User login
Caring About Prognosis
Prognostication continues to be a challenge to the clinician despite over 100 prognostic indices that have been developed during the past few decades to inform clinical practice and medical decision making.[1] Physicians are not accurate in prognostication of patients' risk of death and tend to overestimate survival.[2, 3] In addition, many physicians do not feel comfortable offering a prognosis to patients, despite patients' wishes to be informed.[4, 5] Regardless of the prevalence in the literature and value in improving physicians' prognostic accuracy, prognostic indices of survival are not regularly utilized in the hospital setting. Prognostic tools available for providers are often complicated and may require data about patients that are not readily available.[6, 7, 8] Prognostic indices may be too specific to a patient population, too difficult to remember, or too time consuming to use. A simple, rapid, and practical prognostic index is important in the hospital setting to assist in identifying patients at high risk of death so that primary palliative interventions can be incorporated into the plan of care early in the hospital stay. Patient and family education, advance care planning, formulating the plan of care based on patientfamily goals, and improved resource utilization could be better executed by more accurate risk of death prediction on hospital admission.
The CARING criteria are the only prognostic index to our knowledge that evaluates a patient's risk of death in the next year, with information readily available at the time of hospital admission (Table 1).[9] The CARING criteria are a unique prognostic tool: (1) CARING is a mnemonic acronym, making it more user friendly to the clinician. (2) The 5 prognostic indicators are readily available from the patient's chart on admission; gathering further data by patient or caretaker interviews or by obtaining laboratory data is not needed. (3) The timing for application of the tool on admission to the hospital is an ideal opportunity to intervene and introduce palliative interventions early on the hospital stay. The CARING criteria were developed and validated in a Veteran's Administration hospital setting by Fischer et al.[9] We sought to validate the CARING criteria in a broader patient populationmedical and surgical patients from a tertiary referral university hospital setting and a safety‐net hospital setting.
METHODS
Study Design
This study was a retrospective observational cohort study. The study was approved by the Colorado Multiple Institutional Review Board and the University of Colorado Hospital Research Review Committee.
Study Purpose
To validate the CARING criteria in a tertiary referral university hospital (University of Colorado Hospital [UCH]) and safety‐net hospital (Denver Health and Hospitals [DHH]) setting using similar methodology to that employed by the original CARING criteria study.[9]
Study Setting/Population
All adults (18 years of age) admitted as inpatients to the medical and surgical services of internal medicine, hospitalist, pulmonary, cardiology, hematology/oncology, hepatology, surgery, intensive care unit, and intermediary care unit at UCH and DHH during the study period of July 2005 through August 2005. The only exclusion criteria were those patients who were prisoners or pregnant. Administrative admission data from July 2005 to August 2005 were used to identify names of all persons admitted to the medicine and surgical services of the study hospitals during the specified time period.
The 2 study hospitals, UCH and DHH, provide a range of patients who vary in ethnicity, socioeconomic status, and medical illness. This variability allows for greater generalizability of the results. Both hospitals are affiliated with the University of Colorado School of Medicine internal medicine residency training program and are located in Denver, Colorado.
At the time of the study, UCH was a licensed 550‐bed tertiary referral, academic hospital serving the Denver metropolitan area and the Rocky Mountain region as a specialty care and referral center. DHH was a 398‐bed, academic, safety‐net hospital serving primarily the Denver metropolitan area. DHH provides 42% of the care for the uninsured in Denver and 26% of the uninsured care for the state of Colorado.
Measures
The CARING criteria were developed and validated in a Veteran's Administration (VA) hospital setting by Fischer et al.[9] The purpose of the CARING criteria is to identify patients, at the time of hospital admission, who are at higher risk of death in the following year. The prognostic index uses 5 predictors that can be abstracted from the chart at time of admission. The CARING criteria were developed a priori, and patients were evaluated using only the medical data available at the time of admission. The criteria include items that are already part of the routine physician admission notes and do not require additional data collection or assessments. The criteria include: C=primary diagnosis of cancer, A=2 admissions to the hospital for a chronic illness within the last year; R=resident in a nursing home; I=intensive care unit (ICU) admission with multiorgan failure (MOF), NG=noncancer hospice guidelines (meeting 2 of the National Hospice and Palliative Care Organization's [NHPCO] guidelines).
