User login
An Official Publication of the Society of Hospital Medicine
Copyright © by Society of Hospital Medicine or related companies. All rights reserved. ISSN 1553-5606
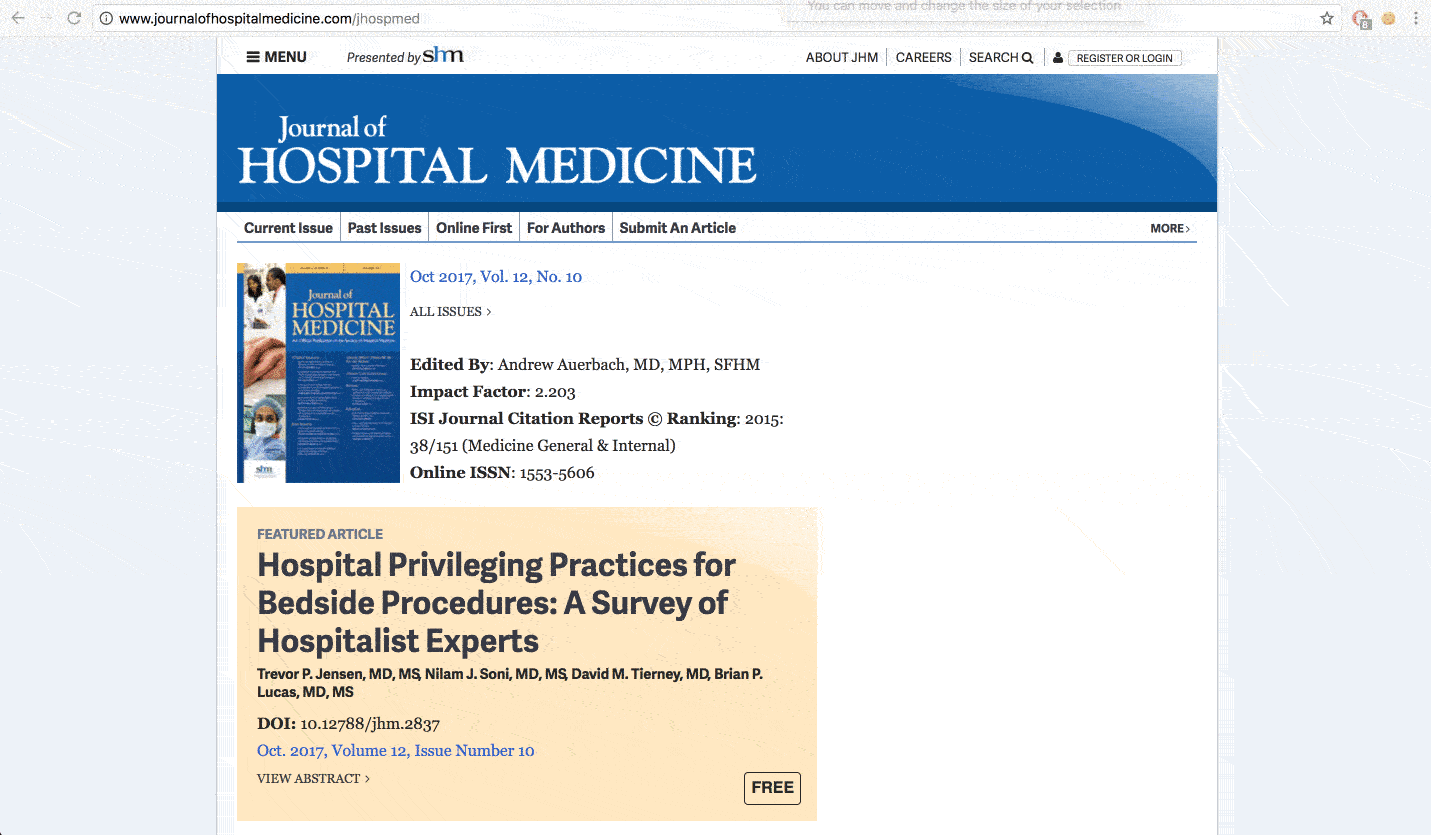
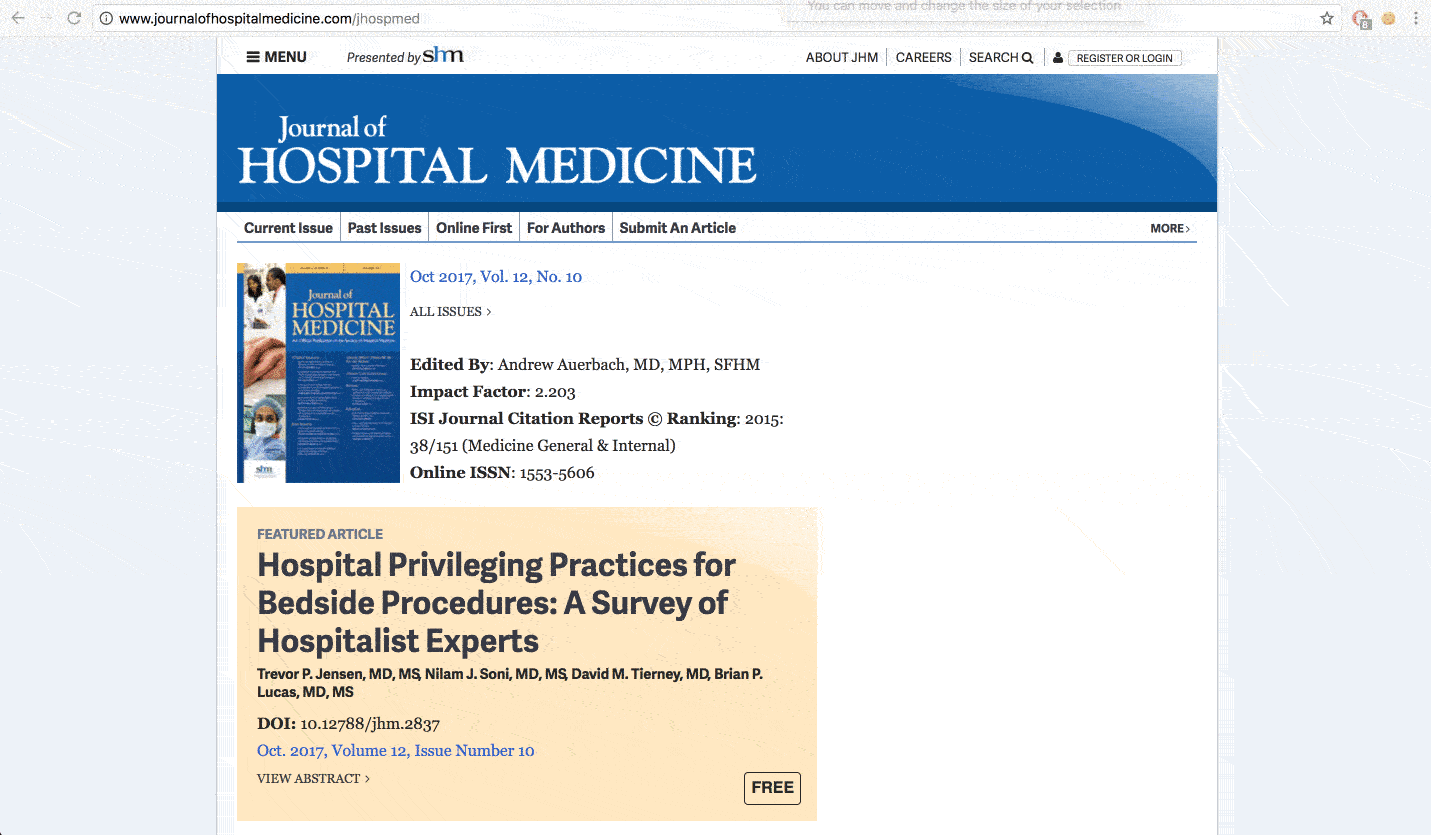
Improving Healthcare Value: Managing Length of Stay and Improving the Hospital Medicine Value Proposition
Healthcare payment model reform has increased pressure on healthcare systems and hospitalists to improve efficiency and reduce the cost of care. These pressures on the healthcare system have been exacerbated by a global pandemic and an aging patient population straining hospital capacity and resources. Hospital capacity constraints may contribute to hospital crowding and can compromise patient outcomes.1 Increasing hospital capacity also contributes to an increase in hospitalist census. This increase in census is accompanied by proportional increases in hospitalist burnout, cost of care, and prolonged length of stay (LOS).2 Managing LOS reduces “waste” (or non–value-added inpatient days) and can improve outcomes and efficiency within the hospital system.
The benefits for LOS reduction when patients are managed by hospitalists compared with primary care practitioners are well described and are associated with decreases in average LOS and cost.3-5 The shorter LOS with hospitalist care is most pronounced in older patients with more complex disease processes, which has temporal importance. The Department of Health and Human Services expects the number of American adults aged >65 years to approach 72 million (20% of the US population) by 2030. Hospitalists are positioned to drive evidence-based care pathways and improve the quality of patient care in this growing patient population. We examine the reasons for managing LOS, summarize factors that contribute to an increased LOS (“waste”), and propose a list of evidence-based value drivers for LOS reduction (Table).2,6-17 Our experience utilizing this approach within Cleveland Clinic Florida following implementation of many of these evidence-based strategies to reduce non–value-added hospital days is also described in the Appendix Figure.
WHY MANAGE LOS?
Barriers to sustainable LOS-reduction strategies have evolved, in part, since the introduction of the Medicare Prospective Payment System, which moved hospital Medicare payments to a predetermined fixed rate for each diagnosis-related group. This led to financial pressures on healthcare systems to identify methods to reduce cost and, in turn, contributed to an increase in postacute facility utilization, with alternative payment models developing in parallel.18,19 These changes along with disaggregated payments between hospitals and postacute facilities have created a formidable challenge to LOS and cost-reduction plans.19
The usual “why” for reducing LOS includes improving constraints on hospital capacity, strains on resources, and deleterious outcomes. In our experience, an evidence-based approach to LOS management should focus on: (1) reduction in patient hospital days through decreased care variation; (2) stabilizing hospitalist workloads; (3) minimizing the fragmentation inherent to the hospitalist care delivery model; and (4) developing service lines to manage patients hospitalized in an observation status and for those patients undergoing procedures deemed medically complex. The literature is mixed on the impact of LOS reductions on other clinical end points, such as readmissions or mortality, with the preponderance indicating no deleterious impact.20-22 Managing LOS using an evidence-based approach that addresses the variability of individual patients is essential to the LOS strategies employed. These strategies should focus on process improvements to drive LOS reduction and utilize metrics under the individual hospitalist control to support their contribution to the hospitalist groups’ overall LOS.23
IMPROVING HOSPITALIST VALUE AROUND LOS MANAGEMENT
Intrinsic factors such as hospitalist staffing fragmentation, high rounding census, failing to prioritize patients ready to be discharged, variability in practice, number of consultants per patient, and hospitalist behaviors contribute to increased LOS.2,6,8 A first precept to management of LOS at the group level is to recognize all hospitalist services are not created equal, and “lumping” hospitalists into a single efficiency metric would not yield actionable information.
The literature is rife with examples of the significant variation in practice styles among hospitalists. A large study including more than 1000 hospitalists identified practice variation as the strongest predictor of variations in mean LOS.7 While Goodwin et al7 identified significant variation among hospitalists’ LOS and the discharge destination of patients, much of the variation could be attributable to the hospitals where they practice. These findings ostensibly highlight the importance of LOS strategies being developed collaboratively among hospitalist groups and the healthcare systems they serve. Similar variation exists among hospitalists on teaching services versus nonteaching services. Our experience parallels that of other studies with regard to teaching services that have found that hospitalists on teaching services often have additional responsibilities and are less able to gain the efficiency of nonresident hospitalists services.3 The impact of teaching services on hospitalist efficiencies is an important component when setting expectations at the hospitalist group level for providers on academic services.
Workload and staffing models for hospitalists have a significant impact on hospitalist efficiency and LOS management. As workload increased, Elliot and colleagues2 identified a proportional increase in LOS. For occupancies of 75% to 85%, LOS increased exponentially above a daily relative value unit of approximately 25 and a census value of approximately 15. The magnitude of this difference in LOS and cost across the range of hospitalist workloads was $262, with an average increase in LOS of 2 days for every unit increase in census. Higher workloads contributed to inferior discussion of treatment options with patients; delays in discharges; delays in placing discharge orders; and unnecessary testing, procedures, and consults.14 To mitigate inefficiency and adverse impacts of higher workloads, hospitalist groups should develop mechanisms to absorb surges in census and unanticipated changes to staffing maintaining the workload within a range appropriate to the patient population.
Decreasing fragmentation, when multiple hospitalists care for the patient during hospitalization, is a necessary component of any LOS-reduction strategy. Studies of pneumonia and heart failure have demonstrated that a 10% increase in hosptialist fragmentation is associated with significant increases in LOS.24 Schedules with hospitalists on 7-day rotating rounding blocks have the intuitive advantage of improving care continuity for patients compared with schedules with a shorter number of consecutive rounding days, resulting in fewer hospitalists caring for each patient and decreased “fragmentation.” Additional value drivers for LOS reduction strategies for hospitalists are listed in the Table.
The 2018 State of Hospital Medicine Report highlighted that, among patients discharged by hospitalist groups, 80.8% were inpatient and 19.2% were outpatient. With nearly one in five patients discharged in observation status, it behooves hospitalist programs to work to effectively manage these patients. Indeed, hospitalist-run observation units have been shown to decrease LOS significantly without an increase in return rates to the emergency department or hospital compared with patients managed prior to the introduction of a dedicated observation unit.9
Although an in-depth discussion is beyond the scope of the present article, it is worth noting the value of hospitalist comanagement (HCoM) strategies. The impact of HCoM teams is demonstrated by reductions in LOS and cost of care resulting from decreases in medical complications, number of consultants per patient, and a decrease in 30-day readmsissions.12 The Society of Hospital Medicine Perioperative Care Work Group has outlined a collaborative framework for hospitalists and healthcare systems to draw from.15
THE CLEVELAND CLINIC INDIAN RIVER HOSPITAL EXPERIENCE
Within the Cleveland Clinic Indian River Hospital (CCIRH) medicine department, many of the aforementioned strategies and tactics were standardized among hospitalist providers. Hospitalists at CCIRH are scheduled on 7-day rotating blocks to reduce fragmentation. In 2019, we targeted a range of 15 to 18 patient contacts per rounding hospitalist per day and utilized a back-up call system to stabilize the hospitalist census. The hospitalist service lines are enhanced through HCoM services with patients cohorted on dedicated HCoM teams. The follow-up to discharge ratio is used to provide feedback at the provider level as both a management and assessment tool.23 The rounding and admitting teams are dedicated to their responsibility (with the occasional exception necessitating the rounding team assist with admissions when the volumes are high). Direct admissions and transfers from outside hospitals are managed by a dedicated hospital medicine “quarterback” to minimize disruption of the admitting and rounding teams. Barriers to discharge are identified at the time of admission by care management and aggressively managed. Prolonged LOS reports are generated daily and disseminated to care managers and physician leadership. In January 2019, the average LOS for inpatients at CCIRH was 4.4 days. In December 2019, the average LOS for the calendar year to-date at CCIRH was 3.9 days (Appendix Figure).
The value proposition for managing LOS should be viewed in the context of the total cost of care over an extended period of time and not viewed in isolation. Readmission rates serve as a counterbalance to LOS-reduction strategies and contribute to higher costs of care when increased. The 30-day readmission rate for this cohort over this same time period was down slightly compared with the previous year to 12.1%. In addition, observation patients at CCIRH are managed in a closed, geographically cohorted unit, staffed by dedicated advanced-practice providers and physicians dedicated to observation medicine. Over this same time period, more than 5500 patients were managed in the observation unit. These patients had an average LOS of 19.2 hours, with approximately four out of every five patients being discharged to home from an observation status.
The impact of COVID-19 and higher hospital volumes are best visualized in the Appendix Figure. Increases in LOS were observed during periods of COVID-19–related “surges” in hospital volume. These reversals in LOS trends during periods of high occupancy echo earlier findings by Elliot et al2 showing that external factors that are not directly under the control of the hospitalist drive LOS and must be considered when developing LOS reduction strategies.
CONCLUSION
The shift toward value-based payment models provides a strong tailwind for healthcare systems to manage LOS. Hospitalists are well positioned to drive LOS-reduction strategies for the healthcare systems they serve and provide value by driving both quality and efficiency. A complete realization of the value proposition of hospitalist programs in driving LOS-reduction initiatives requires the healthcare systems they serve to provide these teams with the appropriate resources and tools.
1. Eriksson CO, Stoner RC, Eden KB, Newgard CD, Guise J-M. The association between hospital capacity strain and inpatient outcomes in highly developed countries: a systematic review. J Gen Intern Med. 2017;32(6):686-696. https://doi.org/10.1007/s11606-016-3936-3
2. Elliott DJ, Young RS, Brice J, Aguiar R, Kolm P. Effect of hospitalist workload on the quality and efficiency of care. JAMA Intern Med. 2014;174(5):786-793. https://doi.org/10.1001/jamainternmed.2014.300
3. Rachoin JS, Skaf J, Cerceo E, et al. The impact of hospitalists on length of stay and costs: systematic review and meta-analysis. Am J Manag Care. 2012;18(1):e23-30.
4. Kuo YF, Goodwin JS. Effect of hospitalists on length of stay in the medicare population: variation according to hospital and patient characteristics. J Am Geriatr Soc. 2010;58(9):1649-1657. https://doi.org/10.1111/j.1532-5415.2010.03007.x
5. Lindenauer PK, Rothberg MB, Pekow PS, Kenwood C, Benjamin EM, Auerbach AD. Outcomes of care by hospitalists, general internists, and family physicians. N Engl J Med. 2007;357(25):2589-2600. https://doi.org/10.1056/NEJMsa067735
6. Epstein K, Juarez E, Epstein A, Loya K, Singer A. The impact of fragmentation of hospitalist care on length of stay. J Hosp Med. 2010;5(6):335-338. https://doi.org/10.1002/jhm.675
7. Goodwin JS, Lin Y-L, Singh S, Kuo Y-F. Variation in length of stay and outcomes among hospitalized patients attributable to hospitals and hospitalists. J Gen Intern Med. 2013;28(3):370-376. https://doi.org/10.1007/s11606-012-2255-6
8. Johnson T, McNutt R, Odwazny R, Patel D, Baker S. Discrepancy between admission and discharge diagnoses as a predictor of hospital length of stay. J Hosp Med. 2009;4(4):234-239. https://doi.org/10.1002/jhm.453
9. Aplin KS, Coutinho McAllister S, Kupersmith E, Rachoin JS. Caring for patients in a hospitalist-run clinical decision unit is associated with decreased length of stay without increasing revisit rates. J Hosp Med. 2014;9(6):391-395. https://doi.org/10.1002/jhm.2188
10. Selker HP, Beshansky JR, Pauker SG, Kassirer JP. The epidemiology of delays in a teaching hospital. The development and use of a tool that detects unnecessary hospital days. Med Care. 1989;27(2):112-129. https://doi.org/10.1097/00005650-198902000-00003
11. Carey MR, Sheth H, Braithwaite RS. A prospective study of reasons for prolonged hospitalizations on a general medicine teaching service. J Gen Intern Med. 2005;20(2):108-115. https://doi.org/10.1111/j.1525-1497.2005.40269.x
12. Rohatgi N, Loftus P, Grujic O, Cullen M, Hopkins J, Ahuja N. Surgical comanagement by hospitalists improves patient outcomes: a propensity score analysis. Ann Surg. 2016;264(2):275-282. https://doi.org/10.1097/SLA.0000000000001629
13. Chen LM, Freitag MH, Franco M, Sullivan CD, Dickson C, Brancati FL. Natural history of late discharges from a general medical ward. J Hosp Med. 2009;4(4):226-233. https://doi.org/10.1002/jhm.413
14. Zoucha J, Hull M, Keniston A, et al. Barriers to early hospital discharge: a cross-sectional study at five academic hospitals. J Hosp Med. 2018;13(12):816-822. https://doi.org/10.12788/jhm.3074
15. Thompson RE, Pfeifer K, Grant PJ, et al. Hospital medicine and perioperative care: a framework for high-quality, high-value collaborative care. J Hosp Med. 2017;12(4):277-282. https://doi.org/10.12788/jhm.2717
16. Fail RE, Meier DE. Improving quality of care for seriously ill patients: opportunities for hospitalists. J Hosp Med. 2018;13(3):194-197. https://doi.org/10.12788/jhm.2896
17. Hoyer EH, Friedman M, Lavezza A, et al. Promoting mobility and reducing length of stay in hospitalized general medicine patients: a quality-improvement project. J Hosp Med. 2016;11(5):341-347. https://doi.org/10.1002/jhm.2546
18. Davis C, Rhodes DJ. The impact of DRGs on the cost and quality of health care in the United States. Health Policy. 1988;9(2):117-131. https://doi.org/10.1016/0168-8510(88)90029-2
19. Rothberg M, Lee N. Reducing readmissions or length of stay-Which is more important? J Hosp Med. 2017;12(8):685-686. https://doi.org/10.12788/jhm.2790
20. Kaboli PJ, Go JT, Hockenberry J, et al. Associations between reduced hospital length of stay and 30-day readmission rate and mortality: 14-year experience in 129 Veterans Affairs hospitals. Ann Intern Med. 2012;157(12):837-845. https://doi.org/10.7326/0003-4819-157-12-201212180-00003
21. Rinne ST, Graves MC, Bastian LA, et al. Association between length of stay and readmission for COPD. Am J Manag Care. 2017;23(8):e253-e258.
22. Sud M, Yu B, Wijeysundera HC, et al. Associations between short or long length of stay and 30-day readmission and mortality in hospitalized patients with heart failure. JACC Heart Fail. 2017;5(8):578-588. https://doi.org/10.1016/j.jchf.2017.03.012
23. Rothman RD, Whinney CM, Pappas MA, Zoller DM, Rosencrance JG, Peter DJ. The relationship between the follow-up to discharge ratio and length of stay. Am J Manag Care. 2020;26(9):396-399. https://doi.org/10.37765/ajmc.2020.88490
24. Epstein K, Juarez E, Epstein A, Loya K, Singer A. The impact of fragmentation of hospitalist care on length of stay. J Hosp Med. 2010;5(6):335-338. https://doi.org/10.1002/jhm.675
Healthcare payment model reform has increased pressure on healthcare systems and hospitalists to improve efficiency and reduce the cost of care. These pressures on the healthcare system have been exacerbated by a global pandemic and an aging patient population straining hospital capacity and resources. Hospital capacity constraints may contribute to hospital crowding and can compromise patient outcomes.1 Increasing hospital capacity also contributes to an increase in hospitalist census. This increase in census is accompanied by proportional increases in hospitalist burnout, cost of care, and prolonged length of stay (LOS).2 Managing LOS reduces “waste” (or non–value-added inpatient days) and can improve outcomes and efficiency within the hospital system.
The benefits for LOS reduction when patients are managed by hospitalists compared with primary care practitioners are well described and are associated with decreases in average LOS and cost.3-5 The shorter LOS with hospitalist care is most pronounced in older patients with more complex disease processes, which has temporal importance. The Department of Health and Human Services expects the number of American adults aged >65 years to approach 72 million (20% of the US population) by 2030. Hospitalists are positioned to drive evidence-based care pathways and improve the quality of patient care in this growing patient population. We examine the reasons for managing LOS, summarize factors that contribute to an increased LOS (“waste”), and propose a list of evidence-based value drivers for LOS reduction (Table).2,6-17 Our experience utilizing this approach within Cleveland Clinic Florida following implementation of many of these evidence-based strategies to reduce non–value-added hospital days is also described in the Appendix Figure.
WHY MANAGE LOS?
Barriers to sustainable LOS-reduction strategies have evolved, in part, since the introduction of the Medicare Prospective Payment System, which moved hospital Medicare payments to a predetermined fixed rate for each diagnosis-related group. This led to financial pressures on healthcare systems to identify methods to reduce cost and, in turn, contributed to an increase in postacute facility utilization, with alternative payment models developing in parallel.18,19 These changes along with disaggregated payments between hospitals and postacute facilities have created a formidable challenge to LOS and cost-reduction plans.19
The usual “why” for reducing LOS includes improving constraints on hospital capacity, strains on resources, and deleterious outcomes. In our experience, an evidence-based approach to LOS management should focus on: (1) reduction in patient hospital days through decreased care variation; (2) stabilizing hospitalist workloads; (3) minimizing the fragmentation inherent to the hospitalist care delivery model; and (4) developing service lines to manage patients hospitalized in an observation status and for those patients undergoing procedures deemed medically complex. The literature is mixed on the impact of LOS reductions on other clinical end points, such as readmissions or mortality, with the preponderance indicating no deleterious impact.20-22 Managing LOS using an evidence-based approach that addresses the variability of individual patients is essential to the LOS strategies employed. These strategies should focus on process improvements to drive LOS reduction and utilize metrics under the individual hospitalist control to support their contribution to the hospitalist groups’ overall LOS.23
IMPROVING HOSPITALIST VALUE AROUND LOS MANAGEMENT
Intrinsic factors such as hospitalist staffing fragmentation, high rounding census, failing to prioritize patients ready to be discharged, variability in practice, number of consultants per patient, and hospitalist behaviors contribute to increased LOS.2,6,8 A first precept to management of LOS at the group level is to recognize all hospitalist services are not created equal, and “lumping” hospitalists into a single efficiency metric would not yield actionable information.
The literature is rife with examples of the significant variation in practice styles among hospitalists. A large study including more than 1000 hospitalists identified practice variation as the strongest predictor of variations in mean LOS.7 While Goodwin et al7 identified significant variation among hospitalists’ LOS and the discharge destination of patients, much of the variation could be attributable to the hospitals where they practice. These findings ostensibly highlight the importance of LOS strategies being developed collaboratively among hospitalist groups and the healthcare systems they serve. Similar variation exists among hospitalists on teaching services versus nonteaching services. Our experience parallels that of other studies with regard to teaching services that have found that hospitalists on teaching services often have additional responsibilities and are less able to gain the efficiency of nonresident hospitalists services.3 The impact of teaching services on hospitalist efficiencies is an important component when setting expectations at the hospitalist group level for providers on academic services.
Workload and staffing models for hospitalists have a significant impact on hospitalist efficiency and LOS management. As workload increased, Elliot and colleagues2 identified a proportional increase in LOS. For occupancies of 75% to 85%, LOS increased exponentially above a daily relative value unit of approximately 25 and a census value of approximately 15. The magnitude of this difference in LOS and cost across the range of hospitalist workloads was $262, with an average increase in LOS of 2 days for every unit increase in census. Higher workloads contributed to inferior discussion of treatment options with patients; delays in discharges; delays in placing discharge orders; and unnecessary testing, procedures, and consults.14 To mitigate inefficiency and adverse impacts of higher workloads, hospitalist groups should develop mechanisms to absorb surges in census and unanticipated changes to staffing maintaining the workload within a range appropriate to the patient population.
Decreasing fragmentation, when multiple hospitalists care for the patient during hospitalization, is a necessary component of any LOS-reduction strategy. Studies of pneumonia and heart failure have demonstrated that a 10% increase in hosptialist fragmentation is associated with significant increases in LOS.24 Schedules with hospitalists on 7-day rotating rounding blocks have the intuitive advantage of improving care continuity for patients compared with schedules with a shorter number of consecutive rounding days, resulting in fewer hospitalists caring for each patient and decreased “fragmentation.” Additional value drivers for LOS reduction strategies for hospitalists are listed in the Table.
The 2018 State of Hospital Medicine Report highlighted that, among patients discharged by hospitalist groups, 80.8% were inpatient and 19.2% were outpatient. With nearly one in five patients discharged in observation status, it behooves hospitalist programs to work to effectively manage these patients. Indeed, hospitalist-run observation units have been shown to decrease LOS significantly without an increase in return rates to the emergency department or hospital compared with patients managed prior to the introduction of a dedicated observation unit.9
Although an in-depth discussion is beyond the scope of the present article, it is worth noting the value of hospitalist comanagement (HCoM) strategies. The impact of HCoM teams is demonstrated by reductions in LOS and cost of care resulting from decreases in medical complications, number of consultants per patient, and a decrease in 30-day readmsissions.12 The Society of Hospital Medicine Perioperative Care Work Group has outlined a collaborative framework for hospitalists and healthcare systems to draw from.15
THE CLEVELAND CLINIC INDIAN RIVER HOSPITAL EXPERIENCE
Within the Cleveland Clinic Indian River Hospital (CCIRH) medicine department, many of the aforementioned strategies and tactics were standardized among hospitalist providers. Hospitalists at CCIRH are scheduled on 7-day rotating blocks to reduce fragmentation. In 2019, we targeted a range of 15 to 18 patient contacts per rounding hospitalist per day and utilized a back-up call system to stabilize the hospitalist census. The hospitalist service lines are enhanced through HCoM services with patients cohorted on dedicated HCoM teams. The follow-up to discharge ratio is used to provide feedback at the provider level as both a management and assessment tool.23 The rounding and admitting teams are dedicated to their responsibility (with the occasional exception necessitating the rounding team assist with admissions when the volumes are high). Direct admissions and transfers from outside hospitals are managed by a dedicated hospital medicine “quarterback” to minimize disruption of the admitting and rounding teams. Barriers to discharge are identified at the time of admission by care management and aggressively managed. Prolonged LOS reports are generated daily and disseminated to care managers and physician leadership. In January 2019, the average LOS for inpatients at CCIRH was 4.4 days. In December 2019, the average LOS for the calendar year to-date at CCIRH was 3.9 days (Appendix Figure).
The value proposition for managing LOS should be viewed in the context of the total cost of care over an extended period of time and not viewed in isolation. Readmission rates serve as a counterbalance to LOS-reduction strategies and contribute to higher costs of care when increased. The 30-day readmission rate for this cohort over this same time period was down slightly compared with the previous year to 12.1%. In addition, observation patients at CCIRH are managed in a closed, geographically cohorted unit, staffed by dedicated advanced-practice providers and physicians dedicated to observation medicine. Over this same time period, more than 5500 patients were managed in the observation unit. These patients had an average LOS of 19.2 hours, with approximately four out of every five patients being discharged to home from an observation status.
The impact of COVID-19 and higher hospital volumes are best visualized in the Appendix Figure. Increases in LOS were observed during periods of COVID-19–related “surges” in hospital volume. These reversals in LOS trends during periods of high occupancy echo earlier findings by Elliot et al2 showing that external factors that are not directly under the control of the hospitalist drive LOS and must be considered when developing LOS reduction strategies.
CONCLUSION
The shift toward value-based payment models provides a strong tailwind for healthcare systems to manage LOS. Hospitalists are well positioned to drive LOS-reduction strategies for the healthcare systems they serve and provide value by driving both quality and efficiency. A complete realization of the value proposition of hospitalist programs in driving LOS-reduction initiatives requires the healthcare systems they serve to provide these teams with the appropriate resources and tools.
Healthcare payment model reform has increased pressure on healthcare systems and hospitalists to improve efficiency and reduce the cost of care. These pressures on the healthcare system have been exacerbated by a global pandemic and an aging patient population straining hospital capacity and resources. Hospital capacity constraints may contribute to hospital crowding and can compromise patient outcomes.1 Increasing hospital capacity also contributes to an increase in hospitalist census. This increase in census is accompanied by proportional increases in hospitalist burnout, cost of care, and prolonged length of stay (LOS).2 Managing LOS reduces “waste” (or non–value-added inpatient days) and can improve outcomes and efficiency within the hospital system.
The benefits for LOS reduction when patients are managed by hospitalists compared with primary care practitioners are well described and are associated with decreases in average LOS and cost.3-5 The shorter LOS with hospitalist care is most pronounced in older patients with more complex disease processes, which has temporal importance. The Department of Health and Human Services expects the number of American adults aged >65 years to approach 72 million (20% of the US population) by 2030. Hospitalists are positioned to drive evidence-based care pathways and improve the quality of patient care in this growing patient population. We examine the reasons for managing LOS, summarize factors that contribute to an increased LOS (“waste”), and propose a list of evidence-based value drivers for LOS reduction (Table).2,6-17 Our experience utilizing this approach within Cleveland Clinic Florida following implementation of many of these evidence-based strategies to reduce non–value-added hospital days is also described in the Appendix Figure.
WHY MANAGE LOS?
Barriers to sustainable LOS-reduction strategies have evolved, in part, since the introduction of the Medicare Prospective Payment System, which moved hospital Medicare payments to a predetermined fixed rate for each diagnosis-related group. This led to financial pressures on healthcare systems to identify methods to reduce cost and, in turn, contributed to an increase in postacute facility utilization, with alternative payment models developing in parallel.18,19 These changes along with disaggregated payments between hospitals and postacute facilities have created a formidable challenge to LOS and cost-reduction plans.19
The usual “why” for reducing LOS includes improving constraints on hospital capacity, strains on resources, and deleterious outcomes. In our experience, an evidence-based approach to LOS management should focus on: (1) reduction in patient hospital days through decreased care variation; (2) stabilizing hospitalist workloads; (3) minimizing the fragmentation inherent to the hospitalist care delivery model; and (4) developing service lines to manage patients hospitalized in an observation status and for those patients undergoing procedures deemed medically complex. The literature is mixed on the impact of LOS reductions on other clinical end points, such as readmissions or mortality, with the preponderance indicating no deleterious impact.20-22 Managing LOS using an evidence-based approach that addresses the variability of individual patients is essential to the LOS strategies employed. These strategies should focus on process improvements to drive LOS reduction and utilize metrics under the individual hospitalist control to support their contribution to the hospitalist groups’ overall LOS.23
IMPROVING HOSPITALIST VALUE AROUND LOS MANAGEMENT
Intrinsic factors such as hospitalist staffing fragmentation, high rounding census, failing to prioritize patients ready to be discharged, variability in practice, number of consultants per patient, and hospitalist behaviors contribute to increased LOS.2,6,8 A first precept to management of LOS at the group level is to recognize all hospitalist services are not created equal, and “lumping” hospitalists into a single efficiency metric would not yield actionable information.
The literature is rife with examples of the significant variation in practice styles among hospitalists. A large study including more than 1000 hospitalists identified practice variation as the strongest predictor of variations in mean LOS.7 While Goodwin et al7 identified significant variation among hospitalists’ LOS and the discharge destination of patients, much of the variation could be attributable to the hospitals where they practice. These findings ostensibly highlight the importance of LOS strategies being developed collaboratively among hospitalist groups and the healthcare systems they serve. Similar variation exists among hospitalists on teaching services versus nonteaching services. Our experience parallels that of other studies with regard to teaching services that have found that hospitalists on teaching services often have additional responsibilities and are less able to gain the efficiency of nonresident hospitalists services.3 The impact of teaching services on hospitalist efficiencies is an important component when setting expectations at the hospitalist group level for providers on academic services.
Workload and staffing models for hospitalists have a significant impact on hospitalist efficiency and LOS management. As workload increased, Elliot and colleagues2 identified a proportional increase in LOS. For occupancies of 75% to 85%, LOS increased exponentially above a daily relative value unit of approximately 25 and a census value of approximately 15. The magnitude of this difference in LOS and cost across the range of hospitalist workloads was $262, with an average increase in LOS of 2 days for every unit increase in census. Higher workloads contributed to inferior discussion of treatment options with patients; delays in discharges; delays in placing discharge orders; and unnecessary testing, procedures, and consults.14 To mitigate inefficiency and adverse impacts of higher workloads, hospitalist groups should develop mechanisms to absorb surges in census and unanticipated changes to staffing maintaining the workload within a range appropriate to the patient population.
Decreasing fragmentation, when multiple hospitalists care for the patient during hospitalization, is a necessary component of any LOS-reduction strategy. Studies of pneumonia and heart failure have demonstrated that a 10% increase in hosptialist fragmentation is associated with significant increases in LOS.24 Schedules with hospitalists on 7-day rotating rounding blocks have the intuitive advantage of improving care continuity for patients compared with schedules with a shorter number of consecutive rounding days, resulting in fewer hospitalists caring for each patient and decreased “fragmentation.” Additional value drivers for LOS reduction strategies for hospitalists are listed in the Table.
The 2018 State of Hospital Medicine Report highlighted that, among patients discharged by hospitalist groups, 80.8% were inpatient and 19.2% were outpatient. With nearly one in five patients discharged in observation status, it behooves hospitalist programs to work to effectively manage these patients. Indeed, hospitalist-run observation units have been shown to decrease LOS significantly without an increase in return rates to the emergency department or hospital compared with patients managed prior to the introduction of a dedicated observation unit.9
Although an in-depth discussion is beyond the scope of the present article, it is worth noting the value of hospitalist comanagement (HCoM) strategies. The impact of HCoM teams is demonstrated by reductions in LOS and cost of care resulting from decreases in medical complications, number of consultants per patient, and a decrease in 30-day readmsissions.12 The Society of Hospital Medicine Perioperative Care Work Group has outlined a collaborative framework for hospitalists and healthcare systems to draw from.15
THE CLEVELAND CLINIC INDIAN RIVER HOSPITAL EXPERIENCE
Within the Cleveland Clinic Indian River Hospital (CCIRH) medicine department, many of the aforementioned strategies and tactics were standardized among hospitalist providers. Hospitalists at CCIRH are scheduled on 7-day rotating blocks to reduce fragmentation. In 2019, we targeted a range of 15 to 18 patient contacts per rounding hospitalist per day and utilized a back-up call system to stabilize the hospitalist census. The hospitalist service lines are enhanced through HCoM services with patients cohorted on dedicated HCoM teams. The follow-up to discharge ratio is used to provide feedback at the provider level as both a management and assessment tool.23 The rounding and admitting teams are dedicated to their responsibility (with the occasional exception necessitating the rounding team assist with admissions when the volumes are high). Direct admissions and transfers from outside hospitals are managed by a dedicated hospital medicine “quarterback” to minimize disruption of the admitting and rounding teams. Barriers to discharge are identified at the time of admission by care management and aggressively managed. Prolonged LOS reports are generated daily and disseminated to care managers and physician leadership. In January 2019, the average LOS for inpatients at CCIRH was 4.4 days. In December 2019, the average LOS for the calendar year to-date at CCIRH was 3.9 days (Appendix Figure).
