User login
In‐hospital CPR Practices
An estimated 200,000 adult patients suffer cardiac arrest in US hospitals each year, of which <20% survive to hospital discharge.[1, 2] Patient survival from in‐hospital cardiac arrest (IHCA), however, varies widely across hospitals, and may be partly attributed to differences in hospital practices.[3, 4, 5] Although there are data to support specific patient‐level practices in the hospital, such as delivery of electrical shock for ventricular fibrillation within 2 minutes of onset of the lethal rhythm,[6] little is known about in‐hospital systems‐level factors. Similar to patient‐level practices, some organizational and systems level practices are supported by international consensus and guideline recommendations.[7, 8] However, the adoption of these practices is poorly understood. As such, we sought to gain a better understanding of current US hospital practices with regard to IHCA and resuscitation with the hopes of identifying potential targets for improvement in quality and outcomes.
METHODS
We conducted a nationally representative mail survey between May 2011 and November 2011, targeting a stratified random sample of 1000 hospitals. We utilized the US Acute‐Care Hospitals (FY2008) database from the American Hospital Association to determine the total population of 3809 community hospitals (ie, nonfederal government, nonpsychiatric, and nonlong‐term care hospitals).[9] This included general medical and surgical, surgical, cancer, heart, orthopedic, and children's hospitals. These hospitals were stratified into tertiles by annual in‐patient days and teaching status (major, minor, nonteaching), from which our sample was randomly selected (Table 1). We identified each hospital's cardiopulmonary resuscitation (CPR) committee (sometimes known as code committee, code blue committee, or cardiac arrest committee) chair or chief medical/quality officer, to whom the paper‐based survey was addressed, with instructions to forward to the most appropriate person if someone other than the recipient. This study was evaluated by the University of Chicago institutional review board and deemed exempt from further review.
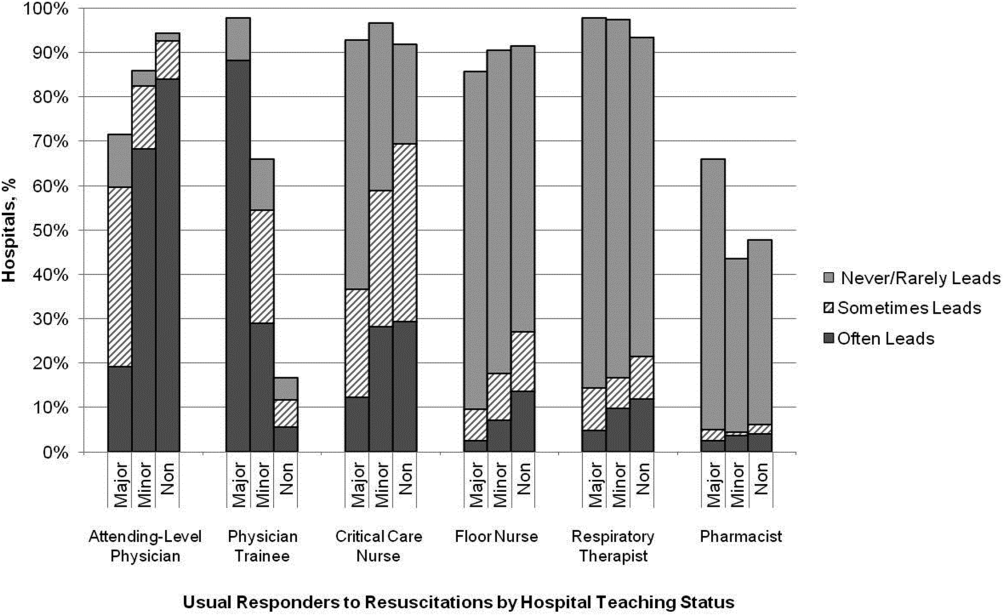
Survey
The survey content was developed by the study investigators and iteratively adapted by consensus and beta testing to require approximately 10 minutes to complete. Questions were edited and formatted by the University of Chicago Survey Lab (Chicago, IL) to be more precise and generalizable. Surveys were mailed in May 2011 and resent twice to nonresponders. A $10 incentive was included in the second mailing. When more than 1 response from a hospital was received, the more complete survey was used, or if equally complete, the responses were combined. All printing, mailing, receipt control, and data entry were performed by the University of Chicago Survey Lab, and data entry was double‐keyed to ensure accuracy.
Response rate was calculated based on the American Association for Public Opinion Research standard response rate formula.[10] It was assumed that the portion of nonresponding cases were ineligible at the same rate of cases for which eligibility was determined. A survey was considered complete if at least 75% of individual questions contained a valid response, partially complete if at least 40% but less than 75% of questions contained a valid response, and a nonresponse if less than 40% was completed. Nonresponses were excluded from the analysis.
Statistical Analysis
Analyses were performed using a statistical software application (Stata version 11.0; StataCorp, College Station, TX). Descriptive statistics were calculated and presented as number (%) or median (interquartile range). A [2] statistic was used to assess bias in response rate. We determined a priori 2 indicators of resource allocation (availability of a CPR committee and dedicated personnel for resuscitation quality improvement) and tested their association with quality improvement initiatives, using logistic regression to adjust for hospital teaching status and number of admissions as potential confounders. All tests of significance used a 2‐sided P<0.05.
RESULTS
Responses were received from 439 hospitals (425 complete and 14 partially complete), yielding a response rate of 44%. One subject ID was removed from the survey and could not be identified, so it was excluded from any analyses. Hospital demographics were similar between responders and nonresponders (P=0.50) (Table 1). Respondents who filled out the surveys included chief medical/quality officers (n=143 [33%]), chairs of CPR committees (n=64 [15%]), members of CPR committees (n=29 [7%]), chiefs of staff (n=33 [8%]), resuscitation officers/nurses (n=27 [6%]), chief nursing officers (n=13 [3%]), and others (n=131 [30%]).
Teaching Status | Annual Inpatient Days | Total | ||
---|---|---|---|---|
<17,695 | 17,695‐52,500 | >52,500 | ||
| ||||
Major | 1/2 (50) | 1/8 (13) | 40/82 (49) | 42/92 (46) |
Minor | 13/39 (33) | 40/89 (45) | 62/133 (47) | 115/261 (44) |
Nonteaching | 141/293 (48) | 100/236 (42) | 40/118 (34) | 281/647 (43) |
Total | 156/335 (47) | 143/335 (43) | 145/336 (43) | 438/1,000 (44) |
Table 2 summarizes structure, equipment, quality improvement, and pre‐ and postarrest practices across the hospitals. Of note, 77% of hospitals (n=334) reported having a predesignated, dedicated code team, and 66% (n=281) reported standardized defibrillator make and model throughout their hospital. However, less than one‐third of hospitals utilized any CPR assist technology (eg, CPR quality sensor or mechanical CPR device). The majority of hospitals reported having a rapid response team (RRT) (n=391 [91%]). Although a therapeutic hypothermia protocol for postarrest care was in place in over half of hospitals (n=252 [58%]), utilization of hypothermia for patients with return of spontaneous circulation was infrequent.
Value | 2010 AHA Guidelines | |
---|---|---|
| ||
Structure | ||
Existing CPR committee | 270 (66) | |
CPR chair | ||
Physician only | 129 (48) | |
Nurse only | 90 (34) | |
Nurse/physician co‐chair | 31 (12) | |
Other | 17 (6) | |
Clinical specialty of chaira | ||
Pulmonary/critical care | 79 (35) | |
Emergency medicine | 71 (31) | |
Anesthesia/critical care | 43 (19) | |
Cardiology | 38 (17) | |
Other | 32 (14) | |
Hospital medicine | 23 (10) | |
Predetermined cardiac arrest team structure | 334 (77) | |
Notifications of respondersa | ||
Hospital‐wide PA system | 406 (93) | |
Pager/calls to individuals | 230 (53) | |
Local alarm | 49 (11) | |
Equipment | ||
AEDs used as primary defibrillator by location | ||
High‐acuity inpatient areas | 69 (16) | |
Low‐acuity inpatient areas | 109 (26) | |
Outpatient areas | 206 (51) | Class IIb, LOE Cb |
Public areas | 263 (78) | Class IIb, LOE Cb |
Defibrillator throughout hospital | ||
Same brand and model | 281 (66) | |
Same brand, different models | 93 (22) | |
Different brands | 54 (13) | |
CPR assist technology useda | ||
None | 291 (70) | |
Capnography | 106 (25) | Class IIb, LOE Cb |
Mechanical CPR | 25 (6) | Class IIb, LOE B/Cbc |
Feedback device | 17 (4) | Class IIa, LOE B |
Quality improvement | ||
IHCA tracked | 336 (82) | Supportedbd |
Data reviewed | Supportedbd | |
Data not tracked/never reviewed | 85 (20) | |
Intermittently | 53 (12) | |
Routinely | 287 (68) | |
Routine cardiac arrest case reviews/debriefing | 149 (34) | Class IIa, LOE C |
Dedicated staff to resuscitation QI | 196 (49) | |
Full‐time equivalent staffing, median (IQR) | 0.5 (0.251.2) | |
Routine simulated resuscitation training | 268 (62) | |
Pre‐ and postarrest measures | ||
Hospitals with RRT | 391 (91) | Class I, LOE Cb |
Formal RRT‐specific training | ||
Never | 50 (14) | |
Once | 110 (30) | |
Recurrent | 163 (45) | |
TH protocol/order set in place | 252 (58) | |
Percent of patients with ROSC receiving TH | Class IIb, LOE Bb | |
<5% | 309 (74) | |
5%25% | 68 (16) | |
26%50% | 11 (3) | |
51%75% | 10 (2) | |
>75% | 18 (4) |
Hospitals reported that routine responders to IHCA events included respiratory therapists (n=414 [95%]), critical care nurses (n=406 [93%]), floor nurses (n=396 [90%]), attending physicians (n=392 [89%]), physician trainees (n=162 [37%]), and pharmacists (n=210 [48%]). Figure 1 shows the distribution of responders and team leaders by hospital type. Of the nonteaching hospitals, attending‐level physicians were likely to respond at 94% (265/281) and routinely lead the resuscitations at 84% (236/281), whereas, of major teaching hospitals, attending physicians were only likely to respond at 71% (30/42) and routinely lead at 19% (8/42).
Two‐thirds of the hospitals had a CPR committee (n=270 [66%]), and 196 (49%) had some staff time dedicated to resuscitation quality improvement. Hospitals with a specific committee dedicated to resuscitation and/or dedicated staff for resuscitation quality improvement were more likely to routinely track cardiac arrest data (odds ratio [OR]: 3.64, 95% confidence interval [CI]: 2.056.47 and OR: 2.02, 95% CI: 1.16‐3.54, respectively) and review the data (OR: 2.67, 95% CI: 1.45‐4.92 and OR: 2.18, 95% CI: 1.22‐3.89, respectively), after adjusting for teaching status and hospital size. These hospitals were also more likely to engage in simulation training and debriefing (Table 3).
CPR Committee, n=406 | Dedicated QI Staff, n=398 | |
---|---|---|
| ||
IHCA tracking | 3.64 (2.056.47) | 2.02 (1.16‐3.54) |
Routinely review | 2.67 (1.45‐4.92) | 2.18 (1.22‐3.89) |
Simulation training | 2.63 (1.66‐4.18) | 1.89 (1.24‐2.89) |
Debriefing | 3.19 (1.89‐5.36) | 2.14 (1.39‐3.32) |
Ninety percent (n=391) of respondents agreed that there is room for improvement in resuscitation practice at my hospital, and 70% (n=302) agreed that improved resuscitation would translate into improved patient outcomes. Overall, 78% (n=338) cited at least 1 barrier to improved resuscitation quality, of which the lack of adequate training (n=233 [54%]) and the lack of an appropriate champion (n=230 [53%]) were the most common. In subgroup analysis, nonteaching hospitals were significantly more likely to report the lack of a champion than their teaching counterparts (P=0.001) (Figure 2). In addition, we analyzed the data by hospitals that reported lack of a champion was not a barrier and compared them to those for whom it was, and found significantly higher adherence across all the measures in Table 2 supported by the 2010 guidelines, with the exception of real‐time feedback (data not shown).
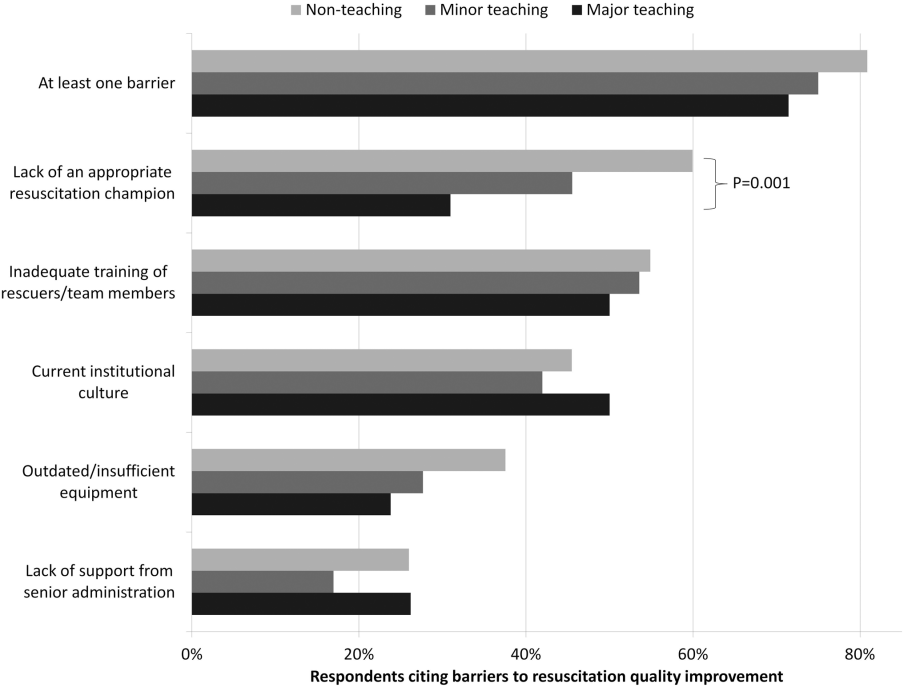
DISCUSSION
In this nationally representative sample of hospitals, we found considerable variability in cardiac arrest and resuscitation structures and processes, suggesting potential areas to target for improvement. Some practices, including use of RRTs and defibrillator standardization, were fairly routine, whereas others, such as therapeutic hypothermia and CPR assist technology, were rarely utilized. Quality initiatives, such as data tracking and review, simulation training, and debriefing were variable.
Several factors likely contribute to the variable implementation of evidence‐based practices. Guidelines alone have been shown to have little impact on practice by physicians in general.[11] This is supported by the lack of correlation we found between the presence, absence or strength of specific American Heart Association (AHA) emergency cardiovascular care treatment recommendations and the percent of hospitals reporting performing that measure. It is possible that other factors, such as a lack of familiarity or agreement with those guidelines, or the presence of external barriers, may be contributing.[12, 13] Specifically, the importance of a clinical champion was supported by our finding that hospitals reporting lack of a champion as a barrier were less likely to be adherent with guidelines. However, because the study did not directly test the impact of a champion, we wanted to be careful to avoid overstating or editorializing our results.
Some of the variability may also be related to the resource intensiveness of the practice. Routine simulation training and debriefing interventions, for example, are time intensive and require trained personnel to institute. That may explain the correlation we noted between these practices and the presence of CPR committee and dedicated personnel. The use of dedicated personnel was rare in this study, with less than half of respondents reporting any dedicated staff and a median of 0.5 full‐time equivalents for those reporting positively. This is in stark contrast to the routine use of resuscitation officers (primarily nurses dedicated to overseeing resuscitation practices and education at the hospital) in the United Kingdom.[14] Such a resuscitation officer model adopted by US hospitals could improve the quality and intensity of resuscitation care approaches.
Particularly surprising was the high rate of respondents (70%) reporting that they do not utilize any CPR assist technology. In the patient who does not have an arterial line, use of quantitative capnography is the best measure of cardiac output during cardiac arrest, yet only one‐quarter of hospitals reported using it, with no discrepancy between hospital type or size. A recent summit of national resuscitation experts expounded on the AHA guidelines suggesting that end‐tidal carbon dioxide should be used in all arrests to guide the quality of CPR with a goal value of >20.[8] Similarly, CPR feedback devices have an even higher level of evidence recommendation in the 2010 AHA guidelines than capnography, yet only 4% of hospitals reported utilizing them. Although it is true that introducing these CPR assist technologies into a hospital would require some effort on the part of hospital leadership, it is important to recognize the potential role such devices might play in the larger context of a resuscitation quality program to optimize clinical outcomes from IHCA.
Several differences were noted between hospitals based on teaching status. Although all hospitals were more likely to rely on physicians to lead resuscitations, nonteaching hospitals were more likely to report routine leadership by nurses and pharmacists. Nonteaching hospitals were also less likely to have a CPR committee, even after adjusting for hospital size. In addition, these hospitals were also more likely to report the lack of a clinical champion as a barrier to quality improvement.
There were several limitations to this study. First, this was a descriptive survey that was not tied to outcomes. As such, we are unable to draw conclusions about which practices correlate with decreased incidence of cardiac arrest and improved survival. Second, this was an optional survey with a somewhat limited response rate. Even though the characteristics of the nonresponding hospitals were similar to the responding hospitals, we cannot rule out the possibility that a selection bias was introduced, which would likely overestimate adherence to the guidelines. Self‐reported responses may have introduced additional errors. Finally, the short interval between the release of the 2010 guidelines and the administration of the first survey may have contributed to the variability in implementation of some practices, but many of the recommendations had been previously included in the 2005 guidelines.
We conclude that there is wide variability between hospitals and within practices for resuscitation care. Future work should seek to understand which practices are associated with improved patient outcomes and how best to implement these practices in a more uniform fashion.
Acknowledgements
The authors thank Nancy Hinckley, who championed the study; David Chearo, Christelle Marpaud, and Martha Van Haitsma of the University of Chicago Survey Lab for their assistance in formulating and distributing the survey; and JoAnne Resnic, Nicole Twu, and Frank Zadravecz for administrative support.
Disclosures: This study was supported by the Society of Hospital Medicine with a grant from Philips Healthcare (Andover, MA). Dr. Edelson is supported by a career development award from the National Heart, Lung, and Blood Institute (K23 HL097157). In addition, she has received research support and honoraria from Philips Healthcare (Andover, MA), research support from the American Heart Association (Dallas, TX) and Laerdal Medical (Stavanger, Norway), and an honorarium from Early Sense (Tel Aviv, Israel). Dr. Hunt has received research support from the Laerdal Foundation for Acute Medicine (Stavanger, Norway), the Hartwell Foundation (Memphis, TN), and the Arthur Vining Davis Foundation (Jacksonville, FL), and honoraria from the Kansas University Endowment (Kansas City, KS), JCCC (Overland Park, KS), and the UVA School of Medicine (Charlottesville, VA) and the European School of Management (Berlin, Germany). Dr. Mancini is supported in part by an Agency for Healthcare Research and Quality grant (R18HS020416). In addition, she has received research support from the American Heart Association (Dallas, TX) and Laerdal Medical (Stavanger, Norway), and honoraria from Sotera Wireless, Inc. (San Diego, CA). Dr. Abella has received research support from the National Institutes of Health (NIH), Medtronic Foundation (Minneapolis, MN), and Philips Healthcare (Andover, MA); has volunteered with the American Heart Association; and received honoraria from Heartsine (Belfast, Ireland), Velomedix (Menlo Park, CA), and Stryker (Kalamazoo, MI). Mr. Miller is employed by the Society of Hospital Medicine.
- Trends in survival after in‐hospital cardiac arrest. N Engl J Med. 2012;367(20):1912–1920. , , , , , .
- Incidence of treated cardiac arrest in hospitalized patients in the United States. Crit Care Med. 2011;39(11):2401–2406. , , , et al.
- Racial differences in survival after in‐hospital cardiac arrest. JAMA. 2009;302(11):1195–1201. , , , et al.
- Hospital variation in time to defibrillation after in‐hospital cardiac arrest. Arch Intern Med. 2009;169(14):1265–1273. , , , , .
- Duration of resuscitation efforts and survival after in‐hospital cardiac arrest: an observational study. Lancet. 2012;380(9852):1473–1481. , , , et al.
- Delayed time to defibrillation after in‐hospital cardiac arrest. N Engl J Med. 2008;358(1):9–17. , , , .
- 2010 American Heart Association guidelines for cardiopulmonary resuscitation and emergency cardiovascular care science. Circulation. 2010;122(18 suppl 3):S640–S946.
- Cardiopulmonary resuscitation quality: improving cardiac resuscitation outcomes both inside and outside the hospital: a consensus statement from the American Heart Association. Circulation. 2013;128(4):417–435. , , , et al.
- American Hospital Association. 2008 AHA annual survey. AHA data viewer: survey instruments. 2012; Available at: http://www.ahadataviewer.com/about/hospital‐database. Accessed October 11, 2013.
- The American Association for Public Opinion Research. Standard Definitions: Final Dispositions of Case Codes and Outcome Rates for Surveys. 7th ed. Deerfield, IL: AAPOR; 2011.
- Do practice guidelines guide practice? The effect of a consensus statement on the practice of physicians. N Engl J Med. 1989;321(19):1306–1311. , , , , , .
- Why don't physicians follow clinical practice guidelines? A framework for improvement. JAMA. 1999;282(15):1458–1465. , , , et al.
- From best evidence to best practice: effective implementation of change in patients' care. Lancet. 2003;362(9391):1225–1230. , .
- Cardiopulmonary resuscitation standards for clinical practice and training in the UK. Accid Emerg Nurs. 2005;13(3):171–179. , , , et al.
An estimated 200,000 adult patients suffer cardiac arrest in US hospitals each year, of which <20% survive to hospital discharge.[1, 2] Patient survival from in‐hospital cardiac arrest (IHCA), however, varies widely across hospitals, and may be partly attributed to differences in hospital practices.[3, 4, 5] Although there are data to support specific patient‐level practices in the hospital, such as delivery of electrical shock for ventricular fibrillation within 2 minutes of onset of the lethal rhythm,[6] little is known about in‐hospital systems‐level factors. Similar to patient‐level practices, some organizational and systems level practices are supported by international consensus and guideline recommendations.[7, 8] However, the adoption of these practices is poorly understood. As such, we sought to gain a better understanding of current US hospital practices with regard to IHCA and resuscitation with the hopes of identifying potential targets for improvement in quality and outcomes.
METHODS
We conducted a nationally representative mail survey between May 2011 and November 2011, targeting a stratified random sample of 1000 hospitals. We utilized the US Acute‐Care Hospitals (FY2008) database from the American Hospital Association to determine the total population of 3809 community hospitals (ie, nonfederal government, nonpsychiatric, and nonlong‐term care hospitals).[9] This included general medical and surgical, surgical, cancer, heart, orthopedic, and children's hospitals. These hospitals were stratified into tertiles by annual in‐patient days and teaching status (major, minor, nonteaching), from which our sample was randomly selected (Table 1). We identified each hospital's cardiopulmonary resuscitation (CPR) committee (sometimes known as code committee, code blue committee, or cardiac arrest committee) chair or chief medical/quality officer, to whom the paper‐based survey was addressed, with instructions to forward to the most appropriate person if someone other than the recipient. This study was evaluated by the University of Chicago institutional review board and deemed exempt from further review.
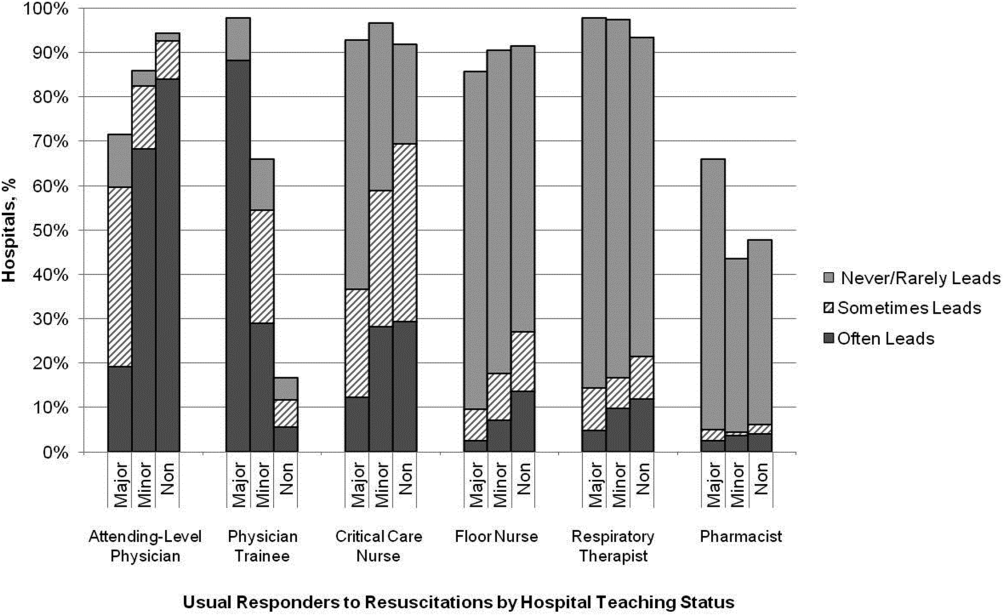
Survey
The survey content was developed by the study investigators and iteratively adapted by consensus and beta testing to require approximately 10 minutes to complete. Questions were edited and formatted by the University of Chicago Survey Lab (Chicago, IL) to be more precise and generalizable. Surveys were mailed in May 2011 and resent twice to nonresponders. A $10 incentive was included in the second mailing. When more than 1 response from a hospital was received, the more complete survey was used, or if equally complete, the responses were combined. All printing, mailing, receipt control, and data entry were performed by the University of Chicago Survey Lab, and data entry was double‐keyed to ensure accuracy.
Response rate was calculated based on the American Association for Public Opinion Research standard response rate formula.[10] It was assumed that the portion of nonresponding cases were ineligible at the same rate of cases for which eligibility was determined. A survey was considered complete if at least 75% of individual questions contained a valid response, partially complete if at least 40% but less than 75% of questions contained a valid response, and a nonresponse if less than 40% was completed. Nonresponses were excluded from the analysis.
Statistical Analysis
Analyses were performed using a statistical software application (Stata version 11.0; StataCorp, College Station, TX). Descriptive statistics were calculated and presented as number (%) or median (interquartile range). A [2] statistic was used to assess bias in response rate. We determined a priori 2 indicators of resource allocation (availability of a CPR committee and dedicated personnel for resuscitation quality improvement) and tested their association with quality improvement initiatives, using logistic regression to adjust for hospital teaching status and number of admissions as potential confounders. All tests of significance used a 2‐sided P<0.05.
RESULTS
Responses were received from 439 hospitals (425 complete and 14 partially complete), yielding a response rate of 44%. One subject ID was removed from the survey and could not be identified, so it was excluded from any analyses. Hospital demographics were similar between responders and nonresponders (P=0.50) (Table 1). Respondents who filled out the surveys included chief medical/quality officers (n=143 [33%]), chairs of CPR committees (n=64 [15%]), members of CPR committees (n=29 [7%]), chiefs of staff (n=33 [8%]), resuscitation officers/nurses (n=27 [6%]), chief nursing officers (n=13 [3%]), and others (n=131 [30%]).
Teaching Status | Annual Inpatient Days | Total | ||
---|---|---|---|---|
<17,695 | 17,695‐52,500 | >52,500 | ||
| ||||
Major | 1/2 (50) | 1/8 (13) | 40/82 (49) | 42/92 (46) |
Minor | 13/39 (33) | 40/89 (45) | 62/133 (47) | 115/261 (44) |
Nonteaching | 141/293 (48) | 100/236 (42) | 40/118 (34) | 281/647 (43) |
Total | 156/335 (47) | 143/335 (43) | 145/336 (43) | 438/1,000 (44) |
Table 2 summarizes structure, equipment, quality improvement, and pre‐ and postarrest practices across the hospitals. Of note, 77% of hospitals (n=334) reported having a predesignated, dedicated code team, and 66% (n=281) reported standardized defibrillator make and model throughout their hospital. However, less than one‐third of hospitals utilized any CPR assist technology (eg, CPR quality sensor or mechanical CPR device). The majority of hospitals reported having a rapid response team (RRT) (n=391 [91%]). Although a therapeutic hypothermia protocol for postarrest care was in place in over half of hospitals (n=252 [58%]), utilization of hypothermia for patients with return of spontaneous circulation was infrequent.
Value | 2010 AHA Guidelines | |
---|---|---|
| ||
Structure | ||
Existing CPR committee | 270 (66) | |
CPR chair | ||
Physician only | 129 (48) | |
Nurse only | 90 (34) | |
Nurse/physician co‐chair | 31 (12) | |
Other | 17 (6) | |
Clinical specialty of chaira | ||
Pulmonary/critical care | 79 (35) | |
Emergency medicine | 71 (31) | |
Anesthesia/critical care | 43 (19) | |
Cardiology | 38 (17) | |
Other | 32 (14) | |
Hospital medicine | 23 (10) | |
Predetermined cardiac arrest team structure | 334 (77) | |
Notifications of respondersa | ||
Hospital‐wide PA system | 406 (93) | |
Pager/calls to individuals | 230 (53) | |
Local alarm | 49 (11) | |
Equipment | ||
AEDs used as primary defibrillator by location | ||
High‐acuity inpatient areas | 69 (16) | |
Low‐acuity inpatient areas | 109 (26) | |
Outpatient areas | 206 (51) | Class IIb, LOE Cb |
Public areas | 263 (78) | Class IIb, LOE Cb |
Defibrillator throughout hospital | ||
Same brand and model | 281 (66) | |
Same brand, different models | 93 (22) | |
Different brands | 54 (13) | |
CPR assist technology useda | ||
None | 291 (70) | |
Capnography | 106 (25) | Class IIb, LOE Cb |
Mechanical CPR | 25 (6) | Class IIb, LOE B/Cbc |
Feedback device | 17 (4) | Class IIa, LOE B |
Quality improvement | ||
IHCA tracked | 336 (82) | Supportedbd |
Data reviewed | Supportedbd | |
Data not tracked/never reviewed | 85 (20) | |
Intermittently | 53 (12) | |
Routinely | 287 (68) | |
Routine cardiac arrest case reviews/debriefing | 149 (34) | Class IIa, LOE C |
Dedicated staff to resuscitation QI | 196 (49) | |
Full‐time equivalent staffing, median (IQR) | 0.5 (0.251.2) | |
Routine simulated resuscitation training | 268 (62) | |
Pre‐ and postarrest measures | ||
Hospitals with RRT | 391 (91) | Class I, LOE Cb |
Formal RRT‐specific training | ||
Never | 50 (14) | |
Once | 110 (30) | |
Recurrent | 163 (45) | |
TH protocol/order set in place | 252 (58) | |
Percent of patients with ROSC receiving TH | Class IIb, LOE Bb | |
<5% | 309 (74) | |
5%25% | 68 (16) | |
26%50% | 11 (3) | |
51%75% | 10 (2) | |
>75% | 18 (4) |
Hospitals reported that routine responders to IHCA events included respiratory therapists (n=414 [95%]), critical care nurses (n=406 [93%]), floor nurses (n=396 [90%]), attending physicians (n=392 [89%]), physician trainees (n=162 [37%]), and pharmacists (n=210 [48%]). Figure 1 shows the distribution of responders and team leaders by hospital type. Of the nonteaching hospitals, attending‐level physicians were likely to respond at 94% (265/281) and routinely lead the resuscitations at 84% (236/281), whereas, of major teaching hospitals, attending physicians were only likely to respond at 71% (30/42) and routinely lead at 19% (8/42).
Two‐thirds of the hospitals had a CPR committee (n=270 [66%]), and 196 (49%) had some staff time dedicated to resuscitation quality improvement. Hospitals with a specific committee dedicated to resuscitation and/or dedicated staff for resuscitation quality improvement were more likely to routinely track cardiac arrest data (odds ratio [OR]: 3.64, 95% confidence interval [CI]: 2.056.47 and OR: 2.02, 95% CI: 1.16‐3.54, respectively) and review the data (OR: 2.67, 95% CI: 1.45‐4.92 and OR: 2.18, 95% CI: 1.22‐3.89, respectively), after adjusting for teaching status and hospital size. These hospitals were also more likely to engage in simulation training and debriefing (Table 3).
CPR Committee, n=406 | Dedicated QI Staff, n=398 | |
---|---|---|
| ||
IHCA tracking | 3.64 (2.056.47) | 2.02 (1.16‐3.54) |
Routinely review | 2.67 (1.45‐4.92) | 2.18 (1.22‐3.89) |
Simulation training | 2.63 (1.66‐4.18) | 1.89 (1.24‐2.89) |
Debriefing | 3.19 (1.89‐5.36) | 2.14 (1.39‐3.32) |
Ninety percent (n=391) of respondents agreed that there is room for improvement in resuscitation practice at my hospital, and 70% (n=302) agreed that improved resuscitation would translate into improved patient outcomes. Overall, 78% (n=338) cited at least 1 barrier to improved resuscitation quality, of which the lack of adequate training (n=233 [54%]) and the lack of an appropriate champion (n=230 [53%]) were the most common. In subgroup analysis, nonteaching hospitals were significantly more likely to report the lack of a champion than their teaching counterparts (P=0.001) (Figure 2). In addition, we analyzed the data by hospitals that reported lack of a champion was not a barrier and compared them to those for whom it was, and found significantly higher adherence across all the measures in Table 2 supported by the 2010 guidelines, with the exception of real‐time feedback (data not shown).
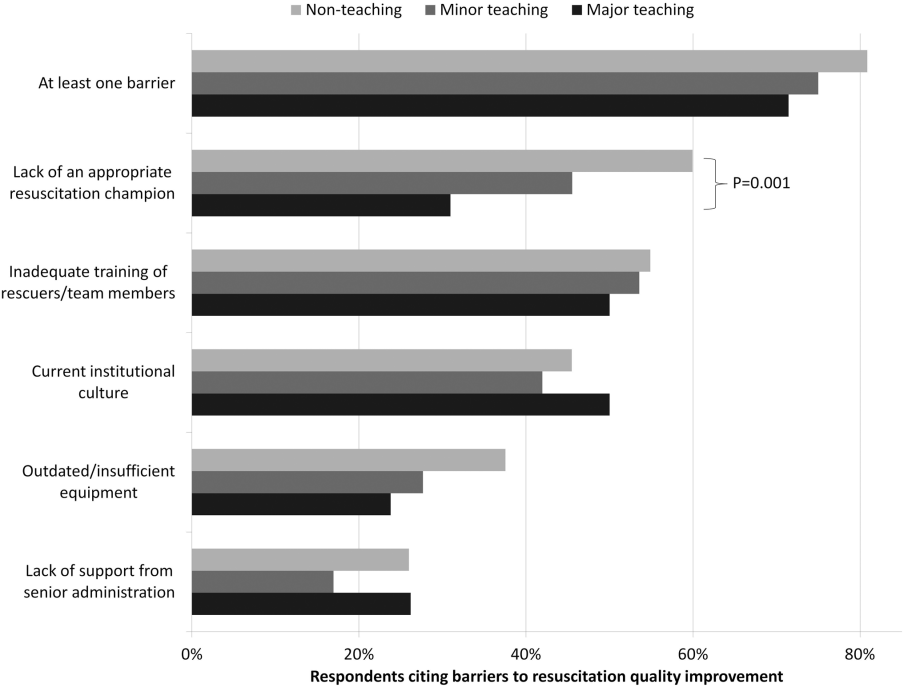
DISCUSSION
In this nationally representative sample of hospitals, we found considerable variability in cardiac arrest and resuscitation structures and processes, suggesting potential areas to target for improvement. Some practices, including use of RRTs and defibrillator standardization, were fairly routine, whereas others, such as therapeutic hypothermia and CPR assist technology, were rarely utilized. Quality initiatives, such as data tracking and review, simulation training, and debriefing were variable.
Several factors likely contribute to the variable implementation of evidence‐based practices. Guidelines alone have been shown to have little impact on practice by physicians in general.[11] This is supported by the lack of correlation we found between the presence, absence or strength of specific American Heart Association (AHA) emergency cardiovascular care treatment recommendations and the percent of hospitals reporting performing that measure. It is possible that other factors, such as a lack of familiarity or agreement with those guidelines, or the presence of external barriers, may be contributing.[12, 13] Specifically, the importance of a clinical champion was supported by our finding that hospitals reporting lack of a champion as a barrier were less likely to be adherent with guidelines. However, because the study did not directly test the impact of a champion, we wanted to be careful to avoid overstating or editorializing our results.
Some of the variability may also be related to the resource intensiveness of the practice. Routine simulation training and debriefing interventions, for example, are time intensive and require trained personnel to institute. That may explain the correlation we noted between these practices and the presence of CPR committee and dedicated personnel. The use of dedicated personnel was rare in this study, with less than half of respondents reporting any dedicated staff and a median of 0.5 full‐time equivalents for those reporting positively. This is in stark contrast to the routine use of resuscitation officers (primarily nurses dedicated to overseeing resuscitation practices and education at the hospital) in the United Kingdom.[14] Such a resuscitation officer model adopted by US hospitals could improve the quality and intensity of resuscitation care approaches.
Particularly surprising was the high rate of respondents (70%) reporting that they do not utilize any CPR assist technology. In the patient who does not have an arterial line, use of quantitative capnography is the best measure of cardiac output during cardiac arrest, yet only one‐quarter of hospitals reported using it, with no discrepancy between hospital type or size. A recent summit of national resuscitation experts expounded on the AHA guidelines suggesting that end‐tidal carbon dioxide should be used in all arrests to guide the quality of CPR with a goal value of >20.[8] Similarly, CPR feedback devices have an even higher level of evidence recommendation in the 2010 AHA guidelines than capnography, yet only 4% of hospitals reported utilizing them. Although it is true that introducing these CPR assist technologies into a hospital would require some effort on the part of hospital leadership, it is important to recognize the potential role such devices might play in the larger context of a resuscitation quality program to optimize clinical outcomes from IHCA.
Several differences were noted between hospitals based on teaching status. Although all hospitals were more likely to rely on physicians to lead resuscitations, nonteaching hospitals were more likely to report routine leadership by nurses and pharmacists. Nonteaching hospitals were also less likely to have a CPR committee, even after adjusting for hospital size. In addition, these hospitals were also more likely to report the lack of a clinical champion as a barrier to quality improvement.
There were several limitations to this study. First, this was a descriptive survey that was not tied to outcomes. As such, we are unable to draw conclusions about which practices correlate with decreased incidence of cardiac arrest and improved survival. Second, this was an optional survey with a somewhat limited response rate. Even though the characteristics of the nonresponding hospitals were similar to the responding hospitals, we cannot rule out the possibility that a selection bias was introduced, which would likely overestimate adherence to the guidelines. Self‐reported responses may have introduced additional errors. Finally, the short interval between the release of the 2010 guidelines and the administration of the first survey may have contributed to the variability in implementation of some practices, but many of the recommendations had been previously included in the 2005 guidelines.
We conclude that there is wide variability between hospitals and within practices for resuscitation care. Future work should seek to understand which practices are associated with improved patient outcomes and how best to implement these practices in a more uniform fashion.
Acknowledgements
The authors thank Nancy Hinckley, who championed the study; David Chearo, Christelle Marpaud, and Martha Van Haitsma of the University of Chicago Survey Lab for their assistance in formulating and distributing the survey; and JoAnne Resnic, Nicole Twu, and Frank Zadravecz for administrative support.
Disclosures: This study was supported by the Society of Hospital Medicine with a grant from Philips Healthcare (Andover, MA). Dr. Edelson is supported by a career development award from the National Heart, Lung, and Blood Institute (K23 HL097157). In addition, she has received research support and honoraria from Philips Healthcare (Andover, MA), research support from the American Heart Association (Dallas, TX) and Laerdal Medical (Stavanger, Norway), and an honorarium from Early Sense (Tel Aviv, Israel). Dr. Hunt has received research support from the Laerdal Foundation for Acute Medicine (Stavanger, Norway), the Hartwell Foundation (Memphis, TN), and the Arthur Vining Davis Foundation (Jacksonville, FL), and honoraria from the Kansas University Endowment (Kansas City, KS), JCCC (Overland Park, KS), and the UVA School of Medicine (Charlottesville, VA) and the European School of Management (Berlin, Germany). Dr. Mancini is supported in part by an Agency for Healthcare Research and Quality grant (R18HS020416). In addition, she has received research support from the American Heart Association (Dallas, TX) and Laerdal Medical (Stavanger, Norway), and honoraria from Sotera Wireless, Inc. (San Diego, CA). Dr. Abella has received research support from the National Institutes of Health (NIH), Medtronic Foundation (Minneapolis, MN), and Philips Healthcare (Andover, MA); has volunteered with the American Heart Association; and received honoraria from Heartsine (Belfast, Ireland), Velomedix (Menlo Park, CA), and Stryker (Kalamazoo, MI). Mr. Miller is employed by the Society of Hospital Medicine.
An estimated 200,000 adult patients suffer cardiac arrest in US hospitals each year, of which <20% survive to hospital discharge.[1, 2] Patient survival from in‐hospital cardiac arrest (IHCA), however, varies widely across hospitals, and may be partly attributed to differences in hospital practices.[3, 4, 5] Although there are data to support specific patient‐level practices in the hospital, such as delivery of electrical shock for ventricular fibrillation within 2 minutes of onset of the lethal rhythm,[6] little is known about in‐hospital systems‐level factors. Similar to patient‐level practices, some organizational and systems level practices are supported by international consensus and guideline recommendations.[7, 8] However, the adoption of these practices is poorly understood. As such, we sought to gain a better understanding of current US hospital practices with regard to IHCA and resuscitation with the hopes of identifying potential targets for improvement in quality and outcomes.
METHODS
We conducted a nationally representative mail survey between May 2011 and November 2011, targeting a stratified random sample of 1000 hospitals. We utilized the US Acute‐Care Hospitals (FY2008) database from the American Hospital Association to determine the total population of 3809 community hospitals (ie, nonfederal government, nonpsychiatric, and nonlong‐term care hospitals).[9] This included general medical and surgical, surgical, cancer, heart, orthopedic, and children's hospitals. These hospitals were stratified into tertiles by annual in‐patient days and teaching status (major, minor, nonteaching), from which our sample was randomly selected (Table 1). We identified each hospital's cardiopulmonary resuscitation (CPR) committee (sometimes known as code committee, code blue committee, or cardiac arrest committee) chair or chief medical/quality officer, to whom the paper‐based survey was addressed, with instructions to forward to the most appropriate person if someone other than the recipient. This study was evaluated by the University of Chicago institutional review board and deemed exempt from further review.
