User login
Rehospitalization after Pneumonia
Pneumonia remains a significant problem in the United States, both in terms of mortality as well as cost to the healthcare system. Each year, over 1 million patients are hospitalized for pneumonia, with costs conservatively estimated at more than $7 billion in 2010.[1] One contributing factor for these costs is that pneumonia is the second most frequent reason for rehospitalization.[2] Rehospitalization after admission for pneumonia is now used as a marker for quality of care.
Given the cost and adverse outcomes of pneumonia, it is important to examine early rehospitalization to better understand what factors play a role. Studies have examined factors associated with readmission following an initial pneumonia‐related hospitalization. Adamuz et al. showed that additional healthcare visits and rehospitalizations within 30 days of discharge from the hospital were common and were associated with worsening of signs or symptoms of pneumonia and/or comorbidities.[3] Other studies demonstrated that instability on hospital discharge, as well as treatment failure, were associated with increased risk of rehospitalization.[4, 5] Jasti et al. found rehospitalizations following pneumonia were usually comorbidity related, primarily cardiopulmonary and/or neurologic disease, rather than due to the index pneumonia.[6] Many of these studies, and others looking at age, activity of daily living score, socioeconomic status, and comorbidity characteristics, were performed in relatively small cohorts. Predictors of rehospitalization have not been studied in a large cohort of patients in an integrated healthcare system.
Our study looks at factors not addressed in prior studies that have used administrative claims data to identify factors associated with early readmission. We also evaluated these admission risk factors in a veteran population, whereas prior studies have primarily focused on those who receive Medicare. The purpose of this study was to examine predictors of early (30 days) readmission in the Veterans Affairs (VA) healthcare system for patients age 65 years and older hospitalized for pneumonia. Our a priori hypothesis was that comorbid illnesses, such as congestive heart failure and chronic obstructive pulmonary disease, and patients with high medical complexity, such as high number of medications and/or prior hospitalizations and nursing home residence, are the primary factors associated with increased risk of rehospitalization.
METHODS
For this national cohort study, we used data from the VA healthcare system administrative and clinical databases that serve as repositories of clinical data from more than 150 VA hospitals and 850 outpatient clinics throughout the United States. The institutional review boards of the University of Texas Health Science Center at San Antonio and VA North Texas Health Care System approved this study. Details regarding the study design and methods were previously published.[7]
Inclusion Criteria
Patients included in this study were hospitalized between October 2001 and September 2007, had a primary diagnosis of pneumonia/emnfluenza (International Classification of Diseases, 9th Revision [ICD‐9] codes 480.0483.99 or 485487) or a secondary discharge diagnosis of pneumonia with a primary diagnosis of respiratory failure (ICD‐9 code 518.81) or sepsis (ICD‐9 code 038.xx),[8] and were aged 65 years or older on the date of admission. To ensure patients obtained their care primarily at the VA, at least 1 VA outpatient clinic visit in the year preceding the index admission and at least 1 active and filled outpatient medication from a VA pharmacy within 90 days of admission were required for inclusion.
If a patient was admitted more than once during the study period, only the first hospitalization was included as the index admission.
Exclusion Criteria
Patients who died during the initial hospitalization were not included in this study. Patients admitted to hospitals with fewer than 25 reported hospitalizations during October 2001 to September 2007 were excluded, as these hospitals are neither representative nor generalizable. This resulted in the removal of 59 patients from 5 different hospitals.
Data Sources and Definitions
We used inpatient and outpatient demographic, utilization, and comorbidity data from the National Patient Care Database. Pharmacy data were extracted from the Decision Support System National Data Extracts and Pharmacy Benefits Management. Vital status information was obtained from the Vital Status file, which incorporates data from veterans' death benefits claims, inpatient deaths, Medicare Vital Status files, and the Social Security Administration's death master file. We used encrypted patient identifiers link to information across these databases.
We obtained demographic information (age, sex, race, marital status) from inpatient and outpatient data. We categorized race as white, black, Hispanic, and other/unknown. To infer active smoking and/or tobacco cessation attempts, we identified ICD‐9 codes for tobacco use (305.1, V15.82), smoking cessation clinic use, and/or use of medications for the treatment of nicotine dependence (Zyban, nicotine replacement, or varenicline). We used VA priority status as a proxy for socioeconomic and disability status. VA priority groups are a way for the VA to focus limited funds to those veterans most in need. The highest group (priority group 1) must have at least a 50% service‐connected disability. Priority groups 2 through 6 include veterans with up to 40% service‐connected disability, former prisoners of war, those awarded certain honors, veterans with lower incomes, and the catastrophically disabled. The lowest groups (priority groups 7 and 8) include veterans with relatively higher incomes who agree to pay copayments.[9]
We also obtained information on comorbid conditions from inpatient and outpatient administrative data. We defined alcohol abuse using ICD‐9 codes 291, 303, 305.0, and illicit drug use with ICD‐9 codes 292, 304, 305 excluding 305.0‐.1. We used the Charlson Comorbidity Index to quantify levels of preexisting comorbidity[10] adapted for administrative databases, using ICD‐9 codes for 19 comorbid conditions from prior outpatient and inpatient encounters. We defined cardiovascular events and lung cancer that were diagnosed during the hospitalization as previously described.[11, 12]
Outcomes
Our primary study outcome was readmission within 30 days of hospital discharge for pneumonia from any VA acute care hospital only. Medicare has used 30‐day readmission as a quality indicator, as readmissions that occur closer to discharge are believed to be more likely due to events during the index hospitalization.[13]
Statistical Analyses
We randomly divided patients from our initial cohort into equal derivation or validation cohorts. We assessed differences between the 2 groups using the Student t test for continuous variables and 2 test for categorical variables. We performed univariate logistic regression analyses in the derivation cohort to examine the relationship between 30‐day rehospitalization and each of our potential covariates. We entered covariates that were significant at P<0.10 in the univariate analyses into a multiple regression model. Significant covariates at P<0.10 were then entered into the final model. For this model, we used bootstrapping with replacement in 1000 replications to obtain standard errors of our coefficients and associated P values. Because some subjects had more than 1 index admission, we used robust variancecovariance matrix estimators to compute standard errors for model coefficients.
We used the C statistic to assess the discrimination of our model. Calibration of this model was measured using the Hosmer‐Lemeshow 2 goodness of fit test, using 10 quantiles to group the data. We evaluated differences in discrimination between the derivation and validation cohorts by comparing C statistics.
Last, using the final model in the derivation cohort, 5 categories of severity were created based on subjects' predicted risk values for readmission. Severity categories in both the derivation and validation cohorts were then graphically evaluated.
We defined statistical significance as a 2‐tailed P value of <0.05. We used Stata 12 (StataCorp, College Station, TX) for all analyses.
RESULTS
Patient Characteristics
Of the 45,134 eligible patients, 13.2% were rehospitalized within 30 days, and rates by hospital ranged from 1.6% to 20.7%. Table 1 presents the comparison of baseline difference between the derivation and validation cohorts. There were no statistically significant differences between the 2 groups. Overall, the mean age was 77 years, 98% were male, and 54% were married. Over 90% of the patients received guideline‐concordant antibiotics, and only 10% required a stay in the intensive care unit (ICU). The average length of stay was 7 days (standard deviation of 11 days).