Patients were identified using name, date of birth, social security number, address, and phone number. This identifying information was then used for tracing death records 1 year after hospital admission.
Mortality at 1 year following the index hospitalization was the primary end point. To minimize missing data and the number of subjects lost to follow‐up, 3 determinants of mortality were used. First, electronic medical records of the 2 participating hospitals and their outpatient clinics were reviewed to determine if a follow‐up appointment had occurred past the study's end point of 1 year (August 2006). For those without a confirmed follow‐up visit, death records from the Colorado Department of Public Health and Vital Records were obtained. For those patients residing outside of Colorado or whose mortality status was still unclear, the National Death Index was accessed.
Medical Record Review
Medical records for all study participants were reviewed by J.Y. (UCH) and B.C. (DHH). Data collection was completed using direct data entry into a Microsoft Access (Microsoft Corp., Redmond, WA) database utilizing a data entry form linked with the database table. This form utilized skip patterns and input masks to ensure quality of data entry and minimize missing or invalid data. Inter‐rater reliability was assessed by an independent rereview (S.F.) of 5% of the total charts. Demographic variables were collected using hospital administrative data. These included personal identifiers of the participants for purposes of mortality follow‐up. Clinical data including the 5 CARING variables and additional descriptive variables were abstracted from the paper hospital chart and the electronic record of the chart (together these constitute the medical record).
Death Follow‐up
A search of Colorado death records was conducted in February 2011 for all subjects. Death records were used to determine mortality and time to death from the index hospitalization. The National Death Index was then searched for any subjects without or record of death in Colorado.
Analysis
All analyses were conducted using the statistical application software SAS for Windows version 9.3 (SAS Institute, Cary, NC). Simple frequencies and means ( standard deviation) were used to describe the baseline characteristics. Multiple logistic regression models were used to model 1‐year mortality. The models were fitted using all of the CARING variables and age. As the aim of the study was to validate the CARING criteria, the variables for the models were selected a priori based on the original index. Two hospital cohorts (DHH and UCH) were modeled separately and as a combined sample. Kaplan‐Meier survival analysis was conducted to compare those subjects who met 1 of the CARING criteria with those who did not through the entire period of mortality follow‐up (20052011). Finally, using the probabilities from the logistic regression models, we again developed a scoring rule appropriate for a non‐VA setting to allow clinicians to easily identify patient risk for 1‐year mortality at the time of hospital admission.
RESULTS
There were a total of 1064 patients admitted to the medical and surgical services during the study period568 patients at DHH and 496 patients at UCH. Sample characteristics of each individual hospital cohort and the entire combined study cohort are detailed in Table 2. Overall, slightly over half the population were male, with a mean age of 50 years, and the ethnic breakdown roughly reflects the population in Denver. A total of 36.5% (n=388) of the study population met 1 of the CARING criteria, and 12.6% (n=134 among 1063 excluding 1 without an admit date) died within 1 year of the index hospitalization. These were younger and healthier patients compared to the VA sample used in developing the CARING criteria.