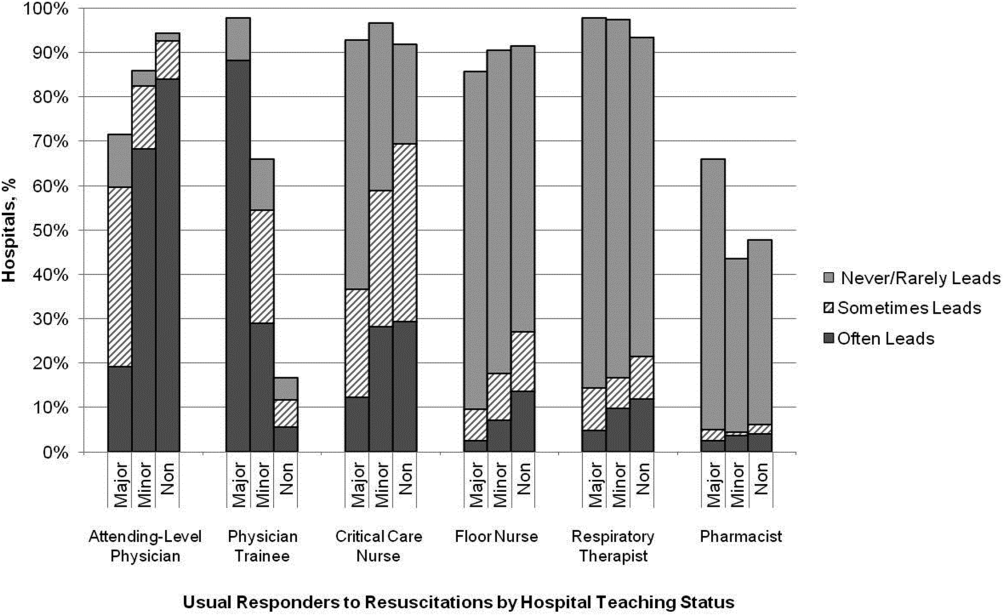
Survey
The survey content was developed by the study investigators and iteratively adapted by consensus and beta testing to require approximately 10 minutes to complete. Questions were edited and formatted by the University of Chicago Survey Lab (Chicago, IL) to be more precise and generalizable. Surveys were mailed in May 2011 and resent twice to nonresponders. A $10 incentive was included in the second mailing. When more than 1 response from a hospital was received, the more complete survey was used, or if equally complete, the responses were combined. All printing, mailing, receipt control, and data entry were performed by the University of Chicago Survey Lab, and data entry was double‐keyed to ensure accuracy.
Response rate was calculated based on the American Association for Public Opinion Research standard response rate formula.[10] It was assumed that the portion of nonresponding cases were ineligible at the same rate of cases for which eligibility was determined. A survey was considered complete if at least 75% of individual questions contained a valid response, partially complete if at least 40% but less than 75% of questions contained a valid response, and a nonresponse if less than 40% was completed. Nonresponses were excluded from the analysis.
Statistical Analysis
Analyses were performed using a statistical software application (Stata version 11.0; StataCorp, College Station, TX). Descriptive statistics were calculated and presented as number (%) or median (interquartile range). A [2] statistic was used to assess bias in response rate. We determined a priori 2 indicators of resource allocation (availability of a CPR committee and dedicated personnel for resuscitation quality improvement) and tested their association with quality improvement initiatives, using logistic regression to adjust for hospital teaching status and number of admissions as potential confounders. All tests of significance used a 2‐sided P<0.05.
RESULTS
Responses were received from 439 hospitals (425 complete and 14 partially complete), yielding a response rate of 44%. One subject ID was removed from the survey and could not be identified, so it was excluded from any analyses. Hospital demographics were similar between responders and nonresponders (P=0.50) (Table 1). Respondents who filled out the surveys included chief medical/quality officers (n=143 [33%]), chairs of CPR committees (n=64 [15%]), members of CPR committees (n=29 [7%]), chiefs of staff (n=33 [8%]), resuscitation officers/nurses (n=27 [6%]), chief nursing officers (n=13 [3%]), and others (n=131 [30%]).
Teaching Status | Annual Inpatient Days | Total | ||
---|---|---|---|---|
<17,695 | 17,695‐52,500 | >52,500 | ||
| ||||
Major | 1/2 (50) | 1/8 (13) | 40/82 (49) | 42/92 (46) |
Minor | 13/39 (33) | 40/89 (45) | 62/133 (47) | 115/261 (44) |
Nonteaching | 141/293 (48) | 100/236 (42) | 40/118 (34) | 281/647 (43) |
Total | 156/335 (47) | 143/335 (43) | 145/336 (43) | 438/1,000 (44) |
Table 2 summarizes structure, equipment, quality improvement, and pre‐ and postarrest practices across the hospitals. Of note, 77% of hospitals (n=334) reported having a predesignated, dedicated code team, and 66% (n=281) reported standardized defibrillator make and model throughout their hospital. However, less than one‐third of hospitals utilized any CPR assist technology (eg, CPR quality sensor or mechanical CPR device). The majority of hospitals reported having a rapid response team (RRT) (n=391 [91%]). Although a therapeutic hypothermia protocol for postarrest care was in place in over half of hospitals (n=252 [58%]), utilization of hypothermia for patients with return of spontaneous circulation was infrequent.
Value | 2010 AHA Guidelines | |
---|---|---|
| ||
Structure | ||
Existing CPR committee | 270 (66) | |
CPR chair | ||
Physician only | 129 (48) | |
Nurse only | 90 (34) | |
Nurse/physician co‐chair | 31 (12) | |
Other | 17 (6) | |
Clinical specialty of chaira | ||
Pulmonary/critical care | 79 (35) | |
Emergency medicine | 71 (31) | |
Anesthesia/critical care | 43 (19) | |
Cardiology | 38 (17) | |
Other | 32 (14) | |
Hospital medicine | 23 (10) | |
Predetermined cardiac arrest team structure | 334 (77) | |
Notifications of respondersa | ||
Hospital‐wide PA system | 406 (93) | |
Pager/calls to individuals | 230 (53) | |
Local alarm | 49 (11) | |
Equipment | ||
AEDs used as primary defibrillator by location | ||
High‐acuity inpatient areas | 69 (16) | |
Low‐acuity inpatient areas | 109 (26) | |
Outpatient areas | 206 (51) | Class IIb, LOE Cb |
Public areas | 263 (78) | Class IIb, LOE Cb |
Defibrillator throughout hospital | ||
Same brand and model | 281 (66) | |
Same brand, different models | 93 (22) | |
Different brands | 54 (13) | |
CPR assist technology useda | ||
None | 291 (70) | |
Capnography | 106 (25) | Class IIb, LOE Cb |
Mechanical CPR | 25 (6) | Class IIb, LOE B/Cbc |
Feedback device | 17 (4) | Class IIa, LOE B |
Quality improvement | ||
IHCA tracked | 336 (82) | Supportedbd |
Data reviewed | Supportedbd | |
Data not tracked/never reviewed | 85 (20) | |
Intermittently | 53 (12) | |
Routinely | 287 (68) | |
Routine cardiac arrest case reviews/debriefing | 149 (34) | Class IIa, LOE C |
Dedicated staff to resuscitation QI | 196 (49) | |
Full‐time equivalent staffing, median (IQR) | 0.5 (0.251.2) | |
Routine simulated resuscitation training | 268 (62) | |
Pre‐ and postarrest measures | ||
Hospitals with RRT | 391 (91) | Class I, LOE Cb |
Formal RRT‐specific training | ||
Never | 50 (14) | |
Once | 110 (30) | |
Recurrent | 163 (45) | |
TH protocol/order set in place | 252 (58) | |
Percent of patients with ROSC receiving TH | Class IIb, LOE Bb | |
<5% | 309 (74) | |
5%25% | 68 (16) | |
26%50% | 11 (3) | |
51%75% | 10 (2) | |
>75% | 18 (4) |
Hospitals reported that routine responders to IHCA events included respiratory therapists (n=414 [95%]), critical care nurses (n=406 [93%]), floor nurses (n=396 [90%]), attending physicians (n=392 [89%]), physician trainees (n=162 [37%]), and pharmacists (n=210 [48%]). Figure 1 shows the distribution of responders and team leaders by hospital type. Of the nonteaching hospitals, attending‐level physicians were likely to respond at 94% (265/281) and routinely lead the resuscitations at 84% (236/281), whereas, of major teaching hospitals, attending physicians were only likely to respond at 71% (30/42) and routinely lead at 19% (8/42).
Two‐thirds of the hospitals had a CPR committee (n=270 [66%]), and 196 (49%) had some staff time dedicated to resuscitation quality improvement. Hospitals with a specific committee dedicated to resuscitation and/or dedicated staff for resuscitation quality improvement were more likely to routinely track cardiac arrest data (odds ratio [OR]: 3.64, 95% confidence interval [CI]: 2.056.47 and OR: 2.02, 95% CI: 1.16‐3.54, respectively) and review the data (OR: 2.67, 95% CI: 1.45‐4.92 and OR: 2.18, 95% CI: 1.22‐3.89, respectively), after adjusting for teaching status and hospital size. These hospitals were also more likely to engage in simulation training and debriefing (Table 3).
CPR Committee, n=406 | Dedicated QI Staff, n=398 | |
---|---|---|
| ||
IHCA tracking | 3.64 (2.056.47) | 2.02 (1.16‐3.54) |
Routinely review | 2.67 (1.45‐4.92) | 2.18 (1.22‐3.89) |
Simulation training | 2.63 (1.66‐4.18) | 1.89 (1.24‐2.89) |
Debriefing | 3.19 (1.89‐5.36) | 2.14 (1.39‐3.32) |
Ninety percent (n=391) of respondents agreed that there is room for improvement in resuscitation practice at my hospital, and 70% (n=302) agreed that improved resuscitation would translate into improved patient outcomes. Overall, 78% (n=338) cited at least 1 barrier to improved resuscitation quality, of which the lack of adequate training (n=233 [54%]) and the lack of an appropriate champion (n=230 [53%]) were the most common. In subgroup analysis, nonteaching hospitals were significantly more likely to report the lack of a champion than their teaching counterparts (P=0.001) (Figure 2). In addition, we analyzed the data by hospitals that reported lack of a champion was not a barrier and compared them to those for whom it was, and found significantly higher adherence across all the measures in Table 2 supported by the 2010 guidelines, with the exception of real‐time feedback (data not shown).
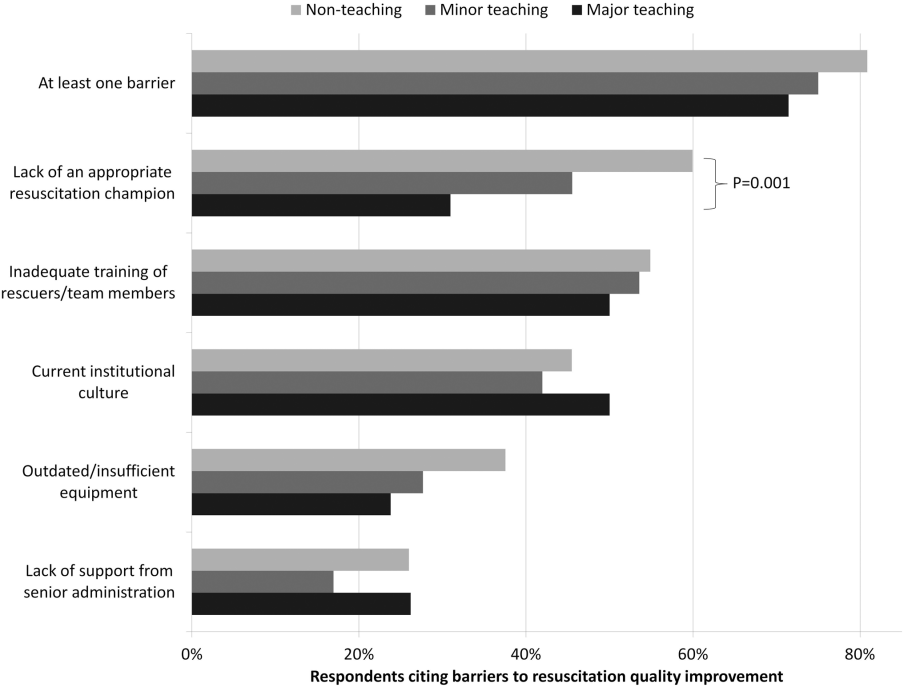
DISCUSSION
In this nationally representative sample of hospitals, we found considerable variability in cardiac arrest and resuscitation structures and processes, suggesting potential areas to target for improvement. Some practices, including use of RRTs and defibrillator standardization, were fairly routine, whereas others, such as therapeutic hypothermia and CPR assist technology, were rarely utilized. Quality initiatives, such as data tracking and review, simulation training, and debriefing were variable.
Several factors likely contribute to the variable implementation of evidence‐based practices. Guidelines alone have been shown to have little impact on practice by physicians in general.[11] This is supported by the lack of correlation we found between the presence, absence or strength of specific American Heart Association (AHA) emergency cardiovascular care treatment recommendations and the percent of hospitals reporting performing that measure. It is possible that other factors, such as a lack of familiarity or agreement with those guidelines, or the presence of external barriers, may be contributing.[12, 13] Specifically, the importance of a clinical champion was supported by our finding that hospitals reporting lack of a champion as a barrier were less likely to be adherent with guidelines. However, because the study did not directly test the impact of a champion, we wanted to be careful to avoid overstating or editorializing our results.
Some of the variability may also be related to the resource intensiveness of the practice. Routine simulation training and debriefing interventions, for example, are time intensive and require trained personnel to institute. That may explain the correlation we noted between these practices and the presence of CPR committee and dedicated personnel. The use of dedicated personnel was rare in this study, with less than half of respondents reporting any dedicated staff and a median of 0.5 full‐time equivalents for those reporting positively. This is in stark contrast to the routine use of resuscitation officers (primarily nurses dedicated to overseeing resuscitation practices and education at the hospital) in the United Kingdom.[14] Such a resuscitation officer model adopted by US hospitals could improve the quality and intensity of resuscitation care approaches.
Particularly surprising was the high rate of respondents (70%) reporting that they do not utilize any CPR assist technology. In the patient who does not have an arterial line, use of quantitative capnography is the best measure of cardiac output during cardiac arrest, yet only one‐quarter of hospitals reported using it, with no discrepancy between hospital type or size. A recent summit of national resuscitation experts expounded on the AHA guidelines suggesting that end‐tidal carbon dioxide should be used in all arrests to guide the quality of CPR with a goal value of >20.[8] Similarly, CPR feedback devices have an even higher level of evidence recommendation in the 2010 AHA guidelines than capnography, yet only 4% of hospitals reported utilizing them. Although it is true that introducing these CPR assist technologies into a hospital would require some effort on the part of hospital leadership, it is important to recognize the potential role such devices might play in the larger context of a resuscitation quality program to optimize clinical outcomes from IHCA.
Several differences were noted between hospitals based on teaching status. Although all hospitals were more likely to rely on physicians to lead resuscitations, nonteaching hospitals were more likely to report routine leadership by nurses and pharmacists. Nonteaching hospitals were also less likely to have a CPR committee, even after adjusting for hospital size. In addition, these hospitals were also more likely to report the lack of a clinical champion as a barrier to quality improvement.
There were several limitations to this study. First, this was a descriptive survey that was not tied to outcomes. As such, we are unable to draw conclusions about which practices correlate with decreased incidence of cardiac arrest and improved survival. Second, this was an optional survey with a somewhat limited response rate. Even though the characteristics of the nonresponding hospitals were similar to the responding hospitals, we cannot rule out the possibility that a selection bias was introduced, which would likely overestimate adherence to the guidelines. Self‐reported responses may have introduced additional errors. Finally, the short interval between the release of the 2010 guidelines and the administration of the first survey may have contributed to the variability in implementation of some practices, but many of the recommendations had been previously included in the 2005 guidelines.
We conclude that there is wide variability between hospitals and within practices for resuscitation care. Future work should seek to understand which practices are associated with improved patient outcomes and how best to implement these practices in a more uniform fashion.
Acknowledgements
The authors thank Nancy Hinckley, who championed the study; David Chearo, Christelle Marpaud, and Martha Van Haitsma of the University of Chicago Survey Lab for their assistance in formulating and distributing the survey; and JoAnne Resnic, Nicole Twu, and Frank Zadravecz for administrative support.
Disclosures: This study was supported by the Society of Hospital Medicine with a grant from Philips Healthcare (Andover, MA). Dr. Edelson is supported by a career development award from the National Heart, Lung, and Blood Institute (K23 HL097157). In addition, she has received research support and honoraria from Philips Healthcare (Andover, MA), research support from the American Heart Association (Dallas, TX) and Laerdal Medical (Stavanger, Norway), and an honorarium from Early Sense (Tel Aviv, Israel). Dr. Hunt has received research support from the Laerdal Foundation for Acute Medicine (Stavanger, Norway), the Hartwell Foundation (Memphis, TN), and the Arthur Vining Davis Foundation (Jacksonville, FL), and honoraria from the Kansas University Endowment (Kansas City, KS), JCCC (Overland Park, KS), and the UVA School of Medicine (Charlottesville, VA) and the European School of Management (Berlin, Germany). Dr. Mancini is supported in part by an Agency for Healthcare Research and Quality grant (R18HS020416). In addition, she has received research support from the American Heart Association (Dallas, TX) and Laerdal Medical (Stavanger, Norway), and honoraria from Sotera Wireless, Inc. (San Diego, CA). Dr. Abella has received research support from the National Institutes of Health (NIH), Medtronic Foundation (Minneapolis, MN), and Philips Healthcare (Andover, MA); has volunteered with the American Heart Association; and received honoraria from Heartsine (Belfast, Ireland), Velomedix (Menlo Park, CA), and Stryker (Kalamazoo, MI). Mr. Miller is employed by the Society of Hospital Medicine.
- Trends in survival after in‐hospital cardiac arrest. N Engl J Med. 2012;367(20):1912–1920. , , , , , .
- Incidence of treated cardiac arrest in hospitalized patients in the United States. Crit Care Med. 2011;39(11):2401–2406. , , , et al.
- Racial differences in survival after in‐hospital cardiac arrest. JAMA. 2009;302(11):1195–1201. , , , et al.
- Hospital variation in time to defibrillation after in‐hospital cardiac arrest. Arch Intern Med. 2009;169(14):1265–1273. , , , , .
- Duration of resuscitation efforts and survival after in‐hospital cardiac arrest: an observational study. Lancet. 2012;380(9852):1473–1481. , , , et al.
- Delayed time to defibrillation after in‐hospital cardiac arrest. N Engl J Med. 2008;358(1):9–17. , , , .
- 2010 American Heart Association guidelines for cardiopulmonary resuscitation and emergency cardiovascular care science. Circulation. 2010;122(18 suppl 3):S640–S946.
- Cardiopulmonary resuscitation quality: improving cardiac resuscitation outcomes both inside and outside the hospital: a consensus statement from the American Heart Association. Circulation. 2013;128(4):417–435. , , , et al.
- American Hospital Association. 2008 AHA annual survey. AHA data viewer: survey instruments. 2012; Available at: http://www.ahadataviewer.com/about/hospital‐database. Accessed October 11, 2013.
- The American Association for Public Opinion Research. Standard Definitions: Final Dispositions of Case Codes and Outcome Rates for Surveys. 7th ed. Deerfield, IL: AAPOR; 2011.
- Do practice guidelines guide practice? The effect of a consensus statement on the practice of physicians. N Engl J Med. 1989;321(19):1306–1311. , , , , , .
- Why don't physicians follow clinical practice guidelines? A framework for improvement. JAMA. 1999;282(15):1458–1465. , , , et al.
- From best evidence to best practice: effective implementation of change in patients' care. Lancet. 2003;362(9391):1225–1230. , .
- Cardiopulmonary resuscitation standards for clinical practice and training in the UK. Accid Emerg Nurs. 2005;13(3):171–179. , , , et al.
- Trends in survival after in‐hospital cardiac arrest. N Engl J Med. 2012;367(20):1912–1920. , , , , , .
- Incidence of treated cardiac arrest in hospitalized patients in the United States. Crit Care Med. 2011;39(11):2401–2406. , , , et al.
- Racial differences in survival after in‐hospital cardiac arrest. JAMA. 2009;302(11):1195–1201. , , , et al.
- Hospital variation in time to defibrillation after in‐hospital cardiac arrest. Arch Intern Med. 2009;169(14):1265–1273. , , , , .
- Duration of resuscitation efforts and survival after in‐hospital cardiac arrest: an observational study. Lancet. 2012;380(9852):1473–1481. , , , et al.
- Delayed time to defibrillation after in‐hospital cardiac arrest. N Engl J Med. 2008;358(1):9–17. , , , .
- 2010 American Heart Association guidelines for cardiopulmonary resuscitation and emergency cardiovascular care science. Circulation. 2010;122(18 suppl 3):S640–S946.
- Cardiopulmonary resuscitation quality: improving cardiac resuscitation outcomes both inside and outside the hospital: a consensus statement from the American Heart Association. Circulation. 2013;128(4):417–435. , , , et al.
- American Hospital Association. 2008 AHA annual survey. AHA data viewer: survey instruments. 2012; Available at: http://www.ahadataviewer.com/about/hospital‐database. Accessed October 11, 2013.
- The American Association for Public Opinion Research. Standard Definitions: Final Dispositions of Case Codes and Outcome Rates for Surveys. 7th ed. Deerfield, IL: AAPOR; 2011.
- Do practice guidelines guide practice? The effect of a consensus statement on the practice of physicians. N Engl J Med. 1989;321(19):1306–1311. , , , , , .
- Why don't physicians follow clinical practice guidelines? A framework for improvement. JAMA. 1999;282(15):1458–1465. , , , et al.
- From best evidence to best practice: effective implementation of change in patients' care. Lancet. 2003;362(9391):1225–1230. , .
- Cardiopulmonary resuscitation standards for clinical practice and training in the UK. Accid Emerg Nurs. 2005;13(3):171–179. , , , et al.
© 2014 Society of Hospital Medicine
Principles and Characteristics of an HMG
With the continuing growth of the specialty of hospital medicine, the capabilities and performance of hospital medicine groups (HMGs) varies significantly. There are few guidelines that HMGs can reference as tools to guide self‐improvement. To address this deficiency, the Society of Hospital Medicine (SHM) Board of Directors authorized a process to identify the key principles and characteristics of an effective HMG.
METHODS
Topic Development and Validation Prework
In providing direction to this effort, the SHM board felt that the principles and characteristics should be directed at both hospitals and hospitalists, addressing the full range of managerial, organizational, clinical, and quality activities necessary to achieve effectiveness. Furthermore, the board defined effectiveness as consisting of 2 components. First, the HMG must assure that the patients managed by hospitalists receive high‐quality care that is sensitive to their needs and preferences. Second, the HMG must understand that the central role of the hospitalist is to coordinate patient care and foster interdisciplinary communication across the care continuum to provide optimal patient outcomes.
The SHM board appointed an HMG Characteristics Workgroup consisting of individuals who have experience with a wide array of HMG models and who could offer expert opinions on the subject. The HMG Characteristics Workgroup felt it important to review the work of other organizations that develop and administer criteria, standards, and/or requirements for healthcare organizations. Examples cited were the American College of Surgeons[1]; The Joint Commission[2]; American Nurse Credentialing Center[3]; the National Committee for Quality Assurance[4]; the American Medical Group Association[5]; and the American Association of Critical‐Care Nurses.[6]
In March 2012 and April 2012, SHM staff reviewed the websites and published materials of these organizations. For each program, information was captured on the qualifications of applicants, history of the program, timing of administering the program, the nature of recognition granted, and the program's keys to success. The summary of these findings was shared with the workgroup.
Background research and the broad scope of characteristics to be addressed led to the workgroup's decision to develop the principles and characteristics using a consensus process, emphasizing expert opinion supplemented by feedback from a broad group of stakeholders.
Initial Draft
During April 2012 and May 2012, the HMG Characteristics Workgroup identified 3 domains for the key characteristics: (1) program structure and operations, (2) clinical care delivery, and (3) organizational performance improvement. Over the course of several meetings, the HMG Characteristics Workgroup developed an initial draft of 83 characteristics, grouped into 29 subgroups within the 3 domains.
From June 2012 to November 2012, this initial draft was reviewed by a broad cross section of the hospital medicine community including members of SHM's committees, a group of academic hospitalists, focus groups in 2 communities (Philadelphia and Boston), and the leaders of several regional and national hospitalist management companies. Quantitative and qualitative feedback was obtained.
In November 2012, the SHM Board of Directors held its annual leadership meeting, attended by approximately 25 national hospitalist thought leaders and chairpersons of SHM committees. At this meeting, a series of exercises were conducted in which these leaders of the hospital medicine movement, including the SHM board members, were each assigned individual characteristics and asked to review and edit them for clarity and appropriateness.
As a result of feedback at that meeting and subsequent discussion by the SHM board, the workgroup was asked to modify the characteristics in 3 ways. First, the list should be streamlined, reducing the number of characteristics. Second, the 3 domains should be eliminated, and a better organizing framework should be created. Third, additional context should be added to the list of characteristics.
Second Draft
During the period from November 2012 to December 2012, the HMG Characteristics Workgroup went through a 2‐step Delphi process to consolidate characteristics and/or eliminate characteristics that were redundant or unnecessary. In the first step, members of the workgroup rated each characteristic from 1 to 3. A rating of 1 meant not important; good quality, but not required for an effective HMG. A rating of 2 meant important; most effective HMGs will meet requirement. A rating of 3 meant highly important; mandatory for an effective HMG. In the second step, members of the workgroup received feedback on the scores for each characteristic and came to a consensus on which characteristics should be eliminated or merged with other characteristics.
As a result, the number of characteristics was reduced and consolidated from 83 to 47, and a new framing structure was defined, replacing the 3 domains with 10 organizing principles. Finally, a rationale for each characteristic was added, defending its inclusion in the list. In addition, consideration was given to including a section describing how an HMG could demonstrate that their organization met each characteristic. However, the workgroup and the board decided that these demonstration requirements should be vetted before they were published.
From January 2013 to June 2013, the revised key principles and characteristics were reviewed by selected chairpersons of SHM committees and by 2 focus groups of HMG leaders. These reviews were conducted at the SHM Annual Meeting. Finally, in June 2013, the Committee on Clinical Leadership of the American Hospital Association reviewed and commented on the draft of the principles and characteristics.
In addition, based on feedback received from the reviewers, the wording of many of the characteristics went through revisions to assure precision and clarity. Before submission to the Journal of Hospital Medicine, a professional editor was engaged to assure that the format and language of the characteristics were clear and consistent.
Final Approval
The final draft of the 10 principles and 47 characteristics was approved for publication at a meeting of the SHM Board of Directors in September 2013 (Figure 1).
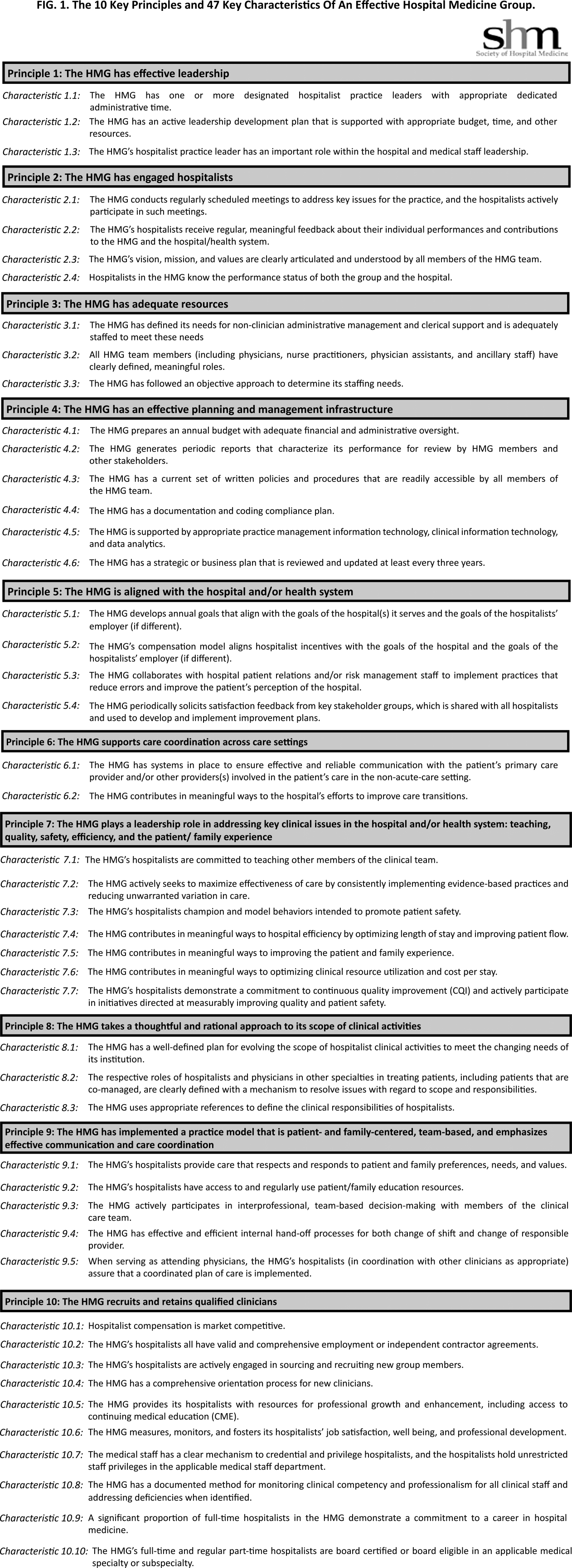
RESULTS
A recurring issue that the workgroup addressed was the applicability of the characteristics from 1 practice setting to another. Confounding factors include the HMG's employment/organizational model (eg, hospital employed, academic, multispecialty group, private practice, and management company), its population served (eg, adult vs pediatric, more than 1 hospital), and the type of hospital served (eg, academic vs community, the hospital has more than 1 HMG). The workgroup has made an effort to assure that all 47 characteristics can be applied to every type of HMG.
In developing the 10 principles, the workgroup attempted to construct a list of the basic ingredients needed to build and sustain an effective HMG. These 10 principles stand on their own, independent of the 47 key characteristics, and include issues such as effective leadership, clinician engagement, adequate resources, management infrastructure, key hospitalist roles and responsibilities, alignment with the hospital, and the recruitment and retention of qualified hospitalists.
A more detailed version of the Key Principles and Characteristics of an Effective HMG is available in the online version of this article (see Supporting Information, Appendix, in the online version of this article). The online Appendix includes the rationales for each of the characteristics, guidance on how to provide feedback to the SHM on the framework, and the SHM's plan for further development of the key principles and characteristics.
DISCUSSION
To address the variability in capabilities and performance of HMGs, these principles and characteristics are designed to provide a framework for HMGs seeking to conduct self‐assessments and develop pathways for improvement.
Although there may be HMG arrangements that do not directly involve the hospital and its executive team, and therefore alternative approaches may make sense, for most HMGs hospitals are directly involved with the HMG as either an employer or a contractor. For that reason, the Key Principles and Characteristics of an Effective HMG is written for 2 audiences: the executive leadership of the hospital (most specifically the chief medical officer or a similar role) and the hospitalists in the HMG (most specifically the practice medical director). To address the key characteristics requires the active participation of both parties. For the hospital executives, the framework establishes expectations for the HMG. For the hospitalists, the framework provides guidance in the development of an improvement plan.
Hospital executives and hospitalists can use the key characteristics in a broad spectrum of ways. The easiest and least formalized approach would be to use the framework as the basis of an ongoing dialogue between the hospital leadership and the HMG. A more formal approach would be to use the framework to guide the planning and budgeting activities of the HMG. Finally, a hospital or health system can use the key principles and characteristics as a way to evaluate their affiliated HMG(s)for example, the HMG must address 80% of the 47 characteristics.
The Key Principles and Characteristics of an Effective HMG should be considered akin to the Core Competencies in Hospital Medicine previously published in the Journal of Hospital Medicine.[7] However, instead of focusing on the competencies of individual physicians, this framework focuses on the characteristics of hospitalist groups. Just as a physician or other healthcare provider is not expected to demonstrate competency for every element in the core competencies document, an HMG does not need to have all 47 characteristics to be effective. Effective hospitalists may have skills other than those listed in the Core Competencies in Hospital Medicine. Similarly, the 47 characteristics do not represent an exhaustive list of every desirable HMG attribute. In general, effective HMGs should possess most of the characteristics.
In applying the framework, the HMG should not simply attempt to evaluate each characteristic with a yes or no assessment. For HMGs responding yes, there may be a wide range of performancefrom meeting the bare minimum requirements to employing sophisticated, expansive measures to excel in the characteristic.
SHM encourages hospital leaders and HMG leaders to use these characteristics to perform an HMG self‐assessment and to develop a plan. The plan could address implementation of selected characteristics that are not currently being addressed by the HMG or the development of additional behaviors, tools, resources, and capabilities that more fully incorporate those characteristics for which the HMG meets only minimum requirements. In addition, the plan could address the impact that a larger organization (eg, health system, hospital, or employer) may have on a given characteristic.
As outlined above, the process used to develop the Key Principles and Characteristics of an Effective HMG was grounded in expert opinion and extensive review and feedback. HMGs that use the framework should recognize that others might have a different opinion. For example, characteristic 5.2 states, The HMG's compensation model aligns hospitalist incentives with the goals of the hospital and the goals of the hospitalist's employer (if different). There are likely to be experienced hospitalist leaders who believe that an effective HMG does not need to have an incentive compensation system. However, the consensus process employed to develop the key characteristics led to the conclusion that an effective HMG should have an incentive compensation system.
The publication of the Key Principles and Characteristics of an Effective HMG may lead to negative and/or unintended consequences. A self‐assessment by an HMG using this framework could require a significant level of effort on behalf of the HMG, whereas implementing remedial efforts to address the characteristics could require an investment of time and money that could take away from other important issues facing the HMG. Many HMGs may be held accountable for addressing these characteristics without the necessary financial support from their hospital or medical group. Finally, the publication of the document could create a backlash from members of the hospitalist community who do not think that the SHM should be in the business of defining what characterizes an effective HMG, rather that this definition should be left to the marketplace.
Despite these concerns, the leadership of the SHM expects that the publication of the Key Principles and Characteristics of an Effective HMG will lead to overall improvement in the capabilities and performance of HMGs.
CONCLUSIONS
The Key Principles and Characteristics of an Effective HMG have been designed to be aspirational, helping to raise the bar for the specialty of hospital medicine. These principles and characteristics could provide a framework for HMGs seeking to conduct self‐assessments, outlining a pathway for improvement, and better defining the central role of hospitalists in coordinating team‐based, patient‐centered care in the acute care setting.
Acknowledgments
Disclosures: Patrick Cawley, MD: none; Steven Deitelzweig, MD: none; Leslie Flores, MHA: provides consulting to hospital medicine groups; Joseph A. Miller, MS: none; John Nelson, MD: provides consulting to hospital medicine groups; Scott Rissmiller, MD: none; Laurence Wellikson, MD: none; Winthrop F. Whitcomb, MD: provides consulting to hospital medicine groups.
- American College of Surgeons. New verification site visit outcomes. Available at: http://www.facs.org/trauma/verifivisitoutcomes.html. Accessed September 3, 2013.
- Hospital accreditation standards 2012. Oakbrook Terrace, IL: The Joint Commission; 2012. Available at: Amazon.com: http://www.amazon.com/Hospital‐Accreditation‐Standards‐Joint‐Commission/dp/1599404257
- The magnet model: components and sources of evidence. Silver Spring, MD: American Nurse Credentialing Center; 2011. Available at: Amazon.com: http://www.amazon.com/Magnet‐Model‐Components‐Sources‐Evidence/dp/1935213229.
- Patient Centered Medical Home Standards and Guidelines. National Committee for Quality Assurance. Available at: https://inetshop01.pub.ncqa.org/Publications/deptCate.asp?dept_id=21(suppl 1):2–95.
With the continuing growth of the specialty of hospital medicine, the capabilities and performance of hospital medicine groups (HMGs) varies significantly. There are few guidelines that HMGs can reference as tools to guide self‐improvement. To address this deficiency, the Society of Hospital Medicine (SHM) Board of Directors authorized a process to identify the key principles and characteristics of an effective HMG.
METHODS
Topic Development and Validation Prework
In providing direction to this effort, the SHM board felt that the principles and characteristics should be directed at both hospitals and hospitalists, addressing the full range of managerial, organizational, clinical, and quality activities necessary to achieve effectiveness. Furthermore, the board defined effectiveness as consisting of 2 components. First, the HMG must assure that the patients managed by hospitalists receive high‐quality care that is sensitive to their needs and preferences. Second, the HMG must understand that the central role of the hospitalist is to coordinate patient care and foster interdisciplinary communication across the care continuum to provide optimal patient outcomes.
The SHM board appointed an HMG Characteristics Workgroup consisting of individuals who have experience with a wide array of HMG models and who could offer expert opinions on the subject. The HMG Characteristics Workgroup felt it important to review the work of other organizations that develop and administer criteria, standards, and/or requirements for healthcare organizations. Examples cited were the American College of Surgeons[1]; The Joint Commission[2]; American Nurse Credentialing Center[3]; the National Committee for Quality Assurance[4]; the American Medical Group Association[5]; and the American Association of Critical‐Care Nurses.[6]
In March 2012 and April 2012, SHM staff reviewed the websites and published materials of these organizations. For each program, information was captured on the qualifications of applicants, history of the program, timing of administering the program, the nature of recognition granted, and the program's keys to success. The summary of these findings was shared with the workgroup.
Background research and the broad scope of characteristics to be addressed led to the workgroup's decision to develop the principles and characteristics using a consensus process, emphasizing expert opinion supplemented by feedback from a broad group of stakeholders.
Initial Draft
During April 2012 and May 2012, the HMG Characteristics Workgroup identified 3 domains for the key characteristics: (1) program structure and operations, (2) clinical care delivery, and (3) organizational performance improvement. Over the course of several meetings, the HMG Characteristics Workgroup developed an initial draft of 83 characteristics, grouped into 29 subgroups within the 3 domains.
From June 2012 to November 2012, this initial draft was reviewed by a broad cross section of the hospital medicine community including members of SHM's committees, a group of academic hospitalists, focus groups in 2 communities (Philadelphia and Boston), and the leaders of several regional and national hospitalist management companies. Quantitative and qualitative feedback was obtained.
In November 2012, the SHM Board of Directors held its annual leadership meeting, attended by approximately 25 national hospitalist thought leaders and chairpersons of SHM committees. At this meeting, a series of exercises were conducted in which these leaders of the hospital medicine movement, including the SHM board members, were each assigned individual characteristics and asked to review and edit them for clarity and appropriateness.
As a result of feedback at that meeting and subsequent discussion by the SHM board, the workgroup was asked to modify the characteristics in 3 ways. First, the list should be streamlined, reducing the number of characteristics. Second, the 3 domains should be eliminated, and a better organizing framework should be created. Third, additional context should be added to the list of characteristics.
Second Draft
During the period from November 2012 to December 2012, the HMG Characteristics Workgroup went through a 2‐step Delphi process to consolidate characteristics and/or eliminate characteristics that were redundant or unnecessary. In the first step, members of the workgroup rated each characteristic from 1 to 3. A rating of 1 meant not important; good quality, but not required for an effective HMG. A rating of 2 meant important; most effective HMGs will meet requirement. A rating of 3 meant highly important; mandatory for an effective HMG. In the second step, members of the workgroup received feedback on the scores for each characteristic and came to a consensus on which characteristics should be eliminated or merged with other characteristics.
As a result, the number of characteristics was reduced and consolidated from 83 to 47, and a new framing structure was defined, replacing the 3 domains with 10 organizing principles. Finally, a rationale for each characteristic was added, defending its inclusion in the list. In addition, consideration was given to including a section describing how an HMG could demonstrate that their organization met each characteristic. However, the workgroup and the board decided that these demonstration requirements should be vetted before they were published.
From January 2013 to June 2013, the revised key principles and characteristics were reviewed by selected chairpersons of SHM committees and by 2 focus groups of HMG leaders. These reviews were conducted at the SHM Annual Meeting. Finally, in June 2013, the Committee on Clinical Leadership of the American Hospital Association reviewed and commented on the draft of the principles and characteristics.
In addition, based on feedback received from the reviewers, the wording of many of the characteristics went through revisions to assure precision and clarity. Before submission to the Journal of Hospital Medicine, a professional editor was engaged to assure that the format and language of the characteristics were clear and consistent.
Final Approval
The final draft of the 10 principles and 47 characteristics was approved for publication at a meeting of the SHM Board of Directors in September 2013 (Figure 1).
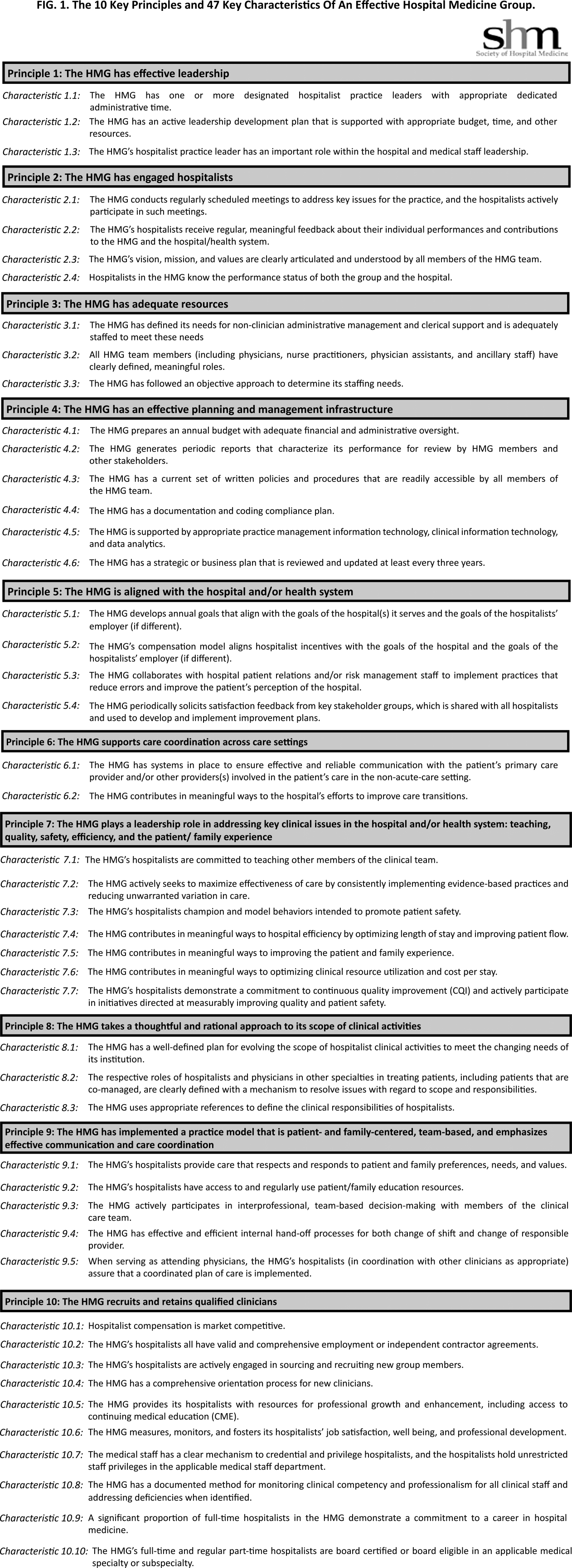
RESULTS
A recurring issue that the workgroup addressed was the applicability of the characteristics from 1 practice setting to another. Confounding factors include the HMG's employment/organizational model (eg, hospital employed, academic, multispecialty group, private practice, and management company), its population served (eg, adult vs pediatric, more than 1 hospital), and the type of hospital served (eg, academic vs community, the hospital has more than 1 HMG). The workgroup has made an effort to assure that all 47 characteristics can be applied to every type of HMG.