Characteristics | Derivation Cohort, N=22,567 | Validation Cohort, N=22,567 | P Value |
---|---|---|---|
| |||
Sociodemographics | |||
Age, mean (SD) | 77.3 (6.5) | 77.2 (6.5) | 0.30 |
Male, no (%) | 22,133 (98.1) | 22,175 (98.3) | 0.14 |
White, no (%) | 18,858 (83.6) | 18,719 (83.0) | 0.08 |
Black, no (%) | 2,562 (11.4) | 2,583 (11.5) | 0.76 |
Hispanic, no (%) | 1,337 (5.9) | 1,414 (6.3) | 0.13 |
Married, no (%) | 12,166 (53.9) | 12,258 (54.3) | 0.39 |
VA priority group, no (%) | 0.28 | ||
1 | 4,286 (19.0) | 4,281 (19.0) | |
26 | 16,306 (72.3) | 16,399 (72.8) | |
78 | 1,954 (8.7) | 1,860 (8.3) | |
Nursing home residence, no (%) | 199 (0.9) | 216 (1.0) | 0.41 |
Smoker status, no (%) | 8,579 (38.0) | 8,677 (38.5) | 0.34 |
Index hospitalization factors | |||
Myocardial infarction during hospitalization, no (%) | 469 (2.1) | 452 (2.0) | 0.57 |
Heart failure during hospitalization, no (%) | 4,817 (21.4) | 4,772 (21.2) | 0.61 |
Arrhythmia during hospitalization, no (%) | 4,356 (19.3) | 4,287 (19.0) | 0.41 |
Length of stay, mean (SD) | 7.2 (10.4) | 7.2 (11.7) | 0.76 |
Lung cancer diagnosis during hospitalization, no (%) | 31 (0.1) | 31 (0.1) | 1.00 |
ICU admission, no (%) | 2,282 (10.1) | 2,336 (10.4) | 0.40 |
Guideline concordant antibiotics, no (%) | 20,724 (91.8) | 20,643 (91.5) | 0.17 |
Invasive mechanical ventilation, no (%) | 793 (3.5) | 832 (3.7) | 0.32 |
Vasopressor use, no (%) | 495 (2.2) | 489 (2.2) | 0.85 |
Hospital complications, no (%) | |||
Renal organ failure | 2,671 (11.8) | 2,640 (11.7) | 0.65 |
Cardiac organ failure | 1,004 (4.5) | 959 (4.3) | 0.30 |
Hepatic organ failure | 33 (0.2) | 40 (0.2) | 0.41 |
Respiratory organ failure | 1,623 (7.2) | 1,583 (7.0) | 0.46 |
Comorbid illnesses | |||
Medical, no (%) | |||
Myocardial infarction | 1,528 (6.8) | 1,542 (6.8) | 0.79 |
Congestive heart failure | 5,815 (25.8) | 5,697 (25.2) | 0.20 |
Peripheral vascular disease | 3,413 (15.1) | 3,454 (15.3) | 0.59 |
Cerebrovascular disease | 3,993 (17.7) | 4,078 (18.1) | 0.30 |
Dementia | 1,141 (5.1) | 1,110 (4.9) | 0.50 |
COPD | 12,168 (53.9) | 12,076 (53.5) | 0.39 |
Rheumatologic disease | 646 (2.9) | 660 (2.9) | 0.69 |
Peptic ulcer disease | 795 (3.5) | 723 (3.2) | 0.06 |
Severe liver disease | 169 (0.8) | 169 (0.8) | 1.00 |
Mild liver disease | 78 (0.4) | 80 (0.4) | 0.87 |
Diabetes | 7,310 (32.4) | 7,410 (32.8) | 0.32 |
Diabetes with complications | 2,229 (9.9) | 2,248 (10.0) | 0.77 |
Chronic renal disease | 2,836 (12.6) | 2,745 (12.2) | 0.19 |
Hemi/paraplegia | 319 (1.4) | 338 (1.5) | 0.46 |
Any prior malignancy | 5,226 (23.2) | 5,269 (23.4) | 0.63 |
Metastatic solid tumor | 748 (3.3) | 795 (3.5) | 0.22 |
AIDS | 56 (0.3) | 49 (0.2) | 0.49 |
HIV | 23 (0.1) | 13 (0.1) | 0.10 |
Alcohol abuse | 897 (4.0) | 934 (4.1) | 0.38 |
Drug abuse | 254 (1.1) | 255 (1.1) | 0.96 |
Psychiatric, no (%) | |||
Anxiety disorder | 1,692 (7.5) | 1,722 (7.6) | 0.59 |
Depression indicator | 3,655 (16.2) | 3,718 (16.5) | 0.42 |
Bipolar disorder | 430 (1.9) | 433 (1.9) | 0.92 |
Cataract indicator | 6,106 (27.1) | 6,180 (27.4) | 0.43 |
Prostatitis indicator | 4,680 (20.7) | 4,593 (20.4) | 0.31 |
Schizophrenia indicator | 685 (3.0) | 676 (3.0) | 0.80 |
Post‐traumatic stress disorder | 844 (3.7) | 862 (3.8) | 0.66 |
Medication history within 90 days | |||
Cardiovascular drugs, mean (SD) | 1.8 (1.6) | 1.8 (1.6) | 0.65 |
Diabetes drugs, mean (SD) | 0.3 (0.7) | 0.3 (0.7) | 0.60 |
Inhaled corticosteroids, mean (SD) | 0.4 (0.9) | 0.4 (0.9) | 0.28 |
Pulmonary drug, mean (SD) | 1.3 (2.0) | 1.3 (2.0) | 0.36 |
Oral corticosteroids, no (%) | 5,363 (23.8) | 5,505 (24.4) | 0.12 |
Prior medical utilization | |||
Number of primary care clinic visits within 1 year, mean (SD) | 4.9 (4.2) | 4.9 (4.2) | 0.67 |
Number of emergency department visits within 1 year, mean (SD) | 1.2 (2.0) | 1.2 (2.0) | 0.86 |
Number of outpatient clinic visits within 1 year, mean (SD) | 14.7 (13.1) | 14.6 (13.0) | 0.33 |
Number of specialty clinic visits within 1 year, mean (SD) | 3.4 (5.5) | 3.3 (5.4) | 0.50 |
Prior hospital admission within 90 days, no (%) | 5,062 (22.4) | 5,141 (22.8) | 0.37 |
Predictors of 30‐Day Readmission
All variables listed in Table 1 were evaluated. The derivation logistic regression model is shown in Table 2, and only includes predictors associated with rehospitalization. Antibiotic concordance and time in the ICU were evaluated and did not predict readmission. Variables that were significantly associated with rehospitalization in the models included age, marital status, the number of emergency department clinic visits a year prior, prior admission within 90 days, number of nonpharmacy clinic visits in a year prior, and hospital length of stay. The increasing age and number of emergency department and clinic visits were associated with higher odds of readmission. The longer the length of stay and those patients who were married had a lower odds of subsequent readmission. Other variables included were the presence of chronic renal disease, prior malignancy, nursing home residence, congestive heart failure, and prior use of oral corticosteroids. The C statistics for the derivation and validation models were 0.615 and 0.613, respectively. There were no significant differences in any of the variables between the derivation and validation cohorts.
Predictors | OR | 95% CI | P Value | |
---|---|---|---|---|
Lower | Upper | |||
| ||||
Sociodemographics | ||||
Age at admission | 1.01 | 1.00 | 1.02 | <0.01 |
White | 1.14 | 1.02 | 1.28 | 0.02 |
Nonwhiteb | 1.00 | |||
Hispanic | 1.26 | 1.07 | 1.47 | 0.01 |
Not Hispanicc | 1.00 | |||
Married | 0.89 | 0.82 | 0.96 | <0.01 |
Not marriedd | 1.00 | |||
Nursing home residence | 1.68 | 1.18 | 2.39 | <0.01 |
Comorbid illnesses | ||||
Peptic ulcer disease | 1.24 | 1.02 | 1.50 | 0.03 |
Chronic renal disease | 1.18 | 1.06 | 1.33 | <0.01 |
Any prior malignancy | 1.17 | 1.06 | 1.28 | <0.01 |
Metastatic solid tumor | 1.20 | 0.98 | 1.47 | 0.08 |
AIDS | 1.99 | 1.06 | 3.74 | 0.03 |
Index admission factors | ||||
Heart failure on initial admission | 1.18 | 1.07 | 1.29 | <0.01 |
Length of stay (per day) | 0.97 | 0.97 | 0.98 | <0.01 |
Medication history | ||||
Cardiovascular drug count 90 days prior | 1.03 | 1.00 | 1.06 | 0.02 |
Oral corticosteroids 90 days prior | 1.16 | 1.06 | 1.27 | <0.01 |
Prior medical utilization | ||||
Number of emergency department visits within 1 year | 1.04 | 1.02 | 1.06 | <0.01 |
Number of specialty clinic visits within 1 year | 1.01 | 1.00 | 1.02 | 0.01 |
Prior hospital admission within 90 days | 1.60 | 1.46 | 1.75 | <0.01 |
Number of nonpharmacy clinic visits within 1 year | 1.00 | 1.00 | 1.01 | <0.01 |
Figure 1 shows that the derivation and validation models are similar in percent rehospitalization group in all severity groups.
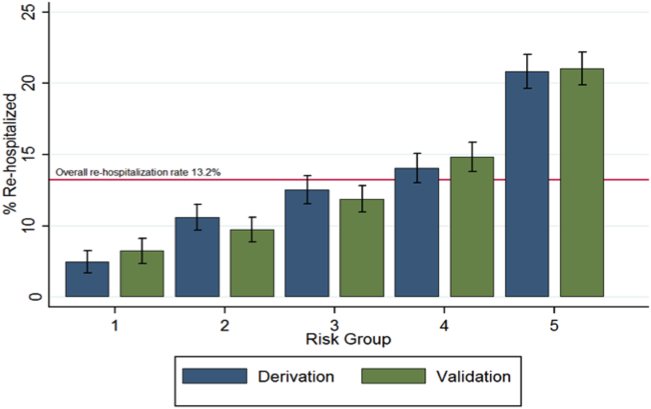
DISCUSSION
Sociodemographics and comorbidities, which are host factors, were significantly associated with rehospitalization of elderly patients admitted for pneumonia and length of hospital stay, the only pneumonia‐specific variable associated with readmission.[6, 14, 15, 16] Higher rates of all types of prior utilization (inpatient, outpatient, emergency department visits) were also associated with higher risk of rehospitalization. Our findings highlight the role that host factors play in rehospitalization of elderly patients with pneumonia and that there are few potentially modifiable targets to help reduce readmissions after pneumonia.