| |
Renal | Dementia |
Stop/decline dialysis | Unable to ambulate independently |
Not candidate for transplant | Urinary or fecal incontinence |
Urine output < 40cc/24 hours | Unable to speak with more than single words |
Creatinine > 8.0 (>6.0 for diabetics) | Unable to bathe independently |
Creatinine clearance 10cc/min | Unable to dress independently |
Uremia | Co‐morbid conditions: |
Persistent serum K + > 7.0 | Aspiration pneumonia |
Co‐morbid conditions: | Pyelonephritis |
Cancer CHF | Decubitus ulcer |
Chronic lung disease AIDS/HIV | Difficulty swallowing or refusal to eat |
Sepsis Cirrhosis | |
Cardiac | Pulmonary |
Ejection fraction < 20% | Dyspnea at rest |
Symptomatic with diuretics and vasodilators | FEV1 < 30% |
Not candidate for transplant | Frequent ER or hospital admits for pulmonary infections or respiratory distress |
History of cardiac arrest | Cor pulmonale or right heart failure |
History of syncope | 02 sat < 88% on 02 |
Systolic BP < 120mmHG | PC02 > 50 |
CVA cardiac origin | Resting tachycardia > 100/min |
Co‐morbid conditions as listed in Renal | Co‐morbid conditions as listed in Renal |
Liver | Stroke/CVA |
End stage cirrhosis | Coma at onset |
Not candidate for transplant | Coma >3 days |
Protime > 5sec and albumin <2.5 | Limb paralysis |
Ascites unresponsive to treatment | Urinary/fecal incontinence |
Hepatorenal syndrome | Impaired sitting balance |
Hepatic encephalopathy | Karnofsky < 50% |
Spontaneous bacterial peritonitis | Recurrent aspiration |
Recurrent variceal bleed | Age > 70 |
Co‐morbid conditions as listed in Renal | Co‐morbid conditions as listed in Renal |
HIV/AIDS | Neuromuscular |
Persistent decline in function | Diminished respiratory function |
Chronic diarrhea 1 year | Chosen not to receive BiPAP/vent |
Decision to stop treatment | Difficulty swallowing |
CNS lymphoma | Diminished functional status |
MAC‐untreated | Incontinence |
Systemic lymphoma | Co‐morbid conditions as listed in Renal |
Dilated cardiomyopathy | |
CD4 < 25 with disease progression | |
Viral load > 100,000 |
Safety‐Net Hospital Cohort, N=568 | Academic Center Cohort, N=496 | Study Cohort,N=1064 | Original CARING Cohort, N=8739 | |
---|---|---|---|---|
| ||||
Mean age ( SD), y | 47.8 (16.5) | 54.4 (17.5) | 50.9 (17.3) | 63 (13) |
Male gender | 59.5% (338) | 50.1% (248) | 55.1% (586) | 98% (856) |
Ethnicity | ||||
African American | 14.1% (80) | 13.5% (65) | 13.8% (145) | 13% (114) |
Asian | 0.4% (2) | 1.5% (7) | 0.9% (9) | Not reported |
Caucasian | 41.7% (237) | 66.3% (318) | 53.0 % (555) | 69% (602) |
Latino | 41.9% (238) | 9.6% (46) | 27.1% (284) | 8% (70) |
Native American | 0.5% (3) | 0.4% (2) | 0.5% (5) | Not reported |
Other | 0.5% (3) | 0.6% (3) | 0.6% (6) | 10% (87) |
Unknown | 0.9% (5) | 8.1% (39) | 4.2% (44) | Not reported |
CARING criteria | ||||
Cancer | 6.2% (35) | 19.4% (96) | 12.3% (131) | 23% (201) |
Admissions to the hospital 2 in past year | 13.6% (77) | 42.7% (212) | 27.2% (289) | 36% (314) |
Resident in a nursing home | 1.8% (10) | 3.4% (17) | 2.5% (27) | 3% (26) |
ICU with MOF | 3.7% (21) | 1.2% (6) | 2.5% (27) | 2% (17) |
NHPCO (2) noncancer guidelines | 1.6% (9) | 5.9% (29) | 3.6% (38) | 8% (70) |
Reliability testing demonstrated excellent inter‐rater reliability. Kappa for each criterion is as follows: (1) primary diagnosis of cancer=1.0, (2) 2 admissions to the hospital in the past year=0.91, (3) resident in a nursing home=1.0, (4) ICU admission with MOF=1.0, and (5) 2 noncancer hospice guidelines=0.78.
This study aimed to validate the CARING criteria9; therefore, all original individual CARING criterion were included in the validation logistic regression models. The 1 exception to this was in the university hospital study cohort, where the ICU criterion was excluded from the model due to small sample size and quasiseparation in the model. The model results are presented in Table 3 for the individual hospitals and combined study cohort.