In developing the 10 principles, the workgroup attempted to construct a list of the basic ingredients needed to build and sustain an effective HMG. These 10 principles stand on their own, independent of the 47 key characteristics, and include issues such as effective leadership, clinician engagement, adequate resources, management infrastructure, key hospitalist roles and responsibilities, alignment with the hospital, and the recruitment and retention of qualified hospitalists.
A more detailed version of the Key Principles and Characteristics of an Effective HMG is available in the online version of this article (see Supporting Information, Appendix, in the online version of this article). The online Appendix includes the rationales for each of the characteristics, guidance on how to provide feedback to the SHM on the framework, and the SHM's plan for further development of the key principles and characteristics.
DISCUSSION
To address the variability in capabilities and performance of HMGs, these principles and characteristics are designed to provide a framework for HMGs seeking to conduct self‐assessments and develop pathways for improvement.
Although there may be HMG arrangements that do not directly involve the hospital and its executive team, and therefore alternative approaches may make sense, for most HMGs hospitals are directly involved with the HMG as either an employer or a contractor. For that reason, the Key Principles and Characteristics of an Effective HMG is written for 2 audiences: the executive leadership of the hospital (most specifically the chief medical officer or a similar role) and the hospitalists in the HMG (most specifically the practice medical director). To address the key characteristics requires the active participation of both parties. For the hospital executives, the framework establishes expectations for the HMG. For the hospitalists, the framework provides guidance in the development of an improvement plan.
Hospital executives and hospitalists can use the key characteristics in a broad spectrum of ways. The easiest and least formalized approach would be to use the framework as the basis of an ongoing dialogue between the hospital leadership and the HMG. A more formal approach would be to use the framework to guide the planning and budgeting activities of the HMG. Finally, a hospital or health system can use the key principles and characteristics as a way to evaluate their affiliated HMG(s)for example, the HMG must address 80% of the 47 characteristics.
The Key Principles and Characteristics of an Effective HMG should be considered akin to the Core Competencies in Hospital Medicine previously published in the Journal of Hospital Medicine.[7] However, instead of focusing on the competencies of individual physicians, this framework focuses on the characteristics of hospitalist groups. Just as a physician or other healthcare provider is not expected to demonstrate competency for every element in the core competencies document, an HMG does not need to have all 47 characteristics to be effective. Effective hospitalists may have skills other than those listed in the Core Competencies in Hospital Medicine. Similarly, the 47 characteristics do not represent an exhaustive list of every desirable HMG attribute. In general, effective HMGs should possess most of the characteristics.
In applying the framework, the HMG should not simply attempt to evaluate each characteristic with a yes or no assessment. For HMGs responding yes, there may be a wide range of performancefrom meeting the bare minimum requirements to employing sophisticated, expansive measures to excel in the characteristic.
SHM encourages hospital leaders and HMG leaders to use these characteristics to perform an HMG self‐assessment and to develop a plan. The plan could address implementation of selected characteristics that are not currently being addressed by the HMG or the development of additional behaviors, tools, resources, and capabilities that more fully incorporate those characteristics for which the HMG meets only minimum requirements. In addition, the plan could address the impact that a larger organization (eg, health system, hospital, or employer) may have on a given characteristic.
As outlined above, the process used to develop the Key Principles and Characteristics of an Effective HMG was grounded in expert opinion and extensive review and feedback. HMGs that use the framework should recognize that others might have a different opinion. For example, characteristic 5.2 states, The HMG's compensation model aligns hospitalist incentives with the goals of the hospital and the goals of the hospitalist's employer (if different). There are likely to be experienced hospitalist leaders who believe that an effective HMG does not need to have an incentive compensation system. However, the consensus process employed to develop the key characteristics led to the conclusion that an effective HMG should have an incentive compensation system.
The publication of the Key Principles and Characteristics of an Effective HMG may lead to negative and/or unintended consequences. A self‐assessment by an HMG using this framework could require a significant level of effort on behalf of the HMG, whereas implementing remedial efforts to address the characteristics could require an investment of time and money that could take away from other important issues facing the HMG. Many HMGs may be held accountable for addressing these characteristics without the necessary financial support from their hospital or medical group. Finally, the publication of the document could create a backlash from members of the hospitalist community who do not think that the SHM should be in the business of defining what characterizes an effective HMG, rather that this definition should be left to the marketplace.
Despite these concerns, the leadership of the SHM expects that the publication of the Key Principles and Characteristics of an Effective HMG will lead to overall improvement in the capabilities and performance of HMGs.
CONCLUSIONS
The Key Principles and Characteristics of an Effective HMG have been designed to be aspirational, helping to raise the bar for the specialty of hospital medicine. These principles and characteristics could provide a framework for HMGs seeking to conduct self‐assessments, outlining a pathway for improvement, and better defining the central role of hospitalists in coordinating team‐based, patient‐centered care in the acute care setting.
Acknowledgments
Disclosures: Patrick Cawley, MD: none; Steven Deitelzweig, MD: none; Leslie Flores, MHA: provides consulting to hospital medicine groups; Joseph A. Miller, MS: none; John Nelson, MD: provides consulting to hospital medicine groups; Scott Rissmiller, MD: none; Laurence Wellikson, MD: none; Winthrop F. Whitcomb, MD: provides consulting to hospital medicine groups.
With the continuing growth of the specialty of hospital medicine, the capabilities and performance of hospital medicine groups (HMGs) varies significantly. There are few guidelines that HMGs can reference as tools to guide self‐improvement. To address this deficiency, the Society of Hospital Medicine (SHM) Board of Directors authorized a process to identify the key principles and characteristics of an effective HMG.
METHODS
Topic Development and Validation Prework
In providing direction to this effort, the SHM board felt that the principles and characteristics should be directed at both hospitals and hospitalists, addressing the full range of managerial, organizational, clinical, and quality activities necessary to achieve effectiveness. Furthermore, the board defined effectiveness as consisting of 2 components. First, the HMG must assure that the patients managed by hospitalists receive high‐quality care that is sensitive to their needs and preferences. Second, the HMG must understand that the central role of the hospitalist is to coordinate patient care and foster interdisciplinary communication across the care continuum to provide optimal patient outcomes.
The SHM board appointed an HMG Characteristics Workgroup consisting of individuals who have experience with a wide array of HMG models and who could offer expert opinions on the subject. The HMG Characteristics Workgroup felt it important to review the work of other organizations that develop and administer criteria, standards, and/or requirements for healthcare organizations. Examples cited were the American College of Surgeons[1]; The Joint Commission[2]; American Nurse Credentialing Center[3]; the National Committee for Quality Assurance[4]; the American Medical Group Association[5]; and the American Association of Critical‐Care Nurses.[6]
In March 2012 and April 2012, SHM staff reviewed the websites and published materials of these organizations. For each program, information was captured on the qualifications of applicants, history of the program, timing of administering the program, the nature of recognition granted, and the program's keys to success. The summary of these findings was shared with the workgroup.
Background research and the broad scope of characteristics to be addressed led to the workgroup's decision to develop the principles and characteristics using a consensus process, emphasizing expert opinion supplemented by feedback from a broad group of stakeholders.
Initial Draft
During April 2012 and May 2012, the HMG Characteristics Workgroup identified 3 domains for the key characteristics: (1) program structure and operations, (2) clinical care delivery, and (3) organizational performance improvement. Over the course of several meetings, the HMG Characteristics Workgroup developed an initial draft of 83 characteristics, grouped into 29 subgroups within the 3 domains.
From June 2012 to November 2012, this initial draft was reviewed by a broad cross section of the hospital medicine community including members of SHM's committees, a group of academic hospitalists, focus groups in 2 communities (Philadelphia and Boston), and the leaders of several regional and national hospitalist management companies. Quantitative and qualitative feedback was obtained.
In November 2012, the SHM Board of Directors held its annual leadership meeting, attended by approximately 25 national hospitalist thought leaders and chairpersons of SHM committees. At this meeting, a series of exercises were conducted in which these leaders of the hospital medicine movement, including the SHM board members, were each assigned individual characteristics and asked to review and edit them for clarity and appropriateness.
As a result of feedback at that meeting and subsequent discussion by the SHM board, the workgroup was asked to modify the characteristics in 3 ways. First, the list should be streamlined, reducing the number of characteristics. Second, the 3 domains should be eliminated, and a better organizing framework should be created. Third, additional context should be added to the list of characteristics.
Second Draft
During the period from November 2012 to December 2012, the HMG Characteristics Workgroup went through a 2‐step Delphi process to consolidate characteristics and/or eliminate characteristics that were redundant or unnecessary. In the first step, members of the workgroup rated each characteristic from 1 to 3. A rating of 1 meant not important; good quality, but not required for an effective HMG. A rating of 2 meant important; most effective HMGs will meet requirement. A rating of 3 meant highly important; mandatory for an effective HMG. In the second step, members of the workgroup received feedback on the scores for each characteristic and came to a consensus on which characteristics should be eliminated or merged with other characteristics.
As a result, the number of characteristics was reduced and consolidated from 83 to 47, and a new framing structure was defined, replacing the 3 domains with 10 organizing principles. Finally, a rationale for each characteristic was added, defending its inclusion in the list. In addition, consideration was given to including a section describing how an HMG could demonstrate that their organization met each characteristic. However, the workgroup and the board decided that these demonstration requirements should be vetted before they were published.
From January 2013 to June 2013, the revised key principles and characteristics were reviewed by selected chairpersons of SHM committees and by 2 focus groups of HMG leaders. These reviews were conducted at the SHM Annual Meeting. Finally, in June 2013, the Committee on Clinical Leadership of the American Hospital Association reviewed and commented on the draft of the principles and characteristics.
In addition, based on feedback received from the reviewers, the wording of many of the characteristics went through revisions to assure precision and clarity. Before submission to the Journal of Hospital Medicine, a professional editor was engaged to assure that the format and language of the characteristics were clear and consistent.
Final Approval
The final draft of the 10 principles and 47 characteristics was approved for publication at a meeting of the SHM Board of Directors in September 2013 (Figure 1).
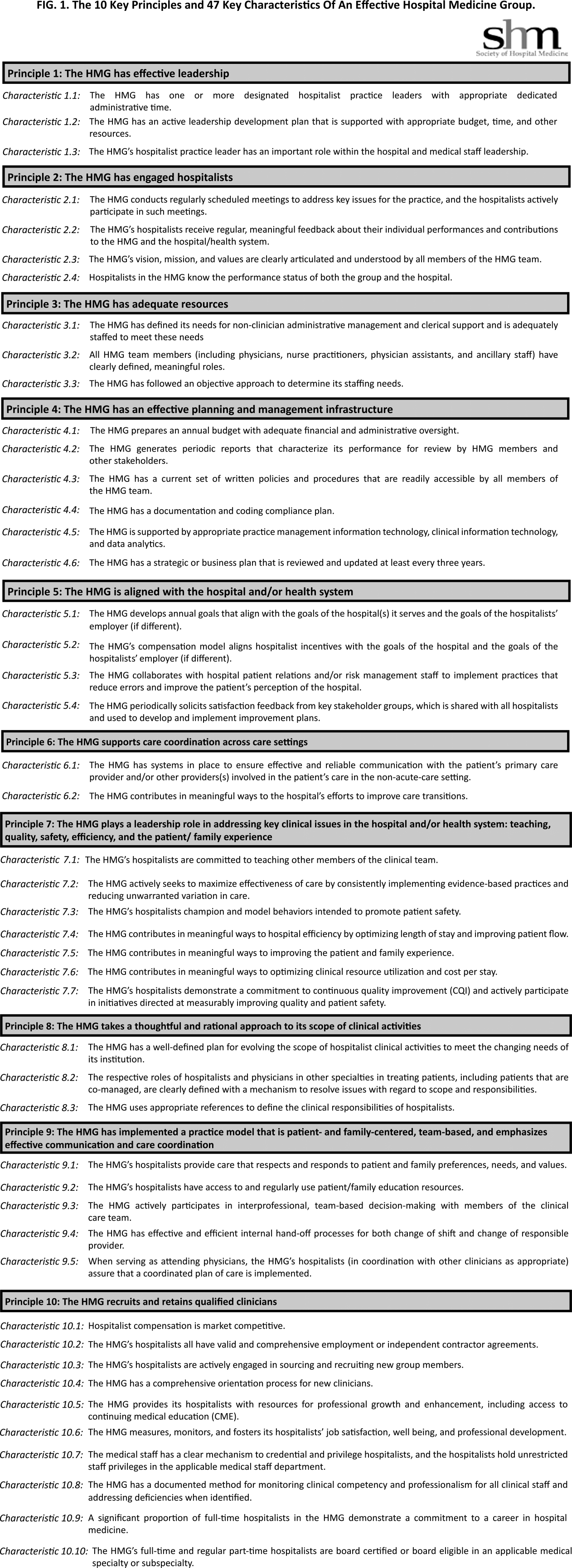
RESULTS
A recurring issue that the workgroup addressed was the applicability of the characteristics from 1 practice setting to another. Confounding factors include the HMG's employment/organizational model (eg, hospital employed, academic, multispecialty group, private practice, and management company), its population served (eg, adult vs pediatric, more than 1 hospital), and the type of hospital served (eg, academic vs community, the hospital has more than 1 HMG). The workgroup has made an effort to assure that all 47 characteristics can be applied to every type of HMG.
In developing the 10 principles, the workgroup attempted to construct a list of the basic ingredients needed to build and sustain an effective HMG. These 10 principles stand on their own, independent of the 47 key characteristics, and include issues such as effective leadership, clinician engagement, adequate resources, management infrastructure, key hospitalist roles and responsibilities, alignment with the hospital, and the recruitment and retention of qualified hospitalists.
A more detailed version of the Key Principles and Characteristics of an Effective HMG is available in the online version of this article (see Supporting Information, Appendix, in the online version of this article). The online Appendix includes the rationales for each of the characteristics, guidance on how to provide feedback to the SHM on the framework, and the SHM's plan for further development of the key principles and characteristics.
DISCUSSION
To address the variability in capabilities and performance of HMGs, these principles and characteristics are designed to provide a framework for HMGs seeking to conduct self‐assessments and develop pathways for improvement.
Although there may be HMG arrangements that do not directly involve the hospital and its executive team, and therefore alternative approaches may make sense, for most HMGs hospitals are directly involved with the HMG as either an employer or a contractor. For that reason, the Key Principles and Characteristics of an Effective HMG is written for 2 audiences: the executive leadership of the hospital (most specifically the chief medical officer or a similar role) and the hospitalists in the HMG (most specifically the practice medical director). To address the key characteristics requires the active participation of both parties. For the hospital executives, the framework establishes expectations for the HMG. For the hospitalists, the framework provides guidance in the development of an improvement plan.
Hospital executives and hospitalists can use the key characteristics in a broad spectrum of ways. The easiest and least formalized approach would be to use the framework as the basis of an ongoing dialogue between the hospital leadership and the HMG. A more formal approach would be to use the framework to guide the planning and budgeting activities of the HMG. Finally, a hospital or health system can use the key principles and characteristics as a way to evaluate their affiliated HMG(s)for example, the HMG must address 80% of the 47 characteristics.
The Key Principles and Characteristics of an Effective HMG should be considered akin to the Core Competencies in Hospital Medicine previously published in the Journal of Hospital Medicine.[7] However, instead of focusing on the competencies of individual physicians, this framework focuses on the characteristics of hospitalist groups. Just as a physician or other healthcare provider is not expected to demonstrate competency for every element in the core competencies document, an HMG does not need to have all 47 characteristics to be effective. Effective hospitalists may have skills other than those listed in the Core Competencies in Hospital Medicine. Similarly, the 47 characteristics do not represent an exhaustive list of every desirable HMG attribute. In general, effective HMGs should possess most of the characteristics.
In applying the framework, the HMG should not simply attempt to evaluate each characteristic with a yes or no assessment. For HMGs responding yes, there may be a wide range of performancefrom meeting the bare minimum requirements to employing sophisticated, expansive measures to excel in the characteristic.
SHM encourages hospital leaders and HMG leaders to use these characteristics to perform an HMG self‐assessment and to develop a plan. The plan could address implementation of selected characteristics that are not currently being addressed by the HMG or the development of additional behaviors, tools, resources, and capabilities that more fully incorporate those characteristics for which the HMG meets only minimum requirements. In addition, the plan could address the impact that a larger organization (eg, health system, hospital, or employer) may have on a given characteristic.
As outlined above, the process used to develop the Key Principles and Characteristics of an Effective HMG was grounded in expert opinion and extensive review and feedback. HMGs that use the framework should recognize that others might have a different opinion. For example, characteristic 5.2 states, The HMG's compensation model aligns hospitalist incentives with the goals of the hospital and the goals of the hospitalist's employer (if different). There are likely to be experienced hospitalist leaders who believe that an effective HMG does not need to have an incentive compensation system. However, the consensus process employed to develop the key characteristics led to the conclusion that an effective HMG should have an incentive compensation system.
The publication of the Key Principles and Characteristics of an Effective HMG may lead to negative and/or unintended consequences. A self‐assessment by an HMG using this framework could require a significant level of effort on behalf of the HMG, whereas implementing remedial efforts to address the characteristics could require an investment of time and money that could take away from other important issues facing the HMG. Many HMGs may be held accountable for addressing these characteristics without the necessary financial support from their hospital or medical group. Finally, the publication of the document could create a backlash from members of the hospitalist community who do not think that the SHM should be in the business of defining what characterizes an effective HMG, rather that this definition should be left to the marketplace.
Despite these concerns, the leadership of the SHM expects that the publication of the Key Principles and Characteristics of an Effective HMG will lead to overall improvement in the capabilities and performance of HMGs.
CONCLUSIONS
The Key Principles and Characteristics of an Effective HMG have been designed to be aspirational, helping to raise the bar for the specialty of hospital medicine. These principles and characteristics could provide a framework for HMGs seeking to conduct self‐assessments, outlining a pathway for improvement, and better defining the central role of hospitalists in coordinating team‐based, patient‐centered care in the acute care setting.
Acknowledgments
Disclosures: Patrick Cawley, MD: none; Steven Deitelzweig, MD: none; Leslie Flores, MHA: provides consulting to hospital medicine groups; Joseph A. Miller, MS: none; John Nelson, MD: provides consulting to hospital medicine groups; Scott Rissmiller, MD: none; Laurence Wellikson, MD: none; Winthrop F. Whitcomb, MD: provides consulting to hospital medicine groups.
- American College of Surgeons. New verification site visit outcomes. Available at: http://www.facs.org/trauma/verifivisitoutcomes.html. Accessed September 3, 2013.
- Hospital accreditation standards 2012. Oakbrook Terrace, IL: The Joint Commission; 2012. Available at: Amazon.com: http://www.amazon.com/Hospital‐Accreditation‐Standards‐Joint‐Commission/dp/1599404257
- The magnet model: components and sources of evidence. Silver Spring, MD: American Nurse Credentialing Center; 2011. Available at: Amazon.com: http://www.amazon.com/Magnet‐Model‐Components‐Sources‐Evidence/dp/1935213229.
- Patient Centered Medical Home Standards and Guidelines. National Committee for Quality Assurance. Available at: https://inetshop01.pub.ncqa.org/Publications/deptCate.asp?dept_id=21(suppl 1):2–95.
- American College of Surgeons. New verification site visit outcomes. Available at: http://www.facs.org/trauma/verifivisitoutcomes.html. Accessed September 3, 2013.
- Hospital accreditation standards 2012. Oakbrook Terrace, IL: The Joint Commission; 2012. Available at: Amazon.com: http://www.amazon.com/Hospital‐Accreditation‐Standards‐Joint‐Commission/dp/1599404257
- The magnet model: components and sources of evidence. Silver Spring, MD: American Nurse Credentialing Center; 2011. Available at: Amazon.com: http://www.amazon.com/Magnet‐Model‐Components‐Sources‐Evidence/dp/1935213229.
- Patient Centered Medical Home Standards and Guidelines. National Committee for Quality Assurance. Available at: https://inetshop01.pub.ncqa.org/Publications/deptCate.asp?dept_id=21(suppl 1):2–95.
Hospitalist‐Job Fit
Person‐organization fit concerns the conditions and consequences of compatibility between people and the organizations for which they work.[1] Studies of other industries have demonstrated that person‐organization fit informs the way individuals join, perform in, and are retained by organizations.[2] Person‐job fit is a closely related subordinate concept that concerns the alignment of workers and their job in as much as workers have needs that their job supplies, or conversely, jobs have requirements that certain workers' abilities can help meet.[3] Explorations of job fit in physicians and their work have recently emerged in a few investigations published in medical journals.[4, 5, 6, 7, 8] Further expanding the understanding of fit between physicians and their employment is important, because the decline of solo practices and recent emphasis on team‐based care have led to a growing number of US physicians working in organizations.[9]
The movement of physicians into employed situations may continue if certain types of Accountable Care Organizations take root.[10] And physicians may be primed to join employer organizations based on current career priorities of individuals in American society. Surveys of medical residents entering the workforce reveal more physicians preferring the security of being employees than starting their own practices.[11] Given these trends, job fit will inform our understanding of how personal and job characteristics facilitate recruitment, performance, satisfaction, and longevity of physician employees.
BACKGROUND
Virtually all hospitalists work in organizationshospitalsand are employees of hospitals, medical schools, physician group practices, or management companies, and therefore invariably function within organizational structures and systems.[7] In spite of their rapid growth in numbers, many employers have faced difficulties recruiting and retaining enough hospitalists to fill their staffing needs. Consequently, the US hospitalist workforce today is characterized by high salaries, work load, and attrition rates.[12]
In this evolving unsaturated market, the attraction‐selection‐attrition framework[13] provides a theoretical construct that predicts that hospitalists and their employers would seek congruence of goals and values early in their relationship through a process of trial and error. This framework assumes that early interactions between workers and organizations serve as opportunities for them to understand if job fit is poor and dissociate or remain affiliated as long as job fit is mutually acceptable. Therefore, job switching on average is expected to increase job fit because workers and organizations gain a better understanding of their own goals and values and choose more wisely the next time.
Other theoretical frameworks, such as the job characteristic model,[14] suggest that over time as workers stay at the same job, they continue to maintain and improve job fit through various workplace‐ or self‐modification strategies. For example, seniority status may have privileges (eg, less undesirable call), or workers may create privileged niches through the acquisition of new skills and abilities over time. Hospitalists' tendency to diversify their work‐related activities by incorporating administrative and teaching responsibilities[15] may thus contribute to improving job fit. Additionally, as a measure of complementarity among people who work together, job fit may be influenced by the quality of relationships among hospitalists and their coworkers through their reorientation to the prevailing organizational climate[16, 17] and increasing socialization.[18] Finally, given that experiential learning is known to contribute to better hospitalist work performance,[19] job fit may affect productivity and clinical outcomes vis‐‐vis quality of work life.
To test the validity of these assumptions in a sample of hospitalists, we critically appraised the following 4 hypotheses:
- Hypothesis 1 (H1): Job attrition and reselection improves job fit among hospitalists entering the job market.
- Hypothesis 2 (H2): Better job fit is achieved through hospitalists engaging a variety of personal skills and abilities.
- Hypothesis 3 (H3): Job fit increases with hospitalists' job duration together with socialization and internalization of organizational values.
- Hypothesis 4 (H4): Job fit is correlated with hospitalists' quality of work life.
METHODS
Analysis was performed on data from the 2009 to 2010 Hospital Medicine Physician Worklife Survey. The sample frame included nonmembers and members of Society of Hospital Medicine (SHM). Details about sampling strategy, data collection, and data quality are available in previous publications.[7, 20] The 118‐item survey instrument, including 9 demographic items and 24 practice and job characteristic items, was administered by mail. Examples of information solicited through these items included respondents' practice model, the number of hospitalist jobs they have held, and the specific kinds of clinical and nonclinical activities they performed as part of their current job.
We used a reliable but broad and generic measure of self‐perceived person‐job fit.[21] The survey items of the 5‐point Likert‐type scale anchored between strongly disagree and strongly agree were: I feel that my work utilizes my full abilities, I feel competent and fully able to handle my job, my job gives me the chance to do the things I feel I do best, I feel that my job and I are well‐matched, I feel I have adequate preparation for the job I now hold. The quality of hospitalists' relationships with physician colleagues, staff, and patients as well as job satisfaction was measured using scales adapted from the Physician Worklife Study.[22] Organizational climate was measured using an adapted scale from the Minimizing Error, Maximizing Outcome study incorporating 3 items from the cohesiveness subscale, 4 items from the organizational trust subscale, and 1 item from the quality emphasis subscale that were most pertinent to hospitalists' relationship with their organizations.[23] Intent to leave practice or reduce work hours was measured using 5 items from the Multi‐Center Hospitalist Survey Project.[24] Frequency of participation in suboptimal patient care was measured by adapting 3 items from the suboptimal reported practice subscale and 2 items from the suboptimal patient care subscale developed by Shanafelt et al.[25] Stress and job burnout were assessed using validated measures.[26, 27] Detailed descriptions of the response rate calculation and imputation of missing item data are available in previous publications.[7, 20]
Mean, variance, range, and skew were used to characterize the responses to the job fit survey scale. A table of respondent characteristics was constructed. A visual representation of job fit by individual hospitalist year in current practice was created, first, by plotting a locally weighted scatterplot smoothing curve to examine the shape of the general relationship, and second, by fitting a similarly contoured functional polynomial curve with 95% confidence intervals (CI) to a plot of the mean and interquartile range of job fit for each year in current practice. Spearman partial correlations were calculated for job fit and each of the 5 items addressing likelihood of leaving practice or reducing workload adjusted for gender to control for the higher proportion of women who plan to work part time. Median (interquartile range) job fit was calculated for categories defined by the number of job changes and compared with the reference category (no job change) using the nonparametric rank sum test for comparing non‐normally distributed data. Multivariate logistic regression models were used to calculate the odds ratio (OR) of participating in each of several clinical and nonclinical hospitalist activities between respondents whose job fit score was optimal (5 on a 5‐point scale) and less than optimal controlling for covariates that influence the likelihood of participating in these activities (years in current practice, practice model, and specialty training). A Spearman correlation matrix was created to assess interscale correlations among organizational parameters (years in current practice, job fit, organizational climate, and relationship with colleagues, staff, and patients). Finally, a separate Spearman correlation matrix was created to assess the interscale correlations among individual worker parameters (job fit, suboptimal patient care, job burnout, stress, and job satisfaction). Statistical significance was defined as P value <0.05, and all analyses were performed on Stata 11.0 (StataCorp, College Station, TX). The Northwestern University institutional review board approved this study.
RESULTS
Respondents included 816 hospitalists belonging to around 700 unique organizations. The adjusted response rate from the stratified sample was 26%. Respondents and nonrespondents were similar with regard to geographic region and model of practice, but respondents were more likely to be members of the SHM than nonrespondents. Panel A of Table 1 shows the demographic characteristics of the respondents. The mean age was 44.3 years, and about one‐third were women. The average hospitalist had about 7 years of experience in the specialty and about 5 years with their current hospitalist job. The majority were trained in internal medicine or one of its subspecialties, whereas pediatricians, family physicians, and physicians with other training made up the remainder.
Panel A | Panel B | ||
---|---|---|---|
Total | Assimilation Period Hospitalists | Advancement Period Hospitalists | |
| |||
Total, n | 816 | 103 | 713 |
Female, n (%) | 284 (35) | 37 (36) | 247 (35) |
Age, mean (SD) | 44.3 (9.0) | 41.9 (9.3) | 44.7 (8.9) |
Years postresidency experience as hospitalist, mean (SD) | 6.9 (4.5) | 4.3 (3.1) | 7.2 (4.6) |
Years in current practice, mean (SD) | 5.1 (3.9) | 0.9 (0.3) | 6.7 (3.8) |
Specialty training, n (%) | |||
Internal medicine | 555 (68) | 75 (73) | 480 (67) |
Pediatrics | 117 (14) | 8 (8) | 109 (15.3) |
Family medicine | 49 (6) | 7 (7) | 42 (6) |
Other | 95 (11) | 13 (13) | 82 (12) |
Job fit was highly skewed toward optimum fit, with a mean of 4.3 on a scale of 1 to 5, with a narrow standard deviation of 0.7. The poorest job fit was reported by 0.3%, whereas optimal fit was reported by 21% of respondents. Job fit plotted against years in current practice had a logarithmic appearance typical of learning curves (Figure 1). An inflection point was visualized at around 2 years. For the purposes of this article, we refer to hospitalists' experience in the first 2 years of a job as an assimilation period, which is marked by a steep increase in job fit early when rapid learning or attrition took place. The years beyond the inflection point are characterized as an advancement period, when a more attenuated rise in job fit was experienced with time. The Spearman correlation between job fit and years in practice during the advancement period was 0.145 (n = 678, P < 0.001). Panel B of Table 1 displays the characteristics of respondents separately for the assimilation and advancement cohorts. Assimilation hospitalists in our sample had a mean age of 41.9 years and mean on‐the‐job experience of 4.3 years, reflecting that many hospitalists in the first 2 years of a job have made at least 1 job change in the past.
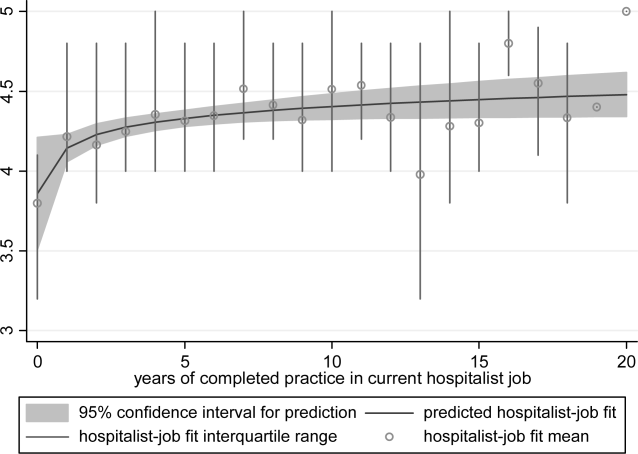
To show the effects of attrition and reselection, we first evaluated the proposition that hospitalists experience attrition (ie, intend to leave their jobs) in response to poor fit. Table 2 shows the correlations between job fit and the self‐reported intent to leave practice or reduce workload separately for the assimilation and advancement periods. For hospitalists in the assimilation period, job fit was negatively correlated with intent to leave current practice within 2 years and to leave hospital medicine within 5 years (P = 0.010 and 0.043, respectively). Hospitalists with <2 years in their current job, therefore, tended to consider attrition but not workload reduction to deal with poor job fit. On the other hand, hospitalists in the advancement period considered both attrition and workload reduction strategies in response to poor fit (all P < 0.001).
Assimilation Period Hospitalists | Advancement Period Hospitalists | |||
---|---|---|---|---|
Rho | P Value | Rho | P Value | |
Likelihood that a hospitalist will: | ||||
Leave current practice within 2 years | 0.253 | 0.010 | 0.367 | <0.001 |
Decrease total work hours within 5 years | 0.060 | 0.548 | 0.179 | <0.001 |
Decrease clinical work hours within 5 years | 0.072 | 0.469 | 0.144 | <0.001 |
Leave hospital medicine within 5 years | 0.200 | 0.043 | 0.231 | <0.001 |
Leave direct patient care within 5 years | 0.040 | 0.691 | 0.212 | <0.001 |
In Table 3, we further compared the median job fit across categories for the number of job switches. The median job fit during the assimilation period of hospitalists who had made 1 job change was slightly but statistically higher than the job fit of their counterparts who never left their first job (4.4 vs 4.0, P = 0.046). This suggests that job switching by hospitalists early in their jobs is associated with improved job fit (H1). However, the fit during the assimilation period of hospitalists who switched jobs twice or more was statistically no different from the fit of those in their first jobs, suggesting that the effect of the attrition‐reselection strategy is weak or inconsistent. The job fit for advancement period hospitalists was also different across the job change and no‐change categories. However, in the case of hospitalists later in their jobs, the median job fit was slightly but statistically lower among those who made job changes, revealing the potential drop in job fit that occurs when a hospitalist already established in his or her job starts over again in a new setting.
n | Age, Mean (95% CI), y | Hospitalist‐Job Fit, Median (IQR) | P Valuea | |
---|---|---|---|---|
| ||||
Assimilation period hospitalistsb | ||||
No job change | 29 | 42.3 (37.347.3) | 4.0 (3.84.4) | Reference |
1 job change | 39 | 40.3 (38.142.5) | 4.4 (4.04.8) | 0.046 |
2 or more job changes | 27 | 43.8 (41.046.6) | 4.4 (3.84.8) | 0.153 |
Advancement period hospitalistsc | ||||
No job change | 390 | 44.5 (43.645.5) | 4.6 (4.05.0) | Reference |
1 job change | 183 | 45.0 (43.746.3) | 4.2 (4.04.8) | 0.002 |
2 or more job changes | 99 | 44.9 (43.146.6) | 4.2 (3.84.8) | 0.002 |
We hypothesized that hospitalists who achieved high job fit within a particular job were more likely to have engaged in activities that utilize a wider spectrum of their abilities. As shown in Table 4, hospitalists in the highest quartile of job fit were associated with a general trend toward higher odds of participating in a variety of common clinical and nonclinical hospitalist activities, but only the odds ratio associated with teaching achieved statistical significance (OR: 1.53, 95% CI: 1.01‐2.31) (H2).
Participation, n/N (%) | Odds Ratio (95% CI) | P Value | |
---|---|---|---|
| |||
Administrative or committee work | 704/816 (86) | 0.73 (0.431.26) | 0.262 |
Quality improvement or patient safety initiatives | 678/816 (83) | 1.13 (0.642.00) | 0.680 |
Information technology design or implementation | 379/816 (46) | 1.18 (0.801.73) | 0.408 |
Any of the above leadership activities | 758/816 (93) | 1.31 (0.563.05) | 0.535 |
Teaching | 442/816 (54) | 1.53 (1.012.31) | 0.046 |
Research | 120/816 (15) | 1.07 (0.601.92) | 0.816 |
Any of the above academic activities | 457/816 (56) | 1.50 (0.992.27) | 0.057 |
Code team or rapid response team | 437/816 (54) | 1.13 (0.771.68) | 0.533 |
Intensive care unit | 254/816 (31) | 0.84 (0.531.35) | 0.469 |
Skilled nursing facility or long‐term acute care facility | 126/816 (15) | 1.06 (0.621.81) | 0.835 |
Outpatient general medical practice | 44/816 (5) | 1.75 (0.813.80) | 0.157 |
Any of the above clinical activities | 681/816 (79) | 1.02 (0.601.76) | 0.930 |
Socialization with peers and the gradual sharing of values within organizations are hypothesized mechanisms for increasing job fit with time. We found that the number of years in current practice was positively correlated with job fit (Spearman coefficient R = 0.149, P < 0.001), organizational climate (R = 0.128, P < 0.001), and relationship with nonphysician staff (R = 0.102, P < 0.01). The association between years in practice and relationship with physician colleagues were weaker (R = 0.079, P < 0.05). Consistent with the episodic nature of patients' encounters with hospitalists, the measure of patient relationships was not significantly associated with length of time in job. In addition, we found substantial correlations among job fit, organizational climate, and all the relational measures (all R > 0.280, P < 0.001), indicating that hospitalists increasingly share the values of their organizations over time (H3).
Finally, we also hypothesized that poor job fit is associated with poor performance and quality of work life. Strong correlations with job fit were noted for stress (R = 0.307, P < 0.001), job burnout (R = 0.360, P < 0.001), and job satisfaction (R = 0.570, P < 0.001). Job fit (R = 0.147, P < 0.001), job burnout (R = 0.236, P < 0.001), stress (R = 0.305, P < 0.001), and job satisfaction (R = 0.224, P < 0.001) were all significantly correlated with the frequency of participating in suboptimal care (H4).
DISCUSSION
In this exploratory analysis, we validated in the hospitalist workforce several assumptions about person‐job fit that have been observed in workers of other industries. We observed attrition‐reselection (ie, job switching) as a strategy used by physicians to achieve better fit early in their job tenure, whereas job modification appeared to be more effective than attrition‐reselection among physicians already established in their jobs. We provided weak but plausible evidence that physicians with optimal job fit had a tendency to participate in activities (eg, teaching) that engage a wider set of interests and abilities. We also demonstrated the growth in hospitalists sharing the values of their organization through the time‐dependent associations among organizational climate, relational measures, and job fit. Finally, we found that physicians with suboptimal job fit were more likely to report poor performance in their work compared to those indicating optimal fit.
Our previous analysis of data from the Hospital Medicine Physician Worklife Survey exposed the widely variable work characteristics of hospitalist jobs in the US market and the equally variable preferences and priorities of individual hospitalists in selecting their work setting.[7] The implication of our present study is that hospitalists achieve the high levels of observed job fit using various strategies that aid their alignment with their employment. One of these strategies involves time, but physician longevity in practice may be both a determinant and product of good job fit. Although early job attrition may be necessary for fitting the right hospitalists to the right jobs, employers may appreciate the importance of retaining experienced hospitalists not only for cost and performance considerations but also for the growth of social capital in organizations consisting of enduring individuals. As our data suggest that hospitalists grow with their jobs, physicians may experience better fit with jobs that flexibly couple their work demands with benefits that address their individual work‐life needs over time. Another implication of this study is that job fit is a useful and predictive measure of job selection, performance, and retention. In light of studies that expose the limitations of job satisfaction as a measure influenced more by workers' dispositional affect (ie, their temperament and outlook) than their compatibility with their jobs,[28] job fit may add a functional dimension to traditional employee feedback measures.
There are limitations to this analysis. The most notable is the low survey response rate. Two reasons contributed to the fairly low rate of return. First, the original sampling frame included many outdated addresses and names of individuals who did not meet inclusion criteria. Although all sampled individuals who would have been excluded from the study could not be identified, we calculated our response rate without accounting for the proportion of potential ineligibles in the denominator population [Response Rate 2 (RR2) according to standards of the American Association of Public Opinion Research].[29] Second, the response rates of physician surveys have seen a steady decline over the years.[30] Respondents to our survey may be older and more experienced than US hospitalists in general. Although concerns about bias from under‐reporting cannot be fully addressed, we believe that the study sample is adequate for this preliminary study intended to translate the evidence of observed phenomena from the nonphysician to the physician workforces. The suboptimal response characteristics (high skew and low variability) of the generic person‐job fit survey scale used in this study indicate that a reliable survey instrument specifically designed to measure physician‐job fit need to be constructed de novo and validated for any future study. Although we performed simple analyses to support our assertions, few of our subanalyses may be underpowered, contributing to overinterpretation of the data. Additional empirical work is also necessary to assess the generalizability of this study's claims in other medical and surgical specialties. Such studies would also allow measurement of the sensitivity and specificity of physicians' self‐identification of poor job fit. Finally, additional investigations of this time‐dependent construct are more appropriately performed using a longitudinal study design to overcome the limitations inherent in this cross‐sectional analysis. Our conclusions about the time‐dependent features of job fit may be explained by other characteristics such as generational and cultural differences among hospitalists with varying experience.
As the US healthcare system reorganizes to bolster accountability,[31] we anticipate increasing interdependence between physicians and their employer organizations. Ultimately, the desired accountability in healthcare is likely to be obtained if physicians function not only as passive and interchangeable employees but as active stakeholders in the achievement of each organization's goals. A methodology for assessing the alignment of physicians and their jobs will continue to be important along the way.
Disclosure
Nothing to report.
- Person‐organization fit: an integrative review of its conceptualizations, measurement, and implications. Personnel Psychol. 1996;49:1–49. .
- Consequences of individuals' fit at work: a meta‐analysis of person‐job, person‐organization, person‐group, and person‐supervisor fit. Personnel Psychol. 2005;58(2):281–342. , , .
- Person‐job fit: a conceptual integration, literature review and methodological critique. In: Cooper CL, Robertson IT, eds. International Review of Industrial and Organizational Psychology. Vol.6. New York, NY:John Wiley 1991. .
- Organizational culture, person‐culture fit, and turnover: a replication in the health care industry. J Organ Behav. 1999;20(2):175–184. .
- Organizational culture and physician satisfaction with dimensions of group practice. Health Serv Res. 2007;42(3 pt 1):1150–1176. , , , .
- Career fit and burnout among academic faculty. Arch Intern Med. 2009;169(10):990–995. , , , et al.
- Job characteristics, satisfaction, and burnout across hospitalist practice models. J Hosp Med. 2012;7(5):402–410. , , , , .
- Provider‐hospital “fit” and patient outcomes: evidence from Massachusetts cardiac surgeons, 2002–2004. Health Serv Res. 2011;46(1 pt 1):1–26. .
- The evolving primary care physician. N Engl J Med. 2012;366(20):1849–1853. .
- Hospitals' race to employ physicians—the logic behind a money‐losing proposition. N Engl J Med. 2011;364(19):1790–1793. , .
- 2011 Survey of Final‐Year Medical Residents. Irving, TX:Merritt Hawkins;2011.
- State of Hospital Medicine: 2010 Report Based on 2009 Data. Englewood, CO:Society of Hospital Medicine and the Medical Group Management Association;2010.
- The ASA framework: an update. Personnel Psychol. 1995;48(4):747–773. , , .
- Work Redesign. Reading. MA:Addison‐Wesley;1980. , .
- The expanding role of hospitalists in the United States. Swiss Med Wkly. 2006;136(37–38):591–596. , .
- Organizational socialization as a learning‐process—the role of information acquisition. Personnel Psychol. 1992;45(4):849–874. , .
- The moderating effect of tenure in person‐environment fit: a field study in educational organizations. J Occup Organ Psych. 1997;70:173–188. , .
- Matching people and organizations—selection and socialization in public accounting firms. Admin Sci Quart. 1991;36(3):459–484. .
- Effects of physician experience on costs and outcomes on an academic general medicine service: results of a trial of hospitalists. Ann Intern Med. 2002;137(11):866–874. , , , et al.
