committee at the Yale University School of Medicine/Yale New Haven Hospital approved an exemption (HIC#0903004927) for the authors to use CMS claims and enrollment data for research analyses and publication.
RESULTS
Model Derivation
After exclusions were applied, the development sample included 150,035 admissions in 2008 at 4537 US hospitals (Figure 1). Factors that were most strongly associated with the risk of mortality included metastatic cancer (odds ratio [OR] 2.34), protein calorie malnutrition (OR 2.18), nonmetastatic cancers of the lung and upper digestive tract, (OR 1.80) cardiorespiratory failure and shock (OR 1.60), and congestive heart failure (OR 1.34) (Table 2).
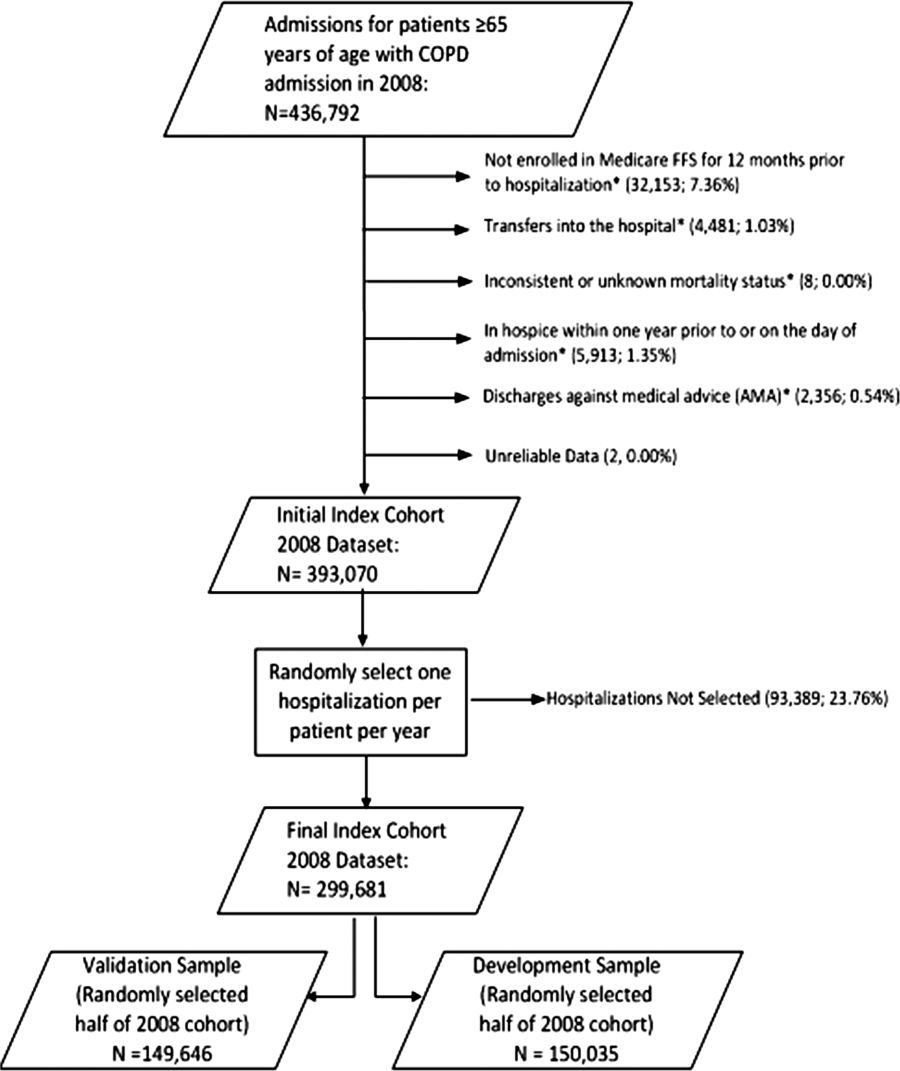
Model Performance, Validation, and Reliability
The model had a C statistic of 0.72, indicating good discrimination, and predicted mortality in the development sample ranged from 1.52% in the lowest decile to 23.74% in the highest. The model validation sample, using the remaining cases from 2008, included 149,646 admissions from 4535 hospitals. Variable frequencies and ORs were similar in both samples (Table 2). Model performance was also similar in the validation samples, with good model discrimination and fit (Table 3). Ten of 12 technical expert panel members responded to the survey, of whom 90% at least somewhat agreed with the statement, the COPD mortality measure provides an accurate reflection of quality. When the model was applied to patients age 18 years and older in the 2006 California Patient Discharge Data, overall discrimination was good (C statistic, 0.74), including in those age 18 to 64 years (C statistic, 0.75; 65 and above C statistic, 0.70).
Development | Validation | Data Years | ||
---|---|---|---|---|
Indices | Sample, 2008 | Sample, 2008 | 2007 | 2009 |
| ||||
Number of admissions | 150,035 | 149,646 | 259,911 | 279,377 |
Number of hospitals | 4537 | 4535 | 4636 | 4571 |
Mean risk‐standardized mortality rate, % (SD) | 8.62 (0.94) | 8.64 (1.07) | 8.97 (1.12) | 8.08 (1.09) |
Calibration, 0, 1 | 0.034, 0.985 | 0.009, 1.004 | 0.095, 1.022 | 0.120, 0.981 |
Discriminationpredictive ability, lowest decile %highest decile % | 1.5223.74 | 1.6023.78 | 1.5424.64 | 1.4222.36 |
Discriminationarea under the ROC curve, C statistic | 0.720 | 0.723 | 0.728 | 0.722 |
Residuals lack of fit, Pearson residual fall % | ||||
2 | 0 | 0 | 0 | 0 |
2, 0 | 91.14 | 91.4 | 91.08 | 91.93 |
0, 2 | 1.66 | 1.7 | 1.96 | 1.42 |
2+ | 6.93 | 6.91 | 6.96 | 6.65 |
Model Wald 2 (number of covariates) | 6982.11 (42) | 7051.50 (42) | 13042.35 (42) | 12542.15 (42) |
P value | <0.0001 | <0.0001 | <0.0001 | <0.0001 |
Between‐hospital variance, (standard error) | 0.067 (0.008) | 0.078 (0.009) | 0.067 (0.006) | 0.072 (0.006) |
Reliability testing demonstrated consistent performance over several years. The frequency and ORs of the variables included in the model showed only minor changes over time. The area under the ROC curve (C statistic) was 0.73 for the model in the 2007 sample and 0.72 for the model using 2009 data (Table 3).
Hospital Risk‐Standardized Mortality Rates
The mean unadjusted hospital 30‐day mortality rate was 8.6% and ranged from 0% to 100% (Figure 2a). Risk‐standardized mortality rates varied across hospitals (Figure 2b). The mean risk‐standardized mortality rate was 8.6% and ranged from 5.9% to 13.5%. The odds of mortality at a hospital 1 standard deviation above average was 1.20 times that of a hospital 1 standard deviation below average.