Safety Net Hospital Cohort, C Index=0.76 | Academic Center Cohort, C Index=0.76 | Combined Hospital Cohort, C Index=0.79 | ||||
---|---|---|---|---|---|---|
Estimate | Odds Ratio (95% CI) | Estimate | Odds Ratio (95% CI) | Estimate | Odds Ratio (95% CI) | |
| ||||||
Cancer | 1.92 | 6.85 (2.83‐16.59)a | 1.85 | 6.36 (3.54‐11.41)a | 1.98 | 7.23 (4.45‐11.75)a |
Admissions to the hospital 2 in past year | 0.55 | 1.74 (0.76‐3.97) | 0.14 | 0.87 (0.51‐1.49) | 0.20 | 1.22 (0.78‐1.91) |
Resident in a nursing home | 0.49 | 0.61 (0.06‐6.56) | 0.27 | 1.31 (0.37‐4.66) | 0.09 | 1.09 (0.36‐3.32) |
ICU with MOF | 1.85 | 6.34 (2.0219.90)a | 1.94 | 6.97 (2.75‐17.68)a | ||
NHPCO (2) noncancer guidelines | 3.04 | 20.86 (4.25102.32)a | 2.62 | 13.73 (5.86‐32.15)a | 2.74 | 15.55 (7.2833.23)a |
Ageb | 0.38 | 1.46 (1.05‐2.03)a | 0.45 | 1.56 (1.23‐1.98)a | 0.47 | 1.60 (1.32‐1.93)a |
In the safety‐net hospital, admission to the hospital with a primary diagnosis related to cancer, 2 noncancer hospice guidelines, ICU admission with MOF, and age by category all were significant predictors of 1‐year mortality. In the university hospital cohort, primary diagnosis of cancer, 2 noncancer hospice guidelines, and age by category were predictive of 1‐year mortality. Finally, in the entire study cohort, primary diagnosis of cancer, ICU with MOF, 2 noncancer hospice guidelines, and age were all predictive of 1‐year mortality. Parameter estimates were similar in 3 of the criteria compared to the VA setting. Differences in patient characteristics may have caused the differences in the estimates. Gender was additionally tested but not significant in any model. One‐year survival was significantly lower for those who met 1 of the CARING criteria versus those who did not (Figure 1).
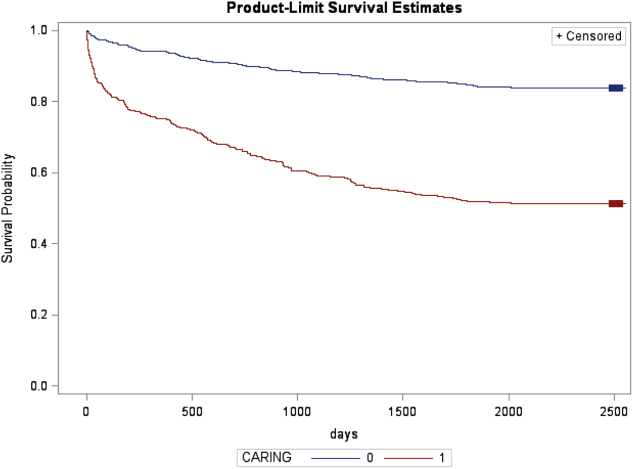
Based on the framework from the original CARING criteria analysis, a scoring rule was developed using the regression results of this validation cohort. To predict a high probability of 1‐year mortality, sensitivity was set to 58% and specificity was set at 86% (error rate=17%). Medium to high probability was set with a sensitivity of 73% and specificity of 72% (error rate=28%). The coefficients from the regression model of the entire study cohort were converted to scores for each of the CARING criteria. The scores are as follows: 0.5 points for admission from a nursing home, 1 point for 2 hospital admissions in the past year for a chronic illness, 10 points for primary diagnosis of cancer, 10 points for ICU admission with MOF, and 14 points for 2 noncancer hospice guidelines. For every age category increase, 2 points are assigned so that 0 points for age <55 years, 2 points for ages 56 to 65 years, 4 points for ages 66 to 75 years, and 6 points for >75 years. Points for individual risk factors were proportional to s (ie, log odds) in the logistic regression model for death at 1 year. Although no linear transformation exists between s and probabilities (of death at 1 year), the aggregated points for combinations of risk factors shown in Table 4 follow the probabilities in an approximately linear fashion, so that different degrees of risk of death can be represented contiguously (as highlighted by differently shaded regions in the scoring matrix) (Table 4). The scoring matrix allows for quick identification for patients at high risk for 1‐year mortality. In this non‐VA setting with healthier patients, low risk is defined at a lower probability threshold (0.1) compared to the VA setting (0.175).