- Worklife and satisfaction of hospitalists: toward flourishing careers. J Gen Intern Med. 2012;27(1):28–36. , , , , .
- Karasek's model in the People's Republic of China: effects of job demands, control, and individual differences. Acad Manage J. 1996;39(6):1594–1618. .
- Measuring physician job satisfaction in a changing workplace and a challenging environment. SGIM Career Satisfaction Study Group. Society of General Internal Medicine. Med Care. 1999;37(11):1174–1182. , , , et al.
- Organizational climate, stress, and error in primary care: The MEMO Study. Adv Patient Saf. 2005;1:65–77. , , , et al.
- Effects of inpatient experience on outcomes and costs in a multicenter trial of academic hospitalists. J Gen Intern Med. 2005;20(suppl 1):141–142. , , , et al.
- Burnout and self‐reported patient care in an internal medicine residency program. Ann Intern Med. 2002;136(5):358–367. , , , .
- Effect of job demands and social support on worker stress—a study of VDT users. Behav Inform Technol. 1995;14(1):32–40. , .
- Validation of a single‐item measure of burnout against the Maslach Burnout Inventory among physicians. Stress Health. 2004;20(2):75–79. , , .
- Job satisfaction: a meta‐analysis of stabilities. J Organ Behav. 2001;22(5):483–504. , .
- The American Association for Public Opinion Research. Standard definitions: final dispositions of case codes and outcome rates for surveys.7th ed. Available at: http://www.aapor.org/Standard_Definitions2.htm. Accessed May 2,2012.
- Response rates and response bias for 50 surveys of pediatricians. Health Serv Res. 2005;40(1):213–226. , , , .
- Accountable care organizations: accountable for what, to whom, and how. JAMA. 2010;304(15):1715–6. , .
Person‐organization fit concerns the conditions and consequences of compatibility between people and the organizations for which they work.[1] Studies of other industries have demonstrated that person‐organization fit informs the way individuals join, perform in, and are retained by organizations.[2] Person‐job fit is a closely related subordinate concept that concerns the alignment of workers and their job in as much as workers have needs that their job supplies, or conversely, jobs have requirements that certain workers' abilities can help meet.[3] Explorations of job fit in physicians and their work have recently emerged in a few investigations published in medical journals.[4, 5, 6, 7, 8] Further expanding the understanding of fit between physicians and their employment is important, because the decline of solo practices and recent emphasis on team‐based care have led to a growing number of US physicians working in organizations.[9]
The movement of physicians into employed situations may continue if certain types of Accountable Care Organizations take root.[10] And physicians may be primed to join employer organizations based on current career priorities of individuals in American society. Surveys of medical residents entering the workforce reveal more physicians preferring the security of being employees than starting their own practices.[11] Given these trends, job fit will inform our understanding of how personal and job characteristics facilitate recruitment, performance, satisfaction, and longevity of physician employees.
BACKGROUND
Virtually all hospitalists work in organizationshospitalsand are employees of hospitals, medical schools, physician group practices, or management companies, and therefore invariably function within organizational structures and systems.[7] In spite of their rapid growth in numbers, many employers have faced difficulties recruiting and retaining enough hospitalists to fill their staffing needs. Consequently, the US hospitalist workforce today is characterized by high salaries, work load, and attrition rates.[12]
In this evolving unsaturated market, the attraction‐selection‐attrition framework[13] provides a theoretical construct that predicts that hospitalists and their employers would seek congruence of goals and values early in their relationship through a process of trial and error. This framework assumes that early interactions between workers and organizations serve as opportunities for them to understand if job fit is poor and dissociate or remain affiliated as long as job fit is mutually acceptable. Therefore, job switching on average is expected to increase job fit because workers and organizations gain a better understanding of their own goals and values and choose more wisely the next time.
Other theoretical frameworks, such as the job characteristic model,[14] suggest that over time as workers stay at the same job, they continue to maintain and improve job fit through various workplace‐ or self‐modification strategies. For example, seniority status may have privileges (eg, less undesirable call), or workers may create privileged niches through the acquisition of new skills and abilities over time. Hospitalists' tendency to diversify their work‐related activities by incorporating administrative and teaching responsibilities[15] may thus contribute to improving job fit. Additionally, as a measure of complementarity among people who work together, job fit may be influenced by the quality of relationships among hospitalists and their coworkers through their reorientation to the prevailing organizational climate[16, 17] and increasing socialization.[18] Finally, given that experiential learning is known to contribute to better hospitalist work performance,[19] job fit may affect productivity and clinical outcomes vis‐‐vis quality of work life.
To test the validity of these assumptions in a sample of hospitalists, we critically appraised the following 4 hypotheses:
- Hypothesis 1 (H1): Job attrition and reselection improves job fit among hospitalists entering the job market.
- Hypothesis 2 (H2): Better job fit is achieved through hospitalists engaging a variety of personal skills and abilities.
- Hypothesis 3 (H3): Job fit increases with hospitalists' job duration together with socialization and internalization of organizational values.
- Hypothesis 4 (H4): Job fit is correlated with hospitalists' quality of work life.
METHODS
Analysis was performed on data from the 2009 to 2010 Hospital Medicine Physician Worklife Survey. The sample frame included nonmembers and members of Society of Hospital Medicine (SHM). Details about sampling strategy, data collection, and data quality are available in previous publications.[7, 20] The 118‐item survey instrument, including 9 demographic items and 24 practice and job characteristic items, was administered by mail. Examples of information solicited through these items included respondents' practice model, the number of hospitalist jobs they have held, and the specific kinds of clinical and nonclinical activities they performed as part of their current job.
We used a reliable but broad and generic measure of self‐perceived person‐job fit.[21] The survey items of the 5‐point Likert‐type scale anchored between strongly disagree and strongly agree were: I feel that my work utilizes my full abilities, I feel competent and fully able to handle my job, my job gives me the chance to do the things I feel I do best, I feel that my job and I are well‐matched, I feel I have adequate preparation for the job I now hold. The quality of hospitalists' relationships with physician colleagues, staff, and patients as well as job satisfaction was measured using scales adapted from the Physician Worklife Study.[22] Organizational climate was measured using an adapted scale from the Minimizing Error, Maximizing Outcome study incorporating 3 items from the cohesiveness subscale, 4 items from the organizational trust subscale, and 1 item from the quality emphasis subscale that were most pertinent to hospitalists' relationship with their organizations.[23] Intent to leave practice or reduce work hours was measured using 5 items from the Multi‐Center Hospitalist Survey Project.[24] Frequency of participation in suboptimal patient care was measured by adapting 3 items from the suboptimal reported practice subscale and 2 items from the suboptimal patient care subscale developed by Shanafelt et al.[25] Stress and job burnout were assessed using validated measures.[26, 27] Detailed descriptions of the response rate calculation and imputation of missing item data are available in previous publications.[7, 20]
Mean, variance, range, and skew were used to characterize the responses to the job fit survey scale. A table of respondent characteristics was constructed. A visual representation of job fit by individual hospitalist year in current practice was created, first, by plotting a locally weighted scatterplot smoothing curve to examine the shape of the general relationship, and second, by fitting a similarly contoured functional polynomial curve with 95% confidence intervals (CI) to a plot of the mean and interquartile range of job fit for each year in current practice. Spearman partial correlations were calculated for job fit and each of the 5 items addressing likelihood of leaving practice or reducing workload adjusted for gender to control for the higher proportion of women who plan to work part time. Median (interquartile range) job fit was calculated for categories defined by the number of job changes and compared with the reference category (no job change) using the nonparametric rank sum test for comparing non‐normally distributed data. Multivariate logistic regression models were used to calculate the odds ratio (OR) of participating in each of several clinical and nonclinical hospitalist activities between respondents whose job fit score was optimal (5 on a 5‐point scale) and less than optimal controlling for covariates that influence the likelihood of participating in these activities (years in current practice, practice model, and specialty training). A Spearman correlation matrix was created to assess interscale correlations among organizational parameters (years in current practice, job fit, organizational climate, and relationship with colleagues, staff, and patients). Finally, a separate Spearman correlation matrix was created to assess the interscale correlations among individual worker parameters (job fit, suboptimal patient care, job burnout, stress, and job satisfaction). Statistical significance was defined as P value <0.05, and all analyses were performed on Stata 11.0 (StataCorp, College Station, TX). The Northwestern University institutional review board approved this study.
RESULTS
Respondents included 816 hospitalists belonging to around 700 unique organizations. The adjusted response rate from the stratified sample was 26%. Respondents and nonrespondents were similar with regard to geographic region and model of practice, but respondents were more likely to be members of the SHM than nonrespondents. Panel A of Table 1 shows the demographic characteristics of the respondents. The mean age was 44.3 years, and about one‐third were women. The average hospitalist had about 7 years of experience in the specialty and about 5 years with their current hospitalist job. The majority were trained in internal medicine or one of its subspecialties, whereas pediatricians, family physicians, and physicians with other training made up the remainder.
Panel A | Panel B | ||
---|---|---|---|
Total | Assimilation Period Hospitalists | Advancement Period Hospitalists | |
| |||
Total, n | 816 | 103 | 713 |
Female, n (%) | 284 (35) | 37 (36) | 247 (35) |
Age, mean (SD) | 44.3 (9.0) | 41.9 (9.3) | 44.7 (8.9) |
Years postresidency experience as hospitalist, mean (SD) | 6.9 (4.5) | 4.3 (3.1) | 7.2 (4.6) |
Years in current practice, mean (SD) | 5.1 (3.9) | 0.9 (0.3) | 6.7 (3.8) |
Specialty training, n (%) | |||
Internal medicine | 555 (68) | 75 (73) | 480 (67) |
Pediatrics | 117 (14) | 8 (8) | 109 (15.3) |
Family medicine | 49 (6) | 7 (7) | 42 (6) |
Other | 95 (11) | 13 (13) | 82 (12) |
Job fit was highly skewed toward optimum fit, with a mean of 4.3 on a scale of 1 to 5, with a narrow standard deviation of 0.7. The poorest job fit was reported by 0.3%, whereas optimal fit was reported by 21% of respondents. Job fit plotted against years in current practice had a logarithmic appearance typical of learning curves (Figure 1). An inflection point was visualized at around 2 years. For the purposes of this article, we refer to hospitalists' experience in the first 2 years of a job as an assimilation period, which is marked by a steep increase in job fit early when rapid learning or attrition took place. The years beyond the inflection point are characterized as an advancement period, when a more attenuated rise in job fit was experienced with time. The Spearman correlation between job fit and years in practice during the advancement period was 0.145 (n = 678, P < 0.001). Panel B of Table 1 displays the characteristics of respondents separately for the assimilation and advancement cohorts. Assimilation hospitalists in our sample had a mean age of 41.9 years and mean on‐the‐job experience of 4.3 years, reflecting that many hospitalists in the first 2 years of a job have made at least 1 job change in the past.
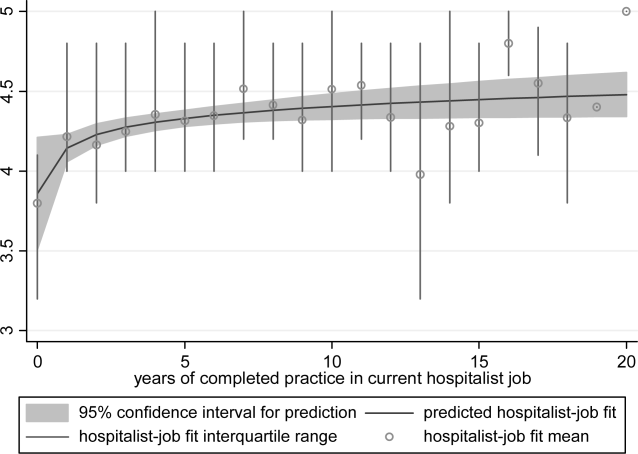
To show the effects of attrition and reselection, we first evaluated the proposition that hospitalists experience attrition (ie, intend to leave their jobs) in response to poor fit. Table 2 shows the correlations between job fit and the self‐reported intent to leave practice or reduce workload separately for the assimilation and advancement periods. For hospitalists in the assimilation period, job fit was negatively correlated with intent to leave current practice within 2 years and to leave hospital medicine within 5 years (P = 0.010 and 0.043, respectively). Hospitalists with <2 years in their current job, therefore, tended to consider attrition but not workload reduction to deal with poor job fit. On the other hand, hospitalists in the advancement period considered both attrition and workload reduction strategies in response to poor fit (all P < 0.001).
Assimilation Period Hospitalists | Advancement Period Hospitalists | |||
---|---|---|---|---|
Rho | P Value | Rho | P Value | |
Likelihood that a hospitalist will: | ||||
Leave current practice within 2 years | 0.253 | 0.010 | 0.367 | <0.001 |
Decrease total work hours within 5 years | 0.060 | 0.548 | 0.179 | <0.001 |
Decrease clinical work hours within 5 years | 0.072 | 0.469 | 0.144 | <0.001 |
Leave hospital medicine within 5 years | 0.200 | 0.043 | 0.231 | <0.001 |
Leave direct patient care within 5 years | 0.040 | 0.691 | 0.212 | <0.001 |
In Table 3, we further compared the median job fit across categories for the number of job switches. The median job fit during the assimilation period of hospitalists who had made 1 job change was slightly but statistically higher than the job fit of their counterparts who never left their first job (4.4 vs 4.0, P = 0.046). This suggests that job switching by hospitalists early in their jobs is associated with improved job fit (H1). However, the fit during the assimilation period of hospitalists who switched jobs twice or more was statistically no different from the fit of those in their first jobs, suggesting that the effect of the attrition‐reselection strategy is weak or inconsistent. The job fit for advancement period hospitalists was also different across the job change and no‐change categories. However, in the case of hospitalists later in their jobs, the median job fit was slightly but statistically lower among those who made job changes, revealing the potential drop in job fit that occurs when a hospitalist already established in his or her job starts over again in a new setting.
n | Age, Mean (95% CI), y | Hospitalist‐Job Fit, Median (IQR) | P Valuea | |
---|---|---|---|---|
| ||||
Assimilation period hospitalistsb | ||||
No job change | 29 | 42.3 (37.347.3) | 4.0 (3.84.4) | Reference |
1 job change | 39 | 40.3 (38.142.5) | 4.4 (4.04.8) | 0.046 |
2 or more job changes | 27 | 43.8 (41.046.6) | 4.4 (3.84.8) | 0.153 |
Advancement period hospitalistsc | ||||
No job change | 390 | 44.5 (43.645.5) | 4.6 (4.05.0) | Reference |
1 job change | 183 | 45.0 (43.746.3) | 4.2 (4.04.8) | 0.002 |
2 or more job changes | 99 | 44.9 (43.146.6) | 4.2 (3.84.8) | 0.002 |
We hypothesized that hospitalists who achieved high job fit within a particular job were more likely to have engaged in activities that utilize a wider spectrum of their abilities. As shown in Table 4, hospitalists in the highest quartile of job fit were associated with a general trend toward higher odds of participating in a variety of common clinical and nonclinical hospitalist activities, but only the odds ratio associated with teaching achieved statistical significance (OR: 1.53, 95% CI: 1.01‐2.31) (H2).
Participation, n/N (%) | Odds Ratio (95% CI) | P Value | |
---|---|---|---|
| |||
Administrative or committee work | 704/816 (86) | 0.73 (0.431.26) | 0.262 |
Quality improvement or patient safety initiatives | 678/816 (83) | 1.13 (0.642.00) | 0.680 |
Information technology design or implementation | 379/816 (46) | 1.18 (0.801.73) | 0.408 |
Any of the above leadership activities | 758/816 (93) | 1.31 (0.563.05) | 0.535 |
Teaching | 442/816 (54) | 1.53 (1.012.31) | 0.046 |
Research | 120/816 (15) | 1.07 (0.601.92) | 0.816 |
Any of the above academic activities | 457/816 (56) | 1.50 (0.992.27) | 0.057 |
Code team or rapid response team | 437/816 (54) | 1.13 (0.771.68) | 0.533 |
Intensive care unit | 254/816 (31) | 0.84 (0.531.35) | 0.469 |
Skilled nursing facility or long‐term acute care facility | 126/816 (15) | 1.06 (0.621.81) | 0.835 |
Outpatient general medical practice | 44/816 (5) | 1.75 (0.813.80) | 0.157 |
Any of the above clinical activities | 681/816 (79) | 1.02 (0.601.76) | 0.930 |
Socialization with peers and the gradual sharing of values within organizations are hypothesized mechanisms for increasing job fit with time. We found that the number of years in current practice was positively correlated with job fit (Spearman coefficient R = 0.149, P < 0.001), organizational climate (R = 0.128, P < 0.001), and relationship with nonphysician staff (R = 0.102, P < 0.01). The association between years in practice and relationship with physician colleagues were weaker (R = 0.079, P < 0.05). Consistent with the episodic nature of patients' encounters with hospitalists, the measure of patient relationships was not significantly associated with length of time in job. In addition, we found substantial correlations among job fit, organizational climate, and all the relational measures (all R > 0.280, P < 0.001), indicating that hospitalists increasingly share the values of their organizations over time (H3).
Finally, we also hypothesized that poor job fit is associated with poor performance and quality of work life. Strong correlations with job fit were noted for stress (R = 0.307, P < 0.001), job burnout (R = 0.360, P < 0.001), and job satisfaction (R = 0.570, P < 0.001). Job fit (R = 0.147, P < 0.001), job burnout (R = 0.236, P < 0.001), stress (R = 0.305, P < 0.001), and job satisfaction (R = 0.224, P < 0.001) were all significantly correlated with the frequency of participating in suboptimal care (H4).
DISCUSSION
In this exploratory analysis, we validated in the hospitalist workforce several assumptions about person‐job fit that have been observed in workers of other industries. We observed attrition‐reselection (ie, job switching) as a strategy used by physicians to achieve better fit early in their job tenure, whereas job modification appeared to be more effective than attrition‐reselection among physicians already established in their jobs. We provided weak but plausible evidence that physicians with optimal job fit had a tendency to participate in activities (eg, teaching) that engage a wider set of interests and abilities. We also demonstrated the growth in hospitalists sharing the values of their organization through the time‐dependent associations among organizational climate, relational measures, and job fit. Finally, we found that physicians with suboptimal job fit were more likely to report poor performance in their work compared to those indicating optimal fit.
Our previous analysis of data from the Hospital Medicine Physician Worklife Survey exposed the widely variable work characteristics of hospitalist jobs in the US market and the equally variable preferences and priorities of individual hospitalists in selecting their work setting.[7] The implication of our present study is that hospitalists achieve the high levels of observed job fit using various strategies that aid their alignment with their employment. One of these strategies involves time, but physician longevity in practice may be both a determinant and product of good job fit. Although early job attrition may be necessary for fitting the right hospitalists to the right jobs, employers may appreciate the importance of retaining experienced hospitalists not only for cost and performance considerations but also for the growth of social capital in organizations consisting of enduring individuals. As our data suggest that hospitalists grow with their jobs, physicians may experience better fit with jobs that flexibly couple their work demands with benefits that address their individual work‐life needs over time. Another implication of this study is that job fit is a useful and predictive measure of job selection, performance, and retention. In light of studies that expose the limitations of job satisfaction as a measure influenced more by workers' dispositional affect (ie, their temperament and outlook) than their compatibility with their jobs,[28] job fit may add a functional dimension to traditional employee feedback measures.
There are limitations to this analysis. The most notable is the low survey response rate. Two reasons contributed to the fairly low rate of return. First, the original sampling frame included many outdated addresses and names of individuals who did not meet inclusion criteria. Although all sampled individuals who would have been excluded from the study could not be identified, we calculated our response rate without accounting for the proportion of potential ineligibles in the denominator population [Response Rate 2 (RR2) according to standards of the American Association of Public Opinion Research].[29] Second, the response rates of physician surveys have seen a steady decline over the years.[30] Respondents to our survey may be older and more experienced than US hospitalists in general. Although concerns about bias from under‐reporting cannot be fully addressed, we believe that the study sample is adequate for this preliminary study intended to translate the evidence of observed phenomena from the nonphysician to the physician workforces. The suboptimal response characteristics (high skew and low variability) of the generic person‐job fit survey scale used in this study indicate that a reliable survey instrument specifically designed to measure physician‐job fit need to be constructed de novo and validated for any future study. Although we performed simple analyses to support our assertions, few of our subanalyses may be underpowered, contributing to overinterpretation of the data. Additional empirical work is also necessary to assess the generalizability of this study's claims in other medical and surgical specialties. Such studies would also allow measurement of the sensitivity and specificity of physicians' self‐identification of poor job fit. Finally, additional investigations of this time‐dependent construct are more appropriately performed using a longitudinal study design to overcome the limitations inherent in this cross‐sectional analysis. Our conclusions about the time‐dependent features of job fit may be explained by other characteristics such as generational and cultural differences among hospitalists with varying experience.
As the US healthcare system reorganizes to bolster accountability,[31] we anticipate increasing interdependence between physicians and their employer organizations. Ultimately, the desired accountability in healthcare is likely to be obtained if physicians function not only as passive and interchangeable employees but as active stakeholders in the achievement of each organization's goals. A methodology for assessing the alignment of physicians and their jobs will continue to be important along the way.
Disclosure
Nothing to report.
Person‐organization fit concerns the conditions and consequences of compatibility between people and the organizations for which they work.[1] Studies of other industries have demonstrated that person‐organization fit informs the way individuals join, perform in, and are retained by organizations.[2] Person‐job fit is a closely related subordinate concept that concerns the alignment of workers and their job in as much as workers have needs that their job supplies, or conversely, jobs have requirements that certain workers' abilities can help meet.[3] Explorations of job fit in physicians and their work have recently emerged in a few investigations published in medical journals.[4, 5, 6, 7, 8] Further expanding the understanding of fit between physicians and their employment is important, because the decline of solo practices and recent emphasis on team‐based care have led to a growing number of US physicians working in organizations.[9]
The movement of physicians into employed situations may continue if certain types of Accountable Care Organizations take root.[10] And physicians may be primed to join employer organizations based on current career priorities of individuals in American society. Surveys of medical residents entering the workforce reveal more physicians preferring the security of being employees than starting their own practices.[11] Given these trends, job fit will inform our understanding of how personal and job characteristics facilitate recruitment, performance, satisfaction, and longevity of physician employees.
BACKGROUND
Virtually all hospitalists work in organizationshospitalsand are employees of hospitals, medical schools, physician group practices, or management companies, and therefore invariably function within organizational structures and systems.[7] In spite of their rapid growth in numbers, many employers have faced difficulties recruiting and retaining enough hospitalists to fill their staffing needs. Consequently, the US hospitalist workforce today is characterized by high salaries, work load, and attrition rates.[12]
In this evolving unsaturated market, the attraction‐selection‐attrition framework[13] provides a theoretical construct that predicts that hospitalists and their employers would seek congruence of goals and values early in their relationship through a process of trial and error. This framework assumes that early interactions between workers and organizations serve as opportunities for them to understand if job fit is poor and dissociate or remain affiliated as long as job fit is mutually acceptable. Therefore, job switching on average is expected to increase job fit because workers and organizations gain a better understanding of their own goals and values and choose more wisely the next time.
Other theoretical frameworks, such as the job characteristic model,[14] suggest that over time as workers stay at the same job, they continue to maintain and improve job fit through various workplace‐ or self‐modification strategies. For example, seniority status may have privileges (eg, less undesirable call), or workers may create privileged niches through the acquisition of new skills and abilities over time. Hospitalists' tendency to diversify their work‐related activities by incorporating administrative and teaching responsibilities[15] may thus contribute to improving job fit. Additionally, as a measure of complementarity among people who work together, job fit may be influenced by the quality of relationships among hospitalists and their coworkers through their reorientation to the prevailing organizational climate[16, 17] and increasing socialization.[18] Finally, given that experiential learning is known to contribute to better hospitalist work performance,[19] job fit may affect productivity and clinical outcomes vis‐‐vis quality of work life.
To test the validity of these assumptions in a sample of hospitalists, we critically appraised the following 4 hypotheses:
- Hypothesis 1 (H1): Job attrition and reselection improves job fit among hospitalists entering the job market.
- Hypothesis 2 (H2): Better job fit is achieved through hospitalists engaging a variety of personal skills and abilities.
- Hypothesis 3 (H3): Job fit increases with hospitalists' job duration together with socialization and internalization of organizational values.
- Hypothesis 4 (H4): Job fit is correlated with hospitalists' quality of work life.
METHODS
Analysis was performed on data from the 2009 to 2010 Hospital Medicine Physician Worklife Survey. The sample frame included nonmembers and members of Society of Hospital Medicine (SHM). Details about sampling strategy, data collection, and data quality are available in previous publications.[7, 20] The 118‐item survey instrument, including 9 demographic items and 24 practice and job characteristic items, was administered by mail. Examples of information solicited through these items included respondents' practice model, the number of hospitalist jobs they have held, and the specific kinds of clinical and nonclinical activities they performed as part of their current job.
We used a reliable but broad and generic measure of self‐perceived person‐job fit.[21] The survey items of the 5‐point Likert‐type scale anchored between strongly disagree and strongly agree were: I feel that my work utilizes my full abilities, I feel competent and fully able to handle my job, my job gives me the chance to do the things I feel I do best, I feel that my job and I are well‐matched, I feel I have adequate preparation for the job I now hold. The quality of hospitalists' relationships with physician colleagues, staff, and patients as well as job satisfaction was measured using scales adapted from the Physician Worklife Study.[22] Organizational climate was measured using an adapted scale from the Minimizing Error, Maximizing Outcome study incorporating 3 items from the cohesiveness subscale, 4 items from the organizational trust subscale, and 1 item from the quality emphasis subscale that were most pertinent to hospitalists' relationship with their organizations.[23] Intent to leave practice or reduce work hours was measured using 5 items from the Multi‐Center Hospitalist Survey Project.[24] Frequency of participation in suboptimal patient care was measured by adapting 3 items from the suboptimal reported practice subscale and 2 items from the suboptimal patient care subscale developed by Shanafelt et al.[25] Stress and job burnout were assessed using validated measures.[26, 27] Detailed descriptions of the response rate calculation and imputation of missing item data are available in previous publications.[7, 20]
Mean, variance, range, and skew were used to characterize the responses to the job fit survey scale. A table of respondent characteristics was constructed. A visual representation of job fit by individual hospitalist year in current practice was created, first, by plotting a locally weighted scatterplot smoothing curve to examine the shape of the general relationship, and second, by fitting a similarly contoured functional polynomial curve with 95% confidence intervals (CI) to a plot of the mean and interquartile range of job fit for each year in current practice. Spearman partial correlations were calculated for job fit and each of the 5 items addressing likelihood of leaving practice or reducing workload adjusted for gender to control for the higher proportion of women who plan to work part time. Median (interquartile range) job fit was calculated for categories defined by the number of job changes and compared with the reference category (no job change) using the nonparametric rank sum test for comparing non‐normally distributed data. Multivariate logistic regression models were used to calculate the odds ratio (OR) of participating in each of several clinical and nonclinical hospitalist activities between respondents whose job fit score was optimal (5 on a 5‐point scale) and less than optimal controlling for covariates that influence the likelihood of participating in these activities (years in current practice, practice model, and specialty training). A Spearman correlation matrix was created to assess interscale correlations among organizational parameters (years in current practice, job fit, organizational climate, and relationship with colleagues, staff, and patients). Finally, a separate Spearman correlation matrix was created to assess the interscale correlations among individual worker parameters (job fit, suboptimal patient care, job burnout, stress, and job satisfaction). Statistical significance was defined as P value <0.05, and all analyses were performed on Stata 11.0 (StataCorp, College Station, TX). The Northwestern University institutional review board approved this study.
RESULTS
Respondents included 816 hospitalists belonging to around 700 unique organizations. The adjusted response rate from the stratified sample was 26%. Respondents and nonrespondents were similar with regard to geographic region and model of practice, but respondents were more likely to be members of the SHM than nonrespondents. Panel A of Table 1 shows the demographic characteristics of the respondents. The mean age was 44.3 years, and about one‐third were women. The average hospitalist had about 7 years of experience in the specialty and about 5 years with their current hospitalist job. The majority were trained in internal medicine or one of its subspecialties, whereas pediatricians, family physicians, and physicians with other training made up the remainder.
Panel A | Panel B | ||
---|---|---|---|
Total | Assimilation Period Hospitalists | Advancement Period Hospitalists | |
| |||
Total, n | 816 | 103 | 713 |
Female, n (%) | 284 (35) | 37 (36) | 247 (35) |
Age, mean (SD) | 44.3 (9.0) | 41.9 (9.3) | 44.7 (8.9) |
Years postresidency experience as hospitalist, mean (SD) | 6.9 (4.5) | 4.3 (3.1) | 7.2 (4.6) |
Years in current practice, mean (SD) | 5.1 (3.9) | 0.9 (0.3) | 6.7 (3.8) |
Specialty training, n (%) | |||
Internal medicine | 555 (68) | 75 (73) | 480 (67) |
Pediatrics | 117 (14) | 8 (8) | 109 (15.3) |
Family medicine | 49 (6) | 7 (7) | 42 (6) |
Other | 95 (11) | 13 (13) | 82 (12) |
Job fit was highly skewed toward optimum fit, with a mean of 4.3 on a scale of 1 to 5, with a narrow standard deviation of 0.7. The poorest job fit was reported by 0.3%, whereas optimal fit was reported by 21% of respondents. Job fit plotted against years in current practice had a logarithmic appearance typical of learning curves (Figure 1). An inflection point was visualized at around 2 years. For the purposes of this article, we refer to hospitalists' experience in the first 2 years of a job as an assimilation period, which is marked by a steep increase in job fit early when rapid learning or attrition took place. The years beyond the inflection point are characterized as an advancement period, when a more attenuated rise in job fit was experienced with time. The Spearman correlation between job fit and years in practice during the advancement period was 0.145 (n = 678, P < 0.001). Panel B of Table 1 displays the characteristics of respondents separately for the assimilation and advancement cohorts. Assimilation hospitalists in our sample had a mean age of 41.9 years and mean on‐the‐job experience of 4.3 years, reflecting that many hospitalists in the first 2 years of a job have made at least 1 job change in the past.
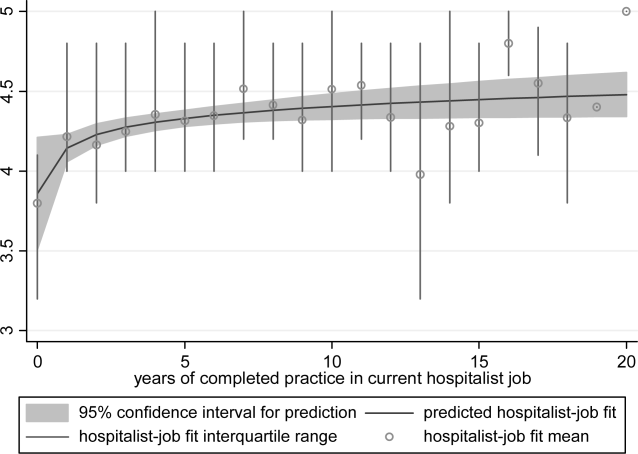
To show the effects of attrition and reselection, we first evaluated the proposition that hospitalists experience attrition (ie, intend to leave their jobs) in response to poor fit. Table 2 shows the correlations between job fit and the self‐reported intent to leave practice or reduce workload separately for the assimilation and advancement periods. For hospitalists in the assimilation period, job fit was negatively correlated with intent to leave current practice within 2 years and to leave hospital medicine within 5 years (P = 0.010 and 0.043, respectively). Hospitalists with <2 years in their current job, therefore, tended to consider attrition but not workload reduction to deal with poor job fit. On the other hand, hospitalists in the advancement period considered both attrition and workload reduction strategies in response to poor fit (all P < 0.001).
Assimilation Period Hospitalists | Advancement Period Hospitalists | |||
---|---|---|---|---|
Rho | P Value | Rho | P Value | |
Likelihood that a hospitalist will: | ||||
Leave current practice within 2 years | 0.253 | 0.010 | 0.367 | <0.001 |
Decrease total work hours within 5 years | 0.060 | 0.548 | 0.179 | <0.001 |
Decrease clinical work hours within 5 years | 0.072 | 0.469 | 0.144 | <0.001 |
Leave hospital medicine within 5 years | 0.200 | 0.043 | 0.231 | <0.001 |
Leave direct patient care within 5 years | 0.040 | 0.691 | 0.212 | <0.001 |
In Table 3, we further compared the median job fit across categories for the number of job switches. The median job fit during the assimilation period of hospitalists who had made 1 job change was slightly but statistically higher than the job fit of their counterparts who never left their first job (4.4 vs 4.0, P = 0.046). This suggests that job switching by hospitalists early in their jobs is associated with improved job fit (H1). However, the fit during the assimilation period of hospitalists who switched jobs twice or more was statistically no different from the fit of those in their first jobs, suggesting that the effect of the attrition‐reselection strategy is weak or inconsistent. The job fit for advancement period hospitalists was also different across the job change and no‐change categories. However, in the case of hospitalists later in their jobs, the median job fit was slightly but statistically lower among those who made job changes, revealing the potential drop in job fit that occurs when a hospitalist already established in his or her job starts over again in a new setting.
n | Age, Mean (95% CI), y | Hospitalist‐Job Fit, Median (IQR) | P Valuea | |
---|---|---|---|---|
| ||||
Assimilation period hospitalistsb | ||||
No job change | 29 | 42.3 (37.347.3) | 4.0 (3.84.4) | Reference |
1 job change | 39 | 40.3 (38.142.5) | 4.4 (4.04.8) | 0.046 |
2 or more job changes | 27 | 43.8 (41.046.6) | 4.4 (3.84.8) | 0.153 |
Advancement period hospitalistsc | ||||
No job change | 390 | 44.5 (43.645.5) | 4.6 (4.05.0) | Reference |
1 job change | 183 | 45.0 (43.746.3) | 4.2 (4.04.8) | 0.002 |
2 or more job changes | 99 | 44.9 (43.146.6) | 4.2 (3.84.8) | 0.002 |
We hypothesized that hospitalists who achieved high job fit within a particular job were more likely to have engaged in activities that utilize a wider spectrum of their abilities. As shown in Table 4, hospitalists in the highest quartile of job fit were associated with a general trend toward higher odds of participating in a variety of common clinical and nonclinical hospitalist activities, but only the odds ratio associated with teaching achieved statistical significance (OR: 1.53, 95% CI: 1.01‐2.31) (H2).
Participation, n/N (%) | Odds Ratio (95% CI) | P Value | |
---|---|---|---|
| |||
Administrative or committee work | 704/816 (86) | 0.73 (0.431.26) | 0.262 |
Quality improvement or patient safety initiatives | 678/816 (83) | 1.13 (0.642.00) | 0.680 |
Information technology design or implementation | 379/816 (46) | 1.18 (0.801.73) | 0.408 |
Any of the above leadership activities | 758/816 (93) | 1.31 (0.563.05) | 0.535 |
Teaching | 442/816 (54) | 1.53 (1.012.31) | 0.046 |
Research | 120/816 (15) | 1.07 (0.601.92) | 0.816 |
Any of the above academic activities | 457/816 (56) | 1.50 (0.992.27) | 0.057 |
Code team or rapid response team | 437/816 (54) | 1.13 (0.771.68) | 0.533 |
Intensive care unit | 254/816 (31) | 0.84 (0.531.35) | 0.469 |
Skilled nursing facility or long‐term acute care facility | 126/816 (15) | 1.06 (0.621.81) | 0.835 |
Outpatient general medical practice | 44/816 (5) | 1.75 (0.813.80) | 0.157 |
Any of the above clinical activities | 681/816 (79) | 1.02 (0.601.76) | 0.930 |
Socialization with peers and the gradual sharing of values within organizations are hypothesized mechanisms for increasing job fit with time. We found that the number of years in current practice was positively correlated with job fit (Spearman coefficient R = 0.149, P < 0.001), organizational climate (R = 0.128, P < 0.001), and relationship with nonphysician staff (R = 0.102, P < 0.01). The association between years in practice and relationship with physician colleagues were weaker (R = 0.079, P < 0.05). Consistent with the episodic nature of patients' encounters with hospitalists, the measure of patient relationships was not significantly associated with length of time in job. In addition, we found substantial correlations among job fit, organizational climate, and all the relational measures (all R > 0.280, P < 0.001), indicating that hospitalists increasingly share the values of their organizations over time (H3).
Finally, we also hypothesized that poor job fit is associated with poor performance and quality of work life. Strong correlations with job fit were noted for stress (R = 0.307, P < 0.001), job burnout (R = 0.360, P < 0.001), and job satisfaction (R = 0.570, P < 0.001). Job fit (R = 0.147, P < 0.001), job burnout (R = 0.236, P < 0.001), stress (R = 0.305, P < 0.001), and job satisfaction (R = 0.224, P < 0.001) were all significantly correlated with the frequency of participating in suboptimal care (H4).
DISCUSSION
In this exploratory analysis, we validated in the hospitalist workforce several assumptions about person‐job fit that have been observed in workers of other industries. We observed attrition‐reselection (ie, job switching) as a strategy used by physicians to achieve better fit early in their job tenure, whereas job modification appeared to be more effective than attrition‐reselection among physicians already established in their jobs. We provided weak but plausible evidence that physicians with optimal job fit had a tendency to participate in activities (eg, teaching) that engage a wider set of interests and abilities. We also demonstrated the growth in hospitalists sharing the values of their organization through the time‐dependent associations among organizational climate, relational measures, and job fit. Finally, we found that physicians with suboptimal job fit were more likely to report poor performance in their work compared to those indicating optimal fit.
Our previous analysis of data from the Hospital Medicine Physician Worklife Survey exposed the widely variable work characteristics of hospitalist jobs in the US market and the equally variable preferences and priorities of individual hospitalists in selecting their work setting.[7] The implication of our present study is that hospitalists achieve the high levels of observed job fit using various strategies that aid their alignment with their employment. One of these strategies involves time, but physician longevity in practice may be both a determinant and product of good job fit. Although early job attrition may be necessary for fitting the right hospitalists to the right jobs, employers may appreciate the importance of retaining experienced hospitalists not only for cost and performance considerations but also for the growth of social capital in organizations consisting of enduring individuals. As our data suggest that hospitalists grow with their jobs, physicians may experience better fit with jobs that flexibly couple their work demands with benefits that address their individual work‐life needs over time. Another implication of this study is that job fit is a useful and predictive measure of job selection, performance, and retention. In light of studies that expose the limitations of job satisfaction as a measure influenced more by workers' dispositional affect (ie, their temperament and outlook) than their compatibility with their jobs,[28] job fit may add a functional dimension to traditional employee feedback measures.
There are limitations to this analysis. The most notable is the low survey response rate. Two reasons contributed to the fairly low rate of return. First, the original sampling frame included many outdated addresses and names of individuals who did not meet inclusion criteria. Although all sampled individuals who would have been excluded from the study could not be identified, we calculated our response rate without accounting for the proportion of potential ineligibles in the denominator population [Response Rate 2 (RR2) according to standards of the American Association of Public Opinion Research].[29] Second, the response rates of physician surveys have seen a steady decline over the years.[30] Respondents to our survey may be older and more experienced than US hospitalists in general. Although concerns about bias from under‐reporting cannot be fully addressed, we believe that the study sample is adequate for this preliminary study intended to translate the evidence of observed phenomena from the nonphysician to the physician workforces. The suboptimal response characteristics (high skew and low variability) of the generic person‐job fit survey scale used in this study indicate that a reliable survey instrument specifically designed to measure physician‐job fit need to be constructed de novo and validated for any future study. Although we performed simple analyses to support our assertions, few of our subanalyses may be underpowered, contributing to overinterpretation of the data. Additional empirical work is also necessary to assess the generalizability of this study's claims in other medical and surgical specialties. Such studies would also allow measurement of the sensitivity and specificity of physicians' self‐identification of poor job fit. Finally, additional investigations of this time‐dependent construct are more appropriately performed using a longitudinal study design to overcome the limitations inherent in this cross‐sectional analysis. Our conclusions about the time‐dependent features of job fit may be explained by other characteristics such as generational and cultural differences among hospitalists with varying experience.
As the US healthcare system reorganizes to bolster accountability,[31] we anticipate increasing interdependence between physicians and their employer organizations. Ultimately, the desired accountability in healthcare is likely to be obtained if physicians function not only as passive and interchangeable employees but as active stakeholders in the achievement of each organization's goals. A methodology for assessing the alignment of physicians and their jobs will continue to be important along the way.
Disclosure
Nothing to report.
- Person‐organization fit: an integrative review of its conceptualizations, measurement, and implications. Personnel Psychol. 1996;49:1–49. .
- Consequences of individuals' fit at work: a meta‐analysis of person‐job, person‐organization, person‐group, and person‐supervisor fit. Personnel Psychol. 2005;58(2):281–342. , , .
- Person‐job fit: a conceptual integration, literature review and methodological critique. In: Cooper CL, Robertson IT, eds. International Review of Industrial and Organizational Psychology. Vol.6. New York, NY:John Wiley 1991. .
- Organizational culture, person‐culture fit, and turnover: a replication in the health care industry. J Organ Behav. 1999;20(2):175–184. .
- Organizational culture and physician satisfaction with dimensions of group practice. Health Serv Res. 2007;42(3 pt 1):1150–1176. , , , .
- Career fit and burnout among academic faculty. Arch Intern Med. 2009;169(10):990–995. , , , et al.
- Job characteristics, satisfaction, and burnout across hospitalist practice models. J Hosp Med. 2012;7(5):402–410. , , , , .
- Provider‐hospital “fit” and patient outcomes: evidence from Massachusetts cardiac surgeons, 2002–2004. Health Serv Res. 2011;46(1 pt 1):1–26. .
- The evolving primary care physician. N Engl J Med. 2012;366(20):1849–1853. .
- Hospitals' race to employ physicians—the logic behind a money‐losing proposition. N Engl J Med. 2011;364(19):1790–1793. , .
- 2011 Survey of Final‐Year Medical Residents. Irving, TX:Merritt Hawkins;2011.
- State of Hospital Medicine: 2010 Report Based on 2009 Data. Englewood, CO:Society of Hospital Medicine and the Medical Group Management Association;2010.
- The ASA framework: an update. Personnel Psychol. 1995;48(4):747–773. , , .
- Work Redesign. Reading. MA:Addison‐Wesley;1980. , .
- The expanding role of hospitalists in the United States. Swiss Med Wkly. 2006;136(37–38):591–596. , .
- Organizational socialization as a learning‐process—the role of information acquisition. Personnel Psychol. 1992;45(4):849–874. , .
- The moderating effect of tenure in person‐environment fit: a field study in educational organizations. J Occup Organ Psych. 1997;70:173–188. , .
- Matching people and organizations—selection and socialization in public accounting firms. Admin Sci Quart. 1991;36(3):459–484. .