The value proposition for managing LOS should be viewed in the context of the total cost of care over an extended period of time and not viewed in isolation. Readmission rates serve as a counterbalance to LOS-reduction strategies and contribute to higher costs of care when increased. The 30-day readmission rate for this cohort over this same time period was down slightly compared with the previous year to 12.1%. In addition, observation patients at CCIRH are managed in a closed, geographically cohorted unit, staffed by dedicated advanced-practice providers and physicians dedicated to observation medicine. Over this same time period, more than 5500 patients were managed in the observation unit. These patients had an average LOS of 19.2 hours, with approximately four out of every five patients being discharged to home from an observation status.
The impact of COVID-19 and higher hospital volumes are best visualized in the Appendix Figure. Increases in LOS were observed during periods of COVID-19–related “surges” in hospital volume. These reversals in LOS trends during periods of high occupancy echo earlier findings by Elliot et al2 showing that external factors that are not directly under the control of the hospitalist drive LOS and must be considered when developing LOS reduction strategies.
CONCLUSION
The shift toward value-based payment models provides a strong tailwind for healthcare systems to manage LOS. Hospitalists are well positioned to drive LOS-reduction strategies for the healthcare systems they serve and provide value by driving both quality and efficiency. A complete realization of the value proposition of hospitalist programs in driving LOS-reduction initiatives requires the healthcare systems they serve to provide these teams with the appropriate resources and tools.
1. Eriksson CO, Stoner RC, Eden KB, Newgard CD, Guise J-M. The association between hospital capacity strain and inpatient outcomes in highly developed countries: a systematic review. J Gen Intern Med. 2017;32(6):686-696. https://doi.org/10.1007/s11606-016-3936-3
2. Elliott DJ, Young RS, Brice J, Aguiar R, Kolm P. Effect of hospitalist workload on the quality and efficiency of care. JAMA Intern Med. 2014;174(5):786-793. https://doi.org/10.1001/jamainternmed.2014.300
3. Rachoin JS, Skaf J, Cerceo E, et al. The impact of hospitalists on length of stay and costs: systematic review and meta-analysis. Am J Manag Care. 2012;18(1):e23-30.
4. Kuo YF, Goodwin JS. Effect of hospitalists on length of stay in the medicare population: variation according to hospital and patient characteristics. J Am Geriatr Soc. 2010;58(9):1649-1657. https://doi.org/10.1111/j.1532-5415.2010.03007.x
5. Lindenauer PK, Rothberg MB, Pekow PS, Kenwood C, Benjamin EM, Auerbach AD. Outcomes of care by hospitalists, general internists, and family physicians. N Engl J Med. 2007;357(25):2589-2600. https://doi.org/10.1056/NEJMsa067735
6. Epstein K, Juarez E, Epstein A, Loya K, Singer A. The impact of fragmentation of hospitalist care on length of stay. J Hosp Med. 2010;5(6):335-338. https://doi.org/10.1002/jhm.675
7. Goodwin JS, Lin Y-L, Singh S, Kuo Y-F. Variation in length of stay and outcomes among hospitalized patients attributable to hospitals and hospitalists. J Gen Intern Med. 2013;28(3):370-376. https://doi.org/10.1007/s11606-012-2255-6
8. Johnson T, McNutt R, Odwazny R, Patel D, Baker S. Discrepancy between admission and discharge diagnoses as a predictor of hospital length of stay. J Hosp Med. 2009;4(4):234-239. https://doi.org/10.1002/jhm.453
9. Aplin KS, Coutinho McAllister S, Kupersmith E, Rachoin JS. Caring for patients in a hospitalist-run clinical decision unit is associated with decreased length of stay without increasing revisit rates. J Hosp Med. 2014;9(6):391-395. https://doi.org/10.1002/jhm.2188
10. Selker HP, Beshansky JR, Pauker SG, Kassirer JP. The epidemiology of delays in a teaching hospital. The development and use of a tool that detects unnecessary hospital days. Med Care. 1989;27(2):112-129. https://doi.org/10.1097/00005650-198902000-00003
11. Carey MR, Sheth H, Braithwaite RS. A prospective study of reasons for prolonged hospitalizations on a general medicine teaching service. J Gen Intern Med. 2005;20(2):108-115. https://doi.org/10.1111/j.1525-1497.2005.40269.x
12. Rohatgi N, Loftus P, Grujic O, Cullen M, Hopkins J, Ahuja N. Surgical comanagement by hospitalists improves patient outcomes: a propensity score analysis. Ann Surg. 2016;264(2):275-282. https://doi.org/10.1097/SLA.0000000000001629
13. Chen LM, Freitag MH, Franco M, Sullivan CD, Dickson C, Brancati FL. Natural history of late discharges from a general medical ward. J Hosp Med. 2009;4(4):226-233. https://doi.org/10.1002/jhm.413
14. Zoucha J, Hull M, Keniston A, et al. Barriers to early hospital discharge: a cross-sectional study at five academic hospitals. J Hosp Med. 2018;13(12):816-822. https://doi.org/10.12788/jhm.3074
15. Thompson RE, Pfeifer K, Grant PJ, et al. Hospital medicine and perioperative care: a framework for high-quality, high-value collaborative care. J Hosp Med. 2017;12(4):277-282. https://doi.org/10.12788/jhm.2717
16. Fail RE, Meier DE. Improving quality of care for seriously ill patients: opportunities for hospitalists. J Hosp Med. 2018;13(3):194-197. https://doi.org/10.12788/jhm.2896
17. Hoyer EH, Friedman M, Lavezza A, et al. Promoting mobility and reducing length of stay in hospitalized general medicine patients: a quality-improvement project. J Hosp Med. 2016;11(5):341-347. https://doi.org/10.1002/jhm.2546
18. Davis C, Rhodes DJ. The impact of DRGs on the cost and quality of health care in the United States. Health Policy. 1988;9(2):117-131. https://doi.org/10.1016/0168-8510(88)90029-2
19. Rothberg M, Lee N. Reducing readmissions or length of stay-Which is more important? J Hosp Med. 2017;12(8):685-686. https://doi.org/10.12788/jhm.2790
20. Kaboli PJ, Go JT, Hockenberry J, et al. Associations between reduced hospital length of stay and 30-day readmission rate and mortality: 14-year experience in 129 Veterans Affairs hospitals. Ann Intern Med. 2012;157(12):837-845. https://doi.org/10.7326/0003-4819-157-12-201212180-00003
21. Rinne ST, Graves MC, Bastian LA, et al. Association between length of stay and readmission for COPD. Am J Manag Care. 2017;23(8):e253-e258.
22. Sud M, Yu B, Wijeysundera HC, et al. Associations between short or long length of stay and 30-day readmission and mortality in hospitalized patients with heart failure. JACC Heart Fail. 2017;5(8):578-588. https://doi.org/10.1016/j.jchf.2017.03.012
23. Rothman RD, Whinney CM, Pappas MA, Zoller DM, Rosencrance JG, Peter DJ. The relationship between the follow-up to discharge ratio and length of stay. Am J Manag Care. 2020;26(9):396-399. https://doi.org/10.37765/ajmc.2020.88490
24. Epstein K, Juarez E, Epstein A, Loya K, Singer A. The impact of fragmentation of hospitalist care on length of stay. J Hosp Med. 2010;5(6):335-338. https://doi.org/10.1002/jhm.675
1. Eriksson CO, Stoner RC, Eden KB, Newgard CD, Guise J-M. The association between hospital capacity strain and inpatient outcomes in highly developed countries: a systematic review. J Gen Intern Med. 2017;32(6):686-696. https://doi.org/10.1007/s11606-016-3936-3
2. Elliott DJ, Young RS, Brice J, Aguiar R, Kolm P. Effect of hospitalist workload on the quality and efficiency of care. JAMA Intern Med. 2014;174(5):786-793. https://doi.org/10.1001/jamainternmed.2014.300
3. Rachoin JS, Skaf J, Cerceo E, et al. The impact of hospitalists on length of stay and costs: systematic review and meta-analysis. Am J Manag Care. 2012;18(1):e23-30.
4. Kuo YF, Goodwin JS. Effect of hospitalists on length of stay in the medicare population: variation according to hospital and patient characteristics. J Am Geriatr Soc. 2010;58(9):1649-1657. https://doi.org/10.1111/j.1532-5415.2010.03007.x
5. Lindenauer PK, Rothberg MB, Pekow PS, Kenwood C, Benjamin EM, Auerbach AD. Outcomes of care by hospitalists, general internists, and family physicians. N Engl J Med. 2007;357(25):2589-2600. https://doi.org/10.1056/NEJMsa067735
6. Epstein K, Juarez E, Epstein A, Loya K, Singer A. The impact of fragmentation of hospitalist care on length of stay. J Hosp Med. 2010;5(6):335-338. https://doi.org/10.1002/jhm.675
7. Goodwin JS, Lin Y-L, Singh S, Kuo Y-F. Variation in length of stay and outcomes among hospitalized patients attributable to hospitals and hospitalists. J Gen Intern Med. 2013;28(3):370-376. https://doi.org/10.1007/s11606-012-2255-6
8. Johnson T, McNutt R, Odwazny R, Patel D, Baker S. Discrepancy between admission and discharge diagnoses as a predictor of hospital length of stay. J Hosp Med. 2009;4(4):234-239. https://doi.org/10.1002/jhm.453
9. Aplin KS, Coutinho McAllister S, Kupersmith E, Rachoin JS. Caring for patients in a hospitalist-run clinical decision unit is associated with decreased length of stay without increasing revisit rates. J Hosp Med. 2014;9(6):391-395. https://doi.org/10.1002/jhm.2188
10. Selker HP, Beshansky JR, Pauker SG, Kassirer JP. The epidemiology of delays in a teaching hospital. The development and use of a tool that detects unnecessary hospital days. Med Care. 1989;27(2):112-129. https://doi.org/10.1097/00005650-198902000-00003
11. Carey MR, Sheth H, Braithwaite RS. A prospective study of reasons for prolonged hospitalizations on a general medicine teaching service. J Gen Intern Med. 2005;20(2):108-115. https://doi.org/10.1111/j.1525-1497.2005.40269.x
12. Rohatgi N, Loftus P, Grujic O, Cullen M, Hopkins J, Ahuja N. Surgical comanagement by hospitalists improves patient outcomes: a propensity score analysis. Ann Surg. 2016;264(2):275-282. https://doi.org/10.1097/SLA.0000000000001629
13. Chen LM, Freitag MH, Franco M, Sullivan CD, Dickson C, Brancati FL. Natural history of late discharges from a general medical ward. J Hosp Med. 2009;4(4):226-233. https://doi.org/10.1002/jhm.413
14. Zoucha J, Hull M, Keniston A, et al. Barriers to early hospital discharge: a cross-sectional study at five academic hospitals. J Hosp Med. 2018;13(12):816-822. https://doi.org/10.12788/jhm.3074
15. Thompson RE, Pfeifer K, Grant PJ, et al. Hospital medicine and perioperative care: a framework for high-quality, high-value collaborative care. J Hosp Med. 2017;12(4):277-282. https://doi.org/10.12788/jhm.2717
16. Fail RE, Meier DE. Improving quality of care for seriously ill patients: opportunities for hospitalists. J Hosp Med. 2018;13(3):194-197. https://doi.org/10.12788/jhm.2896
17. Hoyer EH, Friedman M, Lavezza A, et al. Promoting mobility and reducing length of stay in hospitalized general medicine patients: a quality-improvement project. J Hosp Med. 2016;11(5):341-347. https://doi.org/10.1002/jhm.2546
18. Davis C, Rhodes DJ. The impact of DRGs on the cost and quality of health care in the United States. Health Policy. 1988;9(2):117-131. https://doi.org/10.1016/0168-8510(88)90029-2
19. Rothberg M, Lee N. Reducing readmissions or length of stay-Which is more important? J Hosp Med. 2017;12(8):685-686. https://doi.org/10.12788/jhm.2790
20. Kaboli PJ, Go JT, Hockenberry J, et al. Associations between reduced hospital length of stay and 30-day readmission rate and mortality: 14-year experience in 129 Veterans Affairs hospitals. Ann Intern Med. 2012;157(12):837-845. https://doi.org/10.7326/0003-4819-157-12-201212180-00003
21. Rinne ST, Graves MC, Bastian LA, et al. Association between length of stay and readmission for COPD. Am J Manag Care. 2017;23(8):e253-e258.
22. Sud M, Yu B, Wijeysundera HC, et al. Associations between short or long length of stay and 30-day readmission and mortality in hospitalized patients with heart failure. JACC Heart Fail. 2017;5(8):578-588. https://doi.org/10.1016/j.jchf.2017.03.012
23. Rothman RD, Whinney CM, Pappas MA, Zoller DM, Rosencrance JG, Peter DJ. The relationship between the follow-up to discharge ratio and length of stay. Am J Manag Care. 2020;26(9):396-399. https://doi.org/10.37765/ajmc.2020.88490
24. Epstein K, Juarez E, Epstein A, Loya K, Singer A. The impact of fragmentation of hospitalist care on length of stay. J Hosp Med. 2010;5(6):335-338. https://doi.org/10.1002/jhm.675
© 2021 Society of Hospital Medicine
Factors Associated With COVID-19 Disease Severity in US Children and Adolescents
The COVID-19 pandemic has led to more than 40 million infections and more than 650,000 deaths in the United States alone.1 Morbidity and mortality have disproportionately affected older adults.2-4 However, acute infection and delayed effects, such as multisystem inflammatory syndrome in children (MIS-C), occur and can lead to severe complications, hospitalization, and death in pediatric patients.5,6 Due to higher clinical disease prevalence and morbidity in the adult population, we have learned much about the clinical factors associated with severe adult COVID-19 disease.5,7-9 Such clinical factors include older age, concurrent comorbidities, smoke exposure, and Black race or Hispanic ethnicity, among others.5,7-10 However, there is a paucity of data on severe COVID-19 disease in pediatric patients.5,11,12 In addition, most immunization strategies and pharmacologic treatments for COVID-19 have not been evaluated or approved for use in children.13 To guide targeted prevention and treatment strategies, there is a critical need to identify children and adolescents—who are among the most vulnerable patient populations—at high risk for severe disease.
Identifying the clinical factors associated with severe COVID-19 disease will help with prioritizing and allocating vaccines when they are approved for use in patients younger than 12 years.
METHODS
Study Design
We conducted a multicenter retrospective cohort study of patients presenting for care at pediatric hospitals that report data to the Pediatric Health Information System (PHIS) database. The PHIS administrative database includes billing and utilization data from 45 US tertiary care hospitals affiliated with the Children’s Hospital Association (Lenexa, Kansas). Data quality and reliability are ensured through a joint validation effort between the Children’s Hospital Association and participating hospitals. Hospitals submit discharge data, including demographics, diagnoses, and procedures using International Classification of Diseases, 10th Revision (ICD-10) codes, along with daily detailed information on pharmacy, location of care, and other services.
Study Population
Patients 30 days to 18 years of age discharged from the emergency department (ED) or inpatient setting with a primary diagnosis of COVID-19 (ICD-10 codes U.071 and U.072) between April 1, 2020, and September 30, 2020, were eligible for inclusion.14 In a prior study, the positive predictive value of an ICD-10–coded diagnosis of COVID-19 among hospitalized pediatric patients was 95.5%, compared with reverse transcription polymerase reaction results or presence of MIS-C.15 The diagnostic code for COVID-19 (ICD-10-CM) also had a high sensitivity (98.0%) in the hospitalized population.16 Acknowledging the increasing practice of screening patients upon admission, and in an attempt to minimize potential misclassification, we did not include encounters with secondary diagnoses of COVID-19 in our primary analyses. Pediatric patients with surgical diagnoses and neonates who never left the hospital were also excluded.
Factors Associated With Severe COVID-19 Disease
Exposures of interest were determined a priori based on current evidence in the literature and included patient age (0-4 years, 5-11 years, and 12-18 years), sex, race and ethnicity (non-Hispanic White, non-Hispanic Black, Hispanic, Asian, other non-White race [defined as Pacific Islander, Native American, or other]), payor type, cardiovascular complex chronic conditions (CCC), neuromuscular CCC, obesity/type 2 diabetes mellitus (DM), pulmonary CCC, asthma (defined using ICD-10 codes17), and immunocompromised CCC
Pediatric Complications and Conditions Associated With COVID-19
Based on current evidence and expert opinion of study members, associated diagnoses and complications co-occurring with a COVID-19 diagnosis were defined a priori and identified through ICD-10 codes (Appendix Table 1). These included acute kidney injury, acute liver injury, aseptic meningitis, asthma exacerbation, bronchiolitis, cerebral infarction, croup, encephalitis, encephalopathy, infant fever, febrile seizure, gastroenteritis/dehydration, Kawasaki disease/MIS-C, myocarditis/pericarditis, pneumonia, lung effusion or empyema, respiratory failure, sepsis, nonfebrile seizure, pancreatitis, sickle cell complications, and thrombotic complications.
Outcomes
COVID-19 severity outcomes were assessed as follows: (1) mild = ED discharge; (2) moderate = inpatient admission; (3) severe = intensive care unit (ICU) admission without mechanical ventilation, shock, or death; and (4) very severe = ICU admission with mechanical ventilation, shock, or death.19 This ordinal ranking system did not violate the proportional odds assumption. Potential reasons for admission to the ICU without mechanical ventilation, shock, or death include, but are not limited to, need for noninvasive ventilation, vital sign instability, dysrhythmias, respiratory insufficiency, or complications arising from concurrent conditions (eg, thrombotic events, need for continuous albuterol therapy). We examined several secondary, hospital-based outcomes, including associated diagnoses and complications, all-cause 30-day healthcare reutilization (ED visit or rehospitalization), length of stay (LOS), and ICU LOS.
Statistical Analysis
Demographic characteristics were summarized using frequencies and percentages for categorical variables and geometric means with SD and medians with interquartile ranges (IQR) for continuous variables, as appropriate. Factors associated with hospitalization (encompassing severity levels 2-4) vs ED discharge (severity level 1) were assessed using logistic regression. Factors associated with increasing severity among hospitalized pediatric patients (severity levels 2, 3, and 4) were assessed using ordinal logistic regression. Covariates in these analyses included race and ethnicity, age, sex, payor, cardiovascular CCC, neurologic/neuromuscular CCC, obesity/type 2 DM, pulmonary CCC, asthma, and immunocompromised CCC. Adjusted odds ratios (aOR) and corresponding 95% CI for each risk factor were generated using generalized linear mixed effects models and random intercepts for each hospital. Given the potential for diagnostic misclassification of pediatric patients with COVID-19 based on primary vs secondary diagnoses, we performed sensitivity analyses defining the study population as those with a primary diagnosis of COVID-19 and those with a secondary diagnosis of COVID-19 plus a concurrent primary diagnosis of a condition associated with COVID-19 (Appendix Table 1).
All analyses were performed using SAS version 9.4 (SAS Institute, Inc), and P < .05 was considered statistically significant. The Institutional Review Board at Vanderbilt University Medical Center determined that this study of de-identified data did not meet the criteria for human subjects research.
RESULTS
Study Population
A total of 19,976 encounters were included in the study. Of those, 15,913 (79.7%) were discharged from the ED and 4063 (20.3%) were hospitalized (Table 1). The most common race/ethnicity was Hispanic (9741, 48.8%), followed by non-Hispanic White (4217, 21.1%). Reference race/ethnicity data for the overall 2019 PHIS population can be found in Appendix Table 2.
The severity distribution among the hospitalized population was moderate (3222, 79.3%), severe (431, 11.3%), and very severe (380, 9.4%). The frequency of COVID-19 diagnoses increased late in the study period (Figure). Among those hospitalized, the median LOS for the index admission was 2 days (IQR, 1-4), while among those admitted to the ICU, the median LOS was 3 days (IQR, 2-5).
Overall, 10.1% (n = 2020) of the study population had an all-cause repeat encounter (ie, subsequent ED encounter or hospitalization) within 30 days following the index discharge. Repeat encounters were more frequent among patients hospitalized than among those discharged from the ED (Appendix Table 3).
Prevalence of Conditions and Complications Associated With COVID-19
Overall, 3257 (16.3%) patients had one or more co-occurring diagnoses categorized as a COVID-19–associated condition or complication. The most frequent diagnoses included lower respiratory tract disease (pneumonia, lung effusion, or empyema; n = 1415, 7.1%), gastroenteritis/dehydration (n = 1068, 5.3%), respiratory failure (n = 731, 3.7%), febrile infant (n = 413, 2.1%), and nonfebrile seizure (n = 425, 2.1%). Aside from nonfebrile seizure, neurological complications were less frequent and included febrile seizure (n = 155, 0.8%), encephalopathy (n = 63, 0.3%), aseptic meningitis (n = 16, 0.1%), encephalitis (n = 11, 0.1%), and cerebral infarction (n = 6, <0.1%). Kawasaki disease and MIS-C comprised 1.7% (n = 346) of diagnoses. Thrombotic complications occurred in 0.1% (n = 13) of patients. Overall, these conditions and complications associated with COVID-19 were more frequent in hospitalized patients than in those discharged from the ED (P < .001) (Table 2).
Factors Associated With COVID-19 Disease Severity
Compared to pediatric patients with COVID-19 discharged from the ED, factors associated with increased odds of hospitalization included private payor insurance; obesity/type 2 DM; asthma; and cardiovascular, immunocompromised, neurologic/neuromuscular, and pulmonary CCCs (Table 3). Factors associated with decreased risk of hospitalization included Black race or Hispanic ethnicity compared with White race; female sex; and age 5 to 11 years and age 12 to 17 years (vs age 0-4 years). Among children and adolescents hospitalized with COVID-19, factors associated with greater disease severity included Black or other non-White race; age 5 to 11 years; age 12 to 17 years; obesity/type 2 DM; immunocompromised conditions; and cardiovascular, neurologic/neuromuscular, and pulmonary CCCs (Table 3).
Sensitivity Analysis
We performed a sensitivity analysis that expanded the study population to include those with a secondary diagnosis of COVID-19 plus a diagnosis of a COVID-19–associated condition or complication. Analyses using the expanded population (N = 21,247) were similar to the primary analyses (Appendix Table 4 and Appendix Table 5).
DISCUSSION
In this large multicenter study evaluating COVID-19 disease severity in more than 19,000 patients presenting for emergency care at US pediatric hospitals, approximately 20% were hospitalized, and among those hospitalized almost a quarter required ICU care. Clinical risk factors associated with increased risk of hospitalization include private payor status and selected comorbidities (obesity/type 2 DM; asthma; and cardiovascular, pulmonary, immunocompromised, neurologic/neuromuscular CCCs), while those associated with decreased risk of hospitalization include older age, female sex, and Black race or Hispanic ethnicity. Factors associated with severe disease among hospitalized pediatric patients include Black or other non-White race, school age (≥5 years), and certain chronic conditions (cardiovascular disease, obesity/type 2 DM, neurologic or neuromuscular disease). Sixteen percent of patients had a concurrent diagnosis for a condition or complication associated with COVID-19.
While the study population (ie, children and adolescents presenting to the ED) represents a small fraction of children and adolescents in the community with SARS-CoV-2 infection, the results provide important insight into factors of severe COVID-19 in the pediatric population. A report from France suggested ventilatory or hemodynamic support or death were independently associated with older age (≥10 years), elevated C-reactive protein, and hypoxemia.12 An Italian study found that younger age (0-4 years) was associated with less severe disease, while preexisting conditions were more likely in patients with severe disease.11 A single-center case series of 50 patients (aged ≤21 years) hospitalized at a children’s hospital in New York City found respiratory failure (n = 9) was more common in children older than 1 year, patients with elevated inflammatory markers, and patients with obesity.20
Our study confirms several factors for severe COVID-19 found in these studies, including older age,11,12,20 obesity,20 and preexisting conditions.11 Our findings also expand on these reports, including identification of factors associated with hospitalization. Given the rate of 30-day re-encounters among pediatric patients with COVID-19 (10.1%), identifying risk factors for hospitalization may aid ED providers in determining optimal disposition (eg, home, hospital admission, ICU). We also identified specific comorbidities associated with more severe disease in those hospitalized with COVID-19, such as cardiovascular disease, obesity/type 2 DM, and pulmonary, neurologic, or neuromuscular conditions. We also found that asthma increased the risk for hospitalization but not more severe disease among those hospitalized. This latter finding also aligns with recent single-center studies,21,22 whereas a Turkish study of pediatric patients aged 0 to 18 years found no association between asthma and COVID-19 hospitalizations.23We also examined payor type and racial/ethnic factors in our analysis. In 2019, patients who identified as Black or Hispanic comprised 52.3% of all encounters and 40.7% of hospitalizations recorded in the PHIS database. During the same year, encounters for influenza among Black or Hispanic pediatric patients comprised 58.7% of all influenza diagnoses and 47.0% of pediatric influenza hospitalizations (Appendix Table 2). In this study, patients who identified as Black or Hispanic race represented a disproportionately large share of patients presenting to children’s hospitals (68.5%) and of those hospitalized (60.8%). Hispanic ethnicity, in particular, represented a disproportionate share of patients seeking care for COVID-19 compared to the overall PHIS population (47.7% and 27.1%, respectively). After accounting for other factors, we found Black and other non-White race—but not of Hispanic ethnicity—were independently associated with more disease severity among those hospitalized. This contrasts with findings from a recent adult study by Yehia et al,24 who found (after adjusting for other clinical factors) no significant difference in mortality between Black patients and White patients among adults hospitalized due to COVID-19. It also contrasts with a recent large population-based UK study wherein pediatric patients identifying as Asian, but not Black or mixed race or ethnicity, had an increased risk of hospital admission and admission to the ICU compared to children identifying as White. Children identifying as Black or mixed race had longer hospital admissions.25 However, as the authors of the study note, residual confounders and ascertainment bias due to differences in COVID testing may have influenced these findings.
Our findings of differences in hospitalization and disease severity among those hospitalized by race and ethnicity should be interpreted carefully. These may reflect a constellation of factors that are difficult to measure, including differences in healthcare access, inequalities in care (including hospital admission inequalities), and implicit bias—all of which may reflect structural racism. For example, it is possible that children who identify as Black or Hispanic have different access to care compared to children who identify as White, and this may affect disease severity on presentation.2 Alternatively, it is possible that White pediatric patients are more likely to be hospitalized as compared to non-White pediatric patients with similar illness severity. Our finding that pediatric patients who identify as Hispanic or Black had a lower risk of hospitalization should be also interpreted carefully, as this may reflect higher utilization of the ED for SARS-CoV-2 testing, increased use of nonemergency services among those without access to primary care, or systematic differences in provider decision-making among this segment of the population.2 Further study is needed to determine specific drivers for racial and ethnic differences in healthcare utilization in children and adolescents with COVID-19.26
Complications and co-occurring diagnoses in adults with COVID-19 are well documented.27-30 However, there is little information to date on the co-occurring diagnoses and complications associated with COVID-19 in children and adolescents. We found that complications and co-occurring conditions occurred in 16.3% of the study population, with the most frequent conditions including known complications of viral infections such as pneumonia, respiratory failure, and seizures. Acute kidney and liver injury, as well as thrombotic complications, occurred less commonly than in adults.26-29 Interestingly, neurologic complications were also uncommon compared to adult reports8,31 and less frequent than in other viral illnesses in children and adolescents. For example, neurologic complications occur in approximately 7.5% of children and adolescents hospitalized with influenza.32
Limitations of the present study include the retrospective design, as well as incomplete patient-level clinical data in the PHIS database. The PHIS database only includes children’s hospitals, which may limit the generalizability of findings to community hospitals. We also excluded newborns, and our findings may not be generalizable to this population. We only included children and adolescents with a primary diagnosis of COVID-19, which has the potential for misclassification in cases where COVID-19 was a secondary diagnosis. However, results of our sensitivity analysis, which incorporated secondary diagnoses of COVID-19, were consistent with findings from our main analyses. Our study was designed to examine associations between certain prespecified factors and COVID-19 severity among pediatric patients who visited the ED or were admitted to the hospital during the COVID-19 pandemic. Thus, our findings must be interpreted in light of these considerations and may not be generalizable outside the ED or hospital setting. For example, it could be that some segments of the population utilized ED resources for testing, whereas others avoided the ED and other healthcare settings for fear of exposure to SARS-CoV-2. We also relied on diagnosis codes to identify concurrent diagnoses, as well as mechanical ventilation in our very severe outcome cohort, which resulted in this classification for some of these diagnoses. Despite these limitations, our findings represent an important step in understanding the risk factors associated with severe clinical COVID-19 disease in pediatric patients.
Our findings may inform future research and clinical interventions. Future studies on antiviral therapies and immune modulators targeting SARS-CoV-2 infection in children and adolescents should focus on high-risk populations, such as those identified in the study, as these patients are most likely to benefit from therapeutic interventions. Similarly, vaccine-development efforts may benefit from additional evaluation in high-risk populations, some of which may have altered immune responses. Furthermore, with increasing vaccination among adults and changes in recommendations, societal mitigation efforts (eg, masking, physical distancing) will diminish. Continued vigilance and COVID-19–mitigation efforts among high-risk children, for whom vaccines are not yet available, are critical during this transition.
CONCLUSION
Among children with COVID-19 who received care at children’s hospitals and EDs, 20% were hospitalized, and, of those, 21% were admitted to the ICU. Older children and adolescent patients had a lower risk of hospitalization; however, when hospitalized, they had greater illness severity. Those with selected comorbidities (eg, cardiovascular, obesity/type 2 DM, pulmonary and neurologic or neuromuscular disease) had both increased odds of hospitalization and in-hospital illness severity. While there were observed differences in COVID-19 severity by race and ethnicity, additional research is needed to clarify the drivers of such disparities. These factors should be considered when prioritizing mitigation strategies to prevent infection (eg, remote learning, avoidance of group activities, prioritization of COVID-19 vaccine when approved for children aged <12 years).
1. Centers for Disease Control and Prevention. COVID data tracker. Accessed September 9, 2021. https://covid.cdc.gov/covid-data-tracker/#datatracker-home
2. Levy C, Basmaci R, Bensaid P, et al. Changes in reverse transcription polymerase chain reaction-positive severe acute respiratory syndrome coronavirus 2 rates in adults and children according to the epidemic stages. Pediatr Infect Dis J. 2020;39(11):e369-e372. https://doi.org/10.1097/inf.0000000000002861
3. Gudbjartsson DF, Helgason A, Jonsson H, et al. Spread of SARS-CoV-2 in the Icelandic population. N Engl J Med. 2020;382(24):2302-2315. https://doi.org/10.1056/nejmoa2006100
4. Garg S, Kim L, Whitaker M, et al. Hospitalization rates and characteristics of patients hospitalized with laboratory-confirmed coronavirus disease 2019 - COVID-NET, 14 States, March 1-30, 2020. MMWR Morb Mortal Wkly Rep. 2020;69(15):458-464. https://doi.org/10.15585/mmwr.mm6915e3
5. Castagnoli R, Votto M, Licari A, et al. Severe acute respiratory syndrome coronavirus 2 (SARS-CoV-2) infection in children and adolescents: a systematic review. JAMA Pediatr. 2020;174(9):882-889. https://doi.org/10.1001/jamapediatrics.2020.1467
6. Feldstein LR, Rose EB, Horwitz SM, et al; Overcoming COVID-19 Investigators; CDC COVID-19 Response Team. Multisystem inflammatory syndrome in U.S. children and adolescents. N Engl J Med. 2020;383(4):334-346. https://doi.org/10.1056/nejmoa2021680
7. Magro B, Zuccaro V, Novelli L, et al. Predicting in-hospital mortality from coronavirus disease 2019: a simple validated app for clinical use. PLoS One. 2021;16(1):e0245281. https://doi.org/10.1371/journal.pone.0245281
8. Helms J, Kremer S, Merdji H, et al. Neurologic features in severe SARS-CoV-2 infection. N Engl J Med. 2020;382(23):2268-2270. https://doi.org/10.1056/nejmc2008597
9. Severe Covid GWAS Group; Ellinghaus D, Degenhardt F, Bujanda L, et al. Genomewide association study of severe Covid-19 with respiratory failure. N Engl J Med. 2020;383(16):1522-1534.
10. Kabarriti R, Brodin NP, Maron MI, et al. association of race and ethnicity with comorbidities and survival among patients with COVID-19 at an urban medical center in New York. JAMA Netw Open. 2020;3(9):e2019795. https://doi.org/10.1001/jamanetworkopen.2020.19795
11. Bellino S, Punzo O, Rota MC, et al; COVID-19 Working Group. COVID-19 disease severity risk factors for pediatric patients in Italy. Pediatrics. 2020;146(4):e2020009399. https://doi.org/10.1542/peds.2020-009399
12. Ouldali N, Yang DD, Madhi F, et al; investigator group of the PANDOR study. Factors associated with severe SARS-CoV-2 infection. Pediatrics. 2020;147(3):e2020023432. https://doi.org/10.1542/peds.2020-023432
13. Castells MC, Phillips EJ. Maintaining safety with SARS-CoV-2 vaccines. N Engl J Med. 2021;384(7):643-649. https://doi.org/10.1056/nejmra2035343
14. Antoon JW, Williams DJ, Thurm C, et al. The COVID-19 pandemic and changes in healthcare utilization for pediatric respiratory and nonrespiratory illnesses in the United States. J Hosp Med. 2021;16(5):294-297. https://doi.org/10.12788/jhm.3608
15. Blatz AM, David MZ, Otto WR, Luan X, Gerber JS. Validation of International Classification of Disease-10 code for identifying children hospitalized with coronavirus disease-2019. J Pediatric Infect Dis Soc. 2020;10(4):547-548. https://doi.org/10.1093/jpids/piaa140
16. Kadri SS, Gundrum J, Warner S, et al. Uptake and accuracy of the diagnosis code for COVID-19 among US hospitalizations. JAMA. 2020;324(24):2553-2554. https://doi.org/10.1001/jama.2020.20323
17. Kaiser SV, Rodean J, Bekmezian A, et al; Pediatric Research in Inpatient Settings (PRIS) Network. Effectiveness of pediatric asthma pathways for hospitalized children: a multicenter, national analysis. J Pediatr. 2018;197:165-171.e162. https://doi.org/10.1016/j.jpeds.2018.01.084
18. Feudtner C, Feinstein JA, Zhong W, Hall M, Dai D. Pediatric complex chronic conditions classification system version 2: updated for ICD-10 and complex medical technology dependence and transplantation. BMC Pediatr. 2014;14:199. https://doi.org/10.1186/1471-2431-14-199.
19. Williams DJ, Zhu Y, Grijalva CG, et al. Predicting severe pneumonia outcomes in children. Pediatrics. 2016;138(4):e20161019. https://doi.org/10.1542/peds.2016-1019
20. Zachariah P, Johnson CL, Halabi KC, et al. Epidemiology, clinical features, and disease severity in patients with coronavirus disease 2019 (COVID-19) in a children’s hospital in New York City, New York. JAMA Pediatr. 2020;174(10):e202430. https://doi.org/10.1001/jamapediatrics.2020.2430
21. DeBiasi RL, Song X, Delaney M, et al. Severe coronavirus disease-2019 in children and young adults in the Washington, DC, metropolitan region. J Pediatr. 2020;223:199-203.e191. https://doi.org/10.1016/j.jpeds.2020.05.007
22. Lovinsky-Desir S, Deshpande DR, De A, et al. Asthma among hospitalized patients with COVID-19 and related outcomes. J Allergy Clin Immunol. 2020;146(5):1027-1034.e1024. https://doi.org/10.1016/j.jaci.2020.07.026
23. Beken B, Ozturk GK, Aygun FD, Aydogmus C, Akar HH. Asthma and allergic diseases are not risk factors for hospitalization in children with coronavirus disease 2019. Ann Allergy Asthma Immunol. 2021;126(5):569-575. https://doi.org/10.1016/j.anai.2021.01.018
24. Yehia BR, Winegar A, Fogel R, et al. Association of race with mortality among patients hospitalized with coronavirus disease 2019 (COVID-19) at 92 US hospitals. JAMA Netw Open. 2020;3(8):e2018039. https://doi.org/10.1001/jamanetworkopen.2020.18039
25. Saatci D, Ranger TA, Garriga C, et al. Association between race and COVID-19 outcomes among 2.6 million children in England. JAMA Pediatr. 2021;e211685. https://doi.org/10.1001/jamapediatrics.2021.1685
26. Lopez L, 3rd, Hart LH, 3rd, Katz MH. Racial and ethnic health disparities related to COVID-19. JAMA. 2021;325(8):719-720. https://doi.org/10.1001/jama.2020.26443
27. Altunok ES, Alkan M, Kamat S, et al. Clinical characteristics of adult patients hospitalized with laboratory-confirmed COVID-19 pneumonia. J Infect Chemother. 2020. https://doi.org/10.1016/j.jiac.2020.10.020
28. Ali H, Daoud A, Mohamed MM, et al. Survival rate in acute kidney injury superimposed COVID-19 patients: a systematic review and meta-analysis. Ren Fail. 2020;42(1):393-397. https://doi.org/10.1080/0886022x.2020.1756323
29. Anirvan P, Bharali P, Gogoi M, Thuluvath PJ, Singh SP, Satapathy SK. Liver injury in COVID-19: the hepatic aspect of the respiratory syndrome - what we know so far. World J Hepatol. 2020;12(12):1182-1197. https://doi.org/10.4254/wjh.v12.i12.1182
30. Moschonas IC, Tselepis AD. SARS-CoV-2 infection and thrombotic complications: a narrative review. J Thromb Thrombolysis. 2021;52(1):111-123. https://doi.org/10.1007/s11239-020-02374-3
31. Lee MH, Perl DP, Nair G, et al. Microvascular injury in the brains of patients with Covid-19. N Engl J Med. 2020;384(5):481-483. https://doi.org/10.1056/nejmc2033369
32. Antoon JW, Hall M, Herndon A, et al. Prevalence, risk factors, and outcomes of influenza-associated neurological Complications in Children. J Pediatr. 2021;S0022-3476(21)00657-0. https://doi.org/10.1016/j.jpeds.2021.06.075
The COVID-19 pandemic has led to more than 40 million infections and more than 650,000 deaths in the United States alone.1 Morbidity and mortality have disproportionately affected older adults.2-4 However, acute infection and delayed effects, such as multisystem inflammatory syndrome in children (MIS-C), occur and can lead to severe complications, hospitalization, and death in pediatric patients.5,6 Due to higher clinical disease prevalence and morbidity in the adult population, we have learned much about the clinical factors associated with severe adult COVID-19 disease.5,7-9 Such clinical factors include older age, concurrent comorbidities, smoke exposure, and Black race or Hispanic ethnicity, among others.5,7-10 However, there is a paucity of data on severe COVID-19 disease in pediatric patients.5,11,12 In addition, most immunization strategies and pharmacologic treatments for COVID-19 have not been evaluated or approved for use in children.13 To guide targeted prevention and treatment strategies, there is a critical need to identify children and adolescents—who are among the most vulnerable patient populations—at high risk for severe disease.