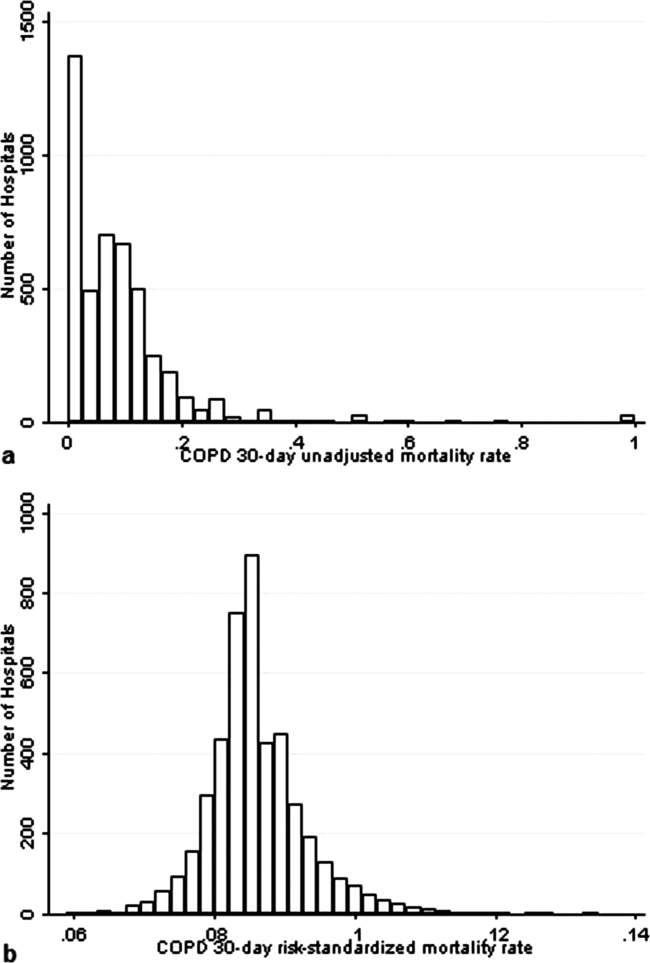
DISCUSSION
We present a hospital‐level risk‐standardized mortality measure for patients admitted with COPD based on administrative claims data that are intended for public reporting and that have achieved endorsement by the National Quality Forum, a voluntary consensus standards‐setting organization. Across more than 4500 US hospitals, the mean 30‐day risk‐standardized mortality rate in 2008 was 8.6%, and we observed considerable variation across institutions, despite adjustment for case mix, suggesting that improvement by lower‐performing institutions may be an achievable goal.
Although improving the delivery of evidence‐based care processes and outcomes of patients with acute myocardial infarction, heart failure, and pneumonia has been the focus of national quality improvement efforts for more than a decade, COPD has largely been overlooked.[23] Within this context, this analysis represents the first attempt to systematically measure, at the hospital level, 30‐day all‐cause mortality for patients admitted to US hospitals for exacerbation of COPD. The model we have developed and validated is intended to be used to compare the performance of hospitals while controlling for differences in the pretreatment risk of mortality of patients and accounting for the clustering of patients within hospitals, and will facilitate surveillance of hospital‐level risk‐adjusted outcomes over time.
In contrast to process‐based measures of quality, such as the percentage of patients with pneumonia who receive appropriate antibiotic therapy, performance measures based on patient outcomes provide a more comprehensive view of care and are more consistent with patients' goals.[24] Additionally, it is well established that hospital performance on individual and composite process measures explains only a small amount of the observed variation in patient outcomes between institutions.[25] In this regard, outcome measures incorporate important, but difficult to measure aspects of care, such as diagnostic accuracy and timing, communication and teamwork, the recognition and response to complications, care coordination at the time of transfers between levels of care, and care settings. Nevertheless, when used for making inferences about the quality of hospital care, individual measures such as the risk‐standardized hospital mortality rate should be interpreted in the context of other performance measures, including readmission, patient experience, and costs of care.
A number of prior investigators have described the outcomes of care for patients hospitalized with exacerbations of COPD, including identifying risk factors for mortality. Patil et al. carried out an analysis of the 1996 Nationwide Inpatient Sample and described an overall in‐hospital mortality rate of 2.5% among patients with COPD, and reported that a multivariable model containing sociodemographic characteristics about the patient and comorbidities had an area under the ROC curve of 0.70.[3] In contrast, this hospital‐level measure includes patients with a principal diagnosis of respiratory failure and focuses on 30‐day rather than inpatient mortality, accounting for the nearly 3‐fold higher mortality rate we observed. In a more recent study that used clinical from a large multistate database, Tabak et al. developed a prediction model for inpatient mortality for patients with COPD that contained only 4 factors: age, blood urea nitrogen, mental status, and pulse, and achieved an area under the ROC curve of 0.72.[4] The simplicity of such a model and its reliance on clinical measurements makes it particularly well suited for bedside application by clinicians, but less valuable for large‐scale public reporting programs that rely on administrative data. In the only other study identified that focused on the assessment of hospital mortality rates, Agabiti et al. analyzed the outcomes of 12,756 patients hospitalized for exacerbations of COPD, using similar ICD‐9‐CM diagnostic criteria as in this study, at 21 hospitals in Rome, Italy.[26] They reported an average crude 30‐day mortality rate of 3.8% among a group of 5 benchmark hospitals and an average mortality of 7.5% (range, 5.2%17.2%) among the remaining institutions.
To put the variation we observed in mortality rates into a broader context, the relative difference in the risk‐standardized hospital mortality rates across the 10th to 90th percentiles of hospital performance was 25% for acute myocardial infarction and 39% for heart failure, whereas rates varied 30% for COPD, from 7.6% to 9.9%.[27] Model discrimination in COPD (C statistic, 0.72) was also similar to that reported for models used for public reporting of hospital mortality in acute myocardial infarction (C statistic, 0.71) and pneumonia (C statistic, 0.72).
This study has a number of important strengths. First, the model was developed from a large sample of recent Medicare claims, achieved good discrimination, and was validated in samples not limited to Medicare beneficiaries. Second, by including patients with a principal diagnosis of COPD, as well as those with a principal diagnosis of acute respiratory failure when accompanied by a secondary diagnosis of COPD with acute exacerbation, this model can be used to assess hospital performance across the full spectrum of disease severity. This broad set of ICD‐9‐CM codes used to define the cohort also ensures that efforts to measure hospital performance will be less influenced by differences in documentation and coding practices across hospitals relating to the diagnosis or sequencing of acute respiratory failure diagnoses. Moreover, the inclusion of patients with respiratory failure is important because these patients have the greatest risk of mortality, and are those in whom efforts to improve the quality and safety of care may have the greatest impact. Third, rather than relying solely on information documented during the index admission, we used ambulatory and inpatient claims from the full year prior to the index admission to identify comorbidities and to distinguish them from potential complications of care. Finally, we did not include factors such as hospital characteristics (eg, number of beds, teaching status) in the model. Although they might have improved overall predictive ability, the goal of the hospital mortality measure is to enable comparisons of mortality rates among hospitals while controlling for differences in patient characteristics. To the extent that factors such as size or teaching status might be independently associated with hospital outcomes, it would be inappropriate to adjust away their effects, because mortality risk should not be influenced by hospital characteristics other than through their effects on quality.
These results should be viewed in light of several limitations. First, we used ICD‐9‐CM codes derived from claims files to define the patient populations included in the measure rather than collecting clinical or physiologic information prospectively or through manual review of medical records, such as the forced expiratory volume in 1 second or whether the patient required long‐term oxygen therapy. Nevertheless, we included a broad set of potential diagnosis codes to capture the full spectrum of COPD exacerbations and to minimize differences in coding across hospitals. Second, because the risk‐adjustment included diagnoses coded in the year prior to the index admission, it is potentially subject to bias due to regional differences in medical care utilization that are not driven by underlying differences in patient illness.[28] Third, using administrative claims data, we observed some paradoxical associations in the model that are difficult to explain on clinical grounds, such as a protective effect of substance and alcohol abuse or prior episodes of respiratory failure. Fourth, although we excluded patients from the analysis who were enrolled in hospice prior to, or on the day of, the index admission, we did not exclude those who choose to withdraw support, transition to comfort measures only, or enrolled in hospice care during a hospitalization. We do not seek to penalize hospitals for being sensitive to the preferences of patients at the end of life. At the same time, it is equally important that the measure is capable of detecting the outcomes of suboptimal care that may in some instances lead a patient or their family to withdraw support or choose hospice. Finally, we did not have the opportunity to validate the model against a clinical registry of patients with COPD, because such data do not currently exist. Nevertheless, the use of claims as a surrogate for chart data for risk adjustment has been validated for several conditions, including acute myocardial infarction, heart failure, and pneumonia.[29, 30]
CONCLUSIONS
Risk‐standardized 30‐day mortality rates for Medicare beneficiaries with COPD vary across hospitals in the US. Calculating and reporting hospital outcomes using validated performance measures may catalyze quality improvement activities and lead to better outcomes. Additional research would be helpful to confirm that hospitals with lower mortality rates achieve care that meets the goals of patients and their families better than at hospitals with higher mortality rates.
