User login
Dyspnea Assessment and Management Survey
Dyspnea, defined as a subjective experience of breathing discomfort,[1] is the seventh most frequent reason adult patients present to the emergency room and the most frequent cause for emergency room visits in patients 65 years or older.[2] Moreover, dyspnea is experienced by 49% of patients hospitalized with a medical condition[3, 4, 5] and by 70% of patients who are seriously ill.[6]
Based on evidence that patients are not treated consistently and effectively for relief of their shortness of breath, the American College of Chest Physicians (ACCP) statement on dyspnea management in patients with advanced lung or heart disease recommended that patients should be asked to rate their dyspnea, and the rating should be routinely documented in the medical record to guide management.[7] Although clinicians may question the utility of routine assessment of dyspnea using a standardized scale, studies have found that the prevalence of dyspnea reported from chart review is much lower than when patients are directly interviewed.[8] This may be the result of underrecognition of dyspnea or poor documentation by physicians, or that patients may not communicate their symptoms unless the physician specifically asks. As is the case with pain, routine assessment of dyspnea severity could lead to improved clinical management and greater patient‐centered care. However, unlike in the case of pain, regulatory bodies, such as the Joint Commission for Accreditation of Healthcare Organization, do not require routine dyspnea assessment.[9]
Currently, there are more than 40,000 hospitalists in the United States, and the vast majority of hospitals with >200 beds have a hospitalist group.[10] Hospitalists care for over 60% of inpatients[11] and play a major role in the management of patients with acute cardiopulmonary diseases. If standardized approaches for the assessment and documentation of dyspnea are to be implemented, hospitalists would be a key stakeholder group for utilizing enhanced clinical information about dyspnea. Therefore, we evaluated attitudes and practices of hospitalists in regard to the assessment and management of dyspnea, including the potential benefits and challenges related to the implementation of standardized assessment. We hypothesized that hospitalists would believe that a dyspnea scale for assessment of severity could improve their management of patients with cardiovascular diseases. Further, we hypothesized that physicians who agreed with the general statement that dyspnea is an important clinical problem would be more likely to believe that routine dyspnea assessment would be valuable.
METHODS
Study Sample
We invited 255 attending hospitalists from 9 geographically and structurally diverse hospitals to complete a survey about the assessment and management of dyspnea. The 9 hospitals represent range of practice environments including 4 academic medical centers, 2 community teaching and 3 nonteaching hospitals, 1 Veterans Administration hospital, and 2 staff‐model HMOs (see Supporting Table 1 in the online version of this article). The survey was distributed online using REDCap (Research Electronic Data Capture), a secure web‐based interface application.[12] A coinvestigator who was a pulmonary critical‐care physician at each site sent an initial email to their hospitalist groups that alerted them to expect a survey from the principal investigator. This notification was subsequently followed by an email invitation containing an informational cover letter and a link to the online survey. The cover letter stated that the completed surveys would not be stored at the local sites and that all the analyzed data would be deidentified. Nonrespondents were sent reminders at 2 and 4 weeks after the initial mailing. A $25 electronic gift card was provided as a gesture of appreciation for their time. The survey was conducted between September 2013 and December 2013.
The study was approved by the Baystate Health Institutional Review Board, Springfield, Massachusetts, with a waiver for written informed consent.
Questionnaire
We developed a 17‐item instrument based on a review of the dyspnea literature and a prior ACCP survey.[12] Questions were piloted with 4 hospitalists at a single institution and modified to improve face validity and clarity (see Supporting Information in the online version of this article for the full survey).
Hospitalists were asked to consider the care of patients admitted for acute cardiopulmonary disease, including heart failure, chronic obstructive pulmonary disease, and pneumonia. A series of 5‐point Likert scales were used to assess the respondents level of agreement with statements related to the following domains: the importance of dyspnea in clinical care, the potential benefits and challenges of routine dyspnea assessment (statements such as: Having a standardized assessment of dyspnea severity would be helpful in management of patients with cardiopulmonary diseases. Dyspnea assessment by a scale should be part of the vital signs for patients with cardiopulmonary diseases.), and management of dyspnea (questions regarding the use of opioids and other nonpharmacological therapies). Additional questions were asked about current assessment practices (questions such as: How often do you assess severity of dyspnea? What is your approach in assessing dyspnea? with options of choosing a categorical or numerical scale), if dyspnea is assessed in their institution by nurses and how often, and the influence of dyspnea severity assessment on their management. The survey had 1 question that solicited comments from the participants: If you don't think that it would be useful to have a standardized dyspnea assessment, please tell us why.
Data Analysis
Responses to survey questions were summarized via counts and percentages in each response category. Adopting the methodology used in the ACCP consensus statement, strongly agree and somewhat agree were combined into a single category of agreement. We also presented percentage of responses in the 2 levels of agreement (strongly agree and somewhat agree) for each question in a bar graph.
Associations between tertiles of physicians' time in practice and attitude toward dyspnea were evaluated via 2 or Fisher exact test.
To examine how answers to the first 2 questions, which assessed attitude toward importance of dyspnea in clinical care, affect answers to the remaining questions, we grouped respondents in 3 categories (strongly agree, agree to these questions, do not agree) and tested the associations using 2 or Fisher exact test.
All analyses were performed using SAS version 9.3 (SAS institute, Inc., Cary, NC) and Stata release 13.1 (StataCorp, College Station, TX).
RESULTS
Overall, 178 (69.8%) of 255 identified hospitalists completed the survey, and all 9 participating hospitals had a response rate greater than 50%. The median number of years in practice was 6 (range, 038 years). A majority (77.5%) of respondents agreed with the statement that dyspnea is 1 of the major symptoms of patients with cardiopulmonary disease, and that its treatment is central to the management of these patients (77.0%) (Figure 1).
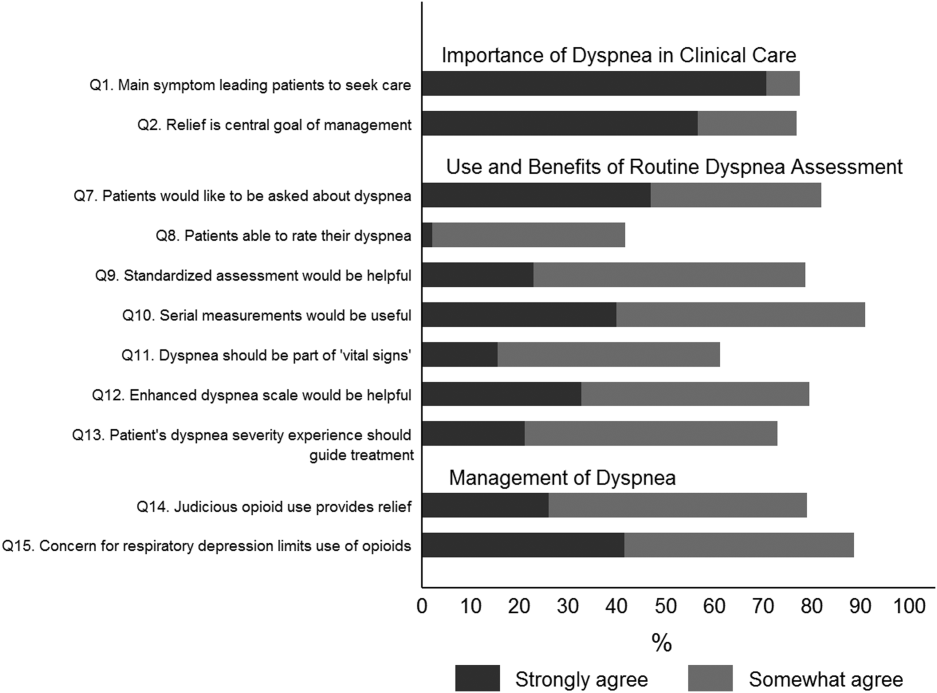
Attitude and Practices Surrounding Dyspnea Assessment
When asked about their current assessment of dyspnea, a majority (84.3%) of the hospitalists stated that they assess dyspnea on a daily basis; two‐thirds indicated that they use a categorical scale (ie, no shortness of breath, improved or worsened compared with a prior date), and one‐third indicated that they ask whether the patient is dyspneic or not. Fifty‐six percent of hospitalists stated that dyspnea is regularly assessed by nurses in their hospital.
The majority of respondents agreed (78.6%, 23.0% strongly and 55.6% somewhat agree) that standardized assessment of dyspnea severity, using a numeric scale and serial measurements as part of the vital signs, would benefit the management of patients with cardiopulmonary diseases. Furthermore, 79.6% (33.0% strongly and 46.6% somewhat agree) reported that using a dyspnea scale that included information to further characterize the patient‐reported experience, such as the level of distress associated with dyspnea, would be helpful in management.
Approximately 90% of the hospitalists indicated that awareness of dyspnea severity has an influence on clinical decision making, including whether to intensify treatment of underlying conditions, to pursue additional diagnostic testing, or to modify discharge timing. Additionally, two‐thirds of hospitalists agreed that awareness of dyspnea severity influences their decision to add opioids, whereas only one‐third prescribed nonpharmacologic symptom‐oriented treatment (Table 1).
Frequency (%) | |
---|---|
| |
When caring for patients with acute cardiopulmonary diseases, how often do you assess severity of dyspnea?* | |
At admission | 66 (37.1) |
At discharge | 59 (33.2) |
Daily until discharge | 150 (84.3) |
More often than daily | 58 (32.6) |
Which description best characterizes your approach to assessing dyspnea severity? | |
I don't regularly ask about dyspnea severity | 3 (1.7) |
I ask the patient whether or not they are having shortness of breath | 50 (28.3) |
I ask the patient to rate the severity of shortness of breath using a numeric scale | 4 (2.3) |
I ask the patient to rate the severity of shortness of breath using a categorical scale (eg, somewhat SOB, no SOB, improved or worsened compared with a prior date) | 120 (67.8) |
When is dyspnea severity assessed and documented by nursing at your hospital?* | |
Dyspnea is not routinely assessed | 60 (33.7) |
At admission | 30 (16.9) |
Daily | 43 (24.2) |
Each shift | 64 (36.0) |
Awareness of dyspnea severity affects my management by:* | |
Influencing my decision to intensify treatment of the patient's underlying condition | 170 (95.5) |
Influencing my decision to pursue additional diagnostic testing | 160 (89.9) |
Influencing my decision to add pharmacologic‐based, symptom‐oriented treatment for dyspnea, such as opioids | 115 (64.6) |
Influencing my decision to add nonpharmacologic‐based, symptom‐oriented treatment for dyspnea, such as fans or pursed lip breathing technique | 58 (32.6) |
Influencing my decision regarding timing of discharge | 162 (91.0) |
Which of the following nonpharmacologic therapies are effective for the relief of dyspnea?* | |
Pursed lip breathing | 113 (63.5) |
Relaxation techniques | 137 (77.0) |
Noninvasive ventilation | 143 (80.3) |
O2 for nonhypoxemic patients | 89 (50.0) |
Cool air/fan | 125 (70.2) |
Cognitive behavioral strategies | 101 (56.7) |
Forty‐two percent of the respondents agreed that patients are able to rate their dyspnea on a scale (2.3% strongly agree and 40.0% agree), and 73.0% indicated that patient experience of dyspnea should guide management independent of physiologic measures such as respiratory rate and oxygen saturation (Figure 1).
Several potential barriers were identified among the 18 participants who did not think that a standardized assessment of dyspnea would be beneficial, including concerns that (1) a dyspnea severity scale is too subjective and numerical scales are not useful for a subjective symptom (19.0%), (2) patients may overrate their symptom or will not be able to rate their dyspnea using a scale (31.0%), or (3) categorical description is sufficient (31.0%).
Practices in Dyspnea Management
Seventy‐nine percent of respondents agreed with the statement that judicious use of opioids can provide relief of dyspnea (26.1% strongly and 52.8% agreed), and 88.7% hospitalists identified the risk of respiratory depression as 1 of the barriers for the limited use of opioids. The majority of physicians (60%80%) considered nonpharmacologic therapies effective for symptomatic treatment of dyspnea, including in the order of agreement: noninvasive ventilation, relaxation techniques, cool air/fan, use of pursed lip breathing, and oxygen for nonhypoxemic patients (Table 1).
Physician Experience and Attitudes Toward Dyspnea Management
When we stratified hospitalists in tertiles of median years of time in practice (median [range]: 2 [04], 6 [58] and 15 [938]), we did not find an association with any of the responses to the questions.
Attitude Regarding the Importance of Dyspnea in Clinical Care and Responses to Subsequent Questions
Respondents who strongly agree or agree that dyspnea is the primary presenting symptom in patients with cardiovascular condition and that dyspnea relief is central to the management of these patients were more likely to believe that patients would like to be asked about their dyspnea (61.2% vs 30.2% vs 29.7%). They also had a more positive attitude about the usefulness of a standardized assessment of dyspnea and the inclusion of the assessment of dyspnea by a scale in the vital signs (Table 2).
Description | Do Not Agree, n (%) | Somewhat Agree, n (%) | Strongly Agree, n (%) | P Value* |
---|---|---|---|---|
| ||||
37 (20.9) | 43 (24.3) | 97 (54.8) | ||
Which description best characterizes your approach to assessing dyspnea severity? | 0.552 | |||
I don't regularly ask about dyspnea severity | 0 (0) | 0 (0) | 3 (3.1) | |
I ask the patient whether or not they are having shortness of breath | 11 (29.7) | 14 (32.6) | 25 (25.8) | |
I ask the patient to rate the severity of shortness of breath using a numeric scale | 2 (5.4) | 1 (2.3) | 1 (1.0) | |
I ask the patient to rate the severity of shortness of breath using a categorical scale (e.g., somewhat shortness of breath, no shortness of breath, improved or worsened compared with a prior date) | 24 (64.9) | 28 (65.1) | 68 (70.1) | |
Patients would like me to ask them about their dyspnea. | <0.0001 | |||
Somewhat agree | 9 (24.3) | 21 (48.8) | 32 (32.7) | |
Strongly agree | 11 (29.7) | 13 (30.2) | 60 (61.2) | |
Patients are able to rate their own dyspnea intensity on a scale of 0‐10. | 0.432 | |||
Somewhat agree | 12 (32.4) | 16 (37.2) | 42 (43.3) | |
Strongly agree | 2 (5.4) | 0 (0) | 2 (2.1) | |
Having a standardized assessment of dyspnea severity would be helpful to me in management of patients with cardiopulmonary diseases. | 0.026 | |||
Somewhat agree | 17 (46.0) | 25 (58.1) | 57 (58.2) | |
Strongly agree | 7 (18.9) | 6 (14.0) | 28 (28.6) | |
Serial measurements of dyspnea would be useful for assessing response to therapy. | 0.042 | |||
Somewhat agree | 14 (37.8) | 28 (65.1) | 48 (49.5) | |
Strongly agree | 16 (43.2) | 12 (27.9) | 43 (44.3) | |
Dyspnea assessment by a scale should be part of the vital signs for patients with cardiopulmonary diseases. | 0.042 | |||
Somewhat agree | 13 (35.1) | 17 (39.5) | 51 (52.0) | |
Strongly agree | 4 (10.8) | 5 (11.6) | 19 (19.4) | |
Using an enhanced dyspnea scale that includes information about the following 4 features 1) Current dyspnea severity, 2) Worst dyspnea ever, 3) Improvement of dyspnea since admission, 4) Acceptability of current level of dyspnea, would be more helpful for my management than a single question focused on dyspnea severity. | 0.03 | |||
Somewhat agree | 14 (40.0) | 24 (55.8) | 44 (44.9) | |
Strongly agree | 9 (25.7) | 9 (20.9) | 40 (40.8) | |
The patients experience of dyspnea should be used to guide treatment decisions independent of objective measures such as respiratory rate and oxygen saturation. | 0.10 | |||
Somewhat agree | 20 (54.0) | 21 (48.8) | 51 (52.0) | |
Strongly agree | 5 (13.5) | 6 (14.0) | 27 (27.6) | |
Judicious use of oral and/or parenteral opioids can provide relief of dyspnea. | 0.21 | |||
Somewhat agree | 20 (54.0) | 23 (54.8) | 50 (51.6) | |
Strongly agree | 10 (27.0) | 6 (14.3) | 30 (30.9) | |
Limited use of opioids for relief of dyspnea in patients with advanced cardiopulmonary disorders is often due to concerns of respiratory depression. | 0.71 | |||
Somewhat agree | 17 (46.0) | 23 (54.8) | 43 (43.9) | |
Strongly agree | 15 (40.5) | 14 (33.3) | 45 (45.9) |
DISCUSSION
In this survey of 178 most hospitalists from a diverse group of 9 US hospitals, we found that most indicate that severity of dyspnea has a profound influence on their clinical practice (including their decision whether to intensify treatments such as diuretics or bronchodilators, to pursue additional diagnostic testing, add opioids or other nonpharmacological treatments) and ultimately their decision regarding the timing of hospital discharge. More importantly, whereas less than half reported experience with standardized assessment of dyspnea severity, most stated that such data would be very useful in their practice.
Despite being a highly prevalent symptom in diverse patient populations, several studies have shown that documentation of dyspnea is sporadic and evaluation of dyspnea quality of care is not routinely performed.[13, 14, 15] Statements from a number of professional societies, including the ACCP, the American Thoracic Society and the Canadian Respiratory Society, recommend that dyspnea management should rely on patient reporting, and that dyspnea severity should be recorded.[1, 4, 7] Assessment is an essential step to guide interventions; however, simply asking about the presence or absence of dyspnea is insufficient.
Several rating scales have been validated and might be implementable in the acute care setting, including the Numerical Rating Scale and the Visual Assessment Scale.[16, 17, 18, 19, 20] Our survey shows that standardized documentation of dyspnea severity in clinical practice is uncommon. However, most hospitalists in our study believed that assessment of dyspnea, using a standardized scale, would positively impact their management of patients with cardiopulmonary disease.
There are a number of potential benefits of routine assessment of dyspnea in hospitalized patients. Implementation of a standardized approach to dyspnea measurement would result in more uniform assessment and documentation practices, and in turn greater awareness among members of the patient‐care team. Though not sufficient to improve care, measurement is necessary because physicians do not always recognize the severity of patients' dyspnea or may not recognize its presence. A retrospective study that assessed the prevalence of symptoms in 410 ambulatory patients showed that one‐quarter of patients had dyspnea, but only half of them told their doctor about it.[21] Two other studies of patients with cancer diagnoses found that 30%70% of patients had dyspnea, but the symptom was recognized in only half of them; even when recognized, dyspnea severity was frequently underrated by physicians.[21, 22] Importantly, underestimation appears to correlate with underutilization of symptomatic management of dyspnea.[8]
Although the results of our survey are encouraging, they highlight a number of potential barriers and misconceptions among hospitalists. For example, although dyspnea can be characterized only by the person experiencing it, only 42% of our survey respondents believed that patients are able to rate their dyspnea intensity on a scale. Some of these responses may be influenced by the fact that dyspnea scales are not currently available to patients under their care. Another explanation is that similar to the case for pain, some hospitalists may believe that patients will exaggerate dyspnea severity. Almost one‐third of the respondents stated that objective measures, such as respiratory rate or oxygen saturation, are more important than a patient's experience of dyspnea in guiding the treatment, and that dyspnea is a subjective symptom and not a vital sign itself. Hospitalists who appreciated the importance of dyspnea in clinical practice were more likely to support the implementation of a standardized dyspnea scale for dyspnea assessment.
Although the potential benefits of including routine measurement of dyspnea in standard hospital practice may seem obvious, evidence that implementing routine assessment improves patient care or outcomes is lacking. Even if hospitalists see the value of dyspnea assessment, asking nurses to collect and document additional information would represent a substantial change in hospital workflow. Finally, without specific protocols to guide care, it is unclear whether physicians will be able to use new information about dyspnea severity effectively. Future studies need to evaluate the impact of implementing routine dyspnea assessment on the management of patients with cardiopulmonary diseases including the use of evidence‐based interventions and reducing the use of less valuable care.
Most hospitalists agreed with the basic principles of dyspnea treatment in patients with advanced cardiopulmonary disease after the primary disease had been stabilized. Effective measures are available, and several guidelines endorse opioids in dyspnea management.[1, 4, 7] However, many clinicians are uncomfortable with this approach for dyspnea, and opioids remain underused. In our study, almost 90% of physicians recognized that concerns about respiratory depression limits opioids use as a treatment. A qualitative study that explored the physicians' perspective toward opioids showed that most physicians were reluctant to prescribe opioids for refractory dyspnea, describing a lack of related knowledge and experience, and fears related to the potential adverse effects. The findings of our study also outline the need to better educate residents and hospitalists on the assessment and management of dyspnea, including prescribing opioids for refractory dyspnea.[23]
Study Strengths and Limitations
This study has several strengths. To our knowledge, it is the first to explore hospitalists' perspectives on incorporating dyspnea assessment in their clinical practice. Hospitalists are the attending physicians for a large majority of inpatients and would be the main users of a dyspnea severity scale. Our questionnaire survey included a large number of hospitalists, from 9 geographically and structurally diverse hospitals, which increased the generalizability of the findings to other hospitals around the country.
The study also has several limitations that need be kept in mind in interpreting the study results. First, desirability bias may have exaggerated some of the positive views expressed by hospitalists toward implementation of routine assessment of dyspnea. Second, because this was a survey, the estimates of dyspnea assessment and documentation practices of both physicians and nurses were based on the respondents' perception and not an objective review of medical records, and the results may be different from actual practice. Third, this was not a population‐based random sample of hospitalists, and it may not be entirely representative; however, those surveyed were from a diverse set of sites with different geographical location, size, academic affiliation, and practice environment, and their time in practice varied widely. Last, we do not have information on nonrespondents, and there is a possibility of nonresponse bias, although the high response rate lessens the risk.
CONCLUSIONS
The results of this survey suggest that most hospitalists believe that routine assessment of dyspnea severity would enhance their clinical decision making and improve patient care. Standardized assessment of dyspnea might result in better awareness of this symptom among providers, reduce undertreatment and mistreatment, and ultimately result in better outcomes for patients. However, implementation of the routine assessment of dyspnea would change current clinical practices and may have a significant effect on existing nursing and physician workflows. Additional research is needed to determine the feasibility and impact on outcomes of routine dyspnea assessment.
Acknowledgements
The authors wish to acknowledge Ms. Anu Joshi for her help with editing the manuscript and assisting with table preparations.
Disclosures
Dr. Stefan is supported by grant K01HL114631‐01A1 from the National Heart, Lung, and Blood Institute of the National Institutes of Health, and by the National Center for Research Resources and the National Center for Advancing Translational Sciences, National Institutes of Health, through grant UL1RR025752. The funder had no role in the design and conduct of the study; collection, management, analysis, and interpretation of the data; preparation, review, or approval of the manuscript; and decision to submit the manuscript for publication. M.S.S. and P.K.L. conceived of the study. M.S.S. acquired the data with the help of all collaborators. M.S.S., P.K.L., P.S.P., and A.P. analyzed and interpreted the data. M.S.S. drafted the manuscript. All authors critically reviewed the manuscript for intellectual content. M.S.S., P.K.L., and A.P. had full access to all of the data in the study and take responsibility for the integrity of the data and the accuracy of the data analysis. M.S.S. is the guarantor for this article, and is responsible for the content of the article, including data and analysis. The authors report no conflicts of interest.
- An Official American Thoracic Society Statement: Update on the Mechanisms, Assessment, and Management of Dyspnea. Am J Respir Crit Care Med. 2012;185(4):435–452. , , , et al.
- CDC/ National Center for Health Statistics. National Hospital Amulatory Medical Care Survey: 2011 Emergency Department Summary Tables. http://www.cdc.gov/nchs/data/ahcd/nhamcs_emergency/2011_ed_web_tables.pdf. Accessed May 15, 2015.
- Signs and symptoms of heart failure: are you asking the right questions? Am J Crit Care. 2010;19(5):443–452. , , , .
- Managing dyspnea in patients with advanced chronic obstructive pulmonary disease: a Canadian Thoracic Society clinical practice guideline. Can Respir J. 2011;18(2):69–78. , , , et al.
- Prevalence of distressing symptoms in hospitalised patients on medical wards: A cross‐sectional study. BMC Palliat Care. 2008;7:16. , .
- Dyspnea in terminally ill cancer patients. Chest. 1986;89(2):234–236. , .
- American College of Chest Physicians consensus statement on the management of dyspnea in patients with advanced lung or heart disease. Chest. 2010;137(3):674–691. , , , et al.
- Common symptoms in ambulatory care: incidence, evaluation, therapy, and outcome. Am J Med. 1989;86(3):262–266. , .
- The Joint Commission. Facts about Pain Management. http://www.jointcommission.org/pain_management/. Accessed May, 15, 2015.
- Hospitalist programs in the age of healthcare reform. J Healthc Manag. 2010;55(6):378–380. .
- The Use of Hospitalists by Small Rural Hospitals: Results of a National Survey. Med Care Res Rev. 2014;71(4):356–366. , , , .
- Tufts CTSI. REDCap [Internet]. Tufts Clinical and Translational Science Institute. http://www.tuftsctsi.org/Services-and-Consultation/REDCap.aspx. Accessed May, 15, 2015.
- Multi‐dimensional Assessment of Dyspnea. Dyspnoea in Advanced Disease: A guide to clinical management; 2005. , .
- Cancer care quality measures: symptoms and end‐of‐life care. Evid Rep Technol Assess (Full Rep). 2006(137):1–77. , , , et al.
- Defining and measuring quality palliative and end‐of‐life care in the intensive care unit. Crit Care Med. 2006;34(11 Suppl):S309–316. .
- Validation of a vertical visual analogue scale as a measure of clinical dyspnea. Rehabil Nurs. 1989;14(6):323–325. .
- Can a self‐rating 0‐10 scale for dyspnea yield a common language that is understood by ED nurses, patients, and their families? J Emerg Nurs. 2000;26(3):233–234. .
- Measurement of dyspnea: word labeled visual analog scale vs. verbal ordinal scale. Respir Physiol Neurobiol. 2003;134(2):77–83. , , .
- Verbal numerical scales are as reliable and sensitive as visual analog scales for rating dyspnea in young and older subjects. Respir Physiol Neurobiol. 2007;157(2‐3):360–365. , , , , , .
- Validation of a three‐factor measurement model of dyspnea in hospitalized adults with heart failure. Heart Lung. 2011;41(1):44–56. , , , , .
- Patient reporting and doctor recognition of dyspnoea in a comprehensive cancer centre. Intern Med J. 2006;36(6):381–384. , , .
- Lung cancer and dyspnea: the patient's perception. Oncol Nurs Forum. 1986;13(5):19–24. , , , .
- Opioids, respiratory function, and dyspnea. Am J Hosp Palliat Care. 2003;20(1):57–61. , , , .
Dyspnea, defined as a subjective experience of breathing discomfort,[1] is the seventh most frequent reason adult patients present to the emergency room and the most frequent cause for emergency room visits in patients 65 years or older.[2] Moreover, dyspnea is experienced by 49% of patients hospitalized with a medical condition[3, 4, 5] and by 70% of patients who are seriously ill.[6]
Based on evidence that patients are not treated consistently and effectively for relief of their shortness of breath, the American College of Chest Physicians (ACCP) statement on dyspnea management in patients with advanced lung or heart disease recommended that patients should be asked to rate their dyspnea, and the rating should be routinely documented in the medical record to guide management.[7] Although clinicians may question the utility of routine assessment of dyspnea using a standardized scale, studies have found that the prevalence of dyspnea reported from chart review is much lower than when patients are directly interviewed.[8] This may be the result of underrecognition of dyspnea or poor documentation by physicians, or that patients may not communicate their symptoms unless the physician specifically asks. As is the case with pain, routine assessment of dyspnea severity could lead to improved clinical management and greater patient‐centered care. However, unlike in the case of pain, regulatory bodies, such as the Joint Commission for Accreditation of Healthcare Organization, do not require routine dyspnea assessment.[9]
Currently, there are more than 40,000 hospitalists in the United States, and the vast majority of hospitals with >200 beds have a hospitalist group.[10] Hospitalists care for over 60% of inpatients[11] and play a major role in the management of patients with acute cardiopulmonary diseases. If standardized approaches for the assessment and documentation of dyspnea are to be implemented, hospitalists would be a key stakeholder group for utilizing enhanced clinical information about dyspnea. Therefore, we evaluated attitudes and practices of hospitalists in regard to the assessment and management of dyspnea, including the potential benefits and challenges related to the implementation of standardized assessment. We hypothesized that hospitalists would believe that a dyspnea scale for assessment of severity could improve their management of patients with cardiovascular diseases. Further, we hypothesized that physicians who agreed with the general statement that dyspnea is an important clinical problem would be more likely to believe that routine dyspnea assessment would be valuable.
METHODS
Study Sample
We invited 255 attending hospitalists from 9 geographically and structurally diverse hospitals to complete a survey about the assessment and management of dyspnea. The 9 hospitals represent range of practice environments including 4 academic medical centers, 2 community teaching and 3 nonteaching hospitals, 1 Veterans Administration hospital, and 2 staff‐model HMOs (see Supporting Table 1 in the online version of this article). The survey was distributed online using REDCap (Research Electronic Data Capture), a secure web‐based interface application.[12] A coinvestigator who was a pulmonary critical‐care physician at each site sent an initial email to their hospitalist groups that alerted them to expect a survey from the principal investigator. This notification was subsequently followed by an email invitation containing an informational cover letter and a link to the online survey. The cover letter stated that the completed surveys would not be stored at the local sites and that all the analyzed data would be deidentified. Nonrespondents were sent reminders at 2 and 4 weeks after the initial mailing. A $25 electronic gift card was provided as a gesture of appreciation for their time. The survey was conducted between September 2013 and December 2013.
The study was approved by the Baystate Health Institutional Review Board, Springfield, Massachusetts, with a waiver for written informed consent.
Questionnaire
We developed a 17‐item instrument based on a review of the dyspnea literature and a prior ACCP survey.[12] Questions were piloted with 4 hospitalists at a single institution and modified to improve face validity and clarity (see Supporting Information in the online version of this article for the full survey).
Hospitalists were asked to consider the care of patients admitted for acute cardiopulmonary disease, including heart failure, chronic obstructive pulmonary disease, and pneumonia. A series of 5‐point Likert scales were used to assess the respondents level of agreement with statements related to the following domains: the importance of dyspnea in clinical care, the potential benefits and challenges of routine dyspnea assessment (statements such as: Having a standardized assessment of dyspnea severity would be helpful in management of patients with cardiopulmonary diseases. Dyspnea assessment by a scale should be part of the vital signs for patients with cardiopulmonary diseases.), and management of dyspnea (questions regarding the use of opioids and other nonpharmacological therapies). Additional questions were asked about current assessment practices (questions such as: How often do you assess severity of dyspnea? What is your approach in assessing dyspnea? with options of choosing a categorical or numerical scale), if dyspnea is assessed in their institution by nurses and how often, and the influence of dyspnea severity assessment on their management. The survey had 1 question that solicited comments from the participants: If you don't think that it would be useful to have a standardized dyspnea assessment, please tell us why.
Data Analysis
Responses to survey questions were summarized via counts and percentages in each response category. Adopting the methodology used in the ACCP consensus statement, strongly agree and somewhat agree were combined into a single category of agreement. We also presented percentage of responses in the 2 levels of agreement (strongly agree and somewhat agree) for each question in a bar graph.
Associations between tertiles of physicians' time in practice and attitude toward dyspnea were evaluated via 2 or Fisher exact test.
To examine how answers to the first 2 questions, which assessed attitude toward importance of dyspnea in clinical care, affect answers to the remaining questions, we grouped respondents in 3 categories (strongly agree, agree to these questions, do not agree) and tested the associations using 2 or Fisher exact test.
All analyses were performed using SAS version 9.3 (SAS institute, Inc., Cary, NC) and Stata release 13.1 (StataCorp, College Station, TX).
RESULTS
Overall, 178 (69.8%) of 255 identified hospitalists completed the survey, and all 9 participating hospitals had a response rate greater than 50%. The median number of years in practice was 6 (range, 038 years). A majority (77.5%) of respondents agreed with the statement that dyspnea is 1 of the major symptoms of patients with cardiopulmonary disease, and that its treatment is central to the management of these patients (77.0%) (Figure 1).
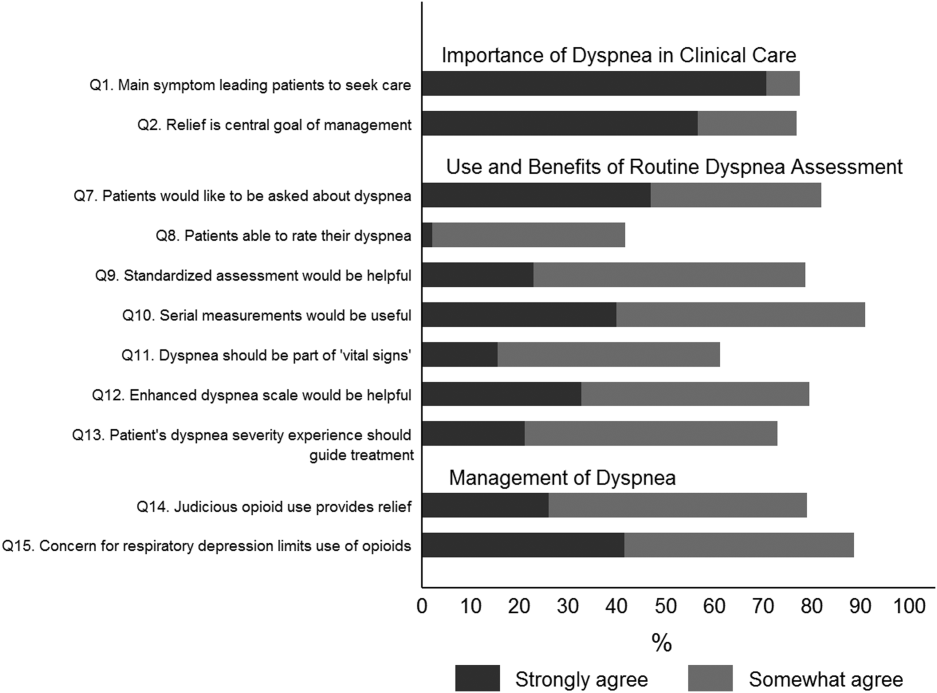
Attitude and Practices Surrounding Dyspnea Assessment
When asked about their current assessment of dyspnea, a majority (84.3%) of the hospitalists stated that they assess dyspnea on a daily basis; two‐thirds indicated that they use a categorical scale (ie, no shortness of breath, improved or worsened compared with a prior date), and one‐third indicated that they ask whether the patient is dyspneic or not. Fifty‐six percent of hospitalists stated that dyspnea is regularly assessed by nurses in their hospital.
The majority of respondents agreed (78.6%, 23.0% strongly and 55.6% somewhat agree) that standardized assessment of dyspnea severity, using a numeric scale and serial measurements as part of the vital signs, would benefit the management of patients with cardiopulmonary diseases. Furthermore, 79.6% (33.0% strongly and 46.6% somewhat agree) reported that using a dyspnea scale that included information to further characterize the patient‐reported experience, such as the level of distress associated with dyspnea, would be helpful in management.
Approximately 90% of the hospitalists indicated that awareness of dyspnea severity has an influence on clinical decision making, including whether to intensify treatment of underlying conditions, to pursue additional diagnostic testing, or to modify discharge timing. Additionally, two‐thirds of hospitalists agreed that awareness of dyspnea severity influences their decision to add opioids, whereas only one‐third prescribed nonpharmacologic symptom‐oriented treatment (Table 1).
Frequency (%) | |
---|---|
| |
When caring for patients with acute cardiopulmonary diseases, how often do you assess severity of dyspnea?* | |
At admission | 66 (37.1) |
At discharge | 59 (33.2) |
Daily until discharge | 150 (84.3) |
More often than daily | 58 (32.6) |
Which description best characterizes your approach to assessing dyspnea severity? | |
I don't regularly ask about dyspnea severity | 3 (1.7) |
I ask the patient whether or not they are having shortness of breath | 50 (28.3) |
I ask the patient to rate the severity of shortness of breath using a numeric scale | 4 (2.3) |
I ask the patient to rate the severity of shortness of breath using a categorical scale (eg, somewhat SOB, no SOB, improved or worsened compared with a prior date) | 120 (67.8) |
When is dyspnea severity assessed and documented by nursing at your hospital?* | |
Dyspnea is not routinely assessed | 60 (33.7) |
At admission | 30 (16.9) |
Daily | 43 (24.2) |
Each shift | 64 (36.0) |
Awareness of dyspnea severity affects my management by:* | |
Influencing my decision to intensify treatment of the patient's underlying condition | 170 (95.5) |
Influencing my decision to pursue additional diagnostic testing | 160 (89.9) |
Influencing my decision to add pharmacologic‐based, symptom‐oriented treatment for dyspnea, such as opioids | 115 (64.6) |
Influencing my decision to add nonpharmacologic‐based, symptom‐oriented treatment for dyspnea, such as fans or pursed lip breathing technique | 58 (32.6) |
Influencing my decision regarding timing of discharge | 162 (91.0) |
Which of the following nonpharmacologic therapies are effective for the relief of dyspnea?* | |
Pursed lip breathing | 113 (63.5) |
Relaxation techniques | 137 (77.0) |
Noninvasive ventilation | 143 (80.3) |
O2 for nonhypoxemic patients | 89 (50.0) |
Cool air/fan | 125 (70.2) |
Cognitive behavioral strategies | 101 (56.7) |
Forty‐two percent of the respondents agreed that patients are able to rate their dyspnea on a scale (2.3% strongly agree and 40.0% agree), and 73.0% indicated that patient experience of dyspnea should guide management independent of physiologic measures such as respiratory rate and oxygen saturation (Figure 1).
Several potential barriers were identified among the 18 participants who did not think that a standardized assessment of dyspnea would be beneficial, including concerns that (1) a dyspnea severity scale is too subjective and numerical scales are not useful for a subjective symptom (19.0%), (2) patients may overrate their symptom or will not be able to rate their dyspnea using a scale (31.0%), or (3) categorical description is sufficient (31.0%).
Practices in Dyspnea Management
Seventy‐nine percent of respondents agreed with the statement that judicious use of opioids can provide relief of dyspnea (26.1% strongly and 52.8% agreed), and 88.7% hospitalists identified the risk of respiratory depression as 1 of the barriers for the limited use of opioids. The majority of physicians (60%80%) considered nonpharmacologic therapies effective for symptomatic treatment of dyspnea, including in the order of agreement: noninvasive ventilation, relaxation techniques, cool air/fan, use of pursed lip breathing, and oxygen for nonhypoxemic patients (Table 1).
Physician Experience and Attitudes Toward Dyspnea Management
When we stratified hospitalists in tertiles of median years of time in practice (median [range]: 2 [04], 6 [58] and 15 [938]), we did not find an association with any of the responses to the questions.
Attitude Regarding the Importance of Dyspnea in Clinical Care and Responses to Subsequent Questions
Respondents who strongly agree or agree that dyspnea is the primary presenting symptom in patients with cardiovascular condition and that dyspnea relief is central to the management of these patients were more likely to believe that patients would like to be asked about their dyspnea (61.2% vs 30.2% vs 29.7%). They also had a more positive attitude about the usefulness of a standardized assessment of dyspnea and the inclusion of the assessment of dyspnea by a scale in the vital signs (Table 2).
Description | Do Not Agree, n (%) | Somewhat Agree, n (%) | Strongly Agree, n (%) | P Value* |
---|---|---|---|---|
| ||||
37 (20.9) | 43 (24.3) | 97 (54.8) | ||
Which description best characterizes your approach to assessing dyspnea severity? | 0.552 | |||
I don't regularly ask about dyspnea severity | 0 (0) | 0 (0) | 3 (3.1) | |
I ask the patient whether or not they are having shortness of breath | 11 (29.7) | 14 (32.6) | 25 (25.8) | |
I ask the patient to rate the severity of shortness of breath using a numeric scale | 2 (5.4) | 1 (2.3) | 1 (1.0) | |
I ask the patient to rate the severity of shortness of breath using a categorical scale (e.g., somewhat shortness of breath, no shortness of breath, improved or worsened compared with a prior date) | 24 (64.9) | 28 (65.1) | 68 (70.1) | |
Patients would like me to ask them about their dyspnea. | <0.0001 | |||
Somewhat agree | 9 (24.3) | 21 (48.8) | 32 (32.7) | |
Strongly agree | 11 (29.7) | 13 (30.2) | 60 (61.2) | |
Patients are able to rate their own dyspnea intensity on a scale of 0‐10. | 0.432 | |||
Somewhat agree | 12 (32.4) | 16 (37.2) | 42 (43.3) | |
Strongly agree | 2 (5.4) | 0 (0) | 2 (2.1) | |
Having a standardized assessment of dyspnea severity would be helpful to me in management of patients with cardiopulmonary diseases. | 0.026 | |||
Somewhat agree | 17 (46.0) | 25 (58.1) | 57 (58.2) | |
Strongly agree | 7 (18.9) | 6 (14.0) | 28 (28.6) | |
Serial measurements of dyspnea would be useful for assessing response to therapy. | 0.042 | |||
Somewhat agree | 14 (37.8) | 28 (65.1) | 48 (49.5) | |
Strongly agree | 16 (43.2) | 12 (27.9) | 43 (44.3) | |
Dyspnea assessment by a scale should be part of the vital signs for patients with cardiopulmonary diseases. | 0.042 | |||
Somewhat agree | 13 (35.1) | 17 (39.5) | 51 (52.0) | |
Strongly agree | 4 (10.8) | 5 (11.6) | 19 (19.4) | |
Using an enhanced dyspnea scale that includes information about the following 4 features 1) Current dyspnea severity, 2) Worst dyspnea ever, 3) Improvement of dyspnea since admission, 4) Acceptability of current level of dyspnea, would be more helpful for my management than a single question focused on dyspnea severity. | 0.03 | |||
Somewhat agree | 14 (40.0) | 24 (55.8) | 44 (44.9) | |
Strongly agree | 9 (25.7) | 9 (20.9) | 40 (40.8) | |
The patients experience of dyspnea should be used to guide treatment decisions independent of objective measures such as respiratory rate and oxygen saturation. | 0.10 | |||
Somewhat agree | 20 (54.0) | 21 (48.8) | 51 (52.0) | |
Strongly agree | 5 (13.5) | 6 (14.0) | 27 (27.6) | |
Judicious use of oral and/or parenteral opioids can provide relief of dyspnea. | 0.21 | |||
Somewhat agree | 20 (54.0) | 23 (54.8) | 50 (51.6) | |
Strongly agree | 10 (27.0) | 6 (14.3) | 30 (30.9) | |
Limited use of opioids for relief of dyspnea in patients with advanced cardiopulmonary disorders is often due to concerns of respiratory depression. | 0.71 | |||
Somewhat agree | 17 (46.0) | 23 (54.8) | 43 (43.9) | |
Strongly agree | 15 (40.5) | 14 (33.3) | 45 (45.9) |
DISCUSSION
In this survey of 178 most hospitalists from a diverse group of 9 US hospitals, we found that most indicate that severity of dyspnea has a profound influence on their clinical practice (including their decision whether to intensify treatments such as diuretics or bronchodilators, to pursue additional diagnostic testing, add opioids or other nonpharmacological treatments) and ultimately their decision regarding the timing of hospital discharge. More importantly, whereas less than half reported experience with standardized assessment of dyspnea severity, most stated that such data would be very useful in their practice.
Despite being a highly prevalent symptom in diverse patient populations, several studies have shown that documentation of dyspnea is sporadic and evaluation of dyspnea quality of care is not routinely performed.[13, 14, 15] Statements from a number of professional societies, including the ACCP, the American Thoracic Society and the Canadian Respiratory Society, recommend that dyspnea management should rely on patient reporting, and that dyspnea severity should be recorded.[1, 4, 7] Assessment is an essential step to guide interventions; however, simply asking about the presence or absence of dyspnea is insufficient.
Several rating scales have been validated and might be implementable in the acute care setting, including the Numerical Rating Scale and the Visual Assessment Scale.[16, 17, 18, 19, 20] Our survey shows that standardized documentation of dyspnea severity in clinical practice is uncommon. However, most hospitalists in our study believed that assessment of dyspnea, using a standardized scale, would positively impact their management of patients with cardiopulmonary disease.
There are a number of potential benefits of routine assessment of dyspnea in hospitalized patients. Implementation of a standardized approach to dyspnea measurement would result in more uniform assessment and documentation practices, and in turn greater awareness among members of the patient‐care team. Though not sufficient to improve care, measurement is necessary because physicians do not always recognize the severity of patients' dyspnea or may not recognize its presence. A retrospective study that assessed the prevalence of symptoms in 410 ambulatory patients showed that one‐quarter of patients had dyspnea, but only half of them told their doctor about it.[21] Two other studies of patients with cancer diagnoses found that 30%70% of patients had dyspnea, but the symptom was recognized in only half of them; even when recognized, dyspnea severity was frequently underrated by physicians.[21, 22] Importantly, underestimation appears to correlate with underutilization of symptomatic management of dyspnea.[8]
Although the results of our survey are encouraging, they highlight a number of potential barriers and misconceptions among hospitalists. For example, although dyspnea can be characterized only by the person experiencing it, only 42% of our survey respondents believed that patients are able to rate their dyspnea intensity on a scale. Some of these responses may be influenced by the fact that dyspnea scales are not currently available to patients under their care. Another explanation is that similar to the case for pain, some hospitalists may believe that patients will exaggerate dyspnea severity. Almost one‐third of the respondents stated that objective measures, such as respiratory rate or oxygen saturation, are more important than a patient's experience of dyspnea in guiding the treatment, and that dyspnea is a subjective symptom and not a vital sign itself. Hospitalists who appreciated the importance of dyspnea in clinical practice were more likely to support the implementation of a standardized dyspnea scale for dyspnea assessment.
Although the potential benefits of including routine measurement of dyspnea in standard hospital practice may seem obvious, evidence that implementing routine assessment improves patient care or outcomes is lacking. Even if hospitalists see the value of dyspnea assessment, asking nurses to collect and document additional information would represent a substantial change in hospital workflow. Finally, without specific protocols to guide care, it is unclear whether physicians will be able to use new information about dyspnea severity effectively. Future studies need to evaluate the impact of implementing routine dyspnea assessment on the management of patients with cardiopulmonary diseases including the use of evidence‐based interventions and reducing the use of less valuable care.
Most hospitalists agreed with the basic principles of dyspnea treatment in patients with advanced cardiopulmonary disease after the primary disease had been stabilized. Effective measures are available, and several guidelines endorse opioids in dyspnea management.[1, 4, 7] However, many clinicians are uncomfortable with this approach for dyspnea, and opioids remain underused. In our study, almost 90% of physicians recognized that concerns about respiratory depression limits opioids use as a treatment. A qualitative study that explored the physicians' perspective toward opioids showed that most physicians were reluctant to prescribe opioids for refractory dyspnea, describing a lack of related knowledge and experience, and fears related to the potential adverse effects. The findings of our study also outline the need to better educate residents and hospitalists on the assessment and management of dyspnea, including prescribing opioids for refractory dyspnea.[23]
Study Strengths and Limitations
This study has several strengths. To our knowledge, it is the first to explore hospitalists' perspectives on incorporating dyspnea assessment in their clinical practice. Hospitalists are the attending physicians for a large majority of inpatients and would be the main users of a dyspnea severity scale. Our questionnaire survey included a large number of hospitalists, from 9 geographically and structurally diverse hospitals, which increased the generalizability of the findings to other hospitals around the country.
The study also has several limitations that need be kept in mind in interpreting the study results. First, desirability bias may have exaggerated some of the positive views expressed by hospitalists toward implementation of routine assessment of dyspnea. Second, because this was a survey, the estimates of dyspnea assessment and documentation practices of both physicians and nurses were based on the respondents' perception and not an objective review of medical records, and the results may be different from actual practice. Third, this was not a population‐based random sample of hospitalists, and it may not be entirely representative; however, those surveyed were from a diverse set of sites with different geographical location, size, academic affiliation, and practice environment, and their time in practice varied widely. Last, we do not have information on nonrespondents, and there is a possibility of nonresponse bias, although the high response rate lessens the risk.
CONCLUSIONS
The results of this survey suggest that most hospitalists believe that routine assessment of dyspnea severity would enhance their clinical decision making and improve patient care. Standardized assessment of dyspnea might result in better awareness of this symptom among providers, reduce undertreatment and mistreatment, and ultimately result in better outcomes for patients. However, implementation of the routine assessment of dyspnea would change current clinical practices and may have a significant effect on existing nursing and physician workflows. Additional research is needed to determine the feasibility and impact on outcomes of routine dyspnea assessment.
Acknowledgements
The authors wish to acknowledge Ms. Anu Joshi for her help with editing the manuscript and assisting with table preparations.
Disclosures
Dr. Stefan is supported by grant K01HL114631‐01A1 from the National Heart, Lung, and Blood Institute of the National Institutes of Health, and by the National Center for Research Resources and the National Center for Advancing Translational Sciences, National Institutes of Health, through grant UL1RR025752. The funder had no role in the design and conduct of the study; collection, management, analysis, and interpretation of the data; preparation, review, or approval of the manuscript; and decision to submit the manuscript for publication. M.S.S. and P.K.L. conceived of the study. M.S.S. acquired the data with the help of all collaborators. M.S.S., P.K.L., P.S.P., and A.P. analyzed and interpreted the data. M.S.S. drafted the manuscript. All authors critically reviewed the manuscript for intellectual content. M.S.S., P.K.L., and A.P. had full access to all of the data in the study and take responsibility for the integrity of the data and the accuracy of the data analysis. M.S.S. is the guarantor for this article, and is responsible for the content of the article, including data and analysis. The authors report no conflicts of interest.
Dyspnea, defined as a subjective experience of breathing discomfort,[1] is the seventh most frequent reason adult patients present to the emergency room and the most frequent cause for emergency room visits in patients 65 years or older.[2] Moreover, dyspnea is experienced by 49% of patients hospitalized with a medical condition[3, 4, 5] and by 70% of patients who are seriously ill.[6]
Based on evidence that patients are not treated consistently and effectively for relief of their shortness of breath, the American College of Chest Physicians (ACCP) statement on dyspnea management in patients with advanced lung or heart disease recommended that patients should be asked to rate their dyspnea, and the rating should be routinely documented in the medical record to guide management.[7] Although clinicians may question the utility of routine assessment of dyspnea using a standardized scale, studies have found that the prevalence of dyspnea reported from chart review is much lower than when patients are directly interviewed.[8] This may be the result of underrecognition of dyspnea or poor documentation by physicians, or that patients may not communicate their symptoms unless the physician specifically asks. As is the case with pain, routine assessment of dyspnea severity could lead to improved clinical management and greater patient‐centered care. However, unlike in the case of pain, regulatory bodies, such as the Joint Commission for Accreditation of Healthcare Organization, do not require routine dyspnea assessment.[9]
Currently, there are more than 40,000 hospitalists in the United States, and the vast majority of hospitals with >200 beds have a hospitalist group.[10] Hospitalists care for over 60% of inpatients[11] and play a major role in the management of patients with acute cardiopulmonary diseases. If standardized approaches for the assessment and documentation of dyspnea are to be implemented, hospitalists would be a key stakeholder group for utilizing enhanced clinical information about dyspnea. Therefore, we evaluated attitudes and practices of hospitalists in regard to the assessment and management of dyspnea, including the potential benefits and challenges related to the implementation of standardized assessment. We hypothesized that hospitalists would believe that a dyspnea scale for assessment of severity could improve their management of patients with cardiovascular diseases. Further, we hypothesized that physicians who agreed with the general statement that dyspnea is an important clinical problem would be more likely to believe that routine dyspnea assessment would be valuable.
METHODS
Study Sample
We invited 255 attending hospitalists from 9 geographically and structurally diverse hospitals to complete a survey about the assessment and management of dyspnea. The 9 hospitals represent range of practice environments including 4 academic medical centers, 2 community teaching and 3 nonteaching hospitals, 1 Veterans Administration hospital, and 2 staff‐model HMOs (see Supporting Table 1 in the online version of this article). The survey was distributed online using REDCap (Research Electronic Data Capture), a secure web‐based interface application.[12] A coinvestigator who was a pulmonary critical‐care physician at each site sent an initial email to their hospitalist groups that alerted them to expect a survey from the principal investigator. This notification was subsequently followed by an email invitation containing an informational cover letter and a link to the online survey. The cover letter stated that the completed surveys would not be stored at the local sites and that all the analyzed data would be deidentified. Nonrespondents were sent reminders at 2 and 4 weeks after the initial mailing. A $25 electronic gift card was provided as a gesture of appreciation for their time. The survey was conducted between September 2013 and December 2013.
The study was approved by the Baystate Health Institutional Review Board, Springfield, Massachusetts, with a waiver for written informed consent.
Questionnaire
We developed a 17‐item instrument based on a review of the dyspnea literature and a prior ACCP survey.[12] Questions were piloted with 4 hospitalists at a single institution and modified to improve face validity and clarity (see Supporting Information in the online version of this article for the full survey).
Hospitalists were asked to consider the care of patients admitted for acute cardiopulmonary disease, including heart failure, chronic obstructive pulmonary disease, and pneumonia. A series of 5‐point Likert scales were used to assess the respondents level of agreement with statements related to the following domains: the importance of dyspnea in clinical care, the potential benefits and challenges of routine dyspnea assessment (statements such as: Having a standardized assessment of dyspnea severity would be helpful in management of patients with cardiopulmonary diseases. Dyspnea assessment by a scale should be part of the vital signs for patients with cardiopulmonary diseases.), and management of dyspnea (questions regarding the use of opioids and other nonpharmacological therapies). Additional questions were asked about current assessment practices (questions such as: How often do you assess severity of dyspnea? What is your approach in assessing dyspnea? with options of choosing a categorical or numerical scale), if dyspnea is assessed in their institution by nurses and how often, and the influence of dyspnea severity assessment on their management. The survey had 1 question that solicited comments from the participants: If you don't think that it would be useful to have a standardized dyspnea assessment, please tell us why.
Data Analysis
Responses to survey questions were summarized via counts and percentages in each response category. Adopting the methodology used in the ACCP consensus statement, strongly agree and somewhat agree were combined into a single category of agreement. We also presented percentage of responses in the 2 levels of agreement (strongly agree and somewhat agree) for each question in a bar graph.
Associations between tertiles of physicians' time in practice and attitude toward dyspnea were evaluated via 2 or Fisher exact test.
To examine how answers to the first 2 questions, which assessed attitude toward importance of dyspnea in clinical care, affect answers to the remaining questions, we grouped respondents in 3 categories (strongly agree, agree to these questions, do not agree) and tested the associations using 2 or Fisher exact test.
All analyses were performed using SAS version 9.3 (SAS institute, Inc., Cary, NC) and Stata release 13.1 (StataCorp, College Station, TX).
RESULTS
Overall, 178 (69.8%) of 255 identified hospitalists completed the survey, and all 9 participating hospitals had a response rate greater than 50%. The median number of years in practice was 6 (range, 038 years). A majority (77.5%) of respondents agreed with the statement that dyspnea is 1 of the major symptoms of patients with cardiopulmonary disease, and that its treatment is central to the management of these patients (77.0%) (Figure 1).
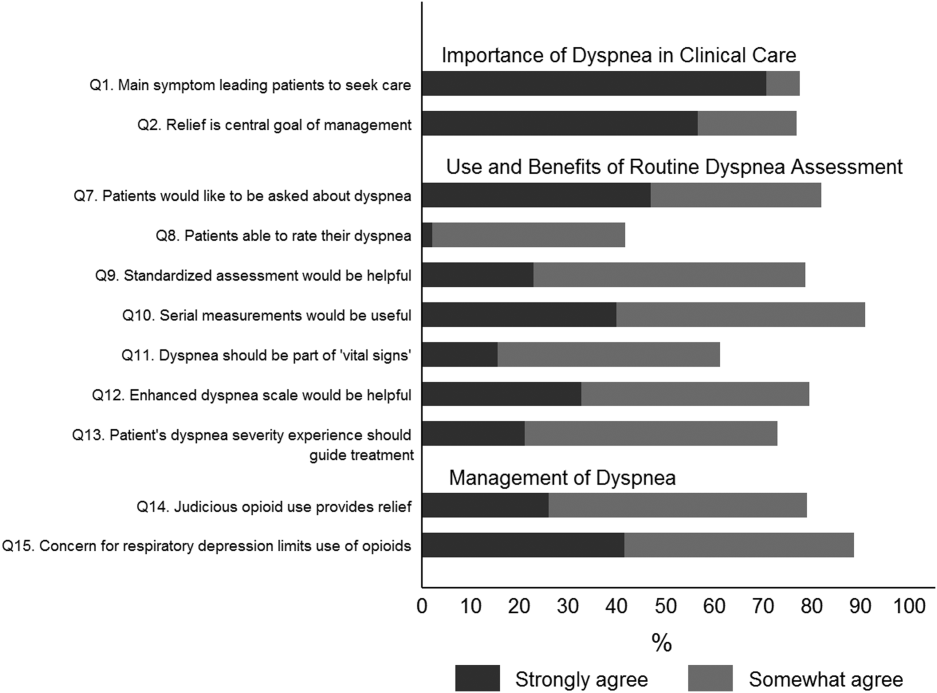
Attitude and Practices Surrounding Dyspnea Assessment
When asked about their current assessment of dyspnea, a majority (84.3%) of the hospitalists stated that they assess dyspnea on a daily basis; two‐thirds indicated that they use a categorical scale (ie, no shortness of breath, improved or worsened compared with a prior date), and one‐third indicated that they ask whether the patient is dyspneic or not. Fifty‐six percent of hospitalists stated that dyspnea is regularly assessed by nurses in their hospital.
The majority of respondents agreed (78.6%, 23.0% strongly and 55.6% somewhat agree) that standardized assessment of dyspnea severity, using a numeric scale and serial measurements as part of the vital signs, would benefit the management of patients with cardiopulmonary diseases. Furthermore, 79.6% (33.0% strongly and 46.6% somewhat agree) reported that using a dyspnea scale that included information to further characterize the patient‐reported experience, such as the level of distress associated with dyspnea, would be helpful in management.
Approximately 90% of the hospitalists indicated that awareness of dyspnea severity has an influence on clinical decision making, including whether to intensify treatment of underlying conditions, to pursue additional diagnostic testing, or to modify discharge timing. Additionally, two‐thirds of hospitalists agreed that awareness of dyspnea severity influences their decision to add opioids, whereas only one‐third prescribed nonpharmacologic symptom‐oriented treatment (Table 1).
Frequency (%) | |
---|---|
| |
When caring for patients with acute cardiopulmonary diseases, how often do you assess severity of dyspnea?* | |
At admission | 66 (37.1) |
At discharge | 59 (33.2) |
Daily until discharge | 150 (84.3) |
More often than daily | 58 (32.6) |
Which description best characterizes your approach to assessing dyspnea severity? | |
I don't regularly ask about dyspnea severity | 3 (1.7) |
I ask the patient whether or not they are having shortness of breath | 50 (28.3) |
I ask the patient to rate the severity of shortness of breath using a numeric scale | 4 (2.3) |
I ask the patient to rate the severity of shortness of breath using a categorical scale (eg, somewhat SOB, no SOB, improved or worsened compared with a prior date) | 120 (67.8) |
When is dyspnea severity assessed and documented by nursing at your hospital?* | |
Dyspnea is not routinely assessed | 60 (33.7) |
At admission | 30 (16.9) |
Daily | 43 (24.2) |
Each shift | 64 (36.0) |
Awareness of dyspnea severity affects my management by:* | |
Influencing my decision to intensify treatment of the patient's underlying condition | 170 (95.5) |
Influencing my decision to pursue additional diagnostic testing | 160 (89.9) |
Influencing my decision to add pharmacologic‐based, symptom‐oriented treatment for dyspnea, such as opioids | 115 (64.6) |
Influencing my decision to add nonpharmacologic‐based, symptom‐oriented treatment for dyspnea, such as fans or pursed lip breathing technique | 58 (32.6) |
Influencing my decision regarding timing of discharge | 162 (91.0) |
Which of the following nonpharmacologic therapies are effective for the relief of dyspnea?* | |
Pursed lip breathing | 113 (63.5) |
Relaxation techniques | 137 (77.0) |
Noninvasive ventilation | 143 (80.3) |
O2 for nonhypoxemic patients | 89 (50.0) |
Cool air/fan | 125 (70.2) |
Cognitive behavioral strategies | 101 (56.7) |
Forty‐two percent of the respondents agreed that patients are able to rate their dyspnea on a scale (2.3% strongly agree and 40.0% agree), and 73.0% indicated that patient experience of dyspnea should guide management independent of physiologic measures such as respiratory rate and oxygen saturation (Figure 1).
Several potential barriers were identified among the 18 participants who did not think that a standardized assessment of dyspnea would be beneficial, including concerns that (1) a dyspnea severity scale is too subjective and numerical scales are not useful for a subjective symptom (19.0%), (2) patients may overrate their symptom or will not be able to rate their dyspnea using a scale (31.0%), or (3) categorical description is sufficient (31.0%).
Practices in Dyspnea Management
Seventy‐nine percent of respondents agreed with the statement that judicious use of opioids can provide relief of dyspnea (26.1% strongly and 52.8% agreed), and 88.7% hospitalists identified the risk of respiratory depression as 1 of the barriers for the limited use of opioids. The majority of physicians (60%80%) considered nonpharmacologic therapies effective for symptomatic treatment of dyspnea, including in the order of agreement: noninvasive ventilation, relaxation techniques, cool air/fan, use of pursed lip breathing, and oxygen for nonhypoxemic patients (Table 1).
Physician Experience and Attitudes Toward Dyspnea Management
When we stratified hospitalists in tertiles of median years of time in practice (median [range]: 2 [04], 6 [58] and 15 [938]), we did not find an association with any of the responses to the questions.
Attitude Regarding the Importance of Dyspnea in Clinical Care and Responses to Subsequent Questions
Respondents who strongly agree or agree that dyspnea is the primary presenting symptom in patients with cardiovascular condition and that dyspnea relief is central to the management of these patients were more likely to believe that patients would like to be asked about their dyspnea (61.2% vs 30.2% vs 29.7%). They also had a more positive attitude about the usefulness of a standardized assessment of dyspnea and the inclusion of the assessment of dyspnea by a scale in the vital signs (Table 2).
Description | Do Not Agree, n (%) | Somewhat Agree, n (%) | Strongly Agree, n (%) | P Value* |
---|---|---|---|---|
| ||||
37 (20.9) | 43 (24.3) | 97 (54.8) | ||
Which description best characterizes your approach to assessing dyspnea severity? | 0.552 | |||
I don't regularly ask about dyspnea severity | 0 (0) | 0 (0) | 3 (3.1) | |
I ask the patient whether or not they are having shortness of breath | 11 (29.7) | 14 (32.6) | 25 (25.8) | |
I ask the patient to rate the severity of shortness of breath using a numeric scale | 2 (5.4) | 1 (2.3) | 1 (1.0) | |
I ask the patient to rate the severity of shortness of breath using a categorical scale (e.g., somewhat shortness of breath, no shortness of breath, improved or worsened compared with a prior date) | 24 (64.9) | 28 (65.1) | 68 (70.1) | |
Patients would like me to ask them about their dyspnea. | <0.0001 | |||
Somewhat agree | 9 (24.3) | 21 (48.8) | 32 (32.7) | |
Strongly agree | 11 (29.7) | 13 (30.2) | 60 (61.2) | |
Patients are able to rate their own dyspnea intensity on a scale of 0‐10. | 0.432 | |||
Somewhat agree | 12 (32.4) | 16 (37.2) | 42 (43.3) | |
Strongly agree | 2 (5.4) | 0 (0) | 2 (2.1) | |
Having a standardized assessment of dyspnea severity would be helpful to me in management of patients with cardiopulmonary diseases. | 0.026 | |||
Somewhat agree | 17 (46.0) | 25 (58.1) | 57 (58.2) | |
Strongly agree | 7 (18.9) | 6 (14.0) | 28 (28.6) | |
Serial measurements of dyspnea would be useful for assessing response to therapy. | 0.042 | |||
Somewhat agree | 14 (37.8) | 28 (65.1) | 48 (49.5) | |
Strongly agree | 16 (43.2) | 12 (27.9) | 43 (44.3) | |
Dyspnea assessment by a scale should be part of the vital signs for patients with cardiopulmonary diseases. | 0.042 | |||
Somewhat agree | 13 (35.1) | 17 (39.5) | 51 (52.0) | |
Strongly agree | 4 (10.8) | 5 (11.6) | 19 (19.4) | |
Using an enhanced dyspnea scale that includes information about the following 4 features 1) Current dyspnea severity, 2) Worst dyspnea ever, 3) Improvement of dyspnea since admission, 4) Acceptability of current level of dyspnea, would be more helpful for my management than a single question focused on dyspnea severity. | 0.03 | |||
Somewhat agree | 14 (40.0) | 24 (55.8) | 44 (44.9) | |
Strongly agree | 9 (25.7) | 9 (20.9) | 40 (40.8) | |
The patients experience of dyspnea should be used to guide treatment decisions independent of objective measures such as respiratory rate and oxygen saturation. | 0.10 | |||
Somewhat agree | 20 (54.0) | 21 (48.8) | 51 (52.0) | |
Strongly agree | 5 (13.5) | 6 (14.0) | 27 (27.6) | |
Judicious use of oral and/or parenteral opioids can provide relief of dyspnea. | 0.21 | |||
Somewhat agree | 20 (54.0) | 23 (54.8) | 50 (51.6) | |
Strongly agree | 10 (27.0) | 6 (14.3) | 30 (30.9) | |
Limited use of opioids for relief of dyspnea in patients with advanced cardiopulmonary disorders is often due to concerns of respiratory depression. | 0.71 | |||
Somewhat agree | 17 (46.0) | 23 (54.8) | 43 (43.9) | |
Strongly agree | 15 (40.5) | 14 (33.3) | 45 (45.9) |
DISCUSSION
In this survey of 178 most hospitalists from a diverse group of 9 US hospitals, we found that most indicate that severity of dyspnea has a profound influence on their clinical practice (including their decision whether to intensify treatments such as diuretics or bronchodilators, to pursue additional diagnostic testing, add opioids or other nonpharmacological treatments) and ultimately their decision regarding the timing of hospital discharge. More importantly, whereas less than half reported experience with standardized assessment of dyspnea severity, most stated that such data would be very useful in their practice.
Despite being a highly prevalent symptom in diverse patient populations, several studies have shown that documentation of dyspnea is sporadic and evaluation of dyspnea quality of care is not routinely performed.[13, 14, 15] Statements from a number of professional societies, including the ACCP, the American Thoracic Society and the Canadian Respiratory Society, recommend that dyspnea management should rely on patient reporting, and that dyspnea severity should be recorded.[1, 4, 7] Assessment is an essential step to guide interventions; however, simply asking about the presence or absence of dyspnea is insufficient.
Several rating scales have been validated and might be implementable in the acute care setting, including the Numerical Rating Scale and the Visual Assessment Scale.[16, 17, 18, 19, 20] Our survey shows that standardized documentation of dyspnea severity in clinical practice is uncommon. However, most hospitalists in our study believed that assessment of dyspnea, using a standardized scale, would positively impact their management of patients with cardiopulmonary disease.
There are a number of potential benefits of routine assessment of dyspnea in hospitalized patients. Implementation of a standardized approach to dyspnea measurement would result in more uniform assessment and documentation practices, and in turn greater awareness among members of the patient‐care team. Though not sufficient to improve care, measurement is necessary because physicians do not always recognize the severity of patients' dyspnea or may not recognize its presence. A retrospective study that assessed the prevalence of symptoms in 410 ambulatory patients showed that one‐quarter of patients had dyspnea, but only half of them told their doctor about it.[21] Two other studies of patients with cancer diagnoses found that 30%70% of patients had dyspnea, but the symptom was recognized in only half of them; even when recognized, dyspnea severity was frequently underrated by physicians.[21, 22] Importantly, underestimation appears to correlate with underutilization of symptomatic management of dyspnea.[8]
Although the results of our survey are encouraging, they highlight a number of potential barriers and misconceptions among hospitalists. For example, although dyspnea can be characterized only by the person experiencing it, only 42% of our survey respondents believed that patients are able to rate their dyspnea intensity on a scale. Some of these responses may be influenced by the fact that dyspnea scales are not currently available to patients under their care. Another explanation is that similar to the case for pain, some hospitalists may believe that patients will exaggerate dyspnea severity. Almost one‐third of the respondents stated that objective measures, such as respiratory rate or oxygen saturation, are more important than a patient's experience of dyspnea in guiding the treatment, and that dyspnea is a subjective symptom and not a vital sign itself. Hospitalists who appreciated the importance of dyspnea in clinical practice were more likely to support the implementation of a standardized dyspnea scale for dyspnea assessment.
Although the potential benefits of including routine measurement of dyspnea in standard hospital practice may seem obvious, evidence that implementing routine assessment improves patient care or outcomes is lacking. Even if hospitalists see the value of dyspnea assessment, asking nurses to collect and document additional information would represent a substantial change in hospital workflow. Finally, without specific protocols to guide care, it is unclear whether physicians will be able to use new information about dyspnea severity effectively. Future studies need to evaluate the impact of implementing routine dyspnea assessment on the management of patients with cardiopulmonary diseases including the use of evidence‐based interventions and reducing the use of less valuable care.
Most hospitalists agreed with the basic principles of dyspnea treatment in patients with advanced cardiopulmonary disease after the primary disease had been stabilized. Effective measures are available, and several guidelines endorse opioids in dyspnea management.[1, 4, 7] However, many clinicians are uncomfortable with this approach for dyspnea, and opioids remain underused. In our study, almost 90% of physicians recognized that concerns about respiratory depression limits opioids use as a treatment. A qualitative study that explored the physicians' perspective toward opioids showed that most physicians were reluctant to prescribe opioids for refractory dyspnea, describing a lack of related knowledge and experience, and fears related to the potential adverse effects. The findings of our study also outline the need to better educate residents and hospitalists on the assessment and management of dyspnea, including prescribing opioids for refractory dyspnea.[23]
Study Strengths and Limitations
This study has several strengths. To our knowledge, it is the first to explore hospitalists' perspectives on incorporating dyspnea assessment in their clinical practice. Hospitalists are the attending physicians for a large majority of inpatients and would be the main users of a dyspnea severity scale. Our questionnaire survey included a large number of hospitalists, from 9 geographically and structurally diverse hospitals, which increased the generalizability of the findings to other hospitals around the country.
The study also has several limitations that need be kept in mind in interpreting the study results. First, desirability bias may have exaggerated some of the positive views expressed by hospitalists toward implementation of routine assessment of dyspnea. Second, because this was a survey, the estimates of dyspnea assessment and documentation practices of both physicians and nurses were based on the respondents' perception and not an objective review of medical records, and the results may be different from actual practice. Third, this was not a population‐based random sample of hospitalists, and it may not be entirely representative; however, those surveyed were from a diverse set of sites with different geographical location, size, academic affiliation, and practice environment, and their time in practice varied widely. Last, we do not have information on nonrespondents, and there is a possibility of nonresponse bias, although the high response rate lessens the risk.
CONCLUSIONS
The results of this survey suggest that most hospitalists believe that routine assessment of dyspnea severity would enhance their clinical decision making and improve patient care. Standardized assessment of dyspnea might result in better awareness of this symptom among providers, reduce undertreatment and mistreatment, and ultimately result in better outcomes for patients. However, implementation of the routine assessment of dyspnea would change current clinical practices and may have a significant effect on existing nursing and physician workflows. Additional research is needed to determine the feasibility and impact on outcomes of routine dyspnea assessment.
Acknowledgements
The authors wish to acknowledge Ms. Anu Joshi for her help with editing the manuscript and assisting with table preparations.
Disclosures
Dr. Stefan is supported by grant K01HL114631‐01A1 from the National Heart, Lung, and Blood Institute of the National Institutes of Health, and by the National Center for Research Resources and the National Center for Advancing Translational Sciences, National Institutes of Health, through grant UL1RR025752. The funder had no role in the design and conduct of the study; collection, management, analysis, and interpretation of the data; preparation, review, or approval of the manuscript; and decision to submit the manuscript for publication. M.S.S. and P.K.L. conceived of the study. M.S.S. acquired the data with the help of all collaborators. M.S.S., P.K.L., P.S.P., and A.P. analyzed and interpreted the data. M.S.S. drafted the manuscript. All authors critically reviewed the manuscript for intellectual content. M.S.S., P.K.L., and A.P. had full access to all of the data in the study and take responsibility for the integrity of the data and the accuracy of the data analysis. M.S.S. is the guarantor for this article, and is responsible for the content of the article, including data and analysis. The authors report no conflicts of interest.
- An Official American Thoracic Society Statement: Update on the Mechanisms, Assessment, and Management of Dyspnea. Am J Respir Crit Care Med. 2012;185(4):435–452. , , , et al.
- CDC/ National Center for Health Statistics. National Hospital Amulatory Medical Care Survey: 2011 Emergency Department Summary Tables. http://www.cdc.gov/nchs/data/ahcd/nhamcs_emergency/2011_ed_web_tables.pdf. Accessed May 15, 2015.
- Signs and symptoms of heart failure: are you asking the right questions? Am J Crit Care. 2010;19(5):443–452. , , , .
- Managing dyspnea in patients with advanced chronic obstructive pulmonary disease: a Canadian Thoracic Society clinical practice guideline. Can Respir J. 2011;18(2):69–78. , , , et al.
- Prevalence of distressing symptoms in hospitalised patients on medical wards: A cross‐sectional study. BMC Palliat Care. 2008;7:16. , .
- Dyspnea in terminally ill cancer patients. Chest. 1986;89(2):234–236. , .
- American College of Chest Physicians consensus statement on the management of dyspnea in patients with advanced lung or heart disease. Chest. 2010;137(3):674–691. , , , et al.
- Common symptoms in ambulatory care: incidence, evaluation, therapy, and outcome. Am J Med. 1989;86(3):262–266. , .
- The Joint Commission. Facts about Pain Management. http://www.jointcommission.org/pain_management/. Accessed May, 15, 2015.
- Hospitalist programs in the age of healthcare reform. J Healthc Manag. 2010;55(6):378–380. .
- The Use of Hospitalists by Small Rural Hospitals: Results of a National Survey. Med Care Res Rev. 2014;71(4):356–366. , , , .
- Tufts CTSI. REDCap [Internet]. Tufts Clinical and Translational Science Institute. http://www.tuftsctsi.org/Services-and-Consultation/REDCap.aspx. Accessed May, 15, 2015.
- Multi‐dimensional Assessment of Dyspnea. Dyspnoea in Advanced Disease: A guide to clinical management; 2005. , .
- Cancer care quality measures: symptoms and end‐of‐life care. Evid Rep Technol Assess (Full Rep). 2006(137):1–77. , , , et al.
- Defining and measuring quality palliative and end‐of‐life care in the intensive care unit. Crit Care Med. 2006;34(11 Suppl):S309–316. .
- Validation of a vertical visual analogue scale as a measure of clinical dyspnea. Rehabil Nurs. 1989;14(6):323–325. .
- Can a self‐rating 0‐10 scale for dyspnea yield a common language that is understood by ED nurses, patients, and their families? J Emerg Nurs. 2000;26(3):233–234. .
- Measurement of dyspnea: word labeled visual analog scale vs. verbal ordinal scale. Respir Physiol Neurobiol. 2003;134(2):77–83. , , .
- Verbal numerical scales are as reliable and sensitive as visual analog scales for rating dyspnea in young and older subjects. Respir Physiol Neurobiol. 2007;157(2‐3):360–365. , , , , , .
- Validation of a three‐factor measurement model of dyspnea in hospitalized adults with heart failure. Heart Lung. 2011;41(1):44–56. , , , , .
- Patient reporting and doctor recognition of dyspnoea in a comprehensive cancer centre. Intern Med J. 2006;36(6):381–384. , , .
- Lung cancer and dyspnea: the patient's perception. Oncol Nurs Forum. 1986;13(5):19–24. , , , .
- Opioids, respiratory function, and dyspnea. Am J Hosp Palliat Care. 2003;20(1):57–61. , , , .
- An Official American Thoracic Society Statement: Update on the Mechanisms, Assessment, and Management of Dyspnea. Am J Respir Crit Care Med. 2012;185(4):435–452. , , , et al.
- CDC/ National Center for Health Statistics. National Hospital Amulatory Medical Care Survey: 2011 Emergency Department Summary Tables. http://www.cdc.gov/nchs/data/ahcd/nhamcs_emergency/2011_ed_web_tables.pdf. Accessed May 15, 2015.
- Signs and symptoms of heart failure: are you asking the right questions? Am J Crit Care. 2010;19(5):443–452. , , , .
- Managing dyspnea in patients with advanced chronic obstructive pulmonary disease: a Canadian Thoracic Society clinical practice guideline. Can Respir J. 2011;18(2):69–78. , , , et al.
- Prevalence of distressing symptoms in hospitalised patients on medical wards: A cross‐sectional study. BMC Palliat Care. 2008;7:16. , .
- Dyspnea in terminally ill cancer patients. Chest. 1986;89(2):234–236. , .
- American College of Chest Physicians consensus statement on the management of dyspnea in patients with advanced lung or heart disease. Chest. 2010;137(3):674–691. , , , et al.
- Common symptoms in ambulatory care: incidence, evaluation, therapy, and outcome. Am J Med. 1989;86(3):262–266. , .
- The Joint Commission. Facts about Pain Management. http://www.jointcommission.org/pain_management/. Accessed May, 15, 2015.
- Hospitalist programs in the age of healthcare reform. J Healthc Manag. 2010;55(6):378–380. .
- The Use of Hospitalists by Small Rural Hospitals: Results of a National Survey. Med Care Res Rev. 2014;71(4):356–366. , , , .
- Tufts CTSI. REDCap [Internet]. Tufts Clinical and Translational Science Institute. http://www.tuftsctsi.org/Services-and-Consultation/REDCap.aspx. Accessed May, 15, 2015.
- Multi‐dimensional Assessment of Dyspnea. Dyspnoea in Advanced Disease: A guide to clinical management; 2005. , .
- Cancer care quality measures: symptoms and end‐of‐life care. Evid Rep Technol Assess (Full Rep). 2006(137):1–77. , , , et al.
- Defining and measuring quality palliative and end‐of‐life care in the intensive care unit. Crit Care Med. 2006;34(11 Suppl):S309–316. .
- Validation of a vertical visual analogue scale as a measure of clinical dyspnea. Rehabil Nurs. 1989;14(6):323–325. .
- Can a self‐rating 0‐10 scale for dyspnea yield a common language that is understood by ED nurses, patients, and their families? J Emerg Nurs. 2000;26(3):233–234. .
- Measurement of dyspnea: word labeled visual analog scale vs. verbal ordinal scale. Respir Physiol Neurobiol. 2003;134(2):77–83. , , .
- Verbal numerical scales are as reliable and sensitive as visual analog scales for rating dyspnea in young and older subjects. Respir Physiol Neurobiol. 2007;157(2‐3):360–365. , , , , , .
- Validation of a three‐factor measurement model of dyspnea in hospitalized adults with heart failure. Heart Lung. 2011;41(1):44–56. , , , , .
- Patient reporting and doctor recognition of dyspnoea in a comprehensive cancer centre. Intern Med J. 2006;36(6):381–384. , , .
- Lung cancer and dyspnea: the patient's perception. Oncol Nurs Forum. 1986;13(5):19–24. , , , .
- Opioids, respiratory function, and dyspnea. Am J Hosp Palliat Care. 2003;20(1):57–61. , , , .
© 2015 Society of Hospital Medicine
Impact of HOCDI on Sepsis Patients
There are approximately 3 million cases of Clostridium difficile infection (CDI) per year in the United States.[1, 2, 3, 4] Of these, 10% result in a hospitalization or occur as a consequence of the exposures and treatments associated with hospitalization.[1, 2, 3, 4] Some patients with CDI experience mild diarrhea that is responsive to therapy, but other patients experience severe, life‐threatening disease that is refractory to treatment, leading to pseudomembranous colitis, toxic megacolon, and sepsis with a 60‐day mortality rate that exceeds 12%.[5, 6, 7, 8, 9, 10, 11, 12, 13, 14]
Hospital‐onset CDI (HOCDI), defined as C difficile‐associated diarrhea and related symptoms with onset more than 48 hours after admission to a healthcare facility,[15] represents a unique marriage of CDI risk factors.[5] A vulnerable patient is introduced into an environment that contains both exposure to C difficile (through other patients or healthcare workers) and treatment with antibacterial agents that may diminish normal flora. Consequently, CDI is common among hospitalized patients.[16, 17, 18] A particularly important group for understanding the burden of disease is patients who initially present to the hospital with sepsis and subsequently develop HOCDI. Sepsis patients are often critically ill and are universally treated with antibiotics.
Determining the incremental cost and mortality risk attributable to HOCDI is methodologically challenging. Because HOCDI is associated with presenting severity, the sickest patients are also the most likely to contract the disease. HOCDI is also associated with time of exposure or length of stay (LOS). Because LOS is a risk factor, comparing LOS between those with and without HOCDI will overestimate the impact if the time to diagnosis is not taken into account.[16, 17, 19, 20] We aimed to examine the impact of HOCDI in hospitalized patients with sepsis using a large, multihospital database with statistical methods that took presenting severity and time to diagnosis into account.
METHODS
Data Source and Subjects
Permission to conduct this study was obtained from the institutional review board at Baystate Medical Center. We used the Premier Healthcare Informatics database, a voluntary, fee‐supported database created to measure quality and healthcare utilization, which has been used extensively in health services research.[21, 22, 23] In addition to the elements found in hospital claims derived from the uniform billing 04 form, Premier data include an itemized, date‐stamped log of all items and services charged to the patient or their insurer, including medications, laboratory tests, and diagnostic and therapeutic services. Approximately 75% of hospitals that submit data also provide information on actual hospital costs, taken from internal cost accounting systems. The rest provide cost estimates based on Medicare cost‐to‐charge ratios. Participating hospitals are similar to the composition of acute care hospitals nationwide, although they are more commonly small‐ to midsized nonteaching facilities and are more likely to be located in the southern United States.
We included medical (nonsurgical) adult patients with sepsis who were admitted to a participating hospital between July 1, 2004 and December 31, 2010. Because we sought to focus on the care of patients who present to the hospital with sepsis, we defined sepsis as the presence of a diagnosis of sepsis plus evidence of both blood cultures and antibiotic treatment within the first 2 days of hospitalization; we used the first 2 days of hospitalization rather than just the first day because, in administrative datasets, the duration of the first hospital day includes partial days that can vary in length. We excluded patients who died or were discharged prior to day 3, because HOCDI is defined as onset after 48 hours in a healthcare facility.[15] We also excluded surviving patients who received less than 3 consecutive days of antibiotics, and patients who were transferred from or to another acute‐care facility; the latter exclusion criterion was used because we could not accurately determine the onset or subsequent course of their illness.
Identification of Patients at Risk for and Diagnosed With HOCDI
Among eligible patients with sepsis, we aimed to identify a cohort at risk for developing CDI during the hospital stay. We excluded patients: (1) with a diagnosis indicating that diarrhea was present on admission, (2) with a diagnosis of CDI that was indicated to be present on admission, (3) who were tested for CDI on the first or second hospital day, and (4) who received an antibiotic that could be consistent with treatment for CDI (oral or intravenous [IV] metronidazole or oral vancomycin) on hospital days 1 or 2.
Next, we aimed to identify sepsis patients at risk for HOCDI who developed HOCDI during their hospital stay. Among eligible patients described above, we considered a patient to have HOCDI if they had an International Classification of Diseases, Ninth Revision, Clinical Modification diagnosis of CDI (primary or secondary but not present on admission), plus evidence of testing for CDI after hospital day 2, and treatment with oral vancomycin or oral or IV metronidazole that was started after hospital day 2 and within 2 days of the C difficile test, and evidence of treatment for CDI for at least 3 days unless the patient was discharged or died.
Patient Information
We recorded patient age, gender, marital status, insurance status, race, and ethnicity. Using software provided by the Healthcare Costs and Utilization Project of the Agency for Healthcare Research and Quality, we categorized information on 30 comorbid conditions. We also created a single numerical comorbidity score based on a previously published and validated combined comorbidity score that predicts 1‐year mortality.[24] Based on a previously described algorithm,[25] we used diagnosis codes to assess the source (lung, abdomen, urinary tract, blood, other) and type of sepsis (Gram positive, Gram negative, mixed, anaerobic, fungal). Because patients can have more than 1 potential source of sepsis (eg, pneumonia and urinary tract infection) and more than 1 organism causing infection (eg, urine with Gram negative rods and blood culture with Gram positive cocci), these categories are not mutually exclusive (see Supporting Table 1 in the online version of this article). We used billing codes to identify the use of therapies, monitoring devices, and pharmacologic treatments to characterize both initial severity of illness and severity at the time of CDI diagnosis. These therapies are included in a validated sepsis mortality prediction model (designed for administrative datasets) with similar discrimination and calibration to clinical intensive care unit (ICU) risk‐adjustment models such as the mortality probability model, version III.[26, 27]
Outcomes
Our primary outcome of interest was in‐hospital mortality. Secondary outcomes included LOS and costs for survivors only and for all patients.
Statistical Methods
We calculated patient‐level summary statistics for all patients using frequencies for binary variables and medians and interquartile percentiles for continuous variables. P values <0.05 were considered statistically significant.
To account for presenting severity and time to diagnosis, we used methods that have been described elsewhere.[12, 13, 18, 20, 28] First, we identified patients who were eligible to develop HOCDI. Second, for all eligible patients, we identified a date of disease onset (index date). For patients who met criteria for HOCDI, this was the date on which the patient was tested for CDI. For eligible patients without disease, this was a date randomly assigned to any time during the hospital stay.[29] Next, we developed a nonparsimonious propensity score model that included all patient characteristics (demographics, comorbidities, sepsis source, and severity of illness on presentation and on the index date; all variables listed in Table 1 were included in the propensity model). Some of the variables for this model (eg, mechanical ventilation and vasopressors) were derived from a validated severity model.[26] We adjusted for correlation within hospital when creating the propensity score using Huber‐White robust standard error estimators clustered at the hospital level.[30] We then created matched pairs with the same LOS prior to the index date and similar propensity for developing CDI. We first matched on index date, and then, within each index‐datematched subset, matched patients with and without HOCDI by their propensity score using a 5‐to‐1 greedy match algorithm.[31] We used the differences in LOS between the cases and controls after the index date to calculate the additional attributable LOS estimates; we also separately estimated the impact on cost and LOS in a group limited to those who survived after discharge because of concerns that death could shorten LOS and reduce costs.
Before Matching | After Matching | |||||
---|---|---|---|---|---|---|
HOCDI, n=2,368, % | No CDI, n=216,547, % | P | HOCDI, n=2,368, % | No CDI, n=2,368, % | P | |
| ||||||
Age, y | 70.9 (15.1) | 68.6 (16.8) | <0.01 | 70.9 (15.1) | 69.8 (15.9) | 0.02 |
Male | 46.8 | 46.0 | 0.44 | 46.8 | 47.2 | 0.79 |
Race | ||||||
White | 61.0 | 63.3 | 61.0 | 58.1 | ||
Black | 15.6 | 14.5 | <0.01 | 15.6 | 17.0 | 0.11 |
Hispanic | 3.2 | 5.4 | 3.2 | 4.1 | ||
Other race | 20.2 | 16.8 | 20.2 | 20.9 | ||
Marital status | ||||||
Married | 31.6 | 36.3 | <0.01 | 31.6 | 32.6 | 0.74 |
Single/divorced | 52.8 | 51.1 | 52.8 | 52.0 | ||
Other/unknown | 15.7 | 12.6 | 15.7 | 14.5 | ||
Insurance status | ||||||
Medicare traditional | 63.2 | 59.5 | 63.2 | 60.3 | ||
Medicare managed | 10.6 | 10.1 | 10.6 | 10.9 | ||
Medicaid traditional | 7.6 | 6.9 | 7.6 | 8.2 | ||
Medicaid managed | 1.8 | 2.0 | <0.01 | 1.8 | 1.8 | 0.50 |
Managed care | 10.8 | 12.3 | 10.8 | 12.0 | ||
Commercial | 2.0 | 3.5 | 2.0 | 2.2 | ||
Self‐pay/other/unknown | 4.0 | 5.7 | 4.0 | 4.7 | ||
Infection source | ||||||
Respiratory | 46.5 | 37.0 | <0.01 | 46.5 | 49.6 | 0.03 |
Skin/bone | 10.1 | 8.6 | 0.01 | 10.1 | 11.2 | 0.21 |
Urinary | 52.2 | 51.3 | 0.38 | 52.2 | 50.3 | 0.18 |
Blood | 11.1 | 15.1 | <0.01 | 11.1 | 11.5 | 0.65 |
Infecting organism | ||||||
Gram negative | 35.0 | 36.6 | <0.01 | 35.0 | 33.1 | 0.18 |
Anaerobe | 1.4 | 0.7 | <0.01 | 1.4 | 1.1 | 0.24 |
Fungal | 17.5 | 7.5 | <0.01 | 17.5 | 18.3 | 0.44 |
Most common comorbid conditions | ||||||
Congestive heart failure | 35.1 | 24.6 | <0.01 | 35.1 | 37.5 | 0.06 |
Chronic lung disease | 31.6 | 27.6 | <0.01 | 31.6 | 32.1 | 0.71 |
Hypertension | 31.5 | 37.7 | <0.01 | 31.5 | 29.7 | 0.16 |
Renal Failure | 29.7 | 23.8 | <0.01 | 29.7 | 31.2 | 0.28 |
Weight Loss | 27.7 | 13.3 | <0.01 | 27.7 | 29.4 | 0.17 |
Treatments by day 2 | ||||||
ICU admission | 40.0 | 29.5 | <0.01 | 40.0 | 40.7 | 0.64 |
Use of bicarbonate | 12.2 | 7.1 | <0.01 | 12.2 | 13.6 | 0.15 |
Fresh frozen plasma | 1.4 | 1.0 | 0.03 | 1.4 | 1.1 | 0.36 |
Inotropes | 1.4 | 0.9 | 0.01 | 1.4 | 2.2 | 0.04 |
Hydrocortisone | 6.7 | 4.7 | <0.01 | 6.7 | 7.4 | 0.33 |
Thiamine | 4.2 | 3.3 | 0.01 | 4.2 | 4.1 | 0.83 |
Psychotropics (eg, haldol for delirium) | 10.0 | 9.2 | 0.21 | 10.0 | 10.8 | 0.36 |
Restraints (eg, for delirium) | 2.0 | 1.5 | 0.05 | 2.0 | 2.5 | 0.29 |
Angiotensin‐converting enzyme inhibitors | 12.1 | 13.2 | 0.12 | 12.1 | 10.9 | 0.20 |
Statins | 18.8 | 21.1 | 0.01 | 18.8 | 16.9 | 0.09 |
Drotrecogin alfa | 0.6 | 0.3 | 0.00 | 0.6 | 0.6 | 0.85 |
Foley catheter | 19.2 | 19.8 | 0.50 | 19.2 | 22.0 | 0.02 |
Diuretics | 28.5 | 25.4 | 0.01 | 28.5 | 29.6 | 0.42 |
Red blood cells | 15.5 | 10.6 | <0.01 | 15.5 | 15.8 | 0.81 |
Calcium channel blockers | 19.3 | 16.8 | 0.01 | 19.3 | 19.1 | 0.82 |
‐Blockers | 32.7 | 29.6 | 0.01 | 32.7 | 30.6 | 0.12 |
Proton pump inhibitors | 59.6 | 53.1 | <0.01 | 59.6 | 61.0 | 0.31 |
Analysis Across Clinical Subgroups
In a secondary analysis, we examined heterogeneity in the association between HOCDI and outcomes within subsets of patients defined by age, combined comorbidity score, and admission to the ICU by day 2. We created separate propensity scores using the same covariates in the primary analysis, but limited matches to within these subsets. For each group, we examined how the covariates in the HOCDI and control groups differed after matching with inference tests that took the paired nature of the data into account. All analyses were carried out using Stata/SE 11.1 (StataCorp, College Station, TX).
RESULTS
We identified 486,943 adult sepsis admissions to a Premier hospital between July 1, 2004 and December 31, 2010. After applying all exclusion criteria, we had a final sample of 218,915 admissions with sepsis (from 400 hospitals) at risk for HOCDI (Figure 1). Of these, 2368 (1.08%) met criteria for diagnosis of CDI after hospital day 2 and were matched to controls using index date and propensity score.
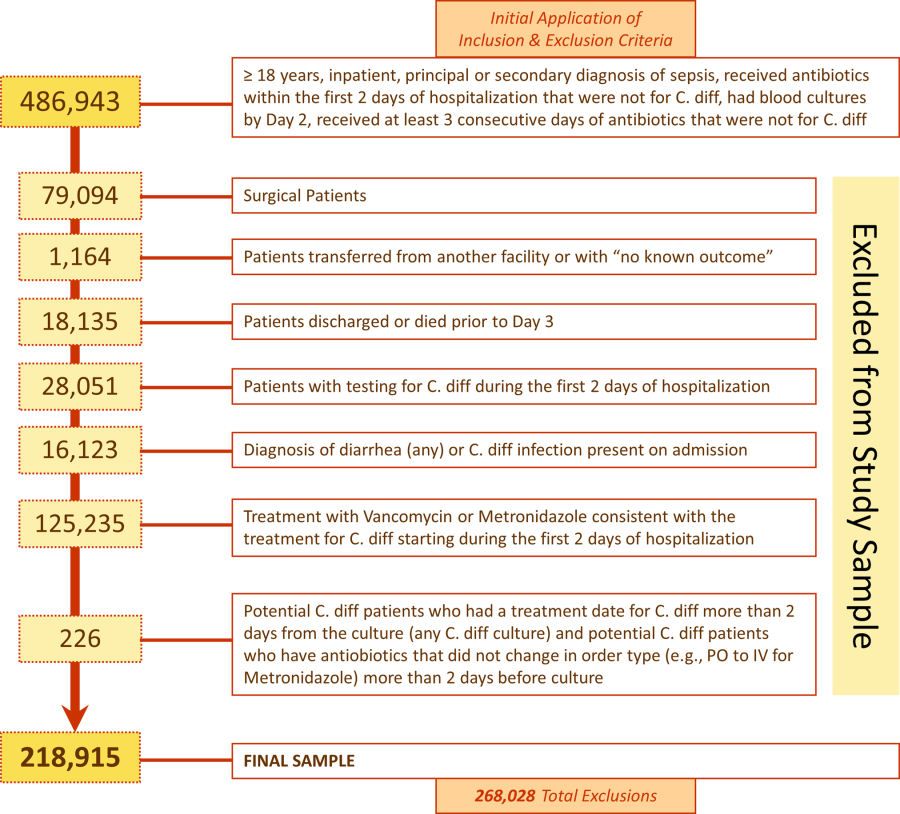
Patient and Hospital Factors
After matching, the median age was 71 years in cases and 70 years in controls (Table 1). Less than half (46%) of the population was male. Most cases (61%) and controls (58%) were white. Heart failure, hypertension, chronic lung disease, renal failure, and weight loss were the most common comorbid conditions. Our propensity model, which had a C statistic of 0.75, identified patients whose risk varied from a mean of 0.1% in the first decile to a mean of 3.8% in the tenth decile. Before matching, 40% of cases and 29% of controls were treated in the ICU by hospital day 2; after matching, 40% of both cases and controls were treated in the ICU by hospital day 2.
Distribution by LOS, Index Day, and Risk for Mortality
The unadjusted and unmatched LOS was longer for cases than controls (19 days vs 8 days, Table 2) (see Supporting Figure 1 in the online version of this article). Approximately 90% of the patients had an index day of 14 or less (Figure 2). Among patients both with and without CDI, the unadjusted mortality risk increased as the index day (and thus the total LOS) increased.
Outcome | HOCDI | No HOCDI | Difference (95% CI) | P |
---|---|---|---|---|
| ||||
Length of stay, d | ||||
Raw results | 19.2 | 8.3 | 8.4 (8.48.5) | <0.01 |
Raw results for survivors only | 18.6 | 8.0 | 10.6 (10.311.0) | <0.01 |
Matched results | 19.2 | 14.2 | 5.1(4.45.7) | <0.01 |
Matched results for survivors only | 18.6 | 13.6 | 5.1 (4.45.8) | <0.01 |
Mortality, % | ||||
Raw results | 24.0 | 10.1 | 13.9 (12.615.1), RR=2.4 (2.22.5) | <0.01 |
Matched results | 24.0 | 15.4 | 8.6 (6.410.9), RR=1.6 (1.41.8) | <0.01 |
Costs, US$ | ||||
Raw results median costs [interquartile range] | $26,187 [$15,117$46,273] | $9,988 [$6,296$17,351] | $16,190 ($15,826$16,555) | <0.01 |
Raw results for survivors only [interquartile range] | $24,038 [$14,169$41,654] | $9,429 [$6,070$15,875] | $14,620 ($14,246$14,996) | <0.01 |
Matched results [interquartile range] | $26,187 [$15,117$46,273] | $19,160 [$12,392$33,777] | $5,308 ($4,521$6,108) | |
Matched results for survivors only [interquartile range] | $24,038 [$14,169$41,654] | $17,811 [$11,614$29,298] | $4,916 ($4,088$5,768) | <0.01 |
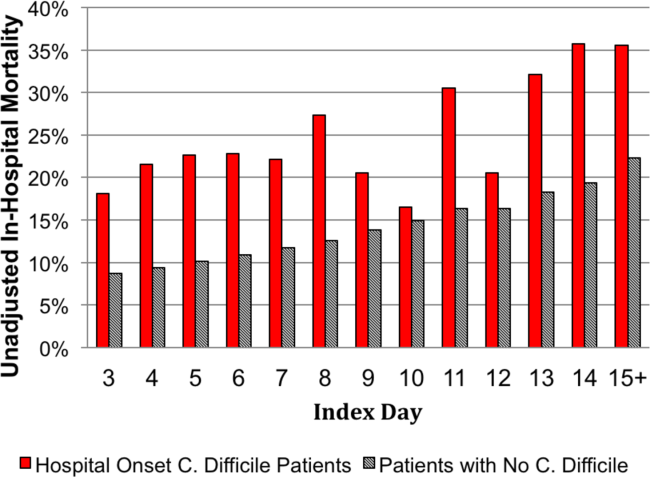
Adjusted Results
Compared to patients without disease, HOCDI patients had an increased unadjusted mortality (24% vs 10%, P<0.001). This translates into a relative risk of 2.4 (95% confidence interval [CI]: 2.2, 2.5). In the matched cohort, the difference in the mortality rates was attenuated, but still significantly higher in the HOCDI patients (24% versus 15%, P<0.001, an absolute difference of 9%; 95% CI: 6.410.8). The adjusted relative risk of mortality for HOCDI was 1.6 (95% CI: 1.41.8; Table 2). After matching, patients with CDI had a LOS of 19.2 days versus 14.2 days in matched controls (difference of 5.1 days; 95% CI: 4.45.7; P<0.001). When the LOS analysis was limited to survivors only, this difference of 5 days remained (P<0.001). In an analysis limited to survivors only, the difference in median costs between cases and controls was $4916 (95% CI: $4088$5768; P<0.001). In a secondary analysis examining heterogeneity between HOCDI and outcomes across clinical subgroups, the absolute difference in mortality and costs between cases and controls varied across demographics, comorbidity, and ICU admission, but the relative risks were similar (Figure 3) (see Supporting Figure 3 in the online version of this article).
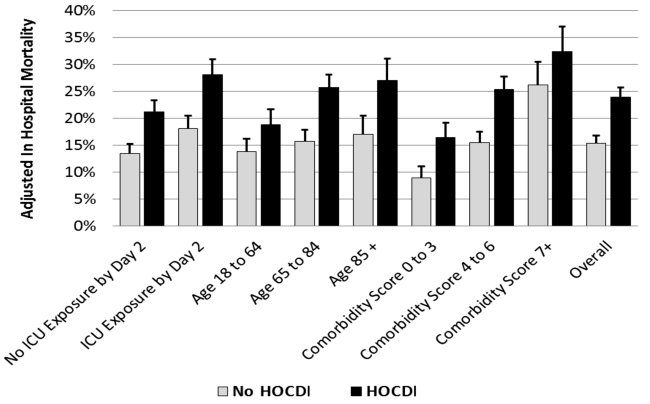
DISCUSSION
In this large cohort of patients with sepsis, we found that approximately 1 in 100 patients with sepsis developed HOCDI. Even after matching with controls based on the date of symptom onset and propensity score, patients who developed HOCDI were more than 1.6 times more likely to die in the hospital. HOCDI also added 5 days to the average hospitalization for patients with sepsis and increased median costs by approximately $5000. These findings suggest that a hospital that prevents 1 case of HOCDI per month in sepsis patients could avoid 1 death and 60 inpatient days annually, achieving an approximate yearly savings of $60,000.
Until now, the incremental cost and mortality attributable to HOCDI in sepsis patients have been poorly understood. Attributing outcomes can be methodologically challenging because patients who are at greatest risk for poor outcomes are the most likely to contract the disease and are at risk for longer periods of time. Therefore, it is necessary to take into account differences in severity of illness and time at risk between diseased and nondiseased populations and to ensure that outcomes attributed to the disease occur after disease onset.[28, 32] The majority of prior studies examining the impact of CDI on hospitalized patients have been limited by a lack of adequate matching to controls, small sample size, or failure to take into account time to infection.[16, 17, 19, 20]
A few studies have taken into account severity, time to infection, or both in estimating the impact of HOCDI. Using a time‐dependent Cox model that accounted for time to infection, Micek et al. found no difference in mortality but a longer LOS in mechanically ventilated patients (not limited to sepsis) with CDI.[33] However, their study was conducted at only 3 centers, did not take into account severity at the time of diagnosis, and did not clearly distinguish between community‐onset CDI and HOCDI. Oake et al. and Forster et al. examined the impact of CDI on patients hospitalized in a 2‐hospital health system in Canada.[12, 13] Using the baseline mortality estimate in a Cox multivariate proportional hazards regression model that accounted for the time‐varying nature of CDI, they found that HOCDI increased absolute risk of death by approximately 10%. Also, notably similar to our study were their findings that HOCDI occurred in approximately 1 in 100 patients and that the attributable median increase in LOS due to hospital‐onset CDI was 6 days. Although methodologically rigorous, these 2 small studies did not assess the impact of CDI on costs of care, were not focused on sepsis patients or even patients who received antibiotics, and also did not clearly distinguish between community‐onset CDI and HOCDI.
Our study therefore has important strengths. It is the first to examine the impact of HOCDI, including costs, on the outcomes of patients hospitalized with sepsis. The fact that we took into account both time to diagnosis and severity at the time of diagnosis (by using an index date for both cases and controls and determining severity on that date) prevented us from overestimating the impact of HOCDI on outcomes. The large differences in outcomes we observed in unadjusted and unmatched data were tempered after multivariate adjustment (eg, difference in LOS from 10.6 days to 5.1 additional days, costs from $14,620 to $4916 additional costs after adjustment). Our patient sample was derived from a large, multihospital database that contains actual hospital costs as derived from internal accounting systems. The fact that our study used data from hundreds of hospitals means that our estimates of cost, LOS, and mortality may be more generalizable than the work of Micek et al., Oake et al., and Forster et al.
This work also has important implications. First, hospital administrators, clinicians, and researchers can use our results to evaluate the cost‐effectiveness of HOCDI prevention measures (eg, hand hygiene programs, antibiotic stewardship). By quantifying the cost per case in sepsis patients, we allow administrators and researchers to compare the incremental costs of HOCDI prevention programs to the dollars and lives saved due to prevention efforts. Second, we found that our propensity model identified patients whose risk varied greatly. This suggests that an opportunity exists to identify subgroups of patients that are at highest risk. Identifying high‐risk subgroups will allow for targeted risk reduction interventions and the opportunity to reduce transmission (eg, by placing high‐risk patients in a private room). Finally, we have reaffirmed that time to diagnosis and presenting severity need to be rigorously addressed prior to making estimates of the impact of CDI burden and other hospital‐acquired conditions and injuries.
There are limitations to this study as well. We did not have access to microbiological data. However, we required a diagnosis code of CDI, evidence of testing, and treatment after the date of testing to confirm a diagnosis. We also adopted detailed exclusion criteria to ensure that CDI that was not present on admission and that controls did not have CDI. These stringent inclusion and exclusion criteria strengthened the internal validity of our estimates of disease impact. We used administrative claims data, which limited our ability to adjust for severity. However, the detailed nature of the database allowed us to use treatments, such as vasopressors and antibiotics, to identify cases; treatments were also used as a validated indicator of severity,[26] which may have helped to reduce some of this potential bias. Although our propensity model included many predictors of CDI, such as use of proton pump inhibitors and factors associated with mortality, not every confounder was completely balanced after propensity matching, although the statistical differences may have been related to our large sample size and therefore might not be clinically significant. We also may have failed to include all possible predictors of CDI in the propensity model.
In a large, diverse cohort of hospitalized patients with sepsis, we found that HOCDI lengthened hospital stay by approximately 5 days, increased risk of in‐hospital mortality by 9%, and increased hospital cost by approximately $5000 per patient. These findings highlight the importance of identifying effective prevention measures and of determining the patient populations at greatest risk for HOCDI.
Disclosures: The study was conducted with funding from the Division of Critical Care and the Center for Quality of Care Research at Baystate Medical Center. Dr. Lagu is supported by the National Heart, Lung, and Blood Institute of the National Institutes of Health under award number K01HL114745. Dr. Stefan is supported by the National Heart, Lung, and Blood Institute of the National Institutes of Health under award number K01HL114631. Drs. Lagu and Lindenauer had full access to all of the data in the study; they take responsibility for the integrity of the data and the accuracy of the data analysis. Drs. Lagu, Lindenauer, Steingrub, Higgins, Stefan, Haessler, and Rothberg conceived of the study. Dr. Lindenauer acquired the data. Drs. Lagu, Lindenauer, Rothberg, Steingrub, Nathanson, Stefan, Haessler, Higgins, and Mr. Hannon analyzed and interpreted the data. Dr. Lagu drafted the manuscript. Drs. Lagu, Lindenauer, Rothberg, Steingrub, Nathanson, Stefan, Haessler, Higgins, and Mr. Hannon critically reviewed the manuscript for important intellectual content. Dr. Nathanson carried out the statistical analyses. Dr. Nathanson, through his company OptiStatim LLC, was paid by the investigators with funding from the Department of Medicine at Baystate Medical Center to assist in conducting the statistical analyses in this study. The authors report no further conflicts of interest.
- Increasing prevalence and severity of Clostridium difficile colitis in hospitalized patients in the United States. Arch Surg. 2007;142(7):624–631; discussion 631. , , , .
- The changing epidemiology of Clostridium difficile infections. Clin Microbiol Rev. 2010;23(3):529–549. , , , et al.
- Clostridium Difficile‐Associated Disease in U.S. Hospitals, 1993–2005. HCUP Statistical Brief #50. April 2008. Agency for Healthcare Research and Quality, Rockville, MD. Available at: http://www.hcup‐us.ahrq.gov/reports/statbriefs/sb50.pdf. Accessed April 4, 2014. , .
- National point prevalence of Clostridium difficile in US health care facility inpatients, 2008. Am J Infect Control. 2009;37(4):263–270. , , , .
- A 76‐year‐old man with recurrent Clostridium difficile‐associated diarrhea: review of C. difficile infection. JAMA. 2009;301(9):954–962. .
- Recurrent Clostridium difficile disease: epidemiology and clinical characteristics. Infect Control Hosp Epidemiol. 1999;20(1):43–50. , , , , , .
- Recurrent Clostridium difficile diarrhea: characteristics of and risk factors for patients enrolled in a prospective, randomized, double‐blinded trial. Clin Infect Dis. 1997;24(3):324–333. , , , , , .
- Narrative review: the new epidemic of Clostridium difficile‐associated enteric disease. Ann Intern Med. 2006;145(10):758–764. .
- Impact of emergency colectomy on survival of patients with fulminant Clostridium difficile colitis during an epidemic caused by a hypervirulent strain. Ann Surg. 2007;245(2):267–272. , , , et al.
- Hospital‐acquired Clostridium difficile‐associated disease in the intensive care unit setting: epidemiology, clinical course and outcome. BMC Infect Dis. 2007;7:42. , , , .
- Factors associated with prolonged symptoms and severe disease due to Clostridium difficile. Age Ageing. 1999;28(2):107–113. , , , , , .
- The effect of hospital‐acquired Clostridium difficile infection on in‐hospital mortality. Arch Intern Med. 2010;170(20):1804–1810. , , , , , .
- The effect of hospital‐acquired infection with Clostridium difficile on length of stay in hospital. CMAJ. 2012;184(1):37–42. , , , , , .
- Clostridium difficile—more difficult than ever. N Engl J Med. 2008;359(18):1932–1940. , .
- Clinical practice guidelines for Clostridium difficile infection in adults: 2010 update by the society for healthcare epidemiology of America (SHEA) and the infectious diseases society of America (IDSA). Infect Control Hosp Epidemiol. 2010;31(5):431–455. , , , et al.
- Health care costs and mortality associated with nosocomial diarrhea due to Clostridium difficile. Clin Infect Dis. 2002;34(3):346–353. , , , .
- Short‐ and long‐term attributable costs of Clostridium difficile‐associated disease in nonsurgical inpatients. Clin Infect Dis. 2008;46(4):497–504. , , , , .
- Estimation of extra hospital stay attributable to nosocomial infections: heterogeneity and timing of events. J Clin Epidemiol. 2000;53(4):409–417. , , , , .
- Attributable outcomes of endemic Clostridium difficile‐associated disease in nonsurgical patients. Emerging Infect Dis. 2008;14(7):1031–1038. , , , et al.
- Excess length of stay, charges, and mortality attributable to medical injuries during hospitalization. JAMA. 2003;290(14):1868–1874. , .
- Association of corticosteroid dose and route of administration with risk of treatment failure in acute exacerbation of chronic obstructive pulmonary disease. JAMA. 2010;303(23):2359–2367. , , , , , .
- The relationship between hospital spending and mortality in patients with sepsis. Arch Intern Med. 2011;171(4):292–299. , , , , , .
- Comparative effectiveness of macrolides and quinolones for patients hospitalized with acute exacerbations of chronic obstructive pulmonary disease (AECOPD). J Hosp Med. 2010;5(5):261–267. , , , , , .
- A combined comorbidity score predicted mortality in elderly patients better than existing scores. J Clin Epidemiol. 2011;64(7):749–759. , , , , .
- Epidemiology of severe sepsis in the United States: analysis of incidence, outcome, and associated costs of care. Crit Care Med. 2001;29(7):1303–1310. , , , , , .
- Development and validation of a model that uses enhanced administrative data to predict mortality in patients with sepsis. Crit Care Med. 2011;39(11):2425–2430. , , , et al.
- Incorporating initial treatments improves performance of a mortality prediction model for patients with sepsis. Pharmacoepidemiol Drug Saf. 2012;21(suppl 2):44–52. , , , , .
- Nosocomial infection, length of stay, and time‐dependent bias. Infect Control Hosp Epidemiol. 2009;30(3):273–276. , , , .
- Length of stay and hospital costs among high‐risk patients with hospital‐origin Clostridium difficile‐associated diarrhea. J Med Econ. 2013;16(3):440–448. , , , , , .
- Rogers. Regression standard errors in clustered samples. Stata Technical Bulletin. 1993;13(13):19–23.
- Reducing bias in a propensity score matched‐pair sample using greedy matching techniques. In: Proceedings of the 26th Annual SAS Users Group International Conference; April 22–25, 2001; Long Beach, CA. Paper 214‐26. Available at: http://www2.sas.com/proceedings/sugi26/p214‐26.pdf. Accessed April 4, 2014. .
- Prolongation of length of stay and Clostridium difficile infection: a review of the methods used to examine length of stay due to healthcare associated infections. Antimicrob Resist Infect Control. 2012;1(1):14. , .
- Clostridium difficile Infection: a multicenter study of epidemiology and outcomes in mechanically ventilated patients. Crit Care Med. 2013;41(8):1968–1975. , , , et al.
There are approximately 3 million cases of Clostridium difficile infection (CDI) per year in the United States.[1, 2, 3, 4] Of these, 10% result in a hospitalization or occur as a consequence of the exposures and treatments associated with hospitalization.[1, 2, 3, 4] Some patients with CDI experience mild diarrhea that is responsive to therapy, but other patients experience severe, life‐threatening disease that is refractory to treatment, leading to pseudomembranous colitis, toxic megacolon, and sepsis with a 60‐day mortality rate that exceeds 12%.[5, 6, 7, 8, 9, 10, 11, 12, 13, 14]
Hospital‐onset CDI (HOCDI), defined as C difficile‐associated diarrhea and related symptoms with onset more than 48 hours after admission to a healthcare facility,[15] represents a unique marriage of CDI risk factors.[5] A vulnerable patient is introduced into an environment that contains both exposure to C difficile (through other patients or healthcare workers) and treatment with antibacterial agents that may diminish normal flora. Consequently, CDI is common among hospitalized patients.[16, 17, 18] A particularly important group for understanding the burden of disease is patients who initially present to the hospital with sepsis and subsequently develop HOCDI. Sepsis patients are often critically ill and are universally treated with antibiotics.
Determining the incremental cost and mortality risk attributable to HOCDI is methodologically challenging. Because HOCDI is associated with presenting severity, the sickest patients are also the most likely to contract the disease. HOCDI is also associated with time of exposure or length of stay (LOS). Because LOS is a risk factor, comparing LOS between those with and without HOCDI will overestimate the impact if the time to diagnosis is not taken into account.[16, 17, 19, 20] We aimed to examine the impact of HOCDI in hospitalized patients with sepsis using a large, multihospital database with statistical methods that took presenting severity and time to diagnosis into account.
METHODS
Data Source and Subjects
Permission to conduct this study was obtained from the institutional review board at Baystate Medical Center. We used the Premier Healthcare Informatics database, a voluntary, fee‐supported database created to measure quality and healthcare utilization, which has been used extensively in health services research.[21, 22, 23] In addition to the elements found in hospital claims derived from the uniform billing 04 form, Premier data include an itemized, date‐stamped log of all items and services charged to the patient or their insurer, including medications, laboratory tests, and diagnostic and therapeutic services. Approximately 75% of hospitals that submit data also provide information on actual hospital costs, taken from internal cost accounting systems. The rest provide cost estimates based on Medicare cost‐to‐charge ratios. Participating hospitals are similar to the composition of acute care hospitals nationwide, although they are more commonly small‐ to midsized nonteaching facilities and are more likely to be located in the southern United States.
We included medical (nonsurgical) adult patients with sepsis who were admitted to a participating hospital between July 1, 2004 and December 31, 2010. Because we sought to focus on the care of patients who present to the hospital with sepsis, we defined sepsis as the presence of a diagnosis of sepsis plus evidence of both blood cultures and antibiotic treatment within the first 2 days of hospitalization; we used the first 2 days of hospitalization rather than just the first day because, in administrative datasets, the duration of the first hospital day includes partial days that can vary in length. We excluded patients who died or were discharged prior to day 3, because HOCDI is defined as onset after 48 hours in a healthcare facility.[15] We also excluded surviving patients who received less than 3 consecutive days of antibiotics, and patients who were transferred from or to another acute‐care facility; the latter exclusion criterion was used because we could not accurately determine the onset or subsequent course of their illness.
Identification of Patients at Risk for and Diagnosed With HOCDI
Among eligible patients with sepsis, we aimed to identify a cohort at risk for developing CDI during the hospital stay. We excluded patients: (1) with a diagnosis indicating that diarrhea was present on admission, (2) with a diagnosis of CDI that was indicated to be present on admission, (3) who were tested for CDI on the first or second hospital day, and (4) who received an antibiotic that could be consistent with treatment for CDI (oral or intravenous [IV] metronidazole or oral vancomycin) on hospital days 1 or 2.
Next, we aimed to identify sepsis patients at risk for HOCDI who developed HOCDI during their hospital stay. Among eligible patients described above, we considered a patient to have HOCDI if they had an International Classification of Diseases, Ninth Revision, Clinical Modification diagnosis of CDI (primary or secondary but not present on admission), plus evidence of testing for CDI after hospital day 2, and treatment with oral vancomycin or oral or IV metronidazole that was started after hospital day 2 and within 2 days of the C difficile test, and evidence of treatment for CDI for at least 3 days unless the patient was discharged or died.
Patient Information
We recorded patient age, gender, marital status, insurance status, race, and ethnicity. Using software provided by the Healthcare Costs and Utilization Project of the Agency for Healthcare Research and Quality, we categorized information on 30 comorbid conditions. We also created a single numerical comorbidity score based on a previously published and validated combined comorbidity score that predicts 1‐year mortality.[24] Based on a previously described algorithm,[25] we used diagnosis codes to assess the source (lung, abdomen, urinary tract, blood, other) and type of sepsis (Gram positive, Gram negative, mixed, anaerobic, fungal). Because patients can have more than 1 potential source of sepsis (eg, pneumonia and urinary tract infection) and more than 1 organism causing infection (eg, urine with Gram negative rods and blood culture with Gram positive cocci), these categories are not mutually exclusive (see Supporting Table 1 in the online version of this article). We used billing codes to identify the use of therapies, monitoring devices, and pharmacologic treatments to characterize both initial severity of illness and severity at the time of CDI diagnosis. These therapies are included in a validated sepsis mortality prediction model (designed for administrative datasets) with similar discrimination and calibration to clinical intensive care unit (ICU) risk‐adjustment models such as the mortality probability model, version III.[26, 27]
Outcomes
Our primary outcome of interest was in‐hospital mortality. Secondary outcomes included LOS and costs for survivors only and for all patients.
Statistical Methods
We calculated patient‐level summary statistics for all patients using frequencies for binary variables and medians and interquartile percentiles for continuous variables. P values <0.05 were considered statistically significant.
To account for presenting severity and time to diagnosis, we used methods that have been described elsewhere.[12, 13, 18, 20, 28] First, we identified patients who were eligible to develop HOCDI. Second, for all eligible patients, we identified a date of disease onset (index date). For patients who met criteria for HOCDI, this was the date on which the patient was tested for CDI. For eligible patients without disease, this was a date randomly assigned to any time during the hospital stay.[29] Next, we developed a nonparsimonious propensity score model that included all patient characteristics (demographics, comorbidities, sepsis source, and severity of illness on presentation and on the index date; all variables listed in Table 1 were included in the propensity model). Some of the variables for this model (eg, mechanical ventilation and vasopressors) were derived from a validated severity model.[26] We adjusted for correlation within hospital when creating the propensity score using Huber‐White robust standard error estimators clustered at the hospital level.[30] We then created matched pairs with the same LOS prior to the index date and similar propensity for developing CDI. We first matched on index date, and then, within each index‐datematched subset, matched patients with and without HOCDI by their propensity score using a 5‐to‐1 greedy match algorithm.[31] We used the differences in LOS between the cases and controls after the index date to calculate the additional attributable LOS estimates; we also separately estimated the impact on cost and LOS in a group limited to those who survived after discharge because of concerns that death could shorten LOS and reduce costs.
Before Matching | After Matching | |||||
---|---|---|---|---|---|---|
HOCDI, n=2,368, % | No CDI, n=216,547, % | P | HOCDI, n=2,368, % | No CDI, n=2,368, % | P | |
| ||||||
Age, y | 70.9 (15.1) | 68.6 (16.8) | <0.01 | 70.9 (15.1) | 69.8 (15.9) | 0.02 |
Male | 46.8 | 46.0 | 0.44 | 46.8 | 47.2 | 0.79 |
Race | ||||||
White | 61.0 | 63.3 | 61.0 | 58.1 | ||
Black | 15.6 | 14.5 | <0.01 | 15.6 | 17.0 | 0.11 |
Hispanic | 3.2 | 5.4 | 3.2 | 4.1 | ||
Other race | 20.2 | 16.8 | 20.2 | 20.9 | ||
Marital status | ||||||
Married | 31.6 | 36.3 | <0.01 | 31.6 | 32.6 | 0.74 |
Single/divorced | 52.8 | 51.1 | 52.8 | 52.0 | ||
Other/unknown | 15.7 | 12.6 | 15.7 | 14.5 | ||
Insurance status | ||||||
Medicare traditional | 63.2 | 59.5 | 63.2 | 60.3 | ||
Medicare managed | 10.6 | 10.1 | 10.6 | 10.9 | ||
Medicaid traditional | 7.6 | 6.9 | 7.6 | 8.2 | ||
Medicaid managed | 1.8 | 2.0 | <0.01 | 1.8 | 1.8 | 0.50 |
Managed care | 10.8 | 12.3 | 10.8 | 12.0 | ||
Commercial | 2.0 | 3.5 | 2.0 | 2.2 | ||
Self‐pay/other/unknown | 4.0 | 5.7 | 4.0 | 4.7 | ||
Infection source | ||||||
Respiratory | 46.5 | 37.0 | <0.01 | 46.5 | 49.6 | 0.03 |
Skin/bone | 10.1 | 8.6 | 0.01 | 10.1 | 11.2 | 0.21 |
Urinary | 52.2 | 51.3 | 0.38 | 52.2 | 50.3 | 0.18 |
Blood | 11.1 | 15.1 | <0.01 | 11.1 | 11.5 | 0.65 |
Infecting organism | ||||||
Gram negative | 35.0 | 36.6 | <0.01 | 35.0 | 33.1 | 0.18 |
Anaerobe | 1.4 | 0.7 | <0.01 | 1.4 | 1.1 | 0.24 |
Fungal | 17.5 | 7.5 | <0.01 | 17.5 | 18.3 | 0.44 |
Most common comorbid conditions | ||||||
Congestive heart failure | 35.1 | 24.6 | <0.01 | 35.1 | 37.5 | 0.06 |
Chronic lung disease | 31.6 | 27.6 | <0.01 | 31.6 | 32.1 | 0.71 |
Hypertension | 31.5 | 37.7 | <0.01 | 31.5 | 29.7 | 0.16 |
Renal Failure | 29.7 | 23.8 | <0.01 | 29.7 | 31.2 | 0.28 |
Weight Loss | 27.7 | 13.3 | <0.01 | 27.7 | 29.4 | 0.17 |
Treatments by day 2 | ||||||
ICU admission | 40.0 | 29.5 | <0.01 | 40.0 | 40.7 | 0.64 |
Use of bicarbonate | 12.2 | 7.1 | <0.01 | 12.2 | 13.6 | 0.15 |
Fresh frozen plasma | 1.4 | 1.0 | 0.03 | 1.4 | 1.1 | 0.36 |
Inotropes | 1.4 | 0.9 | 0.01 | 1.4 | 2.2 | 0.04 |
Hydrocortisone | 6.7 | 4.7 | <0.01 | 6.7 | 7.4 | 0.33 |
Thiamine | 4.2 | 3.3 | 0.01 | 4.2 | 4.1 | 0.83 |
Psychotropics (eg, haldol for delirium) | 10.0 | 9.2 | 0.21 | 10.0 | 10.8 | 0.36 |
Restraints (eg, for delirium) | 2.0 | 1.5 | 0.05 | 2.0 | 2.5 | 0.29 |
Angiotensin‐converting enzyme inhibitors | 12.1 | 13.2 | 0.12 | 12.1 | 10.9 | 0.20 |
Statins | 18.8 | 21.1 | 0.01 | 18.8 | 16.9 | 0.09 |
Drotrecogin alfa | 0.6 | 0.3 | 0.00 | 0.6 | 0.6 | 0.85 |
Foley catheter | 19.2 | 19.8 | 0.50 | 19.2 | 22.0 | 0.02 |
Diuretics | 28.5 | 25.4 | 0.01 | 28.5 | 29.6 | 0.42 |
Red blood cells | 15.5 | 10.6 | <0.01 | 15.5 | 15.8 | 0.81 |
Calcium channel blockers | 19.3 | 16.8 | 0.01 | 19.3 | 19.1 | 0.82 |
‐Blockers | 32.7 | 29.6 | 0.01 | 32.7 | 30.6 | 0.12 |
Proton pump inhibitors | 59.6 | 53.1 | <0.01 | 59.6 | 61.0 | 0.31 |
Analysis Across Clinical Subgroups
In a secondary analysis, we examined heterogeneity in the association between HOCDI and outcomes within subsets of patients defined by age, combined comorbidity score, and admission to the ICU by day 2. We created separate propensity scores using the same covariates in the primary analysis, but limited matches to within these subsets. For each group, we examined how the covariates in the HOCDI and control groups differed after matching with inference tests that took the paired nature of the data into account. All analyses were carried out using Stata/SE 11.1 (StataCorp, College Station, TX).
RESULTS
We identified 486,943 adult sepsis admissions to a Premier hospital between July 1, 2004 and December 31, 2010. After applying all exclusion criteria, we had a final sample of 218,915 admissions with sepsis (from 400 hospitals) at risk for HOCDI (Figure 1). Of these, 2368 (1.08%) met criteria for diagnosis of CDI after hospital day 2 and were matched to controls using index date and propensity score.
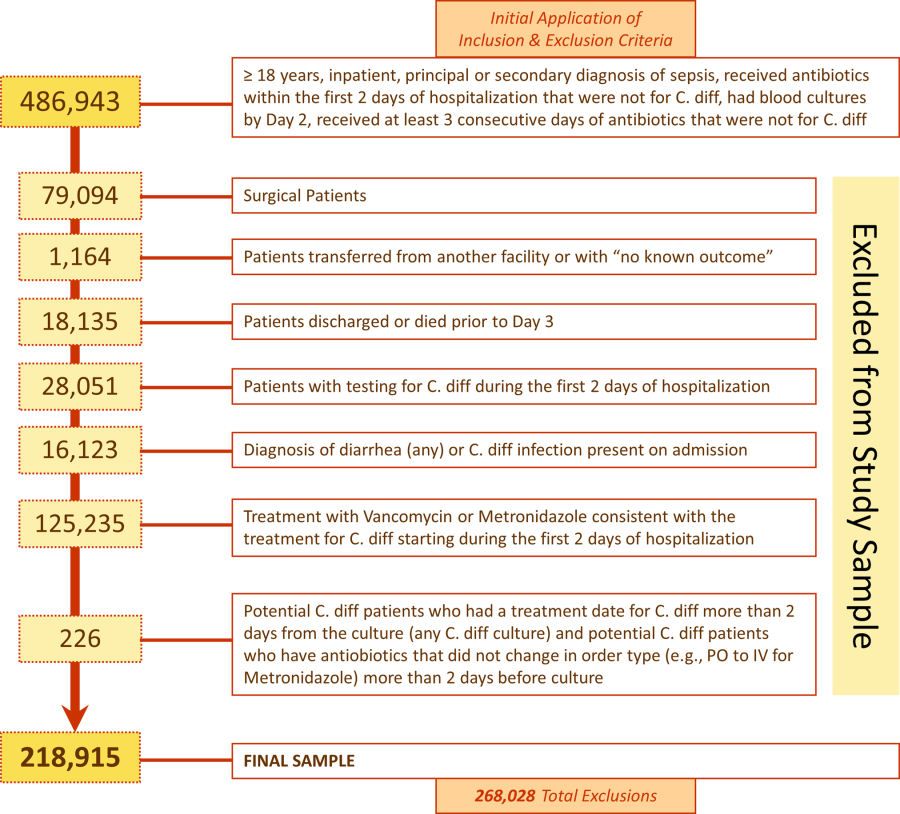
Patient and Hospital Factors
After matching, the median age was 71 years in cases and 70 years in controls (Table 1). Less than half (46%) of the population was male. Most cases (61%) and controls (58%) were white. Heart failure, hypertension, chronic lung disease, renal failure, and weight loss were the most common comorbid conditions. Our propensity model, which had a C statistic of 0.75, identified patients whose risk varied from a mean of 0.1% in the first decile to a mean of 3.8% in the tenth decile. Before matching, 40% of cases and 29% of controls were treated in the ICU by hospital day 2; after matching, 40% of both cases and controls were treated in the ICU by hospital day 2.
Distribution by LOS, Index Day, and Risk for Mortality
The unadjusted and unmatched LOS was longer for cases than controls (19 days vs 8 days, Table 2) (see Supporting Figure 1 in the online version of this article). Approximately 90% of the patients had an index day of 14 or less (Figure 2). Among patients both with and without CDI, the unadjusted mortality risk increased as the index day (and thus the total LOS) increased.
Outcome | HOCDI | No HOCDI | Difference (95% CI) | P |
---|---|---|---|---|
| ||||
Length of stay, d | ||||
Raw results | 19.2 | 8.3 | 8.4 (8.48.5) | <0.01 |
Raw results for survivors only | 18.6 | 8.0 | 10.6 (10.311.0) | <0.01 |
Matched results | 19.2 | 14.2 | 5.1(4.45.7) | <0.01 |
Matched results for survivors only | 18.6 | 13.6 | 5.1 (4.45.8) | <0.01 |
Mortality, % | ||||
Raw results | 24.0 | 10.1 | 13.9 (12.615.1), RR=2.4 (2.22.5) | <0.01 |
Matched results | 24.0 | 15.4 | 8.6 (6.410.9), RR=1.6 (1.41.8) | <0.01 |
Costs, US$ | ||||
Raw results median costs [interquartile range] | $26,187 [$15,117$46,273] | $9,988 [$6,296$17,351] | $16,190 ($15,826$16,555) | <0.01 |
Raw results for survivors only [interquartile range] | $24,038 [$14,169$41,654] | $9,429 [$6,070$15,875] | $14,620 ($14,246$14,996) | <0.01 |
Matched results [interquartile range] | $26,187 [$15,117$46,273] | $19,160 [$12,392$33,777] | $5,308 ($4,521$6,108) | |
Matched results for survivors only [interquartile range] | $24,038 [$14,169$41,654] | $17,811 [$11,614$29,298] | $4,916 ($4,088$5,768) | <0.01 |
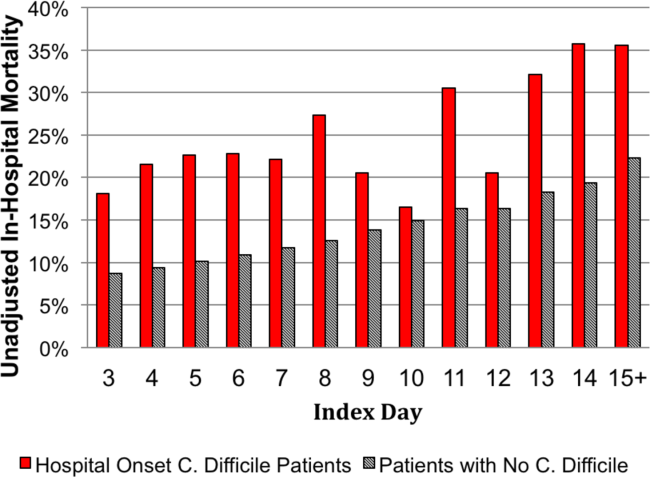
Adjusted Results
Compared to patients without disease, HOCDI patients had an increased unadjusted mortality (24% vs 10%, P<0.001). This translates into a relative risk of 2.4 (95% confidence interval [CI]: 2.2, 2.5). In the matched cohort, the difference in the mortality rates was attenuated, but still significantly higher in the HOCDI patients (24% versus 15%, P<0.001, an absolute difference of 9%; 95% CI: 6.410.8). The adjusted relative risk of mortality for HOCDI was 1.6 (95% CI: 1.41.8; Table 2). After matching, patients with CDI had a LOS of 19.2 days versus 14.2 days in matched controls (difference of 5.1 days; 95% CI: 4.45.7; P<0.001). When the LOS analysis was limited to survivors only, this difference of 5 days remained (P<0.001). In an analysis limited to survivors only, the difference in median costs between cases and controls was $4916 (95% CI: $4088$5768; P<0.001). In a secondary analysis examining heterogeneity between HOCDI and outcomes across clinical subgroups, the absolute difference in mortality and costs between cases and controls varied across demographics, comorbidity, and ICU admission, but the relative risks were similar (Figure 3) (see Supporting Figure 3 in the online version of this article).
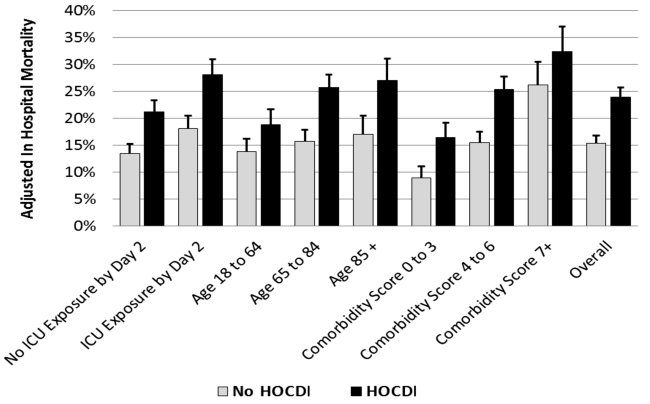
DISCUSSION
In this large cohort of patients with sepsis, we found that approximately 1 in 100 patients with sepsis developed HOCDI. Even after matching with controls based on the date of symptom onset and propensity score, patients who developed HOCDI were more than 1.6 times more likely to die in the hospital. HOCDI also added 5 days to the average hospitalization for patients with sepsis and increased median costs by approximately $5000. These findings suggest that a hospital that prevents 1 case of HOCDI per month in sepsis patients could avoid 1 death and 60 inpatient days annually, achieving an approximate yearly savings of $60,000.
Until now, the incremental cost and mortality attributable to HOCDI in sepsis patients have been poorly understood. Attributing outcomes can be methodologically challenging because patients who are at greatest risk for poor outcomes are the most likely to contract the disease and are at risk for longer periods of time. Therefore, it is necessary to take into account differences in severity of illness and time at risk between diseased and nondiseased populations and to ensure that outcomes attributed to the disease occur after disease onset.[28, 32] The majority of prior studies examining the impact of CDI on hospitalized patients have been limited by a lack of adequate matching to controls, small sample size, or failure to take into account time to infection.[16, 17, 19, 20]
A few studies have taken into account severity, time to infection, or both in estimating the impact of HOCDI. Using a time‐dependent Cox model that accounted for time to infection, Micek et al. found no difference in mortality but a longer LOS in mechanically ventilated patients (not limited to sepsis) with CDI.[33] However, their study was conducted at only 3 centers, did not take into account severity at the time of diagnosis, and did not clearly distinguish between community‐onset CDI and HOCDI. Oake et al. and Forster et al. examined the impact of CDI on patients hospitalized in a 2‐hospital health system in Canada.[12, 13] Using the baseline mortality estimate in a Cox multivariate proportional hazards regression model that accounted for the time‐varying nature of CDI, they found that HOCDI increased absolute risk of death by approximately 10%. Also, notably similar to our study were their findings that HOCDI occurred in approximately 1 in 100 patients and that the attributable median increase in LOS due to hospital‐onset CDI was 6 days. Although methodologically rigorous, these 2 small studies did not assess the impact of CDI on costs of care, were not focused on sepsis patients or even patients who received antibiotics, and also did not clearly distinguish between community‐onset CDI and HOCDI.
Our study therefore has important strengths. It is the first to examine the impact of HOCDI, including costs, on the outcomes of patients hospitalized with sepsis. The fact that we took into account both time to diagnosis and severity at the time of diagnosis (by using an index date for both cases and controls and determining severity on that date) prevented us from overestimating the impact of HOCDI on outcomes. The large differences in outcomes we observed in unadjusted and unmatched data were tempered after multivariate adjustment (eg, difference in LOS from 10.6 days to 5.1 additional days, costs from $14,620 to $4916 additional costs after adjustment). Our patient sample was derived from a large, multihospital database that contains actual hospital costs as derived from internal accounting systems. The fact that our study used data from hundreds of hospitals means that our estimates of cost, LOS, and mortality may be more generalizable than the work of Micek et al., Oake et al., and Forster et al.
This work also has important implications. First, hospital administrators, clinicians, and researchers can use our results to evaluate the cost‐effectiveness of HOCDI prevention measures (eg, hand hygiene programs, antibiotic stewardship). By quantifying the cost per case in sepsis patients, we allow administrators and researchers to compare the incremental costs of HOCDI prevention programs to the dollars and lives saved due to prevention efforts. Second, we found that our propensity model identified patients whose risk varied greatly. This suggests that an opportunity exists to identify subgroups of patients that are at highest risk. Identifying high‐risk subgroups will allow for targeted risk reduction interventions and the opportunity to reduce transmission (eg, by placing high‐risk patients in a private room). Finally, we have reaffirmed that time to diagnosis and presenting severity need to be rigorously addressed prior to making estimates of the impact of CDI burden and other hospital‐acquired conditions and injuries.
There are limitations to this study as well. We did not have access to microbiological data. However, we required a diagnosis code of CDI, evidence of testing, and treatment after the date of testing to confirm a diagnosis. We also adopted detailed exclusion criteria to ensure that CDI that was not present on admission and that controls did not have CDI. These stringent inclusion and exclusion criteria strengthened the internal validity of our estimates of disease impact. We used administrative claims data, which limited our ability to adjust for severity. However, the detailed nature of the database allowed us to use treatments, such as vasopressors and antibiotics, to identify cases; treatments were also used as a validated indicator of severity,[26] which may have helped to reduce some of this potential bias. Although our propensity model included many predictors of CDI, such as use of proton pump inhibitors and factors associated with mortality, not every confounder was completely balanced after propensity matching, although the statistical differences may have been related to our large sample size and therefore might not be clinically significant. We also may have failed to include all possible predictors of CDI in the propensity model.
In a large, diverse cohort of hospitalized patients with sepsis, we found that HOCDI lengthened hospital stay by approximately 5 days, increased risk of in‐hospital mortality by 9%, and increased hospital cost by approximately $5000 per patient. These findings highlight the importance of identifying effective prevention measures and of determining the patient populations at greatest risk for HOCDI.
Disclosures: The study was conducted with funding from the Division of Critical Care and the Center for Quality of Care Research at Baystate Medical Center. Dr. Lagu is supported by the National Heart, Lung, and Blood Institute of the National Institutes of Health under award number K01HL114745. Dr. Stefan is supported by the National Heart, Lung, and Blood Institute of the National Institutes of Health under award number K01HL114631. Drs. Lagu and Lindenauer had full access to all of the data in the study; they take responsibility for the integrity of the data and the accuracy of the data analysis. Drs. Lagu, Lindenauer, Steingrub, Higgins, Stefan, Haessler, and Rothberg conceived of the study. Dr. Lindenauer acquired the data. Drs. Lagu, Lindenauer, Rothberg, Steingrub, Nathanson, Stefan, Haessler, Higgins, and Mr. Hannon analyzed and interpreted the data. Dr. Lagu drafted the manuscript. Drs. Lagu, Lindenauer, Rothberg, Steingrub, Nathanson, Stefan, Haessler, Higgins, and Mr. Hannon critically reviewed the manuscript for important intellectual content. Dr. Nathanson carried out the statistical analyses. Dr. Nathanson, through his company OptiStatim LLC, was paid by the investigators with funding from the Department of Medicine at Baystate Medical Center to assist in conducting the statistical analyses in this study. The authors report no further conflicts of interest.
There are approximately 3 million cases of Clostridium difficile infection (CDI) per year in the United States.[1, 2, 3, 4] Of these, 10% result in a hospitalization or occur as a consequence of the exposures and treatments associated with hospitalization.[1, 2, 3, 4] Some patients with CDI experience mild diarrhea that is responsive to therapy, but other patients experience severe, life‐threatening disease that is refractory to treatment, leading to pseudomembranous colitis, toxic megacolon, and sepsis with a 60‐day mortality rate that exceeds 12%.[5, 6, 7, 8, 9, 10, 11, 12, 13, 14]
Hospital‐onset CDI (HOCDI), defined as C difficile‐associated diarrhea and related symptoms with onset more than 48 hours after admission to a healthcare facility,[15] represents a unique marriage of CDI risk factors.[5] A vulnerable patient is introduced into an environment that contains both exposure to C difficile (through other patients or healthcare workers) and treatment with antibacterial agents that may diminish normal flora. Consequently, CDI is common among hospitalized patients.[16, 17, 18] A particularly important group for understanding the burden of disease is patients who initially present to the hospital with sepsis and subsequently develop HOCDI. Sepsis patients are often critically ill and are universally treated with antibiotics.
Determining the incremental cost and mortality risk attributable to HOCDI is methodologically challenging. Because HOCDI is associated with presenting severity, the sickest patients are also the most likely to contract the disease. HOCDI is also associated with time of exposure or length of stay (LOS). Because LOS is a risk factor, comparing LOS between those with and without HOCDI will overestimate the impact if the time to diagnosis is not taken into account.[16, 17, 19, 20] We aimed to examine the impact of HOCDI in hospitalized patients with sepsis using a large, multihospital database with statistical methods that took presenting severity and time to diagnosis into account.
METHODS
Data Source and Subjects
Permission to conduct this study was obtained from the institutional review board at Baystate Medical Center. We used the Premier Healthcare Informatics database, a voluntary, fee‐supported database created to measure quality and healthcare utilization, which has been used extensively in health services research.[21, 22, 23] In addition to the elements found in hospital claims derived from the uniform billing 04 form, Premier data include an itemized, date‐stamped log of all items and services charged to the patient or their insurer, including medications, laboratory tests, and diagnostic and therapeutic services. Approximately 75% of hospitals that submit data also provide information on actual hospital costs, taken from internal cost accounting systems. The rest provide cost estimates based on Medicare cost‐to‐charge ratios. Participating hospitals are similar to the composition of acute care hospitals nationwide, although they are more commonly small‐ to midsized nonteaching facilities and are more likely to be located in the southern United States.
We included medical (nonsurgical) adult patients with sepsis who were admitted to a participating hospital between July 1, 2004 and December 31, 2010. Because we sought to focus on the care of patients who present to the hospital with sepsis, we defined sepsis as the presence of a diagnosis of sepsis plus evidence of both blood cultures and antibiotic treatment within the first 2 days of hospitalization; we used the first 2 days of hospitalization rather than just the first day because, in administrative datasets, the duration of the first hospital day includes partial days that can vary in length. We excluded patients who died or were discharged prior to day 3, because HOCDI is defined as onset after 48 hours in a healthcare facility.[15] We also excluded surviving patients who received less than 3 consecutive days of antibiotics, and patients who were transferred from or to another acute‐care facility; the latter exclusion criterion was used because we could not accurately determine the onset or subsequent course of their illness.
Identification of Patients at Risk for and Diagnosed With HOCDI
Among eligible patients with sepsis, we aimed to identify a cohort at risk for developing CDI during the hospital stay. We excluded patients: (1) with a diagnosis indicating that diarrhea was present on admission, (2) with a diagnosis of CDI that was indicated to be present on admission, (3) who were tested for CDI on the first or second hospital day, and (4) who received an antibiotic that could be consistent with treatment for CDI (oral or intravenous [IV] metronidazole or oral vancomycin) on hospital days 1 or 2.
Next, we aimed to identify sepsis patients at risk for HOCDI who developed HOCDI during their hospital stay. Among eligible patients described above, we considered a patient to have HOCDI if they had an International Classification of Diseases, Ninth Revision, Clinical Modification diagnosis of CDI (primary or secondary but not present on admission), plus evidence of testing for CDI after hospital day 2, and treatment with oral vancomycin or oral or IV metronidazole that was started after hospital day 2 and within 2 days of the C difficile test, and evidence of treatment for CDI for at least 3 days unless the patient was discharged or died.
Patient Information
We recorded patient age, gender, marital status, insurance status, race, and ethnicity. Using software provided by the Healthcare Costs and Utilization Project of the Agency for Healthcare Research and Quality, we categorized information on 30 comorbid conditions. We also created a single numerical comorbidity score based on a previously published and validated combined comorbidity score that predicts 1‐year mortality.[24] Based on a previously described algorithm,[25] we used diagnosis codes to assess the source (lung, abdomen, urinary tract, blood, other) and type of sepsis (Gram positive, Gram negative, mixed, anaerobic, fungal). Because patients can have more than 1 potential source of sepsis (eg, pneumonia and urinary tract infection) and more than 1 organism causing infection (eg, urine with Gram negative rods and blood culture with Gram positive cocci), these categories are not mutually exclusive (see Supporting Table 1 in the online version of this article). We used billing codes to identify the use of therapies, monitoring devices, and pharmacologic treatments to characterize both initial severity of illness and severity at the time of CDI diagnosis. These therapies are included in a validated sepsis mortality prediction model (designed for administrative datasets) with similar discrimination and calibration to clinical intensive care unit (ICU) risk‐adjustment models such as the mortality probability model, version III.[26, 27]
Outcomes
Our primary outcome of interest was in‐hospital mortality. Secondary outcomes included LOS and costs for survivors only and for all patients.
Statistical Methods
We calculated patient‐level summary statistics for all patients using frequencies for binary variables and medians and interquartile percentiles for continuous variables. P values <0.05 were considered statistically significant.
To account for presenting severity and time to diagnosis, we used methods that have been described elsewhere.[12, 13, 18, 20, 28] First, we identified patients who were eligible to develop HOCDI. Second, for all eligible patients, we identified a date of disease onset (index date). For patients who met criteria for HOCDI, this was the date on which the patient was tested for CDI. For eligible patients without disease, this was a date randomly assigned to any time during the hospital stay.[29] Next, we developed a nonparsimonious propensity score model that included all patient characteristics (demographics, comorbidities, sepsis source, and severity of illness on presentation and on the index date; all variables listed in Table 1 were included in the propensity model). Some of the variables for this model (eg, mechanical ventilation and vasopressors) were derived from a validated severity model.[26] We adjusted for correlation within hospital when creating the propensity score using Huber‐White robust standard error estimators clustered at the hospital level.[30] We then created matched pairs with the same LOS prior to the index date and similar propensity for developing CDI. We first matched on index date, and then, within each index‐datematched subset, matched patients with and without HOCDI by their propensity score using a 5‐to‐1 greedy match algorithm.[31] We used the differences in LOS between the cases and controls after the index date to calculate the additional attributable LOS estimates; we also separately estimated the impact on cost and LOS in a group limited to those who survived after discharge because of concerns that death could shorten LOS and reduce costs.
Before Matching | After Matching | |||||
---|---|---|---|---|---|---|
HOCDI, n=2,368, % | No CDI, n=216,547, % | P | HOCDI, n=2,368, % | No CDI, n=2,368, % | P | |
| ||||||
Age, y | 70.9 (15.1) | 68.6 (16.8) | <0.01 | 70.9 (15.1) | 69.8 (15.9) | 0.02 |
Male | 46.8 | 46.0 | 0.44 | 46.8 | 47.2 | 0.79 |
Race | ||||||
White | 61.0 | 63.3 | 61.0 | 58.1 | ||
Black | 15.6 | 14.5 | <0.01 | 15.6 | 17.0 | 0.11 |
Hispanic | 3.2 | 5.4 | 3.2 | 4.1 | ||
Other race | 20.2 | 16.8 | 20.2 | 20.9 | ||
Marital status | ||||||
Married | 31.6 | 36.3 | <0.01 | 31.6 | 32.6 | 0.74 |
Single/divorced | 52.8 | 51.1 | 52.8 | 52.0 | ||
Other/unknown | 15.7 | 12.6 | 15.7 | 14.5 | ||
Insurance status | ||||||
Medicare traditional | 63.2 | 59.5 | 63.2 | 60.3 | ||
Medicare managed | 10.6 | 10.1 | 10.6 | 10.9 | ||
Medicaid traditional | 7.6 | 6.9 | 7.6 | 8.2 | ||
Medicaid managed | 1.8 | 2.0 | <0.01 | 1.8 | 1.8 | 0.50 |
Managed care | 10.8 | 12.3 | 10.8 | 12.0 | ||
Commercial | 2.0 | 3.5 | 2.0 | 2.2 | ||
Self‐pay/other/unknown | 4.0 | 5.7 | 4.0 | 4.7 | ||
Infection source | ||||||
Respiratory | 46.5 | 37.0 | <0.01 | 46.5 | 49.6 | 0.03 |
Skin/bone | 10.1 | 8.6 | 0.01 | 10.1 | 11.2 | 0.21 |
Urinary | 52.2 | 51.3 | 0.38 | 52.2 | 50.3 | 0.18 |
Blood | 11.1 | 15.1 | <0.01 | 11.1 | 11.5 | 0.65 |
Infecting organism | ||||||
Gram negative | 35.0 | 36.6 | <0.01 | 35.0 | 33.1 | 0.18 |
Anaerobe | 1.4 | 0.7 | <0.01 | 1.4 | 1.1 | 0.24 |
Fungal | 17.5 | 7.5 | <0.01 | 17.5 | 18.3 | 0.44 |
Most common comorbid conditions | ||||||
Congestive heart failure | 35.1 | 24.6 | <0.01 | 35.1 | 37.5 | 0.06 |
Chronic lung disease | 31.6 | 27.6 | <0.01 | 31.6 | 32.1 | 0.71 |
Hypertension | 31.5 | 37.7 | <0.01 | 31.5 | 29.7 | 0.16 |
Renal Failure | 29.7 | 23.8 | <0.01 | 29.7 | 31.2 | 0.28 |
Weight Loss | 27.7 | 13.3 | <0.01 | 27.7 | 29.4 | 0.17 |
Treatments by day 2 | ||||||
ICU admission | 40.0 | 29.5 | <0.01 | 40.0 | 40.7 | 0.64 |
Use of bicarbonate | 12.2 | 7.1 | <0.01 | 12.2 | 13.6 | 0.15 |
Fresh frozen plasma | 1.4 | 1.0 | 0.03 | 1.4 | 1.1 | 0.36 |
Inotropes | 1.4 | 0.9 | 0.01 | 1.4 | 2.2 | 0.04 |
Hydrocortisone | 6.7 | 4.7 | <0.01 | 6.7 | 7.4 | 0.33 |
Thiamine | 4.2 | 3.3 | 0.01 | 4.2 | 4.1 | 0.83 |
Psychotropics (eg, haldol for delirium) | 10.0 | 9.2 | 0.21 | 10.0 | 10.8 | 0.36 |
Restraints (eg, for delirium) | 2.0 | 1.5 | 0.05 | 2.0 | 2.5 | 0.29 |
Angiotensin‐converting enzyme inhibitors | 12.1 | 13.2 | 0.12 | 12.1 | 10.9 | 0.20 |
Statins | 18.8 | 21.1 | 0.01 | 18.8 | 16.9 | 0.09 |
Drotrecogin alfa | 0.6 | 0.3 | 0.00 | 0.6 | 0.6 | 0.85 |
Foley catheter | 19.2 | 19.8 | 0.50 | 19.2 | 22.0 | 0.02 |
Diuretics | 28.5 | 25.4 | 0.01 | 28.5 | 29.6 | 0.42 |
Red blood cells | 15.5 | 10.6 | <0.01 | 15.5 | 15.8 | 0.81 |
Calcium channel blockers | 19.3 | 16.8 | 0.01 | 19.3 | 19.1 | 0.82 |
‐Blockers | 32.7 | 29.6 | 0.01 | 32.7 | 30.6 | 0.12 |
Proton pump inhibitors | 59.6 | 53.1 | <0.01 | 59.6 | 61.0 | 0.31 |
Analysis Across Clinical Subgroups
In a secondary analysis, we examined heterogeneity in the association between HOCDI and outcomes within subsets of patients defined by age, combined comorbidity score, and admission to the ICU by day 2. We created separate propensity scores using the same covariates in the primary analysis, but limited matches to within these subsets. For each group, we examined how the covariates in the HOCDI and control groups differed after matching with inference tests that took the paired nature of the data into account. All analyses were carried out using Stata/SE 11.1 (StataCorp, College Station, TX).
RESULTS
We identified 486,943 adult sepsis admissions to a Premier hospital between July 1, 2004 and December 31, 2010. After applying all exclusion criteria, we had a final sample of 218,915 admissions with sepsis (from 400 hospitals) at risk for HOCDI (Figure 1). Of these, 2368 (1.08%) met criteria for diagnosis of CDI after hospital day 2 and were matched to controls using index date and propensity score.
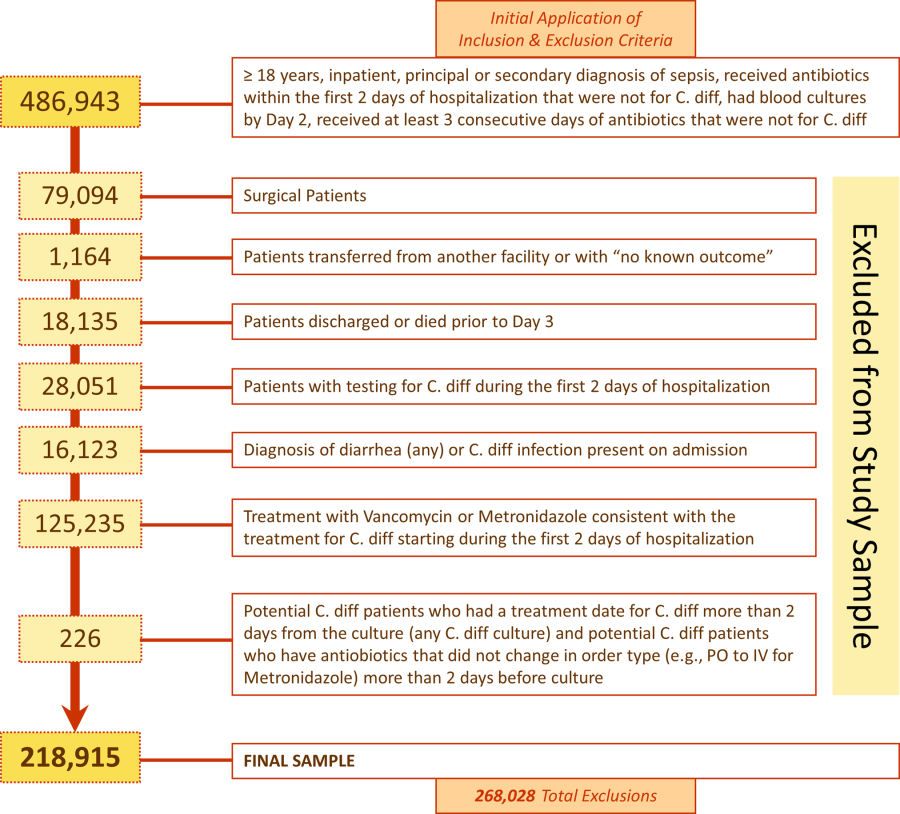
Patient and Hospital Factors
After matching, the median age was 71 years in cases and 70 years in controls (Table 1). Less than half (46%) of the population was male. Most cases (61%) and controls (58%) were white. Heart failure, hypertension, chronic lung disease, renal failure, and weight loss were the most common comorbid conditions. Our propensity model, which had a C statistic of 0.75, identified patients whose risk varied from a mean of 0.1% in the first decile to a mean of 3.8% in the tenth decile. Before matching, 40% of cases and 29% of controls were treated in the ICU by hospital day 2; after matching, 40% of both cases and controls were treated in the ICU by hospital day 2.
Distribution by LOS, Index Day, and Risk for Mortality
The unadjusted and unmatched LOS was longer for cases than controls (19 days vs 8 days, Table 2) (see Supporting Figure 1 in the online version of this article). Approximately 90% of the patients had an index day of 14 or less (Figure 2). Among patients both with and without CDI, the unadjusted mortality risk increased as the index day (and thus the total LOS) increased.
Outcome | HOCDI | No HOCDI | Difference (95% CI) | P |
---|---|---|---|---|
| ||||
Length of stay, d | ||||
Raw results | 19.2 | 8.3 | 8.4 (8.48.5) | <0.01 |
Raw results for survivors only | 18.6 | 8.0 | 10.6 (10.311.0) | <0.01 |
Matched results | 19.2 | 14.2 | 5.1(4.45.7) | <0.01 |
Matched results for survivors only | 18.6 | 13.6 | 5.1 (4.45.8) | <0.01 |
Mortality, % | ||||
Raw results | 24.0 | 10.1 | 13.9 (12.615.1), RR=2.4 (2.22.5) | <0.01 |
Matched results | 24.0 | 15.4 | 8.6 (6.410.9), RR=1.6 (1.41.8) | <0.01 |
Costs, US$ | ||||
Raw results median costs [interquartile range] | $26,187 [$15,117$46,273] | $9,988 [$6,296$17,351] | $16,190 ($15,826$16,555) | <0.01 |
Raw results for survivors only [interquartile range] | $24,038 [$14,169$41,654] | $9,429 [$6,070$15,875] | $14,620 ($14,246$14,996) | <0.01 |
Matched results [interquartile range] | $26,187 [$15,117$46,273] | $19,160 [$12,392$33,777] | $5,308 ($4,521$6,108) | |
Matched results for survivors only [interquartile range] | $24,038 [$14,169$41,654] | $17,811 [$11,614$29,298] | $4,916 ($4,088$5,768) | <0.01 |
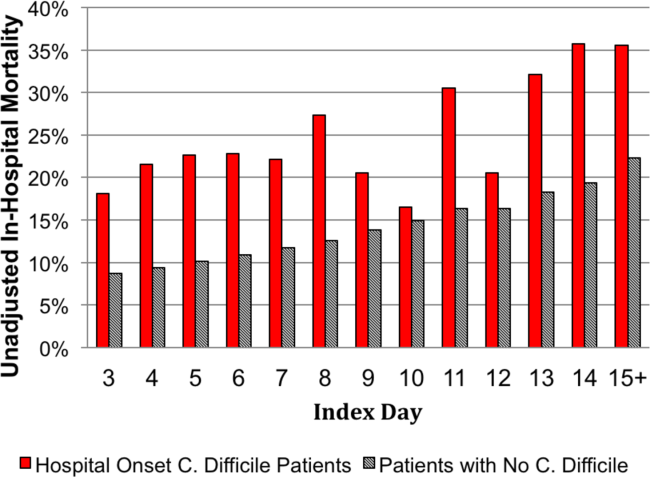
Adjusted Results
Compared to patients without disease, HOCDI patients had an increased unadjusted mortality (24% vs 10%, P<0.001). This translates into a relative risk of 2.4 (95% confidence interval [CI]: 2.2, 2.5). In the matched cohort, the difference in the mortality rates was attenuated, but still significantly higher in the HOCDI patients (24% versus 15%, P<0.001, an absolute difference of 9%; 95% CI: 6.410.8). The adjusted relative risk of mortality for HOCDI was 1.6 (95% CI: 1.41.8; Table 2). After matching, patients with CDI had a LOS of 19.2 days versus 14.2 days in matched controls (difference of 5.1 days; 95% CI: 4.45.7; P<0.001). When the LOS analysis was limited to survivors only, this difference of 5 days remained (P<0.001). In an analysis limited to survivors only, the difference in median costs between cases and controls was $4916 (95% CI: $4088$5768; P<0.001). In a secondary analysis examining heterogeneity between HOCDI and outcomes across clinical subgroups, the absolute difference in mortality and costs between cases and controls varied across demographics, comorbidity, and ICU admission, but the relative risks were similar (Figure 3) (see Supporting Figure 3 in the online version of this article).
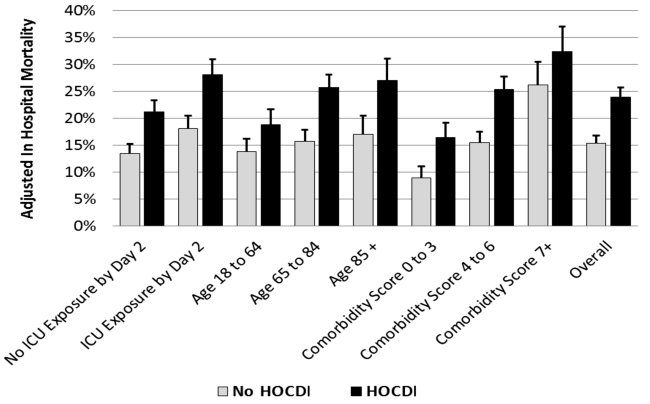
DISCUSSION
In this large cohort of patients with sepsis, we found that approximately 1 in 100 patients with sepsis developed HOCDI. Even after matching with controls based on the date of symptom onset and propensity score, patients who developed HOCDI were more than 1.6 times more likely to die in the hospital. HOCDI also added 5 days to the average hospitalization for patients with sepsis and increased median costs by approximately $5000. These findings suggest that a hospital that prevents 1 case of HOCDI per month in sepsis patients could avoid 1 death and 60 inpatient days annually, achieving an approximate yearly savings of $60,000.
Until now, the incremental cost and mortality attributable to HOCDI in sepsis patients have been poorly understood. Attributing outcomes can be methodologically challenging because patients who are at greatest risk for poor outcomes are the most likely to contract the disease and are at risk for longer periods of time. Therefore, it is necessary to take into account differences in severity of illness and time at risk between diseased and nondiseased populations and to ensure that outcomes attributed to the disease occur after disease onset.[28, 32] The majority of prior studies examining the impact of CDI on hospitalized patients have been limited by a lack of adequate matching to controls, small sample size, or failure to take into account time to infection.[16, 17, 19, 20]
A few studies have taken into account severity, time to infection, or both in estimating the impact of HOCDI. Using a time‐dependent Cox model that accounted for time to infection, Micek et al. found no difference in mortality but a longer LOS in mechanically ventilated patients (not limited to sepsis) with CDI.[33] However, their study was conducted at only 3 centers, did not take into account severity at the time of diagnosis, and did not clearly distinguish between community‐onset CDI and HOCDI. Oake et al. and Forster et al. examined the impact of CDI on patients hospitalized in a 2‐hospital health system in Canada.[12, 13] Using the baseline mortality estimate in a Cox multivariate proportional hazards regression model that accounted for the time‐varying nature of CDI, they found that HOCDI increased absolute risk of death by approximately 10%. Also, notably similar to our study were their findings that HOCDI occurred in approximately 1 in 100 patients and that the attributable median increase in LOS due to hospital‐onset CDI was 6 days. Although methodologically rigorous, these 2 small studies did not assess the impact of CDI on costs of care, were not focused on sepsis patients or even patients who received antibiotics, and also did not clearly distinguish between community‐onset CDI and HOCDI.
Our study therefore has important strengths. It is the first to examine the impact of HOCDI, including costs, on the outcomes of patients hospitalized with sepsis. The fact that we took into account both time to diagnosis and severity at the time of diagnosis (by using an index date for both cases and controls and determining severity on that date) prevented us from overestimating the impact of HOCDI on outcomes. The large differences in outcomes we observed in unadjusted and unmatched data were tempered after multivariate adjustment (eg, difference in LOS from 10.6 days to 5.1 additional days, costs from $14,620 to $4916 additional costs after adjustment). Our patient sample was derived from a large, multihospital database that contains actual hospital costs as derived from internal accounting systems. The fact that our study used data from hundreds of hospitals means that our estimates of cost, LOS, and mortality may be more generalizable than the work of Micek et al., Oake et al., and Forster et al.
This work also has important implications. First, hospital administrators, clinicians, and researchers can use our results to evaluate the cost‐effectiveness of HOCDI prevention measures (eg, hand hygiene programs, antibiotic stewardship). By quantifying the cost per case in sepsis patients, we allow administrators and researchers to compare the incremental costs of HOCDI prevention programs to the dollars and lives saved due to prevention efforts. Second, we found that our propensity model identified patients whose risk varied greatly. This suggests that an opportunity exists to identify subgroups of patients that are at highest risk. Identifying high‐risk subgroups will allow for targeted risk reduction interventions and the opportunity to reduce transmission (eg, by placing high‐risk patients in a private room). Finally, we have reaffirmed that time to diagnosis and presenting severity need to be rigorously addressed prior to making estimates of the impact of CDI burden and other hospital‐acquired conditions and injuries.
There are limitations to this study as well. We did not have access to microbiological data. However, we required a diagnosis code of CDI, evidence of testing, and treatment after the date of testing to confirm a diagnosis. We also adopted detailed exclusion criteria to ensure that CDI that was not present on admission and that controls did not have CDI. These stringent inclusion and exclusion criteria strengthened the internal validity of our estimates of disease impact. We used administrative claims data, which limited our ability to adjust for severity. However, the detailed nature of the database allowed us to use treatments, such as vasopressors and antibiotics, to identify cases; treatments were also used as a validated indicator of severity,[26] which may have helped to reduce some of this potential bias. Although our propensity model included many predictors of CDI, such as use of proton pump inhibitors and factors associated with mortality, not every confounder was completely balanced after propensity matching, although the statistical differences may have been related to our large sample size and therefore might not be clinically significant. We also may have failed to include all possible predictors of CDI in the propensity model.
In a large, diverse cohort of hospitalized patients with sepsis, we found that HOCDI lengthened hospital stay by approximately 5 days, increased risk of in‐hospital mortality by 9%, and increased hospital cost by approximately $5000 per patient. These findings highlight the importance of identifying effective prevention measures and of determining the patient populations at greatest risk for HOCDI.
Disclosures: The study was conducted with funding from the Division of Critical Care and the Center for Quality of Care Research at Baystate Medical Center. Dr. Lagu is supported by the National Heart, Lung, and Blood Institute of the National Institutes of Health under award number K01HL114745. Dr. Stefan is supported by the National Heart, Lung, and Blood Institute of the National Institutes of Health under award number K01HL114631. Drs. Lagu and Lindenauer had full access to all of the data in the study; they take responsibility for the integrity of the data and the accuracy of the data analysis. Drs. Lagu, Lindenauer, Steingrub, Higgins, Stefan, Haessler, and Rothberg conceived of the study. Dr. Lindenauer acquired the data. Drs. Lagu, Lindenauer, Rothberg, Steingrub, Nathanson, Stefan, Haessler, Higgins, and Mr. Hannon analyzed and interpreted the data. Dr. Lagu drafted the manuscript. Drs. Lagu, Lindenauer, Rothberg, Steingrub, Nathanson, Stefan, Haessler, Higgins, and Mr. Hannon critically reviewed the manuscript for important intellectual content. Dr. Nathanson carried out the statistical analyses. Dr. Nathanson, through his company OptiStatim LLC, was paid by the investigators with funding from the Department of Medicine at Baystate Medical Center to assist in conducting the statistical analyses in this study. The authors report no further conflicts of interest.
- Increasing prevalence and severity of Clostridium difficile colitis in hospitalized patients in the United States. Arch Surg. 2007;142(7):624–631; discussion 631. , , , .
- The changing epidemiology of Clostridium difficile infections. Clin Microbiol Rev. 2010;23(3):529–549. , , , et al.
- Clostridium Difficile‐Associated Disease in U.S. Hospitals, 1993–2005. HCUP Statistical Brief #50. April 2008. Agency for Healthcare Research and Quality, Rockville, MD. Available at: http://www.hcup‐us.ahrq.gov/reports/statbriefs/sb50.pdf. Accessed April 4, 2014. , .
- National point prevalence of Clostridium difficile in US health care facility inpatients, 2008. Am J Infect Control. 2009;37(4):263–270. , , , .
- A 76‐year‐old man with recurrent Clostridium difficile‐associated diarrhea: review of C. difficile infection. JAMA. 2009;301(9):954–962. .
- Recurrent Clostridium difficile disease: epidemiology and clinical characteristics. Infect Control Hosp Epidemiol. 1999;20(1):43–50. , , , , , .
- Recurrent Clostridium difficile diarrhea: characteristics of and risk factors for patients enrolled in a prospective, randomized, double‐blinded trial. Clin Infect Dis. 1997;24(3):324–333. , , , , , .
- Narrative review: the new epidemic of Clostridium difficile‐associated enteric disease. Ann Intern Med. 2006;145(10):758–764. .
- Impact of emergency colectomy on survival of patients with fulminant Clostridium difficile colitis during an epidemic caused by a hypervirulent strain. Ann Surg. 2007;245(2):267–272. , , , et al.
- Hospital‐acquired Clostridium difficile‐associated disease in the intensive care unit setting: epidemiology, clinical course and outcome. BMC Infect Dis. 2007;7:42. , , , .
- Factors associated with prolonged symptoms and severe disease due to Clostridium difficile. Age Ageing. 1999;28(2):107–113. , , , , , .
- The effect of hospital‐acquired Clostridium difficile infection on in‐hospital mortality. Arch Intern Med. 2010;170(20):1804–1810. , , , , , .
- The effect of hospital‐acquired infection with Clostridium difficile on length of stay in hospital. CMAJ. 2012;184(1):37–42. , , , , , .
- Clostridium difficile—more difficult than ever. N Engl J Med. 2008;359(18):1932–1940. , .
- Clinical practice guidelines for Clostridium difficile infection in adults: 2010 update by the society for healthcare epidemiology of America (SHEA) and the infectious diseases society of America (IDSA). Infect Control Hosp Epidemiol. 2010;31(5):431–455. , , , et al.
- Health care costs and mortality associated with nosocomial diarrhea due to Clostridium difficile. Clin Infect Dis. 2002;34(3):346–353. , , , .
- Short‐ and long‐term attributable costs of Clostridium difficile‐associated disease in nonsurgical inpatients. Clin Infect Dis. 2008;46(4):497–504. , , , , .
- Estimation of extra hospital stay attributable to nosocomial infections: heterogeneity and timing of events. J Clin Epidemiol. 2000;53(4):409–417. , , , , .
- Attributable outcomes of endemic Clostridium difficile‐associated disease in nonsurgical patients. Emerging Infect Dis. 2008;14(7):1031–1038. , , , et al.
- Excess length of stay, charges, and mortality attributable to medical injuries during hospitalization. JAMA. 2003;290(14):1868–1874. , .
- Association of corticosteroid dose and route of administration with risk of treatment failure in acute exacerbation of chronic obstructive pulmonary disease. JAMA. 2010;303(23):2359–2367. , , , , , .
- The relationship between hospital spending and mortality in patients with sepsis. Arch Intern Med. 2011;171(4):292–299. , , , , , .
- Comparative effectiveness of macrolides and quinolones for patients hospitalized with acute exacerbations of chronic obstructive pulmonary disease (AECOPD). J Hosp Med. 2010;5(5):261–267. , , , , , .
- A combined comorbidity score predicted mortality in elderly patients better than existing scores. J Clin Epidemiol. 2011;64(7):749–759. , , , , .
- Epidemiology of severe sepsis in the United States: analysis of incidence, outcome, and associated costs of care. Crit Care Med. 2001;29(7):1303–1310. , , , , , .
- Development and validation of a model that uses enhanced administrative data to predict mortality in patients with sepsis. Crit Care Med. 2011;39(11):2425–2430. , , , et al.
- Incorporating initial treatments improves performance of a mortality prediction model for patients with sepsis. Pharmacoepidemiol Drug Saf. 2012;21(suppl 2):44–52. , , , , .
- Nosocomial infection, length of stay, and time‐dependent bias. Infect Control Hosp Epidemiol. 2009;30(3):273–276. , , , .
- Length of stay and hospital costs among high‐risk patients with hospital‐origin Clostridium difficile‐associated diarrhea. J Med Econ. 2013;16(3):440–448. , , , , , .
- Rogers. Regression standard errors in clustered samples. Stata Technical Bulletin. 1993;13(13):19–23.
- Reducing bias in a propensity score matched‐pair sample using greedy matching techniques. In: Proceedings of the 26th Annual SAS Users Group International Conference; April 22–25, 2001; Long Beach, CA. Paper 214‐26. Available at: http://www2.sas.com/proceedings/sugi26/p214‐26.pdf. Accessed April 4, 2014. .
- Prolongation of length of stay and Clostridium difficile infection: a review of the methods used to examine length of stay due to healthcare associated infections. Antimicrob Resist Infect Control. 2012;1(1):14. , .
- Clostridium difficile Infection: a multicenter study of epidemiology and outcomes in mechanically ventilated patients. Crit Care Med. 2013;41(8):1968–1975. , , , et al.
- Increasing prevalence and severity of Clostridium difficile colitis in hospitalized patients in the United States. Arch Surg. 2007;142(7):624–631; discussion 631. , , , .
- The changing epidemiology of Clostridium difficile infections. Clin Microbiol Rev. 2010;23(3):529–549. , , , et al.
- Clostridium Difficile‐Associated Disease in U.S. Hospitals, 1993–2005. HCUP Statistical Brief #50. April 2008. Agency for Healthcare Research and Quality, Rockville, MD. Available at: http://www.hcup‐us.ahrq.gov/reports/statbriefs/sb50.pdf. Accessed April 4, 2014. , .
- National point prevalence of Clostridium difficile in US health care facility inpatients, 2008. Am J Infect Control. 2009;37(4):263–270. , , , .
- A 76‐year‐old man with recurrent Clostridium difficile‐associated diarrhea: review of C. difficile infection. JAMA. 2009;301(9):954–962. .
- Recurrent Clostridium difficile disease: epidemiology and clinical characteristics. Infect Control Hosp Epidemiol. 1999;20(1):43–50. , , , , , .
- Recurrent Clostridium difficile diarrhea: characteristics of and risk factors for patients enrolled in a prospective, randomized, double‐blinded trial. Clin Infect Dis. 1997;24(3):324–333. , , , , , .
- Narrative review: the new epidemic of Clostridium difficile‐associated enteric disease. Ann Intern Med. 2006;145(10):758–764. .
- Impact of emergency colectomy on survival of patients with fulminant Clostridium difficile colitis during an epidemic caused by a hypervirulent strain. Ann Surg. 2007;245(2):267–272. , , , et al.
- Hospital‐acquired Clostridium difficile‐associated disease in the intensive care unit setting: epidemiology, clinical course and outcome. BMC Infect Dis. 2007;7:42. , , , .
- Factors associated with prolonged symptoms and severe disease due to Clostridium difficile. Age Ageing. 1999;28(2):107–113. , , , , , .
- The effect of hospital‐acquired Clostridium difficile infection on in‐hospital mortality. Arch Intern Med. 2010;170(20):1804–1810. , , , , , .
- The effect of hospital‐acquired infection with Clostridium difficile on length of stay in hospital. CMAJ. 2012;184(1):37–42. , , , , , .
- Clostridium difficile—more difficult than ever. N Engl J Med. 2008;359(18):1932–1940. , .
- Clinical practice guidelines for Clostridium difficile infection in adults: 2010 update by the society for healthcare epidemiology of America (SHEA) and the infectious diseases society of America (IDSA). Infect Control Hosp Epidemiol. 2010;31(5):431–455. , , , et al.
- Health care costs and mortality associated with nosocomial diarrhea due to Clostridium difficile. Clin Infect Dis. 2002;34(3):346–353. , , , .
- Short‐ and long‐term attributable costs of Clostridium difficile‐associated disease in nonsurgical inpatients. Clin Infect Dis. 2008;46(4):497–504. , , , , .
- Estimation of extra hospital stay attributable to nosocomial infections: heterogeneity and timing of events. J Clin Epidemiol. 2000;53(4):409–417. , , , , .
- Attributable outcomes of endemic Clostridium difficile‐associated disease in nonsurgical patients. Emerging Infect Dis. 2008;14(7):1031–1038. , , , et al.
- Excess length of stay, charges, and mortality attributable to medical injuries during hospitalization. JAMA. 2003;290(14):1868–1874. , .
- Association of corticosteroid dose and route of administration with risk of treatment failure in acute exacerbation of chronic obstructive pulmonary disease. JAMA. 2010;303(23):2359–2367. , , , , , .
- The relationship between hospital spending and mortality in patients with sepsis. Arch Intern Med. 2011;171(4):292–299. , , , , , .
- Comparative effectiveness of macrolides and quinolones for patients hospitalized with acute exacerbations of chronic obstructive pulmonary disease (AECOPD). J Hosp Med. 2010;5(5):261–267. , , , , , .
- A combined comorbidity score predicted mortality in elderly patients better than existing scores. J Clin Epidemiol. 2011;64(7):749–759. , , , , .
- Epidemiology of severe sepsis in the United States: analysis of incidence, outcome, and associated costs of care. Crit Care Med. 2001;29(7):1303–1310. , , , , , .
- Development and validation of a model that uses enhanced administrative data to predict mortality in patients with sepsis. Crit Care Med. 2011;39(11):2425–2430. , , , et al.
- Incorporating initial treatments improves performance of a mortality prediction model for patients with sepsis. Pharmacoepidemiol Drug Saf. 2012;21(suppl 2):44–52. , , , , .
- Nosocomial infection, length of stay, and time‐dependent bias. Infect Control Hosp Epidemiol. 2009;30(3):273–276. , , , .
- Length of stay and hospital costs among high‐risk patients with hospital‐origin Clostridium difficile‐associated diarrhea. J Med Econ. 2013;16(3):440–448. , , , , , .
- Rogers. Regression standard errors in clustered samples. Stata Technical Bulletin. 1993;13(13):19–23.
- Reducing bias in a propensity score matched‐pair sample using greedy matching techniques. In: Proceedings of the 26th Annual SAS Users Group International Conference; April 22–25, 2001; Long Beach, CA. Paper 214‐26. Available at: http://www2.sas.com/proceedings/sugi26/p214‐26.pdf. Accessed April 4, 2014. .
- Prolongation of length of stay and Clostridium difficile infection: a review of the methods used to examine length of stay due to healthcare associated infections. Antimicrob Resist Infect Control. 2012;1(1):14. , .
- Clostridium difficile Infection: a multicenter study of epidemiology and outcomes in mechanically ventilated patients. Crit Care Med. 2013;41(8):1968–1975. , , , et al.
© 2014 Society of Hospital Medicine
Acute Respiratory Failure Epidemiology
Acute respiratory failure (ARF), a common and serious complication in hospitalized patients, may be caused by several conditions including pneumonia, chronic obstructive pulmonary disease (COPD), adult respiratory distress syndrome (ARDS), and congestive heart failure (CHF). Although ARF is conventionally defined by an arterial oxygen tension of <60 mm Hg, an arterial carbon dioxide tension of >45 mm Hg, or both, these thresholds serve as a guide to be used in combination with history and clinical assessment of the patient.[1, 2] Supplemental oxygen and treatment of the underlying cause is the mainstay of therapy for ARF, but in severe cases patients are treated with invasive mechanical ventilation (IMV) or noninvasive ventilation (NIV). ARF is the most frequent reason for admission to the intensive care unit (ICU)[3, 4] and has an in‐hospital mortality rate of 33% to 37% among those who require IMV.[5, 6] The majority of epidemiologic studies of ARF have been limited to patients requiring mechanical ventilation or those admitted to the ICU, and information about the characteristics and outcomes of patients across the full spectrum of severity is much more limited.[5, 7, 8, 9, 10, 11] General improvements in the management of underlying conditions, implementation of more effective ventilation strategies,[12, 13] and increasing use of NIV[14, 15] may have led to better outcomes for patients with ARF, yet empirical evidence of a change in the adjusted mortality rate over time is lacking.
The objective of this study was to provide a broad characterization of the epidemiology of ARF among adults hospitalized in the United States using a large nationally representative database. We sought to evaluate whether incidence, mortality, cost, or ventilation practice associated with ARF in the United States changed over the period of 2001 to 2009.
METHODS
Data Source
We utilized data from the Nationwide Inpatient Sample (NIS) of the Health Care Cost and Utilization Project,[16] which is a 20% stratified probability sample of all US acute‐care hospitals each year. These data are drawn from a sampling frame that contains close to 95% of all discharges in the United States, with the hospital discharge record as the unit of analysis. The NIS has been used to study trends in many different diagnoses.[17, 18, 19] The database contains demographic information, payer information, principal and secondary diagnoses, cost, discharge disposition, and death during hospitalization. It also contains information on hospital characteristics including ownership, size, teaching status, and geographic region.
Definitions
We included patients 18 years old discharged between 2001 and 2009 with a primary or secondary diagnosis of ARF. We identified cases of ARF using diagnostic codes (International Classification of Diseases, Ninth Revision, Clinical Modification [ICD‐9‐CM]) previously used in studies of acute organ dysfunction in sepsis (518.81, 518.82, 518.84, 518.4, 799.1, 786.09).[17, 20, 21] To define ARDS we relied on ICD‐9‐CM codes (518.4, 518.82, 518.5, 786.09) used in prior studies that showed good sensitivity and specificity.[22, 23] The use of ventilatory support was identified using the ICD‐9‐CM procedure codes[24] (93.90, 93.70, 93.71, 93.76). Comorbidities were classified using the Agency for Healthcare Research and Quality's (Rockville, MD) Healthcare Cost and Utilization Project's (HCUP) Comorbidity Software version 3.103.5.[25]
Outcomes
The primary outcomes included the annual number of hospitalizations, population incidence, hospital mortality, and costs of care. Secondary outcomes included length of stay, most common diagnoses associated with ARF, disposition at discharge, and use and type of ventilatory support.
Analysis
We estimated the number of hospitalizations with a diagnosis of ARF/year, and we calculated the weighted frequencies following HCUP‐NIS recommendations using SAS/STAT survey procedures. Using population estimates for the years 2001 to 2009 from the US Census Bureau, we employed direct standardization to calculate age‐, gender‐, and race‐adjusted population incidence and mortality rates of ARF per 100,000 population. Hospital mortality was defined as the ratio of ARF hospitalizations ending in death divided by total number of ARF hospitalizations. Mechanical ventilation rates and rates of selected comorbidities were similarly defined.
We employed indirect standardization to adjust hospital mortality rates for age, sex, race/ethnicity, comorbidities, and hospital characteristics using logistic regression models from 2001 to predict hospital mortality for 2002 to 2009. We used linear regression models to test whether the slope of year was significant for trends in outcomes overtime. Costs were calculated using hospital‐specific cost‐to‐charge ratios when available and a weighted group average at the state level for remaining hospitals. We converted all costs to 2009 US dollars using the Consumer Price Index. Costs and lengths of stay were not normally distributed, so we calculated weighted geometric means (the average of all logarithmic values), then converted back to a base‐10 number. Using a Taylor series expansion, we then calculated standard errors. All analyses were performed using SAS version 9.2 (SAS Institute, Inc., Cary, NC).
The Baystate Medical Center institutional review board determined that the project did not constitute human subjects research.
RESULTS
Hospitalization Trends
The number of hospitalizations with an ARF diagnosis code increased at an average annual rate of 11.3% from 1,007,549 (standard deviation [SD] = 19,268) in 2001 to 1,917,910 (SD = 47,558) in 2009. More than two‐thirds of ARF admissions were associated with medical, rather than surgical, conditions (69.5% in 2001 and 71.2% in 2009). The median age, racial make‐up, and gender did not change significantly. Over the study period we observed an increase in ARF‐related hospitalizations in large, urban, teaching hospitals and in hospitals located in the Midwest (Table 1).
2001 | 2003 | 2005 | 2007 | 2009 | |
---|---|---|---|---|---|
| |||||
Patient characteristics | |||||
All, N (SD) | 1,007,549 (19,268) | 1,184,928 (25,542) | 1,288,594 (30,493) | 1,480,270 (32,002) | 1,917,910 (47,558) |
Age, mean (SE), y | 66.6 (0.2) | 66.0 (0.2) | 66.1 (0.2) | 65.8 (0.2) | 65.8 (0.2) |
Age group, % | |||||
1844 | 11.5 | 12.0 | 11.5 | 11.6 | 10.9 |
4564* | 26.7 | 28.9 | 29.6 | 30.7 | 31.7 |
6584* | 50.2 | 47.8 | 47.0 | 45.7 | 45.3 |
85+ | 11.5 | 11.4 | 11.9 | 12.0 | 12.1 |
Male* | 48.1 | 48.2 | 48.6 | 49.3 | 49.2 |
Race | |||||
White | 75.8 | 71.9 | 76.5 | 71.8 | 73.4 |
Black | 12.7 | 13.6 | 11.2 | 14.2 | 12.5 |
Hispanic | 7.2 | 9.8 | 7.7 | 8.5 | 7.8 |
Other | 4.2 | 4.7 | 4.7 | 5.5 | 6.3 |
Primary ARF | 20.7 | 20.9 | 25.9 | 26.1 | 19.9 |
Secondary ARF | 79.3 | 79.1 | 74.1 | 73.9 | 80.1 |
Medical* | 69.5 | 69.1 | 69.9 | 70.2 | 71.2 |
Surgical* | 30.5 | 30.8 | 30.1 | 29.8 | 28.8 |
Hospital characteristics, % | |||||
Number of beds | |||||
Small | 10.0 | 10.1 | 10.5 | 10.8 | 11.3 |
Medium | 25.2 | 25.3 | 24.6 | 24.0 | 22.7 |
Large | 64.7 | 64.6 | 64.9 | 65.2 | 66.0 |
Region | |||||
South* | 18.5 | 18.5 | 17.6 | 17.0 | 16.3 |
Midwest | 21.4 | 22.0 | 23.6 | 23.2 | 23.5 |
Northeast | 42.6 | 41.7 | 41.4 | 42.2 | 42.1 |
West* | 17.5 | 17.8 | 17.3 | 17.6 | 18.1 |
Hospital type | |||||
Rural | 13.6 | 13.0 | 11.8 | 11.0 | 10.8 |
Urban nonteaching | 45.5 | 44.5 | 50.1 | 45.3 | 45.7 |
Urban teaching | 40.9 | 42.5 | 38.1 | 43.7 | 43.6 |
Patient outcomes | |||||
Ventilation strategy | |||||
IMV* | 48.5 | 48.4 | 47.5 | 46.5 | 42.1 |
NIV* | 3.8 | 5.3 | 6.9 | 9.4 | 10.1 |
IMV or NIV | 50.9 | 51.7 | 52.1 | 52.9 | 49.7 |
Disposition | |||||
Home/home healthcare* | 42.1 | 43.8 | 42.8 | 43.4 | 45.7 |
Transfer to acute care | 5.2 | 4.7 | 4.6 | 4.6 | 4.4 |
Nursing facility* | 24.4 | 24.9 | 27.4 | 28.6 | 29.0 |
Other | 0.7 | 0.8 | 0.9 | 0.9 | 1.0 |
Adjusted mortality, % (SE)* | 27.6 (0.3) | 26.4 (0.4) | 24.9 (0.4) | 22.7 (0.4) | 20.6 (0.3) |
Adjusted mean, LOS/case, d (SE)* | 7.8 (0.1) | 7.9 (0.1) | 7.7 (0.1) | 7.5 (0.1) | 7.1 (0.1) |
Adjusted mean cost/case, 2009 US$, (SE) | 15,818 (251) | 16,981 (419) | 17,236 (411) | 16,941 (436) | 15,987 (402) |
After adjusting for age and sex, the population incidence of ARF increased from 502 (standard error [SE] = 10) cases per 100,000 in 2001 to 784 (SE = 19) cases per 100,000 in 2009 (a 56% increase, P < 0.0001). Hispanics had the lowest rates of ARF, with both black and white groups having similar rates (Table 2).
2001 | 2003 | 2005 | 2007 | 2009 | |
---|---|---|---|---|---|
| |||||
All* | 502 (10) | 569 (12) | 595 (14) | 627 (14) | 784 (19) |
Age group | |||||
1844* | 107 (3) | 130 (4) | 137 (4) | 153 (5) | 189 (6) |
4564* | 422 (9) | 500 (12) | 521 (13) | 580 (14) | 739 (19) |
6584* | 1697 (35) | 1863 (42) | 1950 (50) | 2066 (46) | 2578 (69) |
85+ | 3449 (86) | 3792 (106) | 3981 (120) | 3429 (97) | 4163 (123) |
Sex | |||||
Male* | 491 (10) | 553 (13) | 582 (14) | 629 (14) | 782 (20) |
Female* | 512 (10) | 583 (12) | 607 (15) | 625 (13) | 786 (19) |
Race/ethnicity | |||||
White* | 398 (11) | 427 (12) | 466 (16) | 450 (13) | 699 (21) |
Black* | 423 (27) | 513 (33) | 432 (26) | 574 (38) | 738 (37) |
Hispanic* | 247 (24) | 381 (42) | 307 (27) | 353 (34) | 478 (42) |
Other* | 268 (20) | 342 (29) | 347 (26) | 424 (29) | 713 (77) |
In‐hospital mortality | 140 (3) | 148 (3) | 146 (3) | 140 (3) | 154 (4) |
The most common etiologies of ARF among medical patients were pneumonia, CHF, ARDS, COPD exacerbation, and sepsis. Over the 9‐year study, the proportion of cases secondary to pneumonia and sepsis rose significantly: from 39% to 46% and 13% to 21%, respectively (Figure 1).
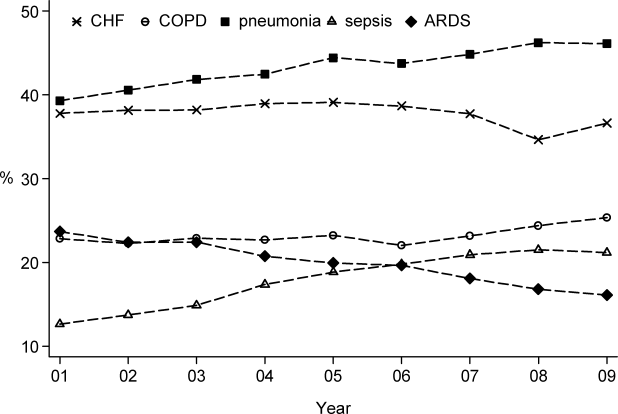
Mortality and Other Outcomes
The number of in‐hospital deaths related to ARF increased from 277,407 deaths in 2001 to 381,155 in 2009 (a 37% increase, P < 0.001). Standardized to the population, deaths increased from 140 in 2001 to 154 cases per 100,000 in 2009 (a 10% increase, P = 0.027). Despite slightly increasing mortality rates at a population level, adjusted in‐hospital mortality improved from 27.6% in 2001 to 20.6% in 2009 (P < 0.001). Mortality declined for both IMV and NIV patients from 35.3% in 2001 to 30.2% in 2009 and from 23.5% to 19%, respectively, but increased for those who required both NIV and IMV (from 26.9% in 2001 to 28% in 2009).
Adjusted hospital length of stay decreased from 7.8 days per patient in 2001 to 7.1 days in 2009 (P < 0.001), with a concomitant increase in discharges to nursing facilities, from 24% in 2001 to 29% in 2009. There was no linear trend in adjusted cost per case, with $15,818 in 2001 and $15,987 in 2009 (in 2009 US dollars) (Table 1).
Ventilation Practices
Overall, 50.9% patients received ventilatory support (NIV or IMV or both) in 2001 and 49.7% in 2009 (P= 0.25). The use of NIV increased from 3.8% to 10.1% (P < 0.001), a 169% increase, whereas the utilization of IMV decreased from 48.5% in 2001 to 42.1% in 2009 (P for trend < 0.0001), a 13% decrease. Uses of both NIV and IMV during hospitalization were seen in 1.4% of cases in 2001 and 2.5% of cases in 2009.
2009 Data Analysis
In 2009 the 1,917,910 hospitalizations with ARF resulted in 381,155 (SD = 8965) deaths and a total inpatient cost of $54 billion. The most common etiologies in patients over 65 years old were pneumonia, CHF, COPD, ARDS, and sepsis. In patients younger than 45 years the most frequent diagnoses were pneumonia, ARDS, sepsis, asthma, drug ingestion, and trauma. Stratified analysis by gender and by age groups showed that mortality rates among men were higher than for women and were highest in patients older than 85 years (Table 3).
Disease | Total | Age <45 Years | 4565 Years | 6584 Years | 85+ Years | Male | Female |
---|---|---|---|---|---|---|---|
| |||||||
Medical | |||||||
Total, N (%) | 1,364,624 (71.2) | 144,715 (10.6) | 416,922 (30.6) | 615,009 (45.1) | 187,977 (13.8) | 647,894 (47.5) | 716,635 (52.5) |
Pneumonia, %* | 46.1 | 41.7 | 42.8 | 46.9 | 54.3 | 48.8 | 43.7 |
CHF, %* | 36.6 | 10.4 | 27.3 | 43.6 | 54.8 | 35.0 | 38.1 |
ARDS, %* | 16.1 | 22.9 | 16.2 | 14.5 | 15.9 | 15.5 | 16.7 |
Sepsis, %* | 21.2 | 18.1 | 21.3 | 21.3 | 23.1 | 22.8 | 19.8 |
COPD, %* | 25.4 | 4.2 | 25.6 | 32.3 | 18.3 | 25.0 | 25.7 |
AMI, %* | 9.0 | 2.6 | 7.1 | 10.5 | 13.3 | 9.3 | 8.8 |
Asthma, %* | 9.2 | 18.1 | 11.6 | 6.7 | 5.4 | 6.2 | 12.0 |
Stroke, %* | 4.8 | 2.3 | 4.1 | 5.5 | 6.0 | 5.0 | 4.7 |
Trauma or burns, %* | 3.4 | 5.4 | 2.9 | 3.0 | 4.1 | 4.3 | 2.5 |
Cardiorespiratory arrest, %* | 4.1 | 3.9 | 4.4 | 4.1 | 3.8 | 4.6 | 3.7 |
Drug, %* | 3.7 | 16.6 | 5.1 | 0.8 | 0.3 | 3.8 | 3.6 |
IMV, %* | 37.7 | 54.6 | 43.7 | 33.5 | 24.8 | 41.1 | 34.5 |
NIV, %* | 11.9 | 7.1 | 11.5 | 13.0 | 12.7 | 11.4 | 12.3 |
In‐hospital mortality (CI) | 22 (21.322.7) | 12.9 (11.913.9) | 18.5 (17.619.4) | 23.9 (23.024.9) | 31.8 (30.633.1) | 24.2 (23.325.1) | 20.9 (20.121.7) |
Surgical | |||||||
Total, N (%) | 552971 (28.8) | 64983 (11.8) | 190225 (34.4) | 254336 (46) | 43426 (7.9) | 295660 (53.5) | 257287 (46.5) |
Pneumonia, %* | 34.9 | 33.0 | 34.0 | 35.0 | 40.5 | 37.1 | 32.2 |
CHF, %* | 27.2 | 8.9 | 21.7 | 33.3 | 42.6 | 26.7 | 27.7 |
ARDS, %* | 45.5 | 51.5 | 45.2 | 44.7 | 42.7 | 45.0 | 46.1 |
Sepsis, %* | 25.1 | 22.8 | 25.4 | 25.2 | 26.1 | 25.4 | 24.7 |
COPD, %* | 8.2 | 1.1 | 7.4 | 10.8 | 7.5 | 8.3 | 8.1 |
AMI, %* | 16.9 | 4.9 | 17.0 | 19.8 | 17.9 | 19.1 | 14.4 |
Asthma, %* | 6.1 | 7.6 | 7.2 | 5.4 | 3.6 | 4.1 | 8.5 |
Stroke, %* | 8.9 | 6.6 | 9.2 | 9.4 | 7.2 | 8.9 | 8.8 |
Trauma or burns, %* | 12.2 | 26.5 | 9.6 | 9.2 | 20.3 | 13.8 | 10.4 |
Cardiorespiratory arrest, %* | 5.5 | 4.4 | 6.0 | 5.4 | 5.2 | 6.1 | 4.7 |
Drug, %* | 0.5 | 1.3 | 0.7 | 0.2 | 0.2 | 0.4 | 0.6 |
IMV, %* | 52.9 | 57.1 | 54.3 | 51.3 | 50.0 | 54.5 | 51.0 |
NIV, %* | 5.8 | 3.5 | 5.5 | 6.4 | 6.4 | 5.6 | 6.0 |
In‐hospital mortality, % (CI) | 18.6 (17.819.5) | 10.7 (9.312.0) | 15.5 (14.216.8) | 20.8 (19.821.9) | 29.4 (27.831.1) | 19.0 (18.219.8) | 18.3 (17.319.2) |
When we examined ventilation practices among medical patients we found that patients older than 85 years, when compared to patients younger than 45 years, were less likely to be treated with IMV (25% vs 55%) and more likely to be treated with NIV (12.7% vs 7%). At the same time, the average cost per case was lowest among patients 85 years and older, and hospital costs per case fell sharply after age 70 years. Costs were considerably higher for those who did not survive during hospitalization, particularly for patients younger than 45 years (Figure 2).
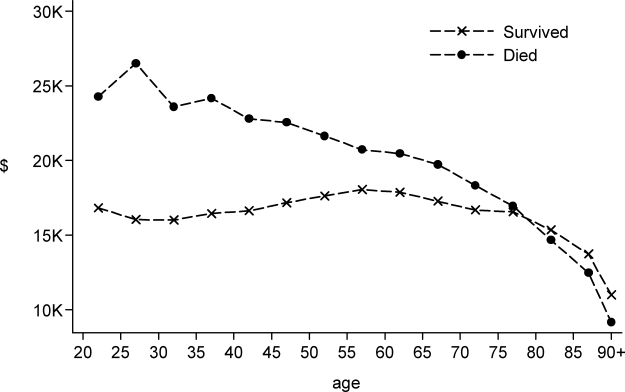
DISCUSSION
In this large population‐based study, we found that the number of hospitalizations associated with a diagnosis of ARF almost doubled over a 9‐year period. In 2009 there were nearly 2 million hospitalizations with ARF in the United States, resulting in approximately 380,000 deaths and inpatient costs of over $54 billion. The population‐adjusted ARF hospitalization rates increased in all age groups, and patients 85 years and older had the highest age‐specific hospitalization rate. Although overall rates of mechanical ventilation (NIV or IMV) remained stable over the 9‐year period, there was an important shift away from IMV (which decreased from 48% in 2001 to 42% in 2009) toward NIV (which increased from 4% in 2001 to 10% in 2009). Overall, there was a significant increase in the number of total deaths despite a decline in adjusted in‐hospital mortality rates. In‐hospital mortality rates decreased for all cases of ARF regardless of ventilation choice.
The findings of this study mirror results of others that have shown that although the incidence of critical care illnesses like sepsis[17, 20, 21, 26] and acute renal failure[27] has increased over the last decade, in‐hospital mortality rates have decreased.[20, 21, 28] Our results also compliment the results of a recent study that looked at hospitalizations for noncardiogenic ARF, which observed a 3.7‐fold increase in the number of cases and a steady decline in case fatality.[11]
Most prior studies addressing the incidence of ARF have included only patients receiving mechanical ventilation. In 1994, the estimated number of cases of ARF requiring IMV was 329,766,[9] which increased to 790,257 in 2005.[10] In our study we found that in 2009, the number of patients with ARF hospitalizations with IMV increased to 806,538. The increase in the overall number of cases with ARF was mainly driven by a surge in cases of sepsis and pneumonia. Our findings are consistent with national trends over time in noncardiogenic ARF[11] and in conditions that predispose patients to ARF such as sepsis[17, 20, 28] and acute renal failure.[27] As the number of claims for ARF doubled and the number of deaths increased, we found that adjusted hospital mortality improved from 27.6% in 2001 to 20.6% in 2009. This decline in hospital mortality was observed among all patients groups, regardless of ventilation choice. The decline in overall case fatality is consistent with prior findings in noncardiogenic ARF,[11] sepsis,[17, 28] and CHF.[29]
There are a number of potential explanations for the reduction in mortality observed over the study period, including improvements in hospital management of the underlying conditions leading to ARF, an increase in the proportion of patients being treated with NIV,[30] and advances in the care of critically ill patients such as the use of low‐tidal volume ventilation.[31, 32] Another contributor may be an increase in the proportion of discharges to nursing facilities, although this change in discharge disposition cannot fully explain our findings. For example, from 2007 to 2009, mortality decreased by 2 percentage points, and nursing home discharges increased by only 0.4 percentage points. Growth and aging of the US population only partially explain the increase we observed in the incidence of ARF, as age‐ and sex‐adjusted population rates increased by 56% from 2001 to 2009. In addition, the NIS captures data on hospital discharges and not individual patients; thus, a patient may have had multiple admissions. Over the last decade adoption of a more intensive practice style has been associated with improved in‐hospital mortality,[33, 34] and although these patients may be living longer they may have multiple readmissions.[35, 36]
We also observed that older patients were less likely to be treated with IMV, had a higher mortality rate, and less expensive care. These results are consistent with other studies and suggest that the intensity of treatment decreases with increasing age, and decisions to withhold or withdraw life‐supporting treatments are more frequent in the elderly.[26, 37] Prior research has shown that severity of illness is more important than age on patients' prognosis,[38, 39] and aggressive treatment strategies are not less cost‐effective when provided to older patients.[40]
Another important finding of this study is the marked increase in the use of NIV paired with a modest reduction in the use of IMV in the treatment of patients with ARF. This finding adds to evidence from other studies, which have similarly reported a dramatic increase in the use of NIV and a decrease in the use of IMV in patients with COPD as well as in ARF of other etiologies.[30, 41]
Our work has several limitations. First, we identified ARF based on ICD‐9‐CM codes and therefore cannot exclude disease misclassification. We did not find any studies in the literature addressing the accuracy and the completeness of ARF coding. However, we employed the same codes used to define ARF as has been used to define organ dysfunction in studies of severe sepsis,[17, 20] and the ICD‐9‐CM codes that we used to identify cases of ARDS have been used in prior studies.[11, 22, 23] Another limitation is that it is not clear to what extent the trends we observed may be due to changes over time in documentation and coding practices. Although this should be considered given the additional reimbursement associated with the diagnosis of ARF, our observation that rates of assisted ventilation have remained almost flat over the 9‐year period of the study suggest that would not wholly account for the rise in ARF. Second, because we did not have access to physiological data such as results of blood gas testing, we could not determine whether the threshold for applying the diagnosis of ARF or for delivering ventilatory support has changed over time. Third, for the purpose of this study we employed a broad definition of ARF, not limiting cases to those requiring mechanical ventilation, and this led to a more heterogeneous cohort including less severe cases of ARF. However, this is not dissimilar to the heterogeneity in disease severity observed among patients who receive a diagnosis of heart failure or acute renal failure. Fourth, survivors of ARF remain at high risk of death in the months after hospitalization,[42] but we assessed only in‐hospital mortality. It is possible that although in‐hospital mortality has improved, 30‐day mortality remained stable. Finally, as the NIS contains only discharge‐level data, we could not distinguish between patients admitted for ARF from those who developed ARF (potentially iatrogenic) after admission.
In summary, over the period of 2001 to 2009, there was a large increase in the number of patients given a diagnosis of ARF and a concomitant reduction in inpatient mortality. Although rates of mechanical ventilation remained relatively constant, there was a significant shift toward greater use of NIV at the expense of IMV.
Disclosures
Dr. Stefan is supported by KM1 CA156726 from the National Cancer Institute (NCI) and by the National Center for Research Resources and the National Center for Advancing Translational Sciences, National Institutes of Health (NIH), through grant UL1 RR025752. The work on this study was supported by a Charlton grant from Tufts University School of Medicine. Dr. Lindenauer and Dr. Pekow are supported by 1R18HL108810‐01 from the National Heart, Lung, and Blood Institute (NHLBI). The content of this publication is solely the responsibility of the authors and does not represent the official views of the NIH, NHLBI, or NCI.
All authors have read and approved the manuscript and none of them have any potential conflicts of interest to report.
Dr. Stefan had full access to all of the data in the study and takes responsibility for the integrity of the data and the accuracy of the data analysis. Conception and design: Mihaela S. Stefan, Penelope S. Pekow, Michael B. Rothberg, Jay Steingrub, Peter K. Lindenauer; analysis and interpretation: Meng‐Shiou Shieh, Mihaela S. Stefan, Penelope S. Pekow, Michael B. Rothberg, Tara Lagu, Peter K. Lindenauer; drafting the manuscript for important intellectual content: Mihaela S. Stefan, Penelope S. Pekow, Michael B. Rothberg, Jay Steingrub, Tara Lagu, and Peter K. Lindenauer.
- Goldman's Cecil Medicine. 24th ed. Amsterdam, the Netherlands: Elsevier Inc.; 2012. , .
- Textbook of Respiratory Medicine. 5th ed. Philadelphia, PA: Saunders; 2010. , .
- Epidemiology and outcome of acute respiratory failure in intensive care unit patients. Crit Care Med. 2003;31(4 suppl):S296–S299. , , .
- Epidemiology of critical care syndromes, organ failures, and life‐support interventions in a suburban US community. Chest. 2011;140(6):1447–1455. , , , et al.
- The changing epidemiology of mechanical ventilation: a population‐based study. J Intensive Care Med. 2006;21(3):173–182. , , , , .
- Mechanical ventilation in Ontario, 1992–2000: incidence, survival, and hospital bed utilization of noncardiac surgery adult patients. Crit Care Med. 2004;32(7):1504–1509. , , , , .
- Contributions to the epidemiology of acute respiratory failure. Crit Care. 2003;7(4):288–290. .
- Incidence, severity, and mortality of acute respiratory failure in Berlin, Germany. Am J Respir Crit Care Med. 1995;151(4):1121–1125. , , , et al.
- Acute respiratory failure in the United States: incidence and 31‐day survival. Chest. 2000;118(4):1100–1105. .
- The epidemiology of mechanical ventilation use in the United States. Crit Care Med. 2010;38(10):1947–1953. , , , , , .
- Trends in the incidence of noncardiogenic acute respiratory failure: the role of race. Crit Care Med. 2012;40(5):1532–1538. , , , .
- Secular trends in nosocomial infections and mortality associated with noninvasive ventilation in patients with exacerbation of COPD and pulmonary edema. JAMA. 2003;290(22):2985–2991. , , , , .
- Association of noninvasive ventilation with nosocomial infections and survival in critically ill patients. JAMA. 2000;284(18):2361–2367. , , , et al.
- Noninvasive versus conventional mechanical ventilation. An epidemiologic survey. Am J Respir Crit Care Med. 2001;163(4):874–880. , , , , .
- Does noninvasive ventilation reduce the ICU nosocomial infection risk? A prospective clinical survey. Intensive Care Med. 1999;25(6):567–573. , , , , , .
- Heathcare Cost and Utilization Project (HCUP). Overview of the Nationwide Inpatient Sample. Available at: http://www.hcup‐us.ahrq.gov/nisoverview.jsp. Accessed December 6, 2011.
- Hospitalizations, costs, and outcomes of severe sepsis in the United States 2003 to 2007. Crit Care Med. 2011;40(3):754–761. , , , , , .
- Association of diagnostic coding with trends in hospitalizations and mortality of patients with pneumonia, 2003–2009. JAMA. 2012;307(13):1405–1413. , , , , .
- Little evidence of correlation between growth in health care spending and reduced mortality. Health Aff (Millwood). 2010;29(8):1523–1531. , , , , .
- The epidemiology of sepsis in the United States from 1979 through 2000. N Engl J Med. 2003;348(16):1546–1554. , , , .
- Rapid increase in hospitalization and mortality rates for severe sepsis in the United States: a trend analysis from 1993 to 2003. Crit Care Med. 2007;35(5):1244–1250. , , , .
- Risk factors for ARDS in the United States: analysis of the 1993 National Mortality Followback Study. Chest. 2001;119(4):1179–1184. , , .
- Acute respiratory distress syndrome: estimated incidence and mortality rate in a 5 million‐person population base. Crit Care. 1998;2(1):29–34. , , , , , .
- Validity of procedure codes in International Classification of Diseases, 9th Revision, Clinical Modification administrative data. Med Care. 2004;42(8):801–809. , , .
- Comorbidity measures for use with administrative data. Med Care. 1998;36(1):8–27. , , , .
- Epidemiology of sepsis: an update. Crit Care Med. 2001;29(7 suppl):S109–S116. , .
- Epidemiology and outcomes of acute renal failure in hospitalized patients: a national survey. Clin J Am Soc Nephrol. 2006;1(1):43–51. , , , , , .
- Facing the challenge: decreasing case fatality rates in severe sepsis despite increasing hospitalizations. Crit Care Med. 2005;33(11):2555–2562. , , , .
- National and regional trends in heart failure hospitalization and mortality rates for Medicare beneficiaries,1998–2008. JAMA. 2011;306(15):1669–1678. , , , .
- Outcomes of noninvasive ventilation for acute exacerbations of chronic obstructive pulmonary disease in the United States, 1998–2008. Am J Respir Crit Care Med. 2011;185(2):152–159. , , , et al.
- A trial of goal‐oriented hemodynamic therapy in critically ill patients. SvO2 Collaborative Group. N Engl J Med. 1995;333(16):1025–1032. , , , et al.
- Ventilation with lower tidal volumes as compared with traditional tidal volumes for acute lung injury. N Engl J Med. 2000;343(11):813; author reply 813–814. , .
- Short‐ and long‐term survival of nonsurgical intensive care patients and its relation to diagnosis, severity of disease, age and comorbidities. Curr Aging Sci. 2009;2(3):240–248. , , .
- The impact of COPD on management and outcomes of patients hospitalized with acute myocardial infarction—a ten‐year retrospective observational study. Chest. 2012;141(6):1441–1448. , , , , , .
- The paradox of health. N Engl J Med. 1988;318(7):414–418. .
- Rehospitalizations among patients in the Medicare fee‐for‐service program. N Engl J Med. 2009;360(14):1418–1428. , , .
- Outcomes and cost‐effectiveness of ventilator support and aggressive care for patients with acute respiratory failure due to pneumonia or acute respiratory distress syndrome. Am J Med. 2000;109(8):614–620. , , , et al.
- Older age, aggressiveness of care, and survival for seriously ill, hospitalized adults. SUPPORT Investigators. Study to Understand Prognoses and Preferences for Outcomes and Risks of Treatments. Ann Intern Med. 1999;131(10):721–728. , , , et al.
- Patient age and decisions to withhold life‐sustaining treatments from seriously ill, hospitalized adults. SUPPORT Investigators. Study to Understand Prognoses and Preferences for Outcomes and Risks of Treatment. Ann Intern Med. 1999;130(2):116–125. , , , et al.
- Are aggressive treatment strategies less cost‐effective for older patients? The case of ventilator support and aggressive care for patients with acute respiratory failure. J Am Geriatr Soc. 2001;49(4):382–390. , , , et al.
- Utilization of non‐invasive ventilation in patients with acute respiratory failure from 2000–2009: a population‐based study. Am J Respir Crit Care Med. 2012;185:A6488. , .
- One‐year outcomes in survivors of the acute respiratory distress syndrome. N Engl J Med. 2003;348(8):683–693. , , , et al.
Acute respiratory failure (ARF), a common and serious complication in hospitalized patients, may be caused by several conditions including pneumonia, chronic obstructive pulmonary disease (COPD), adult respiratory distress syndrome (ARDS), and congestive heart failure (CHF). Although ARF is conventionally defined by an arterial oxygen tension of <60 mm Hg, an arterial carbon dioxide tension of >45 mm Hg, or both, these thresholds serve as a guide to be used in combination with history and clinical assessment of the patient.[1, 2] Supplemental oxygen and treatment of the underlying cause is the mainstay of therapy for ARF, but in severe cases patients are treated with invasive mechanical ventilation (IMV) or noninvasive ventilation (NIV). ARF is the most frequent reason for admission to the intensive care unit (ICU)[3, 4] and has an in‐hospital mortality rate of 33% to 37% among those who require IMV.[5, 6] The majority of epidemiologic studies of ARF have been limited to patients requiring mechanical ventilation or those admitted to the ICU, and information about the characteristics and outcomes of patients across the full spectrum of severity is much more limited.[5, 7, 8, 9, 10, 11] General improvements in the management of underlying conditions, implementation of more effective ventilation strategies,[12, 13] and increasing use of NIV[14, 15] may have led to better outcomes for patients with ARF, yet empirical evidence of a change in the adjusted mortality rate over time is lacking.
The objective of this study was to provide a broad characterization of the epidemiology of ARF among adults hospitalized in the United States using a large nationally representative database. We sought to evaluate whether incidence, mortality, cost, or ventilation practice associated with ARF in the United States changed over the period of 2001 to 2009.
METHODS
Data Source
We utilized data from the Nationwide Inpatient Sample (NIS) of the Health Care Cost and Utilization Project,[16] which is a 20% stratified probability sample of all US acute‐care hospitals each year. These data are drawn from a sampling frame that contains close to 95% of all discharges in the United States, with the hospital discharge record as the unit of analysis. The NIS has been used to study trends in many different diagnoses.[17, 18, 19] The database contains demographic information, payer information, principal and secondary diagnoses, cost, discharge disposition, and death during hospitalization. It also contains information on hospital characteristics including ownership, size, teaching status, and geographic region.
Definitions
We included patients 18 years old discharged between 2001 and 2009 with a primary or secondary diagnosis of ARF. We identified cases of ARF using diagnostic codes (International Classification of Diseases, Ninth Revision, Clinical Modification [ICD‐9‐CM]) previously used in studies of acute organ dysfunction in sepsis (518.81, 518.82, 518.84, 518.4, 799.1, 786.09).[17, 20, 21] To define ARDS we relied on ICD‐9‐CM codes (518.4, 518.82, 518.5, 786.09) used in prior studies that showed good sensitivity and specificity.[22, 23] The use of ventilatory support was identified using the ICD‐9‐CM procedure codes[24] (93.90, 93.70, 93.71, 93.76). Comorbidities were classified using the Agency for Healthcare Research and Quality's (Rockville, MD) Healthcare Cost and Utilization Project's (HCUP) Comorbidity Software version 3.103.5.[25]
Outcomes
The primary outcomes included the annual number of hospitalizations, population incidence, hospital mortality, and costs of care. Secondary outcomes included length of stay, most common diagnoses associated with ARF, disposition at discharge, and use and type of ventilatory support.
Analysis
We estimated the number of hospitalizations with a diagnosis of ARF/year, and we calculated the weighted frequencies following HCUP‐NIS recommendations using SAS/STAT survey procedures. Using population estimates for the years 2001 to 2009 from the US Census Bureau, we employed direct standardization to calculate age‐, gender‐, and race‐adjusted population incidence and mortality rates of ARF per 100,000 population. Hospital mortality was defined as the ratio of ARF hospitalizations ending in death divided by total number of ARF hospitalizations. Mechanical ventilation rates and rates of selected comorbidities were similarly defined.
We employed indirect standardization to adjust hospital mortality rates for age, sex, race/ethnicity, comorbidities, and hospital characteristics using logistic regression models from 2001 to predict hospital mortality for 2002 to 2009. We used linear regression models to test whether the slope of year was significant for trends in outcomes overtime. Costs were calculated using hospital‐specific cost‐to‐charge ratios when available and a weighted group average at the state level for remaining hospitals. We converted all costs to 2009 US dollars using the Consumer Price Index. Costs and lengths of stay were not normally distributed, so we calculated weighted geometric means (the average of all logarithmic values), then converted back to a base‐10 number. Using a Taylor series expansion, we then calculated standard errors. All analyses were performed using SAS version 9.2 (SAS Institute, Inc., Cary, NC).
The Baystate Medical Center institutional review board determined that the project did not constitute human subjects research.
RESULTS
Hospitalization Trends
The number of hospitalizations with an ARF diagnosis code increased at an average annual rate of 11.3% from 1,007,549 (standard deviation [SD] = 19,268) in 2001 to 1,917,910 (SD = 47,558) in 2009. More than two‐thirds of ARF admissions were associated with medical, rather than surgical, conditions (69.5% in 2001 and 71.2% in 2009). The median age, racial make‐up, and gender did not change significantly. Over the study period we observed an increase in ARF‐related hospitalizations in large, urban, teaching hospitals and in hospitals located in the Midwest (Table 1).
2001 | 2003 | 2005 | 2007 | 2009 | |
---|---|---|---|---|---|
| |||||
Patient characteristics | |||||
All, N (SD) | 1,007,549 (19,268) | 1,184,928 (25,542) | 1,288,594 (30,493) | 1,480,270 (32,002) | 1,917,910 (47,558) |
Age, mean (SE), y | 66.6 (0.2) | 66.0 (0.2) | 66.1 (0.2) | 65.8 (0.2) | 65.8 (0.2) |
Age group, % | |||||
1844 | 11.5 | 12.0 | 11.5 | 11.6 | 10.9 |
4564* | 26.7 | 28.9 | 29.6 | 30.7 | 31.7 |
6584* | 50.2 | 47.8 | 47.0 | 45.7 | 45.3 |
85+ | 11.5 | 11.4 | 11.9 | 12.0 | 12.1 |
Male* | 48.1 | 48.2 | 48.6 | 49.3 | 49.2 |
Race | |||||
White | 75.8 | 71.9 | 76.5 | 71.8 | 73.4 |
Black | 12.7 | 13.6 | 11.2 | 14.2 | 12.5 |
Hispanic | 7.2 | 9.8 | 7.7 | 8.5 | 7.8 |
Other | 4.2 | 4.7 | 4.7 | 5.5 | 6.3 |
Primary ARF | 20.7 | 20.9 | 25.9 | 26.1 | 19.9 |
Secondary ARF | 79.3 | 79.1 | 74.1 | 73.9 | 80.1 |
Medical* | 69.5 | 69.1 | 69.9 | 70.2 | 71.2 |
Surgical* | 30.5 | 30.8 | 30.1 | 29.8 | 28.8 |
Hospital characteristics, % | |||||
Number of beds | |||||
Small | 10.0 | 10.1 | 10.5 | 10.8 | 11.3 |
Medium | 25.2 | 25.3 | 24.6 | 24.0 | 22.7 |
Large | 64.7 | 64.6 | 64.9 | 65.2 | 66.0 |
Region | |||||
South* | 18.5 | 18.5 | 17.6 | 17.0 | 16.3 |
Midwest | 21.4 | 22.0 | 23.6 | 23.2 | 23.5 |
Northeast | 42.6 | 41.7 | 41.4 | 42.2 | 42.1 |
West* | 17.5 | 17.8 | 17.3 | 17.6 | 18.1 |
Hospital type | |||||
Rural | 13.6 | 13.0 | 11.8 | 11.0 | 10.8 |
Urban nonteaching | 45.5 | 44.5 | 50.1 | 45.3 | 45.7 |
Urban teaching | 40.9 | 42.5 | 38.1 | 43.7 | 43.6 |
Patient outcomes | |||||
Ventilation strategy | |||||
IMV* | 48.5 | 48.4 | 47.5 | 46.5 | 42.1 |
NIV* | 3.8 | 5.3 | 6.9 | 9.4 | 10.1 |
IMV or NIV | 50.9 | 51.7 | 52.1 | 52.9 | 49.7 |
Disposition | |||||
Home/home healthcare* | 42.1 | 43.8 | 42.8 | 43.4 | 45.7 |
Transfer to acute care | 5.2 | 4.7 | 4.6 | 4.6 | 4.4 |
Nursing facility* | 24.4 | 24.9 | 27.4 | 28.6 | 29.0 |
Other | 0.7 | 0.8 | 0.9 | 0.9 | 1.0 |
Adjusted mortality, % (SE)* | 27.6 (0.3) | 26.4 (0.4) | 24.9 (0.4) | 22.7 (0.4) | 20.6 (0.3) |
Adjusted mean, LOS/case, d (SE)* | 7.8 (0.1) | 7.9 (0.1) | 7.7 (0.1) | 7.5 (0.1) | 7.1 (0.1) |
Adjusted mean cost/case, 2009 US$, (SE) | 15,818 (251) | 16,981 (419) | 17,236 (411) | 16,941 (436) | 15,987 (402) |
After adjusting for age and sex, the population incidence of ARF increased from 502 (standard error [SE] = 10) cases per 100,000 in 2001 to 784 (SE = 19) cases per 100,000 in 2009 (a 56% increase, P < 0.0001). Hispanics had the lowest rates of ARF, with both black and white groups having similar rates (Table 2).
2001 | 2003 | 2005 | 2007 | 2009 | |
---|---|---|---|---|---|
| |||||
All* | 502 (10) | 569 (12) | 595 (14) | 627 (14) | 784 (19) |
Age group | |||||
1844* | 107 (3) | 130 (4) | 137 (4) | 153 (5) | 189 (6) |
4564* | 422 (9) | 500 (12) | 521 (13) | 580 (14) | 739 (19) |
6584* | 1697 (35) | 1863 (42) | 1950 (50) | 2066 (46) | 2578 (69) |
85+ | 3449 (86) | 3792 (106) | 3981 (120) | 3429 (97) | 4163 (123) |
Sex | |||||
Male* | 491 (10) | 553 (13) | 582 (14) | 629 (14) | 782 (20) |
Female* | 512 (10) | 583 (12) | 607 (15) | 625 (13) | 786 (19) |
Race/ethnicity | |||||
White* | 398 (11) | 427 (12) | 466 (16) | 450 (13) | 699 (21) |
Black* | 423 (27) | 513 (33) | 432 (26) | 574 (38) | 738 (37) |
Hispanic* | 247 (24) | 381 (42) | 307 (27) | 353 (34) | 478 (42) |
Other* | 268 (20) | 342 (29) | 347 (26) | 424 (29) | 713 (77) |
In‐hospital mortality | 140 (3) | 148 (3) | 146 (3) | 140 (3) | 154 (4) |
The most common etiologies of ARF among medical patients were pneumonia, CHF, ARDS, COPD exacerbation, and sepsis. Over the 9‐year study, the proportion of cases secondary to pneumonia and sepsis rose significantly: from 39% to 46% and 13% to 21%, respectively (Figure 1).
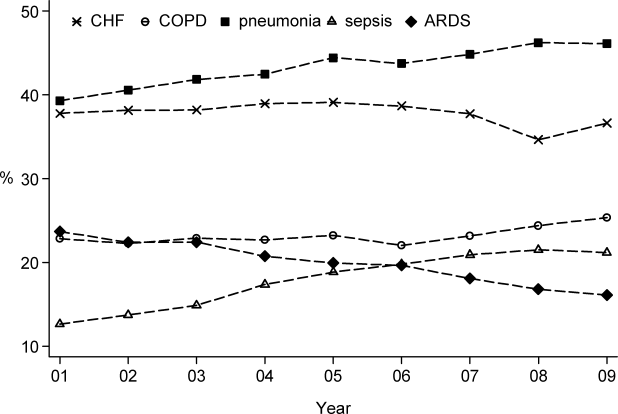
Mortality and Other Outcomes
The number of in‐hospital deaths related to ARF increased from 277,407 deaths in 2001 to 381,155 in 2009 (a 37% increase, P < 0.001). Standardized to the population, deaths increased from 140 in 2001 to 154 cases per 100,000 in 2009 (a 10% increase, P = 0.027). Despite slightly increasing mortality rates at a population level, adjusted in‐hospital mortality improved from 27.6% in 2001 to 20.6% in 2009 (P < 0.001). Mortality declined for both IMV and NIV patients from 35.3% in 2001 to 30.2% in 2009 and from 23.5% to 19%, respectively, but increased for those who required both NIV and IMV (from 26.9% in 2001 to 28% in 2009).
Adjusted hospital length of stay decreased from 7.8 days per patient in 2001 to 7.1 days in 2009 (P < 0.001), with a concomitant increase in discharges to nursing facilities, from 24% in 2001 to 29% in 2009. There was no linear trend in adjusted cost per case, with $15,818 in 2001 and $15,987 in 2009 (in 2009 US dollars) (Table 1).
Ventilation Practices
Overall, 50.9% patients received ventilatory support (NIV or IMV or both) in 2001 and 49.7% in 2009 (P= 0.25). The use of NIV increased from 3.8% to 10.1% (P < 0.001), a 169% increase, whereas the utilization of IMV decreased from 48.5% in 2001 to 42.1% in 2009 (P for trend < 0.0001), a 13% decrease. Uses of both NIV and IMV during hospitalization were seen in 1.4% of cases in 2001 and 2.5% of cases in 2009.
2009 Data Analysis
In 2009 the 1,917,910 hospitalizations with ARF resulted in 381,155 (SD = 8965) deaths and a total inpatient cost of $54 billion. The most common etiologies in patients over 65 years old were pneumonia, CHF, COPD, ARDS, and sepsis. In patients younger than 45 years the most frequent diagnoses were pneumonia, ARDS, sepsis, asthma, drug ingestion, and trauma. Stratified analysis by gender and by age groups showed that mortality rates among men were higher than for women and were highest in patients older than 85 years (Table 3).
Disease | Total | Age <45 Years | 4565 Years | 6584 Years | 85+ Years | Male | Female |
---|---|---|---|---|---|---|---|
| |||||||
Medical | |||||||
Total, N (%) | 1,364,624 (71.2) | 144,715 (10.6) | 416,922 (30.6) | 615,009 (45.1) | 187,977 (13.8) | 647,894 (47.5) | 716,635 (52.5) |
Pneumonia, %* | 46.1 | 41.7 | 42.8 | 46.9 | 54.3 | 48.8 | 43.7 |
CHF, %* | 36.6 | 10.4 | 27.3 | 43.6 | 54.8 | 35.0 | 38.1 |
ARDS, %* | 16.1 | 22.9 | 16.2 | 14.5 | 15.9 | 15.5 | 16.7 |
Sepsis, %* | 21.2 | 18.1 | 21.3 | 21.3 | 23.1 | 22.8 | 19.8 |
COPD, %* | 25.4 | 4.2 | 25.6 | 32.3 | 18.3 | 25.0 | 25.7 |
AMI, %* | 9.0 | 2.6 | 7.1 | 10.5 | 13.3 | 9.3 | 8.8 |
Asthma, %* | 9.2 | 18.1 | 11.6 | 6.7 | 5.4 | 6.2 | 12.0 |
Stroke, %* | 4.8 | 2.3 | 4.1 | 5.5 | 6.0 | 5.0 | 4.7 |
Trauma or burns, %* | 3.4 | 5.4 | 2.9 | 3.0 | 4.1 | 4.3 | 2.5 |
Cardiorespiratory arrest, %* | 4.1 | 3.9 | 4.4 | 4.1 | 3.8 | 4.6 | 3.7 |
Drug, %* | 3.7 | 16.6 | 5.1 | 0.8 | 0.3 | 3.8 | 3.6 |
IMV, %* | 37.7 | 54.6 | 43.7 | 33.5 | 24.8 | 41.1 | 34.5 |
NIV, %* | 11.9 | 7.1 | 11.5 | 13.0 | 12.7 | 11.4 | 12.3 |
In‐hospital mortality (CI) | 22 (21.322.7) | 12.9 (11.913.9) | 18.5 (17.619.4) | 23.9 (23.024.9) | 31.8 (30.633.1) | 24.2 (23.325.1) | 20.9 (20.121.7) |
Surgical | |||||||
Total, N (%) | 552971 (28.8) | 64983 (11.8) | 190225 (34.4) | 254336 (46) | 43426 (7.9) | 295660 (53.5) | 257287 (46.5) |
Pneumonia, %* | 34.9 | 33.0 | 34.0 | 35.0 | 40.5 | 37.1 | 32.2 |
CHF, %* | 27.2 | 8.9 | 21.7 | 33.3 | 42.6 | 26.7 | 27.7 |
ARDS, %* | 45.5 | 51.5 | 45.2 | 44.7 | 42.7 | 45.0 | 46.1 |
Sepsis, %* | 25.1 | 22.8 | 25.4 | 25.2 | 26.1 | 25.4 | 24.7 |
COPD, %* | 8.2 | 1.1 | 7.4 | 10.8 | 7.5 | 8.3 | 8.1 |
AMI, %* | 16.9 | 4.9 | 17.0 | 19.8 | 17.9 | 19.1 | 14.4 |
Asthma, %* | 6.1 | 7.6 | 7.2 | 5.4 | 3.6 | 4.1 | 8.5 |
Stroke, %* | 8.9 | 6.6 | 9.2 | 9.4 | 7.2 | 8.9 | 8.8 |
Trauma or burns, %* | 12.2 | 26.5 | 9.6 | 9.2 | 20.3 | 13.8 | 10.4 |
Cardiorespiratory arrest, %* | 5.5 | 4.4 | 6.0 | 5.4 | 5.2 | 6.1 | 4.7 |
Drug, %* | 0.5 | 1.3 | 0.7 | 0.2 | 0.2 | 0.4 | 0.6 |
IMV, %* | 52.9 | 57.1 | 54.3 | 51.3 | 50.0 | 54.5 | 51.0 |
NIV, %* | 5.8 | 3.5 | 5.5 | 6.4 | 6.4 | 5.6 | 6.0 |
In‐hospital mortality, % (CI) | 18.6 (17.819.5) | 10.7 (9.312.0) | 15.5 (14.216.8) | 20.8 (19.821.9) | 29.4 (27.831.1) | 19.0 (18.219.8) | 18.3 (17.319.2) |
When we examined ventilation practices among medical patients we found that patients older than 85 years, when compared to patients younger than 45 years, were less likely to be treated with IMV (25% vs 55%) and more likely to be treated with NIV (12.7% vs 7%). At the same time, the average cost per case was lowest among patients 85 years and older, and hospital costs per case fell sharply after age 70 years. Costs were considerably higher for those who did not survive during hospitalization, particularly for patients younger than 45 years (Figure 2).
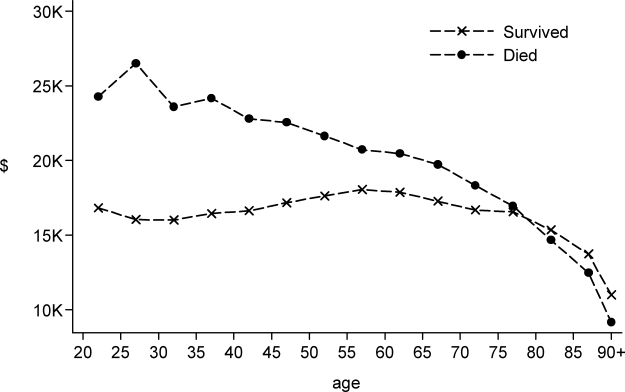
DISCUSSION
In this large population‐based study, we found that the number of hospitalizations associated with a diagnosis of ARF almost doubled over a 9‐year period. In 2009 there were nearly 2 million hospitalizations with ARF in the United States, resulting in approximately 380,000 deaths and inpatient costs of over $54 billion. The population‐adjusted ARF hospitalization rates increased in all age groups, and patients 85 years and older had the highest age‐specific hospitalization rate. Although overall rates of mechanical ventilation (NIV or IMV) remained stable over the 9‐year period, there was an important shift away from IMV (which decreased from 48% in 2001 to 42% in 2009) toward NIV (which increased from 4% in 2001 to 10% in 2009). Overall, there was a significant increase in the number of total deaths despite a decline in adjusted in‐hospital mortality rates. In‐hospital mortality rates decreased for all cases of ARF regardless of ventilation choice.
The findings of this study mirror results of others that have shown that although the incidence of critical care illnesses like sepsis[17, 20, 21, 26] and acute renal failure[27] has increased over the last decade, in‐hospital mortality rates have decreased.[20, 21, 28] Our results also compliment the results of a recent study that looked at hospitalizations for noncardiogenic ARF, which observed a 3.7‐fold increase in the number of cases and a steady decline in case fatality.[11]
Most prior studies addressing the incidence of ARF have included only patients receiving mechanical ventilation. In 1994, the estimated number of cases of ARF requiring IMV was 329,766,[9] which increased to 790,257 in 2005.[10] In our study we found that in 2009, the number of patients with ARF hospitalizations with IMV increased to 806,538. The increase in the overall number of cases with ARF was mainly driven by a surge in cases of sepsis and pneumonia. Our findings are consistent with national trends over time in noncardiogenic ARF[11] and in conditions that predispose patients to ARF such as sepsis[17, 20, 28] and acute renal failure.[27] As the number of claims for ARF doubled and the number of deaths increased, we found that adjusted hospital mortality improved from 27.6% in 2001 to 20.6% in 2009. This decline in hospital mortality was observed among all patients groups, regardless of ventilation choice. The decline in overall case fatality is consistent with prior findings in noncardiogenic ARF,[11] sepsis,[17, 28] and CHF.[29]
There are a number of potential explanations for the reduction in mortality observed over the study period, including improvements in hospital management of the underlying conditions leading to ARF, an increase in the proportion of patients being treated with NIV,[30] and advances in the care of critically ill patients such as the use of low‐tidal volume ventilation.[31, 32] Another contributor may be an increase in the proportion of discharges to nursing facilities, although this change in discharge disposition cannot fully explain our findings. For example, from 2007 to 2009, mortality decreased by 2 percentage points, and nursing home discharges increased by only 0.4 percentage points. Growth and aging of the US population only partially explain the increase we observed in the incidence of ARF, as age‐ and sex‐adjusted population rates increased by 56% from 2001 to 2009. In addition, the NIS captures data on hospital discharges and not individual patients; thus, a patient may have had multiple admissions. Over the last decade adoption of a more intensive practice style has been associated with improved in‐hospital mortality,[33, 34] and although these patients may be living longer they may have multiple readmissions.[35, 36]
We also observed that older patients were less likely to be treated with IMV, had a higher mortality rate, and less expensive care. These results are consistent with other studies and suggest that the intensity of treatment decreases with increasing age, and decisions to withhold or withdraw life‐supporting treatments are more frequent in the elderly.[26, 37] Prior research has shown that severity of illness is more important than age on patients' prognosis,[38, 39] and aggressive treatment strategies are not less cost‐effective when provided to older patients.[40]
Another important finding of this study is the marked increase in the use of NIV paired with a modest reduction in the use of IMV in the treatment of patients with ARF. This finding adds to evidence from other studies, which have similarly reported a dramatic increase in the use of NIV and a decrease in the use of IMV in patients with COPD as well as in ARF of other etiologies.[30, 41]
Our work has several limitations. First, we identified ARF based on ICD‐9‐CM codes and therefore cannot exclude disease misclassification. We did not find any studies in the literature addressing the accuracy and the completeness of ARF coding. However, we employed the same codes used to define ARF as has been used to define organ dysfunction in studies of severe sepsis,[17, 20] and the ICD‐9‐CM codes that we used to identify cases of ARDS have been used in prior studies.[11, 22, 23] Another limitation is that it is not clear to what extent the trends we observed may be due to changes over time in documentation and coding practices. Although this should be considered given the additional reimbursement associated with the diagnosis of ARF, our observation that rates of assisted ventilation have remained almost flat over the 9‐year period of the study suggest that would not wholly account for the rise in ARF. Second, because we did not have access to physiological data such as results of blood gas testing, we could not determine whether the threshold for applying the diagnosis of ARF or for delivering ventilatory support has changed over time. Third, for the purpose of this study we employed a broad definition of ARF, not limiting cases to those requiring mechanical ventilation, and this led to a more heterogeneous cohort including less severe cases of ARF. However, this is not dissimilar to the heterogeneity in disease severity observed among patients who receive a diagnosis of heart failure or acute renal failure. Fourth, survivors of ARF remain at high risk of death in the months after hospitalization,[42] but we assessed only in‐hospital mortality. It is possible that although in‐hospital mortality has improved, 30‐day mortality remained stable. Finally, as the NIS contains only discharge‐level data, we could not distinguish between patients admitted for ARF from those who developed ARF (potentially iatrogenic) after admission.
In summary, over the period of 2001 to 2009, there was a large increase in the number of patients given a diagnosis of ARF and a concomitant reduction in inpatient mortality. Although rates of mechanical ventilation remained relatively constant, there was a significant shift toward greater use of NIV at the expense of IMV.
Disclosures
Dr. Stefan is supported by KM1 CA156726 from the National Cancer Institute (NCI) and by the National Center for Research Resources and the National Center for Advancing Translational Sciences, National Institutes of Health (NIH), through grant UL1 RR025752. The work on this study was supported by a Charlton grant from Tufts University School of Medicine. Dr. Lindenauer and Dr. Pekow are supported by 1R18HL108810‐01 from the National Heart, Lung, and Blood Institute (NHLBI). The content of this publication is solely the responsibility of the authors and does not represent the official views of the NIH, NHLBI, or NCI.
All authors have read and approved the manuscript and none of them have any potential conflicts of interest to report.
Dr. Stefan had full access to all of the data in the study and takes responsibility for the integrity of the data and the accuracy of the data analysis. Conception and design: Mihaela S. Stefan, Penelope S. Pekow, Michael B. Rothberg, Jay Steingrub, Peter K. Lindenauer; analysis and interpretation: Meng‐Shiou Shieh, Mihaela S. Stefan, Penelope S. Pekow, Michael B. Rothberg, Tara Lagu, Peter K. Lindenauer; drafting the manuscript for important intellectual content: Mihaela S. Stefan, Penelope S. Pekow, Michael B. Rothberg, Jay Steingrub, Tara Lagu, and Peter K. Lindenauer.
Acute respiratory failure (ARF), a common and serious complication in hospitalized patients, may be caused by several conditions including pneumonia, chronic obstructive pulmonary disease (COPD), adult respiratory distress syndrome (ARDS), and congestive heart failure (CHF). Although ARF is conventionally defined by an arterial oxygen tension of <60 mm Hg, an arterial carbon dioxide tension of >45 mm Hg, or both, these thresholds serve as a guide to be used in combination with history and clinical assessment of the patient.[1, 2] Supplemental oxygen and treatment of the underlying cause is the mainstay of therapy for ARF, but in severe cases patients are treated with invasive mechanical ventilation (IMV) or noninvasive ventilation (NIV). ARF is the most frequent reason for admission to the intensive care unit (ICU)[3, 4] and has an in‐hospital mortality rate of 33% to 37% among those who require IMV.[5, 6] The majority of epidemiologic studies of ARF have been limited to patients requiring mechanical ventilation or those admitted to the ICU, and information about the characteristics and outcomes of patients across the full spectrum of severity is much more limited.[5, 7, 8, 9, 10, 11] General improvements in the management of underlying conditions, implementation of more effective ventilation strategies,[12, 13] and increasing use of NIV[14, 15] may have led to better outcomes for patients with ARF, yet empirical evidence of a change in the adjusted mortality rate over time is lacking.
The objective of this study was to provide a broad characterization of the epidemiology of ARF among adults hospitalized in the United States using a large nationally representative database. We sought to evaluate whether incidence, mortality, cost, or ventilation practice associated with ARF in the United States changed over the period of 2001 to 2009.
METHODS
Data Source
We utilized data from the Nationwide Inpatient Sample (NIS) of the Health Care Cost and Utilization Project,[16] which is a 20% stratified probability sample of all US acute‐care hospitals each year. These data are drawn from a sampling frame that contains close to 95% of all discharges in the United States, with the hospital discharge record as the unit of analysis. The NIS has been used to study trends in many different diagnoses.[17, 18, 19] The database contains demographic information, payer information, principal and secondary diagnoses, cost, discharge disposition, and death during hospitalization. It also contains information on hospital characteristics including ownership, size, teaching status, and geographic region.
Definitions
We included patients 18 years old discharged between 2001 and 2009 with a primary or secondary diagnosis of ARF. We identified cases of ARF using diagnostic codes (International Classification of Diseases, Ninth Revision, Clinical Modification [ICD‐9‐CM]) previously used in studies of acute organ dysfunction in sepsis (518.81, 518.82, 518.84, 518.4, 799.1, 786.09).[17, 20, 21] To define ARDS we relied on ICD‐9‐CM codes (518.4, 518.82, 518.5, 786.09) used in prior studies that showed good sensitivity and specificity.[22, 23] The use of ventilatory support was identified using the ICD‐9‐CM procedure codes[24] (93.90, 93.70, 93.71, 93.76). Comorbidities were classified using the Agency for Healthcare Research and Quality's (Rockville, MD) Healthcare Cost and Utilization Project's (HCUP) Comorbidity Software version 3.103.5.[25]
Outcomes
The primary outcomes included the annual number of hospitalizations, population incidence, hospital mortality, and costs of care. Secondary outcomes included length of stay, most common diagnoses associated with ARF, disposition at discharge, and use and type of ventilatory support.
Analysis
We estimated the number of hospitalizations with a diagnosis of ARF/year, and we calculated the weighted frequencies following HCUP‐NIS recommendations using SAS/STAT survey procedures. Using population estimates for the years 2001 to 2009 from the US Census Bureau, we employed direct standardization to calculate age‐, gender‐, and race‐adjusted population incidence and mortality rates of ARF per 100,000 population. Hospital mortality was defined as the ratio of ARF hospitalizations ending in death divided by total number of ARF hospitalizations. Mechanical ventilation rates and rates of selected comorbidities were similarly defined.
We employed indirect standardization to adjust hospital mortality rates for age, sex, race/ethnicity, comorbidities, and hospital characteristics using logistic regression models from 2001 to predict hospital mortality for 2002 to 2009. We used linear regression models to test whether the slope of year was significant for trends in outcomes overtime. Costs were calculated using hospital‐specific cost‐to‐charge ratios when available and a weighted group average at the state level for remaining hospitals. We converted all costs to 2009 US dollars using the Consumer Price Index. Costs and lengths of stay were not normally distributed, so we calculated weighted geometric means (the average of all logarithmic values), then converted back to a base‐10 number. Using a Taylor series expansion, we then calculated standard errors. All analyses were performed using SAS version 9.2 (SAS Institute, Inc., Cary, NC).
The Baystate Medical Center institutional review board determined that the project did not constitute human subjects research.
RESULTS
Hospitalization Trends
The number of hospitalizations with an ARF diagnosis code increased at an average annual rate of 11.3% from 1,007,549 (standard deviation [SD] = 19,268) in 2001 to 1,917,910 (SD = 47,558) in 2009. More than two‐thirds of ARF admissions were associated with medical, rather than surgical, conditions (69.5% in 2001 and 71.2% in 2009). The median age, racial make‐up, and gender did not change significantly. Over the study period we observed an increase in ARF‐related hospitalizations in large, urban, teaching hospitals and in hospitals located in the Midwest (Table 1).
2001 | 2003 | 2005 | 2007 | 2009 | |
---|---|---|---|---|---|
| |||||
Patient characteristics | |||||
All, N (SD) | 1,007,549 (19,268) | 1,184,928 (25,542) | 1,288,594 (30,493) | 1,480,270 (32,002) | 1,917,910 (47,558) |
Age, mean (SE), y | 66.6 (0.2) | 66.0 (0.2) | 66.1 (0.2) | 65.8 (0.2) | 65.8 (0.2) |
Age group, % | |||||
1844 | 11.5 | 12.0 | 11.5 | 11.6 | 10.9 |
4564* | 26.7 | 28.9 | 29.6 | 30.7 | 31.7 |
6584* | 50.2 | 47.8 | 47.0 | 45.7 | 45.3 |
85+ | 11.5 | 11.4 | 11.9 | 12.0 | 12.1 |
Male* | 48.1 | 48.2 | 48.6 | 49.3 | 49.2 |
Race | |||||
White | 75.8 | 71.9 | 76.5 | 71.8 | 73.4 |
Black | 12.7 | 13.6 | 11.2 | 14.2 | 12.5 |
Hispanic | 7.2 | 9.8 | 7.7 | 8.5 | 7.8 |
Other | 4.2 | 4.7 | 4.7 | 5.5 | 6.3 |
Primary ARF | 20.7 | 20.9 | 25.9 | 26.1 | 19.9 |
Secondary ARF | 79.3 | 79.1 | 74.1 | 73.9 | 80.1 |
Medical* | 69.5 | 69.1 | 69.9 | 70.2 | 71.2 |
Surgical* | 30.5 | 30.8 | 30.1 | 29.8 | 28.8 |
Hospital characteristics, % | |||||
Number of beds | |||||
Small | 10.0 | 10.1 | 10.5 | 10.8 | 11.3 |
Medium | 25.2 | 25.3 | 24.6 | 24.0 | 22.7 |
Large | 64.7 | 64.6 | 64.9 | 65.2 | 66.0 |
Region | |||||
South* | 18.5 | 18.5 | 17.6 | 17.0 | 16.3 |
Midwest | 21.4 | 22.0 | 23.6 | 23.2 | 23.5 |
Northeast | 42.6 | 41.7 | 41.4 | 42.2 | 42.1 |
West* | 17.5 | 17.8 | 17.3 | 17.6 | 18.1 |
Hospital type | |||||
Rural | 13.6 | 13.0 | 11.8 | 11.0 | 10.8 |
Urban nonteaching | 45.5 | 44.5 | 50.1 | 45.3 | 45.7 |
Urban teaching | 40.9 | 42.5 | 38.1 | 43.7 | 43.6 |
Patient outcomes | |||||
Ventilation strategy | |||||
IMV* | 48.5 | 48.4 | 47.5 | 46.5 | 42.1 |
NIV* | 3.8 | 5.3 | 6.9 | 9.4 | 10.1 |
IMV or NIV | 50.9 | 51.7 | 52.1 | 52.9 | 49.7 |
Disposition | |||||
Home/home healthcare* | 42.1 | 43.8 | 42.8 | 43.4 | 45.7 |
Transfer to acute care | 5.2 | 4.7 | 4.6 | 4.6 | 4.4 |
Nursing facility* | 24.4 | 24.9 | 27.4 | 28.6 | 29.0 |
Other | 0.7 | 0.8 | 0.9 | 0.9 | 1.0 |
Adjusted mortality, % (SE)* | 27.6 (0.3) | 26.4 (0.4) | 24.9 (0.4) | 22.7 (0.4) | 20.6 (0.3) |
Adjusted mean, LOS/case, d (SE)* | 7.8 (0.1) | 7.9 (0.1) | 7.7 (0.1) | 7.5 (0.1) | 7.1 (0.1) |
Adjusted mean cost/case, 2009 US$, (SE) | 15,818 (251) | 16,981 (419) | 17,236 (411) | 16,941 (436) | 15,987 (402) |
After adjusting for age and sex, the population incidence of ARF increased from 502 (standard error [SE] = 10) cases per 100,000 in 2001 to 784 (SE = 19) cases per 100,000 in 2009 (a 56% increase, P < 0.0001). Hispanics had the lowest rates of ARF, with both black and white groups having similar rates (Table 2).
2001 | 2003 | 2005 | 2007 | 2009 | |
---|---|---|---|---|---|
| |||||
All* | 502 (10) | 569 (12) | 595 (14) | 627 (14) | 784 (19) |
Age group | |||||
1844* | 107 (3) | 130 (4) | 137 (4) | 153 (5) | 189 (6) |
4564* | 422 (9) | 500 (12) | 521 (13) | 580 (14) | 739 (19) |
6584* | 1697 (35) | 1863 (42) | 1950 (50) | 2066 (46) | 2578 (69) |
85+ | 3449 (86) | 3792 (106) | 3981 (120) | 3429 (97) | 4163 (123) |
Sex | |||||
Male* | 491 (10) | 553 (13) | 582 (14) | 629 (14) | 782 (20) |
Female* | 512 (10) | 583 (12) | 607 (15) | 625 (13) | 786 (19) |
Race/ethnicity | |||||
White* | 398 (11) | 427 (12) | 466 (16) | 450 (13) | 699 (21) |
Black* | 423 (27) | 513 (33) | 432 (26) | 574 (38) | 738 (37) |
Hispanic* | 247 (24) | 381 (42) | 307 (27) | 353 (34) | 478 (42) |
Other* | 268 (20) | 342 (29) | 347 (26) | 424 (29) | 713 (77) |
In‐hospital mortality | 140 (3) | 148 (3) | 146 (3) | 140 (3) | 154 (4) |
The most common etiologies of ARF among medical patients were pneumonia, CHF, ARDS, COPD exacerbation, and sepsis. Over the 9‐year study, the proportion of cases secondary to pneumonia and sepsis rose significantly: from 39% to 46% and 13% to 21%, respectively (Figure 1).
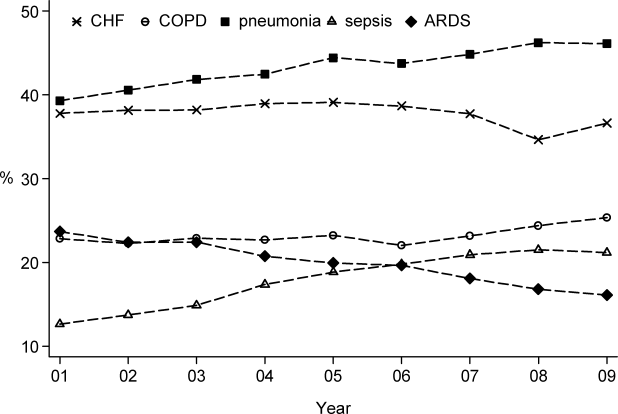
Mortality and Other Outcomes
The number of in‐hospital deaths related to ARF increased from 277,407 deaths in 2001 to 381,155 in 2009 (a 37% increase, P < 0.001). Standardized to the population, deaths increased from 140 in 2001 to 154 cases per 100,000 in 2009 (a 10% increase, P = 0.027). Despite slightly increasing mortality rates at a population level, adjusted in‐hospital mortality improved from 27.6% in 2001 to 20.6% in 2009 (P < 0.001). Mortality declined for both IMV and NIV patients from 35.3% in 2001 to 30.2% in 2009 and from 23.5% to 19%, respectively, but increased for those who required both NIV and IMV (from 26.9% in 2001 to 28% in 2009).
Adjusted hospital length of stay decreased from 7.8 days per patient in 2001 to 7.1 days in 2009 (P < 0.001), with a concomitant increase in discharges to nursing facilities, from 24% in 2001 to 29% in 2009. There was no linear trend in adjusted cost per case, with $15,818 in 2001 and $15,987 in 2009 (in 2009 US dollars) (Table 1).
Ventilation Practices
Overall, 50.9% patients received ventilatory support (NIV or IMV or both) in 2001 and 49.7% in 2009 (P= 0.25). The use of NIV increased from 3.8% to 10.1% (P < 0.001), a 169% increase, whereas the utilization of IMV decreased from 48.5% in 2001 to 42.1% in 2009 (P for trend < 0.0001), a 13% decrease. Uses of both NIV and IMV during hospitalization were seen in 1.4% of cases in 2001 and 2.5% of cases in 2009.
2009 Data Analysis
In 2009 the 1,917,910 hospitalizations with ARF resulted in 381,155 (SD = 8965) deaths and a total inpatient cost of $54 billion. The most common etiologies in patients over 65 years old were pneumonia, CHF, COPD, ARDS, and sepsis. In patients younger than 45 years the most frequent diagnoses were pneumonia, ARDS, sepsis, asthma, drug ingestion, and trauma. Stratified analysis by gender and by age groups showed that mortality rates among men were higher than for women and were highest in patients older than 85 years (Table 3).
Disease | Total | Age <45 Years | 4565 Years | 6584 Years | 85+ Years | Male | Female |
---|---|---|---|---|---|---|---|
| |||||||
Medical | |||||||
Total, N (%) | 1,364,624 (71.2) | 144,715 (10.6) | 416,922 (30.6) | 615,009 (45.1) | 187,977 (13.8) | 647,894 (47.5) | 716,635 (52.5) |
Pneumonia, %* | 46.1 | 41.7 | 42.8 | 46.9 | 54.3 | 48.8 | 43.7 |
CHF, %* | 36.6 | 10.4 | 27.3 | 43.6 | 54.8 | 35.0 | 38.1 |
ARDS, %* | 16.1 | 22.9 | 16.2 | 14.5 | 15.9 | 15.5 | 16.7 |
Sepsis, %* | 21.2 | 18.1 | 21.3 | 21.3 | 23.1 | 22.8 | 19.8 |
COPD, %* | 25.4 | 4.2 | 25.6 | 32.3 | 18.3 | 25.0 | 25.7 |
AMI, %* | 9.0 | 2.6 | 7.1 | 10.5 | 13.3 | 9.3 | 8.8 |
Asthma, %* | 9.2 | 18.1 | 11.6 | 6.7 | 5.4 | 6.2 | 12.0 |
Stroke, %* | 4.8 | 2.3 | 4.1 | 5.5 | 6.0 | 5.0 | 4.7 |
Trauma or burns, %* | 3.4 | 5.4 | 2.9 | 3.0 | 4.1 | 4.3 | 2.5 |
Cardiorespiratory arrest, %* | 4.1 | 3.9 | 4.4 | 4.1 | 3.8 | 4.6 | 3.7 |
Drug, %* | 3.7 | 16.6 | 5.1 | 0.8 | 0.3 | 3.8 | 3.6 |
IMV, %* | 37.7 | 54.6 | 43.7 | 33.5 | 24.8 | 41.1 | 34.5 |
NIV, %* | 11.9 | 7.1 | 11.5 | 13.0 | 12.7 | 11.4 | 12.3 |
In‐hospital mortality (CI) | 22 (21.322.7) | 12.9 (11.913.9) | 18.5 (17.619.4) | 23.9 (23.024.9) | 31.8 (30.633.1) | 24.2 (23.325.1) | 20.9 (20.121.7) |
Surgical | |||||||
Total, N (%) | 552971 (28.8) | 64983 (11.8) | 190225 (34.4) | 254336 (46) | 43426 (7.9) | 295660 (53.5) | 257287 (46.5) |
Pneumonia, %* | 34.9 | 33.0 | 34.0 | 35.0 | 40.5 | 37.1 | 32.2 |
CHF, %* | 27.2 | 8.9 | 21.7 | 33.3 | 42.6 | 26.7 | 27.7 |
ARDS, %* | 45.5 | 51.5 | 45.2 | 44.7 | 42.7 | 45.0 | 46.1 |
Sepsis, %* | 25.1 | 22.8 | 25.4 | 25.2 | 26.1 | 25.4 | 24.7 |
COPD, %* | 8.2 | 1.1 | 7.4 | 10.8 | 7.5 | 8.3 | 8.1 |
AMI, %* | 16.9 | 4.9 | 17.0 | 19.8 | 17.9 | 19.1 | 14.4 |
Asthma, %* | 6.1 | 7.6 | 7.2 | 5.4 | 3.6 | 4.1 | 8.5 |
Stroke, %* | 8.9 | 6.6 | 9.2 | 9.4 | 7.2 | 8.9 | 8.8 |
Trauma or burns, %* | 12.2 | 26.5 | 9.6 | 9.2 | 20.3 | 13.8 | 10.4 |
Cardiorespiratory arrest, %* | 5.5 | 4.4 | 6.0 | 5.4 | 5.2 | 6.1 | 4.7 |
Drug, %* | 0.5 | 1.3 | 0.7 | 0.2 | 0.2 | 0.4 | 0.6 |
IMV, %* | 52.9 | 57.1 | 54.3 | 51.3 | 50.0 | 54.5 | 51.0 |
NIV, %* | 5.8 | 3.5 | 5.5 | 6.4 | 6.4 | 5.6 | 6.0 |
In‐hospital mortality, % (CI) | 18.6 (17.819.5) | 10.7 (9.312.0) | 15.5 (14.216.8) | 20.8 (19.821.9) | 29.4 (27.831.1) | 19.0 (18.219.8) | 18.3 (17.319.2) |
When we examined ventilation practices among medical patients we found that patients older than 85 years, when compared to patients younger than 45 years, were less likely to be treated with IMV (25% vs 55%) and more likely to be treated with NIV (12.7% vs 7%). At the same time, the average cost per case was lowest among patients 85 years and older, and hospital costs per case fell sharply after age 70 years. Costs were considerably higher for those who did not survive during hospitalization, particularly for patients younger than 45 years (Figure 2).
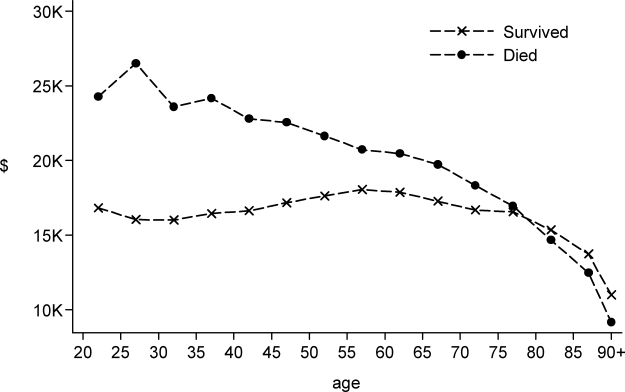
DISCUSSION
In this large population‐based study, we found that the number of hospitalizations associated with a diagnosis of ARF almost doubled over a 9‐year period. In 2009 there were nearly 2 million hospitalizations with ARF in the United States, resulting in approximately 380,000 deaths and inpatient costs of over $54 billion. The population‐adjusted ARF hospitalization rates increased in all age groups, and patients 85 years and older had the highest age‐specific hospitalization rate. Although overall rates of mechanical ventilation (NIV or IMV) remained stable over the 9‐year period, there was an important shift away from IMV (which decreased from 48% in 2001 to 42% in 2009) toward NIV (which increased from 4% in 2001 to 10% in 2009). Overall, there was a significant increase in the number of total deaths despite a decline in adjusted in‐hospital mortality rates. In‐hospital mortality rates decreased for all cases of ARF regardless of ventilation choice.
The findings of this study mirror results of others that have shown that although the incidence of critical care illnesses like sepsis[17, 20, 21, 26] and acute renal failure[27] has increased over the last decade, in‐hospital mortality rates have decreased.[20, 21, 28] Our results also compliment the results of a recent study that looked at hospitalizations for noncardiogenic ARF, which observed a 3.7‐fold increase in the number of cases and a steady decline in case fatality.[11]
Most prior studies addressing the incidence of ARF have included only patients receiving mechanical ventilation. In 1994, the estimated number of cases of ARF requiring IMV was 329,766,[9] which increased to 790,257 in 2005.[10] In our study we found that in 2009, the number of patients with ARF hospitalizations with IMV increased to 806,538. The increase in the overall number of cases with ARF was mainly driven by a surge in cases of sepsis and pneumonia. Our findings are consistent with national trends over time in noncardiogenic ARF[11] and in conditions that predispose patients to ARF such as sepsis[17, 20, 28] and acute renal failure.[27] As the number of claims for ARF doubled and the number of deaths increased, we found that adjusted hospital mortality improved from 27.6% in 2001 to 20.6% in 2009. This decline in hospital mortality was observed among all patients groups, regardless of ventilation choice. The decline in overall case fatality is consistent with prior findings in noncardiogenic ARF,[11] sepsis,[17, 28] and CHF.[29]
There are a number of potential explanations for the reduction in mortality observed over the study period, including improvements in hospital management of the underlying conditions leading to ARF, an increase in the proportion of patients being treated with NIV,[30] and advances in the care of critically ill patients such as the use of low‐tidal volume ventilation.[31, 32] Another contributor may be an increase in the proportion of discharges to nursing facilities, although this change in discharge disposition cannot fully explain our findings. For example, from 2007 to 2009, mortality decreased by 2 percentage points, and nursing home discharges increased by only 0.4 percentage points. Growth and aging of the US population only partially explain the increase we observed in the incidence of ARF, as age‐ and sex‐adjusted population rates increased by 56% from 2001 to 2009. In addition, the NIS captures data on hospital discharges and not individual patients; thus, a patient may have had multiple admissions. Over the last decade adoption of a more intensive practice style has been associated with improved in‐hospital mortality,[33, 34] and although these patients may be living longer they may have multiple readmissions.[35, 36]
We also observed that older patients were less likely to be treated with IMV, had a higher mortality rate, and less expensive care. These results are consistent with other studies and suggest that the intensity of treatment decreases with increasing age, and decisions to withhold or withdraw life‐supporting treatments are more frequent in the elderly.[26, 37] Prior research has shown that severity of illness is more important than age on patients' prognosis,[38, 39] and aggressive treatment strategies are not less cost‐effective when provided to older patients.[40]
Another important finding of this study is the marked increase in the use of NIV paired with a modest reduction in the use of IMV in the treatment of patients with ARF. This finding adds to evidence from other studies, which have similarly reported a dramatic increase in the use of NIV and a decrease in the use of IMV in patients with COPD as well as in ARF of other etiologies.[30, 41]
Our work has several limitations. First, we identified ARF based on ICD‐9‐CM codes and therefore cannot exclude disease misclassification. We did not find any studies in the literature addressing the accuracy and the completeness of ARF coding. However, we employed the same codes used to define ARF as has been used to define organ dysfunction in studies of severe sepsis,[17, 20] and the ICD‐9‐CM codes that we used to identify cases of ARDS have been used in prior studies.[11, 22, 23] Another limitation is that it is not clear to what extent the trends we observed may be due to changes over time in documentation and coding practices. Although this should be considered given the additional reimbursement associated with the diagnosis of ARF, our observation that rates of assisted ventilation have remained almost flat over the 9‐year period of the study suggest that would not wholly account for the rise in ARF. Second, because we did not have access to physiological data such as results of blood gas testing, we could not determine whether the threshold for applying the diagnosis of ARF or for delivering ventilatory support has changed over time. Third, for the purpose of this study we employed a broad definition of ARF, not limiting cases to those requiring mechanical ventilation, and this led to a more heterogeneous cohort including less severe cases of ARF. However, this is not dissimilar to the heterogeneity in disease severity observed among patients who receive a diagnosis of heart failure or acute renal failure. Fourth, survivors of ARF remain at high risk of death in the months after hospitalization,[42] but we assessed only in‐hospital mortality. It is possible that although in‐hospital mortality has improved, 30‐day mortality remained stable. Finally, as the NIS contains only discharge‐level data, we could not distinguish between patients admitted for ARF from those who developed ARF (potentially iatrogenic) after admission.
In summary, over the period of 2001 to 2009, there was a large increase in the number of patients given a diagnosis of ARF and a concomitant reduction in inpatient mortality. Although rates of mechanical ventilation remained relatively constant, there was a significant shift toward greater use of NIV at the expense of IMV.
Disclosures
Dr. Stefan is supported by KM1 CA156726 from the National Cancer Institute (NCI) and by the National Center for Research Resources and the National Center for Advancing Translational Sciences, National Institutes of Health (NIH), through grant UL1 RR025752. The work on this study was supported by a Charlton grant from Tufts University School of Medicine. Dr. Lindenauer and Dr. Pekow are supported by 1R18HL108810‐01 from the National Heart, Lung, and Blood Institute (NHLBI). The content of this publication is solely the responsibility of the authors and does not represent the official views of the NIH, NHLBI, or NCI.
All authors have read and approved the manuscript and none of them have any potential conflicts of interest to report.
Dr. Stefan had full access to all of the data in the study and takes responsibility for the integrity of the data and the accuracy of the data analysis. Conception and design: Mihaela S. Stefan, Penelope S. Pekow, Michael B. Rothberg, Jay Steingrub, Peter K. Lindenauer; analysis and interpretation: Meng‐Shiou Shieh, Mihaela S. Stefan, Penelope S. Pekow, Michael B. Rothberg, Tara Lagu, Peter K. Lindenauer; drafting the manuscript for important intellectual content: Mihaela S. Stefan, Penelope S. Pekow, Michael B. Rothberg, Jay Steingrub, Tara Lagu, and Peter K. Lindenauer.
- Goldman's Cecil Medicine. 24th ed. Amsterdam, the Netherlands: Elsevier Inc.; 2012. , .
- Textbook of Respiratory Medicine. 5th ed. Philadelphia, PA: Saunders; 2010. , .
- Epidemiology and outcome of acute respiratory failure in intensive care unit patients. Crit Care Med. 2003;31(4 suppl):S296–S299. , , .
- Epidemiology of critical care syndromes, organ failures, and life‐support interventions in a suburban US community. Chest. 2011;140(6):1447–1455. , , , et al.
- The changing epidemiology of mechanical ventilation: a population‐based study. J Intensive Care Med. 2006;21(3):173–182. , , , , .
- Mechanical ventilation in Ontario, 1992–2000: incidence, survival, and hospital bed utilization of noncardiac surgery adult patients. Crit Care Med. 2004;32(7):1504–1509. , , , , .
- Contributions to the epidemiology of acute respiratory failure. Crit Care. 2003;7(4):288–290. .
- Incidence, severity, and mortality of acute respiratory failure in Berlin, Germany. Am J Respir Crit Care Med. 1995;151(4):1121–1125. , , , et al.
- Acute respiratory failure in the United States: incidence and 31‐day survival. Chest. 2000;118(4):1100–1105. .
- The epidemiology of mechanical ventilation use in the United States. Crit Care Med. 2010;38(10):1947–1953. , , , , , .
- Trends in the incidence of noncardiogenic acute respiratory failure: the role of race. Crit Care Med. 2012;40(5):1532–1538. , , , .
- Secular trends in nosocomial infections and mortality associated with noninvasive ventilation in patients with exacerbation of COPD and pulmonary edema. JAMA. 2003;290(22):2985–2991. , , , , .
- Association of noninvasive ventilation with nosocomial infections and survival in critically ill patients. JAMA. 2000;284(18):2361–2367. , , , et al.
- Noninvasive versus conventional mechanical ventilation. An epidemiologic survey. Am J Respir Crit Care Med. 2001;163(4):874–880. , , , , .
- Does noninvasive ventilation reduce the ICU nosocomial infection risk? A prospective clinical survey. Intensive Care Med. 1999;25(6):567–573. , , , , , .
- Heathcare Cost and Utilization Project (HCUP). Overview of the Nationwide Inpatient Sample. Available at: http://www.hcup‐us.ahrq.gov/nisoverview.jsp. Accessed December 6, 2011.
- Hospitalizations, costs, and outcomes of severe sepsis in the United States 2003 to 2007. Crit Care Med. 2011;40(3):754–761. , , , , , .
- Association of diagnostic coding with trends in hospitalizations and mortality of patients with pneumonia, 2003–2009. JAMA. 2012;307(13):1405–1413. , , , , .
- Little evidence of correlation between growth in health care spending and reduced mortality. Health Aff (Millwood). 2010;29(8):1523–1531. , , , , .
- The epidemiology of sepsis in the United States from 1979 through 2000. N Engl J Med. 2003;348(16):1546–1554. , , , .
- Rapid increase in hospitalization and mortality rates for severe sepsis in the United States: a trend analysis from 1993 to 2003. Crit Care Med. 2007;35(5):1244–1250. , , , .
- Risk factors for ARDS in the United States: analysis of the 1993 National Mortality Followback Study. Chest. 2001;119(4):1179–1184. , , .
- Acute respiratory distress syndrome: estimated incidence and mortality rate in a 5 million‐person population base. Crit Care. 1998;2(1):29–34. , , , , , .
- Validity of procedure codes in International Classification of Diseases, 9th Revision, Clinical Modification administrative data. Med Care. 2004;42(8):801–809. , , .
- Comorbidity measures for use with administrative data. Med Care. 1998;36(1):8–27. , , , .
- Epidemiology of sepsis: an update. Crit Care Med. 2001;29(7 suppl):S109–S116. , .
- Epidemiology and outcomes of acute renal failure in hospitalized patients: a national survey. Clin J Am Soc Nephrol. 2006;1(1):43–51. , , , , , .
- Facing the challenge: decreasing case fatality rates in severe sepsis despite increasing hospitalizations. Crit Care Med. 2005;33(11):2555–2562. , , , .
- National and regional trends in heart failure hospitalization and mortality rates for Medicare beneficiaries,1998–2008. JAMA. 2011;306(15):1669–1678. , , , .
- Outcomes of noninvasive ventilation for acute exacerbations of chronic obstructive pulmonary disease in the United States, 1998–2008. Am J Respir Crit Care Med. 2011;185(2):152–159. , , , et al.
- A trial of goal‐oriented hemodynamic therapy in critically ill patients. SvO2 Collaborative Group. N Engl J Med. 1995;333(16):1025–1032. , , , et al.
- Ventilation with lower tidal volumes as compared with traditional tidal volumes for acute lung injury. N Engl J Med. 2000;343(11):813; author reply 813–814. , .
- Short‐ and long‐term survival of nonsurgical intensive care patients and its relation to diagnosis, severity of disease, age and comorbidities. Curr Aging Sci. 2009;2(3):240–248. , , .
- The impact of COPD on management and outcomes of patients hospitalized with acute myocardial infarction—a ten‐year retrospective observational study. Chest. 2012;141(6):1441–1448. , , , , , .
- The paradox of health. N Engl J Med. 1988;318(7):414–418. .
- Rehospitalizations among patients in the Medicare fee‐for‐service program. N Engl J Med. 2009;360(14):1418–1428. , , .
- Outcomes and cost‐effectiveness of ventilator support and aggressive care for patients with acute respiratory failure due to pneumonia or acute respiratory distress syndrome. Am J Med. 2000;109(8):614–620. , , , et al.
- Older age, aggressiveness of care, and survival for seriously ill, hospitalized adults. SUPPORT Investigators. Study to Understand Prognoses and Preferences for Outcomes and Risks of Treatments. Ann Intern Med. 1999;131(10):721–728. , , , et al.
- Patient age and decisions to withhold life‐sustaining treatments from seriously ill, hospitalized adults. SUPPORT Investigators. Study to Understand Prognoses and Preferences for Outcomes and Risks of Treatment. Ann Intern Med. 1999;130(2):116–125. , , , et al.
- Are aggressive treatment strategies less cost‐effective for older patients? The case of ventilator support and aggressive care for patients with acute respiratory failure. J Am Geriatr Soc. 2001;49(4):382–390. , , , et al.
- Utilization of non‐invasive ventilation in patients with acute respiratory failure from 2000–2009: a population‐based study. Am J Respir Crit Care Med. 2012;185:A6488. , .
- One‐year outcomes in survivors of the acute respiratory distress syndrome. N Engl J Med. 2003;348(8):683–693. , , , et al.
- Goldman's Cecil Medicine. 24th ed. Amsterdam, the Netherlands: Elsevier Inc.; 2012. , .
- Textbook of Respiratory Medicine. 5th ed. Philadelphia, PA: Saunders; 2010. , .
- Epidemiology and outcome of acute respiratory failure in intensive care unit patients. Crit Care Med. 2003;31(4 suppl):S296–S299. , , .
- Epidemiology of critical care syndromes, organ failures, and life‐support interventions in a suburban US community. Chest. 2011;140(6):1447–1455. , , , et al.
- The changing epidemiology of mechanical ventilation: a population‐based study. J Intensive Care Med. 2006;21(3):173–182. , , , , .
- Mechanical ventilation in Ontario, 1992–2000: incidence, survival, and hospital bed utilization of noncardiac surgery adult patients. Crit Care Med. 2004;32(7):1504–1509. , , , , .
- Contributions to the epidemiology of acute respiratory failure. Crit Care. 2003;7(4):288–290. .
- Incidence, severity, and mortality of acute respiratory failure in Berlin, Germany. Am J Respir Crit Care Med. 1995;151(4):1121–1125. , , , et al.
- Acute respiratory failure in the United States: incidence and 31‐day survival. Chest. 2000;118(4):1100–1105. .
- The epidemiology of mechanical ventilation use in the United States. Crit Care Med. 2010;38(10):1947–1953. , , , , , .
- Trends in the incidence of noncardiogenic acute respiratory failure: the role of race. Crit Care Med. 2012;40(5):1532–1538. , , , .
- Secular trends in nosocomial infections and mortality associated with noninvasive ventilation in patients with exacerbation of COPD and pulmonary edema. JAMA. 2003;290(22):2985–2991. , , , , .
- Association of noninvasive ventilation with nosocomial infections and survival in critically ill patients. JAMA. 2000;284(18):2361–2367. , , , et al.
- Noninvasive versus conventional mechanical ventilation. An epidemiologic survey. Am J Respir Crit Care Med. 2001;163(4):874–880. , , , , .
- Does noninvasive ventilation reduce the ICU nosocomial infection risk? A prospective clinical survey. Intensive Care Med. 1999;25(6):567–573. , , , , , .
- Heathcare Cost and Utilization Project (HCUP). Overview of the Nationwide Inpatient Sample. Available at: http://www.hcup‐us.ahrq.gov/nisoverview.jsp. Accessed December 6, 2011.
- Hospitalizations, costs, and outcomes of severe sepsis in the United States 2003 to 2007. Crit Care Med. 2011;40(3):754–761. , , , , , .
- Association of diagnostic coding with trends in hospitalizations and mortality of patients with pneumonia, 2003–2009. JAMA. 2012;307(13):1405–1413. , , , , .
- Little evidence of correlation between growth in health care spending and reduced mortality. Health Aff (Millwood). 2010;29(8):1523–1531. , , , , .
- The epidemiology of sepsis in the United States from 1979 through 2000. N Engl J Med. 2003;348(16):1546–1554. , , , .
- Rapid increase in hospitalization and mortality rates for severe sepsis in the United States: a trend analysis from 1993 to 2003. Crit Care Med. 2007;35(5):1244–1250. , , , .
- Risk factors for ARDS in the United States: analysis of the 1993 National Mortality Followback Study. Chest. 2001;119(4):1179–1184. , , .
- Acute respiratory distress syndrome: estimated incidence and mortality rate in a 5 million‐person population base. Crit Care. 1998;2(1):29–34. , , , , , .
- Validity of procedure codes in International Classification of Diseases, 9th Revision, Clinical Modification administrative data. Med Care. 2004;42(8):801–809. , , .
- Comorbidity measures for use with administrative data. Med Care. 1998;36(1):8–27. , , , .
- Epidemiology of sepsis: an update. Crit Care Med. 2001;29(7 suppl):S109–S116. , .
- Epidemiology and outcomes of acute renal failure in hospitalized patients: a national survey. Clin J Am Soc Nephrol. 2006;1(1):43–51. , , , , , .
- Facing the challenge: decreasing case fatality rates in severe sepsis despite increasing hospitalizations. Crit Care Med. 2005;33(11):2555–2562. , , , .
- National and regional trends in heart failure hospitalization and mortality rates for Medicare beneficiaries,1998–2008. JAMA. 2011;306(15):1669–1678. , , , .
- Outcomes of noninvasive ventilation for acute exacerbations of chronic obstructive pulmonary disease in the United States, 1998–2008. Am J Respir Crit Care Med. 2011;185(2):152–159. , , , et al.
- A trial of goal‐oriented hemodynamic therapy in critically ill patients. SvO2 Collaborative Group. N Engl J Med. 1995;333(16):1025–1032. , , , et al.
- Ventilation with lower tidal volumes as compared with traditional tidal volumes for acute lung injury. N Engl J Med. 2000;343(11):813; author reply 813–814. , .
- Short‐ and long‐term survival of nonsurgical intensive care patients and its relation to diagnosis, severity of disease, age and comorbidities. Curr Aging Sci. 2009;2(3):240–248. , , .
- The impact of COPD on management and outcomes of patients hospitalized with acute myocardial infarction—a ten‐year retrospective observational study. Chest. 2012;141(6):1441–1448. , , , , , .
- The paradox of health. N Engl J Med. 1988;318(7):414–418. .
- Rehospitalizations among patients in the Medicare fee‐for‐service program. N Engl J Med. 2009;360(14):1418–1428. , , .
- Outcomes and cost‐effectiveness of ventilator support and aggressive care for patients with acute respiratory failure due to pneumonia or acute respiratory distress syndrome. Am J Med. 2000;109(8):614–620. , , , et al.
- Older age, aggressiveness of care, and survival for seriously ill, hospitalized adults. SUPPORT Investigators. Study to Understand Prognoses and Preferences for Outcomes and Risks of Treatments. Ann Intern Med. 1999;131(10):721–728. , , , et al.
- Patient age and decisions to withhold life‐sustaining treatments from seriously ill, hospitalized adults. SUPPORT Investigators. Study to Understand Prognoses and Preferences for Outcomes and Risks of Treatment. Ann Intern Med. 1999;130(2):116–125. , , , et al.
- Are aggressive treatment strategies less cost‐effective for older patients? The case of ventilator support and aggressive care for patients with acute respiratory failure. J Am Geriatr Soc. 2001;49(4):382–390. , , , et al.
- Utilization of non‐invasive ventilation in patients with acute respiratory failure from 2000–2009: a population‐based study. Am J Respir Crit Care Med. 2012;185:A6488. , .
- One‐year outcomes in survivors of the acute respiratory distress syndrome. N Engl J Med. 2003;348(8):683–693. , , , et al.
Copyright © 2012 Society of Hospital Medicine