These findings may indicate 2 things: patients in this cohort are sicker than the general population at baseline and therefore require more medical care, and/or they are being evaluated by more providers increasing the chance they will be readmitted. For the former, an example is the association of prior use of oral corticosteroids with rehospitalization, which may demonstrate poorly controlled comorbidities (eg, chronic obstructive lung disease with recent exacerbation) that require steroid use. Given the VA's integrated healthcare system, greater access to primary care providers may lead to more opportunities for patients to voice their complaints, which can lead to more readmissions among severely ill patients. Weinberger et al. found an increase in hospital readmissions with increased access to primary care.[17] The association of readmission and length of stay is less likely related to pneumonia‐related clinical instabilities on discharge, but rather to the ability to address other potential issues related to the patient's underlying comorbidities.[5]
Our study presents a model looking at rehospitalization of patients with pneumonia, including factors not addressed in a prior publication by Lindenauer et al., the model Centers for Medicare and Medicaid Services (CMS) uses to predict early rehospitalization.[18] Lindenauer's model included 39 variables (of which 37 were clinical variables), including vertebral fractures, other injuries, other gastrointestinal disorders, other urinary tract disorders, as well as demographic and comorbidity factors that were similar to some of those included in our study. Their 30‐day rehospitalization medical record and administrative models had a C statistic of 0.59 and 0.63, respectively. A major difference between our presented model and the latter are the data on utilization of medical care. Despite these additional data, the resulting C statistics of 0.615 and 0.613 were not qualitatively better.
The similar C statistics demonstrates that factors playing a significant role in determining rehospitalization have yet to be identified, and it is not clear if researchers will be able to identify potentially modifiable risk factors for rehospitalization. Examples of other potential factors that should be examined in future studies include family/community support, quality of the transition of care back to their residence, and quality of inpatient care. Calvillo‐King et al. found a broad range of social factors that potentially affect the risk of postdischarge readmission for chronic heart failure.[19] For pneumonia, there were few studies that assessed the risk of social factors on readmission, and these were most commonly rather broad categories such as race/ethnicity or nursing home residence. In the publications that studied social factors and readmissions, there were inconsistent associations between hospital readmission with lower education, low income, and unemployment. Future articles may benefit from taking these additional factors into account.
Potential limitations to our study include the overwhelming majority of patients being male and in a VA population, which may limit generalizability. In addition, no data were available on the quality of the transition of care. Furthermore, limitations include the age of the data and the lack of evaluation of outpatient follow‐up and antibiotics on discharge. Though our data are up to 10 years old, this study evaluates risk factors that are still relevant. Finally, some variables noted in the CMS model were not evaluated in our study, such as protein‐calorie malnutrition, skin ulcers, and fracture history. Given these differences, we were unable to directly compare the performance of the 2 models.
Despite examining novel factors related to prior healthcare utilization, our model still performed suboptimally in identifying subjects at risk for rehospitalization after pneumonia. Future studies need to examine other social factors and patient frailty that may increase the risk of readmissions, as well as examine the impact of clinical stability on discharge. It remains to be determined if readmissions after pneumonia are truly preventable or mostly attributable to the patient's prior health status.
Disclosures: The project described was supported by grant number R01NR010828 from the National Institute of Nursing Research. The content is solely the responsibility of the authors and does not necessarily represent the official views of the National Institute of Nursing Research or the National Institutes of Health. This material is the result of work supported with resources and the use of facilities at the VA North Texas Health Care System. Funding agencies had no role in conducting the study, or role in the preparation, review, or approval of the manuscript. The views expressed in this article are those of the authors and do not necessarily represent the views of the Department of Veterans Affairs.
- Incidence and cost of pneumonia in Medicare beneficiaries. Chest. 2012;142(4):973–981. , , , et al.
- Rehospitalizations among patients in the Medicare fee‐for‐service program. N Engl J Med. 2009;360(14):1418–1428. , , .
- A prospective cohort study of healthcare visits and rehospitalizations after discharge of patients with community‐acquired pneumonia. Respirology. 2011;16(7):1119–1126. , , , et al.
- Pneumonia: criteria for patient instability on hospital discharge. Chest. 2008;134(3):595–600. , , , et al.
- Instability on hospital discharge and the risk of adverse outcomes in patients with pneumonia. Arch Intern Med. 2002;162(11):1278–1284. , , , et al.
- Causes and risk factors for rehospitalization of patients hospitalized with community‐acquired pneumonia. Clin Infect Dis. 2008;46(4):550–556. , , , et al.
- Population‐based study of statins, angiotensin II receptor blockers, and angiotensin‐converting enzyme inhibitors on pneumonia‐related outcomes. Clin Infect Dis. 2012;55(11):1466–1473. , , , et al.
- Quality of care, process, and outcomes in elderly patients with pneumonia. JAMA. 1997;278(23):2080–2084. , , , et al.
- U.S. Department of Veterans Affairs. Health benefits. Priority groups table. Available at: http://www.va.gov/healthbenefits/resources/priority_groups.asp. Accessed July 1, 2013.
- Validation of a combined comorbidity index. J Clin Epidemiol. 1994;47(11):1245–1251. , , , et al.
- Incidence of cardiovascular events after hospital admission for pneumonia. Am J Med. 2011;124(3):244–251. , , , et al.
- Diagnosis of pulmonary malignancy after hospitalization for pneumonia. Am J Med. 2010;123(1):66–71. , , , et al.
- MedPAC. 2013 [cited 2013 June 11]; Available from: http://www.hospitalcompare.gov.
- The relationship between hospital admission rates and rehospitalizations. N Engl J Med. 2011;365(24):2287–2295. , , .
- Predictors of short‐term rehospitalization following discharge of patients hospitalized with community‐acquired pneumonia. Chest. 2009;136(4):1079–1085. , , , et al.
- Effects of previous influenza vaccination on subsequent readmission and mortality in elderly patients hospitalized with pneumonia. Am J Med. 2003;115(6):454–461. , , , et al.
- Does increased access to primary care reduce hospital readmissions? N Engl J Med. 1996;334(22):1441–1447. , , .
- Development, validation, and results of a measure of 30‐day readmission following hospitalization for pneumonia. J Hosp Med. 2011;6(3):142–150. , , , et al.
- Impact of social factors on risk of readmission or mortality in pneumonia and heart failure: systematic review. J Gen Intern Med. 2013;28(2):269–282. , , , et al.
Pneumonia remains a significant problem in the United States, both in terms of mortality as well as cost to the healthcare system. Each year, over 1 million patients are hospitalized for pneumonia, with costs conservatively estimated at more than $7 billion in 2010.[1] One contributing factor for these costs is that pneumonia is the second most frequent reason for rehospitalization.[2] Rehospitalization after admission for pneumonia is now used as a marker for quality of care.
Given the cost and adverse outcomes of pneumonia, it is important to examine early rehospitalization to better understand what factors play a role. Studies have examined factors associated with readmission following an initial pneumonia‐related hospitalization. Adamuz et al. showed that additional healthcare visits and rehospitalizations within 30 days of discharge from the hospital were common and were associated with worsening of signs or symptoms of pneumonia and/or comorbidities.[3] Other studies demonstrated that instability on hospital discharge, as well as treatment failure, were associated with increased risk of rehospitalization.[4, 5] Jasti et al. found rehospitalizations following pneumonia were usually comorbidity related, primarily cardiopulmonary and/or neurologic disease, rather than due to the index pneumonia.[6] Many of these studies, and others looking at age, activity of daily living score, socioeconomic status, and comorbidity characteristics, were performed in relatively small cohorts. Predictors of rehospitalization have not been studied in a large cohort of patients in an integrated healthcare system.
Our study looks at factors not addressed in prior studies that have used administrative claims data to identify factors associated with early readmission. We also evaluated these admission risk factors in a veteran population, whereas prior studies have primarily focused on those who receive Medicare. The purpose of this study was to examine predictors of early (30 days) readmission in the Veterans Affairs (VA) healthcare system for patients age 65 years and older hospitalized for pneumonia. Our a priori hypothesis was that comorbid illnesses, such as congestive heart failure and chronic obstructive pulmonary disease, and patients with high medical complexity, such as high number of medications and/or prior hospitalizations and nursing home residence, are the primary factors associated with increased risk of rehospitalization.
METHODS
For this national cohort study, we used data from the VA healthcare system administrative and clinical databases that serve as repositories of clinical data from more than 150 VA hospitals and 850 outpatient clinics throughout the United States. The institutional review boards of the University of Texas Health Science Center at San Antonio and VA North Texas Health Care System approved this study. Details regarding the study design and methods were previously published.[7]
Inclusion Criteria
Patients included in this study were hospitalized between October 2001 and September 2007, had a primary diagnosis of pneumonia/emnfluenza (International Classification of Diseases, 9th Revision [ICD‐9] codes 480.0483.99 or 485487) or a secondary discharge diagnosis of pneumonia with a primary diagnosis of respiratory failure (ICD‐9 code 518.81) or sepsis (ICD‐9 code 038.xx),[8] and were aged 65 years or older on the date of admission. To ensure patients obtained their care primarily at the VA, at least 1 VA outpatient clinic visit in the year preceding the index admission and at least 1 active and filled outpatient medication from a VA pharmacy within 90 days of admission were required for inclusion.
If a patient was admitted more than once during the study period, only the first hospitalization was included as the index admission.