CARING Criteria Components | |||||||
---|---|---|---|---|---|---|---|
None | Resident in a Nursing Home | Admitted to the Hospital 2 Times in the Past Year | Resident in a Nursing Home Admitted to the Hospital 2 Times in the Past Year | Primary Diagnosis of Cancer | ICU Admission With MOF | Noncancer Hospice Guidelines | |
| |||||||
Age | |||||||
55 years | 0 | 0.5 | 1 | 1.5 | 10 | ||
5565 years | 2 | 2.5 | 3 | 3.5 | 10 | ||
6675 years | 4 | 4.5 | 5 | 5.5 | 10 | ||
>75 years | 6 | 6.5 | 7 | 7.5 | 10 | ||
Risk | |||||||
Low | 3.5 | Probability<0.1 | |||||
Medium | 46.5 | 0.1probability <0.175 | |||||
High | 7 | Probability0.175 |
DISCUSSION
The CARING criteria are a practical prognostic tool that can be easily and rapidly applied to patients admitted to the hospital to estimate risk of death in 1 year, with the goal of identifying patients who may benefit most from incorporating palliative interventions into their plan of care. This study validated the CARING criteria in a tertiary referral university hospital and safety‐net hospital setting, demonstrating applicability in a much broader population than the VA hospital of the original CARING criteria study. The population studied represented a younger population by over 10 years, a more equitable proportion of males to females, a broader ethnic diversity, and lower 1‐year deaths rates than the original study. Despite the broader representation of the population, the significance of each of the individual CARING criterion was maintained except for 2 hospital admissions in the past year for a chronic illness (admission from a nursing home did not meet significance in either study as a sole criterion). As with the original study, meeting 2 of the NHPCO noncancer hospice guidelines demonstrated the highest risk of 1‐year mortality following index hospitalization, followed by primary diagnosis of cancer and ICU admission with MOF. Advancing age, also similar to the original study, conferred increased risk across the criterion.
Hospitalists could be an effective target for utilizing the CARING criteria because they are frequently the first‐line providers in the hospital setting. With the national shortage of palliative care specialists, hospitalists need to be able to identify when a patient has a limited life expectancy so they will be better equipped to make clinical decisions that are aligned with their patients' values, preferences, and goals of care. With the realization that not addressing advance care planning and patient goals of care may be considered medical errors, primary palliative care skills become alarmingly more important as priorities for hospitalists to obtain and feel comfortable using in daily practice.
The CARING criteria are directly applicable to patients who are seen by hospitalists. Other prognostic indices have focused on select patient populations, such as the elderly,[10, 11, 12] require collection of data that are not readily available on admission or would not otherwise be obtained,[10, 13] or apply to patients post‐hospital discharge, thereby missing the opportunity to make an impact earlier in the disease trajectory and incorporate palliative care into the hospital plan of care when key discussions about goals of care and preferences should be encouraged.
Additionally, the CARING criteria could easily be incorporated as a trigger for palliative care consults on hospital admission. Palliative care consults tend to happen late in a hospital stay, limiting the effectiveness of the palliative care team. A trigger system for hospitalists and other primary providers on hospital admission would lend to more effective timing of palliative measures being incorporated into the plan of care. Palliative care consults would not only be initiated earlier, but could be targeted for the more complex and sick patients with the highest risk of death in the next year.
In the time‐pressured environment, the presence of any 1 of the CARING criteria can act as a trigger to begin incorporating primary palliative care measures into the plan of care. The admitting hospitalist provider (ie, physician, nurse practitioner, physician assistant) could access the CARING criteria through an electronic health record prompt when admitting patients. When a more detailed assessment of mortality risk is helpful, the hospitalist can use the scoring matrix, which combines age with the individual criterion to calculate patients at medium or high risk of death within 1 year. Limited resources can then be directed to the patients with the greatest need. Patients with a focused care need, such as advance care planning or hospice referral, can be directed to the social worker or case manager. More complicated patients may be referred to a specialty palliative care team.
Several limitations to this study are recognized, including the small sample size of patients meeting criterion for ICU with MOF in the academic center study cohort. The patient data were collected during a transition time when the university hospital moved to a new campus, resulting in an ICU at each campus that housed patients