- Effects of physician experience on costs and outcomes on an academic general medicine service: results of a trial of hospitalists. Ann Intern Med. 2002;137(11):866–874. , , , et al.
- Worklife and satisfaction of hospitalists: toward flourishing careers. J Gen Intern Med. 2012;27(1):28–36. , , , , .
- Karasek's model in the People's Republic of China: effects of job demands, control, and individual differences. Acad Manage J. 1996;39(6):1594–1618. .
- Measuring physician job satisfaction in a changing workplace and a challenging environment. SGIM Career Satisfaction Study Group. Society of General Internal Medicine. Med Care. 1999;37(11):1174–1182. , , , et al.
- Organizational climate, stress, and error in primary care: The MEMO Study. Adv Patient Saf. 2005;1:65–77. , , , et al.
- Effects of inpatient experience on outcomes and costs in a multicenter trial of academic hospitalists. J Gen Intern Med. 2005;20(suppl 1):141–142. , , , et al.
- Burnout and self‐reported patient care in an internal medicine residency program. Ann Intern Med. 2002;136(5):358–367. , , , .
- Effect of job demands and social support on worker stress—a study of VDT users. Behav Inform Technol. 1995;14(1):32–40. , .
- Validation of a single‐item measure of burnout against the Maslach Burnout Inventory among physicians. Stress Health. 2004;20(2):75–79. , , .
- Job satisfaction: a meta‐analysis of stabilities. J Organ Behav. 2001;22(5):483–504. , .
- The American Association for Public Opinion Research. Standard definitions: final dispositions of case codes and outcome rates for surveys.7th ed. Available at: http://www.aapor.org/Standard_Definitions2.htm. Accessed May 2,2012.
- Response rates and response bias for 50 surveys of pediatricians. Health Serv Res. 2005;40(1):213–226. , , , .
- Accountable care organizations: accountable for what, to whom, and how. JAMA. 2010;304(15):1715–6. , .
- Person‐organization fit: an integrative review of its conceptualizations, measurement, and implications. Personnel Psychol. 1996;49:1–49. .
- Consequences of individuals' fit at work: a meta‐analysis of person‐job, person‐organization, person‐group, and person‐supervisor fit. Personnel Psychol. 2005;58(2):281–342. , , .
- Person‐job fit: a conceptual integration, literature review and methodological critique. In: Cooper CL, Robertson IT, eds. International Review of Industrial and Organizational Psychology. Vol.6. New York, NY:John Wiley 1991. .
- Organizational culture, person‐culture fit, and turnover: a replication in the health care industry. J Organ Behav. 1999;20(2):175–184. .
- Organizational culture and physician satisfaction with dimensions of group practice. Health Serv Res. 2007;42(3 pt 1):1150–1176. , , , .
- Career fit and burnout among academic faculty. Arch Intern Med. 2009;169(10):990–995. , , , et al.
- Job characteristics, satisfaction, and burnout across hospitalist practice models. J Hosp Med. 2012;7(5):402–410. , , , , .
- Provider‐hospital “fit” and patient outcomes: evidence from Massachusetts cardiac surgeons, 2002–2004. Health Serv Res. 2011;46(1 pt 1):1–26. .
- The evolving primary care physician. N Engl J Med. 2012;366(20):1849–1853. .
- Hospitals' race to employ physicians—the logic behind a money‐losing proposition. N Engl J Med. 2011;364(19):1790–1793. , .
- 2011 Survey of Final‐Year Medical Residents. Irving, TX:Merritt Hawkins;2011.
- State of Hospital Medicine: 2010 Report Based on 2009 Data. Englewood, CO:Society of Hospital Medicine and the Medical Group Management Association;2010.
- The ASA framework: an update. Personnel Psychol. 1995;48(4):747–773. , , .
- Work Redesign. Reading. MA:Addison‐Wesley;1980. , .
- The expanding role of hospitalists in the United States. Swiss Med Wkly. 2006;136(37–38):591–596. , .
- Organizational socialization as a learning‐process—the role of information acquisition. Personnel Psychol. 1992;45(4):849–874. , .
- The moderating effect of tenure in person‐environment fit: a field study in educational organizations. J Occup Organ Psych. 1997;70:173–188. , .
- Matching people and organizations—selection and socialization in public accounting firms. Admin Sci Quart. 1991;36(3):459–484. .
- Effects of physician experience on costs and outcomes on an academic general medicine service: results of a trial of hospitalists. Ann Intern Med. 2002;137(11):866–874. , , , et al.
- Worklife and satisfaction of hospitalists: toward flourishing careers. J Gen Intern Med. 2012;27(1):28–36. , , , , .
- Karasek's model in the People's Republic of China: effects of job demands, control, and individual differences. Acad Manage J. 1996;39(6):1594–1618. .
- Measuring physician job satisfaction in a changing workplace and a challenging environment. SGIM Career Satisfaction Study Group. Society of General Internal Medicine. Med Care. 1999;37(11):1174–1182. , , , et al.
- Organizational climate, stress, and error in primary care: The MEMO Study. Adv Patient Saf. 2005;1:65–77. , , , et al.
- Effects of inpatient experience on outcomes and costs in a multicenter trial of academic hospitalists. J Gen Intern Med. 2005;20(suppl 1):141–142. , , , et al.
- Burnout and self‐reported patient care in an internal medicine residency program. Ann Intern Med. 2002;136(5):358–367. , , , .
- Effect of job demands and social support on worker stress—a study of VDT users. Behav Inform Technol. 1995;14(1):32–40. , .
- Validation of a single‐item measure of burnout against the Maslach Burnout Inventory among physicians. Stress Health. 2004;20(2):75–79. , , .
- Job satisfaction: a meta‐analysis of stabilities. J Organ Behav. 2001;22(5):483–504. , .
- The American Association for Public Opinion Research. Standard definitions: final dispositions of case codes and outcome rates for surveys.7th ed. Available at: http://www.aapor.org/Standard_Definitions2.htm. Accessed May 2,2012.
- Response rates and response bias for 50 surveys of pediatricians. Health Serv Res. 2005;40(1):213–226. , , , .
- Accountable care organizations: accountable for what, to whom, and how. JAMA. 2010;304(15):1715–6. , .
Copyright © 2012 Society of Hospital Medicine
Hospitalist Practice Models
Over the past 15 years, there has been dramatic growth in the number of hospitalist physicians in the United States and in the number of hospitals served by them.13 Hospitals are motivated to hire experienced hospitalists to staff their inpatient services,4 with goals that include obtaining cost‐savings and higher quality.59 The rapid growth of Hospital Medicine saw multiple types of hospital practice models emerge with differing job characteristics, clinical duties, workload, and compensation schemes.10 The extent of the variability of hospitalist jobs across practice models is not known.
Intensifying recruitment efforts and the concomitant increase in compensation for hospitalists over the last decade suggest that demand for hospitalists is strong and sustained.11 As a result, today's cohort of hospitalists has a wide range of choices of types of jobs, practice models, and locations. The diversity of available hospitalist jobs is characterized, for example, by setting (community hospital vs academic hospital), employer (hospital vs private practice), job duties (the amount and type of clinical work, and other administrative, teaching, or research duties), and intensity (work hours and duties to maximize income or lifestyle). How these choices relate to job satisfaction and burnout are also unknown.
The Society of Hospital Medicine (SHM) has administered surveys to hospitalist group leaders biennially since 2003.1215 These surveys, however, do not address issues related to individual hospitalist worklife, recruitment, and retention. In 2005, SHM convened a Career Satisfaction Task Force that designed and executed a national survey of hospitalists in 2009‐2010. The objective of this study is to evaluate how job characteristics vary by practice model, and the association of these characteristics and practice models with job satisfaction and burnout.
METHODS
Survey Instrument
A detailed description of the survey design, sampling strategy, data collection, and response rate calculations is described elsewhere.16 Portions of the 118‐item survey instrument assessed characteristics of the respondents' hospitalist group (12 items), details about their individual work patterns (12 items), and demographics (9 items). Work patterns were evaluated by the average number of clinical work days, consecutive days, hours per month, percentage of work assigned to night duty, and number of patient encounters. Average hours spent on nonclinical work, and the percentage of time allocated for clinical, administrative, teaching, and research activities were solicited. Additional items assessed specific clinical responsibilities, pretax earnings in FY2010, the availability of information technology capabilities, and the adequacy of available resources. Job and specialty satisfaction and 11 satisfaction domain measures were measured using validated scales.1726 Burnout symptoms were measured using a validated single‐item measure.26, 27
Sampling Strategy
We surveyed a national stratified sample of hospitalists in the US and Puerto Rico. We used the largest database of hospitalists (>24,000 names) currently available and maintained by the SHM as our sampling frame. We linked hospitalist employer information to hospital statistics from the American Hospital Association database28 to stratify the sample by number of hospital beds, geographic region, employment model, and specialty training, oversampling pediatric hospitalists due to small numbers. A respondent sample of about 700 hospitalists was calculated to be adequate to detect a 0.5 point difference in job satisfaction scores between subgroups assuming 90% power and alpha of 0.05. However, we sampled a total of 5389 addresses from the database to overcome the traditionally low physician response rates, duplicate sampling, bad addresses, and non‐hospitalists being included in the sampling frame. In addition, 2 multistate hospitalist companies (EmCare, In Compass Health) and 1 for‐profit hospital chain (HCA, Inc) financially sponsored this project with the stipulation that all of their hospitalist employees (n = 884) would be surveyed.
Data Collection
The healthcare consulting firm, Press Ganey, provided support with survey layout and administration following the modified Dillman method.29 Three rounds of coded surveys and solicitation letters from the investigators were mailed 2 weeks apart in November and December 2009. Because of low response rates to the mailed survey, an online survey was created using Survey Monkey and sent to 650 surveyees for whom e‐mail addresses were available, and administered at a kiosk for sample physicians during the SHM 2010 annual meeting.
Data Analysis
Nonresponse bias was measured by comparing characteristics between respondents of separate survey waves.30 We determined the validity of mailing addresses immediately following the survey period by mapping each address using Google, and if the address was a hospital, researching online whether or not the intended recipient was currently employed there. Practice characteristics were compared across 5 model categories distilled from the SHM & Medical Group Management Association survey: local hospitalist‐only group, multistate hospitalist group, multispecialty physician group, employer hospital, and university or medical school. Weighted proportions, means, and medians were calculated to account for oversampling of pediatric hospitalists. Differences in categorical measures were assessed using the chi‐square test and the design‐based F test for comparing weighted data. Weighted means (99% confidence intervals) and medians (interquartile ranges) were calculated. Because each parameter yielded a single outlier value across the 5 practice models, differences across weighted means were assessed using generalized linear models with the single outlier value chosen as the reference mean. Pair‐wise Wilcoxon rank sum test was used to compare median values. In these 4‐way comparisons of means and medians, significance was defined as P value of 0.0125 per Bonferroni correction. A single survey item solicited respondents to choose exactly 4 of 13 considerations most pertinent to job satisfaction. The proportion of respondents who scored 4 on a 5‐point Likert scale of the 11 satisfaction domains and 2 global measures of satisfaction, and burnout symptoms defined as 3 on a 5‐point single item measure were bar‐graphed. Chi‐square statistics were used to evaluate for differences across practice models. Statistical significance was defined by alpha less than 0.05, unless otherwise specified. All analyses were performed using STATA version 11.0 (College Station, TX). This study was approved by the Loyola University Institutional Review Board.
Survey data required cleaning prior to analysis. Missing gender information was imputed using the respondents' name. Responses to the item that asked to indicate the proportion of work dedicated to administrative responsibilities, clinical care, teaching, and research that did not add up to 100% were dropped. Two responses that indicated full‐time equivalent (FTE) of 0%, but whose respondents otherwise completed the survey implying they worked as clinical hospitalists, were replaced with values calculated from the given number of work hours relative to the median work hours in our sample. Out of range or implausible responses to the following items were dropped from analyses: the average number of billable encounters during a typical day or shift, number of shifts performing clinical activities during a typical month, pretax earnings, the year the respondent completed residency training, and the number of whole years practiced as a hospitalist. The proportion of selective item nonresponse was small and we did not, otherwise, impute missing data.
RESULTS
Response Rate
Of the 5389 originally sampled addresses, 1868 were undeliverable. Addresses were further excluded if they appeared in duplicate or were outdated. This yielded a total of 3105 eligible surveyees in the sample. As illustrated in Figure 1, 841 responded to the mailed survey and 5 responded to the Web‐based survey. After rejecting 67 non‐hospitalist respondents and 3 duplicate surveys, a total of 776 surveys were included in the final analysis. The adjusted response rate was 25.6% (776/3035). Members of SHM were more likely to return the survey than nonmembers. The adjusted response rate from hospitalists affiliated with the 3 sponsoring institutions was 6% (40/662). Because these respondents were more likely to be non‐members of SHM, we opted to analyze the responses from the sponsor hospitalists together with the sampled hospitalists. The demographics of the resulting pool of 816 respondents affiliated with over 650 unique hospitalist groups were representative of the original survey frame. We analyzed data from 794 of these who responded to the item indicating their hospitalist practice model. Demographic characteristics of responders and nonresponders to the practice model survey item were similar.
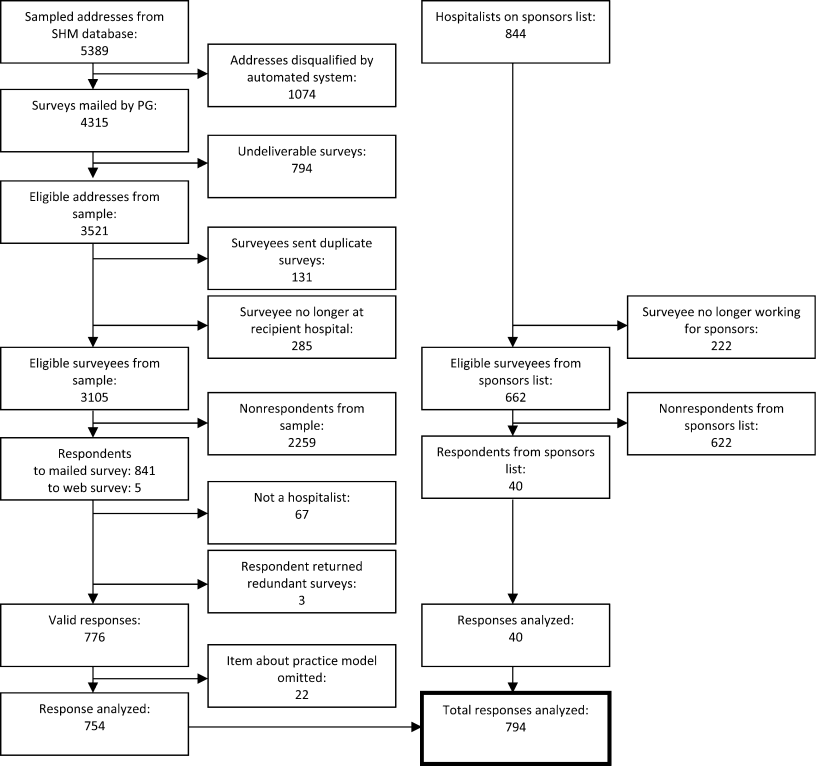
Characteristics of Hospitalists and Their Groups
Table 1 summarizes the characteristics of hospitalist respondents and their organizations by practice model. More (44%) respondents identified their practice model as directly employed by the hospital than other models, including multispecialty physician group (15%), multistate hospitalist group (14%), university or medical school (14%), local hospitalist group (12%), and other (2%). The median age of hospitalist respondents was 42 years, with 6.8 years of mean experience as a hospitalist. One third were women, 84% were married, and 46% had dependent children 6 years old or younger at home. Notably, hospitalists in multistate groups had fewer years of experience, and fewer hospitalists in local and multistate groups were married compared to hospitalists in other practice models.
Local Hospitalist‐Only Group | Multi‐State Hospitalist Group | Multispecialty Physician Group | Employer Hospital | University or Medical School | ||
---|---|---|---|---|---|---|
n = 95 | n = 111 | n = 115 | n = 348 | n = 107 | P Value | |
| ||||||
Hospitalist characteristics | ||||||
Age, weighted mean (99% CI) | 45 (42, 48) | 44 (42, 47) | 45 (43, 47) | 45 (43, 46) | 43 (40, 46) | |
Years hospitalist experience, weighted mean (99% CI) | 8 (6, 9)* | 5 (4, 6)* | 8 (7, 9) | 7 (6, 7) | 8 (6, 9) | <0.010* |
Women, weighted % | 29 | 30 | 39 | 31 | 43 | 0.118 |
Married, weighted % | 76 | 77 | 82 | 89 | 81 | 0.009 |
At least 1 dependent child younger than age 6 living in home, weighted % | 47 | 48 | 43 | 47 | 45 | 0.905 |
Pediatric specialty, n (%) | <10 | <10 | 11 (10%) | 57 (16%) | 36 (34%) | <0.001 |
Hospitalist group characteristics | ||||||
Region, weighted % | <0.001 | |||||
Northeast (AHA 1 & 2) | 13 | 10 | 16 | 27 | 13 | |
South (AHA 3 & 4) | 19 | 37 | 13 | 24 | 21 | |
Midwest (AHA 5 & 6) | 23 | 24 | 25 | 22 | 26 | |
Mountain (AHA 7 & 8) | 22 | 20 | 16 | 13 | 24 | |
West (AHA 9) | 24 | 10 | 31 | 14 | 16 | |
No. beds of primary hospital, weighted % | <0.001 | |||||
Up to 149 | 17 | 26 | 12 | 24 | 14 | |
150299 | 30 | 36 | 36 | 33 | 21 | |
300449 | 26 | 24 | 29 | 20 | 19 | |
450599 | 13 | 8 | 17 | 11 | 21 | |
600 or more | 12 | 6 | 7 | 13 | 24 | |
No. of hospital facilities served by current practice, weighted % | <0.001 | |||||
1 | 53 | 70 | 67 | 77 | 66 | |
2 | 20 | 22 | 20 | 16 | 24 | |
3 or more | 27 | 9 | 13 | 7 | 10 | |
No. of physicians in current practice, median (IQR) | 10 (5, 18) | 8 (6, 12)* | 14 (8, 25)* | 12 (6, 18) | 12 (7, 20) | <0.001*, 0.001 |
No. of non‐physician providers in current practice, median (IQR) | 0 (0, 2) | 0 (0, 2) | 0 (0, 3) | 1 (0, 2) | 0 (0, 2) | |
Available information technology capabilities, weighted % | ||||||
EHR to access physician notes | 57 | 57 | 75 | 58 | 79 | <0.001 |
EHR to access nursing documentations | 68 | 67 | 74 | 75 | 76 | 0.357 |
EHR to access laboratory or test results | 97 | 89 | 95 | 96 | 96 | 0.054 |
Electronic order entry | 30 | 19 | 53 | 38 | 56 | <0.001 |
Electronic billing | 38 | 31 | 36 | 36 | 38 | 0.818 |
Access to EHR at home or off site | 78 | 73 | 78 | 82 | 84 | 0.235 |
Access to Up‐to‐Date or other clinical guideline resources | 80 | 77 | 91 | 92 | 96 | <0.001 |
Access to schedules, calendars, or other organizational resources | 56 | 57 | 66 | 67 | 75 | 0.024 |
E‐mail, Web‐based paging, or other communication resources | 74 | 63 | 88 | 89 | 90 | <0.001 |
Several differences in respondent group characteristics by practice model were found. Respondents in multistate hospitalist groups were more likely from the South and Midwest, while respondents from multispecialty groups were likely from the West. More multistate group practices were based in smaller hospitals, while academic hospitalists tended to practice in hospitals with 600 or more beds. Respondents employed by hospitals were more likely to practice at 1 hospital facility only, while local group practices were more likely to practice at 3 or more facilities. The median number of physicians in a hospitalist group was 11 (interquartile range [IQR] 6, 19). Local and multistate groups had fewer hospitalists compared to other models. Nonphysician providers were employed by nearly half of all hospitalist practices. Although almost all groups had access to some information technology, more academic hospitalists had access to electronic order entry, electronic physician notes, electronic clinical guidelines resources and communication technology, while local and multistate groups were least likely to have access to these resources.
Work Pattern Variations
Table 2 further details hospitalist work hours by practice model. The majority of hospitalists (78%) reported their position was full‐time (FTE 1.0), while 13% reported working less than full‐time (FTE <1.0). Only 5% of local group hospitalists worked part‐time, while 20% of multispecialty group hospitalists did. An additional 9% reported FTE >1.0, indicating their work hours exceeded the definition of a full‐time physician in their practice. Among full‐time hospitalists, local group members worked a greater number of shifts per month than employees of multispecialty groups, hospitals, and academic medical centers. Academic hospitalists reported higher numbers of consecutive clinical days worked on average, but fewer night shifts compared to hospitalists employed by multistate groups, multispecialty groups, and hospitals; fewer billable encounters than hospitalists in local and multistate groups; and more nonclinical work hours than hospitalists of any other practice model. Academic hospitalists also spent more time on teaching and research than other practice models. Hospitalists spent 11%‐18% of their time on administrative and committee responsibilities, with the least amount spent by hospitalists in multistate groups and the most in academic practice.
Local Hospitalist‐Only Group | Multi‐State Hospitalist Group | Multispecialty Physician Group | Employer Hospital | University or Medical School | ||
---|---|---|---|---|---|---|
n = 95 | n = 111 | n = 115 | n = 348 | n = 107 | P Value | |
| ||||||
FTE, weighted % | 0.058 | |||||
FTE < 1.0 | 6 | 13 | 20 | 12 | 14 | |
FTE = 1.0 | 85 | 75 | 74 | 80 | 82 | |
FTE > 1.0 | 10 | 13 | 6 | 8 | 5 | |
Workload parameters, weighted mean (99% CI) | ||||||
Clinical shifts per month for FTE 1.0 | 19 (17, 20)* | 17 (16, 19) | 15 (14, 17)* | 16 (15, 16) | 15 (13, 17) | <0.001* |
Hours per clinical shift | 10 (9, 11) | 11 (10, 11)* | 10 (10, 11.0) | 11 (10, 11.0) | 10 (9, 10)* | 0.006*, 0.002 |
Consecutive days on clinical shift | 8 (6, 9) | 7 (6, 7)* | 6 (6, 7) | 7 (6, 7) | 9 (7, 10)* | 0.002*, <0.001 |
% Clinical shifts on nights | 20 (15, 25) | 23 (18, 28)* | 23 (17, 29) | 21 (17, 24) | 14 (9, 18)* | 0.001*, 0.002 |
% Night shifts spent in hospital | 61 (49, 74)* | 63 (52, 75) | 72 (62, 83) | 73 (67, 80) | 43 (29, 57)* | 0.010*, 0.003, <0.001 |
Billable encounters per clinical shift | 17 (14, 19)* | 17 (16, 18) | 14 (13, 15) | 15 (14, 16) | 13 (11, 14)* | <0.001*, 0.002 |
Hours nonclinical work per month | 23 (12, 34)* | 19 (11, 27) | 31 (20, 42) | 30 (24, 36) | 71 (55, 86)* | <0.001* |
Hours clinical and nonclinical work per month for FTE 1.0 | 202 (186, 219) | 211 (196, 226) | 184 (170, 198)* | 193 (186, 201) | 221 (203, 238)* | <0.001* |
Professional activity, weighted mean % (99% CI) | ||||||
Clinical | 84 (78, 89)* | 86 (81, 90) | 78 (72, 84) | 79 (76, 82) | 58 (51, 64)* | <0.001* |
Teaching | 2.3 (1, 5)* | 3 (1, 4) | 6 (4, 9) | 6 (5, 8) | 17 (14, 20)* | <0.001* |
Administration and Committee work | 13 (8, 19) | 11 (8, 15)* | 16 (10, 21) | 14 (12, 17) | 19 (14, 24)* | 0.001* |
Research | 0 (0, 0)* | 1 (0, 2) | 0 (0, 1) | 1 (0, 1) | 7 (3, 11)* | <0.001* |
Table 3 tabulates other work pattern characteristics. Most hospitalists indicated that their current clinical work as hospitalists involved the general medical wards (100%), medical consultations (98%), and comanagement with specialists (92%). There were wide differences in participation in comanagement (100%, local groups vs 71%, academic), intensive care unit (ICU) responsibilities (94%, multistate groups vs 27%, academic), and nursing home care (30%, local groups vs 8%, academic). Among activities that are potentially not reimbursable, academic hospitalists were less likely to participate in coordination of patient transfers and code or rapid response teams, while multistate groups were least likely to participate in quality improvement activities. In total, 99% of hospitalists reported participating in at least 1 potentially nonreimbursable clinical activity.
Local Hospitalist‐Only Group | Multi‐State Hospitalist Group | Multispecialty Physician Group | Employer Hospital | University or Medical School | ||
---|---|---|---|---|---|---|
n = 95 | n = 111 | n = 115 | n = 348 | n = 107 | P Value | |
| ||||||
Reimbursable activities, overlapping weighted % | ||||||
General medical ward | 100 | 99 | 100 | 99 | 99 | 0.809 |
Medical consultations | 99 | 99 | 100 | 98 | 95 | 0.043 |
Comanagement with specialists | 100 | 96 | 96 | 93 | 71 | <0.001 |
Preoperative evaluations | 92 | 92 | 90 | 88 | 77 | 0.002 |
Intensive care unit | 86 | 94 | 67 | 75 | 27 | <0.001 |
Skilled nursing facility or long‐term acute care facility | 30 | 19 | 12 | 16 | 8 | <0.001 |
Outpatient general medical practice | 4 | 4 | 5 | 5 | 10 | 0.241 |
Potentially nonreimbursable activities, overlapping weighted % | ||||||
Coordination of patient transfers | 92 | 94 | 95 | 93 | 82 | 0.005 |
Quality improvement or patient safety initiatives | 81 | 78 | 83 | 89 | 89 | 0.029 |
Code team or rapid response team | 56 | 57 | 53 | 62 | 37 | <0.001 |
Information technology design or implementation | 42 | 39 | 47 | 51 | 51 | 0.154 |
Admission triage for emergency department | 49 | 46 | 43 | 40 | 31 | 0.132 |
Compensation scheme, weighted % | <0.001 | |||||
Salary only | 18 | 21 | 30 | 29 | 47 | |
Salary plus performance incentive | 54 | 72 | 59 | 67 | 53 | |
Fee‐for‐service | 20 | 1 | 7 | 2 | 0 | |
Capitation | 0 | 0 | 0 | 0 | 0 | |
Other | 9 | 7 | 4 | 3 | 0 | |
Compensation links to incentives, overlapping weighted % | ||||||
No incentives | 40 | 28 | 29 | 29 | 48 | 0.003 |
Patient satisfaction | 23 | 39 | 38 | 38 | 14 | <0.001 |
Length of stay | 18 | 17 | 20 | 13 | 10 | 0.208 |
Overall cost | 8 | 11 | 9 | 5 | 6 | 0.270 |
Test utilization | 2 | 2 | 7 | 1 | 0 | <0.001 |
Clinical processes and outcomes | 26 | 34 | 44 | 43 | 24 | <0.001 |
Other | 17 | 29 | 26 | 31 | 25 | 0.087 |
Earnings, weighted mean dollars (99% CI) | 226,065 (202,891, 249,240)* | 225,613 (210,772, 240,454) | 202,617 (186,036, 219,198) | 206,087 (198,413, 213,460) | 166,478 (151,135, 181,821)* | <0.001* |
Hospitalist compensation schemes were significantly different across the practice models. Salary‐only schemes were most common among academic hospitalists (47%), while 72% of multistate groups used performance incentives in addition to salary. More local groups used fee‐for‐service compensation than other models. Incentives differed by practice model, with more multistate groups having incentives based on patient satisfaction, while more multispecialty physician groups had incentives based on clinical processes and outcomes than other models. Finally, mean earnings for academic hospitalists were significantly lower than for hospitalists of other practice models. Local and multistate group hospitalists earned more than any other practice model (all P <0.001), and $60,000 more than the lowest compensated academic hospitalists.
Components of Job Satisfaction
Hospitalists' rankings of the most important factors for job satisfaction revealed differences across models (Figure 2). Overall, hospitalists were most likely to consider optimal workload and compensation as important factors for job satisfaction from a list of 13 considerations. Local groups and academics were least likely to rank optimal workload as a top factor, and local group hospitalists were more likely to rank optimal autonomy than those of other models. Academic hospitalists had less concern for substantial pay, and more concern for the variety of tasks they perform and recognition by leaders, than other hospitalists.
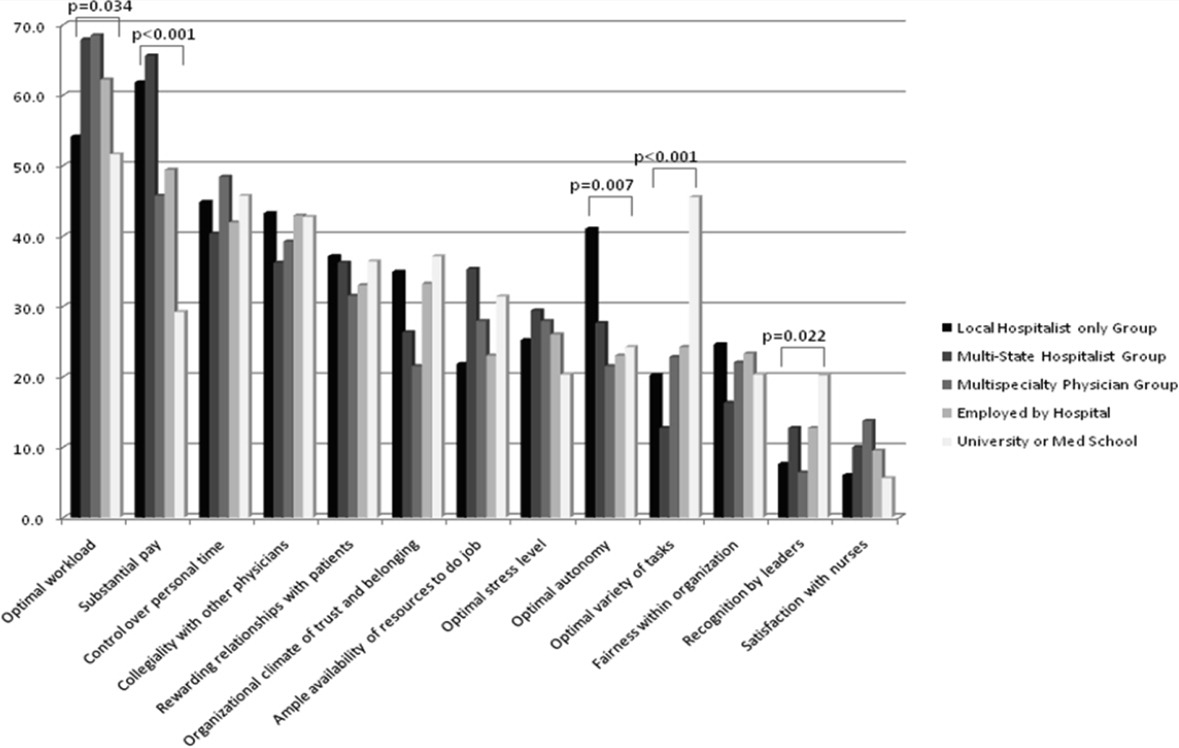
Job Satisfaction and Burnout Risk
Differences in the ratings of 4 of the 11 satisfaction and job characteristic domains were found across the practice models (Figure 3). Multispecialty group hospitalists were less satisfied with autonomy and their relationship with patients than other practice models, and along with multistate groups, reported the highest perceived workload. Organizational fairness was rated much higher by local group hospitalists than other practice models. Despite these differences in work patterns and satisfaction, there were no differences found in level of global job satisfaction, specialty satisfaction, or burnout across the practice models. Overall, 62% of respondents reported high job satisfaction (4 on a 1 to 5 scale), and 30% indicated burnout symptoms.
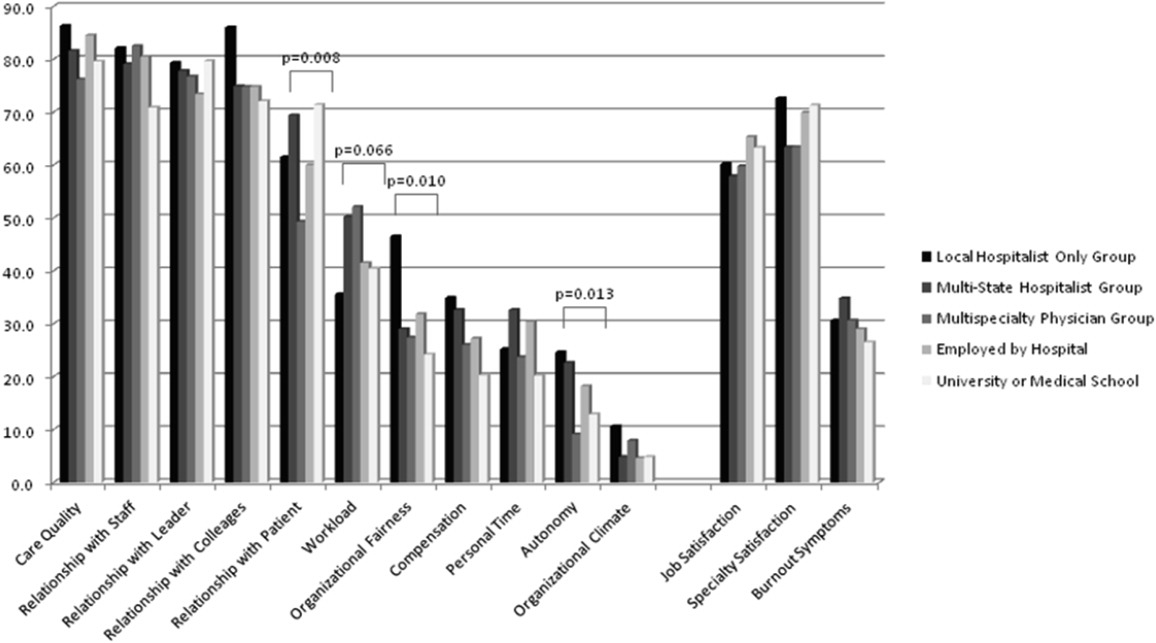
DISCUSSION
In our sample of US hospitalists, we found major differences in work patterns and compensation across hospitalist practice models, but no differences in job satisfaction, specialty satisfaction, and burnout. In particular, differences across these models included variations in hospitalist workload, hours, pay, and distribution of work activities. We found that hospitalists perform a variety of clinical and nonclinical tasks, for many of which there are not standard reimbursement mechanisms. We also found that features of a job that individual hospitalists considered most important vary by practice model.
Previous analysis of this data explored the overall state of hospitalist satisfaction.16 The present analysis offers a glimpse into hospitalists' systems‐orientation through a deeper look at their work patterns. The growth in the number of hospitalists who participate in intensive care medicine, specialty comanagement, and other work that involves close working relationships with specialist physicians confirms collaborative care as one of the dominant drivers of the hospitalist movement. At the level of indirect patient care, nearly all hospitalists contributed to work that facilitates coordination, quality, patient safety, or information technology. Understanding the integrative value of hospitalists outside of their clinical productivity may be of interest to hospital administrators.
Global satisfaction measures were similar across practice models. This finding is particularly interesting given the major differences in job characteristics seen among the practice models. This similarity in global satisfaction despite real differences in the nature of the job suggests that individuals find settings that allow them to address their individual professional goals. Our study demonstrates that, in 2010, Hospital Medicine has evolved enough to accommodate a wide variety of goals and needs.
While global satisfaction did not differ among practice types, hospitalists from various models did report differences in factors considered important to global satisfaction. While workload and pay were rated as influential across most models, the degree of importance was significantly different. In academic settings, substantial pay was not a top consideration for overall job satisfaction, whereas in local and multistate hospitalist groups, pay was a very close second in importance to optimal workload. These results may prove helpful for individual hospitalists trying to find their optimal job. For example, someone who is less concerned about workload, but wants to be paid well and have a high degree of autonomy, may find satisfaction in local hospitalist groups. However, for someone who is willing to sacrifice a higher salary for variety of activities, academic Hospital Medicine may be a better fit.
There is a concerning aspect of hospitalist job satisfaction that different practice models do not seem to solve. Control over personal time is a top consideration for many hospitalists across practice models, yet their satisfaction with personal time is low. As control over personal time is seen as a draw to the Hospital Medicine specialty, group leaders may need to evaluate their programs to ensure that schedules and workload support efforts for hospitalists to balance work and homelife commitments.
There are additional findings that are important for Hospital Medicine group leaders. Regardless of practice model, compensation and workload are often used as tools to recruit and retain hospitalists. While these tools may be effective, leaders may find more nuanced approaches to improving their hospitalists' overall satisfaction. Leaders of local hospitalist groups may find their hospitalists tolerant of heavier workloads as long as they are adequately rewarded and are given real autonomy over their work. However, leaders of academic programs may be missing the primary factor that can improve their hospitalists' satisfaction. Rather than asking for higher salaries to remain competitive, it may be more effective to advocate for time and training for their hospitalists to pursue important other activities beyond direct clinical care. Given that resources will always be limited, group leaders need to understand all of the elements that can contribute to hospitalist job satisfaction.
We point out several limitations to this study. First, our adjusted response rate of 25.6% is low for survey research, in general. As mentioned above, hospitalists are not easily identified in any available national physician database. Therefore, we deliberately designed our sampling strategy to error on the side of including ineligible surveyees to reduce systematic exclusion of practicing hospitalists. Using simple post hoc methods, we identified many nonhospitalists and bad addresses from our sample, but because these methods were exclusionary as opposed to confirmatory, we believe that a significant proportion of remaining nonrespondents may also have been ineligible for the survey. Although this does not fully address concerns about potential response bias, we believe that our sample representing a large number of hospitalist groups is adequate to make estimations about a nationally representative sample of practicing hospitalists. Second, in spite of our inclusive approach, we may still have excluded categories of practicing hospitalists. We were careful not to allow SHM members to represent all US hospitalists and included non‐members in the sampling frame, but the possibility of systematic exclusion that may alter our results remains a concern. Additionally, one of our goals was to characterize pediatric hospitalists independently from their adult‐patient counterparts. Despite oversampling of pediatricians, their sample was too small for a more detailed comparison across practice models. Also, self‐reported data about workload and compensation are subject to inaccuracies related to recall and cognitive biases. Last, this is a cross‐sectional study of hospitalist satisfaction at one point in time. Consequently, our sample may not be representative of very dissatisfied hospitalists who have already left their jobs.
The diversity found across existing practice models and the characteristics of the practices provide physicians with the opportunity to bring their unique skills and motivations to the hospitalist movement. As hospitals and other organizations seek to create, maintain, or grow hospitalist programs, the data provided here may prove useful to understand the relationship between practice characteristics and individual job satisfaction. Additionally, hospitalists looking for a job can consider these results as additional information to guide their choice of practice model and work patterns.
Acknowledgements
The authors thank Kenneth A. Rasinski for assistance with survey items refinement, and members of the SHM Career Satisfaction Task Force for their assistance in survey development.
- The status of hospital medicine groups in the United States.J Hosp Med.2006;1(2):75–80. , , , .
- Growth in the care of older patients by hospitalists in the United States.N Engl J Med.2009;360(11):1102–1112. , , , .
- The state of hospital medicine in 2008.Med Clin North Am.2008;92(2):265–273,vii. .
- Health care market trends and the evolution of hospitalist use and roles.J Gen Intern Med.2005;20(2):101–107. , , , .
- Effects of physician experience on costs and outcomes on an academic general medicine service: results of a trial of hospitalists.Ann Intern Med.2002;137(11):866–874. , , , et al.
- The Park Nicollet experience in establishing a hospitalist system.Ann Intern Med.1999;130(4 pt 2):350–354. .
- Effects of an HMO hospitalist program on inpatient utilization.Am J Manag Care.2001;7(11):1051–1057. , .
- The impact of hospitalists on the cost and quality of inpatient care in the United States: a research synthesis.Med Care Res Rev.2005;62(4):379–406. , .
- Pediatric hospitalists: a systematic review of the literature.Pediatrics.2006;117(5):1736–1744. , , , .
- The hospitalist movement 5 years later.JAMA.2002;287(4):487–494. , .
- Trends in market demand for internal medicine 1999 to 2004: an analysis of physician job advertisements.J Gen Intern Med.2006;21(10):1079–1085. , , , , , .
- SHM. 2003–2004 Survey by the Society of Hospital Medicine on Productivity and Compensation: Analysis of Results. 2004 [updated 2004]. Available at: http://www.hospitalmedicine.org/AM/Template. cfm?Section=Practice_Resources Available at: http://cme.medscape.com/viewarticle/578134. Accessed October 21,2010.
- State of Hospital Medicine: 2010 Report Based on 2009 Data.Englewood, CO and Philadelphia, PA:Medical Group Management Association and Society of Hospital Medicine;2010.
- Worklife and satisfaction of hospitalists: toward flourishing careers.J Gen Intern Med.2011, Jul 20. PMID: 21773849. , , , , .
- Worklife and satisfaction of general internists.Arch Intern Med.2002;162(6):649–656. , , , et al.
- Organizational climate, stress, and error in primary care: the MEMO study. In: Henriksen K, Battles JB, Marks ES, Lewin DI, eds.Advances in Patient Safety: From Research to Implementation. Vol 1: Research Findings.Rockville, MD:Agency for Healthcare Research and Quality;2005;1:65–77. , , , et al.
- Hospitalists and the practice of inpatient medicine: results of a survey of the National Association of Inpatient Physicians.Ann Intern Med.1999;130(4 pt 2):343–349. , , , .
- Physician attitudes toward and prevalence of the hospitalist model of care: results of a national survey.Am J Med.2000;109(8):648–653. , , , , , .
- Taking the Measure of Work: A Guide to Validated Scales for Organizational Research and Diagnosis.Thousand Oaks, CA:Sage Publications;2002. .
- Job Demands and Worker Health.Ann Arbor, MI:University of Michigan, Institute for Social Research;1980. , , , , .
- On the dimensionality of organizational justice: a construct validation of a measure.J Appl Psychol.2001;86(3):386–400. .
- Effect of job demands and social support on worker stress—a study of VDT users.Behav Inform Technol.1995;14(1):32–40. , .
- Measuring physician job satisfaction in a changing workplace and a challenging environment. SGIM Career Satisfaction Study Group. Society of General Internal Medicine.Med Care.1999;37(11):1174–1182. , , , et al.
- Working conditions in primary care: physician reactions and care quality.Ann Intern Med.2009;151(1):28–U48. , , , et al.
- Validation of a single‐item measure of burnout against the Maslach Burnout Inventory among physicians.Stress Health.2004;20(2):75–79. , , .
- American Hospital Association. AHA Hospital Statistics. 2009 [updated 2009]. Available at: http://www.ahadata.com/ahadata/html/AHAStatistics.html. Accessed April 12,2011.
