User login
Association of Healthcare Access With Intensive Care Unit Utilization and Mortality in Patients of Hispanic Ethnicity Hospitalized With COVID-19
In the United States, health disparities in COVID-19 outcomes (including morbidity and mortality) based on race and ethnicity have been described in the scientific literature and mainstream media.1-7 According to the US Centers for Disease Control and Prevention (CDC), Hispanic people are 3.2 times more likely to be hospitalized with COVID-19 than non-Hispanic White people.8 Further, Hispanic people diagnosed with COVID-19 are 2.3 times more likely to die, adjusted for age, than non-Hispanic White people.9 As the epicenter of the COVID-19 pandemic shifted from the Northeast to the South, the CDC reported that, among people who died from COVID-19 in the United States from May to August 2020, the percentage of Hispanic people increased from 16.3% to 26.4%.10
Published studies on the effect of ethnicity on critical illness or mortality for hospitalized COVID-19 patients are limited and inconsistent. While some studies reported a higher mortality rate for Hispanic patients,11-15 others showed no difference.4,16,17 A recent meta-analysis found that intensive care unit (ICU) utilization and mortality were slightly higher among Hispanic COVID-19 inpatients, but this finding did not reach statistical significance.18 Past studies from different healthcare systems were limited by the small sample size of hospitalized Hispanic patients and the heterogeneity of patients. A comprehensive analysis from a large healthcare system with sufficient sample size is needed to understand the impact of ethnicity on clinical outcomes of hospitalized COVID-19 patients.
Texas Health Resources (THR) is a large integrated healthcare system serving the Dallas-Fort Worth-Arlington (DFW) metropolitan area. According to the 2019 US Census Bureau American Community Survey, Hispanic people comprise 18.4% of the population of this geographic area.19 Congruent with the CDC’s findings, Hispanic patients account for a disproportionate share (32.2%) of hospitalized COVID-19 patients at THR relative to the area’s demographic composition. Aware of the increased risk, we undertook an analysis of the clinical outcomes and the clinical, social, and demographic characteristics of Hispanic patients hospitalized at THR with COVID-19. Our primary goal was to investigate whether clinical outcomes differ by ethnicity among patients hospitalized with COVID-19 and, if so, whether inpatient care or preadmission factors contribute to this difference.
Methods
Study Setting and Overview
We collected data from the single electronic health record (EHR) used by 20 THR hospitals located across the DFW metropolitan area. THR is the largest faith-based, nonprofit health system in North Texas, operating 20 acute care hospitals. Including all access points, such as outpatient facilities and physician group practices, THR serves 7 million residents in 16 counties in North Texas, of whom 16.8% are Hispanic, 73.3% are non-Hispanic, and 9.9% are unclassified, congruent with demographics in the DFW area.
The institutional review boards at THR and UT Southwestern Medical Center approved the study under a waiver of informed consent (as a minimal-risk medical record review). After collection, all data were de-identified prior to statistical analysis.
Cohort, Outcomes, and Covariables
The study cohort included 6097 adult patients with laboratory-confirmed COVID-19 (age ≥18 years) who were admitted as inpatients from March 3 to November 5, 2020. The primary outcomes included ICU utilization and death during hospitalization. We described demographic characteristics using the following variables: age (18–49, 50–64, 65–79, ≥80 years), sex, self-reported ethnicity, and primary spoken language.
We defined a severe baseline condition as an elevated respiratory subscore parsed from the overall MSOFA (Modified Sequential Organ Failure Assessment),20 an elevated Epic Deterioration Index (EDI),21 or an elevated C-reactive protein level (CRP) at baseline (any elevated CRP). Baseline referred to the variable mean during the first available 12-hour window of measurement during the COVID-19 hospital admission, including variables obtained in the emergency department (ED). An elevated MSOFA referred to a score of 4, corresponding to an SpO2/FiO2 < 150. Elevated EDI referred to a baseline EDI > 45. An elevated CRP referred to a baseline CRP > 20 mg/dL.22
Variables reflecting access to healthcare included: THR EHR creation year (representing the first time patients accessed the THR health system), insurance payor type, and presence of a primary care provider (PCP). The federal government established the COVID-19 Claims Reimbursement to Health Care Providers and Facilities for Testing, Treatment, and Vaccine Administration for the Uninsured program. The insurance payor for patients covered by this program is designated as COVID-19 HRSA. Presence of a PCP reflects any documented PCP, regardless of affiliation with THR. We selected these access metrics opportunistically, as they were consistently documented in the EHR and readily available for analysis.
We used 12 variables to describe comorbidities or underlying conditions that, according to the CDC, increased patients’ risk of severe illness from COVID-1923: diagnoses of diabetes, hypertension, obesity, chronic obstructive pulmonary disease (COPD), asthma, smoking, other lung disease, heart failure, kidney disease without end-stage renal disease (ESRD), ESRD, liver disease, and cancer. We identified comorbidities by mining the structured diagnosis codes documented in the EHR prior to and during the COVID-19 admission. Sources for diagnoses included final billed diagnosis codes, working diagnosis codes, problem list, and reason for visit. The definition of diabetes included previously recorded diabetes or baseline hemoglobin A1c > 9%. We also recorded the presence of four major COVID-19 treatments: steroids, remdesivir, tocilizumab, and fresh frozen plasma (FFP) from convalescent patients.24-26 Each treatment variable was defined by receipt of one or more doses.
Statistical Analysis
To analyze patient outcomes based on ethnicity, we divided the study cohort into a Hispanic group and a non-Hispanic group based on self-reported ethnicity in the EHR. To study the potential impact of primary language among Hispanic patients, we divided them into English-speaking and non-English-speaking patients based on their self-reported primary language. As a result, we analyzed three groups of patients: (1) non-Hispanic, (2) Hispanic and English speaking, and (3) Hispanic and non-English speaking. We tested differences of a given categorical variable across the three groups using the chi-square test for each age subgroup (18–49, 50–64, 65–79, ≥80 years). The Cochran-Mantel-Haenszel test was used for the overall difference adjusted for age. To assess whether an observed disparity in treatment existed across the three groups, we tested the difference in the administration of four major therapeutics for COVID-19, including steroids, remdesivir, tocilizumab, and convalescent plasma. To determine whether any groups had elevated disease severity at hospital admission (baseline), we tested the difference in four disease-severity metrics across the ethnic-language groups: (1) elevated respiratory MSOFA score, (2) elevated EDI, (3) elevated CRP level, and (4) any of the three conditions.
To study the associations with ICU utilization and death, respectively, we performed a multivariable analysis using a generalized linear mixed model with binomial distribution and a logit link function. In each analysis model, the hospital of admission was included as a random-effect variable to account for the potential treatment variations among different hospitals, while other variables were regarded as fixed effects. In the first multivariable analysis (Model 1), all demographic variables, including age, sex, and ethnicity, and different types of comorbidities and underlying conditions, were included as fixed-effect variables in the initial model, and then backward stepwise variable selection was performed to establish the final model (Model 1). We performed the backward stepwise variable selection separately for the outcome of ICU use or mortality. Based on Akaike information criterion (AIC), during each iteration the fixed-effect variable that led to the largest decrease in the AIC value was removed, and the variable selection process was completed when the AIC value stopped decreasing. In Model 2, we added the disease-severity variable at baseline to the selected variable set derived from Model 1 to explore its effect on the associations between ethnicity and clinical outcomes. In Model 3, we added healthcare access–related variables, including first-time healthsystem access, payor type, and PCP availability to Model 2. We performed all statistical analyses using R, version 4.0.2 (R Foundation for Statistical Computing) in RStudio (version 1.3.1093).
Results
Distinct Demographic and Comorbidity Patterns for Three Ethnic-Language Groups
We identified 6097 adult patients (age ≥18 years) who had confirmed COVID-19 disease and were hospitalized between March 3 and November 5, 2020. Demographic characteristics and comorbidity for these patients are summarized in Table 1. Among these patients, 4139 (67.9%) were non-Hispanic and 1958 (32.1%) were Hispanic. Among the Hispanic patients, 1203 (61.4%) identified English as their primary language and 755 (38.6%) identified a non-English primary language. Age distribution was vastly different among the three ethnic-language groups (Table 1). Unlike the relatively balanced distribution across different age groups in the non-Hispanic group, more than half (55.8%) of the English-speaking Hispanic patients were in the youngest age group (18-49 years). A much lower fraction of Hispanic patients was among the oldest (≥80 years) age group (P < .001). Because COVID-19 clinical outcome is strongly associated with age,27 we used age-stratified analysis when comparing group-level differences in patient outcomes.
Sex distribution also was different among the three groups, with the non-English-speaking Hispanic group having more male patients (53.0%). Diabetes and obesity, which are associated with clinical outcomes of COVID-19 patients, were more prevalent in Hispanic patients (Table 1). Non-English-speaking Hispanic patients had the highest diabetes rate (48.7% with documented diabetes; 15.8% with baseline HbA1c > 9%; P < .001). English-speaking Hispanic patients presented with the highest obesity rate (62.8%; P < .001). Appendix Table 1 provides detailed age-group-specific comorbidity distributions among ethnic-language groups.
Patients of Hispanic Ethnicity Experienced a Higher Rate of ICU Utilization and Mortality
Of the 6097 patients overall, 1365 (22.4%) were admitted to the ICU and 543 (8.9%) died in hospital. For non-Hispanic patients (n = 4139), 883 (21.3%) were admitted to the ICU and 373 (9.0%) died in hospital. For English-speaking Hispanic patients (n = 1203), 241 (20.0%) were admitted to the ICU and 91 (7.6%) died in hospital. For non-English-speaking Hispanic patients (n = 755), 241 (31.9%) were admitted to the ICU and 79 (10.5%) died in hospital. Figure 1 summarizes the age-stratified comparison of ICU utilization and mortality across the three ethnic-language patient groups. In all age groups, non-English-speaking Hispanic patients experienced a significantly higher ICU utilization rate compared to non-Hispanic patients (age-adjusted OR, 1.75; 95% CI, 1.47-2.08; P < .001). English-speaking and non-English-speaking Hispanic patients had a significantly higher mortality rate compared to non-Hispanic patients (age-adjusted OR, 1.53; 95% CI, 1.19-1.98; P = .001 for English-speaking Hispanic patients; age-adjusted OR, 1.43; 95% CI,: 1.10-1.86; P = .01 for non-English-speaking Hispanic patients).
To delineate the risk factors associated with ICU utilization and death, we performed multivariable logistic regression with stepwise variable selection. After adjusting for age, sex, and comorbidity (Model 1), the factors ethnicity and primary language were still strongly associated with ICU utilization and mortality (Appendix Table 2). Non-English-speaking Hispanic patients had an OR of 1.74 (95% CI, 1.41-2.15; P < .001) for ICU utilization and an OR of 1.54 (95% CI, 1.12-2.12; P = .008) for mortality compared to non-Hispanic patients. Similarly, English-speaking Hispanic patients had higher ICU utilization (OR, 1.28; 95% CI, 1.05-1.55; P = .01) and a higher mortality rate (OR, 1.60; 95% CI, 1.19-2.14; P = .002).
No Disparity in COVID-19 Therapeutics Observed Across Three Ethnic-Language Groups
Appendix Figure 1 summarizes the comparison of the administration of four major treatments across the three ethnic-language groups. We did not observe any underuse of COVID-19 therapeutics for Hispanic patients. Usage rates for these therapies were significantly higher, after adjusting for age, in Hispanic groups when compared to non-Hispanic patients (OR ranged from 1.21 to 1.96). Steroids were the most common treatment in all patient groups. Tocilizumab was used almost twice as frequently (OR, 1.96; 95% CI, 1.64-2.33; P < .001) in non-English-speaking Hispanic patients compared to non-Hispanic patients.
Patients of Hispanic Ethnicity Had More Severe Disease at Hospital Admission
Figure 2 shows that non-English-speaking Hispanic patients had a higher rate of severe illness at admission based on each of these metrics: high respiratory MSOFA score (OR, 2.43; 95% CI, 1.77-3.33; P < .001), high EDI (OR, 1.85; 95% CI, 1.41-2.41; P < .001), and high CRP level (OR, 2.06; 95% CI, 1.64-2.58; P < .001). English-speaking Hispanic patients also had a greater rate of high CRP level (OR, 1.48; 95% CI, 1.17-1.86; P = .001) compared to non-Hispanic patients. When considering the presentation of any one of these clinical indicators, the English-speaking and non-English-speaking Hispanic patients had a higher rate of severe baseline condition (OR, 1.33; 95% CI, 1.10-1.61; P = .004 for English-speaking patients; OR, 2.27; 95% CI, 1.89-2.72; P < .001 for non-English-speaking patients).
We then studied how the baseline disease condition affects the association between ethnicity and clinical outcomes. We performed a multivariable analysis including baseline disease severity as a covariable (Model 2, Table 2), which showed that baseline disease severity was strongly associated with ICU admission (OR, 4.52; 95% CI, 3.83-5.33; P < .001) and mortality (OR, 3.32; 95% CI, 2.67-4.13; P < .001). The associations between ethnicity and clinical outcomes were reduced after considering the baseline disease condition. The OR dropped to 1.47 (95% CI, 1.18-1.84; P < .001) and 1.34 (95% CI, 0.97-1.87; P = .08) for ICU utilization and mortality, respectively, when comparing non-English-speaking Hispanic patients to non-Hispanic patients. A similar reduction was observed for English-speaking Hispanic patients. Model comparison showed a significant improvement of Model 2 over Model 1 based on ANOVA test (P < .001) as well as AIC.
Hispanic Patients Had Worse Healthcare Access
To explore the etiology for the more severe disease conditions at hospital admission among Hispanic patients, we analyzed variables related to healthcare access. We found that Hispanic patients were likely to have reduced access to healthcare (Table 1; Appendix Figure 2). For a large proportion (16.9%) of the COVID-19 patients in this study, their medical records were first created at THR in 2020, corresponding to the initial time these patients accessed THR for their healthcare. This surge in 2020, compared to previous years with data (2005–2019), corresponds to the number of new patients seen because of COVID-19 (Appendix Figure 2A). Among this new patient population, the proportion of non-English-speaking Hispanic patients in 2020 was 28.3%, compared to 9.1% from 2005 to 2019 (P < .001). The proportion of new English-speaking Hispanic patients in 2020 was 22.1%, compared to an average of 19.2% from 2005 to 2019 (P < .001). In addition, a much smaller proportion of Hispanic patients had a PCP (P < .001) (Table 1; Appendix Figure 2B), with non-English-speaking Hispanic patients having the smallest proportion (58.5%).
Appendix Figure 2C illustrates the comparison of payor types across the three patient groups. A much higher proportion of Hispanic patients used COVID-19 HRSA (P < .001) compared to non-Hispanic patients. Breaking this down further by primary language, 29.1% of non-English-speaking Hispanic patients relied on COVID-19 HRSA due to otherwise uninsured status, compared to 12.7% of English-speaking Hispanic patients and only 5.1% of non-Hispanic patients. Similarly, non-English-speaking Hispanic patients have the highest self-pay rates (2.3%) compared to English-speaking Hispanic patients (1.4%) and non-Hispanic patients (0.7%). In summary, more Hispanic patients, and especially non-English-speaking Hispanic patients, lacked conventional health insurance and experienced limited access to healthcare.
Further evidence showed a trend of correlation between presentation of severe COVID-19 conditions when arriving at the hospital and each of the healthcare access factors analyzed (Appendix Figure 3).
Discussion
With a large sample size of hospitalized COVID-19 patients at an integrated health system in the DFW metropolitan area, we observed an increased rate of ICU utilization and mortality among Hispanic inpatients. After adjusting for age, we found that non-English-speaking Hispanic patients were 75% more likely to require critical care compared with non-Hispanic patients. English-speaking and non-English-speaking Hispanic patients had an increased mortality rate (age-adjusted) compared to non-Hispanic patients. The association between ethnicity and clinical outcomes remained significant after adjusting for age, sex, and comorbidities. We did not observe any underuse of major COVID-19 therapeutics in Hispanic patients, and excluded in-hospital treatments from the contributors to the outcome differences.
Hispanic patients, especially non-English-speaking Hispanic patients, had a higher rate of severe COVID-19 disease at the time of hospital admission (Figure 2). After including baseline disease severity into the multivariable analysis (Model 2), the overall model improved (P < .001) while the associations between ethnicity and outcomes decreased (Table 2). This suggests disease severity at admission was a main contributor to the observed associations between ethnicity and clinical outcomes. The higher rate of baseline COVID-19 severity in Hispanic patients might also explain their higher rate of receiving major COVID-19 therapeutics (Appendix Figure 1).
This study found that Hispanic patients were less likely to have a PCP and insurance coverage compared with non-Hispanic patients (P < .001). This disparity was more pronounced among non-English-speaking Hispanic patients (Appendix Figure 2). We also observed that a disproportionately larger proportion (50.4%) of patients who visited the healthcare system for the first time in 2020 (the year of the COVID-19 pandemic) was composed of Hispanic patients, compared to merely 28.4% prior to 2020. While there is a possibility that patients had primary care outside THR, the staggering number of Hispanic patients who were new to the health system in 2020, in conjunction with the fact that immigrants tend to be “healthier” compared to their native-born peers (the so-called immigrant paradox),28 led us to conclude that there were few other primary care options for these patients, making THR’s ED the primary care option of choice. The systemic, structural barriers to routine care might be a possible cause for delayed admission and, in turn, elevated baseline COVID-19 severity for Hispanic patients (Appendix Figure 3).
Recent studies have investigated the impact of socioeconomic factors on racial/ethnic disparities in the COVID-19 pandemic.7,16,17 To our knowledge, no study has directly analyzed the link between healthcare access metrics, COVID-19 severity at admission, and the Hispanic population stratified by primary language. Studies exist on this subject for other diseases, however. For example, healthcare access factors have been associated with sepsis-related mortality.29,30 In fact, a recent study that explored the potential effect of language barriers on healthcare access demonstrated an association between limited English proficiency and sepsis-related mortality.31 Our study found that Hispanic patients whose primary language is not English had the worst clinical outcomes, including more severe baseline COVID-19 conditions, and the least access to healthcare, highlighting the importance of addressing language barriers in COVID-19 care. Further research is needed to confirm the relationship between limited English proficiency and clinical outcomes, as well as potential factors that contribute to such a relationship in different types of diseases.
Our study has a number of limitations. First, it was limited to only one large healthcare system, which means the results may not be generalizable. Because THR is an open system, comorbidity data may be incomplete, and we cannot exclude the possibility that patients accessed care outside THR prior to or during the pandemic. We may overcome this limitation in the future with cross-system health information exchange data. Second, we did not have data for the time of symptom onset, so we were unable to analyze the direct evidence of the possible delayed care. As a result, we were unable to analyze whether treatments were administered in a timely manner or appropriately. Third, our analysis was not adjusted for other socioeconomic factors (eg, income, education) due to lack of data. We used self-identification for ethnicity, but unlike new approaches by the U.S. Census Bureau,32 our survey allowed only one choice to be selected.
Conclusion
Sociodemographic factors among Hispanic inpatients hospitalized for COVID-19 at a large integrated health system—including a primary non-English language, lack of a PCP, and insurance status—were associated with measures of reduced access to care and more severe illness at admission. Structural barriers to care, which may be associated with reduced health literacy and less access to health insurance, can result in delayed treatment and more severe illness at admission and underdiagnosis of medical conditions, contributing to worse outcomes in this population. Our findings suggest that interventions to promote early recognition of signs and symptoms of COVID-19 and to encourage prompt clinical care at the community level may reduce the burden of COVID-19 deaths in racial or ethnic minority communities with language and socioeconomic barriers.
1. Lopez L III, Hart LH III, Katz MH. Racial and ethnic health disparities related to COVID-19. JAMA. 2021;325(8):719-720. https://doi.org/10.1001/jama.2020.26443
2. Cooper LA, Williams DR. Excess deaths from COVID-19, community bereavement, and restorative justice for communities of color. JAMA. 2020;324(15):1491-1492. https://doi.org/10.1001/jama.2020.19567
3. Clay LA, Rogus S. Primary and secondary health impacts of COVID-19 among minority individuals in New York State. Int J Environ Res Public Health. 2021;18(2):683. https://doi.org/10.3390/ijerph18020683
4. Rodriguez F, Solomon N, de Lemos JA, et al. Racial and ethnic differences in presentation and outcomes for patients hospitalized with COVID-19: findings from the American Heart Association’s COVID-19 Cardiovascular Disease Registry. Circulation. 2021;143(24):2332-2342. https://doi.org/10.1161/CIRCULATIONAHA.120.052278
5. Moreira A, Chorath K, Rajasekaran K, Burmeister F, Ahmed M, Moreira A. Demographic predictors of hospitalization and mortality in US children with COVID-19. Eur J Pediatr. 2021;180(5):1659-1663. https://doi.org/10.1007/s00431-021-03955-x
6. Kolata G. Social inequities explain racial gaps in pandemic, studies find. The New York Times. December 9, 2020. https://www.nytimes.com/2020/12/09/health/coronavirus-black-hispanic.html
7. Liao TF, De Maio F. Association of social and economic inequality with coronavirus disease 2019 incidence and mortality across US counties. JAMA Netw Open. 2021;4(1):e2034578. https://doi.org/10.1001/jamanetworkopen.2020.34578
8. Centers for Disease Control and Prevention. A Weekly Surveillance Summary of U.S. COVID-19 Activity: Key Updates for Week 2. January 21, 2021. https://www.cdc.gov/coronavirus/2019-ncov/covid-data/pdf/covidview-01-22-2021.pdf
9. Centers for Disease Control and Prevention. Risk for COVID-19 infection, hospitalization, and death by race/ethnicity. Updated September 9, 2021. https://www.cdc.gov/coronavirus/2019-ncov/covid-data/investigations-discovery/hospitalization-death-by-race-ethnicity.html
10. Gold JAW, Rossen LM, Ahmad FB, et al. Race, ethnicity, and age trends in persons who died from COVID-19 – United States, May-August 2020. MMWR Morb Mortal Wkly Rep. 2020;69(42):1517-1521. https://doi.org/10.15585/mmwr.mm6942e1
11. Pennington AF, Kompaniyets L, Summers AD, et al. Risk of clinical severity by age and race/ethnicity among adults hospitalized for COVID-19 – United States, March-September 2020. Open Forum Infect Dis. 2021;8(2):ofaa638. https://doi.org/10.1093/ofid/ofaa638.