Identifying the clinical factors associated with severe COVID-19 disease will help with prioritizing and allocating vaccines when they are approved for use in patients younger than 12 years.
METHODS
Study Design
We conducted a multicenter retrospective cohort study of patients presenting for care at pediatric hospitals that report data to the Pediatric Health Information System (PHIS) database. The PHIS administrative database includes billing and utilization data from 45 US tertiary care hospitals affiliated with the Children’s Hospital Association (Lenexa, Kansas). Data quality and reliability are ensured through a joint validation effort between the Children’s Hospital Association and participating hospitals. Hospitals submit discharge data, including demographics, diagnoses, and procedures using International Classification of Diseases, 10th Revision (ICD-10) codes, along with daily detailed information on pharmacy, location of care, and other services.
Study Population
Patients 30 days to 18 years of age discharged from the emergency department (ED) or inpatient setting with a primary diagnosis of COVID-19 (ICD-10 codes U.071 and U.072) between April 1, 2020, and September 30, 2020, were eligible for inclusion.14 In a prior study, the positive predictive value of an ICD-10–coded diagnosis of COVID-19 among hospitalized pediatric patients was 95.5%, compared with reverse transcription polymerase reaction results or presence of MIS-C.15 The diagnostic code for COVID-19 (ICD-10-CM) also had a high sensitivity (98.0%) in the hospitalized population.16 Acknowledging the increasing practice of screening patients upon admission, and in an attempt to minimize potential misclassification, we did not include encounters with secondary diagnoses of COVID-19 in our primary analyses. Pediatric patients with surgical diagnoses and neonates who never left the hospital were also excluded.
Factors Associated With Severe COVID-19 Disease
Exposures of interest were determined a priori based on current evidence in the literature and included patient age (0-4 years, 5-11 years, and 12-18 years), sex, race and ethnicity (non-Hispanic White, non-Hispanic Black, Hispanic, Asian, other non-White race [defined as Pacific Islander, Native American, or other]), payor type, cardiovascular complex chronic conditions (CCC), neuromuscular CCC, obesity/type 2 diabetes mellitus (DM), pulmonary CCC, asthma (defined using ICD-10 codes17), and immunocompromised CCC
Pediatric Complications and Conditions Associated With COVID-19
Based on current evidence and expert opinion of study members, associated diagnoses and complications co-occurring with a COVID-19 diagnosis were defined a priori and identified through ICD-10 codes (Appendix Table 1). These included acute kidney injury, acute liver injury, aseptic meningitis, asthma exacerbation, bronchiolitis, cerebral infarction, croup, encephalitis, encephalopathy, infant fever, febrile seizure, gastroenteritis/dehydration, Kawasaki disease/MIS-C, myocarditis/pericarditis, pneumonia, lung effusion or empyema, respiratory failure, sepsis, nonfebrile seizure, pancreatitis, sickle cell complications, and thrombotic complications.
Outcomes
COVID-19 severity outcomes were assessed as follows: (1) mild = ED discharge; (2) moderate = inpatient admission; (3) severe = intensive care unit (ICU) admission without mechanical ventilation, shock, or death; and (4) very severe = ICU admission with mechanical ventilation, shock, or death.19 This ordinal ranking system did not violate the proportional odds assumption. Potential reasons for admission to the ICU without mechanical ventilation, shock, or death include, but are not limited to, need for noninvasive ventilation, vital sign instability, dysrhythmias, respiratory insufficiency, or complications arising from concurrent conditions (eg, thrombotic events, need for continuous albuterol therapy). We examined several secondary, hospital-based outcomes, including associated diagnoses and complications, all-cause 30-day healthcare reutilization (ED visit or rehospitalization), length of stay (LOS), and ICU LOS.
Statistical Analysis
Demographic characteristics were summarized using frequencies and percentages for categorical variables and geometric means with SD and medians with interquartile ranges (IQR) for continuous variables, as appropriate. Factors associated with hospitalization (encompassing severity levels 2-4) vs ED discharge (severity level 1) were assessed using logistic regression. Factors associated with increasing severity among hospitalized pediatric patients (severity levels 2, 3, and 4) were assessed using ordinal logistic regression. Covariates in these analyses included race and ethnicity, age, sex, payor, cardiovascular CCC, neurologic/neuromuscular CCC, obesity/type 2 DM, pulmonary CCC, asthma, and immunocompromised CCC. Adjusted odds ratios (aOR) and corresponding 95% CI for each risk factor were generated using generalized linear mixed effects models and random intercepts for each hospital. Given the potential for diagnostic misclassification of pediatric patients with COVID-19 based on primary vs secondary diagnoses, we performed sensitivity analyses defining the study population as those with a primary diagnosis of COVID-19 and those with a secondary diagnosis of COVID-19 plus a concurrent primary diagnosis of a condition associated with COVID-19 (Appendix Table 1).
All analyses were performed using SAS version 9.4 (SAS Institute, Inc), and P < .05 was considered statistically significant. The Institutional Review Board at Vanderbilt University Medical Center determined that this study of de-identified data did not meet the criteria for human subjects research.
RESULTS
Study Population
A total of 19,976 encounters were included in the study. Of those, 15,913 (79.7%) were discharged from the ED and 4063 (20.3%) were hospitalized (Table 1). The most common race/ethnicity was Hispanic (9741, 48.8%), followed by non-Hispanic White (4217, 21.1%). Reference race/ethnicity data for the overall 2019 PHIS population can be found in Appendix Table 2.
The severity distribution among the hospitalized population was moderate (3222, 79.3%), severe (431, 11.3%), and very severe (380, 9.4%). The frequency of COVID-19 diagnoses increased late in the study period (Figure). Among those hospitalized, the median LOS for the index admission was 2 days (IQR, 1-4), while among those admitted to the ICU, the median LOS was 3 days (IQR, 2-5).
Overall, 10.1% (n = 2020) of the study population had an all-cause repeat encounter (ie, subsequent ED encounter or hospitalization) within 30 days following the index discharge. Repeat encounters were more frequent among patients hospitalized than among those discharged from the ED (Appendix Table 3).
Prevalence of Conditions and Complications Associated With COVID-19
Overall, 3257 (16.3%) patients had one or more co-occurring diagnoses categorized as a COVID-19–associated condition or complication. The most frequent diagnoses included lower respiratory tract disease (pneumonia, lung effusion, or empyema; n = 1415, 7.1%), gastroenteritis/dehydration (n = 1068, 5.3%), respiratory failure (n = 731, 3.7%), febrile infant (n = 413, 2.1%), and nonfebrile seizure (n = 425, 2.1%). Aside from nonfebrile seizure, neurological complications were less frequent and included febrile seizure (n = 155, 0.8%), encephalopathy (n = 63, 0.3%), aseptic meningitis (n = 16, 0.1%), encephalitis (n = 11, 0.1%), and cerebral infarction (n = 6, <0.1%). Kawasaki disease and MIS-C comprised 1.7% (n = 346) of diagnoses. Thrombotic complications occurred in 0.1% (n = 13) of patients. Overall, these conditions and complications associated with COVID-19 were more frequent in hospitalized patients than in those discharged from the ED (P < .001) (Table 2).
Factors Associated With COVID-19 Disease Severity
Compared to pediatric patients with COVID-19 discharged from the ED, factors associated with increased odds of hospitalization included private payor insurance; obesity/type 2 DM; asthma; and cardiovascular, immunocompromised, neurologic/neuromuscular, and pulmonary CCCs (Table 3). Factors associated with decreased risk of hospitalization included Black race or Hispanic ethnicity compared with White race; female sex; and age 5 to 11 years and age 12 to 17 years (vs age 0-4 years). Among children and adolescents hospitalized with COVID-19, factors associated with greater disease severity included Black or other non-White race; age 5 to 11 years; age 12 to 17 years; obesity/type 2 DM; immunocompromised conditions; and cardiovascular, neurologic/neuromuscular, and pulmonary CCCs (Table 3).
Sensitivity Analysis
We performed a sensitivity analysis that expanded the study population to include those with a secondary diagnosis of COVID-19 plus a diagnosis of a COVID-19–associated condition or complication. Analyses using the expanded population (N = 21,247) were similar to the primary analyses (Appendix Table 4 and Appendix Table 5).
DISCUSSION
In this large multicenter study evaluating COVID-19 disease severity in more than 19,000 patients presenting for emergency care at US pediatric hospitals, approximately 20% were hospitalized, and among those hospitalized almost a quarter required ICU care. Clinical risk factors associated with increased risk of hospitalization include private payor status and selected comorbidities (obesity/type 2 DM; asthma; and cardiovascular, pulmonary, immunocompromised, neurologic/neuromuscular CCCs), while those associated with decreased risk of hospitalization include older age, female sex, and Black race or Hispanic ethnicity. Factors associated with severe disease among hospitalized pediatric patients include Black or other non-White race, school age (≥5 years), and certain chronic conditions (cardiovascular disease, obesity/type 2 DM, neurologic or neuromuscular disease). Sixteen percent of patients had a concurrent diagnosis for a condition or complication associated with COVID-19.
While the study population (ie, children and adolescents presenting to the ED) represents a small fraction of children and adolescents in the community with SARS-CoV-2 infection, the results provide important insight into factors of severe COVID-19 in the pediatric population. A report from France suggested ventilatory or hemodynamic support or death were independently associated with older age (≥10 years), elevated C-reactive protein, and hypoxemia.12 An Italian study found that younger age (0-4 years) was associated with less severe disease, while preexisting conditions were more likely in patients with severe disease.11 A single-center case series of 50 patients (aged ≤21 years) hospitalized at a children’s hospital in New York City found respiratory failure (n = 9) was more common in children older than 1 year, patients with elevated inflammatory markers, and patients with obesity.20
Our study confirms several factors for severe COVID-19 found in these studies, including older age,11,12,20 obesity,20 and preexisting conditions.11 Our findings also expand on these reports, including identification of factors associated with hospitalization. Given the rate of 30-day re-encounters among pediatric patients with COVID-19 (10.1%), identifying risk factors for hospitalization may aid ED providers in determining optimal disposition (eg, home, hospital admission, ICU). We also identified specific comorbidities associated with more severe disease in those hospitalized with COVID-19, such as cardiovascular disease, obesity/type 2 DM, and pulmonary, neurologic, or neuromuscular conditions. We also found that asthma increased the risk for hospitalization but not more severe disease among those hospitalized. This latter finding also aligns with recent single-center studies,21,22 whereas a Turkish study of pediatric patients aged 0 to 18 years found no association between asthma and COVID-19 hospitalizations.23We also examined payor type and racial/ethnic factors in our analysis. In 2019, patients who identified as Black or Hispanic comprised 52.3% of all encounters and 40.7% of hospitalizations recorded in the PHIS database. During the same year, encounters for influenza among Black or Hispanic pediatric patients comprised 58.7% of all influenza diagnoses and 47.0% of pediatric influenza hospitalizations (Appendix Table 2). In this study, patients who identified as Black or Hispanic race represented a disproportionately large share of patients presenting to children’s hospitals (68.5%) and of those hospitalized (60.8%). Hispanic ethnicity, in particular, represented a disproportionate share of patients seeking care for COVID-19 compared to the overall PHIS population (47.7% and 27.1%, respectively). After accounting for other factors, we found Black and other non-White race—but not of Hispanic ethnicity—were independently associated with more disease severity among those hospitalized. This contrasts with findings from a recent adult study by Yehia et al,24 who found (after adjusting for other clinical factors) no significant difference in mortality between Black patients and White patients among adults hospitalized due to COVID-19. It also contrasts with a recent large population-based UK study wherein pediatric patients identifying as Asian, but not Black or mixed race or ethnicity, had an increased risk of hospital admission and admission to the ICU compared to children identifying as White. Children identifying as Black or mixed race had longer hospital admissions.25 However, as the authors of the study note, residual confounders and ascertainment bias due to differences in COVID testing may have influenced these findings.
Our findings of differences in hospitalization and disease severity among those hospitalized by race and ethnicity should be interpreted carefully. These may reflect a constellation of factors that are difficult to measure, including differences in healthcare access, inequalities in care (including hospital admission inequalities), and implicit bias—all of which may reflect structural racism. For example, it is possible that children who identify as Black or Hispanic have different access to care compared to children who identify as White, and this may affect disease severity on presentation.2 Alternatively, it is possible that White pediatric patients are more likely to be hospitalized as compared to non-White pediatric patients with similar illness severity. Our finding that pediatric patients who identify as Hispanic or Black had a lower risk of hospitalization should be also interpreted carefully, as this may reflect higher utilization of the ED for SARS-CoV-2 testing, increased use of nonemergency services among those without access to primary care, or systematic differences in provider decision-making among this segment of the population.2 Further study is needed to determine specific drivers for racial and ethnic differences in healthcare utilization in children and adolescents with COVID-19.26
Complications and co-occurring diagnoses in adults with COVID-19 are well documented.27-30 However, there is little information to date on the co-occurring diagnoses and complications associated with COVID-19 in children and adolescents. We found that complications and co-occurring conditions occurred in 16.3% of the study population, with the most frequent conditions including known complications of viral infections such as pneumonia, respiratory failure, and seizures. Acute kidney and liver injury, as well as thrombotic complications, occurred less commonly than in adults.26-29 Interestingly, neurologic complications were also uncommon compared to adult reports8,31 and less frequent than in other viral illnesses in children and adolescents. For example, neurologic complications occur in approximately 7.5% of children and adolescents hospitalized with influenza.32
Limitations of the present study include the retrospective design, as well as incomplete patient-level clinical data in the PHIS database. The PHIS database only includes children’s hospitals, which may limit the generalizability of findings to community hospitals. We also excluded newborns, and our findings may not be generalizable to this population. We only included children and adolescents with a primary diagnosis of COVID-19, which has the potential for misclassification in cases where COVID-19 was a secondary diagnosis. However, results of our sensitivity analysis, which incorporated secondary diagnoses of COVID-19, were consistent with findings from our main analyses. Our study was designed to examine associations between certain prespecified factors and COVID-19 severity among pediatric patients who visited the ED or were admitted to the hospital during the COVID-19 pandemic. Thus, our findings must be interpreted in light of these considerations and may not be generalizable outside the ED or hospital setting. For example, it could be that some segments of the population utilized ED resources for testing, whereas others avoided the ED and other healthcare settings for fear of exposure to SARS-CoV-2. We also relied on diagnosis codes to identify concurrent diagnoses, as well as mechanical ventilation in our very severe outcome cohort, which resulted in this classification for some of these diagnoses. Despite these limitations, our findings represent an important step in understanding the risk factors associated with severe clinical COVID-19 disease in pediatric patients.
Our findings may inform future research and clinical interventions. Future studies on antiviral therapies and immune modulators targeting SARS-CoV-2 infection in children and adolescents should focus on high-risk populations, such as those identified in the study, as these patients are most likely to benefit from therapeutic interventions. Similarly, vaccine-development efforts may benefit from additional evaluation in high-risk populations, some of which may have altered immune responses. Furthermore, with increasing vaccination among adults and changes in recommendations, societal mitigation efforts (eg, masking, physical distancing) will diminish. Continued vigilance and COVID-19–mitigation efforts among high-risk children, for whom vaccines are not yet available, are critical during this transition.
CONCLUSION
Among children with COVID-19 who received care at children’s hospitals and EDs, 20% were hospitalized, and, of those, 21% were admitted to the ICU. Older children and adolescent patients had a lower risk of hospitalization; however, when hospitalized, they had greater illness severity. Those with selected comorbidities (eg, cardiovascular, obesity/type 2 DM, pulmonary and neurologic or neuromuscular disease) had both increased odds of hospitalization and in-hospital illness severity. While there were observed differences in COVID-19 severity by race and ethnicity, additional research is needed to clarify the drivers of such disparities. These factors should be considered when prioritizing mitigation strategies to prevent infection (eg, remote learning, avoidance of group activities, prioritization of COVID-19 vaccine when approved for children aged <12 years).
The COVID-19 pandemic has led to more than 40 million infections and more than 650,000 deaths in the United States alone.1 Morbidity and mortality have disproportionately affected older adults.2-4 However, acute infection and delayed effects, such as multisystem inflammatory syndrome in children (MIS-C), occur and can lead to severe complications, hospitalization, and death in pediatric patients.5,6 Due to higher clinical disease prevalence and morbidity in the adult population, we have learned much about the clinical factors associated with severe adult COVID-19 disease.5,7-9 Such clinical factors include older age, concurrent comorbidities, smoke exposure, and Black race or Hispanic ethnicity, among others.5,7-10 However, there is a paucity of data on severe COVID-19 disease in pediatric patients.5,11,12 In addition, most immunization strategies and pharmacologic treatments for COVID-19 have not been evaluated or approved for use in children.13 To guide targeted prevention and treatment strategies, there is a critical need to identify children and adolescents—who are among the most vulnerable patient populations—at high risk for severe disease.
Identifying the clinical factors associated with severe COVID-19 disease will help with prioritizing and allocating vaccines when they are approved for use in patients younger than 12 years.
METHODS
Study Design
We conducted a multicenter retrospective cohort study of patients presenting for care at pediatric hospitals that report data to the Pediatric Health Information System (PHIS) database. The PHIS administrative database includes billing and utilization data from 45 US tertiary care hospitals affiliated with the Children’s Hospital Association (Lenexa, Kansas). Data quality and reliability are ensured through a joint validation effort between the Children’s Hospital Association and participating hospitals. Hospitals submit discharge data, including demographics, diagnoses, and procedures using International Classification of Diseases, 10th Revision (ICD-10) codes, along with daily detailed information on pharmacy, location of care, and other services.
Study Population
Patients 30 days to 18 years of age discharged from the emergency department (ED) or inpatient setting with a primary diagnosis of COVID-19 (ICD-10 codes U.071 and U.072) between April 1, 2020, and September 30, 2020, were eligible for inclusion.14 In a prior study, the positive predictive value of an ICD-10–coded diagnosis of COVID-19 among hospitalized pediatric patients was 95.5%, compared with reverse transcription polymerase reaction results or presence of MIS-C.15 The diagnostic code for COVID-19 (ICD-10-CM) also had a high sensitivity (98.0%) in the hospitalized population.16 Acknowledging the increasing practice of screening patients upon admission, and in an attempt to minimize potential misclassification, we did not include encounters with secondary diagnoses of COVID-19 in our primary analyses. Pediatric patients with surgical diagnoses and neonates who never left the hospital were also excluded.
Factors Associated With Severe COVID-19 Disease
Exposures of interest were determined a priori based on current evidence in the literature and included patient age (0-4 years, 5-11 years, and 12-18 years), sex, race and ethnicity (non-Hispanic White, non-Hispanic Black, Hispanic, Asian, other non-White race [defined as Pacific Islander, Native American, or other]), payor type, cardiovascular complex chronic conditions (CCC), neuromuscular CCC, obesity/type 2 diabetes mellitus (DM), pulmonary CCC, asthma (defined using ICD-10 codes17), and immunocompromised CCC
Pediatric Complications and Conditions Associated With COVID-19
Based on current evidence and expert opinion of study members, associated diagnoses and complications co-occurring with a COVID-19 diagnosis were defined a priori and identified through ICD-10 codes (Appendix Table 1). These included acute kidney injury, acute liver injury, aseptic meningitis, asthma exacerbation, bronchiolitis, cerebral infarction, croup, encephalitis, encephalopathy, infant fever, febrile seizure, gastroenteritis/dehydration, Kawasaki disease/MIS-C, myocarditis/pericarditis, pneumonia, lung effusion or empyema, respiratory failure, sepsis, nonfebrile seizure, pancreatitis, sickle cell complications, and thrombotic complications.
Outcomes
COVID-19 severity outcomes were assessed as follows: (1) mild = ED discharge; (2) moderate = inpatient admission; (3) severe = intensive care unit (ICU) admission without mechanical ventilation, shock, or death; and (4) very severe = ICU admission with mechanical ventilation, shock, or death.19 This ordinal ranking system did not violate the proportional odds assumption. Potential reasons for admission to the ICU without mechanical ventilation, shock, or death include, but are not limited to, need for noninvasive ventilation, vital sign instability, dysrhythmias, respiratory insufficiency, or complications arising from concurrent conditions (eg, thrombotic events, need for continuous albuterol therapy). We examined several secondary, hospital-based outcomes, including associated diagnoses and complications, all-cause 30-day healthcare reutilization (ED visit or rehospitalization), length of stay (LOS), and ICU LOS.
Statistical Analysis
Demographic characteristics were summarized using frequencies and percentages for categorical variables and geometric means with SD and medians with interquartile ranges (IQR) for continuous variables, as appropriate. Factors associated with hospitalization (encompassing severity levels 2-4) vs ED discharge (severity level 1) were assessed using logistic regression. Factors associated with increasing severity among hospitalized pediatric patients (severity levels 2, 3, and 4) were assessed using ordinal logistic regression. Covariates in these analyses included race and ethnicity, age, sex, payor, cardiovascular CCC, neurologic/neuromuscular CCC, obesity/type 2 DM, pulmonary CCC, asthma, and immunocompromised CCC. Adjusted odds ratios (aOR) and corresponding 95% CI for each risk factor were generated using generalized linear mixed effects models and random intercepts for each hospital. Given the potential for diagnostic misclassification of pediatric patients with COVID-19 based on primary vs secondary diagnoses, we performed sensitivity analyses defining the study population as those with a primary diagnosis of COVID-19 and those with a secondary diagnosis of COVID-19 plus a concurrent primary diagnosis of a condition associated with COVID-19 (Appendix Table 1).
All analyses were performed using SAS version 9.4 (SAS Institute, Inc), and P < .05 was considered statistically significant. The Institutional Review Board at Vanderbilt University Medical Center determined that this study of de-identified data did not meet the criteria for human subjects research.
RESULTS
Study Population
A total of 19,976 encounters were included in the study. Of those, 15,913 (79.7%) were discharged from the ED and 4063 (20.3%) were hospitalized (Table 1). The most common race/ethnicity was Hispanic (9741, 48.8%), followed by non-Hispanic White (4217, 21.1%). Reference race/ethnicity data for the overall 2019 PHIS population can be found in Appendix Table 2.
The severity distribution among the hospitalized population was moderate (3222, 79.3%), severe (431, 11.3%), and very severe (380, 9.4%). The frequency of COVID-19 diagnoses increased late in the study period (Figure). Among those hospitalized, the median LOS for the index admission was 2 days (IQR, 1-4), while among those admitted to the ICU, the median LOS was 3 days (IQR, 2-5).
Overall, 10.1% (n = 2020) of the study population had an all-cause repeat encounter (ie, subsequent ED encounter or hospitalization) within 30 days following the index discharge. Repeat encounters were more frequent among patients hospitalized than among those discharged from the ED (Appendix Table 3).
Prevalence of Conditions and Complications Associated With COVID-19
Overall, 3257 (16.3%) patients had one or more co-occurring diagnoses categorized as a COVID-19–associated condition or complication. The most frequent diagnoses included lower respiratory tract disease (pneumonia, lung effusion, or empyema; n = 1415, 7.1%), gastroenteritis/dehydration (n = 1068, 5.3%), respiratory failure (n = 731, 3.7%), febrile infant (n = 413, 2.1%), and nonfebrile seizure (n = 425, 2.1%). Aside from nonfebrile seizure, neurological complications were less frequent and included febrile seizure (n = 155, 0.8%), encephalopathy (n = 63, 0.3%), aseptic meningitis (n = 16, 0.1%), encephalitis (n = 11, 0.1%), and cerebral infarction (n = 6, <0.1%). Kawasaki disease and MIS-C comprised 1.7% (n = 346) of diagnoses. Thrombotic complications occurred in 0.1% (n = 13) of patients. Overall, these conditions and complications associated with COVID-19 were more frequent in hospitalized patients than in those discharged from the ED (P < .001) (Table 2).
Factors Associated With COVID-19 Disease Severity
Compared to pediatric patients with COVID-19 discharged from the ED, factors associated with increased odds of hospitalization included private payor insurance; obesity/type 2 DM; asthma; and cardiovascular, immunocompromised, neurologic/neuromuscular, and pulmonary CCCs (Table 3). Factors associated with decreased risk of hospitalization included Black race or Hispanic ethnicity compared with White race; female sex; and age 5 to 11 years and age 12 to 17 years (vs age 0-4 years). Among children and adolescents hospitalized with COVID-19, factors associated with greater disease severity included Black or other non-White race; age 5 to 11 years; age 12 to 17 years; obesity/type 2 DM; immunocompromised conditions; and cardiovascular, neurologic/neuromuscular, and pulmonary CCCs (Table 3).
Sensitivity Analysis
We performed a sensitivity analysis that expanded the study population to include those with a secondary diagnosis of COVID-19 plus a diagnosis of a COVID-19–associated condition or complication. Analyses using the expanded population (N = 21,247) were similar to the primary analyses (Appendix Table 4 and Appendix Table 5).
DISCUSSION
In this large multicenter study evaluating COVID-19 disease severity in more than 19,000 patients presenting for emergency care at US pediatric hospitals, approximately 20% were hospitalized, and among those hospitalized almost a quarter required ICU care. Clinical risk factors associated with increased risk of hospitalization include private payor status and selected comorbidities (obesity/type 2 DM; asthma; and cardiovascular, pulmonary, immunocompromised, neurologic/neuromuscular CCCs), while those associated with decreased risk of hospitalization include older age, female sex, and Black race or Hispanic ethnicity. Factors associated with severe disease among hospitalized pediatric patients include Black or other non-White race, school age (≥5 years), and certain chronic conditions (cardiovascular disease, obesity/type 2 DM, neurologic or neuromuscular disease). Sixteen percent of patients had a concurrent diagnosis for a condition or complication associated with COVID-19.
While the study population (ie, children and adolescents presenting to the ED) represents a small fraction of children and adolescents in the community with SARS-CoV-2 infection, the results provide important insight into factors of severe COVID-19 in the pediatric population. A report from France suggested ventilatory or hemodynamic support or death were independently associated with older age (≥10 years), elevated C-reactive protein, and hypoxemia.12 An Italian study found that younger age (0-4 years) was associated with less severe disease, while preexisting conditions were more likely in patients with severe disease.11 A single-center case series of 50 patients (aged ≤21 years) hospitalized at a children’s hospital in New York City found respiratory failure (n = 9) was more common in children older than 1 year, patients with elevated inflammatory markers, and patients with obesity.20
Our study confirms several factors for severe COVID-19 found in these studies, including older age,11,12,20 obesity,20 and preexisting conditions.11 Our findings also expand on these reports, including identification of factors associated with hospitalization. Given the rate of 30-day re-encounters among pediatric patients with COVID-19 (10.1%), identifying risk factors for hospitalization may aid ED providers in determining optimal disposition (eg, home, hospital admission, ICU). We also identified specific comorbidities associated with more severe disease in those hospitalized with COVID-19, such as cardiovascular disease, obesity/type 2 DM, and pulmonary, neurologic, or neuromuscular conditions. We also found that asthma increased the risk for hospitalization but not more severe disease among those hospitalized. This latter finding also aligns with recent single-center studies,21,22 whereas a Turkish study of pediatric patients aged 0 to 18 years found no association between asthma and COVID-19 hospitalizations.23We also examined payor type and racial/ethnic factors in our analysis. In 2019, patients who identified as Black or Hispanic comprised 52.3% of all encounters and 40.7% of hospitalizations recorded in the PHIS database. During the same year, encounters for influenza among Black or Hispanic pediatric patients comprised 58.7% of all influenza diagnoses and 47.0% of pediatric influenza hospitalizations (Appendix Table 2). In this study, patients who identified as Black or Hispanic race represented a disproportionately large share of patients presenting to children’s hospitals (68.5%) and of those hospitalized (60.8%). Hispanic ethnicity, in particular, represented a disproportionate share of patients seeking care for COVID-19 compared to the overall PHIS population (47.7% and 27.1%, respectively). After accounting for other factors, we found Black and other non-White race—but not of Hispanic ethnicity—were independently associated with more disease severity among those hospitalized. This contrasts with findings from a recent adult study by Yehia et al,24 who found (after adjusting for other clinical factors) no significant difference in mortality between Black patients and White patients among adults hospitalized due to COVID-19. It also contrasts with a recent large population-based UK study wherein pediatric patients identifying as Asian, but not Black or mixed race or ethnicity, had an increased risk of hospital admission and admission to the ICU compared to children identifying as White. Children identifying as Black or mixed race had longer hospital admissions.25 However, as the authors of the study note, residual confounders and ascertainment bias due to differences in COVID testing may have influenced these findings.
Our findings of differences in hospitalization and disease severity among those hospitalized by race and ethnicity should be interpreted carefully. These may reflect a constellation of factors that are difficult to measure, including differences in healthcare access, inequalities in care (including hospital admission inequalities), and implicit bias—all of which may reflect structural racism. For example, it is possible that children who identify as Black or Hispanic have different access to care compared to children who identify as White, and this may affect disease severity on presentation.2 Alternatively, it is possible that White pediatric patients are more likely to be hospitalized as compared to non-White pediatric patients with similar illness severity. Our finding that pediatric patients who identify as Hispanic or Black had a lower risk of hospitalization should be also interpreted carefully, as this may reflect higher utilization of the ED for SARS-CoV-2 testing, increased use of nonemergency services among those without access to primary care, or systematic differences in provider decision-making among this segment of the population.2 Further study is needed to determine specific drivers for racial and ethnic differences in healthcare utilization in children and adolescents with COVID-19.26
Complications and co-occurring diagnoses in adults with COVID-19 are well documented.27-30 However, there is little information to date on the co-occurring diagnoses and complications associated with COVID-19 in children and adolescents. We found that complications and co-occurring conditions occurred in 16.3% of the study population, with the most frequent conditions including known complications of viral infections such as pneumonia, respiratory failure, and seizures. Acute kidney and liver injury, as well as thrombotic complications, occurred less commonly than in adults.26-29 Interestingly, neurologic complications were also uncommon compared to adult reports8,31 and less frequent than in other viral illnesses in children and adolescents. For example, neurologic complications occur in approximately 7.5% of children and adolescents hospitalized with influenza.32
Limitations of the present study include the retrospective design, as well as incomplete patient-level clinical data in the PHIS database. The PHIS database only includes children’s hospitals, which may limit the generalizability of findings to community hospitals. We also excluded newborns, and our findings may not be generalizable to this population. We only included children and adolescents with a primary diagnosis of COVID-19, which has the potential for misclassification in cases where COVID-19 was a secondary diagnosis. However, results of our sensitivity analysis, which incorporated secondary diagnoses of COVID-19, were consistent with findings from our main analyses. Our study was designed to examine associations between certain prespecified factors and COVID-19 severity among pediatric patients who visited the ED or were admitted to the hospital during the COVID-19 pandemic. Thus, our findings must be interpreted in light of these considerations and may not be generalizable outside the ED or hospital setting. For example, it could be that some segments of the population utilized ED resources for testing, whereas others avoided the ED and other healthcare settings for fear of exposure to SARS-CoV-2. We also relied on diagnosis codes to identify concurrent diagnoses, as well as mechanical ventilation in our very severe outcome cohort, which resulted in this classification for some of these diagnoses. Despite these limitations, our findings represent an important step in understanding the risk factors associated with severe clinical COVID-19 disease in pediatric patients.
Our findings may inform future research and clinical interventions. Future studies on antiviral therapies and immune modulators targeting SARS-CoV-2 infection in children and adolescents should focus on high-risk populations, such as those identified in the study, as these patients are most likely to benefit from therapeutic interventions. Similarly, vaccine-development efforts may benefit from additional evaluation in high-risk populations, some of which may have altered immune responses. Furthermore, with increasing vaccination among adults and changes in recommendations, societal mitigation efforts (eg, masking, physical distancing) will diminish. Continued vigilance and COVID-19–mitigation efforts among high-risk children, for whom vaccines are not yet available, are critical during this transition.
CONCLUSION
Among children with COVID-19 who received care at children’s hospitals and EDs, 20% were hospitalized, and, of those, 21% were admitted to the ICU. Older children and adolescent patients had a lower risk of hospitalization; however, when hospitalized, they had greater illness severity. Those with selected comorbidities (eg, cardiovascular, obesity/type 2 DM, pulmonary and neurologic or neuromuscular disease) had both increased odds of hospitalization and in-hospital illness severity. While there were observed differences in COVID-19 severity by race and ethnicity, additional research is needed to clarify the drivers of such disparities. These factors should be considered when prioritizing mitigation strategies to prevent infection (eg, remote learning, avoidance of group activities, prioritization of COVID-19 vaccine when approved for children aged <12 years).
1. Centers for Disease Control and Prevention. COVID data tracker. Accessed September 9, 2021. https://covid.cdc.gov/covid-data-tracker/#datatracker-home
2. Levy C, Basmaci R, Bensaid P, et al. Changes in reverse transcription polymerase chain reaction-positive severe acute respiratory syndrome coronavirus 2 rates in adults and children according to the epidemic stages. Pediatr Infect Dis J. 2020;39(11):e369-e372. https://doi.org/10.1097/inf.0000000000002861
3. Gudbjartsson DF, Helgason A, Jonsson H, et al. Spread of SARS-CoV-2 in the Icelandic population. N Engl J Med. 2020;382(24):2302-2315. https://doi.org/10.1056/nejmoa2006100
4. Garg S, Kim L, Whitaker M, et al. Hospitalization rates and characteristics of patients hospitalized with laboratory-confirmed coronavirus disease 2019 - COVID-NET, 14 States, March 1-30, 2020. MMWR Morb Mortal Wkly Rep. 2020;69(15):458-464. https://doi.org/10.15585/mmwr.mm6915e3
5. Castagnoli R, Votto M, Licari A, et al. Severe acute respiratory syndrome coronavirus 2 (SARS-CoV-2) infection in children and adolescents: a systematic review. JAMA Pediatr. 2020;174(9):882-889. https://doi.org/10.1001/jamapediatrics.2020.1467
6. Feldstein LR, Rose EB, Horwitz SM, et al; Overcoming COVID-19 Investigators; CDC COVID-19 Response Team. Multisystem inflammatory syndrome in U.S. children and adolescents. N Engl J Med. 2020;383(4):334-346. https://doi.org/10.1056/nejmoa2021680
7. Magro B, Zuccaro V, Novelli L, et al. Predicting in-hospital mortality from coronavirus disease 2019: a simple validated app for clinical use. PLoS One. 2021;16(1):e0245281. https://doi.org/10.1371/journal.pone.0245281
8. Helms J, Kremer S, Merdji H, et al. Neurologic features in severe SARS-CoV-2 infection. N Engl J Med. 2020;382(23):2268-2270. https://doi.org/10.1056/nejmc2008597
9. Severe Covid GWAS Group; Ellinghaus D, Degenhardt F, Bujanda L, et al. Genomewide association study of severe Covid-19 with respiratory failure. N Engl J Med. 2020;383(16):1522-1534.
10. Kabarriti R, Brodin NP, Maron MI, et al. association of race and ethnicity with comorbidities and survival among patients with COVID-19 at an urban medical center in New York. JAMA Netw Open. 2020;3(9):e2019795. https://doi.org/10.1001/jamanetworkopen.2020.19795
11. Bellino S, Punzo O, Rota MC, et al; COVID-19 Working Group. COVID-19 disease severity risk factors for pediatric patients in Italy. Pediatrics. 2020;146(4):e2020009399. https://doi.org/10.1542/peds.2020-009399
12. Ouldali N, Yang DD, Madhi F, et al; investigator group of the PANDOR study. Factors associated with severe SARS-CoV-2 infection. Pediatrics. 2020;147(3):e2020023432. https://doi.org/10.1542/peds.2020-023432
13. Castells MC, Phillips EJ. Maintaining safety with SARS-CoV-2 vaccines. N Engl J Med. 2021;384(7):643-649. https://doi.org/10.1056/nejmra2035343
14. Antoon JW, Williams DJ, Thurm C, et al. The COVID-19 pandemic and changes in healthcare utilization for pediatric respiratory and nonrespiratory illnesses in the United States. J Hosp Med. 2021;16(5):294-297. https://doi.org/10.12788/jhm.3608
15. Blatz AM, David MZ, Otto WR, Luan X, Gerber JS. Validation of International Classification of Disease-10 code for identifying children hospitalized with coronavirus disease-2019. J Pediatric Infect Dis Soc. 2020;10(4):547-548. https://doi.org/10.1093/jpids/piaa140
16. Kadri SS, Gundrum J, Warner S, et al. Uptake and accuracy of the diagnosis code for COVID-19 among US hospitalizations. JAMA. 2020;324(24):2553-2554. https://doi.org/10.1001/jama.2020.20323
17. Kaiser SV, Rodean J, Bekmezian A, et al; Pediatric Research in Inpatient Settings (PRIS) Network. Effectiveness of pediatric asthma pathways for hospitalized children: a multicenter, national analysis. J Pediatr. 2018;197:165-171.e162. https://doi.org/10.1016/j.jpeds.2018.01.084
18. Feudtner C, Feinstein JA, Zhong W, Hall M, Dai D. Pediatric complex chronic conditions classification system version 2: updated for ICD-10 and complex medical technology dependence and transplantation. BMC Pediatr. 2014;14:199. https://doi.org/10.1186/1471-2431-14-199.