- How to obtain excellent response rates when surveying physicians.Fam Pract.2009;26(1):65–68. , , , et al.
- Estimating nonresponse bias in mail surveys.J Marketing Res.1977;14(3):396–402. , .
Over the past 15 years, there has been dramatic growth in the number of hospitalist physicians in the United States and in the number of hospitals served by them.13 Hospitals are motivated to hire experienced hospitalists to staff their inpatient services,4 with goals that include obtaining cost‐savings and higher quality.59 The rapid growth of Hospital Medicine saw multiple types of hospital practice models emerge with differing job characteristics, clinical duties, workload, and compensation schemes.10 The extent of the variability of hospitalist jobs across practice models is not known.
Intensifying recruitment efforts and the concomitant increase in compensation for hospitalists over the last decade suggest that demand for hospitalists is strong and sustained.11 As a result, today's cohort of hospitalists has a wide range of choices of types of jobs, practice models, and locations. The diversity of available hospitalist jobs is characterized, for example, by setting (community hospital vs academic hospital), employer (hospital vs private practice), job duties (the amount and type of clinical work, and other administrative, teaching, or research duties), and intensity (work hours and duties to maximize income or lifestyle). How these choices relate to job satisfaction and burnout are also unknown.
The Society of Hospital Medicine (SHM) has administered surveys to hospitalist group leaders biennially since 2003.1215 These surveys, however, do not address issues related to individual hospitalist worklife, recruitment, and retention. In 2005, SHM convened a Career Satisfaction Task Force that designed and executed a national survey of hospitalists in 2009‐2010. The objective of this study is to evaluate how job characteristics vary by practice model, and the association of these characteristics and practice models with job satisfaction and burnout.
METHODS
Survey Instrument
A detailed description of the survey design, sampling strategy, data collection, and response rate calculations is described elsewhere.16 Portions of the 118‐item survey instrument assessed characteristics of the respondents' hospitalist group (12 items), details about their individual work patterns (12 items), and demographics (9 items). Work patterns were evaluated by the average number of clinical work days, consecutive days, hours per month, percentage of work assigned to night duty, and number of patient encounters. Average hours spent on nonclinical work, and the percentage of time allocated for clinical, administrative, teaching, and research activities were solicited. Additional items assessed specific clinical responsibilities, pretax earnings in FY2010, the availability of information technology capabilities, and the adequacy of available resources. Job and specialty satisfaction and 11 satisfaction domain measures were measured using validated scales.1726 Burnout symptoms were measured using a validated single‐item measure.26, 27
Sampling Strategy
We surveyed a national stratified sample of hospitalists in the US and Puerto Rico. We used the largest database of hospitalists (>24,000 names) currently available and maintained by the SHM as our sampling frame. We linked hospitalist employer information to hospital statistics from the American Hospital Association database28 to stratify the sample by number of hospital beds, geographic region, employment model, and specialty training, oversampling pediatric hospitalists due to small numbers. A respondent sample of about 700 hospitalists was calculated to be adequate to detect a 0.5 point difference in job satisfaction scores between subgroups assuming 90% power and alpha of 0.05. However, we sampled a total of 5389 addresses from the database to overcome the traditionally low physician response rates, duplicate sampling, bad addresses, and non‐hospitalists being included in the sampling frame. In addition, 2 multistate hospitalist companies (EmCare, In Compass Health) and 1 for‐profit hospital chain (HCA, Inc) financially sponsored this project with the stipulation that all of their hospitalist employees (n = 884) would be surveyed.
Data Collection
The healthcare consulting firm, Press Ganey, provided support with survey layout and administration following the modified Dillman method.29 Three rounds of coded surveys and solicitation letters from the investigators were mailed 2 weeks apart in November and December 2009. Because of low response rates to the mailed survey, an online survey was created using Survey Monkey and sent to 650 surveyees for whom e‐mail addresses were available, and administered at a kiosk for sample physicians during the SHM 2010 annual meeting.
Data Analysis
Nonresponse bias was measured by comparing characteristics between respondents of separate survey waves.30 We determined the validity of mailing addresses immediately following the survey period by mapping each address using Google, and if the address was a hospital, researching online whether or not the intended recipient was currently employed there. Practice characteristics were compared across 5 model categories distilled from the SHM & Medical Group Management Association survey: local hospitalist‐only group, multistate hospitalist group, multispecialty physician group, employer hospital, and university or medical school. Weighted proportions, means, and medians were calculated to account for oversampling of pediatric hospitalists. Differences in categorical measures were assessed using the chi‐square test and the design‐based F test for comparing weighted data. Weighted means (99% confidence intervals) and medians (interquartile ranges) were calculated. Because each parameter yielded a single outlier value across the 5 practice models, differences across weighted means were assessed using generalized linear models with the single outlier value chosen as the reference mean. Pair‐wise Wilcoxon rank sum test was used to compare median values. In these 4‐way comparisons of means and medians, significance was defined as P value of 0.0125 per Bonferroni correction. A single survey item solicited respondents to choose exactly 4 of 13 considerations most pertinent to job satisfaction. The proportion of respondents who scored 4 on a 5‐point Likert scale of the 11 satisfaction domains and 2 global measures of satisfaction, and burnout symptoms defined as 3 on a 5‐point single item measure were bar‐graphed. Chi‐square statistics were used to evaluate for differences across practice models. Statistical significance was defined by alpha less than 0.05, unless otherwise specified. All analyses were performed using STATA version 11.0 (College Station, TX). This study was approved by the Loyola University Institutional Review Board.
Survey data required cleaning prior to analysis. Missing gender information was imputed using the respondents' name. Responses to the item that asked to indicate the proportion of work dedicated to administrative responsibilities, clinical care, teaching, and research that did not add up to 100% were dropped. Two responses that indicated full‐time equivalent (FTE) of 0%, but whose respondents otherwise completed the survey implying they worked as clinical hospitalists, were replaced with values calculated from the given number of work hours relative to the median work hours in our sample. Out of range or implausible responses to the following items were dropped from analyses: the average number of billable encounters during a typical day or shift, number of shifts performing clinical activities during a typical month, pretax earnings, the year the respondent completed residency training, and the number of whole years practiced as a hospitalist. The proportion of selective item nonresponse was small and we did not, otherwise, impute missing data.
RESULTS
Response Rate
Of the 5389 originally sampled addresses, 1868 were undeliverable. Addresses were further excluded if they appeared in duplicate or were outdated. This yielded a total of 3105 eligible surveyees in the sample. As illustrated in Figure 1, 841 responded to the mailed survey and 5 responded to the Web‐based survey. After rejecting 67 non‐hospitalist respondents and 3 duplicate surveys, a total of 776 surveys were included in the final analysis. The adjusted response rate was 25.6% (776/3035). Members of SHM were more likely to return the survey than nonmembers. The adjusted response rate from hospitalists affiliated with the 3 sponsoring institutions was 6% (40/662). Because these respondents were more likely to be non‐members of SHM, we opted to analyze the responses from the sponsor hospitalists together with the sampled hospitalists. The demographics of the resulting pool of 816 respondents affiliated with over 650 unique hospitalist groups were representative of the original survey frame. We analyzed data from 794 of these who responded to the item indicating their hospitalist practice model. Demographic characteristics of responders and nonresponders to the practice model survey item were similar.
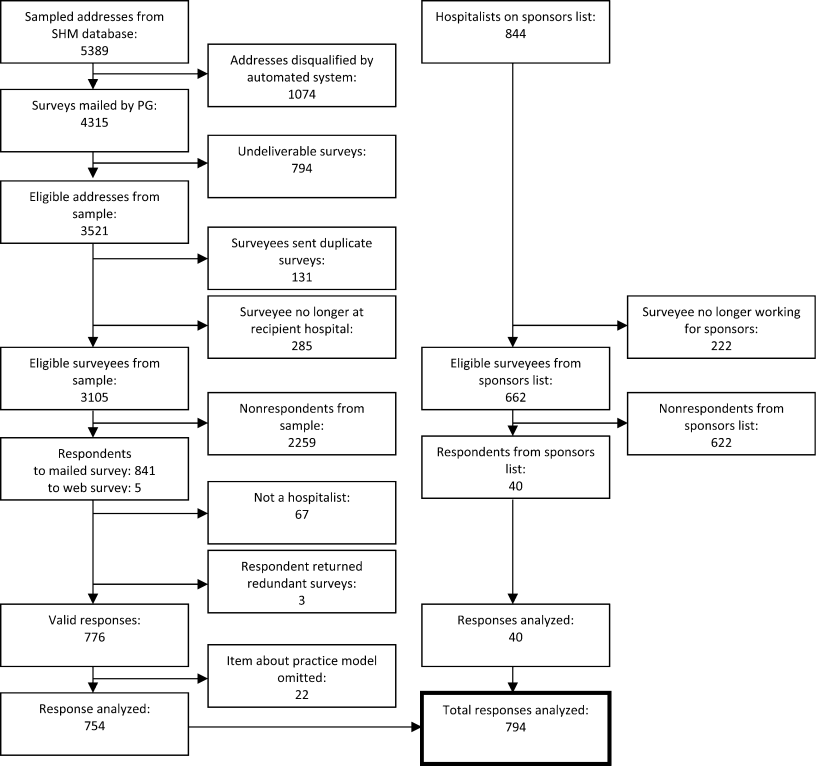
Characteristics of Hospitalists and Their Groups
Table 1 summarizes the characteristics of hospitalist respondents and their organizations by practice model. More (44%) respondents identified their practice model as directly employed by the hospital than other models, including multispecialty physician group (15%), multistate hospitalist group (14%), university or medical school (14%), local hospitalist group (12%), and other (2%). The median age of hospitalist respondents was 42 years, with 6.8 years of mean experience as a hospitalist. One third were women, 84% were married, and 46% had dependent children 6 years old or younger at home. Notably, hospitalists in multistate groups had fewer years of experience, and fewer hospitalists in local and multistate groups were married compared to hospitalists in other practice models.
Local Hospitalist‐Only Group | Multi‐State Hospitalist Group | Multispecialty Physician Group | Employer Hospital | University or Medical School | ||
---|---|---|---|---|---|---|
n = 95 | n = 111 | n = 115 | n = 348 | n = 107 | P Value | |
| ||||||
Hospitalist characteristics | ||||||
Age, weighted mean (99% CI) | 45 (42, 48) | 44 (42, 47) | 45 (43, 47) | 45 (43, 46) | 43 (40, 46) | |
Years hospitalist experience, weighted mean (99% CI) | 8 (6, 9)* | 5 (4, 6)* | 8 (7, 9) | 7 (6, 7) | 8 (6, 9) | <0.010* |
Women, weighted % | 29 | 30 | 39 | 31 | 43 | 0.118 |
Married, weighted % | 76 | 77 | 82 | 89 | 81 | 0.009 |
At least 1 dependent child younger than age 6 living in home, weighted % | 47 | 48 | 43 | 47 | 45 | 0.905 |
Pediatric specialty, n (%) | <10 | <10 | 11 (10%) | 57 (16%) | 36 (34%) | <0.001 |
Hospitalist group characteristics | ||||||
Region, weighted % | <0.001 | |||||
Northeast (AHA 1 & 2) | 13 | 10 | 16 | 27 | 13 | |
South (AHA 3 & 4) | 19 | 37 | 13 | 24 | 21 | |
Midwest (AHA 5 & 6) | 23 | 24 | 25 | 22 | 26 | |
Mountain (AHA 7 & 8) | 22 | 20 | 16 | 13 | 24 | |
West (AHA 9) | 24 | 10 | 31 | 14 | 16 | |
No. beds of primary hospital, weighted % | <0.001 | |||||
Up to 149 | 17 | 26 | 12 | 24 | 14 | |
150299 | 30 | 36 | 36 | 33 | 21 | |
300449 | 26 | 24 | 29 | 20 | 19 | |
450599 | 13 | 8 | 17 | 11 | 21 | |
600 or more | 12 | 6 | 7 | 13 | 24 | |
No. of hospital facilities served by current practice, weighted % | <0.001 | |||||
1 | 53 | 70 | 67 | 77 | 66 | |
2 | 20 | 22 | 20 | 16 | 24 | |
3 or more | 27 | 9 | 13 | 7 | 10 | |
No. of physicians in current practice, median (IQR) | 10 (5, 18) | 8 (6, 12)* | 14 (8, 25)* | 12 (6, 18) | 12 (7, 20) | <0.001*, 0.001 |
No. of non‐physician providers in current practice, median (IQR) | 0 (0, 2) | 0 (0, 2) | 0 (0, 3) | 1 (0, 2) | 0 (0, 2) | |
Available information technology capabilities, weighted % | ||||||
EHR to access physician notes | 57 | 57 | 75 | 58 | 79 | <0.001 |
EHR to access nursing documentations | 68 | 67 | 74 | 75 | 76 | 0.357 |
EHR to access laboratory or test results | 97 | 89 | 95 | 96 | 96 | 0.054 |
Electronic order entry | 30 | 19 | 53 | 38 | 56 | <0.001 |
Electronic billing | 38 | 31 | 36 | 36 | 38 | 0.818 |
Access to EHR at home or off site | 78 | 73 | 78 | 82 | 84 | 0.235 |
Access to Up‐to‐Date or other clinical guideline resources | 80 | 77 | 91 | 92 | 96 | <0.001 |
Access to schedules, calendars, or other organizational resources | 56 | 57 | 66 | 67 | 75 | 0.024 |
E‐mail, Web‐based paging, or other communication resources | 74 | 63 | 88 | 89 | 90 | <0.001 |
Several differences in respondent group characteristics by practice model were found. Respondents in multistate hospitalist groups were more likely from the South and Midwest, while respondents from multispecialty groups were likely from the West. More multistate group practices were based in smaller hospitals, while academic hospitalists tended to practice in hospitals with 600 or more beds. Respondents employed by hospitals were more likely to practice at 1 hospital facility only, while local group practices were more likely to practice at 3 or more facilities. The median number of physicians in a hospitalist group was 11 (interquartile range [IQR] 6, 19). Local and multistate groups had fewer hospitalists compared to other models. Nonphysician providers were employed by nearly half of all hospitalist practices. Although almost all groups had access to some information technology, more academic hospitalists had access to electronic order entry, electronic physician notes, electronic clinical guidelines resources and communication technology, while local and multistate groups were least likely to have access to these resources.
Work Pattern Variations
Table 2 further details hospitalist work hours by practice model. The majority of hospitalists (78%) reported their position was full‐time (FTE 1.0), while 13% reported working less than full‐time (FTE <1.0). Only 5% of local group hospitalists worked part‐time, while 20% of multispecialty group hospitalists did. An additional 9% reported FTE >1.0, indicating their work hours exceeded the definition of a full‐time physician in their practice. Among full‐time hospitalists, local group members worked a greater number of shifts per month than employees of multispecialty groups, hospitals, and academic medical centers. Academic hospitalists reported higher numbers of consecutive clinical days worked on average, but fewer night shifts compared to hospitalists employed by multistate groups, multispecialty groups, and hospitals; fewer billable encounters than hospitalists in local and multistate groups; and more nonclinical work hours than hospitalists of any other practice model. Academic hospitalists also spent more time on teaching and research than other practice models. Hospitalists spent 11%‐18% of their time on administrative and committee responsibilities, with the least amount spent by hospitalists in multistate groups and the most in academic practice.
Local Hospitalist‐Only Group | Multi‐State Hospitalist Group | Multispecialty Physician Group | Employer Hospital | University or Medical School | ||
---|---|---|---|---|---|---|
n = 95 | n = 111 | n = 115 | n = 348 | n = 107 | P Value | |
| ||||||
FTE, weighted % | 0.058 | |||||
FTE < 1.0 | 6 | 13 | 20 | 12 | 14 | |
FTE = 1.0 | 85 | 75 | 74 | 80 | 82 | |
FTE > 1.0 | 10 | 13 | 6 | 8 | 5 | |
Workload parameters, weighted mean (99% CI) | ||||||
Clinical shifts per month for FTE 1.0 | 19 (17, 20)* | 17 (16, 19) | 15 (14, 17)* | 16 (15, 16) | 15 (13, 17) | <0.001* |
Hours per clinical shift | 10 (9, 11) | 11 (10, 11)* | 10 (10, 11.0) | 11 (10, 11.0) | 10 (9, 10)* | 0.006*, 0.002 |
Consecutive days on clinical shift | 8 (6, 9) | 7 (6, 7)* | 6 (6, 7) | 7 (6, 7) | 9 (7, 10)* | 0.002*, <0.001 |
% Clinical shifts on nights | 20 (15, 25) | 23 (18, 28)* | 23 (17, 29) | 21 (17, 24) | 14 (9, 18)* | 0.001*, 0.002 |
% Night shifts spent in hospital | 61 (49, 74)* | 63 (52, 75) | 72 (62, 83) | 73 (67, 80) | 43 (29, 57)* | 0.010*, 0.003, <0.001 |
Billable encounters per clinical shift | 17 (14, 19)* | 17 (16, 18) | 14 (13, 15) | 15 (14, 16) | 13 (11, 14)* | <0.001*, 0.002 |
Hours nonclinical work per month | 23 (12, 34)* | 19 (11, 27) | 31 (20, 42) | 30 (24, 36) | 71 (55, 86)* | <0.001* |
Hours clinical and nonclinical work per month for FTE 1.0 | 202 (186, 219) | 211 (196, 226) | 184 (170, 198)* | 193 (186, 201) | 221 (203, 238)* | <0.001* |
Professional activity, weighted mean % (99% CI) | ||||||
Clinical | 84 (78, 89)* | 86 (81, 90) | 78 (72, 84) | 79 (76, 82) | 58 (51, 64)* | <0.001* |
Teaching | 2.3 (1, 5)* | 3 (1, 4) | 6 (4, 9) | 6 (5, 8) | 17 (14, 20)* | <0.001* |
Administration and Committee work | 13 (8, 19) | 11 (8, 15)* | 16 (10, 21) | 14 (12, 17) | 19 (14, 24)* | 0.001* |
Research | 0 (0, 0)* | 1 (0, 2) | 0 (0, 1) | 1 (0, 1) | 7 (3, 11)* | <0.001* |
Table 3 tabulates other work pattern characteristics. Most hospitalists indicated that their current clinical work as hospitalists involved the general medical wards (100%), medical consultations (98%), and comanagement with specialists (92%). There were wide differences in participation in comanagement (100%, local groups vs 71%, academic), intensive care unit (ICU) responsibilities (94%, multistate groups vs 27%, academic), and nursing home care (30%, local groups vs 8%, academic). Among activities that are potentially not reimbursable, academic hospitalists were less likely to participate in coordination of patient transfers and code or rapid response teams, while multistate groups were least likely to participate in quality improvement activities. In total, 99% of hospitalists reported participating in at least 1 potentially nonreimbursable clinical activity.
Local Hospitalist‐Only Group | Multi‐State Hospitalist Group | Multispecialty Physician Group | Employer Hospital | University or Medical School | ||
---|---|---|---|---|---|---|
n = 95 | n = 111 | n = 115 | n = 348 | n = 107 | P Value | |
| ||||||
Reimbursable activities, overlapping weighted % | ||||||
General medical ward | 100 | 99 | 100 | 99 | 99 | 0.809 |
Medical consultations | 99 | 99 | 100 | 98 | 95 | 0.043 |
Comanagement with specialists | 100 | 96 | 96 | 93 | 71 | <0.001 |
Preoperative evaluations | 92 | 92 | 90 | 88 | 77 | 0.002 |
Intensive care unit | 86 | 94 | 67 | 75 | 27 | <0.001 |
Skilled nursing facility or long‐term acute care facility | 30 | 19 | 12 | 16 | 8 | <0.001 |
Outpatient general medical practice | 4 | 4 | 5 | 5 | 10 | 0.241 |
Potentially nonreimbursable activities, overlapping weighted % | ||||||
Coordination of patient transfers | 92 | 94 | 95 | 93 | 82 | 0.005 |
Quality improvement or patient safety initiatives | 81 | 78 | 83 | 89 | 89 | 0.029 |
Code team or rapid response team | 56 | 57 | 53 | 62 | 37 | <0.001 |
Information technology design or implementation | 42 | 39 | 47 | 51 | 51 | 0.154 |
Admission triage for emergency department | 49 | 46 | 43 | 40 | 31 | 0.132 |
Compensation scheme, weighted % | <0.001 | |||||
Salary only | 18 | 21 | 30 | 29 | 47 | |
Salary plus performance incentive | 54 | 72 | 59 | 67 | 53 | |
Fee‐for‐service | 20 | 1 | 7 | 2 | 0 | |
Capitation | 0 | 0 | 0 | 0 | 0 | |
Other | 9 | 7 | 4 | 3 | 0 | |
Compensation links to incentives, overlapping weighted % | ||||||
No incentives | 40 | 28 | 29 | 29 | 48 | 0.003 |
Patient satisfaction | 23 | 39 | 38 | 38 | 14 | <0.001 |
Length of stay | 18 | 17 | 20 | 13 | 10 | 0.208 |
Overall cost | 8 | 11 | 9 | 5 | 6 | 0.270 |
Test utilization | 2 | 2 | 7 | 1 | 0 | <0.001 |
Clinical processes and outcomes | 26 | 34 | 44 | 43 | 24 | <0.001 |
Other | 17 | 29 | 26 | 31 | 25 | 0.087 |
Earnings, weighted mean dollars (99% CI) | 226,065 (202,891, 249,240)* | 225,613 (210,772, 240,454) | 202,617 (186,036, 219,198) | 206,087 (198,413, 213,460) | 166,478 (151,135, 181,821)* | <0.001* |
Hospitalist compensation schemes were significantly different across the practice models. Salary‐only schemes were most common among academic hospitalists (47%), while 72% of multistate groups used performance incentives in addition to salary. More local groups used fee‐for‐service compensation than other models. Incentives differed by practice model, with more multistate groups having incentives based on patient satisfaction, while more multispecialty physician groups had incentives based on clinical processes and outcomes than other models. Finally, mean earnings for academic hospitalists were significantly lower than for hospitalists of other practice models. Local and multistate group hospitalists earned more than any other practice model (all P <0.001), and $60,000 more than the lowest compensated academic hospitalists.
Components of Job Satisfaction
Hospitalists' rankings of the most important factors for job satisfaction revealed differences across models (Figure 2). Overall, hospitalists were most likely to consider optimal workload and compensation as important factors for job satisfaction from a list of 13 considerations. Local groups and academics were least likely to rank optimal workload as a top factor, and local group hospitalists were more likely to rank optimal autonomy than those of other models. Academic hospitalists had less concern for substantial pay, and more concern for the variety of tasks they perform and recognition by leaders, than other hospitalists.
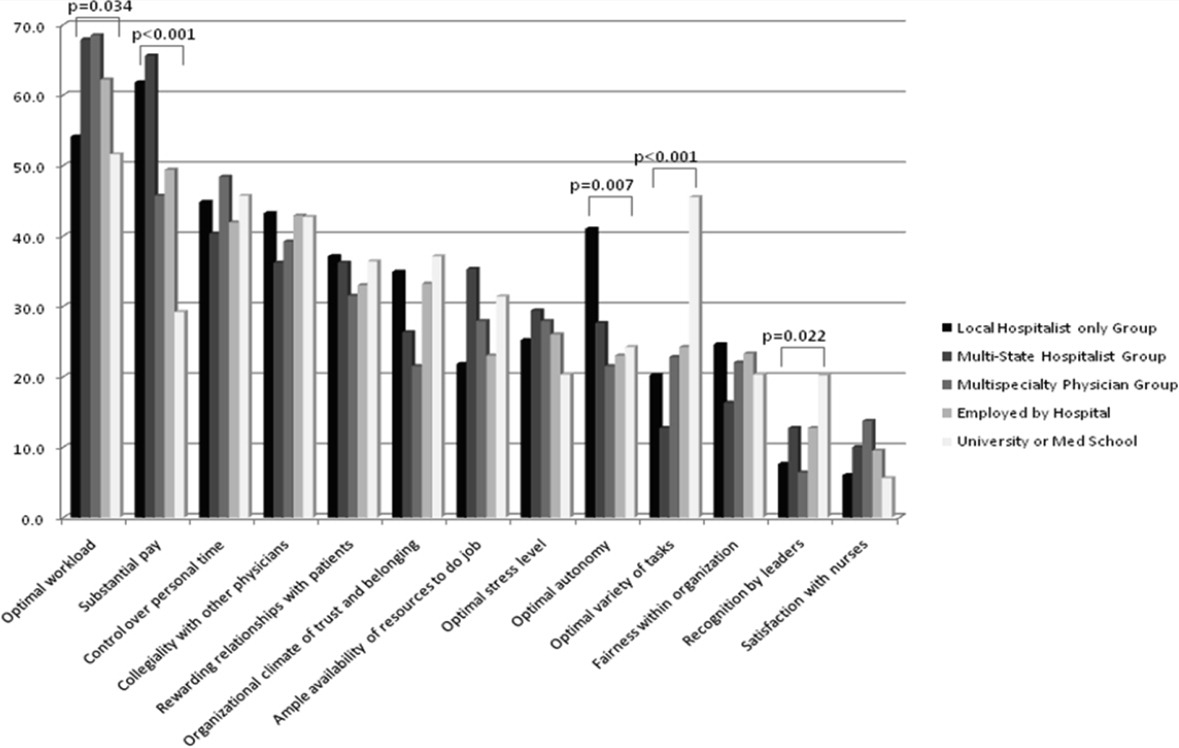
Job Satisfaction and Burnout Risk
Differences in the ratings of 4 of the 11 satisfaction and job characteristic domains were found across the practice models (Figure 3). Multispecialty group hospitalists were less satisfied with autonomy and their relationship with patients than other practice models, and along with multistate groups, reported the highest perceived workload. Organizational fairness was rated much higher by local group hospitalists than other practice models. Despite these differences in work patterns and satisfaction, there were no differences found in level of global job satisfaction, specialty satisfaction, or burnout across the practice models. Overall, 62% of respondents reported high job satisfaction (4 on a 1 to 5 scale), and 30% indicated burnout symptoms.
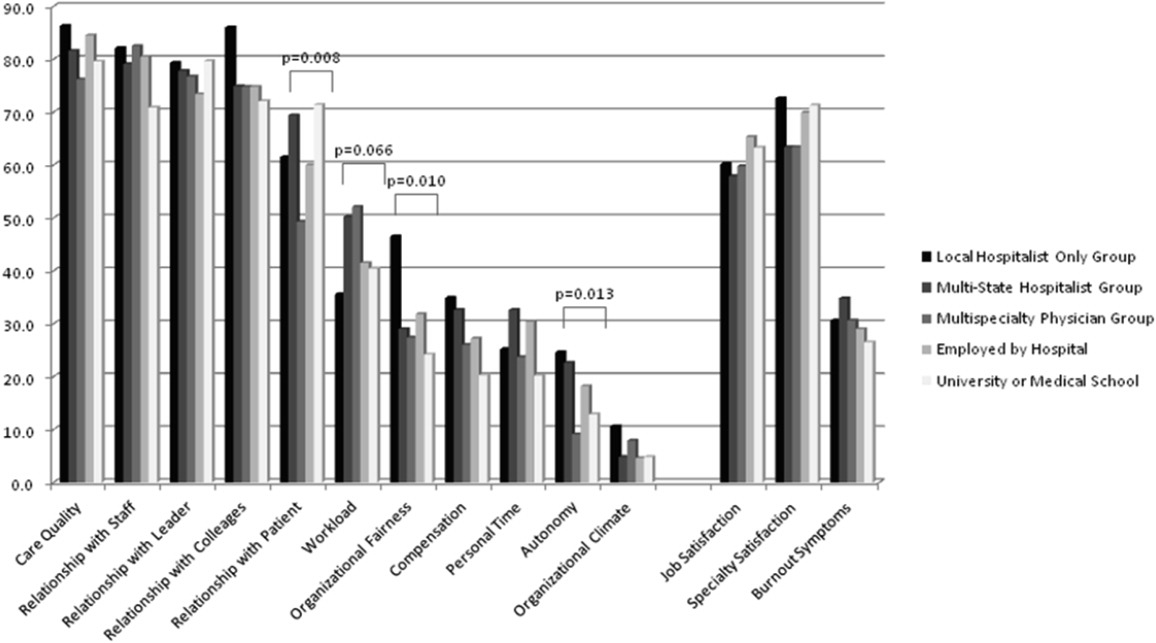
DISCUSSION
In our sample of US hospitalists, we found major differences in work patterns and compensation across hospitalist practice models, but no differences in job satisfaction, specialty satisfaction, and burnout. In particular, differences across these models included variations in hospitalist workload, hours, pay, and distribution of work activities. We found that hospitalists perform a variety of clinical and nonclinical tasks, for many of which there are not standard reimbursement mechanisms. We also found that features of a job that individual hospitalists considered most important vary by practice model.
Previous analysis of this data explored the overall state of hospitalist satisfaction.16 The present analysis offers a glimpse into hospitalists' systems‐orientation through a deeper look at their work patterns. The growth in the number of hospitalists who participate in intensive care medicine, specialty comanagement, and other work that involves close working relationships with specialist physicians confirms collaborative care as one of the dominant drivers of the hospitalist movement. At the level of indirect patient care, nearly all hospitalists contributed to work that facilitates coordination, quality, patient safety, or information technology. Understanding the integrative value of hospitalists outside of their clinical productivity may be of interest to hospital administrators.
Global satisfaction measures were similar across practice models. This finding is particularly interesting given the major differences in job characteristics seen among the practice models. This similarity in global satisfaction despite real differences in the nature of the job suggests that individuals find settings that allow them to address their individual professional goals. Our study demonstrates that, in 2010, Hospital Medicine has evolved enough to accommodate a wide variety of goals and needs.
While global satisfaction did not differ among practice types, hospitalists from various models did report differences in factors considered important to global satisfaction. While workload and pay were rated as influential across most models, the degree of importance was significantly different. In academic settings, substantial pay was not a top consideration for overall job satisfaction, whereas in local and multistate hospitalist groups, pay was a very close second in importance to optimal workload. These results may prove helpful for individual hospitalists trying to find their optimal job. For example, someone who is less concerned about workload, but wants to be paid well and have a high degree of autonomy, may find satisfaction in local hospitalist groups. However, for someone who is willing to sacrifice a higher salary for variety of activities, academic Hospital Medicine may be a better fit.
There is a concerning aspect of hospitalist job satisfaction that different practice models do not seem to solve. Control over personal time is a top consideration for many hospitalists across practice models, yet their satisfaction with personal time is low. As control over personal time is seen as a draw to the Hospital Medicine specialty, group leaders may need to evaluate their programs to ensure that schedules and workload support efforts for hospitalists to balance work and homelife commitments.
There are additional findings that are important for Hospital Medicine group leaders. Regardless of practice model, compensation and workload are often used as tools to recruit and retain hospitalists. While these tools may be effective, leaders may find more nuanced approaches to improving their hospitalists' overall satisfaction. Leaders of local hospitalist groups may find their hospitalists tolerant of heavier workloads as long as they are adequately rewarded and are given real autonomy over their work. However, leaders of academic programs may be missing the primary factor that can improve their hospitalists' satisfaction. Rather than asking for higher salaries to remain competitive, it may be more effective to advocate for time and training for their hospitalists to pursue important other activities beyond direct clinical care. Given that resources will always be limited, group leaders need to understand all of the elements that can contribute to hospitalist job satisfaction.
We point out several limitations to this study. First, our adjusted response rate of 25.6% is low for survey research, in general. As mentioned above, hospitalists are not easily identified in any available national physician database. Therefore, we deliberately designed our sampling strategy to error on the side of including ineligible surveyees to reduce systematic exclusion of practicing hospitalists. Using simple post hoc methods, we identified many nonhospitalists and bad addresses from our sample, but because these methods were exclusionary as opposed to confirmatory, we believe that a significant proportion of remaining nonrespondents may also have been ineligible for the survey. Although this does not fully address concerns about potential response bias, we believe that our sample representing a large number of hospitalist groups is adequate to make estimations about a nationally representative sample of practicing hospitalists. Second, in spite of our inclusive approach, we may still have excluded categories of practicing hospitalists. We were careful not to allow SHM members to represent all US hospitalists and included non‐members in the sampling frame, but the possibility of systematic exclusion that may alter our results remains a concern. Additionally, one of our goals was to characterize pediatric hospitalists independently from their adult‐patient counterparts. Despite oversampling of pediatricians, their sample was too small for a more detailed comparison across practice models. Also, self‐reported data about workload and compensation are subject to inaccuracies related to recall and cognitive biases. Last, this is a cross‐sectional study of hospitalist satisfaction at one point in time. Consequently, our sample may not be representative of very dissatisfied hospitalists who have already left their jobs.
The diversity found across existing practice models and the characteristics of the practices provide physicians with the opportunity to bring their unique skills and motivations to the hospitalist movement. As hospitals and other organizations seek to create, maintain, or grow hospitalist programs, the data provided here may prove useful to understand the relationship between practice characteristics and individual job satisfaction. Additionally, hospitalists looking for a job can consider these results as additional information to guide their choice of practice model and work patterns.
Acknowledgements
The authors thank Kenneth A. Rasinski for assistance with survey items refinement, and members of the SHM Career Satisfaction Task Force for their assistance in survey development.
Over the past 15 years, there has been dramatic growth in the number of hospitalist physicians in the United States and in the number of hospitals served by them.13 Hospitals are motivated to hire experienced hospitalists to staff their inpatient services,4 with goals that include obtaining cost‐savings and higher quality.59 The rapid growth of Hospital Medicine saw multiple types of hospital practice models emerge with differing job characteristics, clinical duties, workload, and compensation schemes.10 The extent of the variability of hospitalist jobs across practice models is not known.
Intensifying recruitment efforts and the concomitant increase in compensation for hospitalists over the last decade suggest that demand for hospitalists is strong and sustained.11 As a result, today's cohort of hospitalists has a wide range of choices of types of jobs, practice models, and locations. The diversity of available hospitalist jobs is characterized, for example, by setting (community hospital vs academic hospital), employer (hospital vs private practice), job duties (the amount and type of clinical work, and other administrative, teaching, or research duties), and intensity (work hours and duties to maximize income or lifestyle). How these choices relate to job satisfaction and burnout are also unknown.
The Society of Hospital Medicine (SHM) has administered surveys to hospitalist group leaders biennially since 2003.1215 These surveys, however, do not address issues related to individual hospitalist worklife, recruitment, and retention. In 2005, SHM convened a Career Satisfaction Task Force that designed and executed a national survey of hospitalists in 2009‐2010. The objective of this study is to evaluate how job characteristics vary by practice model, and the association of these characteristics and practice models with job satisfaction and burnout.
METHODS
Survey Instrument
A detailed description of the survey design, sampling strategy, data collection, and response rate calculations is described elsewhere.16 Portions of the 118‐item survey instrument assessed characteristics of the respondents' hospitalist group (12 items), details about their individual work patterns (12 items), and demographics (9 items). Work patterns were evaluated by the average number of clinical work days, consecutive days, hours per month, percentage of work assigned to night duty, and number of patient encounters. Average hours spent on nonclinical work, and the percentage of time allocated for clinical, administrative, teaching, and research activities were solicited. Additional items assessed specific clinical responsibilities, pretax earnings in FY2010, the availability of information technology capabilities, and the adequacy of available resources. Job and specialty satisfaction and 11 satisfaction domain measures were measured using validated scales.1726 Burnout symptoms were measured using a validated single‐item measure.26, 27
Sampling Strategy
We surveyed a national stratified sample of hospitalists in the US and Puerto Rico. We used the largest database of hospitalists (>24,000 names) currently available and maintained by the SHM as our sampling frame. We linked hospitalist employer information to hospital statistics from the American Hospital Association database28 to stratify the sample by number of hospital beds, geographic region, employment model, and specialty training, oversampling pediatric hospitalists due to small numbers. A respondent sample of about 700 hospitalists was calculated to be adequate to detect a 0.5 point difference in job satisfaction scores between subgroups assuming 90% power and alpha of 0.05. However, we sampled a total of 5389 addresses from the database to overcome the traditionally low physician response rates, duplicate sampling, bad addresses, and non‐hospitalists being included in the sampling frame. In addition, 2 multistate hospitalist companies (EmCare, In Compass Health) and 1 for‐profit hospital chain (HCA, Inc) financially sponsored this project with the stipulation that all of their hospitalist employees (n = 884) would be surveyed.
Data Collection
The healthcare consulting firm, Press Ganey, provided support with survey layout and administration following the modified Dillman method.29 Three rounds of coded surveys and solicitation letters from the investigators were mailed 2 weeks apart in November and December 2009. Because of low response rates to the mailed survey, an online survey was created using Survey Monkey and sent to 650 surveyees for whom e‐mail addresses were available, and administered at a kiosk for sample physicians during the SHM 2010 annual meeting.
Data Analysis
Nonresponse bias was measured by comparing characteristics between respondents of separate survey waves.30 We determined the validity of mailing addresses immediately following the survey period by mapping each address using Google, and if the address was a hospital, researching online whether or not the intended recipient was currently employed there. Practice characteristics were compared across 5 model categories distilled from the SHM & Medical Group Management Association survey: local hospitalist‐only group, multistate hospitalist group, multispecialty physician group, employer hospital, and university or medical school. Weighted proportions, means, and medians were calculated to account for oversampling of pediatric hospitalists. Differences in categorical measures were assessed using the chi‐square test and the design‐based F test for comparing weighted data. Weighted means (99% confidence intervals) and medians (interquartile ranges) were calculated. Because each parameter yielded a single outlier value across the 5 practice models, differences across weighted means were assessed using generalized linear models with the single outlier value chosen as the reference mean. Pair‐wise Wilcoxon rank sum test was used to compare median values. In these 4‐way comparisons of means and medians, significance was defined as P value of 0.0125 per Bonferroni correction. A single survey item solicited respondents to choose exactly 4 of 13 considerations most pertinent to job satisfaction. The proportion of respondents who scored 4 on a 5‐point Likert scale of the 11 satisfaction domains and 2 global measures of satisfaction, and burnout symptoms defined as 3 on a 5‐point single item measure were bar‐graphed. Chi‐square statistics were used to evaluate for differences across practice models. Statistical significance was defined by alpha less than 0.05, unless otherwise specified. All analyses were performed using STATA version 11.0 (College Station, TX). This study was approved by the Loyola University Institutional Review Board.
Survey data required cleaning prior to analysis. Missing gender information was imputed using the respondents' name. Responses to the item that asked to indicate the proportion of work dedicated to administrative responsibilities, clinical care, teaching, and research that did not add up to 100% were dropped. Two responses that indicated full‐time equivalent (FTE) of 0%, but whose respondents otherwise completed the survey implying they worked as clinical hospitalists, were replaced with values calculated from the given number of work hours relative to the median work hours in our sample. Out of range or implausible responses to the following items were dropped from analyses: the average number of billable encounters during a typical day or shift, number of shifts performing clinical activities during a typical month, pretax earnings, the year the respondent completed residency training, and the number of whole years practiced as a hospitalist. The proportion of selective item nonresponse was small and we did not, otherwise, impute missing data.
RESULTS
Response Rate
Of the 5389 originally sampled addresses, 1868 were undeliverable. Addresses were further excluded if they appeared in duplicate or were outdated. This yielded a total of 3105 eligible surveyees in the sample. As illustrated in Figure 1, 841 responded to the mailed survey and 5 responded to the Web‐based survey. After rejecting 67 non‐hospitalist respondents and 3 duplicate surveys, a total of 776 surveys were included in the final analysis. The adjusted response rate was 25.6% (776/3035). Members of SHM were more likely to return the survey than nonmembers. The adjusted response rate from hospitalists affiliated with the 3 sponsoring institutions was 6% (40/662). Because these respondents were more likely to be non‐members of SHM, we opted to analyze the responses from the sponsor hospitalists together with the sampled hospitalists. The demographics of the resulting pool of 816 respondents affiliated with over 650 unique hospitalist groups were representative of the original survey frame. We analyzed data from 794 of these who responded to the item indicating their hospitalist practice model. Demographic characteristics of responders and nonresponders to the practice model survey item were similar.
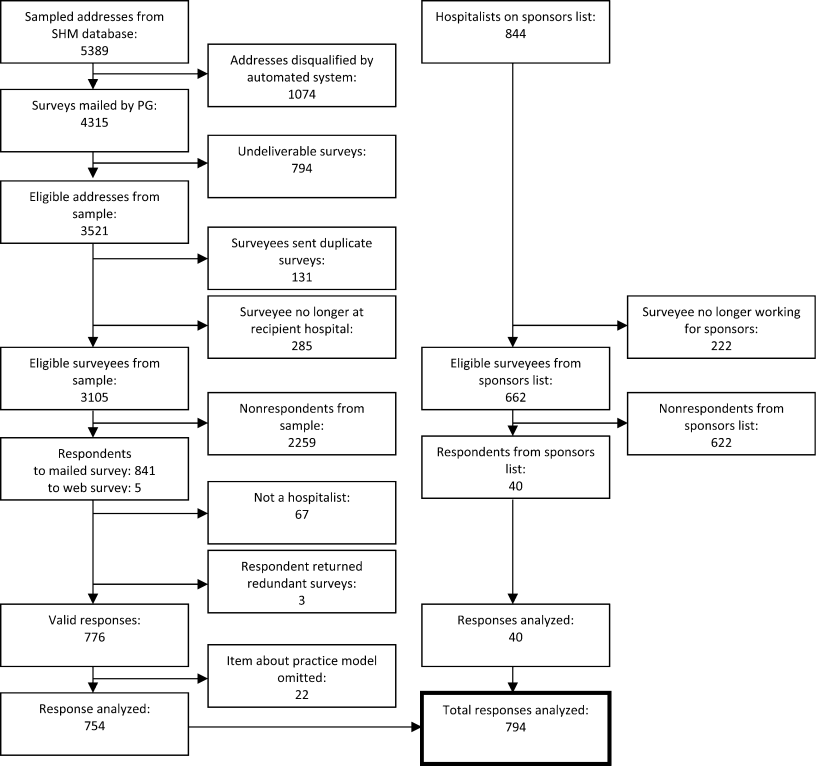
Characteristics of Hospitalists and Their Groups
Table 1 summarizes the characteristics of hospitalist respondents and their organizations by practice model. More (44%) respondents identified their practice model as directly employed by the hospital than other models, including multispecialty physician group (15%), multistate hospitalist group (14%), university or medical school (14%), local hospitalist group (12%), and other (2%). The median age of hospitalist respondents was 42 years, with 6.8 years of mean experience as a hospitalist. One third were women, 84% were married, and 46% had dependent children 6 years old or younger at home. Notably, hospitalists in multistate groups had fewer years of experience, and fewer hospitalists in local and multistate groups were married compared to hospitalists in other practice models.