12. Renelus BD, Khoury NC, Chandrasekaran K, et al. Racial disparities in COVID-19 hospitalization and in-hospital mortality at the height of the New York City pandemic. J Racial Ethn Health Disparities. 2021;8(5):1161-1167. https://doi.org/10.1007/s40615-020-00872-x
13. Wiley Z, Ross-Driscoll K, Wang Z, Smothers L, Mehta AK, Patzer RE. Racial and ethnic differences and clinical outcomes of COVID-19 patients presenting to the emergency department. Clin Infect Dis. 2021 Apr 2. [Epub ahead of print] https://doi.org/10.1093/cid/ciab290
14. Dai CL, Kornilov SA, Roper RT, et al. Characteristics and factors associated with COVID-19 infection, hospitalization, and mortality across race and ethnicity. Clin Infect Dis. 2021 Feb 20. [Epub ahead of print] https://doi.org/10.1093/cid/ciab154
15. Pan AP, Khan O, Meeks JR, et al. Disparities in COVID-19 hospitalizations and mortality among black and Hispanic patients: cross-sectional analysis from the greater Houston metropolitan area. BMC Public Health. 2021;21(1):1330. https://doi.org/10.1186/s12889-021-11431-2
16. Ogedegbe G, Ravenell J, Adhikari S, et al. Assessment of racial/ethnic disparities in hospitalization and mortality in patients with COVID-19 in New York City. JAMA Netw Open. 2020;3(12):e2026881. https://doi.org/10.1001/jamanetworkopen.2020.26881
17. Gershengorn HB, Patel S, Shukla B, et al. Association of race and ethnicity with COVID-19 test positivity and hospitalization is mediated by socioeconomic factors. Ann Am Thorac Soc. 2021;18(8):1326-1334. https://doi.org/10.1513/AnnalsATS.202011-1448OC
18. Sze S, Pan D, Nevill CR, et al. Ethnicity and clinical outcomes in COVID-19: a systematic review and meta-analysis. EClinicalMedicine. 2020;29:100630. https://doi.org/10.1016/j.eclinm.2020.100630
19. U.S. Census Bureau. 2019 U.S Census Bureau American Community Survey. https://www.census.gov/programs-surveys/acs
20. North Texas Mass Critical Care Task Force. North Texas Mass Critical Care Guidelines Document. Hospital and ICU Triage Guidelines for ADULTS. January 2014. https://www.dallas-cms.org/tmaimis/dcms/assets/files/communityhealth/MCC/GuidelinesAdult_JAN2014.pdf
21. Singh K, Valley TS, Tang S, et al. Evaluating a widely implemented proprietary deterioration index model among hospitalized COVID-19 patients. Ann Am Thorac Soc. 2021;18(7):1129-1137. https://doi.org/10.1513/AnnalsATS.202006-698OC
22. Keller MJ, Kitsis EA, Arora S, et al. Effect of systemic glucocorticoids on mortality or mechanical ventilation in patients with COVID-19. J Hosp Med. 2020;15(8):489-493. https://doi.org/10.12788/jhm.3497
23. Centers for Disease Control and Prevention. Science Brief: Evidence used to update the list of underlying medical conditions that increase a person’s risk of severe illness from COVID-19. Updated May 12, 2021. https://www.cdc.gov/coronavirus/2019-ncov/science/science-briefs/underlying-evidence-table.html
24. Gupta S, Wang W, Hayek SS, et al. Association between early treatment with tocilizumab and mortality among critically ill patients with COVID-19. JAMA Intern Med. 2021;181(1):41-51. https://doi.org/10.1001/jamainternmed.2020.6252
25. Baroutjian A, Sanchez C, Boneva D, McKenney M, Elkbuli A. SARS-CoV-2 pharmacologic therapies and their safety/effectiveness according to level of evidence. Am J Emerg Med. 2020;38(11):2405-2415. https://doi.org/10.1016/j.ajem.2020.08.091
26. Janiaud P, Axfors C, Schmitt AM, et al. Association of convalescent plasma treatment with clinical outcomes in patients with COVID-19: a systematic review and meta-analysis. JAMA. 2021;325(12):1185-1195. https://doi.org/10.1001/jama.2021.2747
27. Panagiotou OA, Kosar CM, White EM, et al. Risk factors associated with all-cause 30-day mortality in nursing home residents with COVID-19. JAMA Intern Med. 2021;181(4):439-448. https://doi.org/10.1001/jamainternmed.2020.7968
28. Bacong AM, Menjívar C. Recasting the immigrant health paradox through intersections of legal status and race. J Immigr Minor Health. 2021;23(5):1092-1104. https://doi.org/10.1007/s10903-021-01162-2
29. Plopper GE, Sciarretta KL, Buchman TG. Disparities in sepsis outcomes may be attributable to access to care. Crit Care Med. 2021;49(8):1358-1360. https://doi.org/10.1097/CCM.0000000000005126
30. Jones JM, Fingar KR, Miller MA, et al. Racial disparities in sepsis-related in-hospital mortality: using a broad case capture method and multivariate controls for clinical and hospital variables, 2004-2013. Crit Care Med. 2017;45(12):e1209-e1217. https://doi.org/10.1097/CCM.0000000000002699
31. Jacobs ZG, Prasad PA, Fang MC, Abe-Jones Y, Kangelaris KN. The association between limited English proficiency and sepsis mortality. J Hosp Med. 2019;14:E1-E7. https://doi.org/10.12788/jhm.3334
32. Cohn D. Census considers new approach to asking about race – by not using the term at all. June 18, 2015. https://www.pewresearch.org/fact-tank/2015/06/18/census-considers-new-approach-to-asking-about-race-by-not-using-the-term-at-all/
In the United States, health disparities in COVID-19 outcomes (including morbidity and mortality) based on race and ethnicity have been described in the scientific literature and mainstream media.1-7 According to the US Centers for Disease Control and Prevention (CDC), Hispanic people are 3.2 times more likely to be hospitalized with COVID-19 than non-Hispanic White people.8 Further, Hispanic people diagnosed with COVID-19 are 2.3 times more likely to die, adjusted for age, than non-Hispanic White people.9 As the epicenter of the COVID-19 pandemic shifted from the Northeast to the South, the CDC reported that, among people who died from COVID-19 in the United States from May to August 2020, the percentage of Hispanic people increased from 16.3% to 26.4%.10
Published studies on the effect of ethnicity on critical illness or mortality for hospitalized COVID-19 patients are limited and inconsistent. While some studies reported a higher mortality rate for Hispanic patients,11-15 others showed no difference.4,16,17 A recent meta-analysis found that intensive care unit (ICU) utilization and mortality were slightly higher among Hispanic COVID-19 inpatients, but this finding did not reach statistical significance.18 Past studies from different healthcare systems were limited by the small sample size of hospitalized Hispanic patients and the heterogeneity of patients. A comprehensive analysis from a large healthcare system with sufficient sample size is needed to understand the impact of ethnicity on clinical outcomes of hospitalized COVID-19 patients.
Texas Health Resources (THR) is a large integrated healthcare system serving the Dallas-Fort Worth-Arlington (DFW) metropolitan area. According to the 2019 US Census Bureau American Community Survey, Hispanic people comprise 18.4% of the population of this geographic area.19 Congruent with the CDC’s findings, Hispanic patients account for a disproportionate share (32.2%) of hospitalized COVID-19 patients at THR relative to the area’s demographic composition. Aware of the increased risk, we undertook an analysis of the clinical outcomes and the clinical, social, and demographic characteristics of Hispanic patients hospitalized at THR with COVID-19. Our primary goal was to investigate whether clinical outcomes differ by ethnicity among patients hospitalized with COVID-19 and, if so, whether inpatient care or preadmission factors contribute to this difference.
Methods
Study Setting and Overview
We collected data from the single electronic health record (EHR) used by 20 THR hospitals located across the DFW metropolitan area. THR is the largest faith-based, nonprofit health system in North Texas, operating 20 acute care hospitals. Including all access points, such as outpatient facilities and physician group practices, THR serves 7 million residents in 16 counties in North Texas, of whom 16.8% are Hispanic, 73.3% are non-Hispanic, and 9.9% are unclassified, congruent with demographics in the DFW area.
The institutional review boards at THR and UT Southwestern Medical Center approved the study under a waiver of informed consent (as a minimal-risk medical record review). After collection, all data were de-identified prior to statistical analysis.
Cohort, Outcomes, and Covariables
The study cohort included 6097 adult patients with laboratory-confirmed COVID-19 (age ≥18 years) who were admitted as inpatients from March 3 to November 5, 2020. The primary outcomes included ICU utilization and death during hospitalization. We described demographic characteristics using the following variables: age (18–49, 50–64, 65–79, ≥80 years), sex, self-reported ethnicity, and primary spoken language.
We defined a severe baseline condition as an elevated respiratory subscore parsed from the overall MSOFA (Modified Sequential Organ Failure Assessment),20 an elevated Epic Deterioration Index (EDI),21 or an elevated C-reactive protein level (CRP) at baseline (any elevated CRP). Baseline referred to the variable mean during the first available 12-hour window of measurement during the COVID-19 hospital admission, including variables obtained in the emergency department (ED). An elevated MSOFA referred to a score of 4, corresponding to an SpO2/FiO2 < 150. Elevated EDI referred to a baseline EDI > 45. An elevated CRP referred to a baseline CRP > 20 mg/dL.22
Variables reflecting access to healthcare included: THR EHR creation year (representing the first time patients accessed the THR health system), insurance payor type, and presence of a primary care provider (PCP). The federal government established the COVID-19 Claims Reimbursement to Health Care Providers and Facilities for Testing, Treatment, and Vaccine Administration for the Uninsured program. The insurance payor for patients covered by this program is designated as COVID-19 HRSA. Presence of a PCP reflects any documented PCP, regardless of affiliation with THR. We selected these access metrics opportunistically, as they were consistently documented in the EHR and readily available for analysis.
We used 12 variables to describe comorbidities or underlying conditions that, according to the CDC, increased patients’ risk of severe illness from COVID-1923: diagnoses of diabetes, hypertension, obesity, chronic obstructive pulmonary disease (COPD), asthma, smoking, other lung disease, heart failure, kidney disease without end-stage renal disease (ESRD), ESRD, liver disease, and cancer. We identified comorbidities by mining the structured diagnosis codes documented in the EHR prior to and during the COVID-19 admission. Sources for diagnoses included final billed diagnosis codes, working diagnosis codes, problem list, and reason for visit. The definition of diabetes included previously recorded diabetes or baseline hemoglobin A1c > 9%. We also recorded the presence of four major COVID-19 treatments: steroids, remdesivir, tocilizumab, and fresh frozen plasma (FFP) from convalescent patients.24-26 Each treatment variable was defined by receipt of one or more doses.
Statistical Analysis
To analyze patient outcomes based on ethnicity, we divided the study cohort into a Hispanic group and a non-Hispanic group based on self-reported ethnicity in the EHR. To study the potential impact of primary language among Hispanic patients, we divided them into English-speaking and non-English-speaking patients based on their self-reported primary language. As a result, we analyzed three groups of patients: (1) non-Hispanic, (2) Hispanic and English speaking, and (3) Hispanic and non-English speaking. We tested differences of a given categorical variable across the three groups using the chi-square test for each age subgroup (18–49, 50–64, 65–79, ≥80 years). The Cochran-Mantel-Haenszel test was used for the overall difference adjusted for age. To assess whether an observed disparity in treatment existed across the three groups, we tested the difference in the administration of four major therapeutics for COVID-19, including steroids, remdesivir, tocilizumab, and convalescent plasma. To determine whether any groups had elevated disease severity at hospital admission (baseline), we tested the difference in four disease-severity metrics across the ethnic-language groups: (1) elevated respiratory MSOFA score, (2) elevated EDI, (3) elevated CRP level, and (4) any of the three conditions.
To study the associations with ICU utilization and death, respectively, we performed a multivariable analysis using a generalized linear mixed model with binomial distribution and a logit link function. In each analysis model, the hospital of admission was included as a random-effect variable to account for the potential treatment variations among different hospitals, while other variables were regarded as fixed effects. In the first multivariable analysis (Model 1), all demographic variables, including age, sex, and ethnicity, and different types of comorbidities and underlying conditions, were included as fixed-effect variables in the initial model, and then backward stepwise variable selection was performed to establish the final model (Model 1). We performed the backward stepwise variable selection separately for the outcome of ICU use or mortality. Based on Akaike information criterion (AIC), during each iteration the fixed-effect variable that led to the largest decrease in the AIC value was removed, and the variable selection process was completed when the AIC value stopped decreasing. In Model 2, we added the disease-severity variable at baseline to the selected variable set derived from Model 1 to explore its effect on the associations between ethnicity and clinical outcomes. In Model 3, we added healthcare access–related variables, including first-time healthsystem access, payor type, and PCP availability to Model 2. We performed all statistical analyses using R, version 4.0.2 (R Foundation for Statistical Computing) in RStudio (version 1.3.1093).
Results
Distinct Demographic and Comorbidity Patterns for Three Ethnic-Language Groups
We identified 6097 adult patients (age ≥18 years) who had confirmed COVID-19 disease and were hospitalized between March 3 and November 5, 2020. Demographic characteristics and comorbidity for these patients are summarized in Table 1. Among these patients, 4139 (67.9%) were non-Hispanic and 1958 (32.1%) were Hispanic. Among the Hispanic patients, 1203 (61.4%) identified English as their primary language and 755 (38.6%) identified a non-English primary language. Age distribution was vastly different among the three ethnic-language groups (Table 1). Unlike the relatively balanced distribution across different age groups in the non-Hispanic group, more than half (55.8%) of the English-speaking Hispanic patients were in the youngest age group (18-49 years). A much lower fraction of Hispanic patients was among the oldest (≥80 years) age group (P < .001). Because COVID-19 clinical outcome is strongly associated with age,27 we used age-stratified analysis when comparing group-level differences in patient outcomes.
Sex distribution also was different among the three groups, with the non-English-speaking Hispanic group having more male patients (53.0%). Diabetes and obesity, which are associated with clinical outcomes of COVID-19 patients, were more prevalent in Hispanic patients (Table 1). Non-English-speaking Hispanic patients had the highest diabetes rate (48.7% with documented diabetes; 15.8% with baseline HbA1c > 9%; P < .001). English-speaking Hispanic patients presented with the highest obesity rate (62.8%; P < .001). Appendix Table 1 provides detailed age-group-specific comorbidity distributions among ethnic-language groups.
Patients of Hispanic Ethnicity Experienced a Higher Rate of ICU Utilization and Mortality
Of the 6097 patients overall, 1365 (22.4%) were admitted to the ICU and 543 (8.9%) died in hospital. For non-Hispanic patients (n = 4139), 883 (21.3%) were admitted to the ICU and 373 (9.0%) died in hospital. For English-speaking Hispanic patients (n = 1203), 241 (20.0%) were admitted to the ICU and 91 (7.6%) died in hospital. For non-English-speaking Hispanic patients (n = 755), 241 (31.9%) were admitted to the ICU and 79 (10.5%) died in hospital. Figure 1 summarizes the age-stratified comparison of ICU utilization and mortality across the three ethnic-language patient groups. In all age groups, non-English-speaking Hispanic patients experienced a significantly higher ICU utilization rate compared to non-Hispanic patients (age-adjusted OR, 1.75; 95% CI, 1.47-2.08; P < .001). English-speaking and non-English-speaking Hispanic patients had a significantly higher mortality rate compared to non-Hispanic patients (age-adjusted OR, 1.53; 95% CI, 1.19-1.98; P = .001 for English-speaking Hispanic patients; age-adjusted OR, 1.43; 95% CI,: 1.10-1.86; P = .01 for non-English-speaking Hispanic patients).
To delineate the risk factors associated with ICU utilization and death, we performed multivariable logistic regression with stepwise variable selection. After adjusting for age, sex, and comorbidity (Model 1), the factors ethnicity and primary language were still strongly associated with ICU utilization and mortality (Appendix Table 2). Non-English-speaking Hispanic patients had an OR of 1.74 (95% CI, 1.41-2.15; P < .001) for ICU utilization and an OR of 1.54 (95% CI, 1.12-2.12; P = .008) for mortality compared to non-Hispanic patients. Similarly, English-speaking Hispanic patients had higher ICU utilization (OR, 1.28; 95% CI, 1.05-1.55; P = .01) and a higher mortality rate (OR, 1.60; 95% CI, 1.19-2.14; P = .002).
No Disparity in COVID-19 Therapeutics Observed Across Three Ethnic-Language Groups
Appendix Figure 1 summarizes the comparison of the administration of four major treatments across the three ethnic-language groups. We did not observe any underuse of COVID-19 therapeutics for Hispanic patients. Usage rates for these therapies were significantly higher, after adjusting for age, in Hispanic groups when compared to non-Hispanic patients (OR ranged from 1.21 to 1.96). Steroids were the most common treatment in all patient groups. Tocilizumab was used almost twice as frequently (OR, 1.96; 95% CI, 1.64-2.33; P < .001) in non-English-speaking Hispanic patients compared to non-Hispanic patients.
Patients of Hispanic Ethnicity Had More Severe Disease at Hospital Admission
Figure 2 shows that non-English-speaking Hispanic patients had a higher rate of severe illness at admission based on each of these metrics: high respiratory MSOFA score (OR, 2.43; 95% CI, 1.77-3.33; P < .001), high EDI (OR, 1.85; 95% CI, 1.41-2.41; P < .001), and high CRP level (OR, 2.06; 95% CI, 1.64-2.58; P < .001). English-speaking Hispanic patients also had a greater rate of high CRP level (OR, 1.48; 95% CI, 1.17-1.86; P = .001) compared to non-Hispanic patients. When considering the presentation of any one of these clinical indicators, the English-speaking and non-English-speaking Hispanic patients had a higher rate of severe baseline condition (OR, 1.33; 95% CI, 1.10-1.61; P = .004 for English-speaking patients; OR, 2.27; 95% CI, 1.89-2.72; P < .001 for non-English-speaking patients).
We then studied how the baseline disease condition affects the association between ethnicity and clinical outcomes. We performed a multivariable analysis including baseline disease severity as a covariable (Model 2, Table 2), which showed that baseline disease severity was strongly associated with ICU admission (OR, 4.52; 95% CI, 3.83-5.33; P < .001) and mortality (OR, 3.32; 95% CI, 2.67-4.13; P < .001). The associations between ethnicity and clinical outcomes were reduced after considering the baseline disease condition. The OR dropped to 1.47 (95% CI, 1.18-1.84; P < .001) and 1.34 (95% CI, 0.97-1.87; P = .08) for ICU utilization and mortality, respectively, when comparing non-English-speaking Hispanic patients to non-Hispanic patients. A similar reduction was observed for English-speaking Hispanic patients. Model comparison showed a significant improvement of Model 2 over Model 1 based on ANOVA test (P < .001) as well as AIC.
Hispanic Patients Had Worse Healthcare Access
To explore the etiology for the more severe disease conditions at hospital admission among Hispanic patients, we analyzed variables related to healthcare access. We found that Hispanic patients were likely to have reduced access to healthcare (Table 1; Appendix Figure 2). For a large proportion (16.9%) of the COVID-19 patients in this study, their medical records were first created at THR in 2020, corresponding to the initial time these patients accessed THR for their healthcare. This surge in 2020, compared to previous years with data (2005–2019), corresponds to the number of new patients seen because of COVID-19 (Appendix Figure 2A). Among this new patient population, the proportion of non-English-speaking Hispanic patients in 2020 was 28.3%, compared to 9.1% from 2005 to 2019 (P < .001). The proportion of new English-speaking Hispanic patients in 2020 was 22.1%, compared to an average of 19.2% from 2005 to 2019 (P < .001). In addition, a much smaller proportion of Hispanic patients had a PCP (P < .001) (Table 1; Appendix Figure 2B), with non-English-speaking Hispanic patients having the smallest proportion (58.5%).
Appendix Figure 2C illustrates the comparison of payor types across the three patient groups. A much higher proportion of Hispanic patients used COVID-19 HRSA (P < .001) compared to non-Hispanic patients. Breaking this down further by primary language, 29.1% of non-English-speaking Hispanic patients relied on COVID-19 HRSA due to otherwise uninsured status, compared to 12.7% of English-speaking Hispanic patients and only 5.1% of non-Hispanic patients. Similarly, non-English-speaking Hispanic patients have the highest self-pay rates (2.3%) compared to English-speaking Hispanic patients (1.4%) and non-Hispanic patients (0.7%). In summary, more Hispanic patients, and especially non-English-speaking Hispanic patients, lacked conventional health insurance and experienced limited access to healthcare.
Further evidence showed a trend of correlation between presentation of severe COVID-19 conditions when arriving at the hospital and each of the healthcare access factors analyzed (Appendix Figure 3).
Discussion
With a large sample size of hospitalized COVID-19 patients at an integrated health system in the DFW metropolitan area, we observed an increased rate of ICU utilization and mortality among Hispanic inpatients. After adjusting for age, we found that non-English-speaking Hispanic patients were 75% more likely to require critical care compared with non-Hispanic patients. English-speaking and non-English-speaking Hispanic patients had an increased mortality rate (age-adjusted) compared to non-Hispanic patients. The association between ethnicity and clinical outcomes remained significant after adjusting for age, sex, and comorbidities. We did not observe any underuse of major COVID-19 therapeutics in Hispanic patients, and excluded in-hospital treatments from the contributors to the outcome differences.
Hispanic patients, especially non-English-speaking Hispanic patients, had a higher rate of severe COVID-19 disease at the time of hospital admission (Figure 2). After including baseline disease severity into the multivariable analysis (Model 2), the overall model improved (P < .001) while the associations between ethnicity and outcomes decreased (Table 2). This suggests disease severity at admission was a main contributor to the observed associations between ethnicity and clinical outcomes. The higher rate of baseline COVID-19 severity in Hispanic patients might also explain their higher rate of receiving major COVID-19 therapeutics (Appendix Figure 1).
This study found that Hispanic patients were less likely to have a PCP and insurance coverage compared with non-Hispanic patients (P < .001). This disparity was more pronounced among non-English-speaking Hispanic patients (Appendix Figure 2). We also observed that a disproportionately larger proportion (50.4%) of patients who visited the healthcare system for the first time in 2020 (the year of the COVID-19 pandemic) was composed of Hispanic patients, compared to merely 28.4% prior to 2020. While there is a possibility that patients had primary care outside THR, the staggering number of Hispanic patients who were new to the health system in 2020, in conjunction with the fact that immigrants tend to be “healthier” compared to their native-born peers (the so-called immigrant paradox),28 led us to conclude that there were few other primary care options for these patients, making THR’s ED the primary care option of choice. The systemic, structural barriers to routine care might be a possible cause for delayed admission and, in turn, elevated baseline COVID-19 severity for Hispanic patients (Appendix Figure 3).
Recent studies have investigated the impact of socioeconomic factors on racial/ethnic disparities in the COVID-19 pandemic.7,16,17 To our knowledge, no study has directly analyzed the link between healthcare access metrics, COVID-19 severity at admission, and the Hispanic population stratified by primary language. Studies exist on this subject for other diseases, however. For example, healthcare access factors have been associated with sepsis-related mortality.29,30 In fact, a recent study that explored the potential effect of language barriers on healthcare access demonstrated an association between limited English proficiency and sepsis-related mortality.31 Our study found that Hispanic patients whose primary language is not English had the worst clinical outcomes, including more severe baseline COVID-19 conditions, and the least access to healthcare, highlighting the importance of addressing language barriers in COVID-19 care. Further research is needed to confirm the relationship between limited English proficiency and clinical outcomes, as well as potential factors that contribute to such a relationship in different types of diseases.
Our study has a number of limitations. First, it was limited to only one large healthcare system, which means the results may not be generalizable. Because THR is an open system, comorbidity data may be incomplete, and we cannot exclude the possibility that patients accessed care outside THR prior to or during the pandemic. We may overcome this limitation in the future with cross-system health information exchange data. Second, we did not have data for the time of symptom onset, so we were unable to analyze the direct evidence of the possible delayed care. As a result, we were unable to analyze whether treatments were administered in a timely manner or appropriately. Third, our analysis was not adjusted for other socioeconomic factors (eg, income, education) due to lack of data. We used self-identification for ethnicity, but unlike new approaches by the U.S. Census Bureau,32 our survey allowed only one choice to be selected.
Conclusion
Sociodemographic factors among Hispanic inpatients hospitalized for COVID-19 at a large integrated health system—including a primary non-English language, lack of a PCP, and insurance status—were associated with measures of reduced access to care and more severe illness at admission. Structural barriers to care, which may be associated with reduced health literacy and less access to health insurance, can result in delayed treatment and more severe illness at admission and underdiagnosis of medical conditions, contributing to worse outcomes in this population. Our findings suggest that interventions to promote early recognition of signs and symptoms of COVID-19 and to encourage prompt clinical care at the community level may reduce the burden of COVID-19 deaths in racial or ethnic minority communities with language and socioeconomic barriers.