Acknowledgment
The authors thank the following members of the technical expert panel: Darlene Bainbridge, RN, MS, NHA, CPHQ, CPHRM, President/CEO, Darlene D. Bainbridge & Associates, Inc.; Robert A. Balk, MD, Director of Pulmonary and Critical Care Medicine, Rush University Medical Center; Dale Bratzler, DO, MPH, President and CEO, Oklahoma Foundation for Medical Quality; Scott Cerreta, RRT, Director of Education, COPD Foundation; Gerard J. Criner, MD, Director of Temple Lung Center and Divisions of Pulmonary and Critical Care Medicine, Temple University; Guy D'Andrea, MBA, President, Discern Consulting; Jonathan Fine, MD, Director of Pulmonary Fellowship, Research and Medical Education, Norwalk Hospital; David Hopkins, MS, PhD, Senior Advisor, Pacific Business Group on Health; Fred Martin Jacobs, MD, JD, FACP, FCCP, FCLM, Executive Vice President and Director, Saint Barnabas Quality Institute; Natalie Napolitano, MPH, RRT‐NPS, Respiratory Therapist, Inova Fairfax Hospital; Russell Robbins, MD, MBA, Principal and Senior Clinical Consultant, Mercer. In addition, the authors acknowledge and thank Angela Merrill, Sandi Nelson, Marian Wrobel, and Eric Schone from Mathematica Policy Research, Inc., Sharon‐Lise T. Normand from Harvard Medical School, and Lein Han and Michael Rapp at The Centers for Medicare & Medicaid Services for their contributions to this work.
Disclosures
Peter K. Lindenauer, MD, MSc, is the guarantor of this article, taking responsibility for the integrity of the work as a whole, from inception to published article, and takes responsibility for the content of the manuscript, including the data and data analysis. All authors have made substantial contributions to the conception and design, or acquisition of data, or analysis and interpretation of data; have drafted the submitted article or revised it critically for important intellectual content; and have provided final approval of the version to be published. Preparation of this manuscript was completed under Contract Number: HHSM‐5002008‐0025I/HHSM‐500‐T0001, Modification No. 000007, Option Year 2 Measure Instrument Development and Support (MIDS). Sponsors did not contribute to the development of the research or manuscript. Dr. Au reports being an unpaid research consultant for Bosch Inc. He receives research funding from the NIH, Department of Veterans Affairs, AHRQ, and Gilead Sciences. The views of the this manuscript represent the authors and do not necessarily represent those of the Department of Veterans Affairs. Drs. Drye and Bernheim report receiving contract funding from CMS to develop and maintain quality measures.
Chronic obstructive pulmonary disease (COPD) affects as many as 24 million individuals in the United States, is responsible for more than 700,000 annual hospital admissions, and is currently the nation's third leading cause of death, accounting for nearly $49.9 billion in medical spending in 2010.[1, 2] Reported in‐hospital mortality rates for patients hospitalized for exacerbations of COPD range from 2% to 5%.[3, 4, 5, 6, 7] Information about 30‐day mortality rates following hospitalization for COPD is more limited; however, international studies suggest that rates range from 3% to 9%,[8, 9] and 90‐day mortality rates exceed 15%.[10]
Despite this significant clinical and economic impact, there have been no large‐scale, sustained efforts to measure the quality or outcomes of hospital care for patients with COPD in the United States. What little is known about the treatment of patients with COPD suggests widespread opportunities to increase adherence to guideline‐recommended therapies, to reduce the use of ineffective treatments and tests, and to address variation in care across institutions.[5, 11, 12]
Public reporting of hospital performance is a key strategy for improving the quality and safety of hospital care, both in the United States and internationally.[13] Since 2007, the Centers for Medicare and Medicaid Services (CMS) has reported hospital mortality rates on the Hospital Compare Web site, and COPD is 1 of the conditions highlighted in the Affordable Care Act for future consideration.[14] Such initiatives rely on validated, risk‐adjusted performance measures for comparisons across institutions and to enable outcomes to be tracked over time. We present the development, validation, and results of a model intended for public reporting of risk‐standardized mortality rates for patients hospitalized with exacerbations of COPD that has been endorsed by the National Quality Forum.[15]
METHODS
Approach to Measure Development
We developed this measure in accordance with guidelines described by the National Quality Forum,[16] CMS' Measure Management System,[17] and the American Heart Association scientific statement, Standards for Statistical Models Used for Public Reporting of Health Outcomes.[18] Throughout the process we obtained expert clinical and stakeholder input through meetings with a clinical advisory group and a national technical expert panel (see Acknowledgments). Last, we presented the proposed measure specifications and a summary of the technical expert panel discussions online and made a widely distributed call for public comments. We took the comments into consideration during the final stages of measure development (available at
Data Sources
We used claims data from Medicare inpatient, outpatient, and carrier (physician) Standard Analytic Files from 2008 to develop and validate the model, and examined model reliability using data from 2007 and 2009. The Medicare enrollment database was used to determine Medicare Fee‐for‐Service enrollment and mortality.
Study Cohort
Admissions were considered eligible for inclusion if the patient was 65 years or older, was admitted to a nonfederal acute care hospital in the United States, and had a principal diagnosis of COPD or a principal diagnosis of acute respiratory failure or respiratory arrest when paired with a secondary diagnosis of COPD with exacerbation (Table 1).
ICD‐9‐CM | Description |
---|---|
| |
491.21 | Obstructive chronic bronchitis; with (acute) exacerbation; acute exacerbation of COPD, decompensated COPD, decompensated COPD with exacerbation |
491.22 | Obstructive chronic bronchitis; with acute bronchitis |
491.8 | Other chronic bronchitis; chronic: tracheitis, tracheobronchitis |
491.9 | Unspecified chronic bronchitis |
492.8 | Other emphysema; emphysema (lung or pulmonary): NOS, centriacinar, centrilobular, obstructive, panacinar, panlobular, unilateral, vesicular; MacLeod's syndrome; Swyer‐James syndrome; unilateral hyperlucent lung |
493.20 | Chronic obstructive asthma; asthma with COPD, chronic asthmatic bronchitis, unspecified |
493.21 | Chronic obstructive asthma; asthma with COPD, chronic asthmatic bronchitis, with status asthmaticus |
493.22 | Chronic obstructive asthma; asthma with COPD, chronic asthmatic bronchitis, with (acute) exacerbation |
496 | Chronic: nonspecific lung disease, obstructive lung disease, obstructive pulmonary disease (COPD) NOS. (Note: This code is not to be used with any code from categories 491493.) |
518.81a | Other diseases of lung; acute respiratory failure; respiratory failure NOS |
518.82a | Other diseases of lung; acute respiratory failure; other pulmonary insufficiency, acute respiratory distress |
518.84a | Other diseases of lung; acute respiratory failure; acute and chronic respiratory failure |
799.1a | Other ill‐defined and unknown causes of morbidity and mortality; respiratory arrest, cardiorespiratory failure |
If a patient was discharged and readmitted to a second hospital on the same or the next day, we combined the 2 acute care admissions into a single episode of care and assigned the mortality outcome to the first admitting hospital. We excluded admissions for patients who were enrolled in Medicare Hospice in the 12 months prior to or on the first day of the index hospitalization. An index admission was any eligible admission assessed in the measure for the outcome. We also excluded admissions for patients who were discharged against medical advice, those for whom vital status at 30 days was unknown or recorded inconsistently, and patients with unreliable data (eg, age >115 years). For patients with multiple hospitalizations during a single year, we randomly selected 1 admission per patient to avoid survival bias. Finally, to assure adequate risk adjustment we limited the analysis to patients who had continuous enrollment in Medicare Fee‐for‐Service Parts A and B for the 12 months prior to their index admission so that we could identify comorbid conditions coded during all prior encounters.
Outcomes
The outcome of 30‐day mortality was defined as death from any cause within 30 days of the admission date for the index hospitalization. Mortality was assessed at 30 days to standardize the period of outcome ascertainment,[19] and because 30 days is a clinically meaningful time frame, during which differences in the quality of hospital care may be revealed.