19. Williams DJ, Zhu Y, Grijalva CG, et al. Predicting severe pneumonia outcomes in children. Pediatrics. 2016;138(4):e20161019. https://doi.org/10.1542/peds.2016-1019
20. Zachariah P, Johnson CL, Halabi KC, et al. Epidemiology, clinical features, and disease severity in patients with coronavirus disease 2019 (COVID-19) in a children’s hospital in New York City, New York. JAMA Pediatr. 2020;174(10):e202430. https://doi.org/10.1001/jamapediatrics.2020.2430
21. DeBiasi RL, Song X, Delaney M, et al. Severe coronavirus disease-2019 in children and young adults in the Washington, DC, metropolitan region. J Pediatr. 2020;223:199-203.e191. https://doi.org/10.1016/j.jpeds.2020.05.007
22. Lovinsky-Desir S, Deshpande DR, De A, et al. Asthma among hospitalized patients with COVID-19 and related outcomes. J Allergy Clin Immunol. 2020;146(5):1027-1034.e1024. https://doi.org/10.1016/j.jaci.2020.07.026
23. Beken B, Ozturk GK, Aygun FD, Aydogmus C, Akar HH. Asthma and allergic diseases are not risk factors for hospitalization in children with coronavirus disease 2019. Ann Allergy Asthma Immunol. 2021;126(5):569-575. https://doi.org/10.1016/j.anai.2021.01.018
24. Yehia BR, Winegar A, Fogel R, et al. Association of race with mortality among patients hospitalized with coronavirus disease 2019 (COVID-19) at 92 US hospitals. JAMA Netw Open. 2020;3(8):e2018039. https://doi.org/10.1001/jamanetworkopen.2020.18039
25. Saatci D, Ranger TA, Garriga C, et al. Association between race and COVID-19 outcomes among 2.6 million children in England. JAMA Pediatr. 2021;e211685. https://doi.org/10.1001/jamapediatrics.2021.1685
26. Lopez L, 3rd, Hart LH, 3rd, Katz MH. Racial and ethnic health disparities related to COVID-19. JAMA. 2021;325(8):719-720. https://doi.org/10.1001/jama.2020.26443
27. Altunok ES, Alkan M, Kamat S, et al. Clinical characteristics of adult patients hospitalized with laboratory-confirmed COVID-19 pneumonia. J Infect Chemother. 2020. https://doi.org/10.1016/j.jiac.2020.10.020
28. Ali H, Daoud A, Mohamed MM, et al. Survival rate in acute kidney injury superimposed COVID-19 patients: a systematic review and meta-analysis. Ren Fail. 2020;42(1):393-397. https://doi.org/10.1080/0886022x.2020.1756323
29. Anirvan P, Bharali P, Gogoi M, Thuluvath PJ, Singh SP, Satapathy SK. Liver injury in COVID-19: the hepatic aspect of the respiratory syndrome - what we know so far. World J Hepatol. 2020;12(12):1182-1197. https://doi.org/10.4254/wjh.v12.i12.1182
30. Moschonas IC, Tselepis AD. SARS-CoV-2 infection and thrombotic complications: a narrative review. J Thromb Thrombolysis. 2021;52(1):111-123. https://doi.org/10.1007/s11239-020-02374-3
31. Lee MH, Perl DP, Nair G, et al. Microvascular injury in the brains of patients with Covid-19. N Engl J Med. 2020;384(5):481-483. https://doi.org/10.1056/nejmc2033369
32. Antoon JW, Hall M, Herndon A, et al. Prevalence, risk factors, and outcomes of influenza-associated neurological Complications in Children. J Pediatr. 2021;S0022-3476(21)00657-0. https://doi.org/10.1016/j.jpeds.2021.06.075
1. Centers for Disease Control and Prevention. COVID data tracker. Accessed September 9, 2021. https://covid.cdc.gov/covid-data-tracker/#datatracker-home
2. Levy C, Basmaci R, Bensaid P, et al. Changes in reverse transcription polymerase chain reaction-positive severe acute respiratory syndrome coronavirus 2 rates in adults and children according to the epidemic stages. Pediatr Infect Dis J. 2020;39(11):e369-e372. https://doi.org/10.1097/inf.0000000000002861
3. Gudbjartsson DF, Helgason A, Jonsson H, et al. Spread of SARS-CoV-2 in the Icelandic population. N Engl J Med. 2020;382(24):2302-2315. https://doi.org/10.1056/nejmoa2006100
4. Garg S, Kim L, Whitaker M, et al. Hospitalization rates and characteristics of patients hospitalized with laboratory-confirmed coronavirus disease 2019 - COVID-NET, 14 States, March 1-30, 2020. MMWR Morb Mortal Wkly Rep. 2020;69(15):458-464. https://doi.org/10.15585/mmwr.mm6915e3
5. Castagnoli R, Votto M, Licari A, et al. Severe acute respiratory syndrome coronavirus 2 (SARS-CoV-2) infection in children and adolescents: a systematic review. JAMA Pediatr. 2020;174(9):882-889. https://doi.org/10.1001/jamapediatrics.2020.1467
6. Feldstein LR, Rose EB, Horwitz SM, et al; Overcoming COVID-19 Investigators; CDC COVID-19 Response Team. Multisystem inflammatory syndrome in U.S. children and adolescents. N Engl J Med. 2020;383(4):334-346. https://doi.org/10.1056/nejmoa2021680
7. Magro B, Zuccaro V, Novelli L, et al. Predicting in-hospital mortality from coronavirus disease 2019: a simple validated app for clinical use. PLoS One. 2021;16(1):e0245281. https://doi.org/10.1371/journal.pone.0245281
8. Helms J, Kremer S, Merdji H, et al. Neurologic features in severe SARS-CoV-2 infection. N Engl J Med. 2020;382(23):2268-2270. https://doi.org/10.1056/nejmc2008597
9. Severe Covid GWAS Group; Ellinghaus D, Degenhardt F, Bujanda L, et al. Genomewide association study of severe Covid-19 with respiratory failure. N Engl J Med. 2020;383(16):1522-1534.
10. Kabarriti R, Brodin NP, Maron MI, et al. association of race and ethnicity with comorbidities and survival among patients with COVID-19 at an urban medical center in New York. JAMA Netw Open. 2020;3(9):e2019795. https://doi.org/10.1001/jamanetworkopen.2020.19795
11. Bellino S, Punzo O, Rota MC, et al; COVID-19 Working Group. COVID-19 disease severity risk factors for pediatric patients in Italy. Pediatrics. 2020;146(4):e2020009399. https://doi.org/10.1542/peds.2020-009399
12. Ouldali N, Yang DD, Madhi F, et al; investigator group of the PANDOR study. Factors associated with severe SARS-CoV-2 infection. Pediatrics. 2020;147(3):e2020023432. https://doi.org/10.1542/peds.2020-023432
13. Castells MC, Phillips EJ. Maintaining safety with SARS-CoV-2 vaccines. N Engl J Med. 2021;384(7):643-649. https://doi.org/10.1056/nejmra2035343
14. Antoon JW, Williams DJ, Thurm C, et al. The COVID-19 pandemic and changes in healthcare utilization for pediatric respiratory and nonrespiratory illnesses in the United States. J Hosp Med. 2021;16(5):294-297. https://doi.org/10.12788/jhm.3608
15. Blatz AM, David MZ, Otto WR, Luan X, Gerber JS. Validation of International Classification of Disease-10 code for identifying children hospitalized with coronavirus disease-2019. J Pediatric Infect Dis Soc. 2020;10(4):547-548. https://doi.org/10.1093/jpids/piaa140
16. Kadri SS, Gundrum J, Warner S, et al. Uptake and accuracy of the diagnosis code for COVID-19 among US hospitalizations. JAMA. 2020;324(24):2553-2554. https://doi.org/10.1001/jama.2020.20323
17. Kaiser SV, Rodean J, Bekmezian A, et al; Pediatric Research in Inpatient Settings (PRIS) Network. Effectiveness of pediatric asthma pathways for hospitalized children: a multicenter, national analysis. J Pediatr. 2018;197:165-171.e162. https://doi.org/10.1016/j.jpeds.2018.01.084
18. Feudtner C, Feinstein JA, Zhong W, Hall M, Dai D. Pediatric complex chronic conditions classification system version 2: updated for ICD-10 and complex medical technology dependence and transplantation. BMC Pediatr. 2014;14:199. https://doi.org/10.1186/1471-2431-14-199.
19. Williams DJ, Zhu Y, Grijalva CG, et al. Predicting severe pneumonia outcomes in children. Pediatrics. 2016;138(4):e20161019. https://doi.org/10.1542/peds.2016-1019
20. Zachariah P, Johnson CL, Halabi KC, et al. Epidemiology, clinical features, and disease severity in patients with coronavirus disease 2019 (COVID-19) in a children’s hospital in New York City, New York. JAMA Pediatr. 2020;174(10):e202430. https://doi.org/10.1001/jamapediatrics.2020.2430
21. DeBiasi RL, Song X, Delaney M, et al. Severe coronavirus disease-2019 in children and young adults in the Washington, DC, metropolitan region. J Pediatr. 2020;223:199-203.e191. https://doi.org/10.1016/j.jpeds.2020.05.007
22. Lovinsky-Desir S, Deshpande DR, De A, et al. Asthma among hospitalized patients with COVID-19 and related outcomes. J Allergy Clin Immunol. 2020;146(5):1027-1034.e1024. https://doi.org/10.1016/j.jaci.2020.07.026
23. Beken B, Ozturk GK, Aygun FD, Aydogmus C, Akar HH. Asthma and allergic diseases are not risk factors for hospitalization in children with coronavirus disease 2019. Ann Allergy Asthma Immunol. 2021;126(5):569-575. https://doi.org/10.1016/j.anai.2021.01.018
24. Yehia BR, Winegar A, Fogel R, et al. Association of race with mortality among patients hospitalized with coronavirus disease 2019 (COVID-19) at 92 US hospitals. JAMA Netw Open. 2020;3(8):e2018039. https://doi.org/10.1001/jamanetworkopen.2020.18039
25. Saatci D, Ranger TA, Garriga C, et al. Association between race and COVID-19 outcomes among 2.6 million children in England. JAMA Pediatr. 2021;e211685. https://doi.org/10.1001/jamapediatrics.2021.1685
26. Lopez L, 3rd, Hart LH, 3rd, Katz MH. Racial and ethnic health disparities related to COVID-19. JAMA. 2021;325(8):719-720. https://doi.org/10.1001/jama.2020.26443
27. Altunok ES, Alkan M, Kamat S, et al. Clinical characteristics of adult patients hospitalized with laboratory-confirmed COVID-19 pneumonia. J Infect Chemother. 2020. https://doi.org/10.1016/j.jiac.2020.10.020
28. Ali H, Daoud A, Mohamed MM, et al. Survival rate in acute kidney injury superimposed COVID-19 patients: a systematic review and meta-analysis. Ren Fail. 2020;42(1):393-397. https://doi.org/10.1080/0886022x.2020.1756323
29. Anirvan P, Bharali P, Gogoi M, Thuluvath PJ, Singh SP, Satapathy SK. Liver injury in COVID-19: the hepatic aspect of the respiratory syndrome - what we know so far. World J Hepatol. 2020;12(12):1182-1197. https://doi.org/10.4254/wjh.v12.i12.1182
30. Moschonas IC, Tselepis AD. SARS-CoV-2 infection and thrombotic complications: a narrative review. J Thromb Thrombolysis. 2021;52(1):111-123. https://doi.org/10.1007/s11239-020-02374-3
31. Lee MH, Perl DP, Nair G, et al. Microvascular injury in the brains of patients with Covid-19. N Engl J Med. 2020;384(5):481-483. https://doi.org/10.1056/nejmc2033369
32. Antoon JW, Hall M, Herndon A, et al. Prevalence, risk factors, and outcomes of influenza-associated neurological Complications in Children. J Pediatr. 2021;S0022-3476(21)00657-0. https://doi.org/10.1016/j.jpeds.2021.06.075
© 2021 Society of Hospital Medicine
How Organizations Can Build a Successful and Sustainable Social Media Presence
Horwitz and Detsky1 provide readers with a personal, experientially based primer on how healthcare professionals can more effectively engage on Twitter. As experienced physicians, researchers, and active social media users, the authors outline pragmatic and specific recommendations on how to engage misinformation and add value to social media discourse. We applaud the authors for offering best-practice approaches that are valuable to newcomers as well as seasoned social media users. In highlighting that social media is merely a modern tool for engagement and discussion, the authors underscore the time-held idea that only when a tool is used effectively will it yield the desired outcome. As a medical journal that regularly uses social media as a tool for outreach and dissemination, we could not agree more with the authors’ assertion.
Since 2015, the Journal of Hospital Medicine (JHM) has used social media to engage its readership and extend the impact of the work published in its pages. Like Horwitz and Detsky, JHM has developed insights and experience in how medical journals, organizations, institutions, and other academic programs can use social media effectively. Because of our experience in this area, we are often asked how to build a successful and sustainable social media presence. Here, we share five primary lessons on how to use social media as a tool to disseminate, connect, and engage.
ESTABLISH YOUR GOALS
As the flagship journal for the field of hospital medicine, we seek to disseminate the ideas and research that will inform health policy, optimize healthcare delivery, and improve patient outcomes while also building and sustaining an online community for professional engagement and growth. Our social media goals provide direction on how to interact, allow us to focus attention on what is important, and motivate our growth in this area. Simply put, we believe that using social media without defined goals would be like sailing a ship without a rudder.
KNOW YOUR AUDIENCE
As your organization establishes its goals, it is important to consider with whom you want to connect. Knowing your audience will allow you to better tailor the content you deliver through social media. For instance, we understand that as a journal focused on hospital medicine, our audience consists of busy clinicians, researchers, and medical educators who are trying to efficiently gather the most up-to-date information in our field. Recognizing this, we produce (and make available for download) Visual Abstracts and publish them on Twitter to help our followers assimilate information from new studies quickly and easily.2 Moreover, we recognize that our followers are interested in how to use social media in their professional lives and have published several articles in this topic area.3-5
BUILD YOUR TEAM
We have found that having multiple individuals on our social media team has led to greater creativity and thoughtfulness on how we engage our readership. Our teams span generations, clinical experience, institutions, and cultural backgrounds. This intentional approach has allowed for diversity in thoughts and opinions and has helped shape the JHM social media message. Additionally, we have not only formalized editorial roles through the creation of Digital Media Editor positions, but we have also created the JHM Digital Media Fellowship, a training program and development pipeline for those interested in cultivating organization-based social media experiences and skill sets.6
ENGAGE CONSISTENTLY
Many organizations believe that successful social media outreach means creating an account and posting content when convenient. Experience has taught us that daily postings and regular engagement will build your brand as a regular and reliable source of information for your followers. Additionally, while many academic journals and organizations only occasionally post material and rarely interact with their followers, we have found that engaging and facilitating conversations through our monthly Twitter discussion (#JHMChat) has established a community, created opportunities for professional networking, and further disseminated the work published in JHM.7 As an academic journal or organization entering this field, recognize the product for which people follow you and deliver that product on a consistent basis.
OWN YOUR MISTAKES
It will only be a matter of time before your organization makes a misstep on social media. Instead of hiding, we recommend stepping into that tension and owning the mistake. For example, we recently published an article that contained a culturally offensive term. As a journal, we reflected on our error and took concrete steps to correct it. Further, we shared our thoughts with our followers to ensure transparency.8 Moving forward, we have inserted specific stopgaps in our editorial review process to avoid such missteps in the future.
Although every organization will have different goals and reasons for engaging on social media, we believe these central tenets will help optimize the use of this platform. Although we have established specific objectives for our engagement on social media, we believe Horwitz and Detsky1 put it best when they note that, at the end of the day, our ultimate goal is in “…promoting knowledge and science in a way that helps us all live healthier and happier lives."
1. Horwitz LI, Detsky AS. Tweeting into the void: effective use of social media for healthcare professionals. J Hosp Med. 2021;16(10):581-582. https://doi.org/10.12788/jhm.3684
2. 2021 Visual Abstracts. Accessed September 8, 2021. https://www.journalofhospitalmedicine.com/jhospmed/page/2021-visual-abstracts
3. Kumar A, Chen N, Singh A. #ConsentObtained - patient privacy in the age of social media. J Hosp Med. 2020;15(11):702-704. https://doi.org/10.12788/jhm.3416
4. Minter DJ, Patel A, Ganeshan S, Nematollahi S. Medical communities go virtual. J Hosp Med. 2021;16(6):378-380. https://doi.org/10.12788/jhm.3532
5. Marcelin JR, Cawcutt KA, Shapiro M, Varghese T, O’Glasser A. Moment vs movement: mission-based tweeting for physician advocacy. J Hosp Med. 2021;16(8):507-509. https://doi.org/10.12788/jhm.3636
6. Editorial Fellowships (Digital Media and Editorial). Accessed September 8, 2021. https://www.journalofhospitalmedicine.com/content/editorial-fellowships-digital-media-and-editorial
7. Wray CM, Auerbach AD, Arora VM. The adoption of an online journal club to improve research dissemination and social media engagement among hospitalists. J Hosp Med. 2018;13(11):764-769. https://doi.org/10.12788/jhm.2987
8. Shah SS, Manning KD, Wray CM, Castellanos A, Jerardi KE. Microaggressions, accountability, and our commitment to doing better [editorial]. J Hosp Med. 2021;16(6):325. https://doi.org/10.12788/jhm.3646
Horwitz and Detsky1 provide readers with a personal, experientially based primer on how healthcare professionals can more effectively engage on Twitter. As experienced physicians, researchers, and active social media users, the authors outline pragmatic and specific recommendations on how to engage misinformation and add value to social media discourse. We applaud the authors for offering best-practice approaches that are valuable to newcomers as well as seasoned social media users. In highlighting that social media is merely a modern tool for engagement and discussion, the authors underscore the time-held idea that only when a tool is used effectively will it yield the desired outcome. As a medical journal that regularly uses social media as a tool for outreach and dissemination, we could not agree more with the authors’ assertion.
Since 2015, the Journal of Hospital Medicine (JHM) has used social media to engage its readership and extend the impact of the work published in its pages. Like Horwitz and Detsky, JHM has developed insights and experience in how medical journals, organizations, institutions, and other academic programs can use social media effectively. Because of our experience in this area, we are often asked how to build a successful and sustainable social media presence. Here, we share five primary lessons on how to use social media as a tool to disseminate, connect, and engage.
ESTABLISH YOUR GOALS
As the flagship journal for the field of hospital medicine, we seek to disseminate the ideas and research that will inform health policy, optimize healthcare delivery, and improve patient outcomes while also building and sustaining an online community for professional engagement and growth. Our social media goals provide direction on how to interact, allow us to focus attention on what is important, and motivate our growth in this area. Simply put, we believe that using social media without defined goals would be like sailing a ship without a rudder.
KNOW YOUR AUDIENCE
As your organization establishes its goals, it is important to consider with whom you want to connect. Knowing your audience will allow you to better tailor the content you deliver through social media. For instance, we understand that as a journal focused on hospital medicine, our audience consists of busy clinicians, researchers, and medical educators who are trying to efficiently gather the most up-to-date information in our field. Recognizing this, we produce (and make available for download) Visual Abstracts and publish them on Twitter to help our followers assimilate information from new studies quickly and easily.2 Moreover, we recognize that our followers are interested in how to use social media in their professional lives and have published several articles in this topic area.3-5
BUILD YOUR TEAM
We have found that having multiple individuals on our social media team has led to greater creativity and thoughtfulness on how we engage our readership. Our teams span generations, clinical experience, institutions, and cultural backgrounds. This intentional approach has allowed for diversity in thoughts and opinions and has helped shape the JHM social media message. Additionally, we have not only formalized editorial roles through the creation of Digital Media Editor positions, but we have also created the JHM Digital Media Fellowship, a training program and development pipeline for those interested in cultivating organization-based social media experiences and skill sets.6
ENGAGE CONSISTENTLY
Many organizations believe that successful social media outreach means creating an account and posting content when convenient. Experience has taught us that daily postings and regular engagement will build your brand as a regular and reliable source of information for your followers. Additionally, while many academic journals and organizations only occasionally post material and rarely interact with their followers, we have found that engaging and facilitating conversations through our monthly Twitter discussion (#JHMChat) has established a community, created opportunities for professional networking, and further disseminated the work published in JHM.7 As an academic journal or organization entering this field, recognize the product for which people follow you and deliver that product on a consistent basis.
OWN YOUR MISTAKES
It will only be a matter of time before your organization makes a misstep on social media. Instead of hiding, we recommend stepping into that tension and owning the mistake. For example, we recently published an article that contained a culturally offensive term. As a journal, we reflected on our error and took concrete steps to correct it. Further, we shared our thoughts with our followers to ensure transparency.8 Moving forward, we have inserted specific stopgaps in our editorial review process to avoid such missteps in the future.
Although every organization will have different goals and reasons for engaging on social media, we believe these central tenets will help optimize the use of this platform. Although we have established specific objectives for our engagement on social media, we believe Horwitz and Detsky1 put it best when they note that, at the end of the day, our ultimate goal is in “…promoting knowledge and science in a way that helps us all live healthier and happier lives."
Horwitz and Detsky1 provide readers with a personal, experientially based primer on how healthcare professionals can more effectively engage on Twitter. As experienced physicians, researchers, and active social media users, the authors outline pragmatic and specific recommendations on how to engage misinformation and add value to social media discourse. We applaud the authors for offering best-practice approaches that are valuable to newcomers as well as seasoned social media users. In highlighting that social media is merely a modern tool for engagement and discussion, the authors underscore the time-held idea that only when a tool is used effectively will it yield the desired outcome. As a medical journal that regularly uses social media as a tool for outreach and dissemination, we could not agree more with the authors’ assertion.
Since 2015, the Journal of Hospital Medicine (JHM) has used social media to engage its readership and extend the impact of the work published in its pages. Like Horwitz and Detsky, JHM has developed insights and experience in how medical journals, organizations, institutions, and other academic programs can use social media effectively. Because of our experience in this area, we are often asked how to build a successful and sustainable social media presence. Here, we share five primary lessons on how to use social media as a tool to disseminate, connect, and engage.
ESTABLISH YOUR GOALS
As the flagship journal for the field of hospital medicine, we seek to disseminate the ideas and research that will inform health policy, optimize healthcare delivery, and improve patient outcomes while also building and sustaining an online community for professional engagement and growth. Our social media goals provide direction on how to interact, allow us to focus attention on what is important, and motivate our growth in this area. Simply put, we believe that using social media without defined goals would be like sailing a ship without a rudder.
KNOW YOUR AUDIENCE
As your organization establishes its goals, it is important to consider with whom you want to connect. Knowing your audience will allow you to better tailor the content you deliver through social media. For instance, we understand that as a journal focused on hospital medicine, our audience consists of busy clinicians, researchers, and medical educators who are trying to efficiently gather the most up-to-date information in our field. Recognizing this, we produce (and make available for download) Visual Abstracts and publish them on Twitter to help our followers assimilate information from new studies quickly and easily.2 Moreover, we recognize that our followers are interested in how to use social media in their professional lives and have published several articles in this topic area.3-5
BUILD YOUR TEAM
We have found that having multiple individuals on our social media team has led to greater creativity and thoughtfulness on how we engage our readership. Our teams span generations, clinical experience, institutions, and cultural backgrounds. This intentional approach has allowed for diversity in thoughts and opinions and has helped shape the JHM social media message. Additionally, we have not only formalized editorial roles through the creation of Digital Media Editor positions, but we have also created the JHM Digital Media Fellowship, a training program and development pipeline for those interested in cultivating organization-based social media experiences and skill sets.6
ENGAGE CONSISTENTLY
Many organizations believe that successful social media outreach means creating an account and posting content when convenient. Experience has taught us that daily postings and regular engagement will build your brand as a regular and reliable source of information for your followers. Additionally, while many academic journals and organizations only occasionally post material and rarely interact with their followers, we have found that engaging and facilitating conversations through our monthly Twitter discussion (#JHMChat) has established a community, created opportunities for professional networking, and further disseminated the work published in JHM.7 As an academic journal or organization entering this field, recognize the product for which people follow you and deliver that product on a consistent basis.
OWN YOUR MISTAKES
It will only be a matter of time before your organization makes a misstep on social media. Instead of hiding, we recommend stepping into that tension and owning the mistake. For example, we recently published an article that contained a culturally offensive term. As a journal, we reflected on our error and took concrete steps to correct it. Further, we shared our thoughts with our followers to ensure transparency.8 Moving forward, we have inserted specific stopgaps in our editorial review process to avoid such missteps in the future.
Although every organization will have different goals and reasons for engaging on social media, we believe these central tenets will help optimize the use of this platform. Although we have established specific objectives for our engagement on social media, we believe Horwitz and Detsky1 put it best when they note that, at the end of the day, our ultimate goal is in “…promoting knowledge and science in a way that helps us all live healthier and happier lives."
1. Horwitz LI, Detsky AS. Tweeting into the void: effective use of social media for healthcare professionals. J Hosp Med. 2021;16(10):581-582. https://doi.org/10.12788/jhm.3684
2. 2021 Visual Abstracts. Accessed September 8, 2021. https://www.journalofhospitalmedicine.com/jhospmed/page/2021-visual-abstracts
3. Kumar A, Chen N, Singh A. #ConsentObtained - patient privacy in the age of social media. J Hosp Med. 2020;15(11):702-704. https://doi.org/10.12788/jhm.3416
4. Minter DJ, Patel A, Ganeshan S, Nematollahi S. Medical communities go virtual. J Hosp Med. 2021;16(6):378-380. https://doi.org/10.12788/jhm.3532
5. Marcelin JR, Cawcutt KA, Shapiro M, Varghese T, O’Glasser A. Moment vs movement: mission-based tweeting for physician advocacy. J Hosp Med. 2021;16(8):507-509. https://doi.org/10.12788/jhm.3636
6. Editorial Fellowships (Digital Media and Editorial). Accessed September 8, 2021. https://www.journalofhospitalmedicine.com/content/editorial-fellowships-digital-media-and-editorial
7. Wray CM, Auerbach AD, Arora VM. The adoption of an online journal club to improve research dissemination and social media engagement among hospitalists. J Hosp Med. 2018;13(11):764-769. https://doi.org/10.12788/jhm.2987
8. Shah SS, Manning KD, Wray CM, Castellanos A, Jerardi KE. Microaggressions, accountability, and our commitment to doing better [editorial]. J Hosp Med. 2021;16(6):325. https://doi.org/10.12788/jhm.3646
1. Horwitz LI, Detsky AS. Tweeting into the void: effective use of social media for healthcare professionals. J Hosp Med. 2021;16(10):581-582. https://doi.org/10.12788/jhm.3684
2. 2021 Visual Abstracts. Accessed September 8, 2021. https://www.journalofhospitalmedicine.com/jhospmed/page/2021-visual-abstracts
3. Kumar A, Chen N, Singh A. #ConsentObtained - patient privacy in the age of social media. J Hosp Med. 2020;15(11):702-704. https://doi.org/10.12788/jhm.3416
4. Minter DJ, Patel A, Ganeshan S, Nematollahi S. Medical communities go virtual. J Hosp Med. 2021;16(6):378-380. https://doi.org/10.12788/jhm.3532
5. Marcelin JR, Cawcutt KA, Shapiro M, Varghese T, O’Glasser A. Moment vs movement: mission-based tweeting for physician advocacy. J Hosp Med. 2021;16(8):507-509. https://doi.org/10.12788/jhm.3636
6. Editorial Fellowships (Digital Media and Editorial). Accessed September 8, 2021. https://www.journalofhospitalmedicine.com/content/editorial-fellowships-digital-media-and-editorial
7. Wray CM, Auerbach AD, Arora VM. The adoption of an online journal club to improve research dissemination and social media engagement among hospitalists. J Hosp Med. 2018;13(11):764-769. https://doi.org/10.12788/jhm.2987
8. Shah SS, Manning KD, Wray CM, Castellanos A, Jerardi KE. Microaggressions, accountability, and our commitment to doing better [editorial]. J Hosp Med. 2021;16(6):325. https://doi.org/10.12788/jhm.3646
© 2021 Society of Hospital Medicine
Leadership & Professional Development: How to Teach When You Don’t Know
“By learning you will teach, by teaching you will learn.”
–Latin proverb
The COVID-19 pandemic thrust hospitalists into uncertain clinical situations where scientific evidence was rapidly changing and expert consensus was not always available. Amidst this, our learners were eager to better understand this new disease and how to properly care for patients. This forced hospitalists, as educators, to face the question: “How do you teach when you don’t have the answers?”
Teaching outside a hospitalist’s expertise existed before the COVID-19 pandemic and will continue to exist. It is a challenge encountered frequently by both junior and senior faculty across all disciplines, yet is rarely discussed.1 However, great learning can still occur when we teach at the edge of our comfort zones.
Acknowledge What You Don’t Know
You don’t need to be an expert to be a great teacher. Although most educators know this, we often fear that disclosing our knowledge limitations exposes our weaknesses. But a successful start to the learning journey begins with establishing trust and confidence with your learners. Remaining authentic in your knowledge base will inspire more credibility than false pretenses of content mastery. Phrases like, “This topic is new for me as well. Here’s what I do know and what I don’t know” or “What a great question. I wish I had a great answer. Let me get back to you” set a standard for honesty and reduce teaching pressures. In turn, learners will be more comfortable acknowledging their own uncertainties and will be more likely to voice their hesitations or ask questions on rounds.
Allow Yourself to Be the Student
The field of medicine is steeped in hierarchical structure, where the attending is assumed to have the most knowledge. But this may not always be true, as learners are often more up to date on a subject than the attending. By reexamining traditional hierarchies and instead considering ourselves as part of a learning team, we can promote a more positive educational climate.
When a learner asks a question that you don’t have an answer for, the response “Great question. I can tell you what I think, but I’m interested in first hearing your thoughts” reflects that you respect your learners and their skills and experiences. You can also ask them to do a literature review and report back to you and the team the next morning. By inverting the hierarchy, you are teaching humility, adaptability, and shared responsibility, as well as demonstrating the skills of being a lifelong learner.2
Teach the Skills You Do Have
As educators, we often hold ourselves to unrealistic expectations of being omniscient knowledge vessels. In times of crisis or uncertainty, teaching about how to learn and where to learn become just as important as what to learn. Invite learners to observe how you navigate ambiguity. For example, I recently interacted with a colleague on an unfamiliar case. She said, “Dr Wang, I don’t know much about malaria. Can you share with me what made you consider this diagnosis?” Additionally, admitting to learners when you have made an error not only clarifies their learning, but also role models continuous personal improvement.
By modeling humility by acknowledging our own limits, respecting our learners’ knowledge and experiences, and demonstrating how we manage uncertainty, we can enhance the learning environment and inspire our learners.
1. Huston T. Teaching What You Don’t Know. Harvard University Press; 2012.
2. Heifetz R, Grashow A, Linsky M. Leadership in a (permanent) crisis. Har Bus Rev. 2019;87(7-8):62-69, 153.
“By learning you will teach, by teaching you will learn.”
–Latin proverb
The COVID-19 pandemic thrust hospitalists into uncertain clinical situations where scientific evidence was rapidly changing and expert consensus was not always available. Amidst this, our learners were eager to better understand this new disease and how to properly care for patients. This forced hospitalists, as educators, to face the question: “How do you teach when you don’t have the answers?”
Teaching outside a hospitalist’s expertise existed before the COVID-19 pandemic and will continue to exist. It is a challenge encountered frequently by both junior and senior faculty across all disciplines, yet is rarely discussed.1 However, great learning can still occur when we teach at the edge of our comfort zones.
Acknowledge What You Don’t Know
You don’t need to be an expert to be a great teacher. Although most educators know this, we often fear that disclosing our knowledge limitations exposes our weaknesses. But a successful start to the learning journey begins with establishing trust and confidence with your learners. Remaining authentic in your knowledge base will inspire more credibility than false pretenses of content mastery. Phrases like, “This topic is new for me as well. Here’s what I do know and what I don’t know” or “What a great question. I wish I had a great answer. Let me get back to you” set a standard for honesty and reduce teaching pressures. In turn, learners will be more comfortable acknowledging their own uncertainties and will be more likely to voice their hesitations or ask questions on rounds.
Allow Yourself to Be the Student
The field of medicine is steeped in hierarchical structure, where the attending is assumed to have the most knowledge. But this may not always be true, as learners are often more up to date on a subject than the attending. By reexamining traditional hierarchies and instead considering ourselves as part of a learning team, we can promote a more positive educational climate.
When a learner asks a question that you don’t have an answer for, the response “Great question. I can tell you what I think, but I’m interested in first hearing your thoughts” reflects that you respect your learners and their skills and experiences. You can also ask them to do a literature review and report back to you and the team the next morning. By inverting the hierarchy, you are teaching humility, adaptability, and shared responsibility, as well as demonstrating the skills of being a lifelong learner.2
Teach the Skills You Do Have
As educators, we often hold ourselves to unrealistic expectations of being omniscient knowledge vessels. In times of crisis or uncertainty, teaching about how to learn and where to learn become just as important as what to learn. Invite learners to observe how you navigate ambiguity. For example, I recently interacted with a colleague on an unfamiliar case. She said, “Dr Wang, I don’t know much about malaria. Can you share with me what made you consider this diagnosis?” Additionally, admitting to learners when you have made an error not only clarifies their learning, but also role models continuous personal improvement.
By modeling humility by acknowledging our own limits, respecting our learners’ knowledge and experiences, and demonstrating how we manage uncertainty, we can enhance the learning environment and inspire our learners.
“By learning you will teach, by teaching you will learn.”
–Latin proverb
The COVID-19 pandemic thrust hospitalists into uncertain clinical situations where scientific evidence was rapidly changing and expert consensus was not always available. Amidst this, our learners were eager to better understand this new disease and how to properly care for patients. This forced hospitalists, as educators, to face the question: “How do you teach when you don’t have the answers?”
Teaching outside a hospitalist’s expertise existed before the COVID-19 pandemic and will continue to exist. It is a challenge encountered frequently by both junior and senior faculty across all disciplines, yet is rarely discussed.1 However, great learning can still occur when we teach at the edge of our comfort zones.
Acknowledge What You Don’t Know
You don’t need to be an expert to be a great teacher. Although most educators know this, we often fear that disclosing our knowledge limitations exposes our weaknesses. But a successful start to the learning journey begins with establishing trust and confidence with your learners. Remaining authentic in your knowledge base will inspire more credibility than false pretenses of content mastery. Phrases like, “This topic is new for me as well. Here’s what I do know and what I don’t know” or “What a great question. I wish I had a great answer. Let me get back to you” set a standard for honesty and reduce teaching pressures. In turn, learners will be more comfortable acknowledging their own uncertainties and will be more likely to voice their hesitations or ask questions on rounds.
Allow Yourself to Be the Student
The field of medicine is steeped in hierarchical structure, where the attending is assumed to have the most knowledge. But this may not always be true, as learners are often more up to date on a subject than the attending. By reexamining traditional hierarchies and instead considering ourselves as part of a learning team, we can promote a more positive educational climate.
When a learner asks a question that you don’t have an answer for, the response “Great question. I can tell you what I think, but I’m interested in first hearing your thoughts” reflects that you respect your learners and their skills and experiences. You can also ask them to do a literature review and report back to you and the team the next morning. By inverting the hierarchy, you are teaching humility, adaptability, and shared responsibility, as well as demonstrating the skills of being a lifelong learner.2
Teach the Skills You Do Have
As educators, we often hold ourselves to unrealistic expectations of being omniscient knowledge vessels. In times of crisis or uncertainty, teaching about how to learn and where to learn become just as important as what to learn. Invite learners to observe how you navigate ambiguity. For example, I recently interacted with a colleague on an unfamiliar case. She said, “Dr Wang, I don’t know much about malaria. Can you share with me what made you consider this diagnosis?” Additionally, admitting to learners when you have made an error not only clarifies their learning, but also role models continuous personal improvement.
By modeling humility by acknowledging our own limits, respecting our learners’ knowledge and experiences, and demonstrating how we manage uncertainty, we can enhance the learning environment and inspire our learners.
1. Huston T. Teaching What You Don’t Know. Harvard University Press; 2012.
2. Heifetz R, Grashow A, Linsky M. Leadership in a (permanent) crisis. Har Bus Rev. 2019;87(7-8):62-69, 153.
1. Huston T. Teaching What You Don’t Know. Harvard University Press; 2012.
2. Heifetz R, Grashow A, Linsky M. Leadership in a (permanent) crisis. Har Bus Rev. 2019;87(7-8):62-69, 153.
© 2021 Society of Hospital Medicine
The Limited Academic Footprint of Hospital Medicine: Where Do We Go From Here?
What has been the scholarly output of academic hospital medicine faculty (AHMF) and what academic rank have they achieved at US academic medical centers (AMCs)? Sumarsono et al1 address these questions and add to the growing body of literature exposing the limited academic footprint of hospitalists.
The authors performed a cross-sectional analysis of AHMF affiliated with the top 25 internal medicine training programs (as determined by the physician networking service doximity.com) and used Scopus to determine number of publications, citations, and H-index (a metric of productivity) for each faculty member. They also evaluated predictors for promotion. In contrast, most prior research on this topic relies on data obtained by survey methodology.2-5
Among 1554 AHMF from 22 AMCs, 42 (2.7%) were full professors and 140 (9.0%) were associate professors. The number of publications per AHMF was noticeably low, with a mean of 6.3 and median of 0 (interquartile range, 0-4). The authors found that H-index, completion of chief residency, and graduation from a top 25 medical school were independently associated with promotion.