In the United States, health disparities in COVID-19 outcomes (including morbidity and mortality) based on race and ethnicity have been described in the scientific literature and mainstream media.1-7 According to the US Centers for Disease Control and Prevention (CDC), Hispanic people are 3.2 times more likely to be hospitalized with COVID-19 than non-Hispanic White people.8 Further, Hispanic people diagnosed with COVID-19 are 2.3 times more likely to die, adjusted for age, than non-Hispanic White people.9 As the epicenter of the COVID-19 pandemic shifted from the Northeast to the South, the CDC reported that, among people who died from COVID-19 in the United States from May to August 2020, the percentage of Hispanic people increased from 16.3% to 26.4%.10
Published studies on the effect of ethnicity on critical illness or mortality for hospitalized COVID-19 patients are limited and inconsistent. While some studies reported a higher mortality rate for Hispanic patients,11-15 others showed no difference.4,16,17 A recent meta-analysis found that intensive care unit (ICU) utilization and mortality were slightly higher among Hispanic COVID-19 inpatients, but this finding did not reach statistical significance.18 Past studies from different healthcare systems were limited by the small sample size of hospitalized Hispanic patients and the heterogeneity of patients. A comprehensive analysis from a large healthcare system with sufficient sample size is needed to understand the impact of ethnicity on clinical outcomes of hospitalized COVID-19 patients.
Texas Health Resources (THR) is a large integrated healthcare system serving the Dallas-Fort Worth-Arlington (DFW) metropolitan area. According to the 2019 US Census Bureau American Community Survey, Hispanic people comprise 18.4% of the population of this geographic area.19 Congruent with the CDC’s findings, Hispanic patients account for a disproportionate share (32.2%) of hospitalized COVID-19 patients at THR relative to the area’s demographic composition. Aware of the increased risk, we undertook an analysis of the clinical outcomes and the clinical, social, and demographic characteristics of Hispanic patients hospitalized at THR with COVID-19. Our primary goal was to investigate whether clinical outcomes differ by ethnicity among patients hospitalized with COVID-19 and, if so, whether inpatient care or preadmission factors contribute to this difference.
Methods
Study Setting and Overview
We collected data from the single electronic health record (EHR) used by 20 THR hospitals located across the DFW metropolitan area. THR is the largest faith-based, nonprofit health system in North Texas, operating 20 acute care hospitals. Including all access points, such as outpatient facilities and physician group practices, THR serves 7 million residents in 16 counties in North Texas, of whom 16.8% are Hispanic, 73.3% are non-Hispanic, and 9.9% are unclassified, congruent with demographics in the DFW area.
The institutional review boards at THR and UT Southwestern Medical Center approved the study under a waiver of informed consent (as a minimal-risk medical record review). After collection, all data were de-identified prior to statistical analysis.
Cohort, Outcomes, and Covariables
The study cohort included 6097 adult patients with laboratory-confirmed COVID-19 (age ≥18 years) who were admitted as inpatients from March 3 to November 5, 2020. The primary outcomes included ICU utilization and death during hospitalization. We described demographic characteristics using the following variables: age (18–49, 50–64, 65–79, ≥80 years), sex, self-reported ethnicity, and primary spoken language.
We defined a severe baseline condition as an elevated respiratory subscore parsed from the overall MSOFA (Modified Sequential Organ Failure Assessment),20 an elevated Epic Deterioration Index (EDI),21 or an elevated C-reactive protein level (CRP) at baseline (any elevated CRP). Baseline referred to the variable mean during the first available 12-hour window of measurement during the COVID-19 hospital admission, including variables obtained in the emergency department (ED). An elevated MSOFA referred to a score of 4, corresponding to an SpO2/FiO2 < 150. Elevated EDI referred to a baseline EDI > 45. An elevated CRP referred to a baseline CRP > 20 mg/dL.22
Variables reflecting access to healthcare included: THR EHR creation year (representing the first time patients accessed the THR health system), insurance payor type, and presence of a primary care provider (PCP). The federal government established the COVID-19 Claims Reimbursement to Health Care Providers and Facilities for Testing, Treatment, and Vaccine Administration for the Uninsured program. The insurance payor for patients covered by this program is designated as COVID-19 HRSA. Presence of a PCP reflects any documented PCP, regardless of affiliation with THR. We selected these access metrics opportunistically, as they were consistently documented in the EHR and readily available for analysis.
We used 12 variables to describe comorbidities or underlying conditions that, according to the CDC, increased patients’ risk of severe illness from COVID-1923: diagnoses of diabetes, hypertension, obesity, chronic obstructive pulmonary disease (COPD), asthma, smoking, other lung disease, heart failure, kidney disease without end-stage renal disease (ESRD), ESRD, liver disease, and cancer. We identified comorbidities by mining the structured diagnosis codes documented in the EHR prior to and during the COVID-19 admission. Sources for diagnoses included final billed diagnosis codes, working diagnosis codes, problem list, and reason for visit. The definition of diabetes included previously recorded diabetes or baseline hemoglobin A1c > 9%. We also recorded the presence of four major COVID-19 treatments: steroids, remdesivir, tocilizumab, and fresh frozen plasma (FFP) from convalescent patients.24-26 Each treatment variable was defined by receipt of one or more doses.
Statistical Analysis
To analyze patient outcomes based on ethnicity, we divided the study cohort into a Hispanic group and a non-Hispanic group based on self-reported ethnicity in the EHR. To study the potential impact of primary language among Hispanic patients, we divided them into English-speaking and non-English-speaking patients based on their self-reported primary language. As a result, we analyzed three groups of patients: (1) non-Hispanic, (2) Hispanic and English speaking, and (3) Hispanic and non-English speaking. We tested differences of a given categorical variable across the three groups using the chi-square test for each age subgroup (18–49, 50–64, 65–79, ≥80 years). The Cochran-Mantel-Haenszel test was used for the overall difference adjusted for age. To assess whether an observed disparity in treatment existed across the three groups, we tested the difference in the administration of four major therapeutics for COVID-19, including steroids, remdesivir, tocilizumab, and convalescent plasma. To determine whether any groups had elevated disease severity at hospital admission (baseline), we tested the difference in four disease-severity metrics across the ethnic-language groups: (1) elevated respiratory MSOFA score, (2) elevated EDI, (3) elevated CRP level, and (4) any of the three conditions.
To study the associations with ICU utilization and death, respectively, we performed a multivariable analysis using a generalized linear mixed model with binomial distribution and a logit link function. In each analysis model, the hospital of admission was included as a random-effect variable to account for the potential treatment variations among different hospitals, while other variables were regarded as fixed effects. In the first multivariable analysis (Model 1), all demographic variables, including age, sex, and ethnicity, and different types of comorbidities and underlying conditions, were included as fixed-effect variables in the initial model, and then backward stepwise variable selection was performed to establish the final model (Model 1). We performed the backward stepwise variable selection separately for the outcome of ICU use or mortality. Based on Akaike information criterion (AIC), during each iteration the fixed-effect variable that led to the largest decrease in the AIC value was removed, and the variable selection process was completed when the AIC value stopped decreasing. In Model 2, we added the disease-severity variable at baseline to the selected variable set derived from Model 1 to explore its effect on the associations between ethnicity and clinical outcomes. In Model 3, we added healthcare access–related variables, including first-time healthsystem access, payor type, and PCP availability to Model 2. We performed all statistical analyses using R, version 4.0.2 (R Foundation for Statistical Computing) in RStudio (version 1.3.1093).
Results
Distinct Demographic and Comorbidity Patterns for Three Ethnic-Language Groups
We identified 6097 adult patients (age ≥18 years) who had confirmed COVID-19 disease and were hospitalized between March 3 and November 5, 2020. Demographic characteristics and comorbidity for these patients are summarized in Table 1. Among these patients, 4139 (67.9%) were non-Hispanic and 1958 (32.1%) were Hispanic. Among the Hispanic patients, 1203 (61.4%) identified English as their primary language and 755 (38.6%) identified a non-English primary language. Age distribution was vastly different among the three ethnic-language groups (Table 1). Unlike the relatively balanced distribution across different age groups in the non-Hispanic group, more than half (55.8%) of the English-speaking Hispanic patients were in the youngest age group (18-49 years). A much lower fraction of Hispanic patients was among the oldest (≥80 years) age group (P < .001). Because COVID-19 clinical outcome is strongly associated with age,27 we used age-stratified analysis when comparing group-level differences in patient outcomes.
Sex distribution also was different among the three groups, with the non-English-speaking Hispanic group having more male patients (53.0%). Diabetes and obesity, which are associated with clinical outcomes of COVID-19 patients, were more prevalent in Hispanic patients (Table 1). Non-English-speaking Hispanic patients had the highest diabetes rate (48.7% with documented diabetes; 15.8% with baseline HbA1c > 9%; P < .001). English-speaking Hispanic patients presented with the highest obesity rate (62.8%; P < .001). Appendix Table 1 provides detailed age-group-specific comorbidity distributions among ethnic-language groups.
Patients of Hispanic Ethnicity Experienced a Higher Rate of ICU Utilization and Mortality
Of the 6097 patients overall, 1365 (22.4%) were admitted to the ICU and 543 (8.9%) died in hospital. For non-Hispanic patients (n = 4139), 883 (21.3%) were admitted to the ICU and 373 (9.0%) died in hospital. For English-speaking Hispanic patients (n = 1203), 241 (20.0%) were admitted to the ICU and 91 (7.6%) died in hospital. For non-English-speaking Hispanic patients (n = 755), 241 (31.9%) were admitted to the ICU and 79 (10.5%) died in hospital. Figure 1 summarizes the age-stratified comparison of ICU utilization and mortality across the three ethnic-language patient groups. In all age groups, non-English-speaking Hispanic patients experienced a significantly higher ICU utilization rate compared to non-Hispanic patients (age-adjusted OR, 1.75; 95% CI, 1.47-2.08; P < .001). English-speaking and non-English-speaking Hispanic patients had a significantly higher mortality rate compared to non-Hispanic patients (age-adjusted OR, 1.53; 95% CI, 1.19-1.98; P = .001 for English-speaking Hispanic patients; age-adjusted OR, 1.43; 95% CI,: 1.10-1.86; P = .01 for non-English-speaking Hispanic patients).
To delineate the risk factors associated with ICU utilization and death, we performed multivariable logistic regression with stepwise variable selection. After adjusting for age, sex, and comorbidity (Model 1), the factors ethnicity and primary language were still strongly associated with ICU utilization and mortality (Appendix Table 2). Non-English-speaking Hispanic patients had an OR of 1.74 (95% CI, 1.41-2.15; P < .001) for ICU utilization and an OR of 1.54 (95% CI, 1.12-2.12; P = .008) for mortality compared to non-Hispanic patients. Similarly, English-speaking Hispanic patients had higher ICU utilization (OR, 1.28; 95% CI, 1.05-1.55; P = .01) and a higher mortality rate (OR, 1.60; 95% CI, 1.19-2.14; P = .002).
No Disparity in COVID-19 Therapeutics Observed Across Three Ethnic-Language Groups
Appendix Figure 1 summarizes the comparison of the administration of four major treatments across the three ethnic-language groups. We did not observe any underuse of COVID-19 therapeutics for Hispanic patients. Usage rates for these therapies were significantly higher, after adjusting for age, in Hispanic groups when compared to non-Hispanic patients (OR ranged from 1.21 to 1.96). Steroids were the most common treatment in all patient groups. Tocilizumab was used almost twice as frequently (OR, 1.96; 95% CI, 1.64-2.33; P < .001) in non-English-speaking Hispanic patients compared to non-Hispanic patients.
Patients of Hispanic Ethnicity Had More Severe Disease at Hospital Admission
Figure 2 shows that non-English-speaking Hispanic patients had a higher rate of severe illness at admission based on each of these metrics: high respiratory MSOFA score (OR, 2.43; 95% CI, 1.77-3.33; P < .001), high EDI (OR, 1.85; 95% CI, 1.41-2.41; P < .001), and high CRP level (OR, 2.06; 95% CI, 1.64-2.58; P < .001). English-speaking Hispanic patients also had a greater rate of high CRP level (OR, 1.48; 95% CI, 1.17-1.86; P = .001) compared to non-Hispanic patients. When considering the presentation of any one of these clinical indicators, the English-speaking and non-English-speaking Hispanic patients had a higher rate of severe baseline condition (OR, 1.33; 95% CI, 1.10-1.61; P = .004 for English-speaking patients; OR, 2.27; 95% CI, 1.89-2.72; P < .001 for non-English-speaking patients).
We then studied how the baseline disease condition affects the association between ethnicity and clinical outcomes. We performed a multivariable analysis including baseline disease severity as a covariable (Model 2, Table 2), which showed that baseline disease severity was strongly associated with ICU admission (OR, 4.52; 95% CI, 3.83-5.33; P < .001) and mortality (OR, 3.32; 95% CI, 2.67-4.13; P < .001). The associations between ethnicity and clinical outcomes were reduced after considering the baseline disease condition. The OR dropped to 1.47 (95% CI, 1.18-1.84; P < .001) and 1.34 (95% CI, 0.97-1.87; P = .08) for ICU utilization and mortality, respectively, when comparing non-English-speaking Hispanic patients to non-Hispanic patients. A similar reduction was observed for English-speaking Hispanic patients. Model comparison showed a significant improvement of Model 2 over Model 1 based on ANOVA test (P < .001) as well as AIC.
Hispanic Patients Had Worse Healthcare Access
To explore the etiology for the more severe disease conditions at hospital admission among Hispanic patients, we analyzed variables related to healthcare access. We found that Hispanic patients were likely to have reduced access to healthcare (Table 1; Appendix Figure 2). For a large proportion (16.9%) of the COVID-19 patients in this study, their medical records were first created at THR in 2020, corresponding to the initial time these patients accessed THR for their healthcare. This surge in 2020, compared to previous years with data (2005–2019), corresponds to the number of new patients seen because of COVID-19 (Appendix Figure 2A). Among this new patient population, the proportion of non-English-speaking Hispanic patients in 2020 was 28.3%, compared to 9.1% from 2005 to 2019 (P < .001). The proportion of new English-speaking Hispanic patients in 2020 was 22.1%, compared to an average of 19.2% from 2005 to 2019 (P < .001). In addition, a much smaller proportion of Hispanic patients had a PCP (P < .001) (Table 1; Appendix Figure 2B), with non-English-speaking Hispanic patients having the smallest proportion (58.5%).
Appendix Figure 2C illustrates the comparison of payor types across the three patient groups. A much higher proportion of Hispanic patients used COVID-19 HRSA (P < .001) compared to non-Hispanic patients. Breaking this down further by primary language, 29.1% of non-English-speaking Hispanic patients relied on COVID-19 HRSA due to otherwise uninsured status, compared to 12.7% of English-speaking Hispanic patients and only 5.1% of non-Hispanic patients. Similarly, non-English-speaking Hispanic patients have the highest self-pay rates (2.3%) compared to English-speaking Hispanic patients (1.4%) and non-Hispanic patients (0.7%). In summary, more Hispanic patients, and especially non-English-speaking Hispanic patients, lacked conventional health insurance and experienced limited access to healthcare.
Further evidence showed a trend of correlation between presentation of severe COVID-19 conditions when arriving at the hospital and each of the healthcare access factors analyzed (Appendix Figure 3).
Discussion
With a large sample size of hospitalized COVID-19 patients at an integrated health system in the DFW metropolitan area, we observed an increased rate of ICU utilization and mortality among Hispanic inpatients. After adjusting for age, we found that non-English-speaking Hispanic patients were 75% more likely to require critical care compared with non-Hispanic patients. English-speaking and non-English-speaking Hispanic patients had an increased mortality rate (age-adjusted) compared to non-Hispanic patients. The association between ethnicity and clinical outcomes remained significant after adjusting for age, sex, and comorbidities. We did not observe any underuse of major COVID-19 therapeutics in Hispanic patients, and excluded in-hospital treatments from the contributors to the outcome differences.
Hispanic patients, especially non-English-speaking Hispanic patients, had a higher rate of severe COVID-19 disease at the time of hospital admission (Figure 2). After including baseline disease severity into the multivariable analysis (Model 2), the overall model improved (P < .001) while the associations between ethnicity and outcomes decreased (Table 2). This suggests disease severity at admission was a main contributor to the observed associations between ethnicity and clinical outcomes. The higher rate of baseline COVID-19 severity in Hispanic patients might also explain their higher rate of receiving major COVID-19 therapeutics (Appendix Figure 1).
This study found that Hispanic patients were less likely to have a PCP and insurance coverage compared with non-Hispanic patients (P < .001). This disparity was more pronounced among non-English-speaking Hispanic patients (Appendix Figure 2). We also observed that a disproportionately larger proportion (50.4%) of patients who visited the healthcare system for the first time in 2020 (the year of the COVID-19 pandemic) was composed of Hispanic patients, compared to merely 28.4% prior to 2020. While there is a possibility that patients had primary care outside THR, the staggering number of Hispanic patients who were new to the health system in 2020, in conjunction with the fact that immigrants tend to be “healthier” compared to their native-born peers (the so-called immigrant paradox),28 led us to conclude that there were few other primary care options for these patients, making THR’s ED the primary care option of choice. The systemic, structural barriers to routine care might be a possible cause for delayed admission and, in turn, elevated baseline COVID-19 severity for Hispanic patients (Appendix Figure 3).
Recent studies have investigated the impact of socioeconomic factors on racial/ethnic disparities in the COVID-19 pandemic.7,16,17 To our knowledge, no study has directly analyzed the link between healthcare access metrics, COVID-19 severity at admission, and the Hispanic population stratified by primary language. Studies exist on this subject for other diseases, however. For example, healthcare access factors have been associated with sepsis-related mortality.29,30 In fact, a recent study that explored the potential effect of language barriers on healthcare access demonstrated an association between limited English proficiency and sepsis-related mortality.31 Our study found that Hispanic patients whose primary language is not English had the worst clinical outcomes, including more severe baseline COVID-19 conditions, and the least access to healthcare, highlighting the importance of addressing language barriers in COVID-19 care. Further research is needed to confirm the relationship between limited English proficiency and clinical outcomes, as well as potential factors that contribute to such a relationship in different types of diseases.
Our study has a number of limitations. First, it was limited to only one large healthcare system, which means the results may not be generalizable. Because THR is an open system, comorbidity data may be incomplete, and we cannot exclude the possibility that patients accessed care outside THR prior to or during the pandemic. We may overcome this limitation in the future with cross-system health information exchange data. Second, we did not have data for the time of symptom onset, so we were unable to analyze the direct evidence of the possible delayed care. As a result, we were unable to analyze whether treatments were administered in a timely manner or appropriately. Third, our analysis was not adjusted for other socioeconomic factors (eg, income, education) due to lack of data. We used self-identification for ethnicity, but unlike new approaches by the U.S. Census Bureau,32 our survey allowed only one choice to be selected.
Conclusion
Sociodemographic factors among Hispanic inpatients hospitalized for COVID-19 at a large integrated health system—including a primary non-English language, lack of a PCP, and insurance status—were associated with measures of reduced access to care and more severe illness at admission. Structural barriers to care, which may be associated with reduced health literacy and less access to health insurance, can result in delayed treatment and more severe illness at admission and underdiagnosis of medical conditions, contributing to worse outcomes in this population. Our findings suggest that interventions to promote early recognition of signs and symptoms of COVID-19 and to encourage prompt clinical care at the community level may reduce the burden of COVID-19 deaths in racial or ethnic minority communities with language and socioeconomic barriers.
1. Lopez L III, Hart LH III, Katz MH. Racial and ethnic health disparities related to COVID-19. JAMA. 2021;325(8):719-720. https://doi.org/10.1001/jama.2020.26443
2. Cooper LA, Williams DR. Excess deaths from COVID-19, community bereavement, and restorative justice for communities of color. JAMA. 2020;324(15):1491-1492. https://doi.org/10.1001/jama.2020.19567
3. Clay LA, Rogus S. Primary and secondary health impacts of COVID-19 among minority individuals in New York State. Int J Environ Res Public Health. 2021;18(2):683. https://doi.org/10.3390/ijerph18020683
4. Rodriguez F, Solomon N, de Lemos JA, et al. Racial and ethnic differences in presentation and outcomes for patients hospitalized with COVID-19: findings from the American Heart Association’s COVID-19 Cardiovascular Disease Registry. Circulation. 2021;143(24):2332-2342. https://doi.org/10.1161/CIRCULATIONAHA.120.052278
5. Moreira A, Chorath K, Rajasekaran K, Burmeister F, Ahmed M, Moreira A. Demographic predictors of hospitalization and mortality in US children with COVID-19. Eur J Pediatr. 2021;180(5):1659-1663. https://doi.org/10.1007/s00431-021-03955-x
6. Kolata G. Social inequities explain racial gaps in pandemic, studies find. The New York Times. December 9, 2020. https://www.nytimes.com/2020/12/09/health/coronavirus-black-hispanic.html
7. Liao TF, De Maio F. Association of social and economic inequality with coronavirus disease 2019 incidence and mortality across US counties. JAMA Netw Open. 2021;4(1):e2034578. https://doi.org/10.1001/jamanetworkopen.2020.34578
8. Centers for Disease Control and Prevention. A Weekly Surveillance Summary of U.S. COVID-19 Activity: Key Updates for Week 2. January 21, 2021. https://www.cdc.gov/coronavirus/2019-ncov/covid-data/pdf/covidview-01-22-2021.pdf
9. Centers for Disease Control and Prevention. Risk for COVID-19 infection, hospitalization, and death by race/ethnicity. Updated September 9, 2021. https://www.cdc.gov/coronavirus/2019-ncov/covid-data/investigations-discovery/hospitalization-death-by-race-ethnicity.html
10. Gold JAW, Rossen LM, Ahmad FB, et al. Race, ethnicity, and age trends in persons who died from COVID-19 – United States, May-August 2020. MMWR Morb Mortal Wkly Rep. 2020;69(42):1517-1521. https://doi.org/10.15585/mmwr.mm6942e1
11. Pennington AF, Kompaniyets L, Summers AD, et al. Risk of clinical severity by age and race/ethnicity among adults hospitalized for COVID-19 – United States, March-September 2020. Open Forum Infect Dis. 2021;8(2):ofaa638. https://doi.org/10.1093/ofid/ofaa638.