Risk‐Adjustment Variables
We randomly selected half of all COPD admissions in 2008 that met the inclusion and exclusion criteria to create a model development sample. Candidate variables for inclusion in the risk‐standardized model were selected by a clinician team from diagnostic groups included in the Hierarchical Condition Category clinical classification system[20] and included age and comorbid conditions. Sleep apnea (International Classification of Diseases, 9th Revision, Clinical Modification [ICD‐9‐CM] condition codes 327.20, 327.21, 327.23, 327.27, 327.29, 780.51, 780.53, and 780.57) and mechanical ventilation (ICD‐9‐CM procedure codes 93.90, 96.70, 96.71, and 96.72) were also included as candidate variables.
We defined a condition as present for a given patient if it was coded in the inpatient, outpatient, or physician claims data sources in the preceding 12 months, including the index admission. Because a subset of the condition category variables can represent a complication of care, we did not consider them to be risk factors if they appeared only as secondary diagnosis codes for the index admission and not in claims submitted during the prior year.
We selected final variables for inclusion in the risk‐standardized model based on clinical considerations and a modified approach to stepwise logistic regression. The final patient‐level risk‐adjustment model included 42 variables (Table 2).
Variable | Development Sample (150,035 Admissions at 4537 Hospitals) | Validation Sample (149,646 Admissions at 4535 Hospitals) | ||||
---|---|---|---|---|---|---|
Frequency, % | OR | 95% CI | Frequency, % | OR | 95% CI | |
| ||||||
Demographics | ||||||
Age 65 years (continuous) | 1.03 | 1.03‐1.04 | 1.03 | 1.03‐1.04 | ||
Cardiovascular/respiratory | ||||||
Sleep apnea (ICD‐9‐CM: 327.20, 327.21, 327.23, 327.27, 327.29, 780.51, 780.53, 780.57)a | 9.57 | 0.87 | 0.81‐0.94 | 9.72 | 0.84 | 0.78‐0.90 |
History of mechanical ventilation (ICD‐9‐CM: 93.90, 96.70, 96.71, 96.72)a | 6.00 | 1.19 | 1.11‐1.27 | 6.00 | 1.15 | 1.08‐1.24 |
Respirator dependence/respiratory failure (CC 7778)a | 1.15 | 0.89 | 0.77‐1.02 | 1.20 | 0.78 | 0.68‐0.91 |
Cardiorespiratory failure and shock (CC 79) | 26.35 | 1.60 | 1.53‐1.68 | 26.34 | 1.59 | 1.52‐1.66 |
Congestive heart failure (CC 80) | 41.50 | 1.34 | 1.28‐1.39 | 41.39 | 1.31 | 1.25‐1.36 |
Chronic atherosclerosis (CC 8384)a | 50.44 | 0.87 | 0.83‐0.90 | 50.12 | 0.91 | 0.87‐0.94 |
Arrhythmias (CC 9293) | 37.15 | 1.17 | 1.12‐1.22 | 37.06 | 1.15 | 1.10‐1.20 |
Vascular or circulatory disease (CC 104106) | 38.20 | 1.09 | 1.05‐1.14 | 38.09 | 1.02 | 0.98‐1.06 |
Fibrosis of lung and other chronic lung disorder (CC 109) | 16.96 | 1.08 | 1.03‐1.13 | 17.08 | 1.11 | 1.06‐1.17 |
Asthma (CC 110) | 17.05 | 0.67 | 0.63‐0.70 | 16.90 | 0.67 | 0.63‐0.70 |
Pneumonia (CC 111113) | 49.46 | 1.29 | 1.24‐1.35 | 49.41 | 1.27 | 1.22‐1.33 |
Pleural effusion/pneumothorax (CC 114) | 11.78 | 1.17 | 1.11‐1.23 | 11.54 | 1.18 | 1.12‐1.25 |
Other lung disorders (CC 115) | 53.07 | 0.80 | 0.77‐0.83 | 53.17 | 0.83 | 0.80‐0.87 |
Other comorbid conditions | ||||||
Metastatic cancer and acute leukemia (CC 7) | 2.76 | 2.34 | 2.14‐2.56 | 2.79 | 2.15 | 1.97‐2.35 |
Lung, upper digestive tract, and other severe cancers (CC 8)a | 5.98 | 1.80 | 1.68‐1.92 | 6.02 | 1.98 | 1.85‐2.11 |
Lymphatic, head and neck, brain, and other major cancers; breast, prostate, colorectal and other cancers and tumors; other respiratory and heart neoplasms (CC 911) | 14.13 | 1.03 | 0.97‐1.08 | 14.19 | 1.01 | 0.95‐1.06 |
Other digestive and urinary neoplasms (CC 12) | 6.91 | 0.91 | 0.84‐0.98 | 7.05 | 0.85 | 0.79‐0.92 |
Diabetes and DM complications (CC 1520, 119120) | 38.31 | 0.91 | 0.87‐0.94 | 38.29 | 0.91 | 0.87‐0.94 |
Protein‐calorie malnutrition (CC 21) | 7.40 | 2.18 | 2.07‐2.30 | 7.44 | 2.09 | 1.98‐2.20 |
Disorders of fluid/electrolyte/acid‐base (CC 2223) | 32.05 | 1.13 | 1.08‐1.18 | 32.16 | 1.24 | 1.19‐1.30 |
Other endocrine/metabolic/nutritional disorders (CC 24) | 67.99 | 0.75 | 0.72‐0.78 | 67.88 | 0.76 | 0.73‐0.79 |
Other gastrointestinal disorders (CC 36) | 56.21 | 0.81 | 0.78‐0.84 | 56.18 | 0.78 | 0.75‐0.81 |
Osteoarthritis of hip or knee (CC 40) | 9.32 | 0.74 | 0.69‐0.79 | 9.33 | 0.80 | 0.74‐0.85 |
Other musculoskeletal and connective tissue disorders (CC 43) | 64.14 | 0.83 | 0.80‐0.86 | 64.20 | 0.83 | 0.80‐0.87 |
Iron deficiency and other/unspecified anemias and blood disease (CC 47) | 40.80 | 1.08 | 1.04‐1.12 | 40.72 | 1.08 | 1.04‐1.13 |
Dementia and senility (CC 4950) | 17.06 | 1.09 | 1.04‐1.14 | 16.97 | 1.09 | 1.04‐1.15 |
Drug/alcohol abuse, without dependence (CC 53)a | 23.51 | 0.78 | 0.75‐0.82 | 23.38 | 0.76 | 0.72‐0.80 |
Other psychiatric disorders (CC 60)a | 16.49 | 1.12 | 1.07‐1.18 | 16.43 | 1.12 | 1.06‐1.17 |
Quadriplegia, paraplegia, functional disability (CC 6769, 100102, 177178) | 4.92 | 1.03 | 0.95‐1.12 | 4.92 | 1.08 | 0.99‐1.17 |
Mononeuropathy, other neurological conditions/emnjuries (CC 76) | 11.35 | 0.85 | 0.80‐0.91 | 11.28 | 0.88 | 0.83‐0.93 |
Hypertension and hypertensive disease (CC 9091) | 80.40 | 0.78 | 0.75‐0.82 | 80.35 | 0.79 | 0.75‐0.83 |
Stroke (CC 9596)a | 6.77 | 1.00 | 0.93‐1.08 | 6.73 | 0.98 | 0.91‐1.05 |
Retinal disorders, except detachment and vascular retinopathies (CC 121) | 10.79 | 0.87 | 0.82‐0.93 | 10.69 | 0.90 | 0.85‐0.96 |
Other eye disorders (CC 124)a | 19.05 | 0.90 | 0.86‐0.95 | 19.13 | 0.98 | 0.85‐0.93 |
Other ear, nose, throat, and mouth disorders (CC 127) | 35.21 | 0.83 | 0.80‐0.87 | 35.02 | 0.80 | 0.77‐0.83 |
Renal failure (CC 131)a | 17.92 | 1.12 | 1.07‐1.18 | 18.16 | 1.13 | 1.08‐1.19 |
Decubitus ulcer or chronic skin ulcer (CC 148149) | 7.42 | 1.27 | 1.19‐1.35 | 7.42 | 1.33 | 1.25‐1.42 |
Other dermatological disorders (CC 153) | 28.46 | 0.90 | 0.87‐0.94 | 28.32 | 0.89 | 0.86‐0.93 |
Trauma (CC 154156, 158161) | 9.04 | 1.09 | 1.03‐1.16 | 8.99 | 1.15 | 1.08‐1.22 |
Vertebral fractures (CC 157) | 5.01 | 1.33 | 1.24‐1.44 | 4.97 | 1.29 | 1.20‐1.39 |
Major complications of medical care and trauma (CC 164) | 5.47 | 0.81 | 0.75‐0.88 | 5.55 | 0.82 | 0.76‐0.89 |
Model Derivation
We used hierarchical logistic regression models to model the log‐odds of mortality as a function of patient‐level clinical characteristics and a random hospital‐level intercept. At the patient level, each model adjusts the log‐odds of mortality for age and the selected clinical covariates. The second level models the hospital‐specific intercepts as arising from a normal distribution. The hospital intercept represents the underlying risk of mortality, after accounting for patient risk. If there were no differences among hospitals, then after adjusting for patient risk, the hospital intercepts should be identical across all hospitals.