Exclusion Criteria
Patients who died during the initial hospitalization were not included in this study. Patients admitted to hospitals with fewer than 25 reported hospitalizations during October 2001 to September 2007 were excluded, as these hospitals are neither representative nor generalizable. This resulted in the removal of 59 patients from 5 different hospitals.
Data Sources and Definitions
We used inpatient and outpatient demographic, utilization, and comorbidity data from the National Patient Care Database. Pharmacy data were extracted from the Decision Support System National Data Extracts and Pharmacy Benefits Management. Vital status information was obtained from the Vital Status file, which incorporates data from veterans' death benefits claims, inpatient deaths, Medicare Vital Status files, and the Social Security Administration's death master file. We used encrypted patient identifiers link to information across these databases.
We obtained demographic information (age, sex, race, marital status) from inpatient and outpatient data. We categorized race as white, black, Hispanic, and other/unknown. To infer active smoking and/or tobacco cessation attempts, we identified ICD‐9 codes for tobacco use (305.1, V15.82), smoking cessation clinic use, and/or use of medications for the treatment of nicotine dependence (Zyban, nicotine replacement, or varenicline). We used VA priority status as a proxy for socioeconomic and disability status. VA priority groups are a way for the VA to focus limited funds to those veterans most in need. The highest group (priority group 1) must have at least a 50% service‐connected disability. Priority groups 2 through 6 include veterans with up to 40% service‐connected disability, former prisoners of war, those awarded certain honors, veterans with lower incomes, and the catastrophically disabled. The lowest groups (priority groups 7 and 8) include veterans with relatively higher incomes who agree to pay copayments.[9]
We also obtained information on comorbid conditions from inpatient and outpatient administrative data. We defined alcohol abuse using ICD‐9 codes 291, 303, 305.0, and illicit drug use with ICD‐9 codes 292, 304, 305 excluding 305.0‐.1. We used the Charlson Comorbidity Index to quantify levels of preexisting comorbidity[10] adapted for administrative databases, using ICD‐9 codes for 19 comorbid conditions from prior outpatient and inpatient encounters. We defined cardiovascular events and lung cancer that were diagnosed during the hospitalization as previously described.[11, 12]
Outcomes
Our primary study outcome was readmission within 30 days of hospital discharge for pneumonia from any VA acute care hospital only. Medicare has used 30‐day readmission as a quality indicator, as readmissions that occur closer to discharge are believed to be more likely due to events during the index hospitalization.[13]
Statistical Analyses
We randomly divided patients from our initial cohort into equal derivation or validation cohorts. We assessed differences between the 2 groups using the Student t test for continuous variables and 2 test for categorical variables. We performed univariate logistic regression analyses in the derivation cohort to examine the relationship between 30‐day rehospitalization and each of our potential covariates. We entered covariates that were significant at P<0.10 in the univariate analyses into a multiple regression model. Significant covariates at P<0.10 were then entered into the final model. For this model, we used bootstrapping with replacement in 1000 replications to obtain standard errors of our coefficients and associated P values. Because some subjects had more than 1 index admission, we used robust variancecovariance matrix estimators to compute standard errors for model coefficients.
We used the C statistic to assess the discrimination of our model. Calibration of this model was measured using the Hosmer‐Lemeshow 2 goodness of fit test, using 10 quantiles to group the data. We evaluated differences in discrimination between the derivation and validation cohorts by comparing C statistics.
Last, using the final model in the derivation cohort, 5 categories of severity were created based on subjects' predicted risk values for readmission. Severity categories in both the derivation and validation cohorts were then graphically evaluated.
We defined statistical significance as a 2‐tailed P value of <0.05. We used Stata 12 (StataCorp, College Station, TX) for all analyses.
RESULTS
Patient Characteristics
Of the 45,134 eligible patients, 13.2% were rehospitalized within 30 days, and rates by hospital ranged from 1.6% to 20.7%. Table 1 presents the comparison of baseline difference between the derivation and validation cohorts. There were no statistically significant differences between the 2 groups. Overall, the mean age was 77 years, 98% were male, and 54% were married. Over 90% of the patients received guideline‐concordant antibiotics, and only 10% required a stay in the intensive care unit (ICU). The average length of stay was 7 days (standard deviation of 11 days).
Characteristics | Derivation Cohort, N=22,567 | Validation Cohort, N=22,567 | P Value |
---|---|---|---|
| |||
Sociodemographics | |||
Age, mean (SD) | 77.3 (6.5) | 77.2 (6.5) | 0.30 |
Male, no (%) | 22,133 (98.1) | 22,175 (98.3) | 0.14 |
White, no (%) | 18,858 (83.6) | 18,719 (83.0) | 0.08 |
Black, no (%) | 2,562 (11.4) | 2,583 (11.5) | 0.76 |
Hispanic, no (%) | 1,337 (5.9) | 1,414 (6.3) | 0.13 |
Married, no (%) | 12,166 (53.9) | 12,258 (54.3) | 0.39 |
VA priority group, no (%) | 0.28 | ||
1 | 4,286 (19.0) | 4,281 (19.0) | |
26 | 16,306 (72.3) | 16,399 (72.8) | |
78 | 1,954 (8.7) | 1,860 (8.3) | |
Nursing home residence, no (%) | 199 (0.9) | 216 (1.0) | 0.41 |
Smoker status, no (%) | 8,579 (38.0) | 8,677 (38.5) | 0.34 |
Index hospitalization factors | |||
Myocardial infarction during hospitalization, no (%) | 469 (2.1) | 452 (2.0) | 0.57 |
Heart failure during hospitalization, no (%) | 4,817 (21.4) | 4,772 (21.2) | 0.61 |
Arrhythmia during hospitalization, no (%) | 4,356 (19.3) | 4,287 (19.0) | 0.41 |
Length of stay, mean (SD) | 7.2 (10.4) | 7.2 (11.7) | 0.76 |
Lung cancer diagnosis during hospitalization, no (%) | 31 (0.1) | 31 (0.1) | 1.00 |
ICU admission, no (%) | 2,282 (10.1) | 2,336 (10.4) | 0.40 |
Guideline concordant antibiotics, no (%) | 20,724 (91.8) | 20,643 (91.5) | 0.17 |
Invasive mechanical ventilation, no (%) | 793 (3.5) | 832 (3.7) | 0.32 |
Vasopressor use, no (%) | 495 (2.2) | 489 (2.2) | 0.85 |
Hospital complications, no (%) | |||
Renal organ failure | 2,671 (11.8) | 2,640 (11.7) | 0.65 |
Cardiac organ failure | 1,004 (4.5) | 959 (4.3) | 0.30 |
Hepatic organ failure | 33 (0.2) | 40 (0.2) | 0.41 |
Respiratory organ failure | 1,623 (7.2) | 1,583 (7.0) | 0.46 |
Comorbid illnesses | |||
Medical, no (%) | |||
Myocardial infarction | 1,528 (6.8) | 1,542 (6.8) | 0.79 |
Congestive heart failure | 5,815 (25.8) | 5,697 (25.2) | 0.20 |
Peripheral vascular disease | 3,413 (15.1) | 3,454 (15.3) | 0.59 |
Cerebrovascular disease | 3,993 (17.7) | 4,078 (18.1) | 0.30 |
Dementia | 1,141 (5.1) | 1,110 (4.9) | 0.50 |
COPD | 12,168 (53.9) | 12,076 (53.5) | 0.39 |
Rheumatologic disease | 646 (2.9) | 660 (2.9) | 0.69 |
Peptic ulcer disease | 795 (3.5) | 723 (3.2) | 0.06 |
Severe liver disease | 169 (0.8) | 169 (0.8) | 1.00 |
Mild liver disease | 78 (0.4) | 80 (0.4) | 0.87 |
Diabetes | 7,310 (32.4) | 7,410 (32.8) | 0.32 |
Diabetes with complications | 2,229 (9.9) | 2,248 (10.0) | 0.77 |
Chronic renal disease | 2,836 (12.6) | 2,745 (12.2) | 0.19 |
Hemi/paraplegia | 319 (1.4) | 338 (1.5) | 0.46 |
Any prior malignancy | 5,226 (23.2) | 5,269 (23.4) | 0.63 |
Metastatic solid tumor | 748 (3.3) | 795 (3.5) | 0.22 |
AIDS | 56 (0.3) | 49 (0.2) | 0.49 |
HIV | 23 (0.1) | 13 (0.1) | 0.10 |
Alcohol abuse | 897 (4.0) | 934 (4.1) | 0.38 |
Drug abuse | 254 (1.1) | 255 (1.1) | 0.96 |
Psychiatric, no (%) | |||
Anxiety disorder | 1,692 (7.5) | 1,722 (7.6) | 0.59 |
Depression indicator | 3,655 (16.2) | 3,718 (16.5) | 0.42 |
Bipolar disorder | 430 (1.9) | 433 (1.9) | 0.92 |
Cataract indicator | 6,106 (27.1) | 6,180 (27.4) | 0.43 |
Prostatitis indicator | 4,680 (20.7) | 4,593 (20.4) | 0.31 |
Schizophrenia indicator | 685 (3.0) | 676 (3.0) | 0.80 |
Post‐traumatic stress disorder | 844 (3.7) | 862 (3.8) | 0.66 |
Medication history within 90 days | |||
Cardiovascular drugs, mean (SD) | 1.8 (1.6) | 1.8 (1.6) | 0.65 |
Diabetes drugs, mean (SD) | 0.3 (0.7) | 0.3 (0.7) | 0.60 |
Inhaled corticosteroids, mean (SD) | 0.4 (0.9) | 0.4 (0.9) | 0.28 |
Pulmonary drug, mean (SD) | 1.3 (2.0) | 1.3 (2.0) | 0.36 |
Oral corticosteroids, no (%) | 5,363 (23.8) | 5,505 (24.4) | 0.12 |
Prior medical utilization | |||
Number of primary care clinic visits within 1 year, mean (SD) | 4.9 (4.2) | 4.9 (4.2) | 0.67 |
Number of emergency department visits within 1 year, mean (SD) | 1.2 (2.0) | 1.2 (2.0) | 0.86 |
Number of outpatient clinic visits within 1 year, mean (SD) | 14.7 (13.1) | 14.6 (13.0) | 0.33 |
Number of specialty clinic visits within 1 year, mean (SD) | 3.4 (5.5) | 3.3 (5.4) | 0.50 |
Prior hospital admission within 90 days, no (%) | 5,062 (22.4) | 5,141 (22.8) | 0.37 |
Predictors of 30‐Day Readmission
All variables listed in Table 1 were evaluated. The derivation logistic regression model is shown in Table 2, and only includes predictors associated with rehospitalization. Antibiotic concordance and time in the ICU were evaluated and did not predict readmission. Variables that were significantly associated with rehospitalization in the models included age, marital status, the number of emergency department clinic visits a year prior, prior admission within 90 days, number of nonpharmacy clinic visits in a year prior, and hospital length of stay. The increasing age and number of emergency department and clinic visits were associated with higher odds of readmission. The longer the length of stay and those patients who were married had a lower odds of subsequent readmission. Other variables included were the presence of chronic renal disease, prior malignancy, nursing home residence, congestive heart failure, and prior use of oral corticosteroids. The C statistics for the derivation and validation models were 0.615 and 0.613, respectively. There were no significant differences in any of the variables between the derivation and validation cohorts.