12. Renelus BD, Khoury NC, Chandrasekaran K, et al. Racial disparities in COVID-19 hospitalization and in-hospital mortality at the height of the New York City pandemic. J Racial Ethn Health Disparities. 2021;8(5):1161-1167. https://doi.org/10.1007/s40615-020-00872-x
13. Wiley Z, Ross-Driscoll K, Wang Z, Smothers L, Mehta AK, Patzer RE. Racial and ethnic differences and clinical outcomes of COVID-19 patients presenting to the emergency department. Clin Infect Dis. 2021 Apr 2. [Epub ahead of print] https://doi.org/10.1093/cid/ciab290
14. Dai CL, Kornilov SA, Roper RT, et al. Characteristics and factors associated with COVID-19 infection, hospitalization, and mortality across race and ethnicity. Clin Infect Dis. 2021 Feb 20. [Epub ahead of print] https://doi.org/10.1093/cid/ciab154
15. Pan AP, Khan O, Meeks JR, et al. Disparities in COVID-19 hospitalizations and mortality among black and Hispanic patients: cross-sectional analysis from the greater Houston metropolitan area. BMC Public Health. 2021;21(1):1330. https://doi.org/10.1186/s12889-021-11431-2
16. Ogedegbe G, Ravenell J, Adhikari S, et al. Assessment of racial/ethnic disparities in hospitalization and mortality in patients with COVID-19 in New York City. JAMA Netw Open. 2020;3(12):e2026881. https://doi.org/10.1001/jamanetworkopen.2020.26881
17. Gershengorn HB, Patel S, Shukla B, et al. Association of race and ethnicity with COVID-19 test positivity and hospitalization is mediated by socioeconomic factors. Ann Am Thorac Soc. 2021;18(8):1326-1334. https://doi.org/10.1513/AnnalsATS.202011-1448OC
18. Sze S, Pan D, Nevill CR, et al. Ethnicity and clinical outcomes in COVID-19: a systematic review and meta-analysis. EClinicalMedicine. 2020;29:100630. https://doi.org/10.1016/j.eclinm.2020.100630
19. U.S. Census Bureau. 2019 U.S Census Bureau American Community Survey. https://www.census.gov/programs-surveys/acs
20. North Texas Mass Critical Care Task Force. North Texas Mass Critical Care Guidelines Document. Hospital and ICU Triage Guidelines for ADULTS. January 2014. https://www.dallas-cms.org/tmaimis/dcms/assets/files/communityhealth/MCC/GuidelinesAdult_JAN2014.pdf
21. Singh K, Valley TS, Tang S, et al. Evaluating a widely implemented proprietary deterioration index model among hospitalized COVID-19 patients. Ann Am Thorac Soc. 2021;18(7):1129-1137. https://doi.org/10.1513/AnnalsATS.202006-698OC
22. Keller MJ, Kitsis EA, Arora S, et al. Effect of systemic glucocorticoids on mortality or mechanical ventilation in patients with COVID-19. J Hosp Med. 2020;15(8):489-493. https://doi.org/10.12788/jhm.3497
23. Centers for Disease Control and Prevention. Science Brief: Evidence used to update the list of underlying medical conditions that increase a person’s risk of severe illness from COVID-19. Updated May 12, 2021. https://www.cdc.gov/coronavirus/2019-ncov/science/science-briefs/underlying-evidence-table.html
24. Gupta S, Wang W, Hayek SS, et al. Association between early treatment with tocilizumab and mortality among critically ill patients with COVID-19. JAMA Intern Med. 2021;181(1):41-51. https://doi.org/10.1001/jamainternmed.2020.6252
25. Baroutjian A, Sanchez C, Boneva D, McKenney M, Elkbuli A. SARS-CoV-2 pharmacologic therapies and their safety/effectiveness according to level of evidence. Am J Emerg Med. 2020;38(11):2405-2415. https://doi.org/10.1016/j.ajem.2020.08.091
26. Janiaud P, Axfors C, Schmitt AM, et al. Association of convalescent plasma treatment with clinical outcomes in patients with COVID-19: a systematic review and meta-analysis. JAMA. 2021;325(12):1185-1195. https://doi.org/10.1001/jama.2021.2747
27. Panagiotou OA, Kosar CM, White EM, et al. Risk factors associated with all-cause 30-day mortality in nursing home residents with COVID-19. JAMA Intern Med. 2021;181(4):439-448. https://doi.org/10.1001/jamainternmed.2020.7968
28. Bacong AM, Menjívar C. Recasting the immigrant health paradox through intersections of legal status and race. J Immigr Minor Health. 2021;23(5):1092-1104. https://doi.org/10.1007/s10903-021-01162-2
29. Plopper GE, Sciarretta KL, Buchman TG. Disparities in sepsis outcomes may be attributable to access to care. Crit Care Med. 2021;49(8):1358-1360. https://doi.org/10.1097/CCM.0000000000005126
30. Jones JM, Fingar KR, Miller MA, et al. Racial disparities in sepsis-related in-hospital mortality: using a broad case capture method and multivariate controls for clinical and hospital variables, 2004-2013. Crit Care Med. 2017;45(12):e1209-e1217. https://doi.org/10.1097/CCM.0000000000002699
31. Jacobs ZG, Prasad PA, Fang MC, Abe-Jones Y, Kangelaris KN. The association between limited English proficiency and sepsis mortality. J Hosp Med. 2019;14:E1-E7. https://doi.org/10.12788/jhm.3334
32. Cohn D. Census considers new approach to asking about race – by not using the term at all. June 18, 2015. https://www.pewresearch.org/fact-tank/2015/06/18/census-considers-new-approach-to-asking-about-race-by-not-using-the-term-at-all/
1. Lopez L III, Hart LH III, Katz MH. Racial and ethnic health disparities related to COVID-19. JAMA. 2021;325(8):719-720. https://doi.org/10.1001/jama.2020.26443
2. Cooper LA, Williams DR. Excess deaths from COVID-19, community bereavement, and restorative justice for communities of color. JAMA. 2020;324(15):1491-1492. https://doi.org/10.1001/jama.2020.19567
3. Clay LA, Rogus S. Primary and secondary health impacts of COVID-19 among minority individuals in New York State. Int J Environ Res Public Health. 2021;18(2):683. https://doi.org/10.3390/ijerph18020683
4. Rodriguez F, Solomon N, de Lemos JA, et al. Racial and ethnic differences in presentation and outcomes for patients hospitalized with COVID-19: findings from the American Heart Association’s COVID-19 Cardiovascular Disease Registry. Circulation. 2021;143(24):2332-2342. https://doi.org/10.1161/CIRCULATIONAHA.120.052278
5. Moreira A, Chorath K, Rajasekaran K, Burmeister F, Ahmed M, Moreira A. Demographic predictors of hospitalization and mortality in US children with COVID-19. Eur J Pediatr. 2021;180(5):1659-1663. https://doi.org/10.1007/s00431-021-03955-x
6. Kolata G. Social inequities explain racial gaps in pandemic, studies find. The New York Times. December 9, 2020. https://www.nytimes.com/2020/12/09/health/coronavirus-black-hispanic.html
7. Liao TF, De Maio F. Association of social and economic inequality with coronavirus disease 2019 incidence and mortality across US counties. JAMA Netw Open. 2021;4(1):e2034578. https://doi.org/10.1001/jamanetworkopen.2020.34578
8. Centers for Disease Control and Prevention. A Weekly Surveillance Summary of U.S. COVID-19 Activity: Key Updates for Week 2. January 21, 2021. https://www.cdc.gov/coronavirus/2019-ncov/covid-data/pdf/covidview-01-22-2021.pdf
9. Centers for Disease Control and Prevention. Risk for COVID-19 infection, hospitalization, and death by race/ethnicity. Updated September 9, 2021. https://www.cdc.gov/coronavirus/2019-ncov/covid-data/investigations-discovery/hospitalization-death-by-race-ethnicity.html
10. Gold JAW, Rossen LM, Ahmad FB, et al. Race, ethnicity, and age trends in persons who died from COVID-19 – United States, May-August 2020. MMWR Morb Mortal Wkly Rep. 2020;69(42):1517-1521. https://doi.org/10.15585/mmwr.mm6942e1
11. Pennington AF, Kompaniyets L, Summers AD, et al. Risk of clinical severity by age and race/ethnicity among adults hospitalized for COVID-19 – United States, March-September 2020. Open Forum Infect Dis. 2021;8(2):ofaa638. https://doi.org/10.1093/ofid/ofaa638.
12. Renelus BD, Khoury NC, Chandrasekaran K, et al. Racial disparities in COVID-19 hospitalization and in-hospital mortality at the height of the New York City pandemic. J Racial Ethn Health Disparities. 2021;8(5):1161-1167. https://doi.org/10.1007/s40615-020-00872-x
13. Wiley Z, Ross-Driscoll K, Wang Z, Smothers L, Mehta AK, Patzer RE. Racial and ethnic differences and clinical outcomes of COVID-19 patients presenting to the emergency department. Clin Infect Dis. 2021 Apr 2. [Epub ahead of print] https://doi.org/10.1093/cid/ciab290
14. Dai CL, Kornilov SA, Roper RT, et al. Characteristics and factors associated with COVID-19 infection, hospitalization, and mortality across race and ethnicity. Clin Infect Dis. 2021 Feb 20. [Epub ahead of print] https://doi.org/10.1093/cid/ciab154
15. Pan AP, Khan O, Meeks JR, et al. Disparities in COVID-19 hospitalizations and mortality among black and Hispanic patients: cross-sectional analysis from the greater Houston metropolitan area. BMC Public Health. 2021;21(1):1330. https://doi.org/10.1186/s12889-021-11431-2
16. Ogedegbe G, Ravenell J, Adhikari S, et al. Assessment of racial/ethnic disparities in hospitalization and mortality in patients with COVID-19 in New York City. JAMA Netw Open. 2020;3(12):e2026881. https://doi.org/10.1001/jamanetworkopen.2020.26881
17. Gershengorn HB, Patel S, Shukla B, et al. Association of race and ethnicity with COVID-19 test positivity and hospitalization is mediated by socioeconomic factors. Ann Am Thorac Soc. 2021;18(8):1326-1334. https://doi.org/10.1513/AnnalsATS.202011-1448OC
18. Sze S, Pan D, Nevill CR, et al. Ethnicity and clinical outcomes in COVID-19: a systematic review and meta-analysis. EClinicalMedicine. 2020;29:100630. https://doi.org/10.1016/j.eclinm.2020.100630
19. U.S. Census Bureau. 2019 U.S Census Bureau American Community Survey. https://www.census.gov/programs-surveys/acs
20. North Texas Mass Critical Care Task Force. North Texas Mass Critical Care Guidelines Document. Hospital and ICU Triage Guidelines for ADULTS. January 2014. https://www.dallas-cms.org/tmaimis/dcms/assets/files/communityhealth/MCC/GuidelinesAdult_JAN2014.pdf
21. Singh K, Valley TS, Tang S, et al. Evaluating a widely implemented proprietary deterioration index model among hospitalized COVID-19 patients. Ann Am Thorac Soc. 2021;18(7):1129-1137. https://doi.org/10.1513/AnnalsATS.202006-698OC
22. Keller MJ, Kitsis EA, Arora S, et al. Effect of systemic glucocorticoids on mortality or mechanical ventilation in patients with COVID-19. J Hosp Med. 2020;15(8):489-493. https://doi.org/10.12788/jhm.3497
23. Centers for Disease Control and Prevention. Science Brief: Evidence used to update the list of underlying medical conditions that increase a person’s risk of severe illness from COVID-19. Updated May 12, 2021. https://www.cdc.gov/coronavirus/2019-ncov/science/science-briefs/underlying-evidence-table.html
24. Gupta S, Wang W, Hayek SS, et al. Association between early treatment with tocilizumab and mortality among critically ill patients with COVID-19. JAMA Intern Med. 2021;181(1):41-51. https://doi.org/10.1001/jamainternmed.2020.6252
25. Baroutjian A, Sanchez C, Boneva D, McKenney M, Elkbuli A. SARS-CoV-2 pharmacologic therapies and their safety/effectiveness according to level of evidence. Am J Emerg Med. 2020;38(11):2405-2415. https://doi.org/10.1016/j.ajem.2020.08.091
26. Janiaud P, Axfors C, Schmitt AM, et al. Association of convalescent plasma treatment with clinical outcomes in patients with COVID-19: a systematic review and meta-analysis. JAMA. 2021;325(12):1185-1195. https://doi.org/10.1001/jama.2021.2747
27. Panagiotou OA, Kosar CM, White EM, et al. Risk factors associated with all-cause 30-day mortality in nursing home residents with COVID-19. JAMA Intern Med. 2021;181(4):439-448. https://doi.org/10.1001/jamainternmed.2020.7968
28. Bacong AM, Menjívar C. Recasting the immigrant health paradox through intersections of legal status and race. J Immigr Minor Health. 2021;23(5):1092-1104. https://doi.org/10.1007/s10903-021-01162-2
29. Plopper GE, Sciarretta KL, Buchman TG. Disparities in sepsis outcomes may be attributable to access to care. Crit Care Med. 2021;49(8):1358-1360. https://doi.org/10.1097/CCM.0000000000005126
30. Jones JM, Fingar KR, Miller MA, et al. Racial disparities in sepsis-related in-hospital mortality: using a broad case capture method and multivariate controls for clinical and hospital variables, 2004-2013. Crit Care Med. 2017;45(12):e1209-e1217. https://doi.org/10.1097/CCM.0000000000002699
31. Jacobs ZG, Prasad PA, Fang MC, Abe-Jones Y, Kangelaris KN. The association between limited English proficiency and sepsis mortality. J Hosp Med. 2019;14:E1-E7. https://doi.org/10.12788/jhm.3334
32. Cohn D. Census considers new approach to asking about race – by not using the term at all. June 18, 2015. https://www.pewresearch.org/fact-tank/2015/06/18/census-considers-new-approach-to-asking-about-race-by-not-using-the-term-at-all/
© 2021 Society of Hospital Medicine
Predicting Readmissions from EHR Data
Unplanned hospital readmissions are frequent, costly, and potentially avoidable.[1, 2] Due to major federal financial readmissions penalties targeting excessive 30‐day readmissions, there is increasing attention to implementing hospital‐initiated interventions to reduce readmissions.[3, 4] However, universal enrollment of all hospitalized patients into such programs may be too resource intensive for many hospitals.[5] To optimize efficiency and effectiveness, interventions should be targeted to individuals most likely to benefit.[6, 7] However, existing readmission risk‐prediction models have achieved only modest discrimination, have largely used administrative claims data not available until months after discharge, or are limited to only a subset of patients with Medicare or a specific clinical condition.[8, 9, 10, 11, 12, 13, 14] These limitations have precluded accurate identification of high‐risk individuals in an all‐payer general medical inpatient population to provide actionable information for intervention prior to discharge.
Approaches using electronic health record (EHR) data could allow early identification of high‐risk patients during the index hospitalization to enable initiation of interventions prior to discharge. To date, such strategies have relied largely on EHR data from the day of admission.[15, 16] However, given that variation in 30‐day readmission rates are thought to reflect the quality of in‐hospital care, incorporating EHR data from the entire hospital stay to reflect hospital care processes and clinical trajectory may more accurately identify at‐risk patients.[17, 18, 19, 20] Improved accuracy in risk prediction would help better target intervention efforts in the immediate postdischarge period, an interval characterized by heightened vulnerability for adverse events.[21]
To help hospitals target transitional care interventions more effectively to high‐risk individuals prior to discharge, we derived and validated a readmissions risk‐prediction model incorporating EHR data from the entire course of the index hospitalization, which we termed the full‐stay EHR model. We also compared the full‐stay EHR model performance to our group's previously derived prediction model based on EHR data on the day of admission, termed the first‐day EHR model, as well as to 2 other validated readmission models similarly intended to yield near real‐time risk predictions prior to or shortly after hospital discharge.[9, 10, 15]
METHODS
Study Design, Population, and Data Sources
We conducted an observational cohort study using EHR data from 6 hospitals in the DallasFort Worth metroplex between November 1, 2009 and October 30, 2010 using the same EHR system (Epic Systems Corp., Verona, WI). One site was a university‐affiliated safety net hospital; the remaining 5 sites were teaching and nonteaching community sites.
We included consecutive hospitalizations among adults 18 years old discharged alive from any medicine inpatient service. For individuals with multiple hospitalizations during the study period, we included only the first hospitalization. We excluded individuals who died during the index hospitalization, were transferred to another acute care facility, left against medical advice, or who died outside of the hospital within 30 days of discharge. For model derivation, we randomly split the sample into separate derivation (50%) and validation cohorts (50%).
Outcomes
The primary outcome was 30‐day hospital readmission, defined as a nonelective hospitalization within 30 days of discharge to any of 75 acute care hospitals within a 100‐mile radius of Dallas, ascertained from an all‐payer regional hospitalization database. Nonelective hospitalizations included all hospitalizations classified as a emergency, urgent, or trauma, and excluded those classified as elective as per the Centers for Medicare and Medicaid Services Claim Inpatient Admission Type Code definitions.
Predictor Variables for the Full‐Stay EHR Model
The full‐stay EHR model was iteratively developed from our group's previously derived and validated risk‐prediction model using EHR data available on admission (first‐day EHR model).[15] For the full‐stay EHR model, we included all predictor variables included in our published first‐day EHR model as candidate risk factors. Based on prior literature, we additionally expanded candidate predictors available on admission to include marital status (proxy for social isolation) and socioeconomic disadvantage (percent poverty, unemployment, median income, and educational attainment by zip code of residence as proxy measures of the social and built environment).[22, 23, 24, 25, 26, 27] We also expanded the ascertainment of prior hospitalization to include admissions at both the index hospital and any of 75 acute care hospitals from the same, separate all‐payer regional hospitalization database used to ascertain 30‐day readmissions.
Candidate predictors from the remainder of the hospital stay (ie, following the first 24 hours of admission) were included if they were: (1) available in the EHR of all participating hospitals, (2) routinely collected or available at the time of hospital discharge, and (3) plausible predictors of adverse outcomes based on prior literature and clinical expertise. These included length of stay, in‐hospital complications, transfer to an intensive or coronary care unit, blood transfusions, vital sign instabilities within 24 hours of discharge, select laboratory values at time of discharge, and disposition status. We also assessed trajectories of vital signs and selected laboratory values (defined as changes in these measures from admission to discharge).
Statistical Analysis
Model Derivation
Univariate relationships between readmission and each of the candidate predictors were assessed in the derivation cohort using a prespecified significance threshold of P 0.05. We included all factors from our previously derived and validated first‐day EHR model as candidate predictors.[15] Continuous laboratory and vital sign values at the time of discharge were categorized based on clinically meaningful cutoffs; predictors with missing values were assumed to be normal (<1% missing for each variable). Significant univariate candidate variables were entered in a multivariate logistic regression model using stepwise backward selection with a prespecified significance threshold of P 0.05. We performed several sensitivity analyses to confirm the robustness of our model. First, we alternately derived the full‐stay model using stepwise forward selection. Second, we forced in all significant variables from our first‐day EHR model, and entered the candidate variables from the remainder of the hospital stay using both stepwise backward and forward selection separately. Third, prespecified interactions between variables were evaluated for inclusion. Though final predictors varied slightly between the different approaches, discrimination of each model was similar to the model derived using our primary analytic approach (C statistics 0.01, data not shown).
Model Validation
We assessed model discrimination and calibration of the derived full‐stay EHR model using the validation cohort. Model discrimination was estimated by the C statistic. The C statistic represents the probability that, given 2 hospitalized individuals (1 who was readmitted and the other who was not), the model will predict a higher risk for the readmitted patient than for the nonreadmitted patient. Model calibration was assessed by comparing predicted to observed probabilities of readmission by quintiles of risk, and with the Hosmer‐Lemeshow goodness‐of‐fit test.
Comparison to Existing Models
We compared the full‐stay EHR model performance to 3 previously published models: our group's first‐day EHR model, and the LACE (includes Length of stay, Acute (nonelective) admission status, Charlson Comorbidity Index, and Emergency department visits in the past year) and HOSPITAL (includes Hemoglobin at discharge, discharge from Oncology service, Sodium level at discharge, Procedure during index hospitalization, Index hospitalization Type (nonelective), number of Admissions in the past year, and Length of stay) models, which were both derived to predict 30‐day readmissions among general medical inpatients and were intended to help clinicians identify high‐risk patients to target for discharge interventions.[9, 10, 15] We assessed each model's performance in our validation cohort, calculating the C statistic, integrated discrimination index (IDI), and net reclassification index (NRI) compared to the full‐stay model. IDI is a summary measure of both discrimination and reclassification, where more positive values suggest improvement in model performance in both these domains compared to a reference model.[28] The NRI is defined as the sum of the net proportions of correctly reclassified persons with and without the event of interest.[29] The theoretical range of values is 2 to 2, with more positive values indicating improved net reclassification compared to a reference model. Here, we calculated a category‐based NRI to evaluate the performance of models in correctly classifying individuals with and without readmissions into the highest readmission risk quintile versus the lowest 4 risk quintiles compared to the full‐stay EHR model.[29] This prespecified cutoff is relevant for hospitals interested in identifying the highest‐risk individuals for targeted intervention.[6] Because some hospitals may be able to target a greater number of individuals for intervention, we performed a sensitivity analysis by assessing category‐based NRI for reclassification into the top 2 risk quintiles versus the lowest 3 risk quintiles and found no meaningful difference in our results (data not shown). Finally, we qualitatively assessed calibration of comparator models in our validation cohort by comparing predicted probability to observed probability of readmission by quintiles of risk for each model. We conducted all analyses using Stata 12.1 (StataCorp, College Station, TX). This study was approved by the UT Southwestern Medical Center institutional review board.
RESULTS
Overall, 32,922 index hospitalizations were included in our study cohort; 12.7% resulted in a 30‐day readmission (see Supporting Figure 1 in the online version of this article). Individuals had a mean age of 62 years and had diverse race/ethnicity and primary insurance status; half were female (Table 1). The study sample was randomly split into a derivation cohort (50%, n = 16,492) and validation cohort (50%, n = 16,430). Individuals in the derivation cohort with a 30‐day readmission had markedly different socioeconomic and clinical characteristics compared to those not readmitted (Table 1).
Entire Cohort, N = 32,922 | Derivation Cohort, N = 16,492 | |||
---|---|---|---|---|
No Readmission, N = 14,312 |
Readmission, N = 2,180 |
P Value | ||
| ||||
Demographic characteristics | ||||
Age, y, mean (SD) | 62 (17.3) | 61 (17.4) | 64 (17.0) | 0.001 |
Female, n (%) | 17,715 (53.8) | 7,694 (53.8) | 1,163 (53.3) | 0.72 |
Race/ethnicity | 0.001 | |||
White | 21,359 (64.9) | 9,329 (65.2) | 1,361 (62.4) | |
Black | 5,964 (18.1) | 2,520 (17.6) | 434 (19.9) | |
Hispanic | 4,452 (13.5) | 1,931 (13.5) | 338 (15.5) | |
Other | 1,147 (3.5) | 532 (3.7) | 47 (2.2) | |
Marital status, n (%) | 0.001 | |||
Single | 8,076 (24.5) | 3,516 (24.6) | 514 (23.6) | |
Married | 13,394 (40.7) | 5,950 (41.6) | 812 (37.3) | |
Separated/divorced | 3,468 (10.5) | 1,460 (10.2) | 251 (11.5) | |
Widowed | 4,487 (13.7) | 1,868 (13.1) | 388 (17.8) | |
Other | 3,497 (10.6) | 1,518 (10.6) | 215 (9.9) | |
Primary payer, n (%) | 0.001 | |||
Private | 13,090 (39.8) | 5,855 (40.9) | 726 (33.3) | |
Medicare | 13,015 (39.5) | 5,597 (39.1) | 987 (45.3) | |
Medicaid | 2,204 (6.7) | 852 (5.9) | 242 (11.1) | |
Charity, self‐pay, or other | 4,613 (14.0) | 2,008 (14.0) | 225 (10.3) | |
High‐poverty neighborhood, n (%)* | 7,468 (22.7) | 3,208 (22.4) | 548 (25.1) | 0.001 |
Utilization history | ||||
1 ED visits in past year, n (%) | 9,299 (28.2) | 3,793 (26.5) | 823 (37.8) | 0.001 |
1 hospitalizations in past year, n (%) | 10,189 (30.9) | 4,074 (28.5) | 1,012 (46.4) | 0.001 |
Clinical factors from first day of hospitalization | ||||
Nonelective admission, n (%) | 27,818 (84.5) | 11,960 (83.6) | 1,960 (89.9) | 0.001 |
Charlson Comorbidity Index, median (IQR)∥ | 0 (01) | 0 (00) | 0 (03) | 0.001 |
Laboratory abnormalities within 24 hours of admission | ||||
Albumin <2 g/dL | 355 (1.1) | 119 (0.8) | 46 (2.1) | 0.001 |
Albumin 23 g/dL | 4,732 (14.4) | 1,956 (13.7) | 458 (21.0) | 0.001 |
Aspartate aminotransferase >40 U/L | 4,610 (14.0) | 1,922 (13.4) | 383 (17.6) | 0.001 |
Creatine phosphokinase <60 g/L | 3,728 (11.3) | 1,536 (10.7) | 330 (15.1) | 0.001 |
Mean corpuscular volume >100 fL/red cell | 1,346 (4.1) | 537 (3.8) | 134 (6.2) | 0.001 |
Platelets <90 103/L | 912 (2.8) | 357 (2.5) | 116 (5.3) | 0.001 |
Platelets >350 103/L | 3,332 (10.1) | 1,433 (10.0) | 283 (13.0) | 0.001 |
Prothrombin time >35 seconds | 248 (0.8) | 90 (0.6) | 35 (1.6) | 0.001 |
Clinical factors from remainder of hospital stay | ||||
Length of stay, d, median (IQR) | 4 (26) | 4 (26) | 5 (38) | 0.001 |
ICU transfer after first 24 hours, n (%) | 988 (3.0) | 408 (2.9) | 94 (4.3) | 0.001 |
Hospital complications, n (%) | ||||
Clostridium difficile infection | 119 (0.4) | 44 (0.3) | 24 (1.1) | 0.001 |
Pressure ulcer | 358 (1.1) | 126 (0.9) | 46 (2.1) | 0.001 |
Venous thromboembolism | 301 (0.9) | 112 (0.8) | 34 (1.6) | 0.001 |
Respiratory failure | 1,048 (3.2) | 463 (3.2) | 112 (5.1) | 0.001 |
Central line‐associated bloodstream infection | 22 (0.07) | 6 (0.04) | 5 (0.23) | 0.005 |
Catheter‐associated urinary tract infection | 47 (0.14) | 20 (0.14) | 6 (0.28) | 0.15 |
Acute myocardial infarction | 293 (0.9) | 110 (0.8) | 32 (1.5) | 0.001 |
Pneumonia | 1,754 (5.3) | 719 (5.0) | 154 (7.1) | 0.001 |
Sepsis | 853 (2.6) | 368 (2.6) | 73 (3.4) | 0.04 |
Blood transfusion during hospitalization, n (%) | 4,511 (13.7) | 1,837 (12.8) | 425 (19.5) | 0.001 |
Laboratory abnormalities at discharge# | ||||
Blood urea nitrogen >20 mg/dL, n (%) | 10,014 (30.4) | 4,077 (28.5) | 929 (42.6) | 0.001 |
Sodium <135 mEq/L, n (%) | 4,583 (13.9) | 1,850 (12.9) | 440 (20.2) | 0.001 |
Hematocrit 27 | 3,104 (9.4) | 1,231 (8.6) | 287 (13.2) | 0.001 |
1 vital sign instability at discharge, n (%)# | 6,192 (18.8) | 2,624 (18.3) | 525 (24.1) | 0.001 |
Discharge location, n (%) | 0.001 | |||
Home | 23,339 (70.9) | 10,282 (71.8) | 1,383 (63.4) | |
Home health | 3,185 (9.7) | 1,356 (9.5) | 234 (10.7) | |
Postacute care** | 5,990 (18.2) | 2,496 (17.4) | 549 (25.2) | |
Hospice | 408 (1.2) | 178 (1.2) | 14 (0.6) |
Derivation and Validation of the Full‐Stay EHR Model for 30‐Day Readmission
Our final model included 24 independent variables, including demographic characteristics, utilization history, clinical factors from the first day of admission, and clinical factors from the remainder of the hospital stay (Table 2). The strongest independent predictor of readmission was hospital‐acquired Clostridium difficile infection (adjusted odds ratio [AOR]: 2.03, 95% confidence interval [CI] 1.18‐3.48); other hospital‐acquired complications including pressure ulcers and venous thromboembolism were also significant predictors. Though having Medicaid was associated with increased odds of readmission (AOR: 1.55, 95% CI: 1.31‐1.83), other zip codelevel measures of socioeconomic disadvantage were not predictive and were not included in the final model. Being discharged to hospice was associated with markedly lower odds of readmission (AOR: 0.23, 95% CI: 0.13‐0.40).