Estimation of Hospital Risk‐Standardized Mortality Rate
We calculated a risk‐standardized mortality rate, defined as the ratio of predicted to expected deaths (similar to observed‐to‐expected), multiplied by the national unadjusted mortality rate.[21] The expected number of deaths for each hospital was estimated by applying the estimated regression coefficients to the characteristics of each hospital's patients, adding the average of the hospital‐specific intercepts, transforming the data by using an inverse logit function, and summing the data from all patients in the hospital to obtain the count. The predicted number of deaths was calculated in the same way, substituting the hospital‐specific intercept for the average hospital‐specific intercept.
Model Performance, Validation, and Reliability Testing
We used the remaining admissions in 2008 as the model validation sample. We computed several summary statistics to assess the patient‐level model performance in both the development and validation samples,[22] including over‐fitting indices, predictive ability, area under the receiver operating characteristic (ROC) curve, distribution of residuals, and model 2. In addition, we assessed face validity through a survey of members of the technical expert panel. To assess reliability of the model across data years, we repeated the modeling process using qualifying COPD admissions in both 2007 and 2009. Finally, to assess generalizability we evaluated the model's performance in an all‐payer sample of data from patients admitted to California hospitals in 2006.
Analyses were conducted using SAS version 9.1.3 (SAS Institute Inc., Cary, NC). We estimated the hierarchical models using the GLIMMIX procedure in SAS.
The Human Investigation Committee at the Yale University School of Medicine/Yale New Haven Hospital approved an exemption (HIC#0903004927) for the authors to use CMS claims and enrollment data for research analyses and publication.
RESULTS
Model Derivation
After exclusions were applied, the development sample included 150,035 admissions in 2008 at 4537 US hospitals (Figure 1). Factors that were most strongly associated with the risk of mortality included metastatic cancer (odds ratio [OR] 2.34), protein calorie malnutrition (OR 2.18), nonmetastatic cancers of the lung and upper digestive tract, (OR 1.80) cardiorespiratory failure and shock (OR 1.60), and congestive heart failure (OR 1.34) (Table 2).
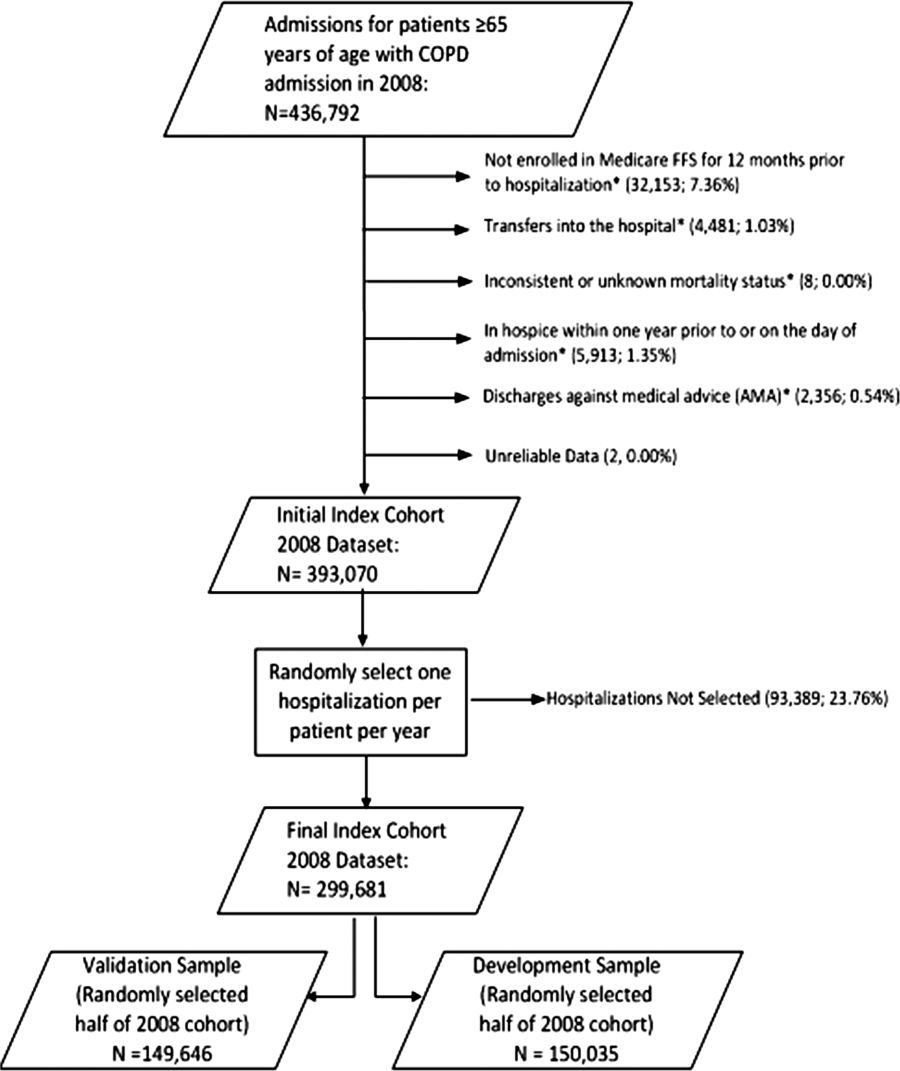
Model Performance, Validation, and Reliability
The model had a C statistic of 0.72, indicating good discrimination, and predicted mortality in the development sample ranged from 1.52% in the lowest decile to 23.74% in the highest. The model validation sample, using the remaining cases from 2008, included 149,646 admissions from 4535 hospitals. Variable frequencies and ORs were similar in both samples (Table 2). Model performance was also similar in the validation samples, with good model discrimination and fit (Table 3). Ten of 12 technical expert panel members responded to the survey, of whom 90% at least somewhat agreed with the statement, the COPD mortality measure provides an accurate reflection of quality. When the model was applied to patients age 18 years and older in the 2006 California Patient Discharge Data, overall discrimination was good (C statistic, 0.74), including in those age 18 to 64 years (C statistic, 0.75; 65 and above C statistic, 0.70).
Development | Validation | Data Years | ||
---|---|---|---|---|
Indices | Sample, 2008 | Sample, 2008 | 2007 | 2009 |
| ||||
Number of admissions | 150,035 | 149,646 | 259,911 | 279,377 |
Number of hospitals | 4537 | 4535 | 4636 | 4571 |
Mean risk‐standardized mortality rate, % (SD) | 8.62 (0.94) | 8.64 (1.07) | 8.97 (1.12) | 8.08 (1.09) |
Calibration, 0, 1 | 0.034, 0.985 | 0.009, 1.004 | 0.095, 1.022 | 0.120, 0.981 |
Discriminationpredictive ability, lowest decile %highest decile % | 1.5223.74 | 1.6023.78 | 1.5424.64 | 1.4222.36 |
Discriminationarea under the ROC curve, C statistic | 0.720 | 0.723 | 0.728 | 0.722 |
Residuals lack of fit, Pearson residual fall % | ||||
2 | 0 | 0 | 0 | 0 |
2, 0 | 91.14 | 91.4 | 91.08 | 91.93 |
0, 2 | 1.66 | 1.7 | 1.96 | 1.42 |
2+ | 6.93 | 6.91 | 6.96 | 6.65 |
Model Wald 2 (number of covariates) | 6982.11 (42) | 7051.50 (42) | 13042.35 (42) | 12542.15 (42) |
P value | <0.0001 | <0.0001 | <0.0001 | <0.0001 |
Between‐hospital variance, (standard error) | 0.067 (0.008) | 0.078 (0.009) | 0.067 (0.006) | 0.072 (0.006) |
Reliability testing demonstrated consistent performance over several years. The frequency and ORs of the variables included in the model showed only minor changes over time. The area under the ROC curve (C statistic) was 0.73 for the model in the 2007 sample and 0.72 for the model using 2009 data (Table 3).