The authors only evaluated AHMF among the most academically rigorous AMCs, an approach that likely overestimates scholarly output of hospitalists across all US AMCs. Conversely, if we presume that promotion is more difficult at these major AMCs, the results may underestimate academic rank of AHMF nationally. Additionally, the authors did not distinguish faculty by tracks (eg, clinician-investigators, clinician-educators), which often have different criteria for academic promotion.
These findings are worrisomely consistent with prior reports, despite the tremendous expansion of the field.2-4 A 2008 survey of academic hospitalists found that 4% of respondents were full professors and 9% were associate professors, values nearly identical to the results in this current analysis,4 suggesting enduring barriers to academic advancement.
We are left with the following questions provoked by this body of literature: How can hospitalists increase their scholarly output and climb the promotional ladder? And how can we increase the academic footprint of hospital medicine? We recently proposed the following strategies based on a survey of academic groups participating in the Hospital Medicine Reengineering Network (HOMERuN) survey5: (1) expand hospital medicine research fellowships, which will provide graduates with research skills to justify dedicated time for research and aid their ability to obtain independent funding; (2) formalize mentorship between research faculty in hospital medicine and other internal medicine disciplines with robust track records for research; (3) invest in research infrastructure and data access within and between institutions; and (4) encourage hospital medicine group leaders to foster academic growth by incentivizing faculty to perform research, present their work at national conferences, and publish manuscripts with their findings.
Although an increase in scholarly output should contribute to higher academic rank, hospitalists routinely make other invaluable contributions beyond clinical care to AMCs, including medical education, hospital leadership, quality improvement, clinical innovation, and social justice advocacy. Also, hospitalists are increasingly disseminating their contributions via newer mediums (eg, social media, podcasts) that arguably have greater reach than traditional scholarship outlets. We believe that promotion committees should update their criteria to reflect the evolution of academic contribution and integrate these within traditional promotion pathways.
Finally, we must address federal funding mechanisms, which currently favor specialty-specific funding over funding that would be more applicable to hospital medicine researchers. Funding agencies are largely specialty- or disease-specific, with limited options for broader-based research.6 Additionally, grant-review committees are largely comprised of specialists, with few generalists and fewer hospitalists. These limitations make it difficult to “argue” the necessity of hospital medicine research. One concrete step would be for the National Institutes of Health (NIH) to create an Office for Hospital Medicine Research, analogous to the Office of Emergency Care Research, which works across NIH institutes and centers to foster research and research training for the emergency setting.
With these strategies, we are hopeful that hospital medicine will continue to expand its academic footprint and be recognized for its ever-growing contributions to the practice of medicine.
1. Sumarsono A, Keshvani N, Saleh SN, et al. Scholarly productivity and rank in academic hospital medicine. J Hosp Med. 2021;16(9):545-548. https://doi.org/10.12788/jhm.3631
2. Chopra V, Burden M, Jones CD, et al. State of research in adult hospital medicine: results of a national survey. J Hosp Med. 2019;14(4):207-211. https://doi.org/10.12788/jhm.3136
3. Miller CS, Fogerty RL, Gann J, et al, the Society of General Internal Medicine Membership Committee. The growth of hospitalists and the future of the society of general internal medicine: results from the 2014 membership survey. J Gen Intern Med. 2017;32(11):1179-1185. https://doi.org/10.1007/s11606-017-4126-7
4. Reid MB, Misky GJ, Harrison RA, Sharpe B, Auerbach A, Glasheen JJ. Mentorship, productivity, and promotion among academic hospitalists. J Gen Intern Med. 2012;27(1):23-27. https://doi.org/10.1007/s11606-011-1892-5
5. Shannon EM, Chopra V, Greysen SR, et al. Dearth of hospitalist investigators in academic medicine: a call to action. J Hosp Med. 2021;16(3):189-191. https://doi.org/10.12788/jhm.3536
6. Levinson W, Linzer M. What is an academic general internist? Career options and training pathways. JAMA. 2002;288(16):2045-2048. https://doi.org/10.1001/jama.288.16.2045
What has been the scholarly output of academic hospital medicine faculty (AHMF) and what academic rank have they achieved at US academic medical centers (AMCs)? Sumarsono et al1 address these questions and add to the growing body of literature exposing the limited academic footprint of hospitalists.
The authors performed a cross-sectional analysis of AHMF affiliated with the top 25 internal medicine training programs (as determined by the physician networking service doximity.com) and used Scopus to determine number of publications, citations, and H-index (a metric of productivity) for each faculty member. They also evaluated predictors for promotion. In contrast, most prior research on this topic relies on data obtained by survey methodology.2-5
Among 1554 AHMF from 22 AMCs, 42 (2.7%) were full professors and 140 (9.0%) were associate professors. The number of publications per AHMF was noticeably low, with a mean of 6.3 and median of 0 (interquartile range, 0-4). The authors found that H-index, completion of chief residency, and graduation from a top 25 medical school were independently associated with promotion.
The authors only evaluated AHMF among the most academically rigorous AMCs, an approach that likely overestimates scholarly output of hospitalists across all US AMCs. Conversely, if we presume that promotion is more difficult at these major AMCs, the results may underestimate academic rank of AHMF nationally. Additionally, the authors did not distinguish faculty by tracks (eg, clinician-investigators, clinician-educators), which often have different criteria for academic promotion.
These findings are worrisomely consistent with prior reports, despite the tremendous expansion of the field.2-4 A 2008 survey of academic hospitalists found that 4% of respondents were full professors and 9% were associate professors, values nearly identical to the results in this current analysis,4 suggesting enduring barriers to academic advancement.
We are left with the following questions provoked by this body of literature: How can hospitalists increase their scholarly output and climb the promotional ladder? And how can we increase the academic footprint of hospital medicine? We recently proposed the following strategies based on a survey of academic groups participating in the Hospital Medicine Reengineering Network (HOMERuN) survey5: (1) expand hospital medicine research fellowships, which will provide graduates with research skills to justify dedicated time for research and aid their ability to obtain independent funding; (2) formalize mentorship between research faculty in hospital medicine and other internal medicine disciplines with robust track records for research; (3) invest in research infrastructure and data access within and between institutions; and (4) encourage hospital medicine group leaders to foster academic growth by incentivizing faculty to perform research, present their work at national conferences, and publish manuscripts with their findings.
Although an increase in scholarly output should contribute to higher academic rank, hospitalists routinely make other invaluable contributions beyond clinical care to AMCs, including medical education, hospital leadership, quality improvement, clinical innovation, and social justice advocacy. Also, hospitalists are increasingly disseminating their contributions via newer mediums (eg, social media, podcasts) that arguably have greater reach than traditional scholarship outlets. We believe that promotion committees should update their criteria to reflect the evolution of academic contribution and integrate these within traditional promotion pathways.
Finally, we must address federal funding mechanisms, which currently favor specialty-specific funding over funding that would be more applicable to hospital medicine researchers. Funding agencies are largely specialty- or disease-specific, with limited options for broader-based research.6 Additionally, grant-review committees are largely comprised of specialists, with few generalists and fewer hospitalists. These limitations make it difficult to “argue” the necessity of hospital medicine research. One concrete step would be for the National Institutes of Health (NIH) to create an Office for Hospital Medicine Research, analogous to the Office of Emergency Care Research, which works across NIH institutes and centers to foster research and research training for the emergency setting.
With these strategies, we are hopeful that hospital medicine will continue to expand its academic footprint and be recognized for its ever-growing contributions to the practice of medicine.
What has been the scholarly output of academic hospital medicine faculty (AHMF) and what academic rank have they achieved at US academic medical centers (AMCs)? Sumarsono et al1 address these questions and add to the growing body of literature exposing the limited academic footprint of hospitalists.
The authors performed a cross-sectional analysis of AHMF affiliated with the top 25 internal medicine training programs (as determined by the physician networking service doximity.com) and used Scopus to determine number of publications, citations, and H-index (a metric of productivity) for each faculty member. They also evaluated predictors for promotion. In contrast, most prior research on this topic relies on data obtained by survey methodology.2-5
Among 1554 AHMF from 22 AMCs, 42 (2.7%) were full professors and 140 (9.0%) were associate professors. The number of publications per AHMF was noticeably low, with a mean of 6.3 and median of 0 (interquartile range, 0-4). The authors found that H-index, completion of chief residency, and graduation from a top 25 medical school were independently associated with promotion.
The authors only evaluated AHMF among the most academically rigorous AMCs, an approach that likely overestimates scholarly output of hospitalists across all US AMCs. Conversely, if we presume that promotion is more difficult at these major AMCs, the results may underestimate academic rank of AHMF nationally. Additionally, the authors did not distinguish faculty by tracks (eg, clinician-investigators, clinician-educators), which often have different criteria for academic promotion.
These findings are worrisomely consistent with prior reports, despite the tremendous expansion of the field.2-4 A 2008 survey of academic hospitalists found that 4% of respondents were full professors and 9% were associate professors, values nearly identical to the results in this current analysis,4 suggesting enduring barriers to academic advancement.
We are left with the following questions provoked by this body of literature: How can hospitalists increase their scholarly output and climb the promotional ladder? And how can we increase the academic footprint of hospital medicine? We recently proposed the following strategies based on a survey of academic groups participating in the Hospital Medicine Reengineering Network (HOMERuN) survey5: (1) expand hospital medicine research fellowships, which will provide graduates with research skills to justify dedicated time for research and aid their ability to obtain independent funding; (2) formalize mentorship between research faculty in hospital medicine and other internal medicine disciplines with robust track records for research; (3) invest in research infrastructure and data access within and between institutions; and (4) encourage hospital medicine group leaders to foster academic growth by incentivizing faculty to perform research, present their work at national conferences, and publish manuscripts with their findings.
Although an increase in scholarly output should contribute to higher academic rank, hospitalists routinely make other invaluable contributions beyond clinical care to AMCs, including medical education, hospital leadership, quality improvement, clinical innovation, and social justice advocacy. Also, hospitalists are increasingly disseminating their contributions via newer mediums (eg, social media, podcasts) that arguably have greater reach than traditional scholarship outlets. We believe that promotion committees should update their criteria to reflect the evolution of academic contribution and integrate these within traditional promotion pathways.
Finally, we must address federal funding mechanisms, which currently favor specialty-specific funding over funding that would be more applicable to hospital medicine researchers. Funding agencies are largely specialty- or disease-specific, with limited options for broader-based research.6 Additionally, grant-review committees are largely comprised of specialists, with few generalists and fewer hospitalists. These limitations make it difficult to “argue” the necessity of hospital medicine research. One concrete step would be for the National Institutes of Health (NIH) to create an Office for Hospital Medicine Research, analogous to the Office of Emergency Care Research, which works across NIH institutes and centers to foster research and research training for the emergency setting.
With these strategies, we are hopeful that hospital medicine will continue to expand its academic footprint and be recognized for its ever-growing contributions to the practice of medicine.
1. Sumarsono A, Keshvani N, Saleh SN, et al. Scholarly productivity and rank in academic hospital medicine. J Hosp Med. 2021;16(9):545-548. https://doi.org/10.12788/jhm.3631
2. Chopra V, Burden M, Jones CD, et al. State of research in adult hospital medicine: results of a national survey. J Hosp Med. 2019;14(4):207-211. https://doi.org/10.12788/jhm.3136
3. Miller CS, Fogerty RL, Gann J, et al, the Society of General Internal Medicine Membership Committee. The growth of hospitalists and the future of the society of general internal medicine: results from the 2014 membership survey. J Gen Intern Med. 2017;32(11):1179-1185. https://doi.org/10.1007/s11606-017-4126-7
4. Reid MB, Misky GJ, Harrison RA, Sharpe B, Auerbach A, Glasheen JJ. Mentorship, productivity, and promotion among academic hospitalists. J Gen Intern Med. 2012;27(1):23-27. https://doi.org/10.1007/s11606-011-1892-5
5. Shannon EM, Chopra V, Greysen SR, et al. Dearth of hospitalist investigators in academic medicine: a call to action. J Hosp Med. 2021;16(3):189-191. https://doi.org/10.12788/jhm.3536
6. Levinson W, Linzer M. What is an academic general internist? Career options and training pathways. JAMA. 2002;288(16):2045-2048. https://doi.org/10.1001/jama.288.16.2045
1. Sumarsono A, Keshvani N, Saleh SN, et al. Scholarly productivity and rank in academic hospital medicine. J Hosp Med. 2021;16(9):545-548. https://doi.org/10.12788/jhm.3631
2. Chopra V, Burden M, Jones CD, et al. State of research in adult hospital medicine: results of a national survey. J Hosp Med. 2019;14(4):207-211. https://doi.org/10.12788/jhm.3136
3. Miller CS, Fogerty RL, Gann J, et al, the Society of General Internal Medicine Membership Committee. The growth of hospitalists and the future of the society of general internal medicine: results from the 2014 membership survey. J Gen Intern Med. 2017;32(11):1179-1185. https://doi.org/10.1007/s11606-017-4126-7
4. Reid MB, Misky GJ, Harrison RA, Sharpe B, Auerbach A, Glasheen JJ. Mentorship, productivity, and promotion among academic hospitalists. J Gen Intern Med. 2012;27(1):23-27. https://doi.org/10.1007/s11606-011-1892-5
5. Shannon EM, Chopra V, Greysen SR, et al. Dearth of hospitalist investigators in academic medicine: a call to action. J Hosp Med. 2021;16(3):189-191. https://doi.org/10.12788/jhm.3536
6. Levinson W, Linzer M. What is an academic general internist? Career options and training pathways. JAMA. 2002;288(16):2045-2048. https://doi.org/10.1001/jama.288.16.2045
© 2021 Society of Hospital Medicine
The Chronic Effects of COVID-19 Hospitalizations: Learning How Patients Can Get “Back to Normal”
As our understanding of SARS-CoV-2 has progressed, researchers, clinicians, and patients have learned that recovery from COVID-19 can last well beyond the acute phase of the illness. As we see fewer fatal cases and more survivors, studies that characterize the postacute sequelae of COVID-19 (PASC) are increasingly important for understanding how to help patients return to their normal lives, especially after hospitalization. Critical to investigating this is knowing patients’ burden of symptoms and disabilities prior to infection. In this issue, a study by Iwashyna et al1 helps us understand patients’ lives after COVID compared to their lives before COVID.
The study analyzed patients with SARS-CoV-2 infection admitted during the third wave of the pandemic to assess for new cardiopulmonary symptoms, new disability, and financial toxicity of hospitalization 1 month after discharge.1 Many patients had new cardiopulmonary symptoms and oxygen use, and a much larger number had new limitations in activities of daily living (ADLs) or instrumental activities of daily living (iADLs). The majority were discharged home without home care services, and new limitations in ADLs or iADLs were common in these cases. Most patients reported not having returned to their cardiopulmonary or functional baseline; however, new cough, shortness of breath, or oxygen use usually did not explain their new disabilities. Financial toxicity was also common, reflecting the effects of COVID-19 on both employment and family finances.
These results complement those of Chopra et al,2 who examined 60-day outcomes for patients hospitalized during the first wave of the pandemic. At 2 months from discharge, many patients had ongoing cough, shortness of breath, oxygen use, and disability, but at lower rates. This likely reflects continuing recovery during the extra 30 days, but other potential explanations deserve consideration. One possibility is improving survival over the course of the pandemic. Many patients who may have passed away earlier in the pandemic now survive to return home, albeit with a heavy burden of symptomatology. This raises the possibility that symptoms among survivors may continue to increase as survival of COVID-19 improves. However, it should be noted that neither study is representative of the national patterns of hospitalization by race or ethnicity.3 Iwashyna et al1 underrepresented Black patients, while Chopra et al2 underrepresented Hispanic patients. Given what we know about outcomes for these populations and their underrepresentation in PASC literature, the impact of COVID-19 for them is likely underestimated. As data from 3, 6, or 12 months become available, we may also see the effect sizes described in this early literature become even larger.
Consistent with the findings of Chopra et al,2 financial toxicity after COVID-19 hospitalization was high. The longer-term financial burden of COVID-19 will likely exceed what is described here, particularly for Black and Hispanic patients, who experienced a disproportionate drain on their savings. These populations are also more likely to be negatively impacted by the COVID economy4 and thus may suffer a “double hit” financially if hospitalized.
Iwashyna et al1 underscore the urgent need for progress in understanding COVID “long-haulers”5 and helping patients with physical and financial recovery. Whether the spectacular innovations identified by the medical community in COVID-19 prevention and treatment of acute illness can be found for long COVID remains to be seen. The fact that so many patients studied by Iwashyna et al did not receive home care services and experienced financial toxicity shows the importance of broader implementation of systems and services to support survivors of COVID-19 hospitalization. Developers of this support must emphasize the importance of physical and cardiopulmonary rehabilitation as well as financial relief, particularly for minorities. For our patients and their families, this may be the best strategy to get “back to normal.”
Acknowledgment
The authors thank Dr Vineet Arora for reviewing and advising on this manuscript.
1. Iwashyna TJ, Kamphuis LA, Gundel SJ, et al. Continuing cardiopulmonary symptoms, disability, and financial toxicity 1 month after hospitalization for third-wave COVID-19: early results from a US nationwide cohort. J Hosp Med. 2021;16(9):531-537. https://doi.org/10.12788/jhm.3660
2. Chopra V, Flanders SA, O’Malley M, Malani AN, Prescott HC. Sixty-day outcomes among patients hospitalized with COVID-19. Ann Intern Med. 2021;174(4):576-578. https://doi.org/10.7326/M20-5661
3. Centers for Disease Control and Prevention. Risk for COVID-19 infection, hospitalization, and death by race/ethnicity. Updated July 16, 2021. Accessed August 19, 2021. https://www.cdc.gov/coronavirus/2019-ncov/covid-data/investigations-discovery/hospitalization-death-by-race-ethnicity.html
4. Robert Wood Johnson Foundation, NPR, Harvard T.H. Chan School of Public Health. The impact of coronavirus on households by race/ethnicity. September 2020. Accessed July 28, 2021. https://www.rwjf.org/en/library/research/2020/09/the-impact-of-coronavirus-on-households-across-america.html
5. Barber C. The problem of ‘long haul’ COVID. December 29, 2020. Accessed July 28, 2021. https://www.scientificamerican.com/article/the-problem-of-long-haul-covid/
As our understanding of SARS-CoV-2 has progressed, researchers, clinicians, and patients have learned that recovery from COVID-19 can last well beyond the acute phase of the illness. As we see fewer fatal cases and more survivors, studies that characterize the postacute sequelae of COVID-19 (PASC) are increasingly important for understanding how to help patients return to their normal lives, especially after hospitalization. Critical to investigating this is knowing patients’ burden of symptoms and disabilities prior to infection. In this issue, a study by Iwashyna et al1 helps us understand patients’ lives after COVID compared to their lives before COVID.
The study analyzed patients with SARS-CoV-2 infection admitted during the third wave of the pandemic to assess for new cardiopulmonary symptoms, new disability, and financial toxicity of hospitalization 1 month after discharge.1 Many patients had new cardiopulmonary symptoms and oxygen use, and a much larger number had new limitations in activities of daily living (ADLs) or instrumental activities of daily living (iADLs). The majority were discharged home without home care services, and new limitations in ADLs or iADLs were common in these cases. Most patients reported not having returned to their cardiopulmonary or functional baseline; however, new cough, shortness of breath, or oxygen use usually did not explain their new disabilities. Financial toxicity was also common, reflecting the effects of COVID-19 on both employment and family finances.
These results complement those of Chopra et al,2 who examined 60-day outcomes for patients hospitalized during the first wave of the pandemic. At 2 months from discharge, many patients had ongoing cough, shortness of breath, oxygen use, and disability, but at lower rates. This likely reflects continuing recovery during the extra 30 days, but other potential explanations deserve consideration. One possibility is improving survival over the course of the pandemic. Many patients who may have passed away earlier in the pandemic now survive to return home, albeit with a heavy burden of symptomatology. This raises the possibility that symptoms among survivors may continue to increase as survival of COVID-19 improves. However, it should be noted that neither study is representative of the national patterns of hospitalization by race or ethnicity.3 Iwashyna et al1 underrepresented Black patients, while Chopra et al2 underrepresented Hispanic patients. Given what we know about outcomes for these populations and their underrepresentation in PASC literature, the impact of COVID-19 for them is likely underestimated. As data from 3, 6, or 12 months become available, we may also see the effect sizes described in this early literature become even larger.
Consistent with the findings of Chopra et al,2 financial toxicity after COVID-19 hospitalization was high. The longer-term financial burden of COVID-19 will likely exceed what is described here, particularly for Black and Hispanic patients, who experienced a disproportionate drain on their savings. These populations are also more likely to be negatively impacted by the COVID economy4 and thus may suffer a “double hit” financially if hospitalized.
Iwashyna et al1 underscore the urgent need for progress in understanding COVID “long-haulers”5 and helping patients with physical and financial recovery. Whether the spectacular innovations identified by the medical community in COVID-19 prevention and treatment of acute illness can be found for long COVID remains to be seen. The fact that so many patients studied by Iwashyna et al did not receive home care services and experienced financial toxicity shows the importance of broader implementation of systems and services to support survivors of COVID-19 hospitalization. Developers of this support must emphasize the importance of physical and cardiopulmonary rehabilitation as well as financial relief, particularly for minorities. For our patients and their families, this may be the best strategy to get “back to normal.”
Acknowledgment
The authors thank Dr Vineet Arora for reviewing and advising on this manuscript.
As our understanding of SARS-CoV-2 has progressed, researchers, clinicians, and patients have learned that recovery from COVID-19 can last well beyond the acute phase of the illness. As we see fewer fatal cases and more survivors, studies that characterize the postacute sequelae of COVID-19 (PASC) are increasingly important for understanding how to help patients return to their normal lives, especially after hospitalization. Critical to investigating this is knowing patients’ burden of symptoms and disabilities prior to infection. In this issue, a study by Iwashyna et al1 helps us understand patients’ lives after COVID compared to their lives before COVID.
The study analyzed patients with SARS-CoV-2 infection admitted during the third wave of the pandemic to assess for new cardiopulmonary symptoms, new disability, and financial toxicity of hospitalization 1 month after discharge.1 Many patients had new cardiopulmonary symptoms and oxygen use, and a much larger number had new limitations in activities of daily living (ADLs) or instrumental activities of daily living (iADLs). The majority were discharged home without home care services, and new limitations in ADLs or iADLs were common in these cases. Most patients reported not having returned to their cardiopulmonary or functional baseline; however, new cough, shortness of breath, or oxygen use usually did not explain their new disabilities. Financial toxicity was also common, reflecting the effects of COVID-19 on both employment and family finances.
These results complement those of Chopra et al,2 who examined 60-day outcomes for patients hospitalized during the first wave of the pandemic. At 2 months from discharge, many patients had ongoing cough, shortness of breath, oxygen use, and disability, but at lower rates. This likely reflects continuing recovery during the extra 30 days, but other potential explanations deserve consideration. One possibility is improving survival over the course of the pandemic. Many patients who may have passed away earlier in the pandemic now survive to return home, albeit with a heavy burden of symptomatology. This raises the possibility that symptoms among survivors may continue to increase as survival of COVID-19 improves. However, it should be noted that neither study is representative of the national patterns of hospitalization by race or ethnicity.3 Iwashyna et al1 underrepresented Black patients, while Chopra et al2 underrepresented Hispanic patients. Given what we know about outcomes for these populations and their underrepresentation in PASC literature, the impact of COVID-19 for them is likely underestimated. As data from 3, 6, or 12 months become available, we may also see the effect sizes described in this early literature become even larger.
Consistent with the findings of Chopra et al,2 financial toxicity after COVID-19 hospitalization was high. The longer-term financial burden of COVID-19 will likely exceed what is described here, particularly for Black and Hispanic patients, who experienced a disproportionate drain on their savings. These populations are also more likely to be negatively impacted by the COVID economy4 and thus may suffer a “double hit” financially if hospitalized.
Iwashyna et al1 underscore the urgent need for progress in understanding COVID “long-haulers”5 and helping patients with physical and financial recovery. Whether the spectacular innovations identified by the medical community in COVID-19 prevention and treatment of acute illness can be found for long COVID remains to be seen. The fact that so many patients studied by Iwashyna et al did not receive home care services and experienced financial toxicity shows the importance of broader implementation of systems and services to support survivors of COVID-19 hospitalization. Developers of this support must emphasize the importance of physical and cardiopulmonary rehabilitation as well as financial relief, particularly for minorities. For our patients and their families, this may be the best strategy to get “back to normal.”
Acknowledgment
The authors thank Dr Vineet Arora for reviewing and advising on this manuscript.
1. Iwashyna TJ, Kamphuis LA, Gundel SJ, et al. Continuing cardiopulmonary symptoms, disability, and financial toxicity 1 month after hospitalization for third-wave COVID-19: early results from a US nationwide cohort. J Hosp Med. 2021;16(9):531-537. https://doi.org/10.12788/jhm.3660
2. Chopra V, Flanders SA, O’Malley M, Malani AN, Prescott HC. Sixty-day outcomes among patients hospitalized with COVID-19. Ann Intern Med. 2021;174(4):576-578. https://doi.org/10.7326/M20-5661
3. Centers for Disease Control and Prevention. Risk for COVID-19 infection, hospitalization, and death by race/ethnicity. Updated July 16, 2021. Accessed August 19, 2021. https://www.cdc.gov/coronavirus/2019-ncov/covid-data/investigations-discovery/hospitalization-death-by-race-ethnicity.html
4. Robert Wood Johnson Foundation, NPR, Harvard T.H. Chan School of Public Health. The impact of coronavirus on households by race/ethnicity. September 2020. Accessed July 28, 2021. https://www.rwjf.org/en/library/research/2020/09/the-impact-of-coronavirus-on-households-across-america.html
5. Barber C. The problem of ‘long haul’ COVID. December 29, 2020. Accessed July 28, 2021. https://www.scientificamerican.com/article/the-problem-of-long-haul-covid/
1. Iwashyna TJ, Kamphuis LA, Gundel SJ, et al. Continuing cardiopulmonary symptoms, disability, and financial toxicity 1 month after hospitalization for third-wave COVID-19: early results from a US nationwide cohort. J Hosp Med. 2021;16(9):531-537. https://doi.org/10.12788/jhm.3660
2. Chopra V, Flanders SA, O’Malley M, Malani AN, Prescott HC. Sixty-day outcomes among patients hospitalized with COVID-19. Ann Intern Med. 2021;174(4):576-578. https://doi.org/10.7326/M20-5661
3. Centers for Disease Control and Prevention. Risk for COVID-19 infection, hospitalization, and death by race/ethnicity. Updated July 16, 2021. Accessed August 19, 2021. https://www.cdc.gov/coronavirus/2019-ncov/covid-data/investigations-discovery/hospitalization-death-by-race-ethnicity.html
4. Robert Wood Johnson Foundation, NPR, Harvard T.H. Chan School of Public Health. The impact of coronavirus on households by race/ethnicity. September 2020. Accessed July 28, 2021. https://www.rwjf.org/en/library/research/2020/09/the-impact-of-coronavirus-on-households-across-america.html
5. Barber C. The problem of ‘long haul’ COVID. December 29, 2020. Accessed July 28, 2021. https://www.scientificamerican.com/article/the-problem-of-long-haul-covid/
© 2021 Society of Hospital Medicine
Leveraging the Care Team to Optimize Disposition Planning
Is this patient a good candidate? In medicine, we subconsciously answer this question for every clinical decision we make. Occasionally, though, a clinical scenario is so complex that it cannot or should not be answered by a single individual. One example is the decision on whether a patient should receive an organ transplant. In this situation, a multidisciplinary committee weighs the complex ethical, clinical, and financial implications of the decision before coming to a verdict. Together, team members discuss the risks and benefits of each patient’s candidacy and, in a united fashion, decide the best course of care. For hospitalists, a far more common question occurs every day and is similarly fraught with multifaceted implications: Is my patient a good candidate for a skilled nursing facility (SNF)? We often rely on a single individual to make the final call, but should we instead be leveraging the expertise of other care team members to assist with this decision?
In this issue, Boyle et al1 describe the implementation of a multidisciplinary team consisting of physicians, case managers, social workers, physical and occupational therapists, and home-health representatives that reviewed all patients with an expected discharge to a SNF. Case managers or social workers began the process by referring eligible patients to the committee for review. If deemed appropriate, the committee discussed each case and reached a consensus recommendation as to whether a SNF was an appropriate discharge destination. The investigators used a matched, preintervention sample as a comparison group, with a primary outcome of total discharges to SNFs, and secondary outcomes consisting of readmissions, time to readmission, and median length of stay. The authors observed a 49.7% relative reduction in total SNF discharges (25.5% of preintervention patients discharged to a SNF vs 12.8% postintervention), as well as a 66.9% relative reduction in new SNF discharges. Despite the significant reduction in SNF utilization, no differences were noted in readmissions, time to readmission, or readmission length of stay.
While this study was performed during the COVID-19 pandemic, several characteristics make its findings applicable beyond this period. First, the structure and workflow of the team are extensively detailed and make the intervention easily generalizable to most hospitals. Second, while not specifically examined, the outcome of SNF reduction likely corresponds to an increase in the patient’s time at home—an important patient-centered target for most posthospitalization plans.2 Finally, the intervention used existing infrastructure and individuals, and did not require new resources to improve patient care, which increases the feasibility of implementation at other institutions.
These findings also reveal potential overutilization of SNFs in the discharge process. On average, a typical SNF stay costs the health system more than $11,000.3 A simple intervention could lead to substantial savings for individuals and the healthcare system. With a nearly 50% reduction in SNF use, understanding why patients who were eligible to go home were ultimately discharged to a SNF will be a crucial question to answer. Are there barriers to patient or family education? Is there a perceived safety difference between a SNF and home for nonskilled nursing needs? Additionally, care should be taken to ensure that decreases in SNF utilization do not disproportionately affect certain populations. Further work should assess the performance of similar models in a non-COVID era and among multiple institutions to verify potential scalability and generalizability.
Like organ transplant committees, Boyle et al’s multidisciplinary approach to reduce SNF discharges had to include thoughtful and intentional decisions. Perhaps it is time we use this same model to transplant patients back into their homes as safely and efficiently as possible.
1. Boyle CA, Ravichandran U, Hankamp V, et al. Safe transitions and congregate living in the age of COVID-19: a retrospective cohort study. J Hosp Med. 2021;16(9):524-530. https://doi.org/10.12788/jhm.3657
2. Barnett ML, Grabowski DC, Mehrotra A. Home-to-home time—measuring what matters to patients and payers. N Engl J Med. 2017;377(1):4-6. https://doi.org/10.1056/NEJMp1703423
3. Werner RM, Coe NB, Qi M, Konetzka RT. Patient outcomes after hospital discharge to home with home health care vs to a skilled nursing facility. JAMA Intern Med. 2019;179(5):617-623. https://doi.org/10.1001/jamainternmed.2018.7998
Is this patient a good candidate? In medicine, we subconsciously answer this question for every clinical decision we make. Occasionally, though, a clinical scenario is so complex that it cannot or should not be answered by a single individual. One example is the decision on whether a patient should receive an organ transplant. In this situation, a multidisciplinary committee weighs the complex ethical, clinical, and financial implications of the decision before coming to a verdict. Together, team members discuss the risks and benefits of each patient’s candidacy and, in a united fashion, decide the best course of care. For hospitalists, a far more common question occurs every day and is similarly fraught with multifaceted implications: Is my patient a good candidate for a skilled nursing facility (SNF)? We often rely on a single individual to make the final call, but should we instead be leveraging the expertise of other care team members to assist with this decision?
In this issue, Boyle et al1 describe the implementation of a multidisciplinary team consisting of physicians, case managers, social workers, physical and occupational therapists, and home-health representatives that reviewed all patients with an expected discharge to a SNF. Case managers or social workers began the process by referring eligible patients to the committee for review. If deemed appropriate, the committee discussed each case and reached a consensus recommendation as to whether a SNF was an appropriate discharge destination. The investigators used a matched, preintervention sample as a comparison group, with a primary outcome of total discharges to SNFs, and secondary outcomes consisting of readmissions, time to readmission, and median length of stay. The authors observed a 49.7% relative reduction in total SNF discharges (25.5% of preintervention patients discharged to a SNF vs 12.8% postintervention), as well as a 66.9% relative reduction in new SNF discharges. Despite the significant reduction in SNF utilization, no differences were noted in readmissions, time to readmission, or readmission length of stay.
While this study was performed during the COVID-19 pandemic, several characteristics make its findings applicable beyond this period. First, the structure and workflow of the team are extensively detailed and make the intervention easily generalizable to most hospitals. Second, while not specifically examined, the outcome of SNF reduction likely corresponds to an increase in the patient’s time at home—an important patient-centered target for most posthospitalization plans.2 Finally, the intervention used existing infrastructure and individuals, and did not require new resources to improve patient care, which increases the feasibility of implementation at other institutions.
These findings also reveal potential overutilization of SNFs in the discharge process. On average, a typical SNF stay costs the health system more than $11,000.3 A simple intervention could lead to substantial savings for individuals and the healthcare system. With a nearly 50% reduction in SNF use, understanding why patients who were eligible to go home were ultimately discharged to a SNF will be a crucial question to answer. Are there barriers to patient or family education? Is there a perceived safety difference between a SNF and home for nonskilled nursing needs? Additionally, care should be taken to ensure that decreases in SNF utilization do not disproportionately affect certain populations. Further work should assess the performance of similar models in a non-COVID era and among multiple institutions to verify potential scalability and generalizability.
Like organ transplant committees, Boyle et al’s multidisciplinary approach to reduce SNF discharges had to include thoughtful and intentional decisions. Perhaps it is time we use this same model to transplant patients back into their homes as safely and efficiently as possible.
Is this patient a good candidate? In medicine, we subconsciously answer this question for every clinical decision we make. Occasionally, though, a clinical scenario is so complex that it cannot or should not be answered by a single individual. One example is the decision on whether a patient should receive an organ transplant. In this situation, a multidisciplinary committee weighs the complex ethical, clinical, and financial implications of the decision before coming to a verdict. Together, team members discuss the risks and benefits of each patient’s candidacy and, in a united fashion, decide the best course of care. For hospitalists, a far more common question occurs every day and is similarly fraught with multifaceted implications: Is my patient a good candidate for a skilled nursing facility (SNF)? We often rely on a single individual to make the final call, but should we instead be leveraging the expertise of other care team members to assist with this decision?
In this issue, Boyle et al1 describe the implementation of a multidisciplinary team consisting of physicians, case managers, social workers, physical and occupational therapists, and home-health representatives that reviewed all patients with an expected discharge to a SNF. Case managers or social workers began the process by referring eligible patients to the committee for review. If deemed appropriate, the committee discussed each case and reached a consensus recommendation as to whether a SNF was an appropriate discharge destination. The investigators used a matched, preintervention sample as a comparison group, with a primary outcome of total discharges to SNFs, and secondary outcomes consisting of readmissions, time to readmission, and median length of stay. The authors observed a 49.7% relative reduction in total SNF discharges (25.5% of preintervention patients discharged to a SNF vs 12.8% postintervention), as well as a 66.9% relative reduction in new SNF discharges. Despite the significant reduction in SNF utilization, no differences were noted in readmissions, time to readmission, or readmission length of stay.
While this study was performed during the COVID-19 pandemic, several characteristics make its findings applicable beyond this period. First, the structure and workflow of the team are extensively detailed and make the intervention easily generalizable to most hospitals. Second, while not specifically examined, the outcome of SNF reduction likely corresponds to an increase in the patient’s time at home—an important patient-centered target for most posthospitalization plans.2 Finally, the intervention used existing infrastructure and individuals, and did not require new resources to improve patient care, which increases the feasibility of implementation at other institutions.
These findings also reveal potential overutilization of SNFs in the discharge process. On average, a typical SNF stay costs the health system more than $11,000.3 A simple intervention could lead to substantial savings for individuals and the healthcare system. With a nearly 50% reduction in SNF use, understanding why patients who were eligible to go home were ultimately discharged to a SNF will be a crucial question to answer. Are there barriers to patient or family education? Is there a perceived safety difference between a SNF and home for nonskilled nursing needs? Additionally, care should be taken to ensure that decreases in SNF utilization do not disproportionately affect certain populations. Further work should assess the performance of similar models in a non-COVID era and among multiple institutions to verify potential scalability and generalizability.
Like organ transplant committees, Boyle et al’s multidisciplinary approach to reduce SNF discharges had to include thoughtful and intentional decisions. Perhaps it is time we use this same model to transplant patients back into their homes as safely and efficiently as possible.
1. Boyle CA, Ravichandran U, Hankamp V, et al. Safe transitions and congregate living in the age of COVID-19: a retrospective cohort study. J Hosp Med. 2021;16(9):524-530. https://doi.org/10.12788/jhm.3657
2. Barnett ML, Grabowski DC, Mehrotra A. Home-to-home time—measuring what matters to patients and payers. N Engl J Med. 2017;377(1):4-6. https://doi.org/10.1056/NEJMp1703423
3. Werner RM, Coe NB, Qi M, Konetzka RT. Patient outcomes after hospital discharge to home with home health care vs to a skilled nursing facility. JAMA Intern Med. 2019;179(5):617-623. https://doi.org/10.1001/jamainternmed.2018.7998
1. Boyle CA, Ravichandran U, Hankamp V, et al. Safe transitions and congregate living in the age of COVID-19: a retrospective cohort study. J Hosp Med. 2021;16(9):524-530. https://doi.org/10.12788/jhm.3657
2. Barnett ML, Grabowski DC, Mehrotra A. Home-to-home time—measuring what matters to patients and payers. N Engl J Med. 2017;377(1):4-6. https://doi.org/10.1056/NEJMp1703423
3. Werner RM, Coe NB, Qi M, Konetzka RT. Patient outcomes after hospital discharge to home with home health care vs to a skilled nursing facility. JAMA Intern Med. 2019;179(5):617-623. https://doi.org/10.1001/jamainternmed.2018.7998
© 2021 Society of Hospital Medicine
A More Intentional Analysis of Race and Racism in Research
Earlier this year, the Journal of Hospital Medicine updated its author guidelines to include recommendations on addressing race and racism.1 These recommendations include explicitly naming racism (rather than race) as a determinant of health. Operationalizing these recommendations into manuscripts represents a fundamental shift in how we ask research questions, structure analyses, and interpret results.