Odds Ratio (95% CI) | ||
---|---|---|
Univariate | Multivariate* | |
| ||
Demographic characteristics | ||
Age, per 10 years | 1.08 (1.051.11) | 1.07 (1.041.10) |
Medicaid | 1.97 (1.702.29) | 1.55 (1.311.83) |
Widow | 1.44 (1.281.63) | 1.27 (1.111.45) |
Utilization history | ||
Prior ED visit, per visit | 1.08 (1.061.10) | 1.04 (1.021.06) |
Prior hospitalization, per hospitalization | 1.30 (1.271.34) | 1.16 (1.121.20) |
Hospital and clinical factors from first day of hospitalization | ||
Nonelective admission | 1.75 (1.512.03) | 1.42 (1.221.65) |
Charlson Comorbidity Index, per point | 1.19 (1.171.21) | 1.06 (1.041.09) |
Laboratory abnormalities within 24 hours of admission | ||
Albumin <2 g/dL | 2.57 (1.823.62) | 1.52 (1.052.21) |
Albumin 23 g/dL | 1.68 (1.501.88) | 1.20 (1.061.36) |
Aspartate aminotransferase >40 U/L | 1.37 (1.221.55) | 1.21 (1.061.38) |
Creatine phosphokinase <60 g/L | 1.48 (1.301.69) | 1.28 (1.111.46) |
Mean corpuscular volume >100 fL/red cell | 1.68 (1.382.04) | 1.32 (1.071.62) |
Platelets <90 103/L | 2.20 (1.772.72) | 1.56 (1.231.97) |
Platelets >350 103/L | 1.34 (1.171.54) | 1.24 (1.081.44) |
Prothrombin time >35 seconds | 2.58 (1.743.82) | 1.92 (1.272.90) |
Hospital and clinical factors from remainder of hospital stay | ||
Length of stay, per day | 1.08 (1.071.09) | 1.06 (1.041.07) |
Hospital complications | ||
Clostridium difficile infection | 3.61 (2.195.95) | 2.03 (1.183.48) |
Pressure ulcer | 2.43 (1.733.41) | 1.64 (1.152.34) |
Venous thromboembolism | 2.01 (1.362.96) | 1.55 (1.032.32) |
Laboratory abnormalities at discharge | ||
Blood urea nitrogen >20 mg/dL | 1.86 (1.702.04) | 1.37 (1.241.52) |
Sodium <135 mEq/L | 1.70 (1.521.91) | 1.34 (1.181.51) |
Hematocrit 27 | 1.61 (1.401.85) | 1.22 (1.051.41) |
Vital sign instability at discharge, per instability | 1.29 (1.201.40) | 1.25 (1.151.36) |
Discharged to hospice | 0.51 (0.300.89) | 0.23 (0.130.40) |
In our validation cohort, the full‐stay EHR model had fair discrimination, with a C statistic of 0.69 (95% CI: 0.68‐0.70) (Table 3). The full‐stay EHR model was well calibrated across all quintiles of risk, with slight overestimation of predicted risk in the lowest and highest quintiles (Figure 1a) (see Supporting Table 5 in the online version of this article). It also effectively stratified individuals across a broad range of predicted readmission risk from 4.1% in the lowest decile to 36.5% in the highest decile (Table 3).
Model Name | C‐Statistic (95% CI) | IDI, % (95% CI) | NRI (95% CI) | Average Predicted Risk, % | |
---|---|---|---|---|---|
Lowest Decile | Highest Decile | ||||
| |||||
Full‐stay EHR model | |||||
Derivation cohort | 0.72 (0.70 to 0.73) | 4.1 | 36.5 | ||
Validation cohort | 0.69 (0.68 to 0.70) | [Reference] | [Reference] | 4.1 | 36.5 |
First‐day EHR model | 0.67 (0.66 to 0.68) | 1.2 (1.4 to 1.0) | 0.020 (0.038 to 0.002) | 5.8 | 31.9 |
LACE model | 0.65 (0.64 to 0.66) | 2.6 (2.9 to 2.3) | 0.046 (0.067 to 0.024) | 6.1 | 27.5 |
HOSPITAL model | 0.64 (0.62 to 0.65) | 3.2 (3.5 to 2.9) | 0.058 (0.080 to 0.035) | 6.7 | 26.6 |
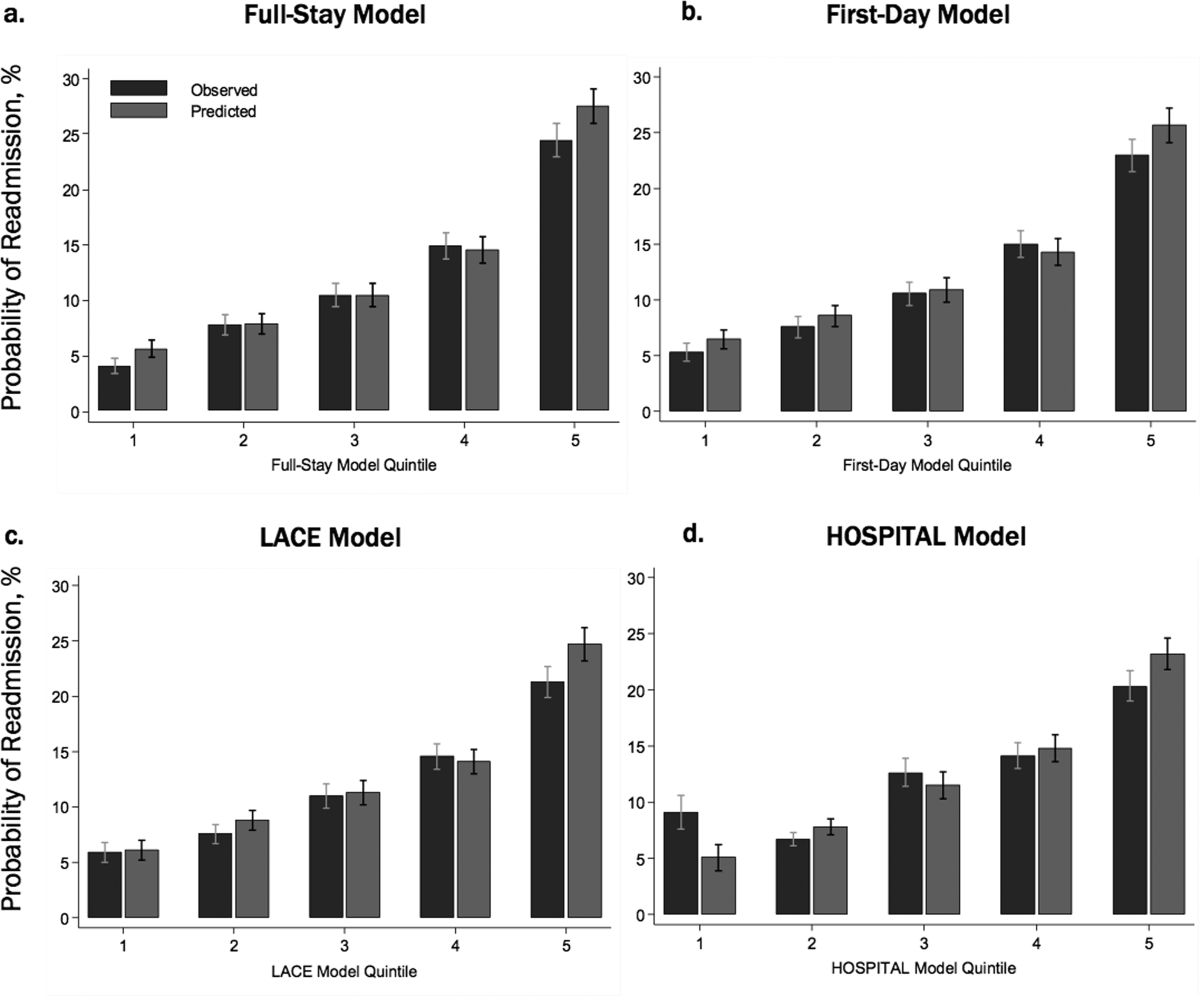
Comparing the Performance of the Full‐Stay EHR Model to Other Models
The full‐stay EHR model had better discrimination compared to the first‐day EHR model and the LACE and HOSPITAL models, though the magnitude of improvement was modest (Table 3). The full‐stay EHR model also stratified individuals across a broader range of readmission risk, and was better able to discriminate and classify those in the highest quintile of risk from those in the lowest 4 quintiles of risk compared to other models as assessed by the IDI and NRI (Table 3) (see Supporting Tables 14 and Supporting Figure 2 in the online version of this article). In terms of model calibration, both the first‐day EHR and LACE models were also well calibrated, whereas the HOSPITAL model was less robust (Figure 1).
The diagnostic accuracy of the full‐stay EHR model in correctly predicting those in the highest quintile of risk was better than that of the first‐day, LACE, and HOSPITAL models, though overall improvements in the sensitivity, specificity, positive and negative predictive values, and positive and negative likelihood ratios were also modest (see Supporting Table 6 in the online version of this article).
DISCUSSION
In this study, we used clinically detailed EHR data from the entire hospitalization on 32,922 individuals treated in 6 diverse hospitals to develop an all‐payer, multicondition readmission risk‐prediction model. To our knowledge, this is the first 30‐day hospital readmission risk‐prediction model to use a comprehensive set of factors from EHR data from the entire hospital stay. Prior EHR‐based models have focused exclusively on data available on or prior to the first day of admission, which account for clinical severity on admission but do not account for factors uncovered during the inpatient stay that influence the chance of a postdischarge adverse outcome.[15, 30] We specifically assessed the prognostic impact of a comprehensive set of factors from the entire index hospitalization, including hospital‐acquired complications, clinical trajectory, and stability on discharge in predicting hospital readmissions. Our full‐stay EHR model had statistically better discrimination, calibration, and diagnostic accuracy than our existing all‐cause first‐day EHR model[15] and 2 previously published readmissions models that included more limited information from hospitalization (such as length of stay).[9, 10] However, although the more complicated full‐stay EHR model was statistically better than previously published models, we were surprised that the predictive performance was only modestly improved despite the inclusion of many additional clinically relevant prognostic factors.
Taken together, our study has several important implications. First, the added complexity and resource intensity of implementing a full‐stay EHR model yields only modestly improved readmission risk prediction. Thus, hospitals and healthcare systems interested in targeting their highest‐risk individuals for interventions to reduce 30‐day readmission should consider doing so within the first day of hospital admission. Our group's previously derived and validated first‐day EHR model, which used data only from the first day of admission, qualitatively performed nearly as well as the full‐stay EHR model.[15] Additionally, a recent study using only preadmission EHR data to predict 30‐day readmissions also achieved similar discrimination and diagnostic accuracy as our full‐stay model.[30]
Second, the field of readmissions risk‐prediction modeling may be reaching the maximum achievable model performance using data that are currently available in the EHR. Our limited ability to accurately predict all‐cause 30‐day readmission risk may reflect the influence of currently unmeasured patient, system, and community factors on readmissions.[31, 32, 33] Due to the constraints of data collected in the EHR, we were unable to include several patient‐level clinical characteristics associated with hospital readmission, including self‐perceived health status, functional impairment, and cognition.[33, 34, 35, 36] However, given their modest effect sizes (ORs ranging from 1.062.10), adequately measuring and including these risk factors in our model may not meaningfully improve model performance and diagnostic accuracy. Further, many social and behavioral patient‐level factors are also not consistently available in EHR data. Though we explored the role of several neighborhood‐level socioeconomic measuresincluding prevalence of poverty, median income, education, and unemploymentwe found that none were significantly associated with 30‐day readmissions. These particular measures may have been inadequate to characterize individual‐level social and behavioral factors, as several previous studies have demonstrated that patient‐level factors such as social support, substance abuse, and medication and visit adherence can influence readmission risk in heart failure and pneumonia.[11, 16, 22, 25] This underscores the need for more standardized routine collection of data across functional, social, and behavioral domains in clinical settings, as recently championed by the Institute of Medicine.[11, 37] Integrating data from outside the EHR on postdischarge health behaviors, self‐management, follow‐up care, recovery, and home environment may be another important but untapped strategy for further improving prediction of readmissions.[25, 38]
Third, a multicondition readmission risk‐prediction model may be a less effective strategy than more customized disease‐specific models for selected conditions associated with high 30‐day readmission rates. Our group's previously derived and internally validated models for heart failure and human immunodeficiency virus had superior discrimination compared to our full‐stay EHR model (C statistic of 0.72 for each).[11, 13] However, given differences in the included population and time periods studied, a head‐to‐head comparison of these different strategies is needed to assess differences in model performance and utility.
Our study had several strengths. To our knowledge, this is the first study to rigorously measure the additive influence of in‐hospital complications, clinical trajectory, and stability on discharge on the risk of 30‐day hospital readmission. Additionally, our study included a large, diverse study population that included all payers, all ages of adults, a mix of community, academic, and safety net hospitals, and individuals from a broad array of racial/ethnic and socioeconomic backgrounds.
Our results should be interpreted in light of several limitations. First, though we sought to represent a diverse group of hospitals, all study sites were located within north Texas and generalizability to other regions is uncertain. Second, our ascertainment of prior hospitalizations and readmissions was more inclusive than what could be typically accomplished in real time using only EHR data from a single clinical site. We performed a sensitivity analysis using only prior utilization data available within the EHR from the index hospital with no meaningful difference in our findings (data not shown). Additionally, a recent study found that 30‐day readmissions occur at the index hospital for over 75% of events, suggesting that 30‐day readmissions are fairly comprehensively captured even with only single‐site data.[39] Third, we were not able to include data on outpatient visits before or after the index hospitalization, which may influence the risk of readmission.[1, 40]
In conclusion, incorporating clinically granular EHR data from the entire course of hospitalization modestly improves prediction of 30‐day readmissions compared to models that only include information from the first 24 hours of hospital admission or models that use far fewer variables. However, given the limited improvement in prediction, our findings suggest that from the practical perspective of implementing real‐time models to identify those at highest risk for readmission, it may not be worth the added complexity of waiting until the end of a hospitalization to leverage additional data on hospital complications, and the trajectory of laboratory and vital sign values currently available in the EHR. Further improvement in prediction of readmissions will likely require accounting for psychosocial, functional, behavioral, and postdischarge factors not currently present in the inpatient EHR.
Disclosures: This study was presented at the Society of Hospital Medicine 2015 Annual Meeting in National Harbor, Maryland, and the Society of General Internal Medicine 2015 Annual Meeting in Toronto, Canada. This work was supported by the Agency for Healthcare Research and Qualityfunded UT Southwestern Center for Patient‐Centered Outcomes Research (1R24HS022418‐01) and the Commonwealth Foundation (#20100323). Drs. Nguyen and Makam received funding from the UT Southwestern KL2 Scholars Program (NIH/NCATS KL2 TR001103). Dr. Halm was also supported in part by NIH/NCATS U54 RFA‐TR‐12‐006. The study sponsors had no role in the design and conduct of the study; collection, management, analysis, and interpretation of the data; and preparation, review, or approval of the manuscript. The authors have no conflicts of interest to disclose.
- Rehospitalizations among patients in the Medicare fee‐for‐service program. N Engl J Med. 2009;360(14):1418–1428. , , .
- Proportion of hospital readmissions deemed avoidable: a systematic review. CMAJ. 2011;183(7):E391–E402. , , , , .
- Hospital‐initiated transitional care interventions as a patient safety strategy: a systematic review. Ann Intern Med. 2013;158(5 pt 2):433–440. , , , , , .
- Interventions to reduce 30‐day rehospitalization: a systematic review. Ann Intern Med. 2011;155(8):520–528. , , , , .
- Interventions to Improve Care Transitions at Hospital Discharge. Rockville, MD: Agency for Healthcare Research and Quality; 2013. , , , , , .
- Allocating scarce resources in real‐time to reduce heart failure readmissions: a prospective, controlled study. BMJ Qual Saf. 2013;22(12):998–1005. , , , et al.
- Implementing electronic health care predictive analytics: considerations and challenges. Health Aff (Millwood). 2014;33(7):1148–1154. , , , , .
- Risk prediction models for hospital readmission: a systematic review. JAMA. 2011;306(15):1688–1698. , , , et al.
- Derivation and validation of an index to predict early death or unplanned readmission after discharge from hospital to the community. CMAJ. 2010;182(6):551–557. , , , et al.
- Potentially avoidable 30‐day hospital readmissions in medical patients: derivation and validation of a prediction model. JAMA Intern Med. 2013;173(8):632–638. , , , .
- An automated model to identify heart failure patients at risk for 30‐day readmission or death using electronic medical record data. Med Care. 2010;48(11):981–988. , , , et al.
- An automated model using electronic medical record data identifies patients with cirrhosis at high risk for readmission. Clin Gastroenterol Hepatol. 2013;11(10):1335–1341.e1331. , , , et al.
- An electronic medical record‐based model to predict 30‐day risk of readmission and death among HIV‐infected inpatients. J Acquir Immune Defic Syndr. 2012;61(3):349–358. , , , , , .
- Development and use of an administrative claims measure for profiling hospital‐wide performance on 30‐day unplanned readmission. Ann Intern Med. 2014;161(10 suppl):S66–S75. , , , et al.
- Electronic medical record‐based multicondition models to predict the risk of 30 day readmission or death among adult medicine patients: validation and comparison to existing models. BMC Med Inform Decis Mak. 2015;15(1):39. , , , et al.
- Linking electronic health record‐extracted psychosocial data in real‐time to risk of readmission for heart failure. Psychosomatics. 2011;52(4):319–327. , , , et al.
- A conceptual framework for the study of early readmission as an indicator of quality of care. Soc Sci Med. 1996;43(11):1533–1541. , .
- Hospital readmission performance and patterns of readmission: retrospective cohort study of Medicare admissions. BMJ. 2013;347:f6571. , , , et al.
- Getting more performance from performance measurement. N Engl J Med. 2014;371(23):2145–2147. , , , , , .
- Hospital strategy uptake and reductions in unplanned readmission rates for patients with heart failure: a prospective study. J Gen Intern Med. 2015;30(5):605–611. , , , et al.
- Post‐hospital syndrome—an acquired, transient condition of generalized risk. N Engl J Med. 2013;368(2):100–102. .
- Impact of social factors on risk of readmission or mortality in pneumonia and heart failure: systematic review. J Gen Intern Med. 2013;28(2):269–282. , , , et al.
- Effect of clinical and social risk factors on hospital profiling for stroke readmission: a cohort study. Ann Intern Med. 2014;161(11):775–784. , , , , , .
- Neighborhood socioeconomic disadvantage and 30‐day rehospitalization: a retrospective cohort study. Ann Intern Med. 2014;161(11):765–774. , , , et al.
- Postdischarge environmental and socioeconomic factors and the likelihood of early hospital readmission among community‐dwelling Medicare beneficiaries. Gerontologist. 2008;48(4):495–504. , , , , , .
- Socioeconomic status and readmissions: evidence from an urban teaching hospital. Health Aff (Millwood). 2014;33(5):778–785. , , .
- Adding socioeconomic data to hospital readmissions calculations may produce more useful results. Health Aff (Millwood). 2014;33(5):786–791. , , , .
- Evaluating the added predictive ability of a new marker: from area under the ROC curve to reclassification and beyond. Stat Med. 2008;27(2):157–172; discussion 207–212. , , , .
- Net reclassification improvement: computation, interpretation, and controversies: a literature review and clinician's guide. Ann Intern Med. 2014;160(2):122–131. , , , , .
- Predicting 30‐day readmissions with preadmission electronic health record data. Med Care. 2015;53(3):283–289. , , , , , .
- Hospital readmissions—not just a measure of quality. JAMA. 2011;306(16):1796–1797. , .
- Thirty‐day readmissions—truth and consequences. N Engl J Med. 2012;366(15):1366–1369. , .
- Functional impairment and hospital readmission in medicare seniors. JAMA Intern Med. 2015;175(4):559–565. , , , .
- Clinical and sociodemographic risk factors for readmission of Medicare beneficiaries. Health Care Financ Rev. 1988;10(1):27–36. , , .
- Mini‐cog performance: novel marker of post discharge risk among patients hospitalized for heart failure. Circ Heart Fail. 2015;8(1):8–16. , , , , , .
- Association of impaired functional status at hospital discharge and subsequent rehospitalization. J Hosp Med. 2014;9(5):277–282. , , , , , .
- Patients in context—EHR capture of social and behavioral determinants of health. N Engl J Med. 2015;372(8):698–701. , .
- Envisioning a social‐health information exchange as a platform to support a patient‐centered medical neighborhood: a feasibility study. J Gen Intern Med. 2015;30(1):60–67. , , , , .
- Patient factors contributing to variation in same‐hospital readmission rate. Med Care Res Review. 2015;72(3):338–358. , , , , , .
- Does increased access to primary care reduce hospital readmissions? Veterans Affairs Cooperative Study Group on Primary Care and Hospital Readmission. N Engl J Med. 1996;334(22):1441–1447. , , .