Hospital Risk‐Standardized Mortality Rates
The mean unadjusted hospital 30‐day mortality rate was 8.6% and ranged from 0% to 100% (Figure 2a). Risk‐standardized mortality rates varied across hospitals (Figure 2b). The mean risk‐standardized mortality rate was 8.6% and ranged from 5.9% to 13.5%. The odds of mortality at a hospital 1 standard deviation above average was 1.20 times that of a hospital 1 standard deviation below average.
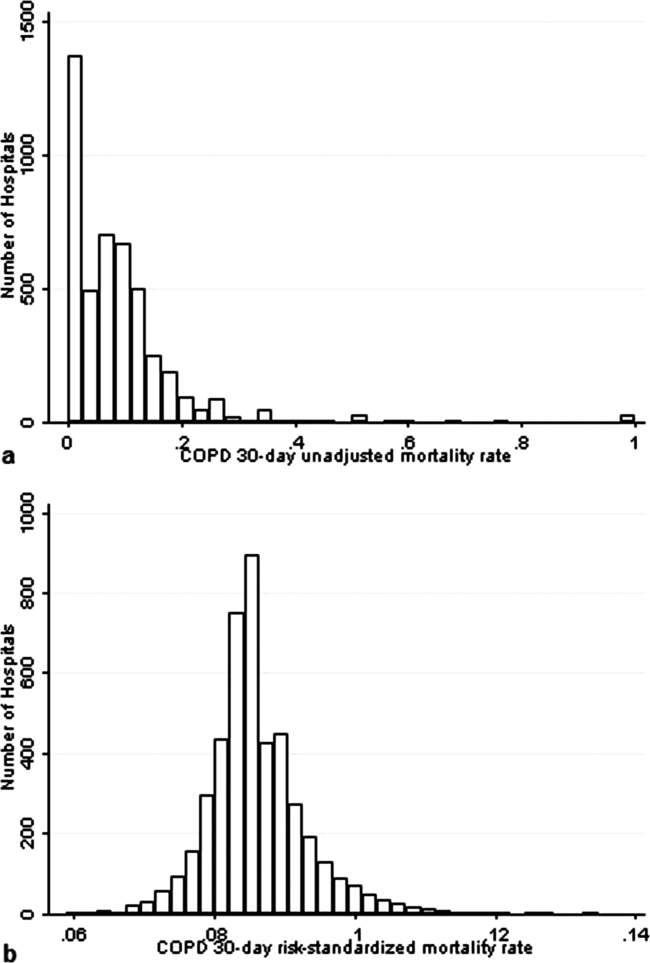
DISCUSSION
We present a hospital‐level risk‐standardized mortality measure for patients admitted with COPD based on administrative claims data that are intended for public reporting and that have achieved endorsement by the National Quality Forum, a voluntary consensus standards‐setting organization. Across more than 4500 US hospitals, the mean 30‐day risk‐standardized mortality rate in 2008 was 8.6%, and we observed considerable variation across institutions, despite adjustment for case mix, suggesting that improvement by lower‐performing institutions may be an achievable goal.
Although improving the delivery of evidence‐based care processes and outcomes of patients with acute myocardial infarction, heart failure, and pneumonia has been the focus of national quality improvement efforts for more than a decade, COPD has largely been overlooked.[23] Within this context, this analysis represents the first attempt to systematically measure, at the hospital level, 30‐day all‐cause mortality for patients admitted to US hospitals for exacerbation of COPD. The model we have developed and validated is intended to be used to compare the performance of hospitals while controlling for differences in the pretreatment risk of mortality of patients and accounting for the clustering of patients within hospitals, and will facilitate surveillance of hospital‐level risk‐adjusted outcomes over time.
In contrast to process‐based measures of quality, such as the percentage of patients with pneumonia who receive appropriate antibiotic therapy, performance measures based on patient outcomes provide a more comprehensive view of care and are more consistent with patients' goals.[24] Additionally, it is well established that hospital performance on individual and composite process measures explains only a small amount of the observed variation in patient outcomes between institutions.[25] In this regard, outcome measures incorporate important, but difficult to measure aspects of care, such as diagnostic accuracy and timing, communication and teamwork, the recognition and response to complications, care coordination at the time of transfers between levels of care, and care settings. Nevertheless, when used for making inferences about the quality of hospital care, individual measures such as the risk‐standardized hospital mortality rate should be interpreted in the context of other performance measures, including readmission, patient experience, and costs of care.
A number of prior investigators have described the outcomes of care for patients hospitalized with exacerbations of COPD, including identifying risk factors for mortality. Patil et al. carried out an analysis of the 1996 Nationwide Inpatient Sample and described an overall in‐hospital mortality rate of 2.5% among patients with COPD, and reported that a multivariable model containing sociodemographic characteristics about the patient and comorbidities had an area under the ROC curve of 0.70.[3] In contrast, this hospital‐level measure includes patients with a principal diagnosis of respiratory failure and focuses on 30‐day rather than inpatient mortality, accounting for the nearly 3‐fold higher mortality rate we observed. In a more recent study that used clinical from a large multistate database, Tabak et al. developed a prediction model for inpatient mortality for patients with COPD that contained only 4 factors: age, blood urea nitrogen, mental status, and pulse, and achieved an area under the ROC curve of 0.72.[4] The simplicity of such a model and its reliance on clinical measurements makes it particularly well suited for bedside application by clinicians, but less valuable for large‐scale public reporting programs that rely on administrative data. In the only other study identified that focused on the assessment of hospital mortality rates, Agabiti et al. analyzed the outcomes of 12,756 patients hospitalized for exacerbations of COPD, using similar ICD‐9‐CM diagnostic criteria as in this study, at 21 hospitals in Rome, Italy.[26] They reported an average crude 30‐day mortality rate of 3.8% among a group of 5 benchmark hospitals and an average mortality of 7.5% (range, 5.2%17.2%) among the remaining institutions.
To put the variation we observed in mortality rates into a broader context, the relative difference in the risk‐standardized hospital mortality rates across the 10th to 90th percentiles of hospital performance was 25% for acute myocardial infarction and 39% for heart failure, whereas rates varied 30% for COPD, from 7.6% to 9.9%.[27] Model discrimination in COPD (C statistic, 0.72) was also similar to that reported for models used for public reporting of hospital mortality in acute myocardial infarction (C statistic, 0.71) and pneumonia (C statistic, 0.72).
This study has a number of important strengths. First, the model was developed from a large sample of recent Medicare claims, achieved good discrimination, and was validated in samples not limited to Medicare beneficiaries. Second, by including patients with a principal diagnosis of COPD, as well as those with a principal diagnosis of acute respiratory failure when accompanied by a secondary diagnosis of COPD with acute exacerbation, this model can be used to assess hospital performance across the full spectrum of disease severity. This broad set of ICD‐9‐CM codes used to define the cohort also ensures that efforts to measure hospital performance will be less influenced by differences in documentation and coding practices across hospitals relating to the diagnosis or sequencing of acute respiratory failure diagnoses. Moreover, the inclusion of patients with respiratory failure is important because these patients have the greatest risk of mortality, and are those in whom efforts to improve the quality and safety of care may have the greatest impact. Third, rather than relying solely on information documented during the index admission, we used ambulatory and inpatient claims from the full year prior to the index admission to identify comorbidities and to distinguish them from potential complications of care. Finally, we did not include factors such as hospital characteristics (eg, number of beds, teaching status) in the model. Although they might have improved overall predictive ability, the goal of the hospital mortality measure is to enable comparisons of mortality rates among hospitals while controlling for differences in patient characteristics. To the extent that factors such as size or teaching status might be independently associated with hospital outcomes, it would be inappropriate to adjust away their effects, because mortality risk should not be influenced by hospital characteristics other than through their effects on quality.