In this issue, Maxwell et al2 illustrate how to disseminate research through this lens in their retrospective cohort study of children with type 1 diabetes hospitalized with diabetic ketoacidosis (DKA). Using 6 years of data from a major academic pediatric medical center, the authors examine the association between risk for DKA admission and three factors: neighborhood poverty level, race, and type of insurance (public or private). Secondary outcomes include DKA severity and length of stay. In their unadjusted model, poverty, race, and insurance were all associated with increased hospitalizations. However, following adjustment, the association between race and hospitalizations disappeared.In line with the journal’s new guidelines, the authors point out that the statistically significant associations of poverty and insurance type with clinical outcomes suggest that racism, rather than race, is a social factor at work in their population. The authors provide further context regarding structural racism in the United States and the history of redlining, which has helped shape a society in which Black individuals are more likely to live in areas of concentrated poverty and be publicly insured.
Two other findings related to the impact of racism are notable. First, in both their univariate and multivariate models, the authors found significant A1c differences between Black and White children—higher than those of previous reports.3 These findings suggest the existence of structural factors at work in the health of their patients. Second, Black patients had longer lengths of stay when compared to White patients with the same severity of DKA. Neither poverty level nor insurance status were significantly associated with length of stay. While the analysis was limited to detecting this difference, rather than identifying its causes, the authors suggest factors at both individual and structural levels that may be impacting outcomes. Specifically, care team bias may impact discharge decisions, and factors such as less flexible times to complete diabetes education, transportation barriers, and childcare challenges could also impact discharge timing.
This work provides a template for how to address the impact of racism on health with intentionality. Moreover, individuals’ lived environments should be considered through alternative economic measurements and neighborhood definitions. The proportion of people within a census tract living below the federal poverty line is just one measure of the complex dynamics that contribute to an individual’s socioeconomic status. An alternative measure is the area deprivation index, which incorporates 17 indicators at the more granular census block group level to describe an individual’s environment4 and could be useful in this area of research.
Perhaps most relevant is the use of public insurance as a marker of socioeconomic status. Medicaid, although not without its flaws, provides fairly comprehensive coverage. However, many Americans have incomes too high to qualify for public insurance but too low to afford adequate insurance coverage. Theoretically, these individuals qualify for subsidies through the Affordable Care Act, yet underinsurance remains a significant issue.5 Future analyses to further understand and describe clinical outcomes could include this population of underinsured children as a distinct at-risk group. Maxwell et al2 provide an excellent example of how we should address race and racism in disseminated literature. Although initially challenging, writing with intentionality regarding this fundamental determinant of health can provide rich and actionable information for practitioners and policy-makers.
1. Andrews AL, Unaka N, Shah SS. New author guidelines for addressing race and racism in the Journal of Hospital Medicine. J Hosp Med. 2021;16(4):197. https://doi.org/10.12788/jhm.3598
2. Maxwell AR, Jones NHY, Taylor S, et al. Socioeconomic and racial disparities in diabetic ketoacidosis admissions in youth with type 1 diabetes. J Hosp Med. 2021;16(9):517-523. https://doi.org/10.12788/jhm.3664
3. Bergenstal RM, Gal RL, Connor CG, et al. Racial differences in the relationship of glucose concentrations and hemoglobin A1c levels. Ann Intern Med. 2017;167(2):95-102. https://doi.org/10.7326/M16-2596
4. Kind AJH, Jencks S, Brock J, et al. Neighborhood socioeconomic disadvantage and 30 day rehospitalization: a retrospective cohort study. Ann Intern Med. 2014(11);161:765-774. https://doi.org/10.7326/M13-2946
5. Strane D, Rosenquist R, Rubin D. Leveraging health care reform to address underinsurance in working families. Health Affairs. June 15, 2021. Accessed August 23, 2021. www.healthaffairs.org/do/10.1377/hblog20210611.153918/full/
Earlier this year, the Journal of Hospital Medicine updated its author guidelines to include recommendations on addressing race and racism.1 These recommendations include explicitly naming racism (rather than race) as a determinant of health. Operationalizing these recommendations into manuscripts represents a fundamental shift in how we ask research questions, structure analyses, and interpret results.
In this issue, Maxwell et al2 illustrate how to disseminate research through this lens in their retrospective cohort study of children with type 1 diabetes hospitalized with diabetic ketoacidosis (DKA). Using 6 years of data from a major academic pediatric medical center, the authors examine the association between risk for DKA admission and three factors: neighborhood poverty level, race, and type of insurance (public or private). Secondary outcomes include DKA severity and length of stay. In their unadjusted model, poverty, race, and insurance were all associated with increased hospitalizations. However, following adjustment, the association between race and hospitalizations disappeared.In line with the journal’s new guidelines, the authors point out that the statistically significant associations of poverty and insurance type with clinical outcomes suggest that racism, rather than race, is a social factor at work in their population. The authors provide further context regarding structural racism in the United States and the history of redlining, which has helped shape a society in which Black individuals are more likely to live in areas of concentrated poverty and be publicly insured.
Two other findings related to the impact of racism are notable. First, in both their univariate and multivariate models, the authors found significant A1c differences between Black and White children—higher than those of previous reports.3 These findings suggest the existence of structural factors at work in the health of their patients. Second, Black patients had longer lengths of stay when compared to White patients with the same severity of DKA. Neither poverty level nor insurance status were significantly associated with length of stay. While the analysis was limited to detecting this difference, rather than identifying its causes, the authors suggest factors at both individual and structural levels that may be impacting outcomes. Specifically, care team bias may impact discharge decisions, and factors such as less flexible times to complete diabetes education, transportation barriers, and childcare challenges could also impact discharge timing.
This work provides a template for how to address the impact of racism on health with intentionality. Moreover, individuals’ lived environments should be considered through alternative economic measurements and neighborhood definitions. The proportion of people within a census tract living below the federal poverty line is just one measure of the complex dynamics that contribute to an individual’s socioeconomic status. An alternative measure is the area deprivation index, which incorporates 17 indicators at the more granular census block group level to describe an individual’s environment4 and could be useful in this area of research.
Perhaps most relevant is the use of public insurance as a marker of socioeconomic status. Medicaid, although not without its flaws, provides fairly comprehensive coverage. However, many Americans have incomes too high to qualify for public insurance but too low to afford adequate insurance coverage. Theoretically, these individuals qualify for subsidies through the Affordable Care Act, yet underinsurance remains a significant issue.5 Future analyses to further understand and describe clinical outcomes could include this population of underinsured children as a distinct at-risk group. Maxwell et al2 provide an excellent example of how we should address race and racism in disseminated literature. Although initially challenging, writing with intentionality regarding this fundamental determinant of health can provide rich and actionable information for practitioners and policy-makers.
Earlier this year, the Journal of Hospital Medicine updated its author guidelines to include recommendations on addressing race and racism.1 These recommendations include explicitly naming racism (rather than race) as a determinant of health. Operationalizing these recommendations into manuscripts represents a fundamental shift in how we ask research questions, structure analyses, and interpret results.
In this issue, Maxwell et al2 illustrate how to disseminate research through this lens in their retrospective cohort study of children with type 1 diabetes hospitalized with diabetic ketoacidosis (DKA). Using 6 years of data from a major academic pediatric medical center, the authors examine the association between risk for DKA admission and three factors: neighborhood poverty level, race, and type of insurance (public or private). Secondary outcomes include DKA severity and length of stay. In their unadjusted model, poverty, race, and insurance were all associated with increased hospitalizations. However, following adjustment, the association between race and hospitalizations disappeared.In line with the journal’s new guidelines, the authors point out that the statistically significant associations of poverty and insurance type with clinical outcomes suggest that racism, rather than race, is a social factor at work in their population. The authors provide further context regarding structural racism in the United States and the history of redlining, which has helped shape a society in which Black individuals are more likely to live in areas of concentrated poverty and be publicly insured.
Two other findings related to the impact of racism are notable. First, in both their univariate and multivariate models, the authors found significant A1c differences between Black and White children—higher than those of previous reports.3 These findings suggest the existence of structural factors at work in the health of their patients. Second, Black patients had longer lengths of stay when compared to White patients with the same severity of DKA. Neither poverty level nor insurance status were significantly associated with length of stay. While the analysis was limited to detecting this difference, rather than identifying its causes, the authors suggest factors at both individual and structural levels that may be impacting outcomes. Specifically, care team bias may impact discharge decisions, and factors such as less flexible times to complete diabetes education, transportation barriers, and childcare challenges could also impact discharge timing.
This work provides a template for how to address the impact of racism on health with intentionality. Moreover, individuals’ lived environments should be considered through alternative economic measurements and neighborhood definitions. The proportion of people within a census tract living below the federal poverty line is just one measure of the complex dynamics that contribute to an individual’s socioeconomic status. An alternative measure is the area deprivation index, which incorporates 17 indicators at the more granular census block group level to describe an individual’s environment4 and could be useful in this area of research.
Perhaps most relevant is the use of public insurance as a marker of socioeconomic status. Medicaid, although not without its flaws, provides fairly comprehensive coverage. However, many Americans have incomes too high to qualify for public insurance but too low to afford adequate insurance coverage. Theoretically, these individuals qualify for subsidies through the Affordable Care Act, yet underinsurance remains a significant issue.5 Future analyses to further understand and describe clinical outcomes could include this population of underinsured children as a distinct at-risk group. Maxwell et al2 provide an excellent example of how we should address race and racism in disseminated literature. Although initially challenging, writing with intentionality regarding this fundamental determinant of health can provide rich and actionable information for practitioners and policy-makers.
1. Andrews AL, Unaka N, Shah SS. New author guidelines for addressing race and racism in the Journal of Hospital Medicine. J Hosp Med. 2021;16(4):197. https://doi.org/10.12788/jhm.3598
2. Maxwell AR, Jones NHY, Taylor S, et al. Socioeconomic and racial disparities in diabetic ketoacidosis admissions in youth with type 1 diabetes. J Hosp Med. 2021;16(9):517-523. https://doi.org/10.12788/jhm.3664
3. Bergenstal RM, Gal RL, Connor CG, et al. Racial differences in the relationship of glucose concentrations and hemoglobin A1c levels. Ann Intern Med. 2017;167(2):95-102. https://doi.org/10.7326/M16-2596
4. Kind AJH, Jencks S, Brock J, et al. Neighborhood socioeconomic disadvantage and 30 day rehospitalization: a retrospective cohort study. Ann Intern Med. 2014(11);161:765-774. https://doi.org/10.7326/M13-2946
5. Strane D, Rosenquist R, Rubin D. Leveraging health care reform to address underinsurance in working families. Health Affairs. June 15, 2021. Accessed August 23, 2021. www.healthaffairs.org/do/10.1377/hblog20210611.153918/full/
1. Andrews AL, Unaka N, Shah SS. New author guidelines for addressing race and racism in the Journal of Hospital Medicine. J Hosp Med. 2021;16(4):197. https://doi.org/10.12788/jhm.3598
2. Maxwell AR, Jones NHY, Taylor S, et al. Socioeconomic and racial disparities in diabetic ketoacidosis admissions in youth with type 1 diabetes. J Hosp Med. 2021;16(9):517-523. https://doi.org/10.12788/jhm.3664
3. Bergenstal RM, Gal RL, Connor CG, et al. Racial differences in the relationship of glucose concentrations and hemoglobin A1c levels. Ann Intern Med. 2017;167(2):95-102. https://doi.org/10.7326/M16-2596
4. Kind AJH, Jencks S, Brock J, et al. Neighborhood socioeconomic disadvantage and 30 day rehospitalization: a retrospective cohort study. Ann Intern Med. 2014(11);161:765-774. https://doi.org/10.7326/M13-2946
5. Strane D, Rosenquist R, Rubin D. Leveraging health care reform to address underinsurance in working families. Health Affairs. June 15, 2021. Accessed August 23, 2021. www.healthaffairs.org/do/10.1377/hblog20210611.153918/full/
© 2021 Society of Hospital Medicine
Safe Transitions and Congregate Living in the Age of COVID-19: A Retrospective Cohort Study
The COVID-19 outbreak in February 2020 at a congregate living facility near Seattle, Washington, signaled the beginning of the pandemic in the United States. In that facility, infected residents had a 54.5% hospitalization rate and 33.7% case-fatality rate.1 Similar to the experience in Washington, all congregate living facilities have proved particularly vulnerable to the effects of COVID-19,2-7 with residents at increased risk for disease severity and mortality.2-7
Due to the COVID-19 emergency, NorthShore University HealthSystem (NUHS), a multihospital, integrated health system in northern Illinois, established a best practice for appropriate use of congregate living facilities after hospitalization. This focused on the safety of discharged patients and mitigation of COVID-19 by putting in place a referral process to a newly established congregate living review committee (CLRC) for review prior to discharge. Although all discharges to congregate living settings are at high risk,2 new placements to skilled nursing facilities (SNFs) were the primary focus of the committee and the sole focus of this study. In this study, we sought to determine whether establishment of the CLRC was associated with a reduction in SNF utilization, whether this was safe and efficient, and whether it was associated with a reduction in COVID-19 incidence in the 30 days following discharge.
METHODS
Setting and Case Review Intervention
We conducted a retrospective cohort study for patients hospitalized within NUHS from March 19, 2019 to July 16, 2020, designed as an interrupted time series. The study was approved by the NUHS Institutional Review Board (EH21-022).
The study exposure was creation of a referral and review process for all patients with expected discharge to a SNF and was implemented as part of usual discharge planning during the COVID-19 pandemic. The key intervention was to establish a multidisciplinary committee, the CLRC, to review all potential discharges to SNFs. The CLRC had dual goals of preventing COVID-19 spread in facilities by limiting placement of new residents and protecting a vulnerable population from a setting that conferred a higher risk of acquiring COVID-19. The CLRC was organized as a multidisciplinary committee with physicians, case managers, social workers, physical therapists, occupational therapists, and the director of NUHS home health agency. Physician members were evenly split as half hospitalists and half ambulatory physicians. The CLRC review was initiated by a patient’s assigned case manager or social worker by consult through a referral in the electronic medical record (EMR). Each case was summarized and then presented to the full CLRC. The CLRC met for 1 hour per day, 6 days per week, to review all planned discharges that met criteria for review. A committee physician chaired each meeting. Three other members were needed for a quorum, with one other member with a title of director or higher. Time required was the 1-hour daily meeting, as well as one full-time position for case review, preparation, and program administration. The case presentation included a clinical summary of the hospitalization as well as COVID-19 status and testing history, previous living situation, level of home support, functional level, psychosocial needs, barrier(s) to discharging home, and long-term residential plans. A structured assessment was then made by each CLRC member in accordance with their professional expertise. Unanimous consensus would be reached before finalizing any recommended adjustments to the discharge, which would be communicated to the inpatient care team via a structured note within the EMR, along with direct communication to the assigned case manager or social worker. When the CLRC suggested adjustments to the discharge, they would work with the assigned case manager or social worker to communicate an appropriate post–acute care plan with the patient or appropriate representative. If there was disagreement or the recommendations could not be followed, the case manager or social worker would place a new referral with additional information for reconsideration. Following a recommendation for SNF, verification would be completed by the CLRC prior to discharge. This process is detailed in Figure 1.
Patient Population
Inclusion criteria for the study were: (1) inpatient hospitalization and (2) eligibility for risk scoring via the organization’s clinical analytics prediction engine (CAPE).8 CAPE is a validated predictive model that includes risk of readmission, in-hospital mortality, and out-of-hospital mortality,8 with extensive adoption at NUHS. CAPE score eligibility was used as an inclusion criterion so that CAPE could be applied for derivation of a matched control. CAPE eligibility criteria include admission age of at least 18 years and that hospitalization is not psychiatric, rehabilitative, or obstetric. Patients must not be enrolled in hospice and must be discharged alive.
Exclusions were patients who tested positive for SARS-CoV-2 prior to or during index hospitalization. Excluding COVID-19 patients from the analysis eliminated a confounder not present in the preintervention group.
For patients with multiple inpatient admissions, the first admission was the only admission used for analysis. Additionally, if a patient had an admission that occurred in both the preintervention and postintervention periods, they were included only in the postintervention period. This was done to avoid any within-subject correlation and ensure unique patients in each group. Confounding from this approach was mitigated through the process of deriving a matched control.
Outcomes Measurement
The primary outcome of interest was total discharges to SNF across NUHS facilities after hospital admission. Patients were identified as discharging to a SNF if discharge destination codes 03, 64, or 83 appeared on the hospital bill. Additionally, new discharges to SNFs were assessed and identified if documentation indicated that the patient’s living arrangement prior to admission was not a SNF but discharge billing destination codes 03, 64, or 83 appeared on the hospital bill.
Secondary outcomes were measurement of readmissions, days to readmission, and median length of stay (LOS). Readmissions and LOS were balancing measures for the primary outcome, with readmissions measured to evaluate the safety of the CLRC process and LOS measured to evaluate its efficiency. A readmission was any patient who had an unplanned inpatient admission at an NUHS facility within 30 days after an index admission. LOS was measured in days from arrival on a hospital unit to time of discharge.
Additional analysis was done to estimate the effect of the intervention on the incidence of COVID-19 in the 30 days following discharge by comparing the observed to expected incidence of COVID-19 by discharge destination. The expected values were derived by estimating COVID-19 cases that would have been expected to occur with rates of preintervention SNF utilization. This was accomplished by multiplying the observed incidence of COVID-19 in the 30 days following discharge by the number of patients who were discharged to SNFs or home/other in the preintervention period. This expected value was then compared with the observed values to estimate the effect size of the intervention on COVID-19 incidence following discharge. This method of deriving an expected value from the observed incidence was utilized because the preintervention period was before COVID-19 was widespread in the community. It was therefore not possible to directly measure COVID-19 incidence in the preintervention period.
Data Source
Data were retrieved from the NUHS Enterprise Data Warehouse, NUHS’s central data repository, which contains a nightly upload of clinical and financial data from the EMR. Data were collected between March 19, 2019, and July 16, 2020.
The preintervention period was defined as March 19, 2019, to March 18, 2020. Data from that interval were compared with the postintervention period, which was from March 19, 2020, to July 16, 2020. The preintervention period, 1 year immediately prior to the intervention, was chosen to limit any effect of temporal trends while also providing a large sample size. The postintervention period began on the first day NUHS implemented the revised approach to SNF use and ended on the last day before the review process was modified.
Data Analysis
An interrupted time series was used to measure the impact of adoption of the CLRC protocol. A matched control was derived from the preintervention population. To derive this matched control, there was an assessment of covariates in the preintervention and postintervention groups using a standardized mean difference (SMD)9 that indicated an imbalance (SMD ≥ 0.1) in some covariates. A propensity score–matching technique10 was applied to address this imbalance and lack of randomization.
The candidate variables for propensity matching were chosen if they had an association with 30-day readmission. Readmission was chosen to find candidate variables because, of the possible outcomes, this was the only one that was not directly impacted by any CLRC decision. Each covariate was assessed using a logistic regression model while controlling for the postintervention group. If there was an association between a covariate and the outcome, it was chosen for propensity matching. Propensity scores were calculated using a logistic regression model with the treatment (1/0) variable as the dependent variable and the chosen covariates as predictors.
There were no indications of strong multicollinearity. The propensity scores generated were then used to derive a matched control using paired matching. MatchIt package in R (R Foundation for Statistical Computing) was used to create a matched dataset with a logit distance and standard caliper of 0.2 times the standard deviations of the logit of the propensity score. If a match was not found within the caliper, the nearest available match was used.
Regression adjustment11 was then performed using multivariate linear/logistic regression with LOS, readmission rate, days to readmission, total SNF discharges, and new SNF discharges as the outcomes. Treatment (1/0) variable and propensity score were used as the predictors. The adjusted coefficients or odds ratios (ORs) of the intervention variable were thus derived, and their associated P values were used to assess the impact of the intervention on the respective outcomes.
RESULTS
The unmatched preintervention population included 14,468 patients, with 4424 patients in the postintervention population. A matched population was derived and, after matching, the population sizes for pre and post intervention were 4424 each. In the matched population, all measured preintervention characteristics had SMDs and P values that were statistically equivalent. Patient characteristics for the unmatched and matched populations are detailed in Table 1.
During the preintervention period, 1130 (25.5%) patients were discharged to a SNF, with 776 (17.5%) patients being new SNF discharges. In the postintervention period, 568 (12.8%) patients were discharged to a SNF, with 257 (5.8%) patients being new SNF discharges. Total SNF discharges postintervention saw a 49.7% relative reduction (OR, 0.42; 95% CI, 0.38-0.47), while new SNF discharges saw a 66.9% relative reduction (OR, 0.29; 95% CI, 0.25-0.34). These results for both total and new SNF discharges were statistically significant, with P values of <.001, respectively.
Readmissions in the preintervention period were 529 (12.0%) patients, compared with 559 (12.6%) patients in the postintervention period (OR, 1.06; 95% CI, 0.93-1.20; P =.406). An OR was also calculated for readmissions, adjusting for discharge disposition, to account for changes observed in SNF use in the postintervention period. This OR was 1.11 (95% CI, 0.97-1.26; P = .131). Days to readmission in the preintervention and postintervention groups were 11.0 days and 12.0 days, respectively (OR, 0.41; 95% CI, –0.61 to 1.43; P = .429).
LOS was 3.61 days in the preintervention group and 3.64 days in the postintervention group, with an interquartile range (IQR) of 2.14 to 5.69 days in the preintervention group and 2.08 to 5.95 in the postintervention group (OR, 0.09; 95% CI, –0.09 to 0.27; P =.316). These results are summarized in Table 2.
DISCUSSION
A COVID-19 outbreak in a SNF presents a grave risk to residents and patients discharged to these facilities. It is critical for healthcare systems to do the utmost to protect the health of this vulnerable population and the public in efforts to limit COVID-19 within SNFs.12-14
In this study, we observed that at NUHS, establishing a multidisciplinary review committee, the CLRC, to assess the appropriateness of discharge to a SNF after hospitalization resulted in a nearly 50% reduction in total SNF discharges and a greater than two-thirds reduction in new SNF discharges, without any increase in LOS or readmissions. Additionally, it was observed that discharging to settings other than a SNF greatly reduced a patient’s risk of being diagnosed with COVID-19 within 30 days, a result that reached statistical significance. Based on the observed 37.2% relative reduction in COVID-19 cases, we estimate that there may have been one COVID-19 infection prevented every 5.6 days from this intervention. Based on published COVID-19 mortality rates for SNF residents,1 the intervention may have prevented one death every 2.6 weeks. Beyond the risk of COVID-19, other benefits of reducing SNF use are patient and family well-being. Although not measured in this study, others have published about the significant psychological burdens placed on SNF residents, who were at high risk for social isolation, anxiety, and depression during the COVID-19 pandemic2,15-19 Family members also may have had increased stress, as they were deprived of the opportunity to visit loved ones, advocate for them, and help maintain their identity, humanity, and quality of life.20
Although other hospitals have established a structured approach to reduce COVID-19 in SNFs,21 to the best of the authors’ knowledge, the approach described in this article is a unique response to the COVID-19 pandemic. As we have demonstrated, it is highly effective and safe and likely prevented many COVID-19 cases and deaths.
Furthermore, a review committee, such as the one we have described, has value well beyond the COVID-19 pandemic. The health and affordability of care for patients, provider success in value-based care models, and the long-term sustainability of the US healthcare system require close attention to appropriate use of expensive services and to ensuring that their use creates high value. SNF use after a hospitalization is one such service that is frequently targeted and thought to contribute to a substantial portion of wasteful medical spending.22,23 Additionally, SNFs are known to be high risk for communicable disease outbreaks other than COVID-19,24,25 as well as a high-risk environment for many other preventable adverse events.25,26 This review committee ultimately serves to help determine the most appropriate postacute setting for patients being discharged with a determination made through considerations for patient safety, rehabilitation potential, and mental and physical well-being. From a population health perspective, this can lead to better outcomes and lower costs.22,23 Therefore, although the risks of COVID-19 infection in SNFs are expected to subside, the work of evaluating appropriate use of SNFs after hospitalization at our institution continues. The broader focus now extends beyond postacute level of service toward ensuring a high-value discharge that results in both appropriate resource use and safe patient care transitions.
Limitations of this study include its retrospective nature, results from a single center, and a number of potentially unmeasured confounders that the COVID-19 pandemic created. One possible confounder is that the reduction in SNF use we observed was a temporal trend related to changing preferences. In addressing this, we reviewed Medicare claims data from the US Department of Health and Human Services in April 2020 and July 2020 compared with the same period in 2019. These data demonstrated only a modest reduction in spending on SNFs in April 2020 that was smaller than the reduction seen in Part A inpatient hospital spending during that same month.27 By July 2020, the spending from Medicare on SNFs exceeded the levels seen in 2019,27 suggesting that the percentage of acute care admissions discharging to SNFs was no lower for Medicare patients in response to COVD-19. We also considered more stringent SNF admission standards as another potential confounder; however, this was not seen at the SNFs in the NUHS geography, where the referral process became less stringent because of COVID-19 waivers for a qualifying stay or skilled need from the Centers for Medicare and Medicaid Services. We were also not able to account for readmissions outside of NUHS, and therefore there may have been differences in the readmission rate that were unmeasured. To address this limitation, we reviewed a data extract from the Illinois Health and Hospital Association and found that the percentage of patients who returned for readmission to a NUHS facility in the year prior to the intervention and during the intervention period were 92.8% and 95.3%, respectively. From this we concluded the unmeasured readmission rate appears to be low, stable, and unlikely to have altered the results of this study. Additionally, when calculating potential COVID-19 cases avoided, the expected number was, by necessity, derived from the observed outcome, given the absence of COVID-19 in the preintervention population. This may have introduced unmeasured confounders, limiting the ability to precisely measure the effect size or draw conclusions on causation. Finally, there may be limitations to the generalizability of these results based on the payor mix of the population at NUHS, which is predominantly insured through Medicare or commercial payors.
CONCLUSION
We believe this model is replicable and the results generalizable and could serve as both a template for reducing the risks of COVID-19 in SNFs and as part of a larger infection-control strategy to mitigate disease spread in vulnerable populations. It could also be applied as a component of value-improvement programs to foster appropriate use of postacute services after an acute care hospitalization, ensuring safe transitions of care through promotion of high-value care practices.
Acknowledgment
The authors thank Wei Ning Chi for editorial assistance.
1. McMichael TM, Currie DW, Clark S, et al. Epidemiology of Covid-19 in a long-term care facility in King County, Washington. N Engl J Med. 2020;382(21):2005-2011. https://doi.org/10.1056/NEJMoa2005412
2. Ouslander JG, Grabowski DC. COVID-19 in nursing homes: calming the perfect storm. J Am Geriatr Soc. 2020;68(10):2153-2162. https://doi.org/10.1111/jgs.16784
3. CDC COVID-19 Response Team. Severe outcomes among patients with coronavirus disease 2019 (COVID-19) - United States, February 12-March 16, 2020. MMWR Morb Mortal Wkly Rep. 2020;69(12):343-346. https://doi.org/10.15585/mmwr.mm6912e2
4. Ko JY, Danielson ML, Town M, et al. Risk factors for coronavirus disease 2019 (COVID-19)-associated hospitalization: COVID-19-Associated Hospitalization Surveillance Network and Behavioral Risk Factor Surveillance System. Clin Infect Dis. 2020;72(11):e695-e703. https://doi.org/10.1093/cid/ciaa1419
5. Davidson PM, Szanton SL. Nursing homes and COVID-19: we can and should do better. J Clin Nurs. 2020;29(15-16):2758-2759. https://doi.org/10.1111/jocn.15297
6. Dosa D, Jump RLP, LaPlante K, Gravenstein S. Long-term care facilities and the coronavirus epidemic: practical guidelines for a population at highest risk. J Am Med Dir Assoc. 2020;21(5):569-571. https://doi.org/10.1016/j.jamda.2020.03.004
7. Fallon A, Dukelow T, Kennelly SP, O’Neill D. COVID-19 in nursing homes. QJM. 2020;113(6):391-392. https://doi.org/10.1093/qjmed/hcaa136
8. Shah N, Konchak C, Chertok D, et al. Clinical Analytics Prediction Engine (CAPE): development, electronic health record integration and prospective validation of hospital mortality, 180-day mortality and 30-day readmission risk prediction models. PLoS One. 2020;15(8):e0238065. https://doi.org/10.1371/journal.pone.0238065
9. Austin PC. Using the standardized difference to compare the prevalence of a binary variable between two groups in observational research. Commun Stat Simul Comput. 2009;38(6):1228-1234. https://doi.org/10.1080/03610910902859574
10. Rosenbaum PR, Rubin DB. Constructing a control group using multivariate matched sampling methods that incorporate the propensity score. Am Stat. 1985;39(1):33-38. https://doi.org/10.2307/2683903
11. Myers JA, Louis TA. Regression adjustment and stratification by propensity score in treatment effect estimation. Johns Hopkins University, Dept of Biostatistics Working Papers. 2010 203(Working Papers):1-27.
12. Lansbury LE, Brown CS, Nguyen-Van-Tam JS. Influenza in long-term care facilities. Influenza Other Respir Viruses. 2017;11(5):356-366. https://doi.org/10.1111/irv.12464
13. Sáez-López E, Marques R, Rodrigues N, et al. Lessons learned from a prolonged norovirus GII.P16-GII.4 Sydney 2012 variant outbreak in a long-term care facility in Portugal, 2017. Infect Control Hosp Epidemiol. 2019;40(10):1164-1169. https://doi.org/10.1017/ice.2019.201
14. Gaspard P, Mosnier A, Stoll-Keller F, Roth C, Larocca S, Bertrand X. Influenza prevention in nursing homes: great significance of seasonal variability and spatio-temporal pattern. Presse Med. 2015;44(10):e311-e319. https://doi.org/10.1016/j.lpm.2015.04.041
15. Pfefferbaum B, North CS. Mental health and the Covid-19 pandemic. N Engl J Med. 2020;383(6):510-512. https://doi.org/10.1056/NEJMp2008017
16. Galea S, Merchant RM, Lurie N. The mental health consequences of COVID-19 and physical distancing: the need for prevention and early intervention. JAMA Intern Med. 2020;180(6):817-818. https://doi.org/10.1001/jamainternmed.2020.1562
17. Armitage R, Nellums LB. COVID-19 and the consequences of isolating the elderly. Lancet Public Health. 2020;5(5):e256. https://doi.org/10.1016/s2468-2667(20)30061-x
18. El Haj M, Altintas E, Chapelet G, Kapogiannis D, Gallouj K. High depression and anxiety in people with Alzheimer’s disease living in retirement homes during the covid-19 crisis. Psychiatry Res. 2020;291:113294. https://doi.org/10.1016/j.psychres.2020.113294
19. Santini ZI, Jose PE, York Cornwell E, et al. Social disconnectedness, perceived isolation, and symptoms of depression and anxiety among older Americans (NSHAP): a longitudinal mediation analysis. Lancet Public Health. 2020;5(1):e62-e70. https://doi.org/10.1016/s2468-2667(19)30230-0
20. Gaugler JE, Anderson KA, Zarit SH, Pearlin LI. Family involvement in nursing homes: effects on stress and well-being. Aging Ment Health. 2004;8(1):65-75. https://doi.org/10.1080/13607860310001613356
21. Kim G, Wang M, Pan H, et al. A health system response to COVID-19 in long-term care and post-acute care: a three-phase approach. J Am Geriatr Soc. 2020;68(6):1155-1161. https://doi.org/10.1111/jgs.16513
22. McWilliams JM, Gilstrap LG, Stevenson DG, Chernew ME, Huskamp HA, Grabowski DC. Changes in postacute care in the Medicare Shared Savings Program. JAMA Intern Med. 2017;177(4):518-526. https://doi.org/10.1001/jamainternmed.2016.9115
23. Ackerly DC, Grabowski DC. Post-acute care reform--beyond the ACA. N Engl J Med. 2014;370(8):689-691. https://doi.org/10.1056/NEJMp1315350
24. Strausbaugh LJ, Sukumar SR, Joseph CL. Infectious disease outbreaks in nursing homes: an unappreciated hazard for frail elderly persons. Clin Infect Dis. 2003;36(7):870-876. https://doi.org/10.1086/368197
25. Kapoor A, Field T, Handler S, et al. Adverse events in long-term care residents transitioning from hospital back to nursing home. JAMA Intern Med. 2019;179(9):1254-1261. https://doi.org/10.1001/jamainternmed.2019.2005
26. Adverse Events in Skilled Nursing Facilities: National Incidence Among Medicare Beneficiaries. Office of Inspector General, US Dept of Health & Human Services; 2014.
27. The Impact of the COVID-19 Pandemic on Medicare Beneficiary Use of Health Care Services and Payments to Providers: Early Data for the First 6 Months of 2020. Office of the Assistant Secretary for Planning and Evaluation, US Dept of Health & Human Services; 2020.
The COVID-19 outbreak in February 2020 at a congregate living facility near Seattle, Washington, signaled the beginning of the pandemic in the United States. In that facility, infected residents had a 54.5% hospitalization rate and 33.7% case-fatality rate.1 Similar to the experience in Washington, all congregate living facilities have proved particularly vulnerable to the effects of COVID-19,2-7 with residents at increased risk for disease severity and mortality.2-7
Due to the COVID-19 emergency, NorthShore University HealthSystem (NUHS), a multihospital, integrated health system in northern Illinois, established a best practice for appropriate use of congregate living facilities after hospitalization. This focused on the safety of discharged patients and mitigation of COVID-19 by putting in place a referral process to a newly established congregate living review committee (CLRC) for review prior to discharge. Although all discharges to congregate living settings are at high risk,2 new placements to skilled nursing facilities (SNFs) were the primary focus of the committee and the sole focus of this study. In this study, we sought to determine whether establishment of the CLRC was associated with a reduction in SNF utilization, whether this was safe and efficient, and whether it was associated with a reduction in COVID-19 incidence in the 30 days following discharge.
METHODS
Setting and Case Review Intervention
We conducted a retrospective cohort study for patients hospitalized within NUHS from March 19, 2019 to July 16, 2020, designed as an interrupted time series. The study was approved by the NUHS Institutional Review Board (EH21-022).
The study exposure was creation of a referral and review process for all patients with expected discharge to a SNF and was implemented as part of usual discharge planning during the COVID-19 pandemic. The key intervention was to establish a multidisciplinary committee, the CLRC, to review all potential discharges to SNFs. The CLRC had dual goals of preventing COVID-19 spread in facilities by limiting placement of new residents and protecting a vulnerable population from a setting that conferred a higher risk of acquiring COVID-19. The CLRC was organized as a multidisciplinary committee with physicians, case managers, social workers, physical therapists, occupational therapists, and the director of NUHS home health agency. Physician members were evenly split as half hospitalists and half ambulatory physicians. The CLRC review was initiated by a patient’s assigned case manager or social worker by consult through a referral in the electronic medical record (EMR). Each case was summarized and then presented to the full CLRC. The CLRC met for 1 hour per day, 6 days per week, to review all planned discharges that met criteria for review. A committee physician chaired each meeting. Three other members were needed for a quorum, with one other member with a title of director or higher. Time required was the 1-hour daily meeting, as well as one full-time position for case review, preparation, and program administration. The case presentation included a clinical summary of the hospitalization as well as COVID-19 status and testing history, previous living situation, level of home support, functional level, psychosocial needs, barrier(s) to discharging home, and long-term residential plans. A structured assessment was then made by each CLRC member in accordance with their professional expertise. Unanimous consensus would be reached before finalizing any recommended adjustments to the discharge, which would be communicated to the inpatient care team via a structured note within the EMR, along with direct communication to the assigned case manager or social worker. When the CLRC suggested adjustments to the discharge, they would work with the assigned case manager or social worker to communicate an appropriate post–acute care plan with the patient or appropriate representative. If there was disagreement or the recommendations could not be followed, the case manager or social worker would place a new referral with additional information for reconsideration. Following a recommendation for SNF, verification would be completed by the CLRC prior to discharge. This process is detailed in Figure 1.
Patient Population
Inclusion criteria for the study were: (1) inpatient hospitalization and (2) eligibility for risk scoring via the organization’s clinical analytics prediction engine (CAPE).8 CAPE is a validated predictive model that includes risk of readmission, in-hospital mortality, and out-of-hospital mortality,8 with extensive adoption at NUHS. CAPE score eligibility was used as an inclusion criterion so that CAPE could be applied for derivation of a matched control. CAPE eligibility criteria include admission age of at least 18 years and that hospitalization is not psychiatric, rehabilitative, or obstetric. Patients must not be enrolled in hospice and must be discharged alive.
Exclusions were patients who tested positive for SARS-CoV-2 prior to or during index hospitalization. Excluding COVID-19 patients from the analysis eliminated a confounder not present in the preintervention group.
For patients with multiple inpatient admissions, the first admission was the only admission used for analysis. Additionally, if a patient had an admission that occurred in both the preintervention and postintervention periods, they were included only in the postintervention period. This was done to avoid any within-subject correlation and ensure unique patients in each group. Confounding from this approach was mitigated through the process of deriving a matched control.
Outcomes Measurement
The primary outcome of interest was total discharges to SNF across NUHS facilities after hospital admission. Patients were identified as discharging to a SNF if discharge destination codes 03, 64, or 83 appeared on the hospital bill. Additionally, new discharges to SNFs were assessed and identified if documentation indicated that the patient’s living arrangement prior to admission was not a SNF but discharge billing destination codes 03, 64, or 83 appeared on the hospital bill.
Secondary outcomes were measurement of readmissions, days to readmission, and median length of stay (LOS). Readmissions and LOS were balancing measures for the primary outcome, with readmissions measured to evaluate the safety of the CLRC process and LOS measured to evaluate its efficiency. A readmission was any patient who had an unplanned inpatient admission at an NUHS facility within 30 days after an index admission. LOS was measured in days from arrival on a hospital unit to time of discharge.