Unplanned hospital readmissions are frequent, costly, and potentially avoidable.[1, 2] Due to major federal financial readmissions penalties targeting excessive 30‐day readmissions, there is increasing attention to implementing hospital‐initiated interventions to reduce readmissions.[3, 4] However, universal enrollment of all hospitalized patients into such programs may be too resource intensive for many hospitals.[5] To optimize efficiency and effectiveness, interventions should be targeted to individuals most likely to benefit.[6, 7] However, existing readmission risk‐prediction models have achieved only modest discrimination, have largely used administrative claims data not available until months after discharge, or are limited to only a subset of patients with Medicare or a specific clinical condition.[8, 9, 10, 11, 12, 13, 14] These limitations have precluded accurate identification of high‐risk individuals in an all‐payer general medical inpatient population to provide actionable information for intervention prior to discharge.
Approaches using electronic health record (EHR) data could allow early identification of high‐risk patients during the index hospitalization to enable initiation of interventions prior to discharge. To date, such strategies have relied largely on EHR data from the day of admission.[15, 16] However, given that variation in 30‐day readmission rates are thought to reflect the quality of in‐hospital care, incorporating EHR data from the entire hospital stay to reflect hospital care processes and clinical trajectory may more accurately identify at‐risk patients.[17, 18, 19, 20] Improved accuracy in risk prediction would help better target intervention efforts in the immediate postdischarge period, an interval characterized by heightened vulnerability for adverse events.[21]
To help hospitals target transitional care interventions more effectively to high‐risk individuals prior to discharge, we derived and validated a readmissions risk‐prediction model incorporating EHR data from the entire course of the index hospitalization, which we termed the full‐stay EHR model. We also compared the full‐stay EHR model performance to our group's previously derived prediction model based on EHR data on the day of admission, termed the first‐day EHR model, as well as to 2 other validated readmission models similarly intended to yield near real‐time risk predictions prior to or shortly after hospital discharge.[9, 10, 15]
METHODS
Study Design, Population, and Data Sources
We conducted an observational cohort study using EHR data from 6 hospitals in the DallasFort Worth metroplex between November 1, 2009 and October 30, 2010 using the same EHR system (Epic Systems Corp., Verona, WI). One site was a university‐affiliated safety net hospital; the remaining 5 sites were teaching and nonteaching community sites.
We included consecutive hospitalizations among adults 18 years old discharged alive from any medicine inpatient service. For individuals with multiple hospitalizations during the study period, we included only the first hospitalization. We excluded individuals who died during the index hospitalization, were transferred to another acute care facility, left against medical advice, or who died outside of the hospital within 30 days of discharge. For model derivation, we randomly split the sample into separate derivation (50%) and validation cohorts (50%).
Outcomes
The primary outcome was 30‐day hospital readmission, defined as a nonelective hospitalization within 30 days of discharge to any of 75 acute care hospitals within a 100‐mile radius of Dallas, ascertained from an all‐payer regional hospitalization database. Nonelective hospitalizations included all hospitalizations classified as a emergency, urgent, or trauma, and excluded those classified as elective as per the Centers for Medicare and Medicaid Services Claim Inpatient Admission Type Code definitions.
Predictor Variables for the Full‐Stay EHR Model
The full‐stay EHR model was iteratively developed from our group's previously derived and validated risk‐prediction model using EHR data available on admission (first‐day EHR model).[15] For the full‐stay EHR model, we included all predictor variables included in our published first‐day EHR model as candidate risk factors. Based on prior literature, we additionally expanded candidate predictors available on admission to include marital status (proxy for social isolation) and socioeconomic disadvantage (percent poverty, unemployment, median income, and educational attainment by zip code of residence as proxy measures of the social and built environment).[22, 23, 24, 25, 26, 27] We also expanded the ascertainment of prior hospitalization to include admissions at both the index hospital and any of 75 acute care hospitals from the same, separate all‐payer regional hospitalization database used to ascertain 30‐day readmissions.
Candidate predictors from the remainder of the hospital stay (ie, following the first 24 hours of admission) were included if they were: (1) available in the EHR of all participating hospitals, (2) routinely collected or available at the time of hospital discharge, and (3) plausible predictors of adverse outcomes based on prior literature and clinical expertise. These included length of stay, in‐hospital complications, transfer to an intensive or coronary care unit, blood transfusions, vital sign instabilities within 24 hours of discharge, select laboratory values at time of discharge, and disposition status. We also assessed trajectories of vital signs and selected laboratory values (defined as changes in these measures from admission to discharge).
Statistical Analysis
Model Derivation
Univariate relationships between readmission and each of the candidate predictors were assessed in the derivation cohort using a prespecified significance threshold of P 0.05. We included all factors from our previously derived and validated first‐day EHR model as candidate predictors.[15] Continuous laboratory and vital sign values at the time of discharge were categorized based on clinically meaningful cutoffs; predictors with missing values were assumed to be normal (<1% missing for each variable). Significant univariate candidate variables were entered in a multivariate logistic regression model using stepwise backward selection with a prespecified significance threshold of P 0.05. We performed several sensitivity analyses to confirm the robustness of our model. First, we alternately derived the full‐stay model using stepwise forward selection. Second, we forced in all significant variables from our first‐day EHR model, and entered the candidate variables from the remainder of the hospital stay using both stepwise backward and forward selection separately. Third, prespecified interactions between variables were evaluated for inclusion. Though final predictors varied slightly between the different approaches, discrimination of each model was similar to the model derived using our primary analytic approach (C statistics 0.01, data not shown).
Model Validation
We assessed model discrimination and calibration of the derived full‐stay EHR model using the validation cohort. Model discrimination was estimated by the C statistic. The C statistic represents the probability that, given 2 hospitalized individuals (1 who was readmitted and the other who was not), the model will predict a higher risk for the readmitted patient than for the nonreadmitted patient. Model calibration was assessed by comparing predicted to observed probabilities of readmission by quintiles of risk, and with the Hosmer‐Lemeshow goodness‐of‐fit test.
Comparison to Existing Models
We compared the full‐stay EHR model performance to 3 previously published models: our group's first‐day EHR model, and the LACE (includes Length of stay, Acute (nonelective) admission status, Charlson Comorbidity Index, and Emergency department visits in the past year) and HOSPITAL (includes Hemoglobin at discharge, discharge from Oncology service, Sodium level at discharge, Procedure during index hospitalization, Index hospitalization Type (nonelective), number of Admissions in the past year, and Length of stay) models, which were both derived to predict 30‐day readmissions among general medical inpatients and were intended to help clinicians identify high‐risk patients to target for discharge interventions.[9, 10, 15] We assessed each model's performance in our validation cohort, calculating the C statistic, integrated discrimination index (IDI), and net reclassification index (NRI) compared to the full‐stay model. IDI is a summary measure of both discrimination and reclassification, where more positive values suggest improvement in model performance in both these domains compared to a reference model.[28] The NRI is defined as the sum of the net proportions of correctly reclassified persons with and without the event of interest.[29] The theoretical range of values is 2 to 2, with more positive values indicating improved net reclassification compared to a reference model. Here, we calculated a category‐based NRI to evaluate the performance of models in correctly classifying individuals with and without readmissions into the highest readmission risk quintile versus the lowest 4 risk quintiles compared to the full‐stay EHR model.[29] This prespecified cutoff is relevant for hospitals interested in identifying the highest‐risk individuals for targeted intervention.[6] Because some hospitals may be able to target a greater number of individuals for intervention, we performed a sensitivity analysis by assessing category‐based NRI for reclassification into the top 2 risk quintiles versus the lowest 3 risk quintiles and found no meaningful difference in our results (data not shown). Finally, we qualitatively assessed calibration of comparator models in our validation cohort by comparing predicted probability to observed probability of readmission by quintiles of risk for each model. We conducted all analyses using Stata 12.1 (StataCorp, College Station, TX). This study was approved by the UT Southwestern Medical Center institutional review board.
RESULTS
Overall, 32,922 index hospitalizations were included in our study cohort; 12.7% resulted in a 30‐day readmission (see Supporting Figure 1 in the online version of this article). Individuals had a mean age of 62 years and had diverse race/ethnicity and primary insurance status; half were female (Table 1). The study sample was randomly split into a derivation cohort (50%, n = 16,492) and validation cohort (50%, n = 16,430). Individuals in the derivation cohort with a 30‐day readmission had markedly different socioeconomic and clinical characteristics compared to those not readmitted (Table 1).
Entire Cohort, N = 32,922 | Derivation Cohort, N = 16,492 | |||
---|---|---|---|---|
No Readmission, N = 14,312 |
Readmission, N = 2,180 |
P Value | ||
| ||||
Demographic characteristics | ||||
Age, y, mean (SD) | 62 (17.3) | 61 (17.4) | 64 (17.0) | 0.001 |
Female, n (%) | 17,715 (53.8) | 7,694 (53.8) | 1,163 (53.3) | 0.72 |
Race/ethnicity | 0.001 | |||
White | 21,359 (64.9) | 9,329 (65.2) | 1,361 (62.4) | |
Black | 5,964 (18.1) | 2,520 (17.6) | 434 (19.9) | |
Hispanic | 4,452 (13.5) | 1,931 (13.5) | 338 (15.5) | |
Other | 1,147 (3.5) | 532 (3.7) | 47 (2.2) | |
Marital status, n (%) | 0.001 | |||
Single | 8,076 (24.5) | 3,516 (24.6) | 514 (23.6) | |
Married | 13,394 (40.7) | 5,950 (41.6) | 812 (37.3) | |
Separated/divorced | 3,468 (10.5) | 1,460 (10.2) | 251 (11.5) | |
Widowed | 4,487 (13.7) | 1,868 (13.1) | 388 (17.8) | |
Other | 3,497 (10.6) | 1,518 (10.6) | 215 (9.9) | |
Primary payer, n (%) | 0.001 | |||
Private | 13,090 (39.8) | 5,855 (40.9) | 726 (33.3) | |
Medicare | 13,015 (39.5) | 5,597 (39.1) | 987 (45.3) | |
Medicaid | 2,204 (6.7) | 852 (5.9) | 242 (11.1) | |
Charity, self‐pay, or other | 4,613 (14.0) | 2,008 (14.0) | 225 (10.3) | |
High‐poverty neighborhood, n (%)* | 7,468 (22.7) | 3,208 (22.4) | 548 (25.1) | 0.001 |
Utilization history | ||||
1 ED visits in past year, n (%) | 9,299 (28.2) | 3,793 (26.5) | 823 (37.8) | 0.001 |
1 hospitalizations in past year, n (%) | 10,189 (30.9) | 4,074 (28.5) | 1,012 (46.4) | 0.001 |
Clinical factors from first day of hospitalization | ||||
Nonelective admission, n (%) | 27,818 (84.5) | 11,960 (83.6) | 1,960 (89.9) | 0.001 |
Charlson Comorbidity Index, median (IQR)∥ | 0 (01) | 0 (00) | 0 (03) | 0.001 |
Laboratory abnormalities within 24 hours of admission | ||||
Albumin <2 g/dL | 355 (1.1) | 119 (0.8) | 46 (2.1) | 0.001 |
Albumin 23 g/dL | 4,732 (14.4) | 1,956 (13.7) | 458 (21.0) | 0.001 |
Aspartate aminotransferase >40 U/L | 4,610 (14.0) | 1,922 (13.4) | 383 (17.6) | 0.001 |
Creatine phosphokinase <60 g/L | 3,728 (11.3) | 1,536 (10.7) | 330 (15.1) | 0.001 |
Mean corpuscular volume >100 fL/red cell | 1,346 (4.1) | 537 (3.8) | 134 (6.2) | 0.001 |
Platelets <90 103/L | 912 (2.8) | 357 (2.5) | 116 (5.3) | 0.001 |
Platelets >350 103/L | 3,332 (10.1) | 1,433 (10.0) | 283 (13.0) | 0.001 |
Prothrombin time >35 seconds | 248 (0.8) | 90 (0.6) | 35 (1.6) | 0.001 |
Clinical factors from remainder of hospital stay | ||||
Length of stay, d, median (IQR) | 4 (26) | 4 (26) | 5 (38) | 0.001 |
ICU transfer after first 24 hours, n (%) | 988 (3.0) | 408 (2.9) | 94 (4.3) | 0.001 |
Hospital complications, n (%) | ||||
Clostridium difficile infection | 119 (0.4) | 44 (0.3) | 24 (1.1) | 0.001 |
Pressure ulcer | 358 (1.1) | 126 (0.9) | 46 (2.1) | 0.001 |
Venous thromboembolism | 301 (0.9) | 112 (0.8) | 34 (1.6) | 0.001 |
Respiratory failure | 1,048 (3.2) | 463 (3.2) | 112 (5.1) | 0.001 |
Central line‐associated bloodstream infection | 22 (0.07) | 6 (0.04) | 5 (0.23) | 0.005 |
Catheter‐associated urinary tract infection | 47 (0.14) | 20 (0.14) | 6 (0.28) | 0.15 |
Acute myocardial infarction | 293 (0.9) | 110 (0.8) | 32 (1.5) | 0.001 |
Pneumonia | 1,754 (5.3) | 719 (5.0) | 154 (7.1) | 0.001 |
Sepsis | 853 (2.6) | 368 (2.6) | 73 (3.4) | 0.04 |
Blood transfusion during hospitalization, n (%) | 4,511 (13.7) | 1,837 (12.8) | 425 (19.5) | 0.001 |
Laboratory abnormalities at discharge# | ||||
Blood urea nitrogen >20 mg/dL, n (%) | 10,014 (30.4) | 4,077 (28.5) | 929 (42.6) | 0.001 |
Sodium <135 mEq/L, n (%) | 4,583 (13.9) | 1,850 (12.9) | 440 (20.2) | 0.001 |
Hematocrit 27 | 3,104 (9.4) | 1,231 (8.6) | 287 (13.2) | 0.001 |
1 vital sign instability at discharge, n (%)# | 6,192 (18.8) | 2,624 (18.3) | 525 (24.1) | 0.001 |
Discharge location, n (%) | 0.001 | |||
Home | 23,339 (70.9) | 10,282 (71.8) | 1,383 (63.4) | |
Home health | 3,185 (9.7) | 1,356 (9.5) | 234 (10.7) | |
Postacute care** | 5,990 (18.2) | 2,496 (17.4) | 549 (25.2) | |
Hospice | 408 (1.2) | 178 (1.2) | 14 (0.6) |
Derivation and Validation of the Full‐Stay EHR Model for 30‐Day Readmission
Our final model included 24 independent variables, including demographic characteristics, utilization history, clinical factors from the first day of admission, and clinical factors from the remainder of the hospital stay (Table 2). The strongest independent predictor of readmission was hospital‐acquired Clostridium difficile infection (adjusted odds ratio [AOR]: 2.03, 95% confidence interval [CI] 1.18‐3.48); other hospital‐acquired complications including pressure ulcers and venous thromboembolism were also significant predictors. Though having Medicaid was associated with increased odds of readmission (AOR: 1.55, 95% CI: 1.31‐1.83), other zip codelevel measures of socioeconomic disadvantage were not predictive and were not included in the final model. Being discharged to hospice was associated with markedly lower odds of readmission (AOR: 0.23, 95% CI: 0.13‐0.40).
Odds Ratio (95% CI) | ||
---|---|---|
Univariate | Multivariate* | |
| ||
Demographic characteristics | ||
Age, per 10 years | 1.08 (1.051.11) | 1.07 (1.041.10) |
Medicaid | 1.97 (1.702.29) | 1.55 (1.311.83) |
Widow | 1.44 (1.281.63) | 1.27 (1.111.45) |
Utilization history | ||
Prior ED visit, per visit | 1.08 (1.061.10) | 1.04 (1.021.06) |
Prior hospitalization, per hospitalization | 1.30 (1.271.34) | 1.16 (1.121.20) |
Hospital and clinical factors from first day of hospitalization | ||
Nonelective admission | 1.75 (1.512.03) | 1.42 (1.221.65) |
Charlson Comorbidity Index, per point | 1.19 (1.171.21) | 1.06 (1.041.09) |
Laboratory abnormalities within 24 hours of admission | ||
Albumin <2 g/dL | 2.57 (1.823.62) | 1.52 (1.052.21) |
Albumin 23 g/dL | 1.68 (1.501.88) | 1.20 (1.061.36) |
Aspartate aminotransferase >40 U/L | 1.37 (1.221.55) | 1.21 (1.061.38) |
Creatine phosphokinase <60 g/L | 1.48 (1.301.69) | 1.28 (1.111.46) |
Mean corpuscular volume >100 fL/red cell | 1.68 (1.382.04) | 1.32 (1.071.62) |
Platelets <90 103/L | 2.20 (1.772.72) | 1.56 (1.231.97) |
Platelets >350 103/L | 1.34 (1.171.54) | 1.24 (1.081.44) |
Prothrombin time >35 seconds | 2.58 (1.743.82) | 1.92 (1.272.90) |
Hospital and clinical factors from remainder of hospital stay | ||
Length of stay, per day | 1.08 (1.071.09) | 1.06 (1.041.07) |
Hospital complications | ||
Clostridium difficile infection | 3.61 (2.195.95) | 2.03 (1.183.48) |
Pressure ulcer | 2.43 (1.733.41) | 1.64 (1.152.34) |
Venous thromboembolism | 2.01 (1.362.96) | 1.55 (1.032.32) |
Laboratory abnormalities at discharge | ||
Blood urea nitrogen >20 mg/dL | 1.86 (1.702.04) | 1.37 (1.241.52) |
Sodium <135 mEq/L | 1.70 (1.521.91) | 1.34 (1.181.51) |
Hematocrit 27 | 1.61 (1.401.85) | 1.22 (1.051.41) |
Vital sign instability at discharge, per instability | 1.29 (1.201.40) | 1.25 (1.151.36) |
Discharged to hospice | 0.51 (0.300.89) | 0.23 (0.130.40) |
In our validation cohort, the full‐stay EHR model had fair discrimination, with a C statistic of 0.69 (95% CI: 0.68‐0.70) (Table 3). The full‐stay EHR model was well calibrated across all quintiles of risk, with slight overestimation of predicted risk in the lowest and highest quintiles (Figure 1a) (see Supporting Table 5 in the online version of this article). It also effectively stratified individuals across a broad range of predicted readmission risk from 4.1% in the lowest decile to 36.5% in the highest decile (Table 3).
Model Name | C‐Statistic (95% CI) | IDI, % (95% CI) | NRI (95% CI) | Average Predicted Risk, % | |
---|---|---|---|---|---|
Lowest Decile | Highest Decile | ||||
| |||||
Full‐stay EHR model | |||||
Derivation cohort | 0.72 (0.70 to 0.73) | 4.1 | 36.5 | ||
Validation cohort | 0.69 (0.68 to 0.70) | [Reference] | [Reference] | 4.1 | 36.5 |
First‐day EHR model | 0.67 (0.66 to 0.68) | 1.2 (1.4 to 1.0) | 0.020 (0.038 to 0.002) | 5.8 | 31.9 |
LACE model | 0.65 (0.64 to 0.66) | 2.6 (2.9 to 2.3) | 0.046 (0.067 to 0.024) | 6.1 | 27.5 |
HOSPITAL model | 0.64 (0.62 to 0.65) | 3.2 (3.5 to 2.9) | 0.058 (0.080 to 0.035) | 6.7 | 26.6 |
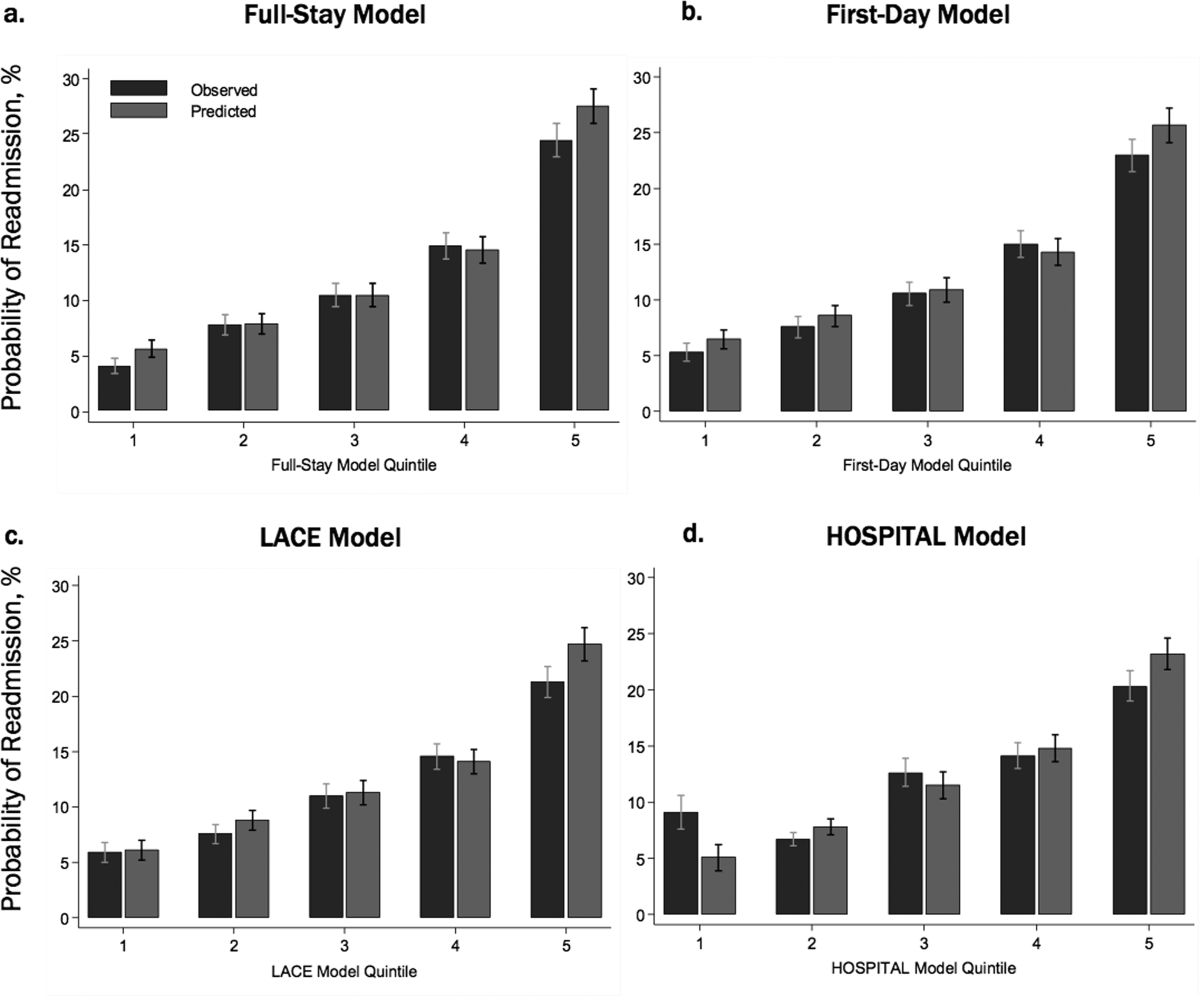
Comparing the Performance of the Full‐Stay EHR Model to Other Models
The full‐stay EHR model had better discrimination compared to the first‐day EHR model and the LACE and HOSPITAL models, though the magnitude of improvement was modest (Table 3). The full‐stay EHR model also stratified individuals across a broader range of readmission risk, and was better able to discriminate and classify those in the highest quintile of risk from those in the lowest 4 quintiles of risk compared to other models as assessed by the IDI and NRI (Table 3) (see Supporting Tables 14 and Supporting Figure 2 in the online version of this article). In terms of model calibration, both the first‐day EHR and LACE models were also well calibrated, whereas the HOSPITAL model was less robust (Figure 1).
The diagnostic accuracy of the full‐stay EHR model in correctly predicting those in the highest quintile of risk was better than that of the first‐day, LACE, and HOSPITAL models, though overall improvements in the sensitivity, specificity, positive and negative predictive values, and positive and negative likelihood ratios were also modest (see Supporting Table 6 in the online version of this article).