Local Hospitalist‐Only Group | Multi‐State Hospitalist Group | Multispecialty Physician Group | Employer Hospital | University or Medical School | ||
---|---|---|---|---|---|---|
n = 95 | n = 111 | n = 115 | n = 348 | n = 107 | P Value | |
| ||||||
Hospitalist characteristics | ||||||
Age, weighted mean (99% CI) | 45 (42, 48) | 44 (42, 47) | 45 (43, 47) | 45 (43, 46) | 43 (40, 46) | |
Years hospitalist experience, weighted mean (99% CI) | 8 (6, 9)* | 5 (4, 6)* | 8 (7, 9) | 7 (6, 7) | 8 (6, 9) | <0.010* |
Women, weighted % | 29 | 30 | 39 | 31 | 43 | 0.118 |
Married, weighted % | 76 | 77 | 82 | 89 | 81 | 0.009 |
At least 1 dependent child younger than age 6 living in home, weighted % | 47 | 48 | 43 | 47 | 45 | 0.905 |
Pediatric specialty, n (%) | <10 | <10 | 11 (10%) | 57 (16%) | 36 (34%) | <0.001 |
Hospitalist group characteristics | ||||||
Region, weighted % | <0.001 | |||||
Northeast (AHA 1 & 2) | 13 | 10 | 16 | 27 | 13 | |
South (AHA 3 & 4) | 19 | 37 | 13 | 24 | 21 | |
Midwest (AHA 5 & 6) | 23 | 24 | 25 | 22 | 26 | |
Mountain (AHA 7 & 8) | 22 | 20 | 16 | 13 | 24 | |
West (AHA 9) | 24 | 10 | 31 | 14 | 16 | |
No. beds of primary hospital, weighted % | <0.001 | |||||
Up to 149 | 17 | 26 | 12 | 24 | 14 | |
150299 | 30 | 36 | 36 | 33 | 21 | |
300449 | 26 | 24 | 29 | 20 | 19 | |
450599 | 13 | 8 | 17 | 11 | 21 | |
600 or more | 12 | 6 | 7 | 13 | 24 | |
No. of hospital facilities served by current practice, weighted % | <0.001 | |||||
1 | 53 | 70 | 67 | 77 | 66 | |
2 | 20 | 22 | 20 | 16 | 24 | |
3 or more | 27 | 9 | 13 | 7 | 10 | |
No. of physicians in current practice, median (IQR) | 10 (5, 18) | 8 (6, 12)* | 14 (8, 25)* | 12 (6, 18) | 12 (7, 20) | <0.001*, 0.001 |
No. of non‐physician providers in current practice, median (IQR) | 0 (0, 2) | 0 (0, 2) | 0 (0, 3) | 1 (0, 2) | 0 (0, 2) | |
Available information technology capabilities, weighted % | ||||||
EHR to access physician notes | 57 | 57 | 75 | 58 | 79 | <0.001 |
EHR to access nursing documentations | 68 | 67 | 74 | 75 | 76 | 0.357 |
EHR to access laboratory or test results | 97 | 89 | 95 | 96 | 96 | 0.054 |
Electronic order entry | 30 | 19 | 53 | 38 | 56 | <0.001 |
Electronic billing | 38 | 31 | 36 | 36 | 38 | 0.818 |
Access to EHR at home or off site | 78 | 73 | 78 | 82 | 84 | 0.235 |
Access to Up‐to‐Date or other clinical guideline resources | 80 | 77 | 91 | 92 | 96 | <0.001 |
Access to schedules, calendars, or other organizational resources | 56 | 57 | 66 | 67 | 75 | 0.024 |
E‐mail, Web‐based paging, or other communication resources | 74 | 63 | 88 | 89 | 90 | <0.001 |
Several differences in respondent group characteristics by practice model were found. Respondents in multistate hospitalist groups were more likely from the South and Midwest, while respondents from multispecialty groups were likely from the West. More multistate group practices were based in smaller hospitals, while academic hospitalists tended to practice in hospitals with 600 or more beds. Respondents employed by hospitals were more likely to practice at 1 hospital facility only, while local group practices were more likely to practice at 3 or more facilities. The median number of physicians in a hospitalist group was 11 (interquartile range [IQR] 6, 19). Local and multistate groups had fewer hospitalists compared to other models. Nonphysician providers were employed by nearly half of all hospitalist practices. Although almost all groups had access to some information technology, more academic hospitalists had access to electronic order entry, electronic physician notes, electronic clinical guidelines resources and communication technology, while local and multistate groups were least likely to have access to these resources.
Work Pattern Variations
Table 2 further details hospitalist work hours by practice model. The majority of hospitalists (78%) reported their position was full‐time (FTE 1.0), while 13% reported working less than full‐time (FTE <1.0). Only 5% of local group hospitalists worked part‐time, while 20% of multispecialty group hospitalists did. An additional 9% reported FTE >1.0, indicating their work hours exceeded the definition of a full‐time physician in their practice. Among full‐time hospitalists, local group members worked a greater number of shifts per month than employees of multispecialty groups, hospitals, and academic medical centers. Academic hospitalists reported higher numbers of consecutive clinical days worked on average, but fewer night shifts compared to hospitalists employed by multistate groups, multispecialty groups, and hospitals; fewer billable encounters than hospitalists in local and multistate groups; and more nonclinical work hours than hospitalists of any other practice model. Academic hospitalists also spent more time on teaching and research than other practice models. Hospitalists spent 11%‐18% of their time on administrative and committee responsibilities, with the least amount spent by hospitalists in multistate groups and the most in academic practice.
Local Hospitalist‐Only Group | Multi‐State Hospitalist Group | Multispecialty Physician Group | Employer Hospital | University or Medical School | ||
---|---|---|---|---|---|---|
n = 95 | n = 111 | n = 115 | n = 348 | n = 107 | P Value | |
| ||||||
FTE, weighted % | 0.058 | |||||
FTE < 1.0 | 6 | 13 | 20 | 12 | 14 | |
FTE = 1.0 | 85 | 75 | 74 | 80 | 82 | |
FTE > 1.0 | 10 | 13 | 6 | 8 | 5 | |
Workload parameters, weighted mean (99% CI) | ||||||
Clinical shifts per month for FTE 1.0 | 19 (17, 20)* | 17 (16, 19) | 15 (14, 17)* | 16 (15, 16) | 15 (13, 17) | <0.001* |
Hours per clinical shift | 10 (9, 11) | 11 (10, 11)* | 10 (10, 11.0) | 11 (10, 11.0) | 10 (9, 10)* | 0.006*, 0.002 |
Consecutive days on clinical shift | 8 (6, 9) | 7 (6, 7)* | 6 (6, 7) | 7 (6, 7) | 9 (7, 10)* | 0.002*, <0.001 |
% Clinical shifts on nights | 20 (15, 25) | 23 (18, 28)* | 23 (17, 29) | 21 (17, 24) | 14 (9, 18)* | 0.001*, 0.002 |
% Night shifts spent in hospital | 61 (49, 74)* | 63 (52, 75) | 72 (62, 83) | 73 (67, 80) | 43 (29, 57)* | 0.010*, 0.003, <0.001 |
Billable encounters per clinical shift | 17 (14, 19)* | 17 (16, 18) | 14 (13, 15) | 15 (14, 16) | 13 (11, 14)* | <0.001*, 0.002 |
Hours nonclinical work per month | 23 (12, 34)* | 19 (11, 27) | 31 (20, 42) | 30 (24, 36) | 71 (55, 86)* | <0.001* |
Hours clinical and nonclinical work per month for FTE 1.0 | 202 (186, 219) | 211 (196, 226) | 184 (170, 198)* | 193 (186, 201) | 221 (203, 238)* | <0.001* |
Professional activity, weighted mean % (99% CI) | ||||||
Clinical | 84 (78, 89)* | 86 (81, 90) | 78 (72, 84) | 79 (76, 82) | 58 (51, 64)* | <0.001* |
Teaching | 2.3 (1, 5)* | 3 (1, 4) | 6 (4, 9) | 6 (5, 8) | 17 (14, 20)* | <0.001* |
Administration and Committee work | 13 (8, 19) | 11 (8, 15)* | 16 (10, 21) | 14 (12, 17) | 19 (14, 24)* | 0.001* |
Research | 0 (0, 0)* | 1 (0, 2) | 0 (0, 1) | 1 (0, 1) | 7 (3, 11)* | <0.001* |
Table 3 tabulates other work pattern characteristics. Most hospitalists indicated that their current clinical work as hospitalists involved the general medical wards (100%), medical consultations (98%), and comanagement with specialists (92%). There were wide differences in participation in comanagement (100%, local groups vs 71%, academic), intensive care unit (ICU) responsibilities (94%, multistate groups vs 27%, academic), and nursing home care (30%, local groups vs 8%, academic). Among activities that are potentially not reimbursable, academic hospitalists were less likely to participate in coordination of patient transfers and code or rapid response teams, while multistate groups were least likely to participate in quality improvement activities. In total, 99% of hospitalists reported participating in at least 1 potentially nonreimbursable clinical activity.
Local Hospitalist‐Only Group | Multi‐State Hospitalist Group | Multispecialty Physician Group | Employer Hospital | University or Medical School | ||
---|---|---|---|---|---|---|
n = 95 | n = 111 | n = 115 | n = 348 | n = 107 | P Value | |
| ||||||
Reimbursable activities, overlapping weighted % | ||||||
General medical ward | 100 | 99 | 100 | 99 | 99 | 0.809 |
Medical consultations | 99 | 99 | 100 | 98 | 95 | 0.043 |
Comanagement with specialists | 100 | 96 | 96 | 93 | 71 | <0.001 |
Preoperative evaluations | 92 | 92 | 90 | 88 | 77 | 0.002 |
Intensive care unit | 86 | 94 | 67 | 75 | 27 | <0.001 |
Skilled nursing facility or long‐term acute care facility | 30 | 19 | 12 | 16 | 8 | <0.001 |
Outpatient general medical practice | 4 | 4 | 5 | 5 | 10 | 0.241 |
Potentially nonreimbursable activities, overlapping weighted % | ||||||
Coordination of patient transfers | 92 | 94 | 95 | 93 | 82 | 0.005 |
Quality improvement or patient safety initiatives | 81 | 78 | 83 | 89 | 89 | 0.029 |
Code team or rapid response team | 56 | 57 | 53 | 62 | 37 | <0.001 |
Information technology design or implementation | 42 | 39 | 47 | 51 | 51 | 0.154 |
Admission triage for emergency department | 49 | 46 | 43 | 40 | 31 | 0.132 |
Compensation scheme, weighted % | <0.001 | |||||
Salary only | 18 | 21 | 30 | 29 | 47 | |
Salary plus performance incentive | 54 | 72 | 59 | 67 | 53 | |
Fee‐for‐service | 20 | 1 | 7 | 2 | 0 | |
Capitation | 0 | 0 | 0 | 0 | 0 | |
Other | 9 | 7 | 4 | 3 | 0 | |
Compensation links to incentives, overlapping weighted % | ||||||
No incentives | 40 | 28 | 29 | 29 | 48 | 0.003 |
Patient satisfaction | 23 | 39 | 38 | 38 | 14 | <0.001 |
Length of stay | 18 | 17 | 20 | 13 | 10 | 0.208 |
Overall cost | 8 | 11 | 9 | 5 | 6 | 0.270 |
Test utilization | 2 | 2 | 7 | 1 | 0 | <0.001 |
Clinical processes and outcomes | 26 | 34 | 44 | 43 | 24 | <0.001 |
Other | 17 | 29 | 26 | 31 | 25 | 0.087 |
Earnings, weighted mean dollars (99% CI) | 226,065 (202,891, 249,240)* | 225,613 (210,772, 240,454) | 202,617 (186,036, 219,198) | 206,087 (198,413, 213,460) | 166,478 (151,135, 181,821)* | <0.001* |
Hospitalist compensation schemes were significantly different across the practice models. Salary‐only schemes were most common among academic hospitalists (47%), while 72% of multistate groups used performance incentives in addition to salary. More local groups used fee‐for‐service compensation than other models. Incentives differed by practice model, with more multistate groups having incentives based on patient satisfaction, while more multispecialty physician groups had incentives based on clinical processes and outcomes than other models. Finally, mean earnings for academic hospitalists were significantly lower than for hospitalists of other practice models. Local and multistate group hospitalists earned more than any other practice model (all P <0.001), and $60,000 more than the lowest compensated academic hospitalists.
Components of Job Satisfaction
Hospitalists' rankings of the most important factors for job satisfaction revealed differences across models (Figure 2). Overall, hospitalists were most likely to consider optimal workload and compensation as important factors for job satisfaction from a list of 13 considerations. Local groups and academics were least likely to rank optimal workload as a top factor, and local group hospitalists were more likely to rank optimal autonomy than those of other models. Academic hospitalists had less concern for substantial pay, and more concern for the variety of tasks they perform and recognition by leaders, than other hospitalists.
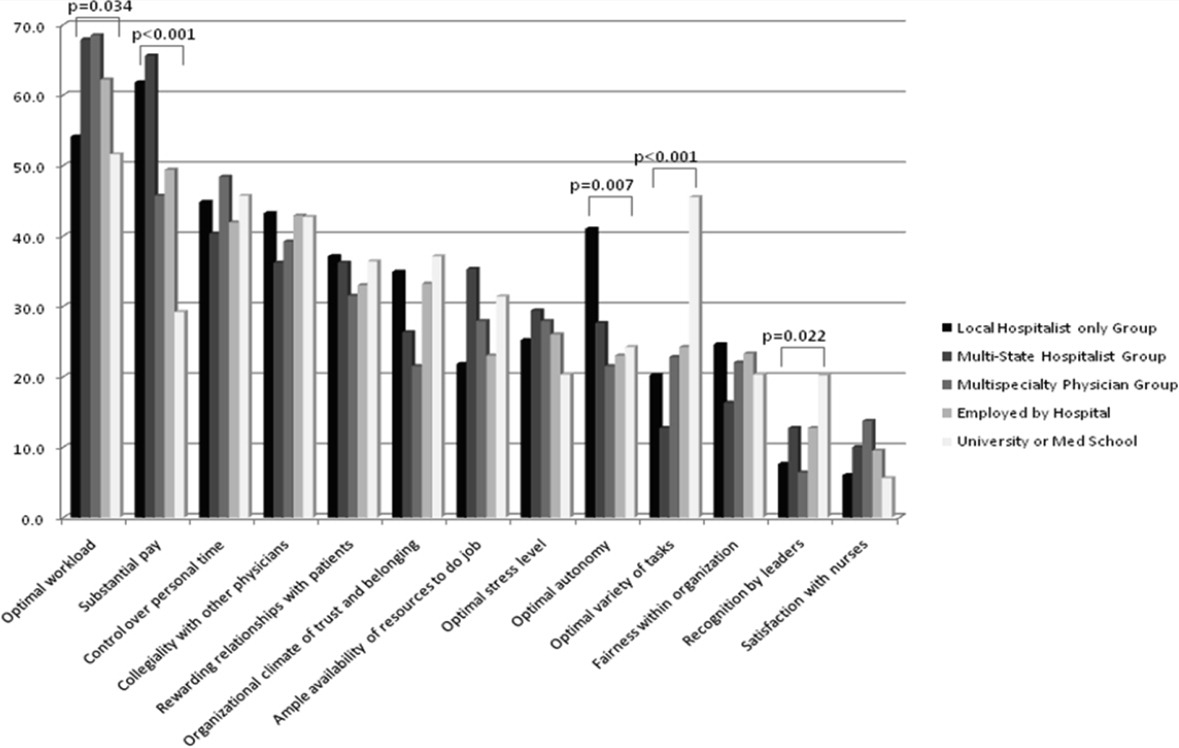
Job Satisfaction and Burnout Risk
Differences in the ratings of 4 of the 11 satisfaction and job characteristic domains were found across the practice models (Figure 3). Multispecialty group hospitalists were less satisfied with autonomy and their relationship with patients than other practice models, and along with multistate groups, reported the highest perceived workload. Organizational fairness was rated much higher by local group hospitalists than other practice models. Despite these differences in work patterns and satisfaction, there were no differences found in level of global job satisfaction, specialty satisfaction, or burnout across the practice models. Overall, 62% of respondents reported high job satisfaction (4 on a 1 to 5 scale), and 30% indicated burnout symptoms.
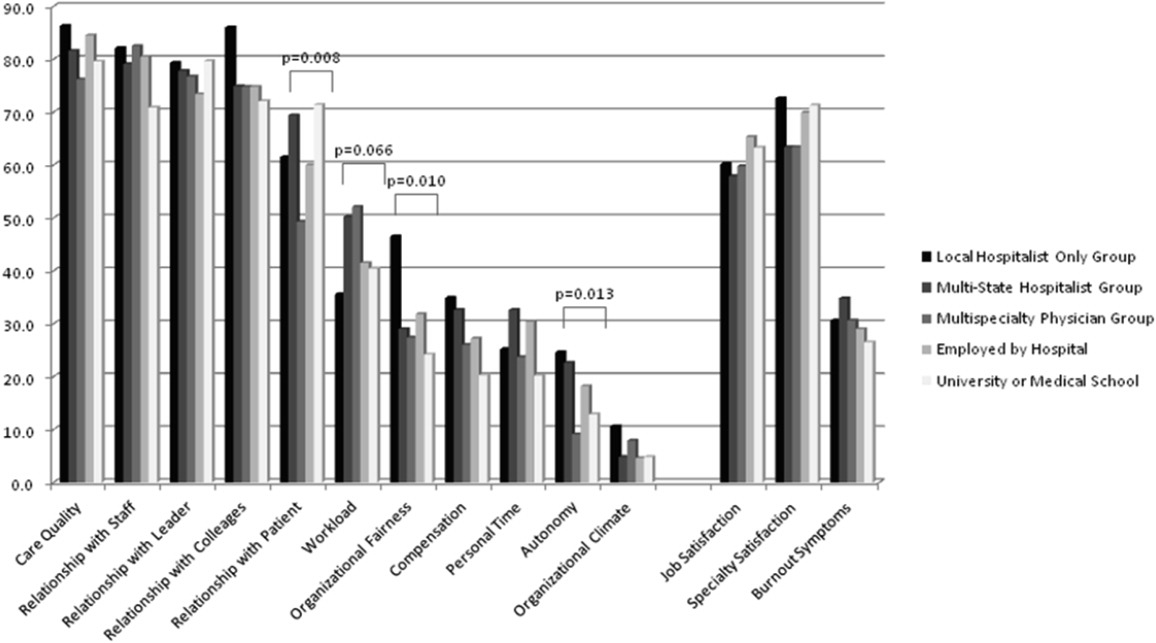
DISCUSSION
In our sample of US hospitalists, we found major differences in work patterns and compensation across hospitalist practice models, but no differences in job satisfaction, specialty satisfaction, and burnout. In particular, differences across these models included variations in hospitalist workload, hours, pay, and distribution of work activities. We found that hospitalists perform a variety of clinical and nonclinical tasks, for many of which there are not standard reimbursement mechanisms. We also found that features of a job that individual hospitalists considered most important vary by practice model.
Previous analysis of this data explored the overall state of hospitalist satisfaction.16 The present analysis offers a glimpse into hospitalists' systems‐orientation through a deeper look at their work patterns. The growth in the number of hospitalists who participate in intensive care medicine, specialty comanagement, and other work that involves close working relationships with specialist physicians confirms collaborative care as one of the dominant drivers of the hospitalist movement. At the level of indirect patient care, nearly all hospitalists contributed to work that facilitates coordination, quality, patient safety, or information technology. Understanding the integrative value of hospitalists outside of their clinical productivity may be of interest to hospital administrators.
Global satisfaction measures were similar across practice models. This finding is particularly interesting given the major differences in job characteristics seen among the practice models. This similarity in global satisfaction despite real differences in the nature of the job suggests that individuals find settings that allow them to address their individual professional goals. Our study demonstrates that, in 2010, Hospital Medicine has evolved enough to accommodate a wide variety of goals and needs.
While global satisfaction did not differ among practice types, hospitalists from various models did report differences in factors considered important to global satisfaction. While workload and pay were rated as influential across most models, the degree of importance was significantly different. In academic settings, substantial pay was not a top consideration for overall job satisfaction, whereas in local and multistate hospitalist groups, pay was a very close second in importance to optimal workload. These results may prove helpful for individual hospitalists trying to find their optimal job. For example, someone who is less concerned about workload, but wants to be paid well and have a high degree of autonomy, may find satisfaction in local hospitalist groups. However, for someone who is willing to sacrifice a higher salary for variety of activities, academic Hospital Medicine may be a better fit.
There is a concerning aspect of hospitalist job satisfaction that different practice models do not seem to solve. Control over personal time is a top consideration for many hospitalists across practice models, yet their satisfaction with personal time is low. As control over personal time is seen as a draw to the Hospital Medicine specialty, group leaders may need to evaluate their programs to ensure that schedules and workload support efforts for hospitalists to balance work and homelife commitments.
There are additional findings that are important for Hospital Medicine group leaders. Regardless of practice model, compensation and workload are often used as tools to recruit and retain hospitalists. While these tools may be effective, leaders may find more nuanced approaches to improving their hospitalists' overall satisfaction. Leaders of local hospitalist groups may find their hospitalists tolerant of heavier workloads as long as they are adequately rewarded and are given real autonomy over their work. However, leaders of academic programs may be missing the primary factor that can improve their hospitalists' satisfaction. Rather than asking for higher salaries to remain competitive, it may be more effective to advocate for time and training for their hospitalists to pursue important other activities beyond direct clinical care. Given that resources will always be limited, group leaders need to understand all of the elements that can contribute to hospitalist job satisfaction.
We point out several limitations to this study. First, our adjusted response rate of 25.6% is low for survey research, in general. As mentioned above, hospitalists are not easily identified in any available national physician database. Therefore, we deliberately designed our sampling strategy to error on the side of including ineligible surveyees to reduce systematic exclusion of practicing hospitalists. Using simple post hoc methods, we identified many nonhospitalists and bad addresses from our sample, but because these methods were exclusionary as opposed to confirmatory, we believe that a significant proportion of remaining nonrespondents may also have been ineligible for the survey. Although this does not fully address concerns about potential response bias, we believe that our sample representing a large number of hospitalist groups is adequate to make estimations about a nationally representative sample of practicing hospitalists. Second, in spite of our inclusive approach, we may still have excluded categories of practicing hospitalists. We were careful not to allow SHM members to represent all US hospitalists and included non‐members in the sampling frame, but the possibility of systematic exclusion that may alter our results remains a concern. Additionally, one of our goals was to characterize pediatric hospitalists independently from their adult‐patient counterparts. Despite oversampling of pediatricians, their sample was too small for a more detailed comparison across practice models. Also, self‐reported data about workload and compensation are subject to inaccuracies related to recall and cognitive biases. Last, this is a cross‐sectional study of hospitalist satisfaction at one point in time. Consequently, our sample may not be representative of very dissatisfied hospitalists who have already left their jobs.
The diversity found across existing practice models and the characteristics of the practices provide physicians with the opportunity to bring their unique skills and motivations to the hospitalist movement. As hospitals and other organizations seek to create, maintain, or grow hospitalist programs, the data provided here may prove useful to understand the relationship between practice characteristics and individual job satisfaction. Additionally, hospitalists looking for a job can consider these results as additional information to guide their choice of practice model and work patterns.
Acknowledgements
The authors thank Kenneth A. Rasinski for assistance with survey items refinement, and members of the SHM Career Satisfaction Task Force for their assistance in survey development.
- The status of hospital medicine groups in the United States.J Hosp Med.2006;1(2):75–80. , , , .
- Growth in the care of older patients by hospitalists in the United States.N Engl J Med.2009;360(11):1102–1112. , , , .
- The state of hospital medicine in 2008.Med Clin North Am.2008;92(2):265–273,vii. .
- Health care market trends and the evolution of hospitalist use and roles.J Gen Intern Med.2005;20(2):101–107. , , , .
- Effects of physician experience on costs and outcomes on an academic general medicine service: results of a trial of hospitalists.Ann Intern Med.2002;137(11):866–874. , , , et al.
- The Park Nicollet experience in establishing a hospitalist system.Ann Intern Med.1999;130(4 pt 2):350–354. .
- Effects of an HMO hospitalist program on inpatient utilization.Am J Manag Care.2001;7(11):1051–1057. , .
- The impact of hospitalists on the cost and quality of inpatient care in the United States: a research synthesis.Med Care Res Rev.2005;62(4):379–406. , .
- Pediatric hospitalists: a systematic review of the literature.Pediatrics.2006;117(5):1736–1744. , , , .
- The hospitalist movement 5 years later.JAMA.2002;287(4):487–494. , .
- Trends in market demand for internal medicine 1999 to 2004: an analysis of physician job advertisements.J Gen Intern Med.2006;21(10):1079–1085. , , , , , .
- SHM. 2003–2004 Survey by the Society of Hospital Medicine on Productivity and Compensation: Analysis of Results. 2004 [updated 2004]. Available at: http://www.hospitalmedicine.org/AM/Template. cfm?Section=Practice_Resources Available at: http://cme.medscape.com/viewarticle/578134. Accessed October 21,2010.
- State of Hospital Medicine: 2010 Report Based on 2009 Data.Englewood, CO and Philadelphia, PA:Medical Group Management Association and Society of Hospital Medicine;2010.
- Worklife and satisfaction of hospitalists: toward flourishing careers.J Gen Intern Med.2011, Jul 20. PMID: 21773849. , , , , .
- Worklife and satisfaction of general internists.Arch Intern Med.2002;162(6):649–656. , , , et al.
- Organizational climate, stress, and error in primary care: the MEMO study. In: Henriksen K, Battles JB, Marks ES, Lewin DI, eds.Advances in Patient Safety: From Research to Implementation. Vol 1: Research Findings.Rockville, MD:Agency for Healthcare Research and Quality;2005;1:65–77. , , , et al.
- Hospitalists and the practice of inpatient medicine: results of a survey of the National Association of Inpatient Physicians.Ann Intern Med.1999;130(4 pt 2):343–349. , , , .
- Physician attitudes toward and prevalence of the hospitalist model of care: results of a national survey.Am J Med.2000;109(8):648–653. , , , , , .
- Taking the Measure of Work: A Guide to Validated Scales for Organizational Research and Diagnosis.Thousand Oaks, CA:Sage Publications;2002. .
- Job Demands and Worker Health.Ann Arbor, MI:University of Michigan, Institute for Social Research;1980. , , , , .
- On the dimensionality of organizational justice: a construct validation of a measure.J Appl Psychol.2001;86(3):386–400. .
- Effect of job demands and social support on worker stress—a study of VDT users.Behav Inform Technol.1995;14(1):32–40. , .
- Measuring physician job satisfaction in a changing workplace and a challenging environment. SGIM Career Satisfaction Study Group. Society of General Internal Medicine.Med Care.1999;37(11):1174–1182. , , , et al.
- Working conditions in primary care: physician reactions and care quality.Ann Intern Med.2009;151(1):28–U48. , , , et al.
- Validation of a single‐item measure of burnout against the Maslach Burnout Inventory among physicians.Stress Health.2004;20(2):75–79. , , .
- American Hospital Association. AHA Hospital Statistics. 2009 [updated 2009]. Available at: http://www.ahadata.com/ahadata/html/AHAStatistics.html. Accessed April 12,2011.
- How to obtain excellent response rates when surveying physicians.Fam Pract.2009;26(1):65–68. , , , et al.
- Estimating nonresponse bias in mail surveys.J Marketing Res.1977;14(3):396–402. , .
- The status of hospital medicine groups in the United States.J Hosp Med.2006;1(2):75–80. , , , .
- Growth in the care of older patients by hospitalists in the United States.N Engl J Med.2009;360(11):1102–1112. , , , .
- The state of hospital medicine in 2008.Med Clin North Am.2008;92(2):265–273,vii. .
- Health care market trends and the evolution of hospitalist use and roles.J Gen Intern Med.2005;20(2):101–107. , , , .
- Effects of physician experience on costs and outcomes on an academic general medicine service: results of a trial of hospitalists.Ann Intern Med.2002;137(11):866–874. , , , et al.
- The Park Nicollet experience in establishing a hospitalist system.Ann Intern Med.1999;130(4 pt 2):350–354. .
- Effects of an HMO hospitalist program on inpatient utilization.Am J Manag Care.2001;7(11):1051–1057. , .
- The impact of hospitalists on the cost and quality of inpatient care in the United States: a research synthesis.Med Care Res Rev.2005;62(4):379–406. , .
- Pediatric hospitalists: a systematic review of the literature.Pediatrics.2006;117(5):1736–1744. , , , .
- The hospitalist movement 5 years later.JAMA.2002;287(4):487–494. , .
- Trends in market demand for internal medicine 1999 to 2004: an analysis of physician job advertisements.J Gen Intern Med.2006;21(10):1079–1085. , , , , , .
- SHM. 2003–2004 Survey by the Society of Hospital Medicine on Productivity and Compensation: Analysis of Results. 2004 [updated 2004]. Available at: http://www.hospitalmedicine.org/AM/Template. cfm?Section=Practice_Resources Available at: http://cme.medscape.com/viewarticle/578134. Accessed October 21,2010.
- State of Hospital Medicine: 2010 Report Based on 2009 Data.Englewood, CO and Philadelphia, PA:Medical Group Management Association and Society of Hospital Medicine;2010.
- Worklife and satisfaction of hospitalists: toward flourishing careers.J Gen Intern Med.2011, Jul 20. PMID: 21773849. , , , , .
- Worklife and satisfaction of general internists.Arch Intern Med.2002;162(6):649–656. , , , et al.
- Organizational climate, stress, and error in primary care: the MEMO study. In: Henriksen K, Battles JB, Marks ES, Lewin DI, eds.Advances in Patient Safety: From Research to Implementation. Vol 1: Research Findings.Rockville, MD:Agency for Healthcare Research and Quality;2005;1:65–77. , , , et al.
- Hospitalists and the practice of inpatient medicine: results of a survey of the National Association of Inpatient Physicians.Ann Intern Med.1999;130(4 pt 2):343–349. , , , .
- Physician attitudes toward and prevalence of the hospitalist model of care: results of a national survey.Am J Med.2000;109(8):648–653. , , , , , .
- Taking the Measure of Work: A Guide to Validated Scales for Organizational Research and Diagnosis.Thousand Oaks, CA:Sage Publications;2002. .
- Job Demands and Worker Health.Ann Arbor, MI:University of Michigan, Institute for Social Research;1980. , , , , .
- On the dimensionality of organizational justice: a construct validation of a measure.J Appl Psychol.2001;86(3):386–400. .
- Effect of job demands and social support on worker stress—a study of VDT users.Behav Inform Technol.1995;14(1):32–40. , .
- Measuring physician job satisfaction in a changing workplace and a challenging environment. SGIM Career Satisfaction Study Group. Society of General Internal Medicine.Med Care.1999;37(11):1174–1182. , , , et al.
- Working conditions in primary care: physician reactions and care quality.Ann Intern Med.2009;151(1):28–U48. , , , et al.
- Validation of a single‐item measure of burnout against the Maslach Burnout Inventory among physicians.Stress Health.2004;20(2):75–79. , , .
- American Hospital Association. AHA Hospital Statistics. 2009 [updated 2009]. Available at: http://www.ahadata.com/ahadata/html/AHAStatistics.html. Accessed April 12,2011.
- How to obtain excellent response rates when surveying physicians.Fam Pract.2009;26(1):65–68. , , , et al.
- Estimating nonresponse bias in mail surveys.J Marketing Res.1977;14(3):396–402. , .
Copyright © 2012 Society of Hospital Medicine
Status of US Hospital Medicine Groups
The term hospitalist was coined in 1996 in an article1 that appeared in the New England Journal of Medicine. Robert M. Wachter, MD, and Lee Goldman, MD, of the University of California, San Francisco, defined hospitalists as hospital‐based physicians who take responsibility for managing medical inpatients. Hospitalists were described as having responsibility for seeing unassigned hospital patients and being available for in‐hospital consultations. Several years later, the Society of Hospital Medicine posted the definition of a hospitalist as someone whose primary professional focus is the medical care of hospitalized patientsin patient care, education, research, and administrative activities.
In January 2002, Wachter and Goldman published a follow‐up article,2 The Hospitalist Movement 5 Years Later, in the Journal of the American Medical Association. This formal review of 19 published studies analyzed the impact of hospital medicine groups on financial and clinical outcomes. Wachter and Goldman concluded, Empirical research supports the premise that hospitalists improve inpatient efficiency without harmful effects on quality or patient satisfaction. These studies indicate an average reduction of cost per stay of 13.4% and an average reduction in length of stay of 16.6%.
The evolution of the hospitalist movement has been fast paced and extensive. Given the recent pace of growth, a scholarly analysis estimated that the mature hospitalist workforce in the United States will eventually total 20,000, making it the equivalent of the cardiology specialty.3 Beyond sheer growth, medical literature has demonstrated positive effects of the hospitalist model on patient quality outcomes, including readmission rates, postoperative complications, and mortality.47
In addition to peer‐reviewed medical literature, there is anecdotal evidence about the growth and effects of the hospitalist movement:
The Society of Hospital Medicine (SHM), the hospitalist professional society, estimated that in 2003 there were 8000 physicians practicing as hospitalists in the United States.8
Twelve of the country's top 15 hospitals have hospital medicine groups.8
As hospital medicine groups have proliferated, 4 major employment models have evolved. Hospitalists can be employees of: 1) a hospital or a hospital subsidiary; 2) a multispecialty or primary care physician group; 3) a medical group (local or national) of independent hospitalists; or 4) a university or medical school. However, there is little published data on the prevalence of each of these hospitalist employment models, nationally or by type of hospital.
To better understand the extent and nature of the hospitalist movement, the American Hospital Association (AHA) utilized its 2003 Annual Survey to gather data on hospital medicine groups in the United States
DATA AND METHODS
The data for our analysis came from the 2003 AHA Annual Survey. Conducted since 1946, this survey is the principal data collection mechanism of the American Hospital Association and is a basic source of data on hospitals in the United States about the availability of services, utilization, personnel, finances, and governance. Its main purpose is to provide a cross‐sectional view of hospitals and hospital performance over time. In the 2003 survey, a series of items were added about hospitalists including whether hospitals had hospital medicine groups, the number of hospitalists operating in such groups, and the employment model used.
The study population for this analysis was limited to US community hospitals (n = 4895). Community hospitals are defined as all adult and pediatric nonfederal, short‐term general, and specialty hospitals whose facilities and services are available to the public. Excluded from the analysis were all federal hospitals, long‐term care hospitals, and psychiatric hospitals.
Imputation of Missing Data
In the 2003 survey, 77% of the 4895 US community hospitals answered the question on specific use of hospitalists. To get a complete picture of the number of groups and hospitalists, we imputed data for the nonresponding hospitals.
We performed logistic regression analysis of data from the responding hospitals to estimate the number of nonresponding hospitals that had a group and the number of hospitalists in these groups. The dependent variable in the regression was whether a hospital had a group, and the independent variables included hospital characteristics for which data were available for all US hospitals, both survey respondents and nonrespondents. The results of the regression analysis were then applied to the data for each nonresponding hospital to estimate its probability of having a group. These probabilities were summed over the various nonresponding hospitals to estimate the total number of nonresponding hospitals that had groups.
To impute the number of hospitalists in the nonresponding set of hospitals, the additional number of groups was stratified into the 9 US Census Divisions. On the basis of reported data, the average number of hospitalists per group was calculated at the Census Division level. The per‐group value was then applied to the number of additional groups, and the result was added to the total number of reported hospitalists. The Census Division values were then summed to produce the national total. To produce results for all other control groupings, the national total was then apportioned across the categories according to percentage of hospitalists by category on the basis of the reported data.
Analytical Plan
In analyzing the hospitalist movement across the country, we realized there are 2 dimensions of diffusion, which can be characterized as breadth and depth. In the present study:
The measure of breadth is the percentage of hospital medicine groups in a given group of hospitals. In the Results section, this measure is sometimes referred to as penetration.
The measure of depth is the number of hospitalists for each average daily census (ADC) of 100 patients. For instance, for a hospital with an average daily census of 100 that has 4 hospitalists, that measure is 4. To compute this metric for a given category of hospitals (eg, major teaching hospitals), the numerator is the number of hospitalists and the denominator is the ADC at hospitals that have hospital medicine groups. The metric reflects the in‐hospital impact of hospital medicine groups at their hospitals.
Using these 2 measures, it is possible to differentiate between a group of hospitals that has many hospital medicine groups but each group has a minimal impact at the hospital versus a group of hospitals that has few hospital medicine groups but each group has a major impact at the hospital.
The analysis also characterizes the employment status of hospitalists by comparing the proportion of hospitals in each of the employment models by category of hospital.
RESULTS
Diffusion and Impact
Overall, the penetration of hospital medicine groups across the 4895 hospitals in the United States is 29% and the in‐hospital impact at hospitals with hospital medicine groups is 3.93 hospitalists per 100 ADC. The average hospital medicine group has 7.9 hospitalists at a hospital with an ADC of 200.6.
Geographic Categories (Tables 1A and 2A)
The Northeast (46%) and the Pacific (40%) divisions have the greatest penetration of hospital medicine groups. The West North Central Division (16%) has the lowest penetration of hospital medicine groups. Hospital medicine groups in the West South Central Division average 11.1 hospitalists, which partially explains why this region has the greatest in‐hospital impact (6.24 hospitalists per 100 ADC). At the other end of the spectrum are the Middle Atlantic and East South Central divisions with (2.42 and 2.83 hospitalists per 100 ADC, respectively.
Category | Hospitals | Hospital medicine groups | Hospitals with hospital medicine groups (%) |
---|---|---|---|
| |||
Region | |||
1: Northeast | 203 | 94 | 46% |
2: Mid‐Atlantic | 486 | 172 | 35% |
3: South‐Atlantic | 731 | 272 | 37% |
4: East North Central | 732 | 209 | 29% |
5: East South Central | 427 | 92 | 22% |
6: West North Central | 675 | 106 | 16% |
7: West South Central | 737 | 164 | 22% |
8: Mountain | 348 | 83 | 24% |
9: Pacific | 556 | 223 | 40% |
Rural/urban | |||
Rural | 2166 | 235 | 11% |
Small urban | 1285 | 488 | 38% |
Large urban | 1444 | 692 | 48% |
Total | 4895 | 1415 | 29% |
Category | Groups (hospitals) | Hospitalists | Hospitalists per group | Hospitalists per 100 census |
---|---|---|---|---|
| ||||
Region | ||||
1: Northeast | 94 | 669 | 7.1 | 3.62 |
2: Mid‐Atlantic | 172 | 1133 | 6.6 | 2.42 |
3: South Atlantic | 272 | 1933 | 7.1 | 3.21 |
4: East North Central | 209 | 2087 | 10.0 | 4.65 |
5: East South Central | 92 | 433 | 4.7 | 2.83 |
6: West North Central | 106 | 887 | 8.4 | 4.37 |
7: West South Central | 164 | 1828 | 11.1 | 6.24 |
8: Mountain | 83 | 644 | 7.8 | 4.43 |
9: Pacific | 223 | 1546 | 6.9 | 4.56 |
Rural/urban | ||||
Rural | 235 | 893 | 3.8 | 4.85 |
Small urban | 488 | 3236 | 6.6 | 3.03 |
Large urban | 692 | 7030 | 10.2 | 4.43 |
Total | 1415 | 11 159 | 7.9 | 3.93 |
There are more hospital medicine groups in urban locations. The penetration of hospital medicine groups is 48% at hospitals in large metropolitan locations (ie, with a population of more than 1 million), 38% at hospitals in small metropolitan locations, and 11% at hospitals in rural areas. However, rural hospitals have a relatively high in‐hospital impact (4.85 hospitalists per 100 ADC), explained by an average group size of 3.8 and an average ADC of 78.4.
Hospital Size, Control/Ownership, and Teaching Status (Tables 1B and 2B)
The penetration of hospital medicine groups increases as the size of the hospital increases. Six percent of hospitals with 6‐24 beds have groups, whereas 71% of hospitals with 500+ beds have groups. Among hospitals with 200 or more beds, 55% have hospital medicine groups compared to 19% of hospitals with fewer than 200 beds. As would be expected, larger hospitals have larger hospital medicine groups: hospitals with 6‐24 beds average 2.1 hospitalists, whereas hospitals with 500+ beds average 14.2 hospitalists. However, hospitalists have a proportionately greater impact at smaller hospitals. Their greatest impact is at hospitals with 6‐24 beds (46.34 hospitalists per 100 ADC); their smallest impact is at hospitals with 500+ beds (2.47 hospitalists per 100 ADC).
Category | Hospitals | Hospital medicine groups | Hospitals with hospital medicine groups (%) |
---|---|---|---|
| |||
Size | |||
6‐24 beds | 327 | 18 | 6% |
25‐49 beds | 965 | 88 | 9% |
50‐99 beds | 1031 | 168 | 16% |
100‐199 beds | 1168 | 372 | 32% |
200‐299 beds | 624 | 287 | 46% |
300‐399 beds | 349 | 183 | 52% |
400‐499 beds | 172 | 116 | 67% |
500+ beds | 259 | 183 | 71% |
Control | |||
Government | 1121 | 161 | 14% |
Not for profit | 2984 | 1032 | 35% |
For profit | 790 | 222 | 28% |
Teaching status | |||
Nonteaching | 3800 | 823 | 22% |
Other teaching | 779 | 382 | 49% |
Major teaching | 316 | 210 | 66% |
Total | 4895 | 1415 | 29% |
Category | Groups (hospitals) | Hospitalists | Hospitalists per group | Hospitalists per 100 census |
---|---|---|---|---|
| ||||
Size | ||||
6‐24 beds | 18 | 38 | 2.1 | 46.34 |
25‐49 beds | 88 | 260 | 3.0 | 17.94 |
50‐99 beds | 168 | 885 | 5.3 | 12.75 |
100‐199 beds | 372 | 1757 | 4.7 | 5.29 |
200‐299 beds | 287 | 2308 | 8.0 | 4.72 |
300‐399 beds | 183 | 1,553 | 8.5 | 3.29 |
400‐499 beds | 116 | 1751 | 15.1 | 4.35 |
500+ beds | 183 | 2,607 | 14.2 | 2.47 |
Control | ||||
Government | 161 | 1,674 | 10.4 | 5.85 |
Not for profit | 1032 | 8,481 | 8.2 | 3.64 |
For profit | 222 | 1,004 | 4.5 | 4.47 |
Teaching Status | ||||
Nonteaching | 823 | 4,910 | 6.0 | 4.85 |
Other teaching | 382 | 2,678 | 7.0 | 3.25 |
Major teaching | 210 | 3,571 | 17.0 | 3.57 |
Total | 1415 | 11 159 | 7.9 | 3.93 |
Of the 3 categories of control, government groups have the lowest penetration of hospital medicine groups (14%). However, the hospital medicine groups at these government‐controlled hospitals are large (10.4 hospitalists), and they have a significant in‐hospital impact on care at these hospitals (5.85 hospitalists per 100 ADC). Not‐for‐profit hospitals have the highest penetration of hospital medicine groups (35%), whereas hospital medicine groups at for‐profit hospitals have the lowest average size (4.5 hospitalists).