These results should be viewed in light of several limitations. First, we used ICD‐9‐CM codes derived from claims files to define the patient populations included in the measure rather than collecting clinical or physiologic information prospectively or through manual review of medical records, such as the forced expiratory volume in 1 second or whether the patient required long‐term oxygen therapy. Nevertheless, we included a broad set of potential diagnosis codes to capture the full spectrum of COPD exacerbations and to minimize differences in coding across hospitals. Second, because the risk‐adjustment included diagnoses coded in the year prior to the index admission, it is potentially subject to bias due to regional differences in medical care utilization that are not driven by underlying differences in patient illness.[28] Third, using administrative claims data, we observed some paradoxical associations in the model that are difficult to explain on clinical grounds, such as a protective effect of substance and alcohol abuse or prior episodes of respiratory failure. Fourth, although we excluded patients from the analysis who were enrolled in hospice prior to, or on the day of, the index admission, we did not exclude those who choose to withdraw support, transition to comfort measures only, or enrolled in hospice care during a hospitalization. We do not seek to penalize hospitals for being sensitive to the preferences of patients at the end of life. At the same time, it is equally important that the measure is capable of detecting the outcomes of suboptimal care that may in some instances lead a patient or their family to withdraw support or choose hospice. Finally, we did not have the opportunity to validate the model against a clinical registry of patients with COPD, because such data do not currently exist. Nevertheless, the use of claims as a surrogate for chart data for risk adjustment has been validated for several conditions, including acute myocardial infarction, heart failure, and pneumonia.[29, 30]
CONCLUSIONS
Risk‐standardized 30‐day mortality rates for Medicare beneficiaries with COPD vary across hospitals in the US. Calculating and reporting hospital outcomes using validated performance measures may catalyze quality improvement activities and lead to better outcomes. Additional research would be helpful to confirm that hospitals with lower mortality rates achieve care that meets the goals of patients and their families better than at hospitals with higher mortality rates.
Acknowledgment
The authors thank the following members of the technical expert panel: Darlene Bainbridge, RN, MS, NHA, CPHQ, CPHRM, President/CEO, Darlene D. Bainbridge & Associates, Inc.; Robert A. Balk, MD, Director of Pulmonary and Critical Care Medicine, Rush University Medical Center; Dale Bratzler, DO, MPH, President and CEO, Oklahoma Foundation for Medical Quality; Scott Cerreta, RRT, Director of Education, COPD Foundation; Gerard J. Criner, MD, Director of Temple Lung Center and Divisions of Pulmonary and Critical Care Medicine, Temple University; Guy D'Andrea, MBA, President, Discern Consulting; Jonathan Fine, MD, Director of Pulmonary Fellowship, Research and Medical Education, Norwalk Hospital; David Hopkins, MS, PhD, Senior Advisor, Pacific Business Group on Health; Fred Martin Jacobs, MD, JD, FACP, FCCP, FCLM, Executive Vice President and Director, Saint Barnabas Quality Institute; Natalie Napolitano, MPH, RRT‐NPS, Respiratory Therapist, Inova Fairfax Hospital; Russell Robbins, MD, MBA, Principal and Senior Clinical Consultant, Mercer. In addition, the authors acknowledge and thank Angela Merrill, Sandi Nelson, Marian Wrobel, and Eric Schone from Mathematica Policy Research, Inc., Sharon‐Lise T. Normand from Harvard Medical School, and Lein Han and Michael Rapp at The Centers for Medicare & Medicaid Services for their contributions to this work.
Disclosures
Peter K. Lindenauer, MD, MSc, is the guarantor of this article, taking responsibility for the integrity of the work as a whole, from inception to published article, and takes responsibility for the content of the manuscript, including the data and data analysis. All authors have made substantial contributions to the conception and design, or acquisition of data, or analysis and interpretation of data; have drafted the submitted article or revised it critically for important intellectual content; and have provided final approval of the version to be published. Preparation of this manuscript was completed under Contract Number: HHSM‐5002008‐0025I/HHSM‐500‐T0001, Modification No. 000007, Option Year 2 Measure Instrument Development and Support (MIDS). Sponsors did not contribute to the development of the research or manuscript. Dr. Au reports being an unpaid research consultant for Bosch Inc. He receives research funding from the NIH, Department of Veterans Affairs, AHRQ, and Gilead Sciences. The views of the this manuscript represent the authors and do not necessarily represent those of the Department of Veterans Affairs. Drs. Drye and Bernheim report receiving contract funding from CMS to develop and maintain quality measures.
- FASTSTATS—chronic lower respiratory disease. Available at: http://www.cdc.gov/nchs/fastats/copd.htm. Accessed September 18, 2010.
- National Heart, Lung and Blood Institute. Morbidity and mortality chartbook. Available at: http://www.nhlbi.nih.gov/resources/docs/cht‐book.htm. Accessed April 27, 2010.
- In‐hospital mortality following acute exacerbations of chronic obstructive pulmonary disease. Arch Intern Med. 2003;163(10):1180–1186. , , , .
- Mortality and need for mechanical ventilation in acute exacerbations of chronic obstructive pulmonary disease: development and validation of a simple risk score. Arch Intern Med. 2009;169(17):1595–1602. , , , , .
- Quality of care for patients hospitalized for acute exacerbations of chronic obstructive pulmonary disease. Ann Intern Med. 2006;144(12):894–903. , , , , , .
- Use of beta blockers and the risk of death in hospitalised patients with acute exacerbations of COPD. Thorax. 2008;63(4):301–305. , , , , .
- HCUP facts and figures: statistics on hospital‐based care in the United States, 2007. 2009. Available at: http://www.hcup‐us.ahrq.gov/reports.jsp. Accessed August 6, 2012. , , , , .
- Predictors of long‐term survival in elderly patients hospitalized for acute exacerbations of chronic obstructive pulmonary disease. Respirology. 2008;13(6):851–855. , .
- The impact on risk‐factor analysis of different mortality outcomes in COPD patients. Eur Respir J 2008;32(3):629–636. , , , , , .
- Clinical audit indicators of outcome following admission to hospital with acute exacerbation of chronic obstructive pulmonary disease. Thorax. 2002;57(2):137–141. , , , , , .
- The quality of obstructive lung disease care for adults in the United States as measured by adherence to recommended processes. Chest. 2006;130(6):1844–1850. , , , et al.
- Management of acute exacerbations of chronic obstructive pulmonary disease in the elderly: physician practices in the community hospital setting. J Okla State Med Assoc. 2004;97(6):227–232. , , , , , .
- Leadership by Example: Coordinating Government Roles in Improving Health Care Quality. Washington, DC: National Academies Press; 2002. , , .
- Patient Protection and Affordable Care Act [H.R. 3590], Pub. L. No. 111–148, §2702, 124 Stat. 119, 318–319 (March 23, 2010). Available at: http://www.gpo.gov/fdsys/pkg/PLAW‐111publ148/html/PLAW‐111publ148.htm. Accessed July 15, 2012.
- National Quality Forum. NQF Endorses Additional Pulmonary Measure. 2013. Available at: http://www.qualityforum.org/News_And_Resources/Press_Releases/2013/NQF_Endorses_Additional_Pulmonary_Measure.aspx. Accessed January 11, 2013.
- National Quality Forum. National voluntary consensus standards for patient outcomes: a consensus report. Washington, DC: National Quality Forum; 2011.
- The Measures Management System. The Centers for Medicare and Medicaid Services. Available at: http://www.cms.gov/Medicare/Quality‐Initiatives‐Patient‐Assessment‐Instruments/MMS/index.html?redirect=/MMS/. Accessed August 6, 2012.