DISCUSSION
In this study, we used clinically detailed EHR data from the entire hospitalization on 32,922 individuals treated in 6 diverse hospitals to develop an all‐payer, multicondition readmission risk‐prediction model. To our knowledge, this is the first 30‐day hospital readmission risk‐prediction model to use a comprehensive set of factors from EHR data from the entire hospital stay. Prior EHR‐based models have focused exclusively on data available on or prior to the first day of admission, which account for clinical severity on admission but do not account for factors uncovered during the inpatient stay that influence the chance of a postdischarge adverse outcome.[15, 30] We specifically assessed the prognostic impact of a comprehensive set of factors from the entire index hospitalization, including hospital‐acquired complications, clinical trajectory, and stability on discharge in predicting hospital readmissions. Our full‐stay EHR model had statistically better discrimination, calibration, and diagnostic accuracy than our existing all‐cause first‐day EHR model[15] and 2 previously published readmissions models that included more limited information from hospitalization (such as length of stay).[9, 10] However, although the more complicated full‐stay EHR model was statistically better than previously published models, we were surprised that the predictive performance was only modestly improved despite the inclusion of many additional clinically relevant prognostic factors.
Taken together, our study has several important implications. First, the added complexity and resource intensity of implementing a full‐stay EHR model yields only modestly improved readmission risk prediction. Thus, hospitals and healthcare systems interested in targeting their highest‐risk individuals for interventions to reduce 30‐day readmission should consider doing so within the first day of hospital admission. Our group's previously derived and validated first‐day EHR model, which used data only from the first day of admission, qualitatively performed nearly as well as the full‐stay EHR model.[15] Additionally, a recent study using only preadmission EHR data to predict 30‐day readmissions also achieved similar discrimination and diagnostic accuracy as our full‐stay model.[30]
Second, the field of readmissions risk‐prediction modeling may be reaching the maximum achievable model performance using data that are currently available in the EHR. Our limited ability to accurately predict all‐cause 30‐day readmission risk may reflect the influence of currently unmeasured patient, system, and community factors on readmissions.[31, 32, 33] Due to the constraints of data collected in the EHR, we were unable to include several patient‐level clinical characteristics associated with hospital readmission, including self‐perceived health status, functional impairment, and cognition.[33, 34, 35, 36] However, given their modest effect sizes (ORs ranging from 1.062.10), adequately measuring and including these risk factors in our model may not meaningfully improve model performance and diagnostic accuracy. Further, many social and behavioral patient‐level factors are also not consistently available in EHR data. Though we explored the role of several neighborhood‐level socioeconomic measuresincluding prevalence of poverty, median income, education, and unemploymentwe found that none were significantly associated with 30‐day readmissions. These particular measures may have been inadequate to characterize individual‐level social and behavioral factors, as several previous studies have demonstrated that patient‐level factors such as social support, substance abuse, and medication and visit adherence can influence readmission risk in heart failure and pneumonia.[11, 16, 22, 25] This underscores the need for more standardized routine collection of data across functional, social, and behavioral domains in clinical settings, as recently championed by the Institute of Medicine.[11, 37] Integrating data from outside the EHR on postdischarge health behaviors, self‐management, follow‐up care, recovery, and home environment may be another important but untapped strategy for further improving prediction of readmissions.[25, 38]
Third, a multicondition readmission risk‐prediction model may be a less effective strategy than more customized disease‐specific models for selected conditions associated with high 30‐day readmission rates. Our group's previously derived and internally validated models for heart failure and human immunodeficiency virus had superior discrimination compared to our full‐stay EHR model (C statistic of 0.72 for each).[11, 13] However, given differences in the included population and time periods studied, a head‐to‐head comparison of these different strategies is needed to assess differences in model performance and utility.
Our study had several strengths. To our knowledge, this is the first study to rigorously measure the additive influence of in‐hospital complications, clinical trajectory, and stability on discharge on the risk of 30‐day hospital readmission. Additionally, our study included a large, diverse study population that included all payers, all ages of adults, a mix of community, academic, and safety net hospitals, and individuals from a broad array of racial/ethnic and socioeconomic backgrounds.
Our results should be interpreted in light of several limitations. First, though we sought to represent a diverse group of hospitals, all study sites were located within north Texas and generalizability to other regions is uncertain. Second, our ascertainment of prior hospitalizations and readmissions was more inclusive than what could be typically accomplished in real time using only EHR data from a single clinical site. We performed a sensitivity analysis using only prior utilization data available within the EHR from the index hospital with no meaningful difference in our findings (data not shown). Additionally, a recent study found that 30‐day readmissions occur at the index hospital for over 75% of events, suggesting that 30‐day readmissions are fairly comprehensively captured even with only single‐site data.[39] Third, we were not able to include data on outpatient visits before or after the index hospitalization, which may influence the risk of readmission.[1, 40]
In conclusion, incorporating clinically granular EHR data from the entire course of hospitalization modestly improves prediction of 30‐day readmissions compared to models that only include information from the first 24 hours of hospital admission or models that use far fewer variables. However, given the limited improvement in prediction, our findings suggest that from the practical perspective of implementing real‐time models to identify those at highest risk for readmission, it may not be worth the added complexity of waiting until the end of a hospitalization to leverage additional data on hospital complications, and the trajectory of laboratory and vital sign values currently available in the EHR. Further improvement in prediction of readmissions will likely require accounting for psychosocial, functional, behavioral, and postdischarge factors not currently present in the inpatient EHR.
Disclosures: This study was presented at the Society of Hospital Medicine 2015 Annual Meeting in National Harbor, Maryland, and the Society of General Internal Medicine 2015 Annual Meeting in Toronto, Canada. This work was supported by the Agency for Healthcare Research and Qualityfunded UT Southwestern Center for Patient‐Centered Outcomes Research (1R24HS022418‐01) and the Commonwealth Foundation (#20100323). Drs. Nguyen and Makam received funding from the UT Southwestern KL2 Scholars Program (NIH/NCATS KL2 TR001103). Dr. Halm was also supported in part by NIH/NCATS U54 RFA‐TR‐12‐006. The study sponsors had no role in the design and conduct of the study; collection, management, analysis, and interpretation of the data; and preparation, review, or approval of the manuscript. The authors have no conflicts of interest to disclose.
Unplanned hospital readmissions are frequent, costly, and potentially avoidable.[1, 2] Due to major federal financial readmissions penalties targeting excessive 30‐day readmissions, there is increasing attention to implementing hospital‐initiated interventions to reduce readmissions.[3, 4] However, universal enrollment of all hospitalized patients into such programs may be too resource intensive for many hospitals.[5] To optimize efficiency and effectiveness, interventions should be targeted to individuals most likely to benefit.[6, 7] However, existing readmission risk‐prediction models have achieved only modest discrimination, have largely used administrative claims data not available until months after discharge, or are limited to only a subset of patients with Medicare or a specific clinical condition.[8, 9, 10, 11, 12, 13, 14] These limitations have precluded accurate identification of high‐risk individuals in an all‐payer general medical inpatient population to provide actionable information for intervention prior to discharge.
Approaches using electronic health record (EHR) data could allow early identification of high‐risk patients during the index hospitalization to enable initiation of interventions prior to discharge. To date, such strategies have relied largely on EHR data from the day of admission.[15, 16] However, given that variation in 30‐day readmission rates are thought to reflect the quality of in‐hospital care, incorporating EHR data from the entire hospital stay to reflect hospital care processes and clinical trajectory may more accurately identify at‐risk patients.[17, 18, 19, 20] Improved accuracy in risk prediction would help better target intervention efforts in the immediate postdischarge period, an interval characterized by heightened vulnerability for adverse events.[21]
To help hospitals target transitional care interventions more effectively to high‐risk individuals prior to discharge, we derived and validated a readmissions risk‐prediction model incorporating EHR data from the entire course of the index hospitalization, which we termed the full‐stay EHR model. We also compared the full‐stay EHR model performance to our group's previously derived prediction model based on EHR data on the day of admission, termed the first‐day EHR model, as well as to 2 other validated readmission models similarly intended to yield near real‐time risk predictions prior to or shortly after hospital discharge.[9, 10, 15]
METHODS
Study Design, Population, and Data Sources
We conducted an observational cohort study using EHR data from 6 hospitals in the DallasFort Worth metroplex between November 1, 2009 and October 30, 2010 using the same EHR system (Epic Systems Corp., Verona, WI). One site was a university‐affiliated safety net hospital; the remaining 5 sites were teaching and nonteaching community sites.
We included consecutive hospitalizations among adults 18 years old discharged alive from any medicine inpatient service. For individuals with multiple hospitalizations during the study period, we included only the first hospitalization. We excluded individuals who died during the index hospitalization, were transferred to another acute care facility, left against medical advice, or who died outside of the hospital within 30 days of discharge. For model derivation, we randomly split the sample into separate derivation (50%) and validation cohorts (50%).
Outcomes
The primary outcome was 30‐day hospital readmission, defined as a nonelective hospitalization within 30 days of discharge to any of 75 acute care hospitals within a 100‐mile radius of Dallas, ascertained from an all‐payer regional hospitalization database. Nonelective hospitalizations included all hospitalizations classified as a emergency, urgent, or trauma, and excluded those classified as elective as per the Centers for Medicare and Medicaid Services Claim Inpatient Admission Type Code definitions.
Predictor Variables for the Full‐Stay EHR Model
The full‐stay EHR model was iteratively developed from our group's previously derived and validated risk‐prediction model using EHR data available on admission (first‐day EHR model).[15] For the full‐stay EHR model, we included all predictor variables included in our published first‐day EHR model as candidate risk factors. Based on prior literature, we additionally expanded candidate predictors available on admission to include marital status (proxy for social isolation) and socioeconomic disadvantage (percent poverty, unemployment, median income, and educational attainment by zip code of residence as proxy measures of the social and built environment).[22, 23, 24, 25, 26, 27] We also expanded the ascertainment of prior hospitalization to include admissions at both the index hospital and any of 75 acute care hospitals from the same, separate all‐payer regional hospitalization database used to ascertain 30‐day readmissions.
Candidate predictors from the remainder of the hospital stay (ie, following the first 24 hours of admission) were included if they were: (1) available in the EHR of all participating hospitals, (2) routinely collected or available at the time of hospital discharge, and (3) plausible predictors of adverse outcomes based on prior literature and clinical expertise. These included length of stay, in‐hospital complications, transfer to an intensive or coronary care unit, blood transfusions, vital sign instabilities within 24 hours of discharge, select laboratory values at time of discharge, and disposition status. We also assessed trajectories of vital signs and selected laboratory values (defined as changes in these measures from admission to discharge).
Statistical Analysis
Model Derivation
Univariate relationships between readmission and each of the candidate predictors were assessed in the derivation cohort using a prespecified significance threshold of P 0.05. We included all factors from our previously derived and validated first‐day EHR model as candidate predictors.[15] Continuous laboratory and vital sign values at the time of discharge were categorized based on clinically meaningful cutoffs; predictors with missing values were assumed to be normal (<1% missing for each variable). Significant univariate candidate variables were entered in a multivariate logistic regression model using stepwise backward selection with a prespecified significance threshold of P 0.05. We performed several sensitivity analyses to confirm the robustness of our model. First, we alternately derived the full‐stay model using stepwise forward selection. Second, we forced in all significant variables from our first‐day EHR model, and entered the candidate variables from the remainder of the hospital stay using both stepwise backward and forward selection separately. Third, prespecified interactions between variables were evaluated for inclusion. Though final predictors varied slightly between the different approaches, discrimination of each model was similar to the model derived using our primary analytic approach (C statistics 0.01, data not shown).
Model Validation
We assessed model discrimination and calibration of the derived full‐stay EHR model using the validation cohort. Model discrimination was estimated by the C statistic. The C statistic represents the probability that, given 2 hospitalized individuals (1 who was readmitted and the other who was not), the model will predict a higher risk for the readmitted patient than for the nonreadmitted patient. Model calibration was assessed by comparing predicted to observed probabilities of readmission by quintiles of risk, and with the Hosmer‐Lemeshow goodness‐of‐fit test.
Comparison to Existing Models
We compared the full‐stay EHR model performance to 3 previously published models: our group's first‐day EHR model, and the LACE (includes Length of stay, Acute (nonelective) admission status, Charlson Comorbidity Index, and Emergency department visits in the past year) and HOSPITAL (includes Hemoglobin at discharge, discharge from Oncology service, Sodium level at discharge, Procedure during index hospitalization, Index hospitalization Type (nonelective), number of Admissions in the past year, and Length of stay) models, which were both derived to predict 30‐day readmissions among general medical inpatients and were intended to help clinicians identify high‐risk patients to target for discharge interventions.[9, 10, 15] We assessed each model's performance in our validation cohort, calculating the C statistic, integrated discrimination index (IDI), and net reclassification index (NRI) compared to the full‐stay model. IDI is a summary measure of both discrimination and reclassification, where more positive values suggest improvement in model performance in both these domains compared to a reference model.[28] The NRI is defined as the sum of the net proportions of correctly reclassified persons with and without the event of interest.[29] The theoretical range of values is 2 to 2, with more positive values indicating improved net reclassification compared to a reference model. Here, we calculated a category‐based NRI to evaluate the performance of models in correctly classifying individuals with and without readmissions into the highest readmission risk quintile versus the lowest 4 risk quintiles compared to the full‐stay EHR model.[29] This prespecified cutoff is relevant for hospitals interested in identifying the highest‐risk individuals for targeted intervention.[6] Because some hospitals may be able to target a greater number of individuals for intervention, we performed a sensitivity analysis by assessing category‐based NRI for reclassification into the top 2 risk quintiles versus the lowest 3 risk quintiles and found no meaningful difference in our results (data not shown). Finally, we qualitatively assessed calibration of comparator models in our validation cohort by comparing predicted probability to observed probability of readmission by quintiles of risk for each model. We conducted all analyses using Stata 12.1 (StataCorp, College Station, TX). This study was approved by the UT Southwestern Medical Center institutional review board.
RESULTS
Overall, 32,922 index hospitalizations were included in our study cohort; 12.7% resulted in a 30‐day readmission (see Supporting Figure 1 in the online version of this article). Individuals had a mean age of 62 years and had diverse race/ethnicity and primary insurance status; half were female (Table 1). The study sample was randomly split into a derivation cohort (50%, n = 16,492) and validation cohort (50%, n = 16,430). Individuals in the derivation cohort with a 30‐day readmission had markedly different socioeconomic and clinical characteristics compared to those not readmitted (Table 1).
Entire Cohort, N = 32,922 | Derivation Cohort, N = 16,492 | |||
---|---|---|---|---|
No Readmission, N = 14,312 |
Readmission, N = 2,180 |
P Value | ||
| ||||
Demographic characteristics | ||||
Age, y, mean (SD) | 62 (17.3) | 61 (17.4) | 64 (17.0) | 0.001 |
Female, n (%) | 17,715 (53.8) | 7,694 (53.8) | 1,163 (53.3) | 0.72 |
Race/ethnicity | 0.001 | |||
White | 21,359 (64.9) | 9,329 (65.2) | 1,361 (62.4) | |
Black | 5,964 (18.1) | 2,520 (17.6) | 434 (19.9) | |
Hispanic | 4,452 (13.5) | 1,931 (13.5) | 338 (15.5) | |
Other | 1,147 (3.5) | 532 (3.7) | 47 (2.2) | |
Marital status, n (%) | 0.001 | |||
Single | 8,076 (24.5) | 3,516 (24.6) | 514 (23.6) | |
Married | 13,394 (40.7) | 5,950 (41.6) | 812 (37.3) | |
Separated/divorced | 3,468 (10.5) | 1,460 (10.2) | 251 (11.5) | |
Widowed | 4,487 (13.7) | 1,868 (13.1) | 388 (17.8) | |
Other | 3,497 (10.6) | 1,518 (10.6) | 215 (9.9) | |
Primary payer, n (%) | 0.001 | |||
Private | 13,090 (39.8) | 5,855 (40.9) | 726 (33.3) | |
Medicare | 13,015 (39.5) | 5,597 (39.1) | 987 (45.3) | |
Medicaid | 2,204 (6.7) | 852 (5.9) | 242 (11.1) | |
Charity, self‐pay, or other | 4,613 (14.0) | 2,008 (14.0) | 225 (10.3) | |
High‐poverty neighborhood, n (%)* | 7,468 (22.7) | 3,208 (22.4) | 548 (25.1) | 0.001 |
Utilization history | ||||
1 ED visits in past year, n (%) | 9,299 (28.2) | 3,793 (26.5) | 823 (37.8) | 0.001 |
1 hospitalizations in past year, n (%) | 10,189 (30.9) | 4,074 (28.5) | 1,012 (46.4) | 0.001 |
Clinical factors from first day of hospitalization | ||||
Nonelective admission, n (%) | 27,818 (84.5) | 11,960 (83.6) | 1,960 (89.9) | 0.001 |
Charlson Comorbidity Index, median (IQR)∥ | 0 (01) | 0 (00) | 0 (03) | 0.001 |
Laboratory abnormalities within 24 hours of admission | ||||
Albumin <2 g/dL | 355 (1.1) | 119 (0.8) | 46 (2.1) | 0.001 |
Albumin 23 g/dL | 4,732 (14.4) | 1,956 (13.7) | 458 (21.0) | 0.001 |
Aspartate aminotransferase >40 U/L | 4,610 (14.0) | 1,922 (13.4) | 383 (17.6) | 0.001 |
Creatine phosphokinase <60 g/L | 3,728 (11.3) | 1,536 (10.7) | 330 (15.1) | 0.001 |
Mean corpuscular volume >100 fL/red cell | 1,346 (4.1) | 537 (3.8) | 134 (6.2) | 0.001 |
Platelets <90 103/L | 912 (2.8) | 357 (2.5) | 116 (5.3) | 0.001 |
Platelets >350 103/L | 3,332 (10.1) | 1,433 (10.0) | 283 (13.0) | 0.001 |
Prothrombin time >35 seconds | 248 (0.8) | 90 (0.6) | 35 (1.6) | 0.001 |
Clinical factors from remainder of hospital stay | ||||
Length of stay, d, median (IQR) | 4 (26) | 4 (26) | 5 (38) | 0.001 |
ICU transfer after first 24 hours, n (%) | 988 (3.0) | 408 (2.9) | 94 (4.3) | 0.001 |
Hospital complications, n (%) | ||||
Clostridium difficile infection | 119 (0.4) | 44 (0.3) | 24 (1.1) | 0.001 |
Pressure ulcer | 358 (1.1) | 126 (0.9) | 46 (2.1) | 0.001 |
Venous thromboembolism | 301 (0.9) | 112 (0.8) | 34 (1.6) | 0.001 |
Respiratory failure | 1,048 (3.2) | 463 (3.2) | 112 (5.1) | 0.001 |
Central line‐associated bloodstream infection | 22 (0.07) | 6 (0.04) | 5 (0.23) | 0.005 |
Catheter‐associated urinary tract infection | 47 (0.14) | 20 (0.14) | 6 (0.28) | 0.15 |
Acute myocardial infarction | 293 (0.9) | 110 (0.8) | 32 (1.5) | 0.001 |
Pneumonia | 1,754 (5.3) | 719 (5.0) | 154 (7.1) | 0.001 |
Sepsis | 853 (2.6) | 368 (2.6) | 73 (3.4) | 0.04 |
Blood transfusion during hospitalization, n (%) | 4,511 (13.7) | 1,837 (12.8) | 425 (19.5) | 0.001 |
Laboratory abnormalities at discharge# | ||||
Blood urea nitrogen >20 mg/dL, n (%) | 10,014 (30.4) | 4,077 (28.5) | 929 (42.6) | 0.001 |
Sodium <135 mEq/L, n (%) | 4,583 (13.9) | 1,850 (12.9) | 440 (20.2) | 0.001 |
Hematocrit 27 | 3,104 (9.4) | 1,231 (8.6) | 287 (13.2) | 0.001 |
1 vital sign instability at discharge, n (%)# | 6,192 (18.8) | 2,624 (18.3) | 525 (24.1) | 0.001 |
Discharge location, n (%) | 0.001 | |||
Home | 23,339 (70.9) | 10,282 (71.8) | 1,383 (63.4) | |
Home health | 3,185 (9.7) | 1,356 (9.5) | 234 (10.7) | |
Postacute care** | 5,990 (18.2) | 2,496 (17.4) | 549 (25.2) | |
Hospice | 408 (1.2) | 178 (1.2) | 14 (0.6) |
Derivation and Validation of the Full‐Stay EHR Model for 30‐Day Readmission
Our final model included 24 independent variables, including demographic characteristics, utilization history, clinical factors from the first day of admission, and clinical factors from the remainder of the hospital stay (Table 2). The strongest independent predictor of readmission was hospital‐acquired Clostridium difficile infection (adjusted odds ratio [AOR]: 2.03, 95% confidence interval [CI] 1.18‐3.48); other hospital‐acquired complications including pressure ulcers and venous thromboembolism were also significant predictors. Though having Medicaid was associated with increased odds of readmission (AOR: 1.55, 95% CI: 1.31‐1.83), other zip codelevel measures of socioeconomic disadvantage were not predictive and were not included in the final model. Being discharged to hospice was associated with markedly lower odds of readmission (AOR: 0.23, 95% CI: 0.13‐0.40).
Odds Ratio (95% CI) | ||
---|---|---|
Univariate | Multivariate* | |
| ||
Demographic characteristics | ||
Age, per 10 years | 1.08 (1.051.11) | 1.07 (1.041.10) |
Medicaid | 1.97 (1.702.29) | 1.55 (1.311.83) |
Widow | 1.44 (1.281.63) | 1.27 (1.111.45) |
Utilization history | ||
Prior ED visit, per visit | 1.08 (1.061.10) | 1.04 (1.021.06) |
Prior hospitalization, per hospitalization | 1.30 (1.271.34) | 1.16 (1.121.20) |
Hospital and clinical factors from first day of hospitalization | ||
Nonelective admission | 1.75 (1.512.03) | 1.42 (1.221.65) |
Charlson Comorbidity Index, per point | 1.19 (1.171.21) | 1.06 (1.041.09) |
Laboratory abnormalities within 24 hours of admission | ||
Albumin <2 g/dL | 2.57 (1.823.62) | 1.52 (1.052.21) |
Albumin 23 g/dL | 1.68 (1.501.88) | 1.20 (1.061.36) |
Aspartate aminotransferase >40 U/L | 1.37 (1.221.55) | 1.21 (1.061.38) |
Creatine phosphokinase <60 g/L | 1.48 (1.301.69) | 1.28 (1.111.46) |
Mean corpuscular volume >100 fL/red cell | 1.68 (1.382.04) | 1.32 (1.071.62) |
Platelets <90 103/L | 2.20 (1.772.72) | 1.56 (1.231.97) |
Platelets >350 103/L | 1.34 (1.171.54) | 1.24 (1.081.44) |
Prothrombin time >35 seconds | 2.58 (1.743.82) | 1.92 (1.272.90) |
Hospital and clinical factors from remainder of hospital stay | ||
Length of stay, per day | 1.08 (1.071.09) | 1.06 (1.041.07) |
Hospital complications | ||
Clostridium difficile infection | 3.61 (2.195.95) | 2.03 (1.183.48) |
Pressure ulcer | 2.43 (1.733.41) | 1.64 (1.152.34) |
Venous thromboembolism | 2.01 (1.362.96) | 1.55 (1.032.32) |
Laboratory abnormalities at discharge | ||
Blood urea nitrogen >20 mg/dL | 1.86 (1.702.04) | 1.37 (1.241.52) |
Sodium <135 mEq/L | 1.70 (1.521.91) | 1.34 (1.181.51) |
Hematocrit 27 | 1.61 (1.401.85) | 1.22 (1.051.41) |
Vital sign instability at discharge, per instability | 1.29 (1.201.40) | 1.25 (1.151.36) |
Discharged to hospice | 0.51 (0.300.89) | 0.23 (0.130.40) |
In our validation cohort, the full‐stay EHR model had fair discrimination, with a C statistic of 0.69 (95% CI: 0.68‐0.70) (Table 3). The full‐stay EHR model was well calibrated across all quintiles of risk, with slight overestimation of predicted risk in the lowest and highest quintiles (Figure 1a) (see Supporting Table 5 in the online version of this article). It also effectively stratified individuals across a broad range of predicted readmission risk from 4.1% in the lowest decile to 36.5% in the highest decile (Table 3).