Predictors | OR | 95% CI | P Value | |
---|---|---|---|---|
Lower | Upper | |||
| ||||
Sociodemographics | ||||
Age at admission | 1.01 | 1.00 | 1.02 | <0.01 |
White | 1.14 | 1.02 | 1.28 | 0.02 |
Nonwhiteb | 1.00 | |||
Hispanic | 1.26 | 1.07 | 1.47 | 0.01 |
Not Hispanicc | 1.00 | |||
Married | 0.89 | 0.82 | 0.96 | <0.01 |
Not marriedd | 1.00 | |||
Nursing home residence | 1.68 | 1.18 | 2.39 | <0.01 |
Comorbid illnesses | ||||
Peptic ulcer disease | 1.24 | 1.02 | 1.50 | 0.03 |
Chronic renal disease | 1.18 | 1.06 | 1.33 | <0.01 |
Any prior malignancy | 1.17 | 1.06 | 1.28 | <0.01 |
Metastatic solid tumor | 1.20 | 0.98 | 1.47 | 0.08 |
AIDS | 1.99 | 1.06 | 3.74 | 0.03 |
Index admission factors | ||||
Heart failure on initial admission | 1.18 | 1.07 | 1.29 | <0.01 |
Length of stay (per day) | 0.97 | 0.97 | 0.98 | <0.01 |
Medication history | ||||
Cardiovascular drug count 90 days prior | 1.03 | 1.00 | 1.06 | 0.02 |
Oral corticosteroids 90 days prior | 1.16 | 1.06 | 1.27 | <0.01 |
Prior medical utilization | ||||
Number of emergency department visits within 1 year | 1.04 | 1.02 | 1.06 | <0.01 |
Number of specialty clinic visits within 1 year | 1.01 | 1.00 | 1.02 | 0.01 |
Prior hospital admission within 90 days | 1.60 | 1.46 | 1.75 | <0.01 |
Number of nonpharmacy clinic visits within 1 year | 1.00 | 1.00 | 1.01 | <0.01 |
Figure 1 shows that the derivation and validation models are similar in percent rehospitalization group in all severity groups.
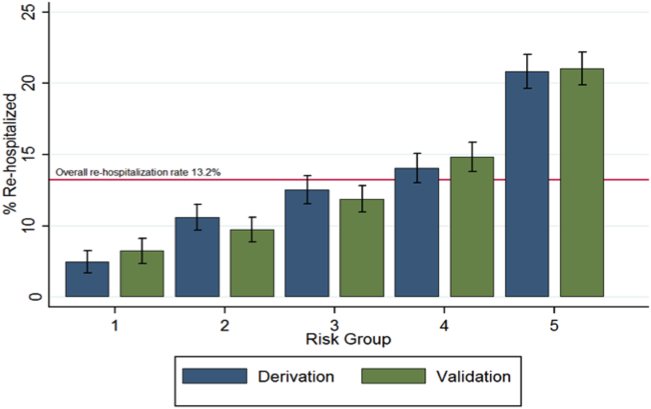
DISCUSSION
Sociodemographics and comorbidities, which are host factors, were significantly associated with rehospitalization of elderly patients admitted for pneumonia and length of hospital stay, the only pneumonia‐specific variable associated with readmission.[6, 14, 15, 16] Higher rates of all types of prior utilization (inpatient, outpatient, emergency department visits) were also associated with higher risk of rehospitalization. Our findings highlight the role that host factors play in rehospitalization of elderly patients with pneumonia and that there are few potentially modifiable targets to help reduce readmissions after pneumonia.
These findings may indicate 2 things: patients in this cohort are sicker than the general population at baseline and therefore require more medical care, and/or they are being evaluated by more providers increasing the chance they will be readmitted. For the former, an example is the association of prior use of oral corticosteroids with rehospitalization, which may demonstrate poorly controlled comorbidities (eg, chronic obstructive lung disease with recent exacerbation) that require steroid use. Given the VA's integrated healthcare system, greater access to primary care providers may lead to more opportunities for patients to voice their complaints, which can lead to more readmissions among severely ill patients. Weinberger et al. found an increase in hospital readmissions with increased access to primary care.[17] The association of readmission and length of stay is less likely related to pneumonia‐related clinical instabilities on discharge, but rather to the ability to address other potential issues related to the patient's underlying comorbidities.[5]
Our study presents a model looking at rehospitalization of patients with pneumonia, including factors not addressed in a prior publication by Lindenauer et al., the model Centers for Medicare and Medicaid Services (CMS) uses to predict early rehospitalization.[18] Lindenauer's model included 39 variables (of which 37 were clinical variables), including vertebral fractures, other injuries, other gastrointestinal disorders, other urinary tract disorders, as well as demographic and comorbidity factors that were similar to some of those included in our study. Their 30‐day rehospitalization medical record and administrative models had a C statistic of 0.59 and 0.63, respectively. A major difference between our presented model and the latter are the data on utilization of medical care. Despite these additional data, the resulting C statistics of 0.615 and 0.613 were not qualitatively better.
The similar C statistics demonstrates that factors playing a significant role in determining rehospitalization have yet to be identified, and it is not clear if researchers will be able to identify potentially modifiable risk factors for rehospitalization. Examples of other potential factors that should be examined in future studies include family/community support, quality of the transition of care back to their residence, and quality of inpatient care. Calvillo‐King et al. found a broad range of social factors that potentially affect the risk of postdischarge readmission for chronic heart failure.[19] For pneumonia, there were few studies that assessed the risk of social factors on readmission, and these were most commonly rather broad categories such as race/ethnicity or nursing home residence. In the publications that studied social factors and readmissions, there were inconsistent associations between hospital readmission with lower education, low income, and unemployment. Future articles may benefit from taking these additional factors into account.
Potential limitations to our study include the overwhelming majority of patients being male and in a VA population, which may limit generalizability. In addition, no data were available on the quality of the transition of care. Furthermore, limitations include the age of the data and the lack of evaluation of outpatient follow‐up and antibiotics on discharge. Though our data are up to 10 years old, this study evaluates risk factors that are still relevant. Finally, some variables noted in the CMS model were not evaluated in our study, such as protein‐calorie malnutrition, skin ulcers, and fracture history. Given these differences, we were unable to directly compare the performance of the 2 models.
Despite examining novel factors related to prior healthcare utilization, our model still performed suboptimally in identifying subjects at risk for rehospitalization after pneumonia. Future studies need to examine other social factors and patient frailty that may increase the risk of readmissions, as well as examine the impact of clinical stability on discharge. It remains to be determined if readmissions after pneumonia are truly preventable or mostly attributable to the patient's prior health status.
Disclosures: The project described was supported by grant number R01NR010828 from the National Institute of Nursing Research. The content is solely the responsibility of the authors and does not necessarily represent the official views of the National Institute of Nursing Research or the National Institutes of Health. This material is the result of work supported with resources and the use of facilities at the VA North Texas Health Care System. Funding agencies had no role in conducting the study, or role in the preparation, review, or approval of the manuscript. The views expressed in this article are those of the authors and do not necessarily represent the views of the Department of Veterans Affairs.