There appears to be a relationship between teaching status and the likelihood that a hospital has a hospital medicine group. The penetration of hospital medicine groups is 66% at major teaching hospitals, 49% at other teaching hospitals, and 22% at nonteaching hospitals. However, nonteaching hospitals have a relatively high in‐hospital impact (4.85 hospitalists per 100 ADC). This is explained by their having an average group size of 6.0, but an average ADC of only 123.0 (compared to 477.0 for major teaching hospitals and 215.7 for other teaching hospitals).
Employment Models
The results of the analysis of hospitalist employment models (data not shown) can be summarized as follows:
Employees of hospitals: This employment model averaged 33% of all groups, with an average size of 9.8 hospitalists. The employees of hospital model was more prevalent in the Mid‐Atlantic (56%), New England (49%), and West North Central (45%) regions and in rural hospitals (45%). The East South Central (16%) and West South Central (12%) regions and for‐profit hospitals (20%) had fewer hospital employee groups.
Employees of medical groups: This employment model averaged 29% of all groups, with an average of 7.4 hospitalists. More hospitals in the East South Central (35%) and New England (34%) regions had this employment model. Fewer hospitals in the Mid‐Atlantic (18%) and West North Central (18%) regions and rural (18%) hospitals had medical group‐based groups.
Employees of independent hospitalist groups: This employment group averaged 25% of all groups and had the smallest mean number of hospitalists (6.9). This employment model was more prevalent in for‐profit hospitals (43%) and was less prevalent in the New England (9%) and Mid‐Atlantic (11%) regions and in major teaching hospitals (11%) and government hospitals (19%).
CONCLUSIONS
Hospital medicine groups appear to have become part of the mainstream delivery of health care. With more than 11 000 hospitalists, the specialty is equivalent in size to the gastroenterology medical specialty.9 Fifty‐five percent of hospitals with more than 200 beds have hospital medicine groups. Furthermore, it appears that the growth of the hospitalist movement has not peaked. It is likely that the number of hospitals with hospital medicine groups will increase and that existing hospital medicine groups will continue to add hospitalists.
No one employment model of hospital medicine group appears to dominate the health care landscape. We expect that there will continue to be diversity among the organizations that choose to establish hospital medicine groups.
In light of this growth and diversity, hospital medicine groups appear to be valued by a wide range of stakeholders in the health care industry. The potential benefits provided by hospitalists include financial savings, improved throughput efficiency, improved quality and safety, improved medical education, and better provider satisfaction.
Despite this success story, the hospitalist movement has maintained a relatively low profile among consumers and some segments of the health care industry. This is likely to change. As the hospital medicine specialty gains recognition, hospitalists will receive increased scrutiny and attention. This emerging specialty will need to be able to clearly define its role and document its performance in the constantly changing health care industry.
ADDENDUM
Subsequent to the acceptance of this manuscript, the authors received results of the 2004 Annual Survey of the American Hospital Association. Some highlights of the new data and comparisons to the 2003 results are as follows:
The penetration of hospitals with hospital medicine groups grew from 29% to 34% (for hospitals with 200+ beds, the penetration grew from 55% to 63%)
An estimated 1,661 hospitals have hospital medicine groups (an increase of 17% from 2003)
The average size of a hospital medicine group decreased from 7.9 physicians to 7.5 physicians (a decrease of 5%)
It is estimated that there are 12,504 hospitalists in the U.S. (an increase of 12% from 2003)
Hospital medicine groups remain equally distributed among the three employment models: employees of hospitals 30%, employees of medical groups 29%, employees of independent hospitalist groups 29%
These updated results indicate strong hospitalist growth over the one year period and continued diversity among hospital medicine programs, reinforcing the conclusions of the manuscript.
APPENDIX
AHA Annual Survey Overview
Conducted since 1946, the AHA Annual Survey is the principal data collection mechanism of the American Hospital Association and is a basic source of data on hospitals in the United States. Its main purpose is to provide a cross‐sectional view of the hospital field each year and to make it possible to monitor hospital performance over time. The information that it gathers from a universe of approximately 5700 hospitals concerns primarily the availability of services, utilization, personnel, finances, and governance. Newly added to the 2003 survey were the following questions regarding hospitalists: Do hospitalists provide care for patients in your hospital? YES □ NO □
Hospitalist is defined as a physician whose primary professional focus is the care of hospitalized medical patients (through clinical, education, administrative and research activity).
If yes, please report the number of full time and part time hospitalists?
Full‐time ______
Part‐time ______
Full‐time equivalent (FTE) is the total number of hours worked by all employees over the full (12 month) reporting period divided by the normal number of hours worked by a full‐time employee for that same period. For example, if your hospital considers a normal workweek for a full‐time employee to be 40 hours, a total of 2080 hours would be worked over a full year (52 weeks). If the total number of hours worked by all employees on the payroll is 208 000, then the number of FTEs is 100 (employees). The FTE calculation for a specific occupational category such as registered nurses is exactly the same. The calculation for each occupational category should be based on the number of hours worked by staff employed in that specific category.
If yes, please select the category below that best describes the employment model for your hospitalists:
□ Independent provider group
□ Employed by your hospital
□ Employed by a physician group
□ Employed by a university or school program
□ Other
It is the results from these questions that are the subject of this analysis and the manuscript.
- The emerging role of “hospitalists” in the American health care system.N Eng J Med.1996;335:514–517. , .
- The hospitalist movement 5 years later.JAMA.2002;287:487–494. , .
- The potential size of the hospitalist workforce in the United StatesAm J Med.1999;106:441–445. , , , , .
- Implementation of a hospitalist service at a community hospital: evolution of service utilization, costs, and patient outcomes [abstract]. National Association of Inpatient Physicians, 3rd Annual Meeting. Philadelphia, Penn, April 11‐12,2000. .
- Decreased length of stay, costs, and mortality in a randomized trial of academic hospitalists [abstract]. National Association of Inpatient Physicians, 4th Annual Meeting, Atlanta, GA, March 27‐28,2001. , , , , , .
- The effect of full‐time faculty hospitalists on the efficiency of care at a community teaching hospital.Ann Intern Med.1998;129:197–203. , .
- Program description: a hospitalist run, medical short‐stay unit in a teaching hospital.CMAJ.2000;163:1477–1480. , , , .
- Society of Hospital Medicine. Growth of hospital medicine nationwide. July 2003. Available at: http://www.hospitalmedicine.org/presentation/apps/indlist/intro.asp?flag=18. Accessed February2005.
- American Medical Association.Physician characteristics and distribution in the US, 2004.Chicago, Ill:American Medical Association,2004.
The term hospitalist was coined in 1996 in an article1 that appeared in the New England Journal of Medicine. Robert M. Wachter, MD, and Lee Goldman, MD, of the University of California, San Francisco, defined hospitalists as hospital‐based physicians who take responsibility for managing medical inpatients. Hospitalists were described as having responsibility for seeing unassigned hospital patients and being available for in‐hospital consultations. Several years later, the Society of Hospital Medicine posted the definition of a hospitalist as someone whose primary professional focus is the medical care of hospitalized patientsin patient care, education, research, and administrative activities.
In January 2002, Wachter and Goldman published a follow‐up article,2 The Hospitalist Movement 5 Years Later, in the Journal of the American Medical Association. This formal review of 19 published studies analyzed the impact of hospital medicine groups on financial and clinical outcomes. Wachter and Goldman concluded, Empirical research supports the premise that hospitalists improve inpatient efficiency without harmful effects on quality or patient satisfaction. These studies indicate an average reduction of cost per stay of 13.4% and an average reduction in length of stay of 16.6%.
The evolution of the hospitalist movement has been fast paced and extensive. Given the recent pace of growth, a scholarly analysis estimated that the mature hospitalist workforce in the United States will eventually total 20,000, making it the equivalent of the cardiology specialty.3 Beyond sheer growth, medical literature has demonstrated positive effects of the hospitalist model on patient quality outcomes, including readmission rates, postoperative complications, and mortality.47
In addition to peer‐reviewed medical literature, there is anecdotal evidence about the growth and effects of the hospitalist movement:
The Society of Hospital Medicine (SHM), the hospitalist professional society, estimated that in 2003 there were 8000 physicians practicing as hospitalists in the United States.8
Twelve of the country's top 15 hospitals have hospital medicine groups.8
As hospital medicine groups have proliferated, 4 major employment models have evolved. Hospitalists can be employees of: 1) a hospital or a hospital subsidiary; 2) a multispecialty or primary care physician group; 3) a medical group (local or national) of independent hospitalists; or 4) a university or medical school. However, there is little published data on the prevalence of each of these hospitalist employment models, nationally or by type of hospital.
To better understand the extent and nature of the hospitalist movement, the American Hospital Association (AHA) utilized its 2003 Annual Survey to gather data on hospital medicine groups in the United States
DATA AND METHODS
The data for our analysis came from the 2003 AHA Annual Survey. Conducted since 1946, this survey is the principal data collection mechanism of the American Hospital Association and is a basic source of data on hospitals in the United States about the availability of services, utilization, personnel, finances, and governance. Its main purpose is to provide a cross‐sectional view of hospitals and hospital performance over time. In the 2003 survey, a series of items were added about hospitalists including whether hospitals had hospital medicine groups, the number of hospitalists operating in such groups, and the employment model used.
The study population for this analysis was limited to US community hospitals (n = 4895). Community hospitals are defined as all adult and pediatric nonfederal, short‐term general, and specialty hospitals whose facilities and services are available to the public. Excluded from the analysis were all federal hospitals, long‐term care hospitals, and psychiatric hospitals.
Imputation of Missing Data
In the 2003 survey, 77% of the 4895 US community hospitals answered the question on specific use of hospitalists. To get a complete picture of the number of groups and hospitalists, we imputed data for the nonresponding hospitals.
We performed logistic regression analysis of data from the responding hospitals to estimate the number of nonresponding hospitals that had a group and the number of hospitalists in these groups. The dependent variable in the regression was whether a hospital had a group, and the independent variables included hospital characteristics for which data were available for all US hospitals, both survey respondents and nonrespondents. The results of the regression analysis were then applied to the data for each nonresponding hospital to estimate its probability of having a group. These probabilities were summed over the various nonresponding hospitals to estimate the total number of nonresponding hospitals that had groups.
To impute the number of hospitalists in the nonresponding set of hospitals, the additional number of groups was stratified into the 9 US Census Divisions. On the basis of reported data, the average number of hospitalists per group was calculated at the Census Division level. The per‐group value was then applied to the number of additional groups, and the result was added to the total number of reported hospitalists. The Census Division values were then summed to produce the national total. To produce results for all other control groupings, the national total was then apportioned across the categories according to percentage of hospitalists by category on the basis of the reported data.
Analytical Plan
In analyzing the hospitalist movement across the country, we realized there are 2 dimensions of diffusion, which can be characterized as breadth and depth. In the present study:
The measure of breadth is the percentage of hospital medicine groups in a given group of hospitals. In the Results section, this measure is sometimes referred to as penetration.
The measure of depth is the number of hospitalists for each average daily census (ADC) of 100 patients. For instance, for a hospital with an average daily census of 100 that has 4 hospitalists, that measure is 4. To compute this metric for a given category of hospitals (eg, major teaching hospitals), the numerator is the number of hospitalists and the denominator is the ADC at hospitals that have hospital medicine groups. The metric reflects the in‐hospital impact of hospital medicine groups at their hospitals.
Using these 2 measures, it is possible to differentiate between a group of hospitals that has many hospital medicine groups but each group has a minimal impact at the hospital versus a group of hospitals that has few hospital medicine groups but each group has a major impact at the hospital.
The analysis also characterizes the employment status of hospitalists by comparing the proportion of hospitals in each of the employment models by category of hospital.
RESULTS
Diffusion and Impact
Overall, the penetration of hospital medicine groups across the 4895 hospitals in the United States is 29% and the in‐hospital impact at hospitals with hospital medicine groups is 3.93 hospitalists per 100 ADC. The average hospital medicine group has 7.9 hospitalists at a hospital with an ADC of 200.6.
Geographic Categories (Tables 1A and 2A)
The Northeast (46%) and the Pacific (40%) divisions have the greatest penetration of hospital medicine groups. The West North Central Division (16%) has the lowest penetration of hospital medicine groups. Hospital medicine groups in the West South Central Division average 11.1 hospitalists, which partially explains why this region has the greatest in‐hospital impact (6.24 hospitalists per 100 ADC). At the other end of the spectrum are the Middle Atlantic and East South Central divisions with (2.42 and 2.83 hospitalists per 100 ADC, respectively.
Category | Hospitals | Hospital medicine groups | Hospitals with hospital medicine groups (%) |
---|---|---|---|
| |||
Region | |||
1: Northeast | 203 | 94 | 46% |
2: Mid‐Atlantic | 486 | 172 | 35% |
3: South‐Atlantic | 731 | 272 | 37% |
4: East North Central | 732 | 209 | 29% |
5: East South Central | 427 | 92 | 22% |
6: West North Central | 675 | 106 | 16% |
7: West South Central | 737 | 164 | 22% |
8: Mountain | 348 | 83 | 24% |
9: Pacific | 556 | 223 | 40% |
Rural/urban | |||
Rural | 2166 | 235 | 11% |
Small urban | 1285 | 488 | 38% |
Large urban | 1444 | 692 | 48% |
Total | 4895 | 1415 | 29% |
Category | Groups (hospitals) | Hospitalists | Hospitalists per group | Hospitalists per 100 census |
---|---|---|---|---|
| ||||
Region | ||||
1: Northeast | 94 | 669 | 7.1 | 3.62 |
2: Mid‐Atlantic | 172 | 1133 | 6.6 | 2.42 |
3: South Atlantic | 272 | 1933 | 7.1 | 3.21 |
4: East North Central | 209 | 2087 | 10.0 | 4.65 |
5: East South Central | 92 | 433 | 4.7 | 2.83 |
6: West North Central | 106 | 887 | 8.4 | 4.37 |
7: West South Central | 164 | 1828 | 11.1 | 6.24 |
8: Mountain | 83 | 644 | 7.8 | 4.43 |
9: Pacific | 223 | 1546 | 6.9 | 4.56 |
Rural/urban | ||||
Rural | 235 | 893 | 3.8 | 4.85 |
Small urban | 488 | 3236 | 6.6 | 3.03 |
Large urban | 692 | 7030 | 10.2 | 4.43 |
Total | 1415 | 11 159 | 7.9 | 3.93 |
There are more hospital medicine groups in urban locations. The penetration of hospital medicine groups is 48% at hospitals in large metropolitan locations (ie, with a population of more than 1 million), 38% at hospitals in small metropolitan locations, and 11% at hospitals in rural areas. However, rural hospitals have a relatively high in‐hospital impact (4.85 hospitalists per 100 ADC), explained by an average group size of 3.8 and an average ADC of 78.4.
Hospital Size, Control/Ownership, and Teaching Status (Tables 1B and 2B)
The penetration of hospital medicine groups increases as the size of the hospital increases. Six percent of hospitals with 6‐24 beds have groups, whereas 71% of hospitals with 500+ beds have groups. Among hospitals with 200 or more beds, 55% have hospital medicine groups compared to 19% of hospitals with fewer than 200 beds. As would be expected, larger hospitals have larger hospital medicine groups: hospitals with 6‐24 beds average 2.1 hospitalists, whereas hospitals with 500+ beds average 14.2 hospitalists. However, hospitalists have a proportionately greater impact at smaller hospitals. Their greatest impact is at hospitals with 6‐24 beds (46.34 hospitalists per 100 ADC); their smallest impact is at hospitals with 500+ beds (2.47 hospitalists per 100 ADC).
Category | Hospitals | Hospital medicine groups | Hospitals with hospital medicine groups (%) |
---|---|---|---|
| |||
Size | |||
6‐24 beds | 327 | 18 | 6% |
25‐49 beds | 965 | 88 | 9% |
50‐99 beds | 1031 | 168 | 16% |
100‐199 beds | 1168 | 372 | 32% |
200‐299 beds | 624 | 287 | 46% |
300‐399 beds | 349 | 183 | 52% |
400‐499 beds | 172 | 116 | 67% |
500+ beds | 259 | 183 | 71% |
Control | |||
Government | 1121 | 161 | 14% |
Not for profit | 2984 | 1032 | 35% |
For profit | 790 | 222 | 28% |
Teaching status | |||
Nonteaching | 3800 | 823 | 22% |
Other teaching | 779 | 382 | 49% |
Major teaching | 316 | 210 | 66% |
Total | 4895 | 1415 | 29% |
Category | Groups (hospitals) | Hospitalists | Hospitalists per group | Hospitalists per 100 census |
---|---|---|---|---|
| ||||
Size | ||||
6‐24 beds | 18 | 38 | 2.1 | 46.34 |
25‐49 beds | 88 | 260 | 3.0 | 17.94 |
50‐99 beds | 168 | 885 | 5.3 | 12.75 |
100‐199 beds | 372 | 1757 | 4.7 | 5.29 |
200‐299 beds | 287 | 2308 | 8.0 | 4.72 |
300‐399 beds | 183 | 1,553 | 8.5 | 3.29 |
400‐499 beds | 116 | 1751 | 15.1 | 4.35 |
500+ beds | 183 | 2,607 | 14.2 | 2.47 |
Control | ||||
Government | 161 | 1,674 | 10.4 | 5.85 |
Not for profit | 1032 | 8,481 | 8.2 | 3.64 |
For profit | 222 | 1,004 | 4.5 | 4.47 |
Teaching Status | ||||
Nonteaching | 823 | 4,910 | 6.0 | 4.85 |
Other teaching | 382 | 2,678 | 7.0 | 3.25 |
Major teaching | 210 | 3,571 | 17.0 | 3.57 |
Total | 1415 | 11 159 | 7.9 | 3.93 |
Of the 3 categories of control, government groups have the lowest penetration of hospital medicine groups (14%). However, the hospital medicine groups at these government‐controlled hospitals are large (10.4 hospitalists), and they have a significant in‐hospital impact on care at these hospitals (5.85 hospitalists per 100 ADC). Not‐for‐profit hospitals have the highest penetration of hospital medicine groups (35%), whereas hospital medicine groups at for‐profit hospitals have the lowest average size (4.5 hospitalists).
There appears to be a relationship between teaching status and the likelihood that a hospital has a hospital medicine group. The penetration of hospital medicine groups is 66% at major teaching hospitals, 49% at other teaching hospitals, and 22% at nonteaching hospitals. However, nonteaching hospitals have a relatively high in‐hospital impact (4.85 hospitalists per 100 ADC). This is explained by their having an average group size of 6.0, but an average ADC of only 123.0 (compared to 477.0 for major teaching hospitals and 215.7 for other teaching hospitals).
Employment Models
The results of the analysis of hospitalist employment models (data not shown) can be summarized as follows:
Employees of hospitals: This employment model averaged 33% of all groups, with an average size of 9.8 hospitalists. The employees of hospital model was more prevalent in the Mid‐Atlantic (56%), New England (49%), and West North Central (45%) regions and in rural hospitals (45%). The East South Central (16%) and West South Central (12%) regions and for‐profit hospitals (20%) had fewer hospital employee groups.
Employees of medical groups: This employment model averaged 29% of all groups, with an average of 7.4 hospitalists. More hospitals in the East South Central (35%) and New England (34%) regions had this employment model. Fewer hospitals in the Mid‐Atlantic (18%) and West North Central (18%) regions and rural (18%) hospitals had medical group‐based groups.
Employees of independent hospitalist groups: This employment group averaged 25% of all groups and had the smallest mean number of hospitalists (6.9). This employment model was more prevalent in for‐profit hospitals (43%) and was less prevalent in the New England (9%) and Mid‐Atlantic (11%) regions and in major teaching hospitals (11%) and government hospitals (19%).
CONCLUSIONS
Hospital medicine groups appear to have become part of the mainstream delivery of health care. With more than 11 000 hospitalists, the specialty is equivalent in size to the gastroenterology medical specialty.9 Fifty‐five percent of hospitals with more than 200 beds have hospital medicine groups. Furthermore, it appears that the growth of the hospitalist movement has not peaked. It is likely that the number of hospitals with hospital medicine groups will increase and that existing hospital medicine groups will continue to add hospitalists.
No one employment model of hospital medicine group appears to dominate the health care landscape. We expect that there will continue to be diversity among the organizations that choose to establish hospital medicine groups.
In light of this growth and diversity, hospital medicine groups appear to be valued by a wide range of stakeholders in the health care industry. The potential benefits provided by hospitalists include financial savings, improved throughput efficiency, improved quality and safety, improved medical education, and better provider satisfaction.
Despite this success story, the hospitalist movement has maintained a relatively low profile among consumers and some segments of the health care industry. This is likely to change. As the hospital medicine specialty gains recognition, hospitalists will receive increased scrutiny and attention. This emerging specialty will need to be able to clearly define its role and document its performance in the constantly changing health care industry.
ADDENDUM
Subsequent to the acceptance of this manuscript, the authors received results of the 2004 Annual Survey of the American Hospital Association. Some highlights of the new data and comparisons to the 2003 results are as follows:
The penetration of hospitals with hospital medicine groups grew from 29% to 34% (for hospitals with 200+ beds, the penetration grew from 55% to 63%)
An estimated 1,661 hospitals have hospital medicine groups (an increase of 17% from 2003)
The average size of a hospital medicine group decreased from 7.9 physicians to 7.5 physicians (a decrease of 5%)
It is estimated that there are 12,504 hospitalists in the U.S. (an increase of 12% from 2003)
Hospital medicine groups remain equally distributed among the three employment models: employees of hospitals 30%, employees of medical groups 29%, employees of independent hospitalist groups 29%
These updated results indicate strong hospitalist growth over the one year period and continued diversity among hospital medicine programs, reinforcing the conclusions of the manuscript.
APPENDIX
AHA Annual Survey Overview
Conducted since 1946, the AHA Annual Survey is the principal data collection mechanism of the American Hospital Association and is a basic source of data on hospitals in the United States. Its main purpose is to provide a cross‐sectional view of the hospital field each year and to make it possible to monitor hospital performance over time. The information that it gathers from a universe of approximately 5700 hospitals concerns primarily the availability of services, utilization, personnel, finances, and governance. Newly added to the 2003 survey were the following questions regarding hospitalists: Do hospitalists provide care for patients in your hospital? YES □ NO □
Hospitalist is defined as a physician whose primary professional focus is the care of hospitalized medical patients (through clinical, education, administrative and research activity).
If yes, please report the number of full time and part time hospitalists?
Full‐time ______
Part‐time ______
Full‐time equivalent (FTE) is the total number of hours worked by all employees over the full (12 month) reporting period divided by the normal number of hours worked by a full‐time employee for that same period. For example, if your hospital considers a normal workweek for a full‐time employee to be 40 hours, a total of 2080 hours would be worked over a full year (52 weeks). If the total number of hours worked by all employees on the payroll is 208 000, then the number of FTEs is 100 (employees). The FTE calculation for a specific occupational category such as registered nurses is exactly the same. The calculation for each occupational category should be based on the number of hours worked by staff employed in that specific category.
If yes, please select the category below that best describes the employment model for your hospitalists:
□ Independent provider group
□ Employed by your hospital
□ Employed by a physician group
□ Employed by a university or school program
□ Other
It is the results from these questions that are the subject of this analysis and the manuscript.
The term hospitalist was coined in 1996 in an article1 that appeared in the New England Journal of Medicine. Robert M. Wachter, MD, and Lee Goldman, MD, of the University of California, San Francisco, defined hospitalists as hospital‐based physicians who take responsibility for managing medical inpatients. Hospitalists were described as having responsibility for seeing unassigned hospital patients and being available for in‐hospital consultations. Several years later, the Society of Hospital Medicine posted the definition of a hospitalist as someone whose primary professional focus is the medical care of hospitalized patientsin patient care, education, research, and administrative activities.
In January 2002, Wachter and Goldman published a follow‐up article,2 The Hospitalist Movement 5 Years Later, in the Journal of the American Medical Association. This formal review of 19 published studies analyzed the impact of hospital medicine groups on financial and clinical outcomes. Wachter and Goldman concluded, Empirical research supports the premise that hospitalists improve inpatient efficiency without harmful effects on quality or patient satisfaction. These studies indicate an average reduction of cost per stay of 13.4% and an average reduction in length of stay of 16.6%.
The evolution of the hospitalist movement has been fast paced and extensive. Given the recent pace of growth, a scholarly analysis estimated that the mature hospitalist workforce in the United States will eventually total 20,000, making it the equivalent of the cardiology specialty.3 Beyond sheer growth, medical literature has demonstrated positive effects of the hospitalist model on patient quality outcomes, including readmission rates, postoperative complications, and mortality.47
In addition to peer‐reviewed medical literature, there is anecdotal evidence about the growth and effects of the hospitalist movement:
The Society of Hospital Medicine (SHM), the hospitalist professional society, estimated that in 2003 there were 8000 physicians practicing as hospitalists in the United States.8
Twelve of the country's top 15 hospitals have hospital medicine groups.8
As hospital medicine groups have proliferated, 4 major employment models have evolved. Hospitalists can be employees of: 1) a hospital or a hospital subsidiary; 2) a multispecialty or primary care physician group; 3) a medical group (local or national) of independent hospitalists; or 4) a university or medical school. However, there is little published data on the prevalence of each of these hospitalist employment models, nationally or by type of hospital.
To better understand the extent and nature of the hospitalist movement, the American Hospital Association (AHA) utilized its 2003 Annual Survey to gather data on hospital medicine groups in the United States
DATA AND METHODS
The data for our analysis came from the 2003 AHA Annual Survey. Conducted since 1946, this survey is the principal data collection mechanism of the American Hospital Association and is a basic source of data on hospitals in the United States about the availability of services, utilization, personnel, finances, and governance. Its main purpose is to provide a cross‐sectional view of hospitals and hospital performance over time. In the 2003 survey, a series of items were added about hospitalists including whether hospitals had hospital medicine groups, the number of hospitalists operating in such groups, and the employment model used.
The study population for this analysis was limited to US community hospitals (n = 4895). Community hospitals are defined as all adult and pediatric nonfederal, short‐term general, and specialty hospitals whose facilities and services are available to the public. Excluded from the analysis were all federal hospitals, long‐term care hospitals, and psychiatric hospitals.
Imputation of Missing Data
In the 2003 survey, 77% of the 4895 US community hospitals answered the question on specific use of hospitalists. To get a complete picture of the number of groups and hospitalists, we imputed data for the nonresponding hospitals.
We performed logistic regression analysis of data from the responding hospitals to estimate the number of nonresponding hospitals that had a group and the number of hospitalists in these groups. The dependent variable in the regression was whether a hospital had a group, and the independent variables included hospital characteristics for which data were available for all US hospitals, both survey respondents and nonrespondents. The results of the regression analysis were then applied to the data for each nonresponding hospital to estimate its probability of having a group. These probabilities were summed over the various nonresponding hospitals to estimate the total number of nonresponding hospitals that had groups.
To impute the number of hospitalists in the nonresponding set of hospitals, the additional number of groups was stratified into the 9 US Census Divisions. On the basis of reported data, the average number of hospitalists per group was calculated at the Census Division level. The per‐group value was then applied to the number of additional groups, and the result was added to the total number of reported hospitalists. The Census Division values were then summed to produce the national total. To produce results for all other control groupings, the national total was then apportioned across the categories according to percentage of hospitalists by category on the basis of the reported data.
Analytical Plan
In analyzing the hospitalist movement across the country, we realized there are 2 dimensions of diffusion, which can be characterized as breadth and depth. In the present study:
The measure of breadth is the percentage of hospital medicine groups in a given group of hospitals. In the Results section, this measure is sometimes referred to as penetration.
The measure of depth is the number of hospitalists for each average daily census (ADC) of 100 patients. For instance, for a hospital with an average daily census of 100 that has 4 hospitalists, that measure is 4. To compute this metric for a given category of hospitals (eg, major teaching hospitals), the numerator is the number of hospitalists and the denominator is the ADC at hospitals that have hospital medicine groups. The metric reflects the in‐hospital impact of hospital medicine groups at their hospitals.
Using these 2 measures, it is possible to differentiate between a group of hospitals that has many hospital medicine groups but each group has a minimal impact at the hospital versus a group of hospitals that has few hospital medicine groups but each group has a major impact at the hospital.
The analysis also characterizes the employment status of hospitalists by comparing the proportion of hospitals in each of the employment models by category of hospital.
RESULTS
Diffusion and Impact
Overall, the penetration of hospital medicine groups across the 4895 hospitals in the United States is 29% and the in‐hospital impact at hospitals with hospital medicine groups is 3.93 hospitalists per 100 ADC. The average hospital medicine group has 7.9 hospitalists at a hospital with an ADC of 200.6.
Geographic Categories (Tables 1A and 2A)
The Northeast (46%) and the Pacific (40%) divisions have the greatest penetration of hospital medicine groups. The West North Central Division (16%) has the lowest penetration of hospital medicine groups. Hospital medicine groups in the West South Central Division average 11.1 hospitalists, which partially explains why this region has the greatest in‐hospital impact (6.24 hospitalists per 100 ADC). At the other end of the spectrum are the Middle Atlantic and East South Central divisions with (2.42 and 2.83 hospitalists per 100 ADC, respectively.
Category | Hospitals | Hospital medicine groups | Hospitals with hospital medicine groups (%) |
---|---|---|---|
| |||
Region | |||
1: Northeast | 203 | 94 | 46% |
2: Mid‐Atlantic | 486 | 172 | 35% |
3: South‐Atlantic | 731 | 272 | 37% |
4: East North Central | 732 | 209 | 29% |
5: East South Central | 427 | 92 | 22% |
6: West North Central | 675 | 106 | 16% |
7: West South Central | 737 | 164 | 22% |
8: Mountain | 348 | 83 | 24% |
9: Pacific | 556 | 223 | 40% |
Rural/urban | |||
Rural | 2166 | 235 | 11% |
Small urban | 1285 | 488 | 38% |
Large urban | 1444 | 692 | 48% |
Total | 4895 | 1415 | 29% |
Category | Groups (hospitals) | Hospitalists | Hospitalists per group | Hospitalists per 100 census |
---|---|---|---|---|
| ||||
Region | ||||
1: Northeast | 94 | 669 | 7.1 | 3.62 |
2: Mid‐Atlantic | 172 | 1133 | 6.6 | 2.42 |
3: South Atlantic | 272 | 1933 | 7.1 | 3.21 |
4: East North Central | 209 | 2087 | 10.0 | 4.65 |
5: East South Central | 92 | 433 | 4.7 | 2.83 |
6: West North Central | 106 | 887 | 8.4 | 4.37 |
7: West South Central | 164 | 1828 | 11.1 | 6.24 |
8: Mountain | 83 | 644 | 7.8 | 4.43 |
9: Pacific | 223 | 1546 | 6.9 | 4.56 |
Rural/urban | ||||
Rural | 235 | 893 | 3.8 | 4.85 |
Small urban | 488 | 3236 | 6.6 | 3.03 |
Large urban | 692 | 7030 | 10.2 | 4.43 |
Total | 1415 | 11 159 | 7.9 | 3.93 |
There are more hospital medicine groups in urban locations. The penetration of hospital medicine groups is 48% at hospitals in large metropolitan locations (ie, with a population of more than 1 million), 38% at hospitals in small metropolitan locations, and 11% at hospitals in rural areas. However, rural hospitals have a relatively high in‐hospital impact (4.85 hospitalists per 100 ADC), explained by an average group size of 3.8 and an average ADC of 78.4.
Hospital Size, Control/Ownership, and Teaching Status (Tables 1B and 2B)
The penetration of hospital medicine groups increases as the size of the hospital increases. Six percent of hospitals with 6‐24 beds have groups, whereas 71% of hospitals with 500+ beds have groups. Among hospitals with 200 or more beds, 55% have hospital medicine groups compared to 19% of hospitals with fewer than 200 beds. As would be expected, larger hospitals have larger hospital medicine groups: hospitals with 6‐24 beds average 2.1 hospitalists, whereas hospitals with 500+ beds average 14.2 hospitalists. However, hospitalists have a proportionately greater impact at smaller hospitals. Their greatest impact is at hospitals with 6‐24 beds (46.34 hospitalists per 100 ADC); their smallest impact is at hospitals with 500+ beds (2.47 hospitalists per 100 ADC).
Category | Hospitals | Hospital medicine groups | Hospitals with hospital medicine groups (%) |
---|---|---|---|
| |||
Size | |||
6‐24 beds | 327 | 18 | 6% |
25‐49 beds | 965 | 88 | 9% |
50‐99 beds | 1031 | 168 | 16% |
100‐199 beds | 1168 | 372 | 32% |
200‐299 beds | 624 | 287 | 46% |
300‐399 beds | 349 | 183 | 52% |
400‐499 beds | 172 | 116 | 67% |
500+ beds | 259 | 183 | 71% |
Control | |||
Government | 1121 | 161 | 14% |
Not for profit | 2984 | 1032 | 35% |
For profit | 790 | 222 | 28% |
Teaching status | |||
Nonteaching | 3800 | 823 | 22% |
Other teaching | 779 | 382 | 49% |
Major teaching | 316 | 210 | 66% |
Total | 4895 | 1415 | 29% |
Category | Groups (hospitals) | Hospitalists | Hospitalists per group | Hospitalists per 100 census |
---|---|---|---|---|
| ||||
Size | ||||
6‐24 beds | 18 | 38 | 2.1 | 46.34 |
25‐49 beds | 88 | 260 | 3.0 | 17.94 |
50‐99 beds | 168 | 885 | 5.3 | 12.75 |
100‐199 beds | 372 | 1757 | 4.7 | 5.29 |
200‐299 beds | 287 | 2308 | 8.0 | 4.72 |
300‐399 beds | 183 | 1,553 | 8.5 | 3.29 |
400‐499 beds | 116 | 1751 | 15.1 | 4.35 |
500+ beds | 183 | 2,607 | 14.2 | 2.47 |
Control | ||||
Government | 161 | 1,674 | 10.4 | 5.85 |
Not for profit | 1032 | 8,481 | 8.2 | 3.64 |
For profit | 222 | 1,004 | 4.5 | 4.47 |
Teaching Status | ||||
Nonteaching | 823 | 4,910 | 6.0 | 4.85 |
Other teaching | 382 | 2,678 | 7.0 | 3.25 |
Major teaching | 210 | 3,571 | 17.0 | 3.57 |
Total | 1415 | 11 159 | 7.9 | 3.93 |
Of the 3 categories of control, government groups have the lowest penetration of hospital medicine groups (14%). However, the hospital medicine groups at these government‐controlled hospitals are large (10.4 hospitalists), and they have a significant in‐hospital impact on care at these hospitals (5.85 hospitalists per 100 ADC). Not‐for‐profit hospitals have the highest penetration of hospital medicine groups (35%), whereas hospital medicine groups at for‐profit hospitals have the lowest average size (4.5 hospitalists).
There appears to be a relationship between teaching status and the likelihood that a hospital has a hospital medicine group. The penetration of hospital medicine groups is 66% at major teaching hospitals, 49% at other teaching hospitals, and 22% at nonteaching hospitals. However, nonteaching hospitals have a relatively high in‐hospital impact (4.85 hospitalists per 100 ADC). This is explained by their having an average group size of 6.0, but an average ADC of only 123.0 (compared to 477.0 for major teaching hospitals and 215.7 for other teaching hospitals).
Employment Models
The results of the analysis of hospitalist employment models (data not shown) can be summarized as follows:
Employees of hospitals: This employment model averaged 33% of all groups, with an average size of 9.8 hospitalists. The employees of hospital model was more prevalent in the Mid‐Atlantic (56%), New England (49%), and West North Central (45%) regions and in rural hospitals (45%). The East South Central (16%) and West South Central (12%) regions and for‐profit hospitals (20%) had fewer hospital employee groups.
Employees of medical groups: This employment model averaged 29% of all groups, with an average of 7.4 hospitalists. More hospitals in the East South Central (35%) and New England (34%) regions had this employment model. Fewer hospitals in the Mid‐Atlantic (18%) and West North Central (18%) regions and rural (18%) hospitals had medical group‐based groups.
Employees of independent hospitalist groups: This employment group averaged 25% of all groups and had the smallest mean number of hospitalists (6.9). This employment model was more prevalent in for‐profit hospitals (43%) and was less prevalent in the New England (9%) and Mid‐Atlantic (11%) regions and in major teaching hospitals (11%) and government hospitals (19%).
CONCLUSIONS
Hospital medicine groups appear to have become part of the mainstream delivery of health care. With more than 11 000 hospitalists, the specialty is equivalent in size to the gastroenterology medical specialty.9 Fifty‐five percent of hospitals with more than 200 beds have hospital medicine groups. Furthermore, it appears that the growth of the hospitalist movement has not peaked. It is likely that the number of hospitals with hospital medicine groups will increase and that existing hospital medicine groups will continue to add hospitalists.
No one employment model of hospital medicine group appears to dominate the health care landscape. We expect that there will continue to be diversity among the organizations that choose to establish hospital medicine groups.
In light of this growth and diversity, hospital medicine groups appear to be valued by a wide range of stakeholders in the health care industry. The potential benefits provided by hospitalists include financial savings, improved throughput efficiency, improved quality and safety, improved medical education, and better provider satisfaction.
Despite this success story, the hospitalist movement has maintained a relatively low profile among consumers and some segments of the health care industry. This is likely to change. As the hospital medicine specialty gains recognition, hospitalists will receive increased scrutiny and attention. This emerging specialty will need to be able to clearly define its role and document its performance in the constantly changing health care industry.
ADDENDUM
Subsequent to the acceptance of this manuscript, the authors received results of the 2004 Annual Survey of the American Hospital Association. Some highlights of the new data and comparisons to the 2003 results are as follows:
The penetration of hospitals with hospital medicine groups grew from 29% to 34% (for hospitals with 200+ beds, the penetration grew from 55% to 63%)
An estimated 1,661 hospitals have hospital medicine groups (an increase of 17% from 2003)
The average size of a hospital medicine group decreased from 7.9 physicians to 7.5 physicians (a decrease of 5%)
It is estimated that there are 12,504 hospitalists in the U.S. (an increase of 12% from 2003)
Hospital medicine groups remain equally distributed among the three employment models: employees of hospitals 30%, employees of medical groups 29%, employees of independent hospitalist groups 29%
These updated results indicate strong hospitalist growth over the one year period and continued diversity among hospital medicine programs, reinforcing the conclusions of the manuscript.
APPENDIX
AHA Annual Survey Overview
Conducted since 1946, the AHA Annual Survey is the principal data collection mechanism of the American Hospital Association and is a basic source of data on hospitals in the United States. Its main purpose is to provide a cross‐sectional view of the hospital field each year and to make it possible to monitor hospital performance over time. The information that it gathers from a universe of approximately 5700 hospitals concerns primarily the availability of services, utilization, personnel, finances, and governance. Newly added to the 2003 survey were the following questions regarding hospitalists: Do hospitalists provide care for patients in your hospital? YES □ NO □
Hospitalist is defined as a physician whose primary professional focus is the care of hospitalized medical patients (through clinical, education, administrative and research activity).
If yes, please report the number of full time and part time hospitalists?
Full‐time ______
Part‐time ______
Full‐time equivalent (FTE) is the total number of hours worked by all employees over the full (12 month) reporting period divided by the normal number of hours worked by a full‐time employee for that same period. For example, if your hospital considers a normal workweek for a full‐time employee to be 40 hours, a total of 2080 hours would be worked over a full year (52 weeks). If the total number of hours worked by all employees on the payroll is 208 000, then the number of FTEs is 100 (employees). The FTE calculation for a specific occupational category such as registered nurses is exactly the same. The calculation for each occupational category should be based on the number of hours worked by staff employed in that specific category.
If yes, please select the category below that best describes the employment model for your hospitalists:
□ Independent provider group
□ Employed by your hospital
□ Employed by a physician group
□ Employed by a university or school program
□ Other
It is the results from these questions that are the subject of this analysis and the manuscript.
- The emerging role of “hospitalists” in the American health care system.N Eng J Med.1996;335:514–517. , .
- The hospitalist movement 5 years later.JAMA.2002;287:487–494. , .
- The potential size of the hospitalist workforce in the United StatesAm J Med.1999;106:441–445. , , , , .
- Implementation of a hospitalist service at a community hospital: evolution of service utilization, costs, and patient outcomes [abstract]. National Association of Inpatient Physicians, 3rd Annual Meeting. Philadelphia, Penn, April 11‐12,2000. .
- Decreased length of stay, costs, and mortality in a randomized trial of academic hospitalists [abstract]. National Association of Inpatient Physicians, 4th Annual Meeting, Atlanta, GA, March 27‐28,2001. , , , , , .
- The effect of full‐time faculty hospitalists on the efficiency of care at a community teaching hospital.Ann Intern Med.1998;129:197–203. , .
- Program description: a hospitalist run, medical short‐stay unit in a teaching hospital.CMAJ.2000;163:1477–1480. , , , .
- Society of Hospital Medicine. Growth of hospital medicine nationwide. July 2003. Available at: http://www.hospitalmedicine.org/presentation/apps/indlist/intro.asp?flag=18. Accessed February2005.
- American Medical Association.Physician characteristics and distribution in the US, 2004.Chicago, Ill:American Medical Association,2004.
- The emerging role of “hospitalists” in the American health care system.N Eng J Med.1996;335:514–517. , .
- The hospitalist movement 5 years later.JAMA.2002;287:487–494. , .
- The potential size of the hospitalist workforce in the United StatesAm J Med.1999;106:441–445. , , , , .
- Implementation of a hospitalist service at a community hospital: evolution of service utilization, costs, and patient outcomes [abstract]. National Association of Inpatient Physicians, 3rd Annual Meeting. Philadelphia, Penn, April 11‐12,2000. .
- Decreased length of stay, costs, and mortality in a randomized trial of academic hospitalists [abstract]. National Association of Inpatient Physicians, 4th Annual Meeting, Atlanta, GA, March 27‐28,2001. , , , , , .
- The effect of full‐time faculty hospitalists on the efficiency of care at a community teaching hospital.Ann Intern Med.1998;129:197–203. , .
- Program description: a hospitalist run, medical short‐stay unit in a teaching hospital.CMAJ.2000;163:1477–1480. , , , .
- Society of Hospital Medicine. Growth of hospital medicine nationwide. July 2003. Available at: http://www.hospitalmedicine.org/presentation/apps/indlist/intro.asp?flag=18. Accessed February2005.
- American Medical Association.Physician characteristics and distribution in the US, 2004.Chicago, Ill:American Medical Association,2004.
Copyright © 2006 Society of Hospital Medicine