Model Name | C‐Statistic (95% CI) | IDI, % (95% CI) | NRI (95% CI) | Average Predicted Risk, % | |
---|---|---|---|---|---|
Lowest Decile | Highest Decile | ||||
| |||||
Full‐stay EHR model | |||||
Derivation cohort | 0.72 (0.70 to 0.73) | 4.1 | 36.5 | ||
Validation cohort | 0.69 (0.68 to 0.70) | [Reference] | [Reference] | 4.1 | 36.5 |
First‐day EHR model | 0.67 (0.66 to 0.68) | 1.2 (1.4 to 1.0) | 0.020 (0.038 to 0.002) | 5.8 | 31.9 |
LACE model | 0.65 (0.64 to 0.66) | 2.6 (2.9 to 2.3) | 0.046 (0.067 to 0.024) | 6.1 | 27.5 |
HOSPITAL model | 0.64 (0.62 to 0.65) | 3.2 (3.5 to 2.9) | 0.058 (0.080 to 0.035) | 6.7 | 26.6 |
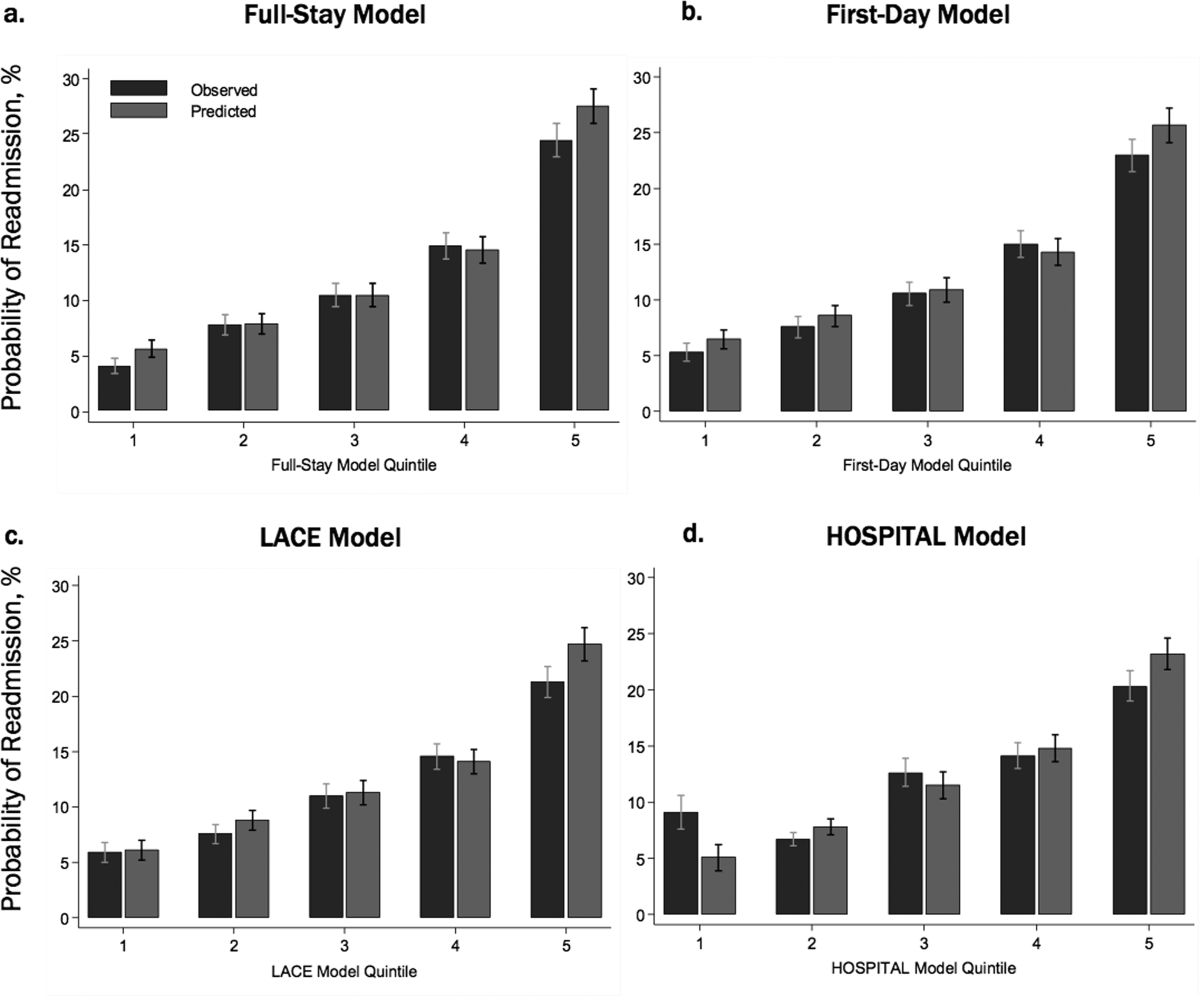
Comparing the Performance of the Full‐Stay EHR Model to Other Models
The full‐stay EHR model had better discrimination compared to the first‐day EHR model and the LACE and HOSPITAL models, though the magnitude of improvement was modest (Table 3). The full‐stay EHR model also stratified individuals across a broader range of readmission risk, and was better able to discriminate and classify those in the highest quintile of risk from those in the lowest 4 quintiles of risk compared to other models as assessed by the IDI and NRI (Table 3) (see Supporting Tables 14 and Supporting Figure 2 in the online version of this article). In terms of model calibration, both the first‐day EHR and LACE models were also well calibrated, whereas the HOSPITAL model was less robust (Figure 1).
The diagnostic accuracy of the full‐stay EHR model in correctly predicting those in the highest quintile of risk was better than that of the first‐day, LACE, and HOSPITAL models, though overall improvements in the sensitivity, specificity, positive and negative predictive values, and positive and negative likelihood ratios were also modest (see Supporting Table 6 in the online version of this article).
DISCUSSION
In this study, we used clinically detailed EHR data from the entire hospitalization on 32,922 individuals treated in 6 diverse hospitals to develop an all‐payer, multicondition readmission risk‐prediction model. To our knowledge, this is the first 30‐day hospital readmission risk‐prediction model to use a comprehensive set of factors from EHR data from the entire hospital stay. Prior EHR‐based models have focused exclusively on data available on or prior to the first day of admission, which account for clinical severity on admission but do not account for factors uncovered during the inpatient stay that influence the chance of a postdischarge adverse outcome.[15, 30] We specifically assessed the prognostic impact of a comprehensive set of factors from the entire index hospitalization, including hospital‐acquired complications, clinical trajectory, and stability on discharge in predicting hospital readmissions. Our full‐stay EHR model had statistically better discrimination, calibration, and diagnostic accuracy than our existing all‐cause first‐day EHR model[15] and 2 previously published readmissions models that included more limited information from hospitalization (such as length of stay).[9, 10] However, although the more complicated full‐stay EHR model was statistically better than previously published models, we were surprised that the predictive performance was only modestly improved despite the inclusion of many additional clinically relevant prognostic factors.
Taken together, our study has several important implications. First, the added complexity and resource intensity of implementing a full‐stay EHR model yields only modestly improved readmission risk prediction. Thus, hospitals and healthcare systems interested in targeting their highest‐risk individuals for interventions to reduce 30‐day readmission should consider doing so within the first day of hospital admission. Our group's previously derived and validated first‐day EHR model, which used data only from the first day of admission, qualitatively performed nearly as well as the full‐stay EHR model.[15] Additionally, a recent study using only preadmission EHR data to predict 30‐day readmissions also achieved similar discrimination and diagnostic accuracy as our full‐stay model.[30]
Second, the field of readmissions risk‐prediction modeling may be reaching the maximum achievable model performance using data that are currently available in the EHR. Our limited ability to accurately predict all‐cause 30‐day readmission risk may reflect the influence of currently unmeasured patient, system, and community factors on readmissions.[31, 32, 33] Due to the constraints of data collected in the EHR, we were unable to include several patient‐level clinical characteristics associated with hospital readmission, including self‐perceived health status, functional impairment, and cognition.[33, 34, 35, 36] However, given their modest effect sizes (ORs ranging from 1.062.10), adequately measuring and including these risk factors in our model may not meaningfully improve model performance and diagnostic accuracy. Further, many social and behavioral patient‐level factors are also not consistently available in EHR data. Though we explored the role of several neighborhood‐level socioeconomic measuresincluding prevalence of poverty, median income, education, and unemploymentwe found that none were significantly associated with 30‐day readmissions. These particular measures may have been inadequate to characterize individual‐level social and behavioral factors, as several previous studies have demonstrated that patient‐level factors such as social support, substance abuse, and medication and visit adherence can influence readmission risk in heart failure and pneumonia.[11, 16, 22, 25] This underscores the need for more standardized routine collection of data across functional, social, and behavioral domains in clinical settings, as recently championed by the Institute of Medicine.[11, 37] Integrating data from outside the EHR on postdischarge health behaviors, self‐management, follow‐up care, recovery, and home environment may be another important but untapped strategy for further improving prediction of readmissions.[25, 38]
Third, a multicondition readmission risk‐prediction model may be a less effective strategy than more customized disease‐specific models for selected conditions associated with high 30‐day readmission rates. Our group's previously derived and internally validated models for heart failure and human immunodeficiency virus had superior discrimination compared to our full‐stay EHR model (C statistic of 0.72 for each).[11, 13] However, given differences in the included population and time periods studied, a head‐to‐head comparison of these different strategies is needed to assess differences in model performance and utility.
Our study had several strengths. To our knowledge, this is the first study to rigorously measure the additive influence of in‐hospital complications, clinical trajectory, and stability on discharge on the risk of 30‐day hospital readmission. Additionally, our study included a large, diverse study population that included all payers, all ages of adults, a mix of community, academic, and safety net hospitals, and individuals from a broad array of racial/ethnic and socioeconomic backgrounds.
Our results should be interpreted in light of several limitations. First, though we sought to represent a diverse group of hospitals, all study sites were located within north Texas and generalizability to other regions is uncertain. Second, our ascertainment of prior hospitalizations and readmissions was more inclusive than what could be typically accomplished in real time using only EHR data from a single clinical site. We performed a sensitivity analysis using only prior utilization data available within the EHR from the index hospital with no meaningful difference in our findings (data not shown). Additionally, a recent study found that 30‐day readmissions occur at the index hospital for over 75% of events, suggesting that 30‐day readmissions are fairly comprehensively captured even with only single‐site data.[39] Third, we were not able to include data on outpatient visits before or after the index hospitalization, which may influence the risk of readmission.[1, 40]
In conclusion, incorporating clinically granular EHR data from the entire course of hospitalization modestly improves prediction of 30‐day readmissions compared to models that only include information from the first 24 hours of hospital admission or models that use far fewer variables. However, given the limited improvement in prediction, our findings suggest that from the practical perspective of implementing real‐time models to identify those at highest risk for readmission, it may not be worth the added complexity of waiting until the end of a hospitalization to leverage additional data on hospital complications, and the trajectory of laboratory and vital sign values currently available in the EHR. Further improvement in prediction of readmissions will likely require accounting for psychosocial, functional, behavioral, and postdischarge factors not currently present in the inpatient EHR.
Disclosures: This study was presented at the Society of Hospital Medicine 2015 Annual Meeting in National Harbor, Maryland, and the Society of General Internal Medicine 2015 Annual Meeting in Toronto, Canada. This work was supported by the Agency for Healthcare Research and Qualityfunded UT Southwestern Center for Patient‐Centered Outcomes Research (1R24HS022418‐01) and the Commonwealth Foundation (#20100323). Drs. Nguyen and Makam received funding from the UT Southwestern KL2 Scholars Program (NIH/NCATS KL2 TR001103). Dr. Halm was also supported in part by NIH/NCATS U54 RFA‐TR‐12‐006. The study sponsors had no role in the design and conduct of the study; collection, management, analysis, and interpretation of the data; and preparation, review, or approval of the manuscript. The authors have no conflicts of interest to disclose.
- Rehospitalizations among patients in the Medicare fee‐for‐service program. N Engl J Med. 2009;360(14):1418–1428. , , .
- Proportion of hospital readmissions deemed avoidable: a systematic review. CMAJ. 2011;183(7):E391–E402. , , , , .
- Hospital‐initiated transitional care interventions as a patient safety strategy: a systematic review. Ann Intern Med. 2013;158(5 pt 2):433–440. , , , , , .
- Interventions to reduce 30‐day rehospitalization: a systematic review. Ann Intern Med. 2011;155(8):520–528. , , , , .
- Interventions to Improve Care Transitions at Hospital Discharge. Rockville, MD: Agency for Healthcare Research and Quality; 2013. , , , , , .
- Allocating scarce resources in real‐time to reduce heart failure readmissions: a prospective, controlled study. BMJ Qual Saf. 2013;22(12):998–1005. , , , et al.
- Implementing electronic health care predictive analytics: considerations and challenges. Health Aff (Millwood). 2014;33(7):1148–1154. , , , , .
- Risk prediction models for hospital readmission: a systematic review. JAMA. 2011;306(15):1688–1698. , , , et al.
- Derivation and validation of an index to predict early death or unplanned readmission after discharge from hospital to the community. CMAJ. 2010;182(6):551–557. , , , et al.
- Potentially avoidable 30‐day hospital readmissions in medical patients: derivation and validation of a prediction model. JAMA Intern Med. 2013;173(8):632–638. , , , .
- An automated model to identify heart failure patients at risk for 30‐day readmission or death using electronic medical record data. Med Care. 2010;48(11):981–988. , , , et al.
- An automated model using electronic medical record data identifies patients with cirrhosis at high risk for readmission. Clin Gastroenterol Hepatol. 2013;11(10):1335–1341.e1331. , , , et al.
- An electronic medical record‐based model to predict 30‐day risk of readmission and death among HIV‐infected inpatients. J Acquir Immune Defic Syndr. 2012;61(3):349–358. , , , , , .
- Development and use of an administrative claims measure for profiling hospital‐wide performance on 30‐day unplanned readmission. Ann Intern Med. 2014;161(10 suppl):S66–S75. , , , et al.
- Electronic medical record‐based multicondition models to predict the risk of 30 day readmission or death among adult medicine patients: validation and comparison to existing models. BMC Med Inform Decis Mak. 2015;15(1):39. , , , et al.
- Linking electronic health record‐extracted psychosocial data in real‐time to risk of readmission for heart failure. Psychosomatics. 2011;52(4):319–327. , , , et al.
- A conceptual framework for the study of early readmission as an indicator of quality of care. Soc Sci Med. 1996;43(11):1533–1541. , .
- Hospital readmission performance and patterns of readmission: retrospective cohort study of Medicare admissions. BMJ. 2013;347:f6571. , , , et al.
- Getting more performance from performance measurement. N Engl J Med. 2014;371(23):2145–2147. , , , , , .
- Hospital strategy uptake and reductions in unplanned readmission rates for patients with heart failure: a prospective study. J Gen Intern Med. 2015;30(5):605–611. , , , et al.
- Post‐hospital syndrome—an acquired, transient condition of generalized risk. N Engl J Med. 2013;368(2):100–102. .
- Impact of social factors on risk of readmission or mortality in pneumonia and heart failure: systematic review. J Gen Intern Med. 2013;28(2):269–282. , , , et al.
- Effect of clinical and social risk factors on hospital profiling for stroke readmission: a cohort study. Ann Intern Med. 2014;161(11):775–784. , , , , , .
- Neighborhood socioeconomic disadvantage and 30‐day rehospitalization: a retrospective cohort study. Ann Intern Med. 2014;161(11):765–774. , , , et al.
- Postdischarge environmental and socioeconomic factors and the likelihood of early hospital readmission among community‐dwelling Medicare beneficiaries. Gerontologist. 2008;48(4):495–504. , , , , , .
- Socioeconomic status and readmissions: evidence from an urban teaching hospital. Health Aff (Millwood). 2014;33(5):778–785. , , .
- Adding socioeconomic data to hospital readmissions calculations may produce more useful results. Health Aff (Millwood). 2014;33(5):786–791. , , , .
- Evaluating the added predictive ability of a new marker: from area under the ROC curve to reclassification and beyond. Stat Med. 2008;27(2):157–172; discussion 207–212. , , , .
- Net reclassification improvement: computation, interpretation, and controversies: a literature review and clinician's guide. Ann Intern Med. 2014;160(2):122–131. , , , , .
- Predicting 30‐day readmissions with preadmission electronic health record data. Med Care. 2015;53(3):283–289. , , , , , .
- Hospital readmissions—not just a measure of quality. JAMA. 2011;306(16):1796–1797. , .
- Thirty‐day readmissions—truth and consequences. N Engl J Med. 2012;366(15):1366–1369. , .
- Functional impairment and hospital readmission in medicare seniors. JAMA Intern Med. 2015;175(4):559–565. , , , .
- Clinical and sociodemographic risk factors for readmission of Medicare beneficiaries. Health Care Financ Rev. 1988;10(1):27–36. , , .
- Mini‐cog performance: novel marker of post discharge risk among patients hospitalized for heart failure. Circ Heart Fail. 2015;8(1):8–16. , , , , , .
- Association of impaired functional status at hospital discharge and subsequent rehospitalization. J Hosp Med. 2014;9(5):277–282. , , , , , .
- Patients in context—EHR capture of social and behavioral determinants of health. N Engl J Med. 2015;372(8):698–701. , .
- Envisioning a social‐health information exchange as a platform to support a patient‐centered medical neighborhood: a feasibility study. J Gen Intern Med. 2015;30(1):60–67. , , , , .
- Patient factors contributing to variation in same‐hospital readmission rate. Med Care Res Review. 2015;72(3):338–358. , , , , , .
- Does increased access to primary care reduce hospital readmissions? Veterans Affairs Cooperative Study Group on Primary Care and Hospital Readmission. N Engl J Med. 1996;334(22):1441–1447. , , .
- Rehospitalizations among patients in the Medicare fee‐for‐service program. N Engl J Med. 2009;360(14):1418–1428. , , .
- Proportion of hospital readmissions deemed avoidable: a systematic review. CMAJ. 2011;183(7):E391–E402. , , , , .
- Hospital‐initiated transitional care interventions as a patient safety strategy: a systematic review. Ann Intern Med. 2013;158(5 pt 2):433–440. , , , , , .
- Interventions to reduce 30‐day rehospitalization: a systematic review. Ann Intern Med. 2011;155(8):520–528. , , , , .
- Interventions to Improve Care Transitions at Hospital Discharge. Rockville, MD: Agency for Healthcare Research and Quality; 2013. , , , , , .
- Allocating scarce resources in real‐time to reduce heart failure readmissions: a prospective, controlled study. BMJ Qual Saf. 2013;22(12):998–1005. , , , et al.
- Implementing electronic health care predictive analytics: considerations and challenges. Health Aff (Millwood). 2014;33(7):1148–1154. , , , , .
- Risk prediction models for hospital readmission: a systematic review. JAMA. 2011;306(15):1688–1698. , , , et al.
- Derivation and validation of an index to predict early death or unplanned readmission after discharge from hospital to the community. CMAJ. 2010;182(6):551–557. , , , et al.
- Potentially avoidable 30‐day hospital readmissions in medical patients: derivation and validation of a prediction model. JAMA Intern Med. 2013;173(8):632–638. , , , .
- An automated model to identify heart failure patients at risk for 30‐day readmission or death using electronic medical record data. Med Care. 2010;48(11):981–988. , , , et al.
- An automated model using electronic medical record data identifies patients with cirrhosis at high risk for readmission. Clin Gastroenterol Hepatol. 2013;11(10):1335–1341.e1331. , , , et al.
- An electronic medical record‐based model to predict 30‐day risk of readmission and death among HIV‐infected inpatients. J Acquir Immune Defic Syndr. 2012;61(3):349–358. , , , , , .
- Development and use of an administrative claims measure for profiling hospital‐wide performance on 30‐day unplanned readmission. Ann Intern Med. 2014;161(10 suppl):S66–S75. , , , et al.
- Electronic medical record‐based multicondition models to predict the risk of 30 day readmission or death among adult medicine patients: validation and comparison to existing models. BMC Med Inform Decis Mak. 2015;15(1):39. , , , et al.
- Linking electronic health record‐extracted psychosocial data in real‐time to risk of readmission for heart failure. Psychosomatics. 2011;52(4):319–327. , , , et al.
- A conceptual framework for the study of early readmission as an indicator of quality of care. Soc Sci Med. 1996;43(11):1533–1541. , .
- Hospital readmission performance and patterns of readmission: retrospective cohort study of Medicare admissions. BMJ. 2013;347:f6571. , , , et al.
- Getting more performance from performance measurement. N Engl J Med. 2014;371(23):2145–2147. , , , , , .
- Hospital strategy uptake and reductions in unplanned readmission rates for patients with heart failure: a prospective study. J Gen Intern Med. 2015;30(5):605–611. , , , et al.
- Post‐hospital syndrome—an acquired, transient condition of generalized risk. N Engl J Med. 2013;368(2):100–102. .
- Impact of social factors on risk of readmission or mortality in pneumonia and heart failure: systematic review. J Gen Intern Med. 2013;28(2):269–282. , , , et al.
- Effect of clinical and social risk factors on hospital profiling for stroke readmission: a cohort study. Ann Intern Med. 2014;161(11):775–784. , , , , , .
- Neighborhood socioeconomic disadvantage and 30‐day rehospitalization: a retrospective cohort study. Ann Intern Med. 2014;161(11):765–774. , , , et al.
- Postdischarge environmental and socioeconomic factors and the likelihood of early hospital readmission among community‐dwelling Medicare beneficiaries. Gerontologist. 2008;48(4):495–504. , , , , , .
- Socioeconomic status and readmissions: evidence from an urban teaching hospital. Health Aff (Millwood). 2014;33(5):778–785. , , .
- Adding socioeconomic data to hospital readmissions calculations may produce more useful results. Health Aff (Millwood). 2014;33(5):786–791. , , , .
- Evaluating the added predictive ability of a new marker: from area under the ROC curve to reclassification and beyond. Stat Med. 2008;27(2):157–172; discussion 207–212. , , , .
- Net reclassification improvement: computation, interpretation, and controversies: a literature review and clinician's guide. Ann Intern Med. 2014;160(2):122–131. , , , , .
- Predicting 30‐day readmissions with preadmission electronic health record data. Med Care. 2015;53(3):283–289. , , , , , .
- Hospital readmissions—not just a measure of quality. JAMA. 2011;306(16):1796–1797. , .
- Thirty‐day readmissions—truth and consequences. N Engl J Med. 2012;366(15):1366–1369. , .
- Functional impairment and hospital readmission in medicare seniors. JAMA Intern Med. 2015;175(4):559–565. , , , .
- Clinical and sociodemographic risk factors for readmission of Medicare beneficiaries. Health Care Financ Rev. 1988;10(1):27–36. , , .
- Mini‐cog performance: novel marker of post discharge risk among patients hospitalized for heart failure. Circ Heart Fail. 2015;8(1):8–16. , , , , , .
- Association of impaired functional status at hospital discharge and subsequent rehospitalization. J Hosp Med. 2014;9(5):277–282. , , , , , .
- Patients in context—EHR capture of social and behavioral determinants of health. N Engl J Med. 2015;372(8):698–701. , .
- Envisioning a social‐health information exchange as a platform to support a patient‐centered medical neighborhood: a feasibility study. J Gen Intern Med. 2015;30(1):60–67. , , , , .
- Patient factors contributing to variation in same‐hospital readmission rate. Med Care Res Review. 2015;72(3):338–358. , , , , , .
- Does increased access to primary care reduce hospital readmissions? Veterans Affairs Cooperative Study Group on Primary Care and Hospital Readmission. N Engl J Med. 1996;334(22):1441–1447. , , .