Pneumonia remains a significant problem in the United States, both in terms of mortality as well as cost to the healthcare system. Each year, over 1 million patients are hospitalized for pneumonia, with costs conservatively estimated at more than $7 billion in 2010.[1] One contributing factor for these costs is that pneumonia is the second most frequent reason for rehospitalization.[2] Rehospitalization after admission for pneumonia is now used as a marker for quality of care.
Given the cost and adverse outcomes of pneumonia, it is important to examine early rehospitalization to better understand what factors play a role. Studies have examined factors associated with readmission following an initial pneumonia‐related hospitalization. Adamuz et al. showed that additional healthcare visits and rehospitalizations within 30 days of discharge from the hospital were common and were associated with worsening of signs or symptoms of pneumonia and/or comorbidities.[3] Other studies demonstrated that instability on hospital discharge, as well as treatment failure, were associated with increased risk of rehospitalization.[4, 5] Jasti et al. found rehospitalizations following pneumonia were usually comorbidity related, primarily cardiopulmonary and/or neurologic disease, rather than due to the index pneumonia.[6] Many of these studies, and others looking at age, activity of daily living score, socioeconomic status, and comorbidity characteristics, were performed in relatively small cohorts. Predictors of rehospitalization have not been studied in a large cohort of patients in an integrated healthcare system.
Our study looks at factors not addressed in prior studies that have used administrative claims data to identify factors associated with early readmission. We also evaluated these admission risk factors in a veteran population, whereas prior studies have primarily focused on those who receive Medicare. The purpose of this study was to examine predictors of early (30 days) readmission in the Veterans Affairs (VA) healthcare system for patients age 65 years and older hospitalized for pneumonia. Our a priori hypothesis was that comorbid illnesses, such as congestive heart failure and chronic obstructive pulmonary disease, and patients with high medical complexity, such as high number of medications and/or prior hospitalizations and nursing home residence, are the primary factors associated with increased risk of rehospitalization.
METHODS
For this national cohort study, we used data from the VA healthcare system administrative and clinical databases that serve as repositories of clinical data from more than 150 VA hospitals and 850 outpatient clinics throughout the United States. The institutional review boards of the University of Texas Health Science Center at San Antonio and VA North Texas Health Care System approved this study. Details regarding the study design and methods were previously published.[7]
Inclusion Criteria
Patients included in this study were hospitalized between October 2001 and September 2007, had a primary diagnosis of pneumonia/emnfluenza (International Classification of Diseases, 9th Revision [ICD‐9] codes 480.0483.99 or 485487) or a secondary discharge diagnosis of pneumonia with a primary diagnosis of respiratory failure (ICD‐9 code 518.81) or sepsis (ICD‐9 code 038.xx),[8] and were aged 65 years or older on the date of admission. To ensure patients obtained their care primarily at the VA, at least 1 VA outpatient clinic visit in the year preceding the index admission and at least 1 active and filled outpatient medication from a VA pharmacy within 90 days of admission were required for inclusion.
If a patient was admitted more than once during the study period, only the first hospitalization was included as the index admission.
Exclusion Criteria
Patients who died during the initial hospitalization were not included in this study. Patients admitted to hospitals with fewer than 25 reported hospitalizations during October 2001 to September 2007 were excluded, as these hospitals are neither representative nor generalizable. This resulted in the removal of 59 patients from 5 different hospitals.
Data Sources and Definitions
We used inpatient and outpatient demographic, utilization, and comorbidity data from the National Patient Care Database. Pharmacy data were extracted from the Decision Support System National Data Extracts and Pharmacy Benefits Management. Vital status information was obtained from the Vital Status file, which incorporates data from veterans' death benefits claims, inpatient deaths, Medicare Vital Status files, and the Social Security Administration's death master file. We used encrypted patient identifiers link to information across these databases.
We obtained demographic information (age, sex, race, marital status) from inpatient and outpatient data. We categorized race as white, black, Hispanic, and other/unknown. To infer active smoking and/or tobacco cessation attempts, we identified ICD‐9 codes for tobacco use (305.1, V15.82), smoking cessation clinic use, and/or use of medications for the treatment of nicotine dependence (Zyban, nicotine replacement, or varenicline). We used VA priority status as a proxy for socioeconomic and disability status. VA priority groups are a way for the VA to focus limited funds to those veterans most in need. The highest group (priority group 1) must have at least a 50% service‐connected disability. Priority groups 2 through 6 include veterans with up to 40% service‐connected disability, former prisoners of war, those awarded certain honors, veterans with lower incomes, and the catastrophically disabled. The lowest groups (priority groups 7 and 8) include veterans with relatively higher incomes who agree to pay copayments.[9]
We also obtained information on comorbid conditions from inpatient and outpatient administrative data. We defined alcohol abuse using ICD‐9 codes 291, 303, 305.0, and illicit drug use with ICD‐9 codes 292, 304, 305 excluding 305.0‐.1. We used the Charlson Comorbidity Index to quantify levels of preexisting comorbidity[10] adapted for administrative databases, using ICD‐9 codes for 19 comorbid conditions from prior outpatient and inpatient encounters. We defined cardiovascular events and lung cancer that were diagnosed during the hospitalization as previously described.[11, 12]
Outcomes
Our primary study outcome was readmission within 30 days of hospital discharge for pneumonia from any VA acute care hospital only. Medicare has used 30‐day readmission as a quality indicator, as readmissions that occur closer to discharge are believed to be more likely due to events during the index hospitalization.[13]
Statistical Analyses
We randomly divided patients from our initial cohort into equal derivation or validation cohorts. We assessed differences between the 2 groups using the Student t test for continuous variables and 2 test for categorical variables. We performed univariate logistic regression analyses in the derivation cohort to examine the relationship between 30‐day rehospitalization and each of our potential covariates. We entered covariates that were significant at P<0.10 in the univariate analyses into a multiple regression model. Significant covariates at P<0.10 were then entered into the final model. For this model, we used bootstrapping with replacement in 1000 replications to obtain standard errors of our coefficients and associated P values. Because some subjects had more than 1 index admission, we used robust variancecovariance matrix estimators to compute standard errors for model coefficients.
We used the C statistic to assess the discrimination of our model. Calibration of this model was measured using the Hosmer‐Lemeshow 2 goodness of fit test, using 10 quantiles to group the data. We evaluated differences in discrimination between the derivation and validation cohorts by comparing C statistics.
Last, using the final model in the derivation cohort, 5 categories of severity were created based on subjects' predicted risk values for readmission. Severity categories in both the derivation and validation cohorts were then graphically evaluated.
We defined statistical significance as a 2‐tailed P value of <0.05. We used Stata 12 (StataCorp, College Station, TX) for all analyses.
RESULTS
Patient Characteristics
Of the 45,134 eligible patients, 13.2% were rehospitalized within 30 days, and rates by hospital ranged from 1.6% to 20.7%. Table 1 presents the comparison of baseline difference between the derivation and validation cohorts. There were no statistically significant differences between the 2 groups. Overall, the mean age was 77 years, 98% were male, and 54% were married. Over 90% of the patients received guideline‐concordant antibiotics, and only 10% required a stay in the intensive care unit (ICU). The average length of stay was 7 days (standard deviation of 11 days).