Additional analysis was done to estimate the effect of the intervention on the incidence of COVID-19 in the 30 days following discharge by comparing the observed to expected incidence of COVID-19 by discharge destination. The expected values were derived by estimating COVID-19 cases that would have been expected to occur with rates of preintervention SNF utilization. This was accomplished by multiplying the observed incidence of COVID-19 in the 30 days following discharge by the number of patients who were discharged to SNFs or home/other in the preintervention period. This expected value was then compared with the observed values to estimate the effect size of the intervention on COVID-19 incidence following discharge. This method of deriving an expected value from the observed incidence was utilized because the preintervention period was before COVID-19 was widespread in the community. It was therefore not possible to directly measure COVID-19 incidence in the preintervention period.
Data Source
Data were retrieved from the NUHS Enterprise Data Warehouse, NUHS’s central data repository, which contains a nightly upload of clinical and financial data from the EMR. Data were collected between March 19, 2019, and July 16, 2020.
The preintervention period was defined as March 19, 2019, to March 18, 2020. Data from that interval were compared with the postintervention period, which was from March 19, 2020, to July 16, 2020. The preintervention period, 1 year immediately prior to the intervention, was chosen to limit any effect of temporal trends while also providing a large sample size. The postintervention period began on the first day NUHS implemented the revised approach to SNF use and ended on the last day before the review process was modified.
Data Analysis
An interrupted time series was used to measure the impact of adoption of the CLRC protocol. A matched control was derived from the preintervention population. To derive this matched control, there was an assessment of covariates in the preintervention and postintervention groups using a standardized mean difference (SMD)9 that indicated an imbalance (SMD ≥ 0.1) in some covariates. A propensity score–matching technique10 was applied to address this imbalance and lack of randomization.
The candidate variables for propensity matching were chosen if they had an association with 30-day readmission. Readmission was chosen to find candidate variables because, of the possible outcomes, this was the only one that was not directly impacted by any CLRC decision. Each covariate was assessed using a logistic regression model while controlling for the postintervention group. If there was an association between a covariate and the outcome, it was chosen for propensity matching. Propensity scores were calculated using a logistic regression model with the treatment (1/0) variable as the dependent variable and the chosen covariates as predictors.
There were no indications of strong multicollinearity. The propensity scores generated were then used to derive a matched control using paired matching. MatchIt package in R (R Foundation for Statistical Computing) was used to create a matched dataset with a logit distance and standard caliper of 0.2 times the standard deviations of the logit of the propensity score. If a match was not found within the caliper, the nearest available match was used.
Regression adjustment11 was then performed using multivariate linear/logistic regression with LOS, readmission rate, days to readmission, total SNF discharges, and new SNF discharges as the outcomes. Treatment (1/0) variable and propensity score were used as the predictors. The adjusted coefficients or odds ratios (ORs) of the intervention variable were thus derived, and their associated P values were used to assess the impact of the intervention on the respective outcomes.
RESULTS
The unmatched preintervention population included 14,468 patients, with 4424 patients in the postintervention population. A matched population was derived and, after matching, the population sizes for pre and post intervention were 4424 each. In the matched population, all measured preintervention characteristics had SMDs and P values that were statistically equivalent. Patient characteristics for the unmatched and matched populations are detailed in Table 1.
During the preintervention period, 1130 (25.5%) patients were discharged to a SNF, with 776 (17.5%) patients being new SNF discharges. In the postintervention period, 568 (12.8%) patients were discharged to a SNF, with 257 (5.8%) patients being new SNF discharges. Total SNF discharges postintervention saw a 49.7% relative reduction (OR, 0.42; 95% CI, 0.38-0.47), while new SNF discharges saw a 66.9% relative reduction (OR, 0.29; 95% CI, 0.25-0.34). These results for both total and new SNF discharges were statistically significant, with P values of <.001, respectively.
Readmissions in the preintervention period were 529 (12.0%) patients, compared with 559 (12.6%) patients in the postintervention period (OR, 1.06; 95% CI, 0.93-1.20; P =.406). An OR was also calculated for readmissions, adjusting for discharge disposition, to account for changes observed in SNF use in the postintervention period. This OR was 1.11 (95% CI, 0.97-1.26; P = .131). Days to readmission in the preintervention and postintervention groups were 11.0 days and 12.0 days, respectively (OR, 0.41; 95% CI, –0.61 to 1.43; P = .429).
LOS was 3.61 days in the preintervention group and 3.64 days in the postintervention group, with an interquartile range (IQR) of 2.14 to 5.69 days in the preintervention group and 2.08 to 5.95 in the postintervention group (OR, 0.09; 95% CI, –0.09 to 0.27; P =.316). These results are summarized in Table 2.
DISCUSSION
A COVID-19 outbreak in a SNF presents a grave risk to residents and patients discharged to these facilities. It is critical for healthcare systems to do the utmost to protect the health of this vulnerable population and the public in efforts to limit COVID-19 within SNFs.12-14
In this study, we observed that at NUHS, establishing a multidisciplinary review committee, the CLRC, to assess the appropriateness of discharge to a SNF after hospitalization resulted in a nearly 50% reduction in total SNF discharges and a greater than two-thirds reduction in new SNF discharges, without any increase in LOS or readmissions. Additionally, it was observed that discharging to settings other than a SNF greatly reduced a patient’s risk of being diagnosed with COVID-19 within 30 days, a result that reached statistical significance. Based on the observed 37.2% relative reduction in COVID-19 cases, we estimate that there may have been one COVID-19 infection prevented every 5.6 days from this intervention. Based on published COVID-19 mortality rates for SNF residents,1 the intervention may have prevented one death every 2.6 weeks. Beyond the risk of COVID-19, other benefits of reducing SNF use are patient and family well-being. Although not measured in this study, others have published about the significant psychological burdens placed on SNF residents, who were at high risk for social isolation, anxiety, and depression during the COVID-19 pandemic2,15-19 Family members also may have had increased stress, as they were deprived of the opportunity to visit loved ones, advocate for them, and help maintain their identity, humanity, and quality of life.20
Although other hospitals have established a structured approach to reduce COVID-19 in SNFs,21 to the best of the authors’ knowledge, the approach described in this article is a unique response to the COVID-19 pandemic. As we have demonstrated, it is highly effective and safe and likely prevented many COVID-19 cases and deaths.
Furthermore, a review committee, such as the one we have described, has value well beyond the COVID-19 pandemic. The health and affordability of care for patients, provider success in value-based care models, and the long-term sustainability of the US healthcare system require close attention to appropriate use of expensive services and to ensuring that their use creates high value. SNF use after a hospitalization is one such service that is frequently targeted and thought to contribute to a substantial portion of wasteful medical spending.22,23 Additionally, SNFs are known to be high risk for communicable disease outbreaks other than COVID-19,24,25 as well as a high-risk environment for many other preventable adverse events.25,26 This review committee ultimately serves to help determine the most appropriate postacute setting for patients being discharged with a determination made through considerations for patient safety, rehabilitation potential, and mental and physical well-being. From a population health perspective, this can lead to better outcomes and lower costs.22,23 Therefore, although the risks of COVID-19 infection in SNFs are expected to subside, the work of evaluating appropriate use of SNFs after hospitalization at our institution continues. The broader focus now extends beyond postacute level of service toward ensuring a high-value discharge that results in both appropriate resource use and safe patient care transitions.
Limitations of this study include its retrospective nature, results from a single center, and a number of potentially unmeasured confounders that the COVID-19 pandemic created. One possible confounder is that the reduction in SNF use we observed was a temporal trend related to changing preferences. In addressing this, we reviewed Medicare claims data from the US Department of Health and Human Services in April 2020 and July 2020 compared with the same period in 2019. These data demonstrated only a modest reduction in spending on SNFs in April 2020 that was smaller than the reduction seen in Part A inpatient hospital spending during that same month.27 By July 2020, the spending from Medicare on SNFs exceeded the levels seen in 2019,27 suggesting that the percentage of acute care admissions discharging to SNFs was no lower for Medicare patients in response to COVD-19. We also considered more stringent SNF admission standards as another potential confounder; however, this was not seen at the SNFs in the NUHS geography, where the referral process became less stringent because of COVID-19 waivers for a qualifying stay or skilled need from the Centers for Medicare and Medicaid Services. We were also not able to account for readmissions outside of NUHS, and therefore there may have been differences in the readmission rate that were unmeasured. To address this limitation, we reviewed a data extract from the Illinois Health and Hospital Association and found that the percentage of patients who returned for readmission to a NUHS facility in the year prior to the intervention and during the intervention period were 92.8% and 95.3%, respectively. From this we concluded the unmeasured readmission rate appears to be low, stable, and unlikely to have altered the results of this study. Additionally, when calculating potential COVID-19 cases avoided, the expected number was, by necessity, derived from the observed outcome, given the absence of COVID-19 in the preintervention population. This may have introduced unmeasured confounders, limiting the ability to precisely measure the effect size or draw conclusions on causation. Finally, there may be limitations to the generalizability of these results based on the payor mix of the population at NUHS, which is predominantly insured through Medicare or commercial payors.
CONCLUSION
We believe this model is replicable and the results generalizable and could serve as both a template for reducing the risks of COVID-19 in SNFs and as part of a larger infection-control strategy to mitigate disease spread in vulnerable populations. It could also be applied as a component of value-improvement programs to foster appropriate use of postacute services after an acute care hospitalization, ensuring safe transitions of care through promotion of high-value care practices.
Acknowledgment
The authors thank Wei Ning Chi for editorial assistance.
The COVID-19 outbreak in February 2020 at a congregate living facility near Seattle, Washington, signaled the beginning of the pandemic in the United States. In that facility, infected residents had a 54.5% hospitalization rate and 33.7% case-fatality rate.1 Similar to the experience in Washington, all congregate living facilities have proved particularly vulnerable to the effects of COVID-19,2-7 with residents at increased risk for disease severity and mortality.2-7
Due to the COVID-19 emergency, NorthShore University HealthSystem (NUHS), a multihospital, integrated health system in northern Illinois, established a best practice for appropriate use of congregate living facilities after hospitalization. This focused on the safety of discharged patients and mitigation of COVID-19 by putting in place a referral process to a newly established congregate living review committee (CLRC) for review prior to discharge. Although all discharges to congregate living settings are at high risk,2 new placements to skilled nursing facilities (SNFs) were the primary focus of the committee and the sole focus of this study. In this study, we sought to determine whether establishment of the CLRC was associated with a reduction in SNF utilization, whether this was safe and efficient, and whether it was associated with a reduction in COVID-19 incidence in the 30 days following discharge.
METHODS
Setting and Case Review Intervention
We conducted a retrospective cohort study for patients hospitalized within NUHS from March 19, 2019 to July 16, 2020, designed as an interrupted time series. The study was approved by the NUHS Institutional Review Board (EH21-022).
The study exposure was creation of a referral and review process for all patients with expected discharge to a SNF and was implemented as part of usual discharge planning during the COVID-19 pandemic. The key intervention was to establish a multidisciplinary committee, the CLRC, to review all potential discharges to SNFs. The CLRC had dual goals of preventing COVID-19 spread in facilities by limiting placement of new residents and protecting a vulnerable population from a setting that conferred a higher risk of acquiring COVID-19. The CLRC was organized as a multidisciplinary committee with physicians, case managers, social workers, physical therapists, occupational therapists, and the director of NUHS home health agency. Physician members were evenly split as half hospitalists and half ambulatory physicians. The CLRC review was initiated by a patient’s assigned case manager or social worker by consult through a referral in the electronic medical record (EMR). Each case was summarized and then presented to the full CLRC. The CLRC met for 1 hour per day, 6 days per week, to review all planned discharges that met criteria for review. A committee physician chaired each meeting. Three other members were needed for a quorum, with one other member with a title of director or higher. Time required was the 1-hour daily meeting, as well as one full-time position for case review, preparation, and program administration. The case presentation included a clinical summary of the hospitalization as well as COVID-19 status and testing history, previous living situation, level of home support, functional level, psychosocial needs, barrier(s) to discharging home, and long-term residential plans. A structured assessment was then made by each CLRC member in accordance with their professional expertise. Unanimous consensus would be reached before finalizing any recommended adjustments to the discharge, which would be communicated to the inpatient care team via a structured note within the EMR, along with direct communication to the assigned case manager or social worker. When the CLRC suggested adjustments to the discharge, they would work with the assigned case manager or social worker to communicate an appropriate post–acute care plan with the patient or appropriate representative. If there was disagreement or the recommendations could not be followed, the case manager or social worker would place a new referral with additional information for reconsideration. Following a recommendation for SNF, verification would be completed by the CLRC prior to discharge. This process is detailed in Figure 1.
Patient Population
Inclusion criteria for the study were: (1) inpatient hospitalization and (2) eligibility for risk scoring via the organization’s clinical analytics prediction engine (CAPE).8 CAPE is a validated predictive model that includes risk of readmission, in-hospital mortality, and out-of-hospital mortality,8 with extensive adoption at NUHS. CAPE score eligibility was used as an inclusion criterion so that CAPE could be applied for derivation of a matched control. CAPE eligibility criteria include admission age of at least 18 years and that hospitalization is not psychiatric, rehabilitative, or obstetric. Patients must not be enrolled in hospice and must be discharged alive.
Exclusions were patients who tested positive for SARS-CoV-2 prior to or during index hospitalization. Excluding COVID-19 patients from the analysis eliminated a confounder not present in the preintervention group.
For patients with multiple inpatient admissions, the first admission was the only admission used for analysis. Additionally, if a patient had an admission that occurred in both the preintervention and postintervention periods, they were included only in the postintervention period. This was done to avoid any within-subject correlation and ensure unique patients in each group. Confounding from this approach was mitigated through the process of deriving a matched control.
Outcomes Measurement
The primary outcome of interest was total discharges to SNF across NUHS facilities after hospital admission. Patients were identified as discharging to a SNF if discharge destination codes 03, 64, or 83 appeared on the hospital bill. Additionally, new discharges to SNFs were assessed and identified if documentation indicated that the patient’s living arrangement prior to admission was not a SNF but discharge billing destination codes 03, 64, or 83 appeared on the hospital bill.
Secondary outcomes were measurement of readmissions, days to readmission, and median length of stay (LOS). Readmissions and LOS were balancing measures for the primary outcome, with readmissions measured to evaluate the safety of the CLRC process and LOS measured to evaluate its efficiency. A readmission was any patient who had an unplanned inpatient admission at an NUHS facility within 30 days after an index admission. LOS was measured in days from arrival on a hospital unit to time of discharge.
Additional analysis was done to estimate the effect of the intervention on the incidence of COVID-19 in the 30 days following discharge by comparing the observed to expected incidence of COVID-19 by discharge destination. The expected values were derived by estimating COVID-19 cases that would have been expected to occur with rates of preintervention SNF utilization. This was accomplished by multiplying the observed incidence of COVID-19 in the 30 days following discharge by the number of patients who were discharged to SNFs or home/other in the preintervention period. This expected value was then compared with the observed values to estimate the effect size of the intervention on COVID-19 incidence following discharge. This method of deriving an expected value from the observed incidence was utilized because the preintervention period was before COVID-19 was widespread in the community. It was therefore not possible to directly measure COVID-19 incidence in the preintervention period.
Data Source
Data were retrieved from the NUHS Enterprise Data Warehouse, NUHS’s central data repository, which contains a nightly upload of clinical and financial data from the EMR. Data were collected between March 19, 2019, and July 16, 2020.
The preintervention period was defined as March 19, 2019, to March 18, 2020. Data from that interval were compared with the postintervention period, which was from March 19, 2020, to July 16, 2020. The preintervention period, 1 year immediately prior to the intervention, was chosen to limit any effect of temporal trends while also providing a large sample size. The postintervention period began on the first day NUHS implemented the revised approach to SNF use and ended on the last day before the review process was modified.
Data Analysis
An interrupted time series was used to measure the impact of adoption of the CLRC protocol. A matched control was derived from the preintervention population. To derive this matched control, there was an assessment of covariates in the preintervention and postintervention groups using a standardized mean difference (SMD)9 that indicated an imbalance (SMD ≥ 0.1) in some covariates. A propensity score–matching technique10 was applied to address this imbalance and lack of randomization.
The candidate variables for propensity matching were chosen if they had an association with 30-day readmission. Readmission was chosen to find candidate variables because, of the possible outcomes, this was the only one that was not directly impacted by any CLRC decision. Each covariate was assessed using a logistic regression model while controlling for the postintervention group. If there was an association between a covariate and the outcome, it was chosen for propensity matching. Propensity scores were calculated using a logistic regression model with the treatment (1/0) variable as the dependent variable and the chosen covariates as predictors.
There were no indications of strong multicollinearity. The propensity scores generated were then used to derive a matched control using paired matching. MatchIt package in R (R Foundation for Statistical Computing) was used to create a matched dataset with a logit distance and standard caliper of 0.2 times the standard deviations of the logit of the propensity score. If a match was not found within the caliper, the nearest available match was used.
Regression adjustment11 was then performed using multivariate linear/logistic regression with LOS, readmission rate, days to readmission, total SNF discharges, and new SNF discharges as the outcomes. Treatment (1/0) variable and propensity score were used as the predictors. The adjusted coefficients or odds ratios (ORs) of the intervention variable were thus derived, and their associated P values were used to assess the impact of the intervention on the respective outcomes.
RESULTS
The unmatched preintervention population included 14,468 patients, with 4424 patients in the postintervention population. A matched population was derived and, after matching, the population sizes for pre and post intervention were 4424 each. In the matched population, all measured preintervention characteristics had SMDs and P values that were statistically equivalent. Patient characteristics for the unmatched and matched populations are detailed in Table 1.
During the preintervention period, 1130 (25.5%) patients were discharged to a SNF, with 776 (17.5%) patients being new SNF discharges. In the postintervention period, 568 (12.8%) patients were discharged to a SNF, with 257 (5.8%) patients being new SNF discharges. Total SNF discharges postintervention saw a 49.7% relative reduction (OR, 0.42; 95% CI, 0.38-0.47), while new SNF discharges saw a 66.9% relative reduction (OR, 0.29; 95% CI, 0.25-0.34). These results for both total and new SNF discharges were statistically significant, with P values of <.001, respectively.
Readmissions in the preintervention period were 529 (12.0%) patients, compared with 559 (12.6%) patients in the postintervention period (OR, 1.06; 95% CI, 0.93-1.20; P =.406). An OR was also calculated for readmissions, adjusting for discharge disposition, to account for changes observed in SNF use in the postintervention period. This OR was 1.11 (95% CI, 0.97-1.26; P = .131). Days to readmission in the preintervention and postintervention groups were 11.0 days and 12.0 days, respectively (OR, 0.41; 95% CI, –0.61 to 1.43; P = .429).
LOS was 3.61 days in the preintervention group and 3.64 days in the postintervention group, with an interquartile range (IQR) of 2.14 to 5.69 days in the preintervention group and 2.08 to 5.95 in the postintervention group (OR, 0.09; 95% CI, –0.09 to 0.27; P =.316). These results are summarized in Table 2.
DISCUSSION
A COVID-19 outbreak in a SNF presents a grave risk to residents and patients discharged to these facilities. It is critical for healthcare systems to do the utmost to protect the health of this vulnerable population and the public in efforts to limit COVID-19 within SNFs.12-14
In this study, we observed that at NUHS, establishing a multidisciplinary review committee, the CLRC, to assess the appropriateness of discharge to a SNF after hospitalization resulted in a nearly 50% reduction in total SNF discharges and a greater than two-thirds reduction in new SNF discharges, without any increase in LOS or readmissions. Additionally, it was observed that discharging to settings other than a SNF greatly reduced a patient’s risk of being diagnosed with COVID-19 within 30 days, a result that reached statistical significance. Based on the observed 37.2% relative reduction in COVID-19 cases, we estimate that there may have been one COVID-19 infection prevented every 5.6 days from this intervention. Based on published COVID-19 mortality rates for SNF residents,1 the intervention may have prevented one death every 2.6 weeks. Beyond the risk of COVID-19, other benefits of reducing SNF use are patient and family well-being. Although not measured in this study, others have published about the significant psychological burdens placed on SNF residents, who were at high risk for social isolation, anxiety, and depression during the COVID-19 pandemic2,15-19 Family members also may have had increased stress, as they were deprived of the opportunity to visit loved ones, advocate for them, and help maintain their identity, humanity, and quality of life.20
Although other hospitals have established a structured approach to reduce COVID-19 in SNFs,21 to the best of the authors’ knowledge, the approach described in this article is a unique response to the COVID-19 pandemic. As we have demonstrated, it is highly effective and safe and likely prevented many COVID-19 cases and deaths.
Furthermore, a review committee, such as the one we have described, has value well beyond the COVID-19 pandemic. The health and affordability of care for patients, provider success in value-based care models, and the long-term sustainability of the US healthcare system require close attention to appropriate use of expensive services and to ensuring that their use creates high value. SNF use after a hospitalization is one such service that is frequently targeted and thought to contribute to a substantial portion of wasteful medical spending.22,23 Additionally, SNFs are known to be high risk for communicable disease outbreaks other than COVID-19,24,25 as well as a high-risk environment for many other preventable adverse events.25,26 This review committee ultimately serves to help determine the most appropriate postacute setting for patients being discharged with a determination made through considerations for patient safety, rehabilitation potential, and mental and physical well-being. From a population health perspective, this can lead to better outcomes and lower costs.22,23 Therefore, although the risks of COVID-19 infection in SNFs are expected to subside, the work of evaluating appropriate use of SNFs after hospitalization at our institution continues. The broader focus now extends beyond postacute level of service toward ensuring a high-value discharge that results in both appropriate resource use and safe patient care transitions.
Limitations of this study include its retrospective nature, results from a single center, and a number of potentially unmeasured confounders that the COVID-19 pandemic created. One possible confounder is that the reduction in SNF use we observed was a temporal trend related to changing preferences. In addressing this, we reviewed Medicare claims data from the US Department of Health and Human Services in April 2020 and July 2020 compared with the same period in 2019. These data demonstrated only a modest reduction in spending on SNFs in April 2020 that was smaller than the reduction seen in Part A inpatient hospital spending during that same month.27 By July 2020, the spending from Medicare on SNFs exceeded the levels seen in 2019,27 suggesting that the percentage of acute care admissions discharging to SNFs was no lower for Medicare patients in response to COVD-19. We also considered more stringent SNF admission standards as another potential confounder; however, this was not seen at the SNFs in the NUHS geography, where the referral process became less stringent because of COVID-19 waivers for a qualifying stay or skilled need from the Centers for Medicare and Medicaid Services. We were also not able to account for readmissions outside of NUHS, and therefore there may have been differences in the readmission rate that were unmeasured. To address this limitation, we reviewed a data extract from the Illinois Health and Hospital Association and found that the percentage of patients who returned for readmission to a NUHS facility in the year prior to the intervention and during the intervention period were 92.8% and 95.3%, respectively. From this we concluded the unmeasured readmission rate appears to be low, stable, and unlikely to have altered the results of this study. Additionally, when calculating potential COVID-19 cases avoided, the expected number was, by necessity, derived from the observed outcome, given the absence of COVID-19 in the preintervention population. This may have introduced unmeasured confounders, limiting the ability to precisely measure the effect size or draw conclusions on causation. Finally, there may be limitations to the generalizability of these results based on the payor mix of the population at NUHS, which is predominantly insured through Medicare or commercial payors.
CONCLUSION
We believe this model is replicable and the results generalizable and could serve as both a template for reducing the risks of COVID-19 in SNFs and as part of a larger infection-control strategy to mitigate disease spread in vulnerable populations. It could also be applied as a component of value-improvement programs to foster appropriate use of postacute services after an acute care hospitalization, ensuring safe transitions of care through promotion of high-value care practices.
Acknowledgment
The authors thank Wei Ning Chi for editorial assistance.
1. McMichael TM, Currie DW, Clark S, et al. Epidemiology of Covid-19 in a long-term care facility in King County, Washington. N Engl J Med. 2020;382(21):2005-2011. https://doi.org/10.1056/NEJMoa2005412
2. Ouslander JG, Grabowski DC. COVID-19 in nursing homes: calming the perfect storm. J Am Geriatr Soc. 2020;68(10):2153-2162. https://doi.org/10.1111/jgs.16784
3. CDC COVID-19 Response Team. Severe outcomes among patients with coronavirus disease 2019 (COVID-19) - United States, February 12-March 16, 2020. MMWR Morb Mortal Wkly Rep. 2020;69(12):343-346. https://doi.org/10.15585/mmwr.mm6912e2
4. Ko JY, Danielson ML, Town M, et al. Risk factors for coronavirus disease 2019 (COVID-19)-associated hospitalization: COVID-19-Associated Hospitalization Surveillance Network and Behavioral Risk Factor Surveillance System. Clin Infect Dis. 2020;72(11):e695-e703. https://doi.org/10.1093/cid/ciaa1419
5. Davidson PM, Szanton SL. Nursing homes and COVID-19: we can and should do better. J Clin Nurs. 2020;29(15-16):2758-2759. https://doi.org/10.1111/jocn.15297
6. Dosa D, Jump RLP, LaPlante K, Gravenstein S. Long-term care facilities and the coronavirus epidemic: practical guidelines for a population at highest risk. J Am Med Dir Assoc. 2020;21(5):569-571. https://doi.org/10.1016/j.jamda.2020.03.004
7. Fallon A, Dukelow T, Kennelly SP, O’Neill D. COVID-19 in nursing homes. QJM. 2020;113(6):391-392. https://doi.org/10.1093/qjmed/hcaa136
8. Shah N, Konchak C, Chertok D, et al. Clinical Analytics Prediction Engine (CAPE): development, electronic health record integration and prospective validation of hospital mortality, 180-day mortality and 30-day readmission risk prediction models. PLoS One. 2020;15(8):e0238065. https://doi.org/10.1371/journal.pone.0238065
9. Austin PC. Using the standardized difference to compare the prevalence of a binary variable between two groups in observational research. Commun Stat Simul Comput. 2009;38(6):1228-1234. https://doi.org/10.1080/03610910902859574
10. Rosenbaum PR, Rubin DB. Constructing a control group using multivariate matched sampling methods that incorporate the propensity score. Am Stat. 1985;39(1):33-38. https://doi.org/10.2307/2683903
11. Myers JA, Louis TA. Regression adjustment and stratification by propensity score in treatment effect estimation. Johns Hopkins University, Dept of Biostatistics Working Papers. 2010 203(Working Papers):1-27.
12. Lansbury LE, Brown CS, Nguyen-Van-Tam JS. Influenza in long-term care facilities. Influenza Other Respir Viruses. 2017;11(5):356-366. https://doi.org/10.1111/irv.12464
13. Sáez-López E, Marques R, Rodrigues N, et al. Lessons learned from a prolonged norovirus GII.P16-GII.4 Sydney 2012 variant outbreak in a long-term care facility in Portugal, 2017. Infect Control Hosp Epidemiol. 2019;40(10):1164-1169. https://doi.org/10.1017/ice.2019.201
14. Gaspard P, Mosnier A, Stoll-Keller F, Roth C, Larocca S, Bertrand X. Influenza prevention in nursing homes: great significance of seasonal variability and spatio-temporal pattern. Presse Med. 2015;44(10):e311-e319. https://doi.org/10.1016/j.lpm.2015.04.041
15. Pfefferbaum B, North CS. Mental health and the Covid-19 pandemic. N Engl J Med. 2020;383(6):510-512. https://doi.org/10.1056/NEJMp2008017
16. Galea S, Merchant RM, Lurie N. The mental health consequences of COVID-19 and physical distancing: the need for prevention and early intervention. JAMA Intern Med. 2020;180(6):817-818. https://doi.org/10.1001/jamainternmed.2020.1562
17. Armitage R, Nellums LB. COVID-19 and the consequences of isolating the elderly. Lancet Public Health. 2020;5(5):e256. https://doi.org/10.1016/s2468-2667(20)30061-x
18. El Haj M, Altintas E, Chapelet G, Kapogiannis D, Gallouj K. High depression and anxiety in people with Alzheimer’s disease living in retirement homes during the covid-19 crisis. Psychiatry Res. 2020;291:113294. https://doi.org/10.1016/j.psychres.2020.113294
19. Santini ZI, Jose PE, York Cornwell E, et al. Social disconnectedness, perceived isolation, and symptoms of depression and anxiety among older Americans (NSHAP): a longitudinal mediation analysis. Lancet Public Health. 2020;5(1):e62-e70. https://doi.org/10.1016/s2468-2667(19)30230-0
20. Gaugler JE, Anderson KA, Zarit SH, Pearlin LI. Family involvement in nursing homes: effects on stress and well-being. Aging Ment Health. 2004;8(1):65-75. https://doi.org/10.1080/13607860310001613356
21. Kim G, Wang M, Pan H, et al. A health system response to COVID-19 in long-term care and post-acute care: a three-phase approach. J Am Geriatr Soc. 2020;68(6):1155-1161. https://doi.org/10.1111/jgs.16513
22. McWilliams JM, Gilstrap LG, Stevenson DG, Chernew ME, Huskamp HA, Grabowski DC. Changes in postacute care in the Medicare Shared Savings Program. JAMA Intern Med. 2017;177(4):518-526. https://doi.org/10.1001/jamainternmed.2016.9115
23. Ackerly DC, Grabowski DC. Post-acute care reform--beyond the ACA. N Engl J Med. 2014;370(8):689-691. https://doi.org/10.1056/NEJMp1315350
24. Strausbaugh LJ, Sukumar SR, Joseph CL. Infectious disease outbreaks in nursing homes: an unappreciated hazard for frail elderly persons. Clin Infect Dis. 2003;36(7):870-876. https://doi.org/10.1086/368197
25. Kapoor A, Field T, Handler S, et al. Adverse events in long-term care residents transitioning from hospital back to nursing home. JAMA Intern Med. 2019;179(9):1254-1261. https://doi.org/10.1001/jamainternmed.2019.2005
26. Adverse Events in Skilled Nursing Facilities: National Incidence Among Medicare Beneficiaries. Office of Inspector General, US Dept of Health & Human Services; 2014.
27. The Impact of the COVID-19 Pandemic on Medicare Beneficiary Use of Health Care Services and Payments to Providers: Early Data for the First 6 Months of 2020. Office of the Assistant Secretary for Planning and Evaluation, US Dept of Health & Human Services; 2020.
1. McMichael TM, Currie DW, Clark S, et al. Epidemiology of Covid-19 in a long-term care facility in King County, Washington. N Engl J Med. 2020;382(21):2005-2011. https://doi.org/10.1056/NEJMoa2005412
2. Ouslander JG, Grabowski DC. COVID-19 in nursing homes: calming the perfect storm. J Am Geriatr Soc. 2020;68(10):2153-2162. https://doi.org/10.1111/jgs.16784
3. CDC COVID-19 Response Team. Severe outcomes among patients with coronavirus disease 2019 (COVID-19) - United States, February 12-March 16, 2020. MMWR Morb Mortal Wkly Rep. 2020;69(12):343-346. https://doi.org/10.15585/mmwr.mm6912e2
4. Ko JY, Danielson ML, Town M, et al. Risk factors for coronavirus disease 2019 (COVID-19)-associated hospitalization: COVID-19-Associated Hospitalization Surveillance Network and Behavioral Risk Factor Surveillance System. Clin Infect Dis. 2020;72(11):e695-e703. https://doi.org/10.1093/cid/ciaa1419
5. Davidson PM, Szanton SL. Nursing homes and COVID-19: we can and should do better. J Clin Nurs. 2020;29(15-16):2758-2759. https://doi.org/10.1111/jocn.15297
6. Dosa D, Jump RLP, LaPlante K, Gravenstein S. Long-term care facilities and the coronavirus epidemic: practical guidelines for a population at highest risk. J Am Med Dir Assoc. 2020;21(5):569-571. https://doi.org/10.1016/j.jamda.2020.03.004
7. Fallon A, Dukelow T, Kennelly SP, O’Neill D. COVID-19 in nursing homes. QJM. 2020;113(6):391-392. https://doi.org/10.1093/qjmed/hcaa136
8. Shah N, Konchak C, Chertok D, et al. Clinical Analytics Prediction Engine (CAPE): development, electronic health record integration and prospective validation of hospital mortality, 180-day mortality and 30-day readmission risk prediction models. PLoS One. 2020;15(8):e0238065. https://doi.org/10.1371/journal.pone.0238065
9. Austin PC. Using the standardized difference to compare the prevalence of a binary variable between two groups in observational research. Commun Stat Simul Comput. 2009;38(6):1228-1234. https://doi.org/10.1080/03610910902859574
10. Rosenbaum PR, Rubin DB. Constructing a control group using multivariate matched sampling methods that incorporate the propensity score. Am Stat. 1985;39(1):33-38. https://doi.org/10.2307/2683903
11. Myers JA, Louis TA. Regression adjustment and stratification by propensity score in treatment effect estimation. Johns Hopkins University, Dept of Biostatistics Working Papers. 2010 203(Working Papers):1-27.
12. Lansbury LE, Brown CS, Nguyen-Van-Tam JS. Influenza in long-term care facilities. Influenza Other Respir Viruses. 2017;11(5):356-366. https://doi.org/10.1111/irv.12464
13. Sáez-López E, Marques R, Rodrigues N, et al. Lessons learned from a prolonged norovirus GII.P16-GII.4 Sydney 2012 variant outbreak in a long-term care facility in Portugal, 2017. Infect Control Hosp Epidemiol. 2019;40(10):1164-1169. https://doi.org/10.1017/ice.2019.201
14. Gaspard P, Mosnier A, Stoll-Keller F, Roth C, Larocca S, Bertrand X. Influenza prevention in nursing homes: great significance of seasonal variability and spatio-temporal pattern. Presse Med. 2015;44(10):e311-e319. https://doi.org/10.1016/j.lpm.2015.04.041
15. Pfefferbaum B, North CS. Mental health and the Covid-19 pandemic. N Engl J Med. 2020;383(6):510-512. https://doi.org/10.1056/NEJMp2008017
16. Galea S, Merchant RM, Lurie N. The mental health consequences of COVID-19 and physical distancing: the need for prevention and early intervention. JAMA Intern Med. 2020;180(6):817-818. https://doi.org/10.1001/jamainternmed.2020.1562
17. Armitage R, Nellums LB. COVID-19 and the consequences of isolating the elderly. Lancet Public Health. 2020;5(5):e256. https://doi.org/10.1016/s2468-2667(20)30061-x
18. El Haj M, Altintas E, Chapelet G, Kapogiannis D, Gallouj K. High depression and anxiety in people with Alzheimer’s disease living in retirement homes during the covid-19 crisis. Psychiatry Res. 2020;291:113294. https://doi.org/10.1016/j.psychres.2020.113294
19. Santini ZI, Jose PE, York Cornwell E, et al. Social disconnectedness, perceived isolation, and symptoms of depression and anxiety among older Americans (NSHAP): a longitudinal mediation analysis. Lancet Public Health. 2020;5(1):e62-e70. https://doi.org/10.1016/s2468-2667(19)30230-0
20. Gaugler JE, Anderson KA, Zarit SH, Pearlin LI. Family involvement in nursing homes: effects on stress and well-being. Aging Ment Health. 2004;8(1):65-75. https://doi.org/10.1080/13607860310001613356
21. Kim G, Wang M, Pan H, et al. A health system response to COVID-19 in long-term care and post-acute care: a three-phase approach. J Am Geriatr Soc. 2020;68(6):1155-1161. https://doi.org/10.1111/jgs.16513
22. McWilliams JM, Gilstrap LG, Stevenson DG, Chernew ME, Huskamp HA, Grabowski DC. Changes in postacute care in the Medicare Shared Savings Program. JAMA Intern Med. 2017;177(4):518-526. https://doi.org/10.1001/jamainternmed.2016.9115
23. Ackerly DC, Grabowski DC. Post-acute care reform--beyond the ACA. N Engl J Med. 2014;370(8):689-691. https://doi.org/10.1056/NEJMp1315350
24. Strausbaugh LJ, Sukumar SR, Joseph CL. Infectious disease outbreaks in nursing homes: an unappreciated hazard for frail elderly persons. Clin Infect Dis. 2003;36(7):870-876. https://doi.org/10.1086/368197
25. Kapoor A, Field T, Handler S, et al. Adverse events in long-term care residents transitioning from hospital back to nursing home. JAMA Intern Med. 2019;179(9):1254-1261. https://doi.org/10.1001/jamainternmed.2019.2005
26. Adverse Events in Skilled Nursing Facilities: National Incidence Among Medicare Beneficiaries. Office of Inspector General, US Dept of Health & Human Services; 2014.
27. The Impact of the COVID-19 Pandemic on Medicare Beneficiary Use of Health Care Services and Payments to Providers: Early Data for the First 6 Months of 2020. Office of the Assistant Secretary for Planning and Evaluation, US Dept of Health & Human Services; 2020.
© 2021 Society of Hospital Medicine
Things We Do for No Reason™: Tumor Markers CA125, CA19-9, and CEA in the Initial Diagnosis of Malignancy
Inspired by the ABIM Foundation’s Choosing Wisely® campaign, the “Things We Do for No Reason™” (TWDFNR) series reviews practices that have become common parts of hospital care but may provide little value to our patients. Practices reviewed in the TWDFNR series do not represent clear-cut conclusions or clinical practice standards but are meant as a starting place for research and active discussions among hospitalists and patients. We invite you to be part of that discussion.
CLINICAL SCENARIO
A 56-year-old woman presents to the emergency department with a 2-week history of abdominal pain associated with nausea and an episode of nonbilious, nonbloody emesis. Her last bowel movement was 2 days prior to her presentation. The patient has tachycardia to 105 beats per minute but otherwise normal vital signs. Findings on her physical examination include dry mucous membranes and increased bowel sounds. A review of systems reveals an unintentional weight loss of 15 kg over the past 4 months and increased fatigue. Computed tomography scan of the abdomen and pelvis with contrast reveals multiple areas of attenuation in the liver and small bowel obstruction. The hospitalist admits the patient to the medicine service for supportive treatment and workup for underlying malignancy. Her admitting team orders serum tumor biomarkers on admission to expedite the diagnosis.