- Standards for statistical models used for public reporting of health outcomes: an American Heart Association Scientific Statement from the Quality of Care and Outcomes Research Interdisciplinary Writing Group: cosponsored by the Council on Epidemiology and Prevention and the Stroke Council. Endorsed by the American College of Cardiology Foundation. Circulation. 2006;113(3):456–462. , , , et al.
- Comparison of hospital risk‐standardized mortality rates calculated by using in‐hospital and 30‐day models: an observational study with implications for hospital profiling. Ann Intern Med. 2012;156(1 pt 1):19–26. , , , et al.
- Diagnostic cost group hierarchical condition category models for Medicare risk adjustment. Report prepared for the Health Care Financing Administration. Health Economics Research, Inc.; 2000. Available at: http://www.cms.gov/Research‐Statistics‐Data‐and‐Systems/Statistics‐Trends‐and‐Reports/Reports/downloads/pope_2000_2.pdf. Accessed November 7, 2009. , , , et al.
- Statistical and clinical aspects of hospital outcomes profiling. Stat Sci. 2007;22(2):206–226. , .
- Using full probability models to compute probabilities of actual interest to decision makers. Int J Technol Assess Health Care. 2001;17(1):17–26. , .
- COPD performance measures: missing opportunities for improving care. Chest. 2010;137(5):1181–1189. , , .
- Measuring Performance For Treating Heart Attacks And Heart Failure: The Case For Outcomes Measurement. Health Aff. 2007;26(1):75–85. , , , , .
- Hospital quality for acute myocardial infarction: correlation among process measures and relationship with short‐term mortality. JAMA. 2006;296(1):72–78. , , , et al.
- Profiling hospital performance to monitor the quality of care: the case of COPD. Eur Respir J. 2010;35(5):1031–1038. , , , et al.
- Patterns of hospital performance in acute myocardial infarction and heart failure 30‐day mortality and readmission. Circ Cardiovasc Qual Outcomes. 2009;2(5):407–413. , , , et al.
- Geographic variation in diagnosis frequency and risk of death among Medicare beneficiaries. JAMA. 2011;305(11):1113–1118. , , , , .
- An administrative claims model for profiling hospital 30‐day mortality rates for pneumonia patients. PLoS ONE. 2011;6(4):e17401. , , , et al.
- An Administrative Claims Model Suitable for Profiling Hospital Performance Based on 30‐Day Mortality Rates Among Patients With Heart Failure. Circulation. 2006;113(13):1693–1701. , , , et al.
- FASTSTATS—chronic lower respiratory disease. Available at: http://www.cdc.gov/nchs/fastats/copd.htm. Accessed September 18, 2010.
- National Heart, Lung and Blood Institute. Morbidity and mortality chartbook. Available at: http://www.nhlbi.nih.gov/resources/docs/cht‐book.htm. Accessed April 27, 2010.
- In‐hospital mortality following acute exacerbations of chronic obstructive pulmonary disease. Arch Intern Med. 2003;163(10):1180–1186. , , , .
- Mortality and need for mechanical ventilation in acute exacerbations of chronic obstructive pulmonary disease: development and validation of a simple risk score. Arch Intern Med. 2009;169(17):1595–1602. , , , , .
- Quality of care for patients hospitalized for acute exacerbations of chronic obstructive pulmonary disease. Ann Intern Med. 2006;144(12):894–903. , , , , , .
- Use of beta blockers and the risk of death in hospitalised patients with acute exacerbations of COPD. Thorax. 2008;63(4):301–305. , , , , .
- HCUP facts and figures: statistics on hospital‐based care in the United States, 2007. 2009. Available at: http://www.hcup‐us.ahrq.gov/reports.jsp. Accessed August 6, 2012. , , , , .
- Predictors of long‐term survival in elderly patients hospitalized for acute exacerbations of chronic obstructive pulmonary disease. Respirology. 2008;13(6):851–855. , .
- The impact on risk‐factor analysis of different mortality outcomes in COPD patients. Eur Respir J 2008;32(3):629–636. , , , , , .
- Clinical audit indicators of outcome following admission to hospital with acute exacerbation of chronic obstructive pulmonary disease. Thorax. 2002;57(2):137–141. , , , , , .
- The quality of obstructive lung disease care for adults in the United States as measured by adherence to recommended processes. Chest. 2006;130(6):1844–1850. , , , et al.
- Management of acute exacerbations of chronic obstructive pulmonary disease in the elderly: physician practices in the community hospital setting. J Okla State Med Assoc. 2004;97(6):227–232. , , , , , .
- Leadership by Example: Coordinating Government Roles in Improving Health Care Quality. Washington, DC: National Academies Press; 2002. , , .
- Patient Protection and Affordable Care Act [H.R. 3590], Pub. L. No. 111–148, §2702, 124 Stat. 119, 318–319 (March 23, 2010). Available at: http://www.gpo.gov/fdsys/pkg/PLAW‐111publ148/html/PLAW‐111publ148.htm. Accessed July 15, 2012.
- National Quality Forum. NQF Endorses Additional Pulmonary Measure. 2013. Available at: http://www.qualityforum.org/News_And_Resources/Press_Releases/2013/NQF_Endorses_Additional_Pulmonary_Measure.aspx. Accessed January 11, 2013.
- National Quality Forum. National voluntary consensus standards for patient outcomes: a consensus report. Washington, DC: National Quality Forum; 2011.
- The Measures Management System. The Centers for Medicare and Medicaid Services. Available at: http://www.cms.gov/Medicare/Quality‐Initiatives‐Patient‐Assessment‐Instruments/MMS/index.html?redirect=/MMS/. Accessed August 6, 2012.
- Standards for statistical models used for public reporting of health outcomes: an American Heart Association Scientific Statement from the Quality of Care and Outcomes Research Interdisciplinary Writing Group: cosponsored by the Council on Epidemiology and Prevention and the Stroke Council. Endorsed by the American College of Cardiology Foundation. Circulation. 2006;113(3):456–462. , , , et al.
- Comparison of hospital risk‐standardized mortality rates calculated by using in‐hospital and 30‐day models: an observational study with implications for hospital profiling. Ann Intern Med. 2012;156(1 pt 1):19–26. , , , et al.
- Diagnostic cost group hierarchical condition category models for Medicare risk adjustment. Report prepared for the Health Care Financing Administration. Health Economics Research, Inc.; 2000. Available at: http://www.cms.gov/Research‐Statistics‐Data‐and‐Systems/Statistics‐Trends‐and‐Reports/Reports/downloads/pope_2000_2.pdf. Accessed November 7, 2009. , , , et al.
- Statistical and clinical aspects of hospital outcomes profiling. Stat Sci. 2007;22(2):206–226. , .
- Using full probability models to compute probabilities of actual interest to decision makers. Int J Technol Assess Health Care. 2001;17(1):17–26. , .
- COPD performance measures: missing opportunities for improving care. Chest. 2010;137(5):1181–1189. , , .
- Measuring Performance For Treating Heart Attacks And Heart Failure: The Case For Outcomes Measurement. Health Aff. 2007;26(1):75–85. , , , , .
- Hospital quality for acute myocardial infarction: correlation among process measures and relationship with short‐term mortality. JAMA. 2006;296(1):72–78. , , , et al.
- Profiling hospital performance to monitor the quality of care: the case of COPD. Eur Respir J. 2010;35(5):1031–1038. , , , et al.
- Patterns of hospital performance in acute myocardial infarction and heart failure 30‐day mortality and readmission. Circ Cardiovasc Qual Outcomes. 2009;2(5):407–413. , , , et al.
- Geographic variation in diagnosis frequency and risk of death among Medicare beneficiaries. JAMA. 2011;305(11):1113–1118. , , , , .
- An administrative claims model for profiling hospital 30‐day mortality rates for pneumonia patients. PLoS ONE. 2011;6(4):e17401. , , , et al.
- An Administrative Claims Model Suitable for Profiling Hospital Performance Based on 30‐Day Mortality Rates Among Patients With Heart Failure. Circulation. 2006;113(13):1693–1701. , , , et al.
Copyright © 2013 Society of Hospital Medicine