Characteristics | Derivation Cohort, N=22,567 | Validation Cohort, N=22,567 | P Value |
---|---|---|---|
| |||
Sociodemographics | |||
Age, mean (SD) | 77.3 (6.5) | 77.2 (6.5) | 0.30 |
Male, no (%) | 22,133 (98.1) | 22,175 (98.3) | 0.14 |
White, no (%) | 18,858 (83.6) | 18,719 (83.0) | 0.08 |
Black, no (%) | 2,562 (11.4) | 2,583 (11.5) | 0.76 |
Hispanic, no (%) | 1,337 (5.9) | 1,414 (6.3) | 0.13 |
Married, no (%) | 12,166 (53.9) | 12,258 (54.3) | 0.39 |
VA priority group, no (%) | 0.28 | ||
1 | 4,286 (19.0) | 4,281 (19.0) | |
26 | 16,306 (72.3) | 16,399 (72.8) | |
78 | 1,954 (8.7) | 1,860 (8.3) | |
Nursing home residence, no (%) | 199 (0.9) | 216 (1.0) | 0.41 |
Smoker status, no (%) | 8,579 (38.0) | 8,677 (38.5) | 0.34 |
Index hospitalization factors | |||
Myocardial infarction during hospitalization, no (%) | 469 (2.1) | 452 (2.0) | 0.57 |
Heart failure during hospitalization, no (%) | 4,817 (21.4) | 4,772 (21.2) | 0.61 |
Arrhythmia during hospitalization, no (%) | 4,356 (19.3) | 4,287 (19.0) | 0.41 |
Length of stay, mean (SD) | 7.2 (10.4) | 7.2 (11.7) | 0.76 |
Lung cancer diagnosis during hospitalization, no (%) | 31 (0.1) | 31 (0.1) | 1.00 |
ICU admission, no (%) | 2,282 (10.1) | 2,336 (10.4) | 0.40 |
Guideline concordant antibiotics, no (%) | 20,724 (91.8) | 20,643 (91.5) | 0.17 |
Invasive mechanical ventilation, no (%) | 793 (3.5) | 832 (3.7) | 0.32 |
Vasopressor use, no (%) | 495 (2.2) | 489 (2.2) | 0.85 |
Hospital complications, no (%) | |||
Renal organ failure | 2,671 (11.8) | 2,640 (11.7) | 0.65 |
Cardiac organ failure | 1,004 (4.5) | 959 (4.3) | 0.30 |
Hepatic organ failure | 33 (0.2) | 40 (0.2) | 0.41 |
Respiratory organ failure | 1,623 (7.2) | 1,583 (7.0) | 0.46 |
Comorbid illnesses | |||
Medical, no (%) | |||
Myocardial infarction | 1,528 (6.8) | 1,542 (6.8) | 0.79 |
Congestive heart failure | 5,815 (25.8) | 5,697 (25.2) | 0.20 |
Peripheral vascular disease | 3,413 (15.1) | 3,454 (15.3) | 0.59 |
Cerebrovascular disease | 3,993 (17.7) | 4,078 (18.1) | 0.30 |
Dementia | 1,141 (5.1) | 1,110 (4.9) | 0.50 |
COPD | 12,168 (53.9) | 12,076 (53.5) | 0.39 |
Rheumatologic disease | 646 (2.9) | 660 (2.9) | 0.69 |
Peptic ulcer disease | 795 (3.5) | 723 (3.2) | 0.06 |
Severe liver disease | 169 (0.8) | 169 (0.8) | 1.00 |
Mild liver disease | 78 (0.4) | 80 (0.4) | 0.87 |
Diabetes | 7,310 (32.4) | 7,410 (32.8) | 0.32 |
Diabetes with complications | 2,229 (9.9) | 2,248 (10.0) | 0.77 |
Chronic renal disease | 2,836 (12.6) | 2,745 (12.2) | 0.19 |
Hemi/paraplegia | 319 (1.4) | 338 (1.5) | 0.46 |
Any prior malignancy | 5,226 (23.2) | 5,269 (23.4) | 0.63 |
Metastatic solid tumor | 748 (3.3) | 795 (3.5) | 0.22 |
AIDS | 56 (0.3) | 49 (0.2) | 0.49 |
HIV | 23 (0.1) | 13 (0.1) | 0.10 |
Alcohol abuse | 897 (4.0) | 934 (4.1) | 0.38 |
Drug abuse | 254 (1.1) | 255 (1.1) | 0.96 |
Psychiatric, no (%) | |||
Anxiety disorder | 1,692 (7.5) | 1,722 (7.6) | 0.59 |
Depression indicator | 3,655 (16.2) | 3,718 (16.5) | 0.42 |
Bipolar disorder | 430 (1.9) | 433 (1.9) | 0.92 |
Cataract indicator | 6,106 (27.1) | 6,180 (27.4) | 0.43 |
Prostatitis indicator | 4,680 (20.7) | 4,593 (20.4) | 0.31 |
Schizophrenia indicator | 685 (3.0) | 676 (3.0) | 0.80 |
Post‐traumatic stress disorder | 844 (3.7) | 862 (3.8) | 0.66 |
Medication history within 90 days | |||
Cardiovascular drugs, mean (SD) | 1.8 (1.6) | 1.8 (1.6) | 0.65 |
Diabetes drugs, mean (SD) | 0.3 (0.7) | 0.3 (0.7) | 0.60 |
Inhaled corticosteroids, mean (SD) | 0.4 (0.9) | 0.4 (0.9) | 0.28 |
Pulmonary drug, mean (SD) | 1.3 (2.0) | 1.3 (2.0) | 0.36 |
Oral corticosteroids, no (%) | 5,363 (23.8) | 5,505 (24.4) | 0.12 |
Prior medical utilization | |||
Number of primary care clinic visits within 1 year, mean (SD) | 4.9 (4.2) | 4.9 (4.2) | 0.67 |
Number of emergency department visits within 1 year, mean (SD) | 1.2 (2.0) | 1.2 (2.0) | 0.86 |
Number of outpatient clinic visits within 1 year, mean (SD) | 14.7 (13.1) | 14.6 (13.0) | 0.33 |
Number of specialty clinic visits within 1 year, mean (SD) | 3.4 (5.5) | 3.3 (5.4) | 0.50 |
Prior hospital admission within 90 days, no (%) | 5,062 (22.4) | 5,141 (22.8) | 0.37 |
Predictors of 30‐Day Readmission
All variables listed in Table 1 were evaluated. The derivation logistic regression model is shown in Table 2, and only includes predictors associated with rehospitalization. Antibiotic concordance and time in the ICU were evaluated and did not predict readmission. Variables that were significantly associated with rehospitalization in the models included age, marital status, the number of emergency department clinic visits a year prior, prior admission within 90 days, number of nonpharmacy clinic visits in a year prior, and hospital length of stay. The increasing age and number of emergency department and clinic visits were associated with higher odds of readmission. The longer the length of stay and those patients who were married had a lower odds of subsequent readmission. Other variables included were the presence of chronic renal disease, prior malignancy, nursing home residence, congestive heart failure, and prior use of oral corticosteroids. The C statistics for the derivation and validation models were 0.615 and 0.613, respectively. There were no significant differences in any of the variables between the derivation and validation cohorts.
Predictors | OR | 95% CI | P Value | |
---|---|---|---|---|
Lower | Upper | |||
| ||||
Sociodemographics | ||||
Age at admission | 1.01 | 1.00 | 1.02 | <0.01 |
White | 1.14 | 1.02 | 1.28 | 0.02 |
Nonwhiteb | 1.00 | |||
Hispanic | 1.26 | 1.07 | 1.47 | 0.01 |
Not Hispanicc | 1.00 | |||
Married | 0.89 | 0.82 | 0.96 | <0.01 |
Not marriedd | 1.00 | |||
Nursing home residence | 1.68 | 1.18 | 2.39 | <0.01 |
Comorbid illnesses | ||||
Peptic ulcer disease | 1.24 | 1.02 | 1.50 | 0.03 |
Chronic renal disease | 1.18 | 1.06 | 1.33 | <0.01 |
Any prior malignancy | 1.17 | 1.06 | 1.28 | <0.01 |
Metastatic solid tumor | 1.20 | 0.98 | 1.47 | 0.08 |
AIDS | 1.99 | 1.06 | 3.74 | 0.03 |
Index admission factors | ||||
Heart failure on initial admission | 1.18 | 1.07 | 1.29 | <0.01 |
Length of stay (per day) | 0.97 | 0.97 | 0.98 | <0.01 |
Medication history | ||||
Cardiovascular drug count 90 days prior | 1.03 | 1.00 | 1.06 | 0.02 |
Oral corticosteroids 90 days prior | 1.16 | 1.06 | 1.27 | <0.01 |
Prior medical utilization | ||||
Number of emergency department visits within 1 year | 1.04 | 1.02 | 1.06 | <0.01 |
Number of specialty clinic visits within 1 year | 1.01 | 1.00 | 1.02 | 0.01 |
Prior hospital admission within 90 days | 1.60 | 1.46 | 1.75 | <0.01 |
Number of nonpharmacy clinic visits within 1 year | 1.00 | 1.00 | 1.01 | <0.01 |
Figure 1 shows that the derivation and validation models are similar in percent rehospitalization group in all severity groups.
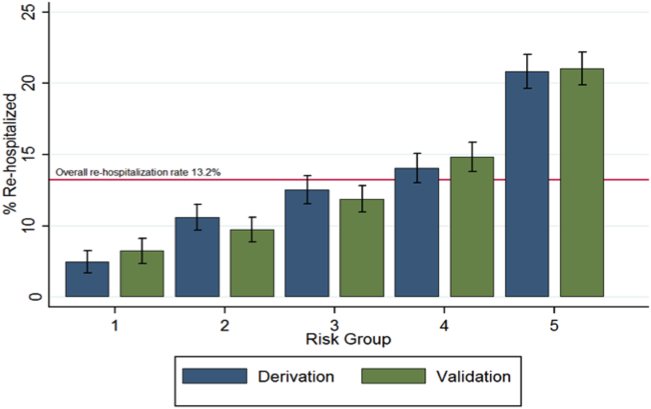
DISCUSSION
Sociodemographics and comorbidities, which are host factors, were significantly associated with rehospitalization of elderly patients admitted for pneumonia and length of hospital stay, the only pneumonia‐specific variable associated with readmission.[6, 14, 15, 16] Higher rates of all types of prior utilization (inpatient, outpatient, emergency department visits) were also associated with higher risk of rehospitalization. Our findings highlight the role that host factors play in rehospitalization of elderly patients with pneumonia and that there are few potentially modifiable targets to help reduce readmissions after pneumonia.