BACKGROUND
When patients present with unexplained weight loss or with metastasis from an unknown primary location, the initial workup often includes imaging and a tumor biomarker panel (eg, cancer antigen 125 [CA125], carbohydrate antigen 19-9 [CA19-9], carcinoembryonic antigen [CEA]). The CA125, CA19-9, and CEA biomarkers are traditionally associated with ovarian, pancreatic, and colorectal cancer, respectively.1 While clinicians initially used these serum biomarkers to monitor for cancer recurrence or treatment response, they have since become widely used in multiple clinical stages of oncological evaluation.
WHY YOU MIGHT THINK CA125, CA19-9, AND CEA ARE HELPFUL IN THE DIAGNOSIS OF CANCER
Hospitalists routinely order biomarkers as part of the malignancy workup. More than a dozen oncology biomarkers are used in the clinical setting to risk stratify, plan treatment, and monitor for recurrence. For example, studies associate elevated preoperative levels of CEA and CA19-9 with metastatic invasion of colorectal2 and gastric3 cancers and with poor prognosis of intrahepatic cholangiocarcinoma. Similarly, CA125 has demonstrated utility in monitoring response to ovarian cancer treatment.4 Specific biomarkers, such as alpha-fetoprotein, improve diagnosis of liver and nonseminomatous testicular tumors.5 Clinicians often apply the same paradigm to other biomarkers due to their widespread availability, noninvasiveness, reproducibility, and ease of use, particularly in acute settings wherein any new information is perceived to be potentially helpful.
WHY YOU SHOULD NOT USE CA125, CA19-9, AND CEA TO DIAGNOSE CANCER
Utilizing these serum biomarkers to diagnose cancer has the potential for diagnostic error and can result in unnecessary patient anxiety and follow-up testing. Since tissue sampling is necessary and remains the gold standard in most cancer diagnoses, obtaining these tumor biomarkers in the early diagnostic stage does not change management and may even lead to harm. Furthermore, due to their poor sensitivity and specificity, these biomarkers cannot rule in or rule out cancer. Elevated CA125, CA19-9, and CEA biomarkers occur in a variety of malignancies, including gastric, gallbladder, hepatocellular, bladder, and breast cancers.1,3,6 In addition, these biomarkers have a very limited role in the workup of cancer of unknown primary origin.7
Even in the setting of a known pelvic mass, the use of CA125 alone has poor sensitivity at a cut-off level of 35 U/mL as a biomarker for the diagnosis of early ovarian cancer.8
Serum CA19-9 is not a useful diagnostic biomarker as elevated CA19-9 can occur in benign conditions, including cirrhosis, chronic pancreatitis, and cholangitis. In a systematic review of patients with histologic confirmation of pancreatic malignancy, the median positive predictive value of CA19-9 was 72% (interquartile range, 41%-95%).9 Additionally, patients with Lewis-null blood type, which is present in 5% to 10% of the Caucasian population, do not produce CA19-9.10 Therefore, CA19-9 will be 0% specific for tumors in this population.
The use of CEA in the diagnosis of colorectal cancer is also questionable. In stage I colorectal cancer, CEA was only 38.1% sensitive at a cut-off level of 2.41 ng/mL; it was 78.3% sensitive in stage IV disease.11 The specificity of CEA is limited since elevated CEA occurs in benign conditions, such as inflammatory bowel disease, smoking, hypothyroidism, pancreatitis, biliary obstruction, peptic ulcers, and cirrhosis—though CEA levels in these conditions are rarely >10 ng/mL.11 Regardless of the results of biomarker testing, definitive diagnosis requires tissue biopsy; therefore, biomarker findings are of little utility in the initial workup.
In addition to variable diagnostic utility, overreliance on these biomarkers has the potential for serious patient harm. In a study examining patients with established rectal cancer, combination CEA and CA19-9 testing alone was insufficient to predict the pathologic stage of disease correctly.2 A cancer misdiagnosis not only traumatizes patients but also erodes their trust in clinicians and creates anxiety during future clinical encounters. Overutilization of these tumor biomarkers is also costly and contributes to waste in the US healthcare system.
WHEN YOU SHOULD USE CA125, CA19-9, AND CEA
There is a role for tumor biomarker testing in specific cancers after the primary source of malignancy has been determined. When evaluating a known pelvic mass, CA125 testing is performed in conjunction with transvaginal ultrasound and assessment of menopausal status in the risk of ovarian malignancy algorithm for prognostication of disease prior to surgery.12 This algorithm takes into account levels of CA125 in addition to levels of human epididymis protein 4 and patient age, yielding an area under the curve as high as 0.93 for ovarian cancer risk classification.8 Beyond the prognostication process, oncologists follow CA125 to monitor response to first-line ovarian cancer treatment. However, CA125 has a less defined role in surveillance for ovarian cancer recurrence.
CA19-9 has demonstrated utility for pancreatic cancer and cholangiocarcinoma survival estimates. A national cohort analysis of patients with established intrahepatic cholangiocarcinoma found that CA19-9 independently predicted increased mortality. Patients with elevated CA19-9 also had significantly more nodal metastases and positive-margin resections.6 A study of 353 patients with pancreatic ductal adenocarcinoma undergoing radical resection further demonstrated the utility of CA19-9. In this study, patients with postoperative CA19-9 normalization had improved survival by almost 12 months when compared to those with consistently elevated CA19-9.13
Last, the literature describes CEA biomarker testing in the surveillance of patients after curative treatment of colon and rectal cancer. The American Society of Colon and Rectal Surgeons recommends regularly tracking this biomarker following curative resection, in conjunction with colonoscopy and chest and liver imaging studies.14 A prospective randomized controlled study that followed this monitoring protocol in cured asymptomatic patients on a bimonthly basis found that early diagnosis of recurrent colorectal cancer improved survival.15 The use of CEA testing as a monitoring tool should therefore be a point of discussion between providers and patients, as its utility varies based on patient comorbidities, their ability to tolerate surgery or chemotherapy, risk factors for recurrence, performance status, compliance, age, and preference.14
WHAT YOU SHOULD DO INSTEAD
The use of CA125, CA19-9, and CEA testing alone as initial diagnostic tools for malignancy are problematic due to their poor sensitivities and/or positive predictive value. Multiple studies have demonstrated their utility as markers of metastasis or malignancy progression rather than as clinically useful markers for the detection of any one type of cancer.1,3,6 In an undiagnosed symptomatic patient with unexplained weight loss or symptoms of a tumor mass, elevated CA125, CA19-9, and CEA add no new information as metastatic pancreatic, colorectal, ovarian, gastric, gallbladder, hepatocellular, bladder, ovarian, and breast cancers all remain in the differential diagnosis. Clinicians should approach the initial diagnosis of cancer in such patients with appropriate imaging studies, a thorough physical examination, and prompt biopsy of abnormal findings, as long as these are consistent with the patient’s goals of care. After establishing a tissue diagnosis, some tumor biomarkers have valid prognostic, staging, and monitoring roles.6,13,14
RECOMMENDATIONS
- Do not routinely order CA125, CA19-9, and CEA tests for the initial diagnostic workup of visceral malignancy of unknown origin regardless of whether imaging studies have been obtained.
- Use appropriate imaging, perform a thorough physical examination, and obtain tissue biopsy in the initial diagnostic workup of a visceral malignancy of unknown origin.
CONCLUSION
Clinicians should use serum biomarkers, like any other diagnostic test, to maximize benefit while preventing patient harm. In general, CA125, CA19-9, and CEA do not have a role in cancer diagnosis. The patient described in our clinical scenario would not benefit from a serum tumor biomarker panel at the time of admission. Regardless of findings from these tests, a tissue sample is required to make a definitive diagnosis of underlying malignancy in this patient.
Do you think this is a low-value practice? Is this truly a “Thing We Do for No Reason™”? Share what you do in your practice and join in the conversation online by retweeting it on Twitter (#TWDFNR) and liking it on Facebook. We invite you to propose ideas for other “Things We Do for No Reason™” topics by emailing [email protected]
1. Yotsukura S, Mamitsuka H. Evaluation of serum-based cancer biomarkers: a brief review from a clinical and computational viewpoint. Crit Rev Oncol Hematol. 2015;93(2):103-115. https://doi.org/10.1016/j.critrevonc.2014.10.002
2. Zhang B, Sun Z, Song M, et al. Ultrasound/CT combined with serum CEA/CA19.9 in the diagnosis and prognosis of rectal cancer. J Buon. 2018;23(3):592-597.
3. Zhou YC, Zhao HJ, Shen LZ. Preoperative serum CEA and CA19-9 in gastric cancer--a single tertiary hospital study of 1,075 cases. Asian Pac J Cancer Prev. 2015;16(7):2685-2691. https://doi.org/10.7314/apjcp.2015.16.7.2685
4. Karam AK, Karlan BY. Ovarian cancer: the duplicity of CA125 measurement. Nat Rev Clin Oncol. 2010;7(6):335-339. https://doi.org/10.1038/nrclinonc.2010.44
5. Gilligan TD, Seidenfeld J, Basch EM, et al; American Society of Clinical Oncology. American Society of Clinical Oncology Clinical Practice Guideline on uses of serum tumor markers in adult males with germ cell tumors. J Clin Oncol. 2010;28(20):3388-3404. https://doi.org/10.1200/jco.2009.26.4481
6. Bergquist JR, Ivanics T, Storlie CB, et al. Implications of CA19-9 elevation for survival, staging, and treatment sequencing in intrahepatic cholangiocarcinoma: a national cohort analysis. J Surg Oncol. 2016;114(4):475-482. https://doi.org/10.1002/jso.24381
7. Milovic M, Popov I, Jelic S. Tumor markers in metastatic disease from cancer of unknown primary origin. Med Sci Monit. 2002;8(2):MT25-MT30.
8. Dochez V, Caillon H, Vaucel E, Dimet J, Winer N. Biomarkers and algorithms for diagnosis of ovarian cancer: CA125, HE4, RMI and ROMA, a review. J Ovarian Res. 2019;12(1):28. https://doi.org/10.1186/s13048-019-0503-7
9. Goonetilleke KS, Siriwardena AK. Systematic review of carbohydrate antigen (CA 19-9) as a biochemical marker in the diagnosis of pancreatic cancer. Eur J Surg Oncol. 2007;33(3):266-270. https://doi.org/10.1016/j.ejso.2006.10.004
10. Loosen SH, Neumann UP, Trautwein C, Roderburg C, Luedde T. Current and future biomarkers for pancreatic adenocarcinoma. Tumour Biol. 2017;39(6):1010428317692231. https://doi.org/10.1177/1010428317692231
11. Polat E, Duman U, Duman M, et al. Diagnostic value of preoperative serum carcinoembryonic antigen and carbohydrate antigen 19-9 in colorectal cancer. Curr Oncol. 2014;21(1):e1-e7. https://doi.org/10.3747/co.21.1711
12. Sölétormos G, Duffy MJ, Othman Abu Hassan S, et al. Clinical use of cancer biomarkers in epithelial ovarian cancer: updated guidelines from the European Group on Tumor Markers. Int J Gynecol Cancer. 2016;26(1):43-51. https://doi.org/10.1097/igc.0000000000000586
13. Xu HX, Liu L, Xiang JF, et al. Postoperative serum CEA and CA125 levels are supplementary to perioperative CA19-9 levels in predicting operative outcomes of pancreatic ductal adenocarcinoma. Surgery. 2017;161(2):373-384. https://doi.org/10.1016/j.surg.2016.08.005
14. Steele SR, Chang GJ, Hendren S, et al. Practice guideline for the surveillance of patients after curative treatment of colon and rectal cancer. Dis Colon Rectum. 2015;58(8):713-725. https://doi.org/10.1097/dcr.0000000000000410
15. Verberne CJ, Zhan Z, van den Heuvel E, et al. Intensified follow-up in colorectal cancer patients using frequent Carcino-Embryonic Antigen (CEA) measurements and CEA-triggered imaging: results of the randomized “CEAwatch” trial. Eur J Surg Oncol. 2015;41(9):1188-1196. https://doi.org/10.1016/j.ejso.2015.06.008
Inspired by the ABIM Foundation’s Choosing Wisely® campaign, the “Things We Do for No Reason™” (TWDFNR) series reviews practices that have become common parts of hospital care but may provide little value to our patients. Practices reviewed in the TWDFNR series do not represent clear-cut conclusions or clinical practice standards but are meant as a starting place for research and active discussions among hospitalists and patients. We invite you to be part of that discussion.
CLINICAL SCENARIO
A 56-year-old woman presents to the emergency department with a 2-week history of abdominal pain associated with nausea and an episode of nonbilious, nonbloody emesis. Her last bowel movement was 2 days prior to her presentation. The patient has tachycardia to 105 beats per minute but otherwise normal vital signs. Findings on her physical examination include dry mucous membranes and increased bowel sounds. A review of systems reveals an unintentional weight loss of 15 kg over the past 4 months and increased fatigue. Computed tomography scan of the abdomen and pelvis with contrast reveals multiple areas of attenuation in the liver and small bowel obstruction. The hospitalist admits the patient to the medicine service for supportive treatment and workup for underlying malignancy. Her admitting team orders serum tumor biomarkers on admission to expedite the diagnosis.
BACKGROUND
When patients present with unexplained weight loss or with metastasis from an unknown primary location, the initial workup often includes imaging and a tumor biomarker panel (eg, cancer antigen 125 [CA125], carbohydrate antigen 19-9 [CA19-9], carcinoembryonic antigen [CEA]). The CA125, CA19-9, and CEA biomarkers are traditionally associated with ovarian, pancreatic, and colorectal cancer, respectively.1 While clinicians initially used these serum biomarkers to monitor for cancer recurrence or treatment response, they have since become widely used in multiple clinical stages of oncological evaluation.
WHY YOU MIGHT THINK CA125, CA19-9, AND CEA ARE HELPFUL IN THE DIAGNOSIS OF CANCER
Hospitalists routinely order biomarkers as part of the malignancy workup. More than a dozen oncology biomarkers are used in the clinical setting to risk stratify, plan treatment, and monitor for recurrence. For example, studies associate elevated preoperative levels of CEA and CA19-9 with metastatic invasion of colorectal2 and gastric3 cancers and with poor prognosis of intrahepatic cholangiocarcinoma. Similarly, CA125 has demonstrated utility in monitoring response to ovarian cancer treatment.4 Specific biomarkers, such as alpha-fetoprotein, improve diagnosis of liver and nonseminomatous testicular tumors.5 Clinicians often apply the same paradigm to other biomarkers due to their widespread availability, noninvasiveness, reproducibility, and ease of use, particularly in acute settings wherein any new information is perceived to be potentially helpful.
WHY YOU SHOULD NOT USE CA125, CA19-9, AND CEA TO DIAGNOSE CANCER
Utilizing these serum biomarkers to diagnose cancer has the potential for diagnostic error and can result in unnecessary patient anxiety and follow-up testing. Since tissue sampling is necessary and remains the gold standard in most cancer diagnoses, obtaining these tumor biomarkers in the early diagnostic stage does not change management and may even lead to harm. Furthermore, due to their poor sensitivity and specificity, these biomarkers cannot rule in or rule out cancer. Elevated CA125, CA19-9, and CEA biomarkers occur in a variety of malignancies, including gastric, gallbladder, hepatocellular, bladder, and breast cancers.1,3,6 In addition, these biomarkers have a very limited role in the workup of cancer of unknown primary origin.7
Even in the setting of a known pelvic mass, the use of CA125 alone has poor sensitivity at a cut-off level of 35 U/mL as a biomarker for the diagnosis of early ovarian cancer.8
Serum CA19-9 is not a useful diagnostic biomarker as elevated CA19-9 can occur in benign conditions, including cirrhosis, chronic pancreatitis, and cholangitis. In a systematic review of patients with histologic confirmation of pancreatic malignancy, the median positive predictive value of CA19-9 was 72% (interquartile range, 41%-95%).9 Additionally, patients with Lewis-null blood type, which is present in 5% to 10% of the Caucasian population, do not produce CA19-9.10 Therefore, CA19-9 will be 0% specific for tumors in this population.
The use of CEA in the diagnosis of colorectal cancer is also questionable. In stage I colorectal cancer, CEA was only 38.1% sensitive at a cut-off level of 2.41 ng/mL; it was 78.3% sensitive in stage IV disease.11 The specificity of CEA is limited since elevated CEA occurs in benign conditions, such as inflammatory bowel disease, smoking, hypothyroidism, pancreatitis, biliary obstruction, peptic ulcers, and cirrhosis—though CEA levels in these conditions are rarely >10 ng/mL.11 Regardless of the results of biomarker testing, definitive diagnosis requires tissue biopsy; therefore, biomarker findings are of little utility in the initial workup.
In addition to variable diagnostic utility, overreliance on these biomarkers has the potential for serious patient harm. In a study examining patients with established rectal cancer, combination CEA and CA19-9 testing alone was insufficient to predict the pathologic stage of disease correctly.2 A cancer misdiagnosis not only traumatizes patients but also erodes their trust in clinicians and creates anxiety during future clinical encounters. Overutilization of these tumor biomarkers is also costly and contributes to waste in the US healthcare system.
WHEN YOU SHOULD USE CA125, CA19-9, AND CEA
There is a role for tumor biomarker testing in specific cancers after the primary source of malignancy has been determined. When evaluating a known pelvic mass, CA125 testing is performed in conjunction with transvaginal ultrasound and assessment of menopausal status in the risk of ovarian malignancy algorithm for prognostication of disease prior to surgery.12 This algorithm takes into account levels of CA125 in addition to levels of human epididymis protein 4 and patient age, yielding an area under the curve as high as 0.93 for ovarian cancer risk classification.8 Beyond the prognostication process, oncologists follow CA125 to monitor response to first-line ovarian cancer treatment. However, CA125 has a less defined role in surveillance for ovarian cancer recurrence.
CA19-9 has demonstrated utility for pancreatic cancer and cholangiocarcinoma survival estimates. A national cohort analysis of patients with established intrahepatic cholangiocarcinoma found that CA19-9 independently predicted increased mortality. Patients with elevated CA19-9 also had significantly more nodal metastases and positive-margin resections.6 A study of 353 patients with pancreatic ductal adenocarcinoma undergoing radical resection further demonstrated the utility of CA19-9. In this study, patients with postoperative CA19-9 normalization had improved survival by almost 12 months when compared to those with consistently elevated CA19-9.13
Last, the literature describes CEA biomarker testing in the surveillance of patients after curative treatment of colon and rectal cancer. The American Society of Colon and Rectal Surgeons recommends regularly tracking this biomarker following curative resection, in conjunction with colonoscopy and chest and liver imaging studies.14 A prospective randomized controlled study that followed this monitoring protocol in cured asymptomatic patients on a bimonthly basis found that early diagnosis of recurrent colorectal cancer improved survival.15 The use of CEA testing as a monitoring tool should therefore be a point of discussion between providers and patients, as its utility varies based on patient comorbidities, their ability to tolerate surgery or chemotherapy, risk factors for recurrence, performance status, compliance, age, and preference.14
WHAT YOU SHOULD DO INSTEAD
The use of CA125, CA19-9, and CEA testing alone as initial diagnostic tools for malignancy are problematic due to their poor sensitivities and/or positive predictive value. Multiple studies have demonstrated their utility as markers of metastasis or malignancy progression rather than as clinically useful markers for the detection of any one type of cancer.1,3,6 In an undiagnosed symptomatic patient with unexplained weight loss or symptoms of a tumor mass, elevated CA125, CA19-9, and CEA add no new information as metastatic pancreatic, colorectal, ovarian, gastric, gallbladder, hepatocellular, bladder, ovarian, and breast cancers all remain in the differential diagnosis. Clinicians should approach the initial diagnosis of cancer in such patients with appropriate imaging studies, a thorough physical examination, and prompt biopsy of abnormal findings, as long as these are consistent with the patient’s goals of care. After establishing a tissue diagnosis, some tumor biomarkers have valid prognostic, staging, and monitoring roles.6,13,14
RECOMMENDATIONS
- Do not routinely order CA125, CA19-9, and CEA tests for the initial diagnostic workup of visceral malignancy of unknown origin regardless of whether imaging studies have been obtained.
- Use appropriate imaging, perform a thorough physical examination, and obtain tissue biopsy in the initial diagnostic workup of a visceral malignancy of unknown origin.
CONCLUSION
Clinicians should use serum biomarkers, like any other diagnostic test, to maximize benefit while preventing patient harm. In general, CA125, CA19-9, and CEA do not have a role in cancer diagnosis. The patient described in our clinical scenario would not benefit from a serum tumor biomarker panel at the time of admission. Regardless of findings from these tests, a tissue sample is required to make a definitive diagnosis of underlying malignancy in this patient.
Do you think this is a low-value practice? Is this truly a “Thing We Do for No Reason™”? Share what you do in your practice and join in the conversation online by retweeting it on Twitter (#TWDFNR) and liking it on Facebook. We invite you to propose ideas for other “Things We Do for No Reason™” topics by emailing [email protected]
Inspired by the ABIM Foundation’s Choosing Wisely® campaign, the “Things We Do for No Reason™” (TWDFNR) series reviews practices that have become common parts of hospital care but may provide little value to our patients. Practices reviewed in the TWDFNR series do not represent clear-cut conclusions or clinical practice standards but are meant as a starting place for research and active discussions among hospitalists and patients. We invite you to be part of that discussion.
CLINICAL SCENARIO
A 56-year-old woman presents to the emergency department with a 2-week history of abdominal pain associated with nausea and an episode of nonbilious, nonbloody emesis. Her last bowel movement was 2 days prior to her presentation. The patient has tachycardia to 105 beats per minute but otherwise normal vital signs. Findings on her physical examination include dry mucous membranes and increased bowel sounds. A review of systems reveals an unintentional weight loss of 15 kg over the past 4 months and increased fatigue. Computed tomography scan of the abdomen and pelvis with contrast reveals multiple areas of attenuation in the liver and small bowel obstruction. The hospitalist admits the patient to the medicine service for supportive treatment and workup for underlying malignancy. Her admitting team orders serum tumor biomarkers on admission to expedite the diagnosis.
BACKGROUND
When patients present with unexplained weight loss or with metastasis from an unknown primary location, the initial workup often includes imaging and a tumor biomarker panel (eg, cancer antigen 125 [CA125], carbohydrate antigen 19-9 [CA19-9], carcinoembryonic antigen [CEA]). The CA125, CA19-9, and CEA biomarkers are traditionally associated with ovarian, pancreatic, and colorectal cancer, respectively.1 While clinicians initially used these serum biomarkers to monitor for cancer recurrence or treatment response, they have since become widely used in multiple clinical stages of oncological evaluation.
WHY YOU MIGHT THINK CA125, CA19-9, AND CEA ARE HELPFUL IN THE DIAGNOSIS OF CANCER
Hospitalists routinely order biomarkers as part of the malignancy workup. More than a dozen oncology biomarkers are used in the clinical setting to risk stratify, plan treatment, and monitor for recurrence. For example, studies associate elevated preoperative levels of CEA and CA19-9 with metastatic invasion of colorectal2 and gastric3 cancers and with poor prognosis of intrahepatic cholangiocarcinoma. Similarly, CA125 has demonstrated utility in monitoring response to ovarian cancer treatment.4 Specific biomarkers, such as alpha-fetoprotein, improve diagnosis of liver and nonseminomatous testicular tumors.5 Clinicians often apply the same paradigm to other biomarkers due to their widespread availability, noninvasiveness, reproducibility, and ease of use, particularly in acute settings wherein any new information is perceived to be potentially helpful.
WHY YOU SHOULD NOT USE CA125, CA19-9, AND CEA TO DIAGNOSE CANCER
Utilizing these serum biomarkers to diagnose cancer has the potential for diagnostic error and can result in unnecessary patient anxiety and follow-up testing. Since tissue sampling is necessary and remains the gold standard in most cancer diagnoses, obtaining these tumor biomarkers in the early diagnostic stage does not change management and may even lead to harm. Furthermore, due to their poor sensitivity and specificity, these biomarkers cannot rule in or rule out cancer. Elevated CA125, CA19-9, and CEA biomarkers occur in a variety of malignancies, including gastric, gallbladder, hepatocellular, bladder, and breast cancers.1,3,6 In addition, these biomarkers have a very limited role in the workup of cancer of unknown primary origin.7
Even in the setting of a known pelvic mass, the use of CA125 alone has poor sensitivity at a cut-off level of 35 U/mL as a biomarker for the diagnosis of early ovarian cancer.8
Serum CA19-9 is not a useful diagnostic biomarker as elevated CA19-9 can occur in benign conditions, including cirrhosis, chronic pancreatitis, and cholangitis. In a systematic review of patients with histologic confirmation of pancreatic malignancy, the median positive predictive value of CA19-9 was 72% (interquartile range, 41%-95%).9 Additionally, patients with Lewis-null blood type, which is present in 5% to 10% of the Caucasian population, do not produce CA19-9.10 Therefore, CA19-9 will be 0% specific for tumors in this population.
The use of CEA in the diagnosis of colorectal cancer is also questionable. In stage I colorectal cancer, CEA was only 38.1% sensitive at a cut-off level of 2.41 ng/mL; it was 78.3% sensitive in stage IV disease.11 The specificity of CEA is limited since elevated CEA occurs in benign conditions, such as inflammatory bowel disease, smoking, hypothyroidism, pancreatitis, biliary obstruction, peptic ulcers, and cirrhosis—though CEA levels in these conditions are rarely >10 ng/mL.11 Regardless of the results of biomarker testing, definitive diagnosis requires tissue biopsy; therefore, biomarker findings are of little utility in the initial workup.
In addition to variable diagnostic utility, overreliance on these biomarkers has the potential for serious patient harm. In a study examining patients with established rectal cancer, combination CEA and CA19-9 testing alone was insufficient to predict the pathologic stage of disease correctly.2 A cancer misdiagnosis not only traumatizes patients but also erodes their trust in clinicians and creates anxiety during future clinical encounters. Overutilization of these tumor biomarkers is also costly and contributes to waste in the US healthcare system.
WHEN YOU SHOULD USE CA125, CA19-9, AND CEA
There is a role for tumor biomarker testing in specific cancers after the primary source of malignancy has been determined. When evaluating a known pelvic mass, CA125 testing is performed in conjunction with transvaginal ultrasound and assessment of menopausal status in the risk of ovarian malignancy algorithm for prognostication of disease prior to surgery.12 This algorithm takes into account levels of CA125 in addition to levels of human epididymis protein 4 and patient age, yielding an area under the curve as high as 0.93 for ovarian cancer risk classification.8 Beyond the prognostication process, oncologists follow CA125 to monitor response to first-line ovarian cancer treatment. However, CA125 has a less defined role in surveillance for ovarian cancer recurrence.
CA19-9 has demonstrated utility for pancreatic cancer and cholangiocarcinoma survival estimates. A national cohort analysis of patients with established intrahepatic cholangiocarcinoma found that CA19-9 independently predicted increased mortality. Patients with elevated CA19-9 also had significantly more nodal metastases and positive-margin resections.6 A study of 353 patients with pancreatic ductal adenocarcinoma undergoing radical resection further demonstrated the utility of CA19-9. In this study, patients with postoperative CA19-9 normalization had improved survival by almost 12 months when compared to those with consistently elevated CA19-9.13
Last, the literature describes CEA biomarker testing in the surveillance of patients after curative treatment of colon and rectal cancer. The American Society of Colon and Rectal Surgeons recommends regularly tracking this biomarker following curative resection, in conjunction with colonoscopy and chest and liver imaging studies.14 A prospective randomized controlled study that followed this monitoring protocol in cured asymptomatic patients on a bimonthly basis found that early diagnosis of recurrent colorectal cancer improved survival.15 The use of CEA testing as a monitoring tool should therefore be a point of discussion between providers and patients, as its utility varies based on patient comorbidities, their ability to tolerate surgery or chemotherapy, risk factors for recurrence, performance status, compliance, age, and preference.14
WHAT YOU SHOULD DO INSTEAD
The use of CA125, CA19-9, and CEA testing alone as initial diagnostic tools for malignancy are problematic due to their poor sensitivities and/or positive predictive value. Multiple studies have demonstrated their utility as markers of metastasis or malignancy progression rather than as clinically useful markers for the detection of any one type of cancer.1,3,6 In an undiagnosed symptomatic patient with unexplained weight loss or symptoms of a tumor mass, elevated CA125, CA19-9, and CEA add no new information as metastatic pancreatic, colorectal, ovarian, gastric, gallbladder, hepatocellular, bladder, ovarian, and breast cancers all remain in the differential diagnosis. Clinicians should approach the initial diagnosis of cancer in such patients with appropriate imaging studies, a thorough physical examination, and prompt biopsy of abnormal findings, as long as these are consistent with the patient’s goals of care. After establishing a tissue diagnosis, some tumor biomarkers have valid prognostic, staging, and monitoring roles.6,13,14
RECOMMENDATIONS
- Do not routinely order CA125, CA19-9, and CEA tests for the initial diagnostic workup of visceral malignancy of unknown origin regardless of whether imaging studies have been obtained.
- Use appropriate imaging, perform a thorough physical examination, and obtain tissue biopsy in the initial diagnostic workup of a visceral malignancy of unknown origin.
CONCLUSION
Clinicians should use serum biomarkers, like any other diagnostic test, to maximize benefit while preventing patient harm. In general, CA125, CA19-9, and CEA do not have a role in cancer diagnosis. The patient described in our clinical scenario would not benefit from a serum tumor biomarker panel at the time of admission. Regardless of findings from these tests, a tissue sample is required to make a definitive diagnosis of underlying malignancy in this patient.
Do you think this is a low-value practice? Is this truly a “Thing We Do for No Reason™”? Share what you do in your practice and join in the conversation online by retweeting it on Twitter (#TWDFNR) and liking it on Facebook. We invite you to propose ideas for other “Things We Do for No Reason™” topics by emailing [email protected]
1. Yotsukura S, Mamitsuka H. Evaluation of serum-based cancer biomarkers: a brief review from a clinical and computational viewpoint. Crit Rev Oncol Hematol. 2015;93(2):103-115. https://doi.org/10.1016/j.critrevonc.2014.10.002
2. Zhang B, Sun Z, Song M, et al. Ultrasound/CT combined with serum CEA/CA19.9 in the diagnosis and prognosis of rectal cancer. J Buon. 2018;23(3):592-597.
3. Zhou YC, Zhao HJ, Shen LZ. Preoperative serum CEA and CA19-9 in gastric cancer--a single tertiary hospital study of 1,075 cases. Asian Pac J Cancer Prev. 2015;16(7):2685-2691. https://doi.org/10.7314/apjcp.2015.16.7.2685
4. Karam AK, Karlan BY. Ovarian cancer: the duplicity of CA125 measurement. Nat Rev Clin Oncol. 2010;7(6):335-339. https://doi.org/10.1038/nrclinonc.2010.44
5. Gilligan TD, Seidenfeld J, Basch EM, et al; American Society of Clinical Oncology. American Society of Clinical Oncology Clinical Practice Guideline on uses of serum tumor markers in adult males with germ cell tumors. J Clin Oncol. 2010;28(20):3388-3404. https://doi.org/10.1200/jco.2009.26.4481
6. Bergquist JR, Ivanics T, Storlie CB, et al. Implications of CA19-9 elevation for survival, staging, and treatment sequencing in intrahepatic cholangiocarcinoma: a national cohort analysis. J Surg Oncol. 2016;114(4):475-482. https://doi.org/10.1002/jso.24381
7. Milovic M, Popov I, Jelic S. Tumor markers in metastatic disease from cancer of unknown primary origin. Med Sci Monit. 2002;8(2):MT25-MT30.
8. Dochez V, Caillon H, Vaucel E, Dimet J, Winer N. Biomarkers and algorithms for diagnosis of ovarian cancer: CA125, HE4, RMI and ROMA, a review. J Ovarian Res. 2019;12(1):28. https://doi.org/10.1186/s13048-019-0503-7
9. Goonetilleke KS, Siriwardena AK. Systematic review of carbohydrate antigen (CA 19-9) as a biochemical marker in the diagnosis of pancreatic cancer. Eur J Surg Oncol. 2007;33(3):266-270. https://doi.org/10.1016/j.ejso.2006.10.004
10. Loosen SH, Neumann UP, Trautwein C, Roderburg C, Luedde T. Current and future biomarkers for pancreatic adenocarcinoma. Tumour Biol. 2017;39(6):1010428317692231. https://doi.org/10.1177/1010428317692231
11. Polat E, Duman U, Duman M, et al. Diagnostic value of preoperative serum carcinoembryonic antigen and carbohydrate antigen 19-9 in colorectal cancer. Curr Oncol. 2014;21(1):e1-e7. https://doi.org/10.3747/co.21.1711
12. Sölétormos G, Duffy MJ, Othman Abu Hassan S, et al. Clinical use of cancer biomarkers in epithelial ovarian cancer: updated guidelines from the European Group on Tumor Markers. Int J Gynecol Cancer. 2016;26(1):43-51. https://doi.org/10.1097/igc.0000000000000586
13. Xu HX, Liu L, Xiang JF, et al. Postoperative serum CEA and CA125 levels are supplementary to perioperative CA19-9 levels in predicting operative outcomes of pancreatic ductal adenocarcinoma. Surgery. 2017;161(2):373-384. https://doi.org/10.1016/j.surg.2016.08.005
14. Steele SR, Chang GJ, Hendren S, et al. Practice guideline for the surveillance of patients after curative treatment of colon and rectal cancer. Dis Colon Rectum. 2015;58(8):713-725. https://doi.org/10.1097/dcr.0000000000000410
15. Verberne CJ, Zhan Z, van den Heuvel E, et al. Intensified follow-up in colorectal cancer patients using frequent Carcino-Embryonic Antigen (CEA) measurements and CEA-triggered imaging: results of the randomized “CEAwatch” trial. Eur J Surg Oncol. 2015;41(9):1188-1196. https://doi.org/10.1016/j.ejso.2015.06.008
1. Yotsukura S, Mamitsuka H. Evaluation of serum-based cancer biomarkers: a brief review from a clinical and computational viewpoint. Crit Rev Oncol Hematol. 2015;93(2):103-115. https://doi.org/10.1016/j.critrevonc.2014.10.002
2. Zhang B, Sun Z, Song M, et al. Ultrasound/CT combined with serum CEA/CA19.9 in the diagnosis and prognosis of rectal cancer. J Buon. 2018;23(3):592-597.
3. Zhou YC, Zhao HJ, Shen LZ. Preoperative serum CEA and CA19-9 in gastric cancer--a single tertiary hospital study of 1,075 cases. Asian Pac J Cancer Prev. 2015;16(7):2685-2691. https://doi.org/10.7314/apjcp.2015.16.7.2685
4. Karam AK, Karlan BY. Ovarian cancer: the duplicity of CA125 measurement. Nat Rev Clin Oncol. 2010;7(6):335-339. https://doi.org/10.1038/nrclinonc.2010.44
5. Gilligan TD, Seidenfeld J, Basch EM, et al; American Society of Clinical Oncology. American Society of Clinical Oncology Clinical Practice Guideline on uses of serum tumor markers in adult males with germ cell tumors. J Clin Oncol. 2010;28(20):3388-3404. https://doi.org/10.1200/jco.2009.26.4481
6. Bergquist JR, Ivanics T, Storlie CB, et al. Implications of CA19-9 elevation for survival, staging, and treatment sequencing in intrahepatic cholangiocarcinoma: a national cohort analysis. J Surg Oncol. 2016;114(4):475-482. https://doi.org/10.1002/jso.24381
7. Milovic M, Popov I, Jelic S. Tumor markers in metastatic disease from cancer of unknown primary origin. Med Sci Monit. 2002;8(2):MT25-MT30.
8. Dochez V, Caillon H, Vaucel E, Dimet J, Winer N. Biomarkers and algorithms for diagnosis of ovarian cancer: CA125, HE4, RMI and ROMA, a review. J Ovarian Res. 2019;12(1):28. https://doi.org/10.1186/s13048-019-0503-7
9. Goonetilleke KS, Siriwardena AK. Systematic review of carbohydrate antigen (CA 19-9) as a biochemical marker in the diagnosis of pancreatic cancer. Eur J Surg Oncol. 2007;33(3):266-270. https://doi.org/10.1016/j.ejso.2006.10.004
10. Loosen SH, Neumann UP, Trautwein C, Roderburg C, Luedde T. Current and future biomarkers for pancreatic adenocarcinoma. Tumour Biol. 2017;39(6):1010428317692231. https://doi.org/10.1177/1010428317692231
11. Polat E, Duman U, Duman M, et al. Diagnostic value of preoperative serum carcinoembryonic antigen and carbohydrate antigen 19-9 in colorectal cancer. Curr Oncol. 2014;21(1):e1-e7. https://doi.org/10.3747/co.21.1711
12. Sölétormos G, Duffy MJ, Othman Abu Hassan S, et al. Clinical use of cancer biomarkers in epithelial ovarian cancer: updated guidelines from the European Group on Tumor Markers. Int J Gynecol Cancer. 2016;26(1):43-51. https://doi.org/10.1097/igc.0000000000000586
13. Xu HX, Liu L, Xiang JF, et al. Postoperative serum CEA and CA125 levels are supplementary to perioperative CA19-9 levels in predicting operative outcomes of pancreatic ductal adenocarcinoma. Surgery. 2017;161(2):373-384. https://doi.org/10.1016/j.surg.2016.08.005
14. Steele SR, Chang GJ, Hendren S, et al. Practice guideline for the surveillance of patients after curative treatment of colon and rectal cancer. Dis Colon Rectum. 2015;58(8):713-725. https://doi.org/10.1097/dcr.0000000000000410
15. Verberne CJ, Zhan Z, van den Heuvel E, et al. Intensified follow-up in colorectal cancer patients using frequent Carcino-Embryonic Antigen (CEA) measurements and CEA-triggered imaging: results of the randomized “CEAwatch” trial. Eur J Surg Oncol. 2015;41(9):1188-1196. https://doi.org/10.1016/j.ejso.2015.06.008
© 2021 Society of Hospital Medicine