These findings may indicate 2 things: patients in this cohort are sicker than the general population at baseline and therefore require more medical care, and/or they are being evaluated by more providers increasing the chance they will be readmitted. For the former, an example is the association of prior use of oral corticosteroids with rehospitalization, which may demonstrate poorly controlled comorbidities (eg, chronic obstructive lung disease with recent exacerbation) that require steroid use. Given the VA's integrated healthcare system, greater access to primary care providers may lead to more opportunities for patients to voice their complaints, which can lead to more readmissions among severely ill patients. Weinberger et al. found an increase in hospital readmissions with increased access to primary care.[17] The association of readmission and length of stay is less likely related to pneumonia‐related clinical instabilities on discharge, but rather to the ability to address other potential issues related to the patient's underlying comorbidities.[5]
Our study presents a model looking at rehospitalization of patients with pneumonia, including factors not addressed in a prior publication by Lindenauer et al., the model Centers for Medicare and Medicaid Services (CMS) uses to predict early rehospitalization.[18] Lindenauer's model included 39 variables (of which 37 were clinical variables), including vertebral fractures, other injuries, other gastrointestinal disorders, other urinary tract disorders, as well as demographic and comorbidity factors that were similar to some of those included in our study. Their 30‐day rehospitalization medical record and administrative models had a C statistic of 0.59 and 0.63, respectively. A major difference between our presented model and the latter are the data on utilization of medical care. Despite these additional data, the resulting C statistics of 0.615 and 0.613 were not qualitatively better.
The similar C statistics demonstrates that factors playing a significant role in determining rehospitalization have yet to be identified, and it is not clear if researchers will be able to identify potentially modifiable risk factors for rehospitalization. Examples of other potential factors that should be examined in future studies include family/community support, quality of the transition of care back to their residence, and quality of inpatient care. Calvillo‐King et al. found a broad range of social factors that potentially affect the risk of postdischarge readmission for chronic heart failure.[19] For pneumonia, there were few studies that assessed the risk of social factors on readmission, and these were most commonly rather broad categories such as race/ethnicity or nursing home residence. In the publications that studied social factors and readmissions, there were inconsistent associations between hospital readmission with lower education, low income, and unemployment. Future articles may benefit from taking these additional factors into account.
Potential limitations to our study include the overwhelming majority of patients being male and in a VA population, which may limit generalizability. In addition, no data were available on the quality of the transition of care. Furthermore, limitations include the age of the data and the lack of evaluation of outpatient follow‐up and antibiotics on discharge. Though our data are up to 10 years old, this study evaluates risk factors that are still relevant. Finally, some variables noted in the CMS model were not evaluated in our study, such as protein‐calorie malnutrition, skin ulcers, and fracture history. Given these differences, we were unable to directly compare the performance of the 2 models.
Despite examining novel factors related to prior healthcare utilization, our model still performed suboptimally in identifying subjects at risk for rehospitalization after pneumonia. Future studies need to examine other social factors and patient frailty that may increase the risk of readmissions, as well as examine the impact of clinical stability on discharge. It remains to be determined if readmissions after pneumonia are truly preventable or mostly attributable to the patient's prior health status.
Disclosures: The project described was supported by grant number R01NR010828 from the National Institute of Nursing Research. The content is solely the responsibility of the authors and does not necessarily represent the official views of the National Institute of Nursing Research or the National Institutes of Health. This material is the result of work supported with resources and the use of facilities at the VA North Texas Health Care System. Funding agencies had no role in conducting the study, or role in the preparation, review, or approval of the manuscript. The views expressed in this article are those of the authors and do not necessarily represent the views of the Department of Veterans Affairs.
- Incidence and cost of pneumonia in Medicare beneficiaries. Chest. 2012;142(4):973–981. , , , et al.
- Rehospitalizations among patients in the Medicare fee‐for‐service program. N Engl J Med. 2009;360(14):1418–1428. , , .
- A prospective cohort study of healthcare visits and rehospitalizations after discharge of patients with community‐acquired pneumonia. Respirology. 2011;16(7):1119–1126. , , , et al.
- Pneumonia: criteria for patient instability on hospital discharge. Chest. 2008;134(3):595–600. , , , et al.
- Instability on hospital discharge and the risk of adverse outcomes in patients with pneumonia. Arch Intern Med. 2002;162(11):1278–1284. , , , et al.
- Causes and risk factors for rehospitalization of patients hospitalized with community‐acquired pneumonia. Clin Infect Dis. 2008;46(4):550–556. , , , et al.
- Population‐based study of statins, angiotensin II receptor blockers, and angiotensin‐converting enzyme inhibitors on pneumonia‐related outcomes. Clin Infect Dis. 2012;55(11):1466–1473. , , , et al.
- Quality of care, process, and outcomes in elderly patients with pneumonia. JAMA. 1997;278(23):2080–2084. , , , et al.
- U.S. Department of Veterans Affairs. Health benefits. Priority groups table. Available at: http://www.va.gov/healthbenefits/resources/priority_groups.asp. Accessed July 1, 2013.
- Validation of a combined comorbidity index. J Clin Epidemiol. 1994;47(11):1245–1251. , , , et al.
- Incidence of cardiovascular events after hospital admission for pneumonia. Am J Med. 2011;124(3):244–251. , , , et al.
- Diagnosis of pulmonary malignancy after hospitalization for pneumonia. Am J Med. 2010;123(1):66–71. , , , et al.
- MedPAC. 2013 [cited 2013 June 11]; Available from: http://www.hospitalcompare.gov.
- The relationship between hospital admission rates and rehospitalizations. N Engl J Med. 2011;365(24):2287–2295. , , .
- Predictors of short‐term rehospitalization following discharge of patients hospitalized with community‐acquired pneumonia. Chest. 2009;136(4):1079–1085. , , , et al.
- Effects of previous influenza vaccination on subsequent readmission and mortality in elderly patients hospitalized with pneumonia. Am J Med. 2003;115(6):454–461. , , , et al.
- Does increased access to primary care reduce hospital readmissions? N Engl J Med. 1996;334(22):1441–1447. , , .
- Development, validation, and results of a measure of 30‐day readmission following hospitalization for pneumonia. J Hosp Med. 2011;6(3):142–150. , , , et al.
- Impact of social factors on risk of readmission or mortality in pneumonia and heart failure: systematic review. J Gen Intern Med. 2013;28(2):269–282. , , , et al.
- Incidence and cost of pneumonia in Medicare beneficiaries. Chest. 2012;142(4):973–981. , , , et al.
- Rehospitalizations among patients in the Medicare fee‐for‐service program. N Engl J Med. 2009;360(14):1418–1428. , , .
- A prospective cohort study of healthcare visits and rehospitalizations after discharge of patients with community‐acquired pneumonia. Respirology. 2011;16(7):1119–1126. , , , et al.
- Pneumonia: criteria for patient instability on hospital discharge. Chest. 2008;134(3):595–600. , , , et al.
- Instability on hospital discharge and the risk of adverse outcomes in patients with pneumonia. Arch Intern Med. 2002;162(11):1278–1284. , , , et al.
- Causes and risk factors for rehospitalization of patients hospitalized with community‐acquired pneumonia. Clin Infect Dis. 2008;46(4):550–556. , , , et al.
- Population‐based study of statins, angiotensin II receptor blockers, and angiotensin‐converting enzyme inhibitors on pneumonia‐related outcomes. Clin Infect Dis. 2012;55(11):1466–1473. , , , et al.
- Quality of care, process, and outcomes in elderly patients with pneumonia. JAMA. 1997;278(23):2080–2084. , , , et al.
- U.S. Department of Veterans Affairs. Health benefits. Priority groups table. Available at: http://www.va.gov/healthbenefits/resources/priority_groups.asp. Accessed July 1, 2013.
- Validation of a combined comorbidity index. J Clin Epidemiol. 1994;47(11):1245–1251. , , , et al.
- Incidence of cardiovascular events after hospital admission for pneumonia. Am J Med. 2011;124(3):244–251. , , , et al.
- Diagnosis of pulmonary malignancy after hospitalization for pneumonia. Am J Med. 2010;123(1):66–71. , , , et al.
- MedPAC. 2013 [cited 2013 June 11]; Available from: http://www.hospitalcompare.gov.
- The relationship between hospital admission rates and rehospitalizations. N Engl J Med. 2011;365(24):2287–2295. , , .
- Predictors of short‐term rehospitalization following discharge of patients hospitalized with community‐acquired pneumonia. Chest. 2009;136(4):1079–1085. , , , et al.
- Effects of previous influenza vaccination on subsequent readmission and mortality in elderly patients hospitalized with pneumonia. Am J Med. 2003;115(6):454–461. , , , et al.
- Does increased access to primary care reduce hospital readmissions? N Engl J Med. 1996;334(22):1441–1447. , , .
- Development, validation, and results of a measure of 30‐day readmission following hospitalization for pneumonia. J Hosp Med. 2011;6(3):142–150. , , , et al.
- Impact of social factors on risk of readmission or mortality in pneumonia and heart failure: systematic review. J Gen Intern Med. 2013;28(2):269–282. , , , et al.
2014 Society of Hospital Medicine. This article is a US government work and, as such, is in the public domain in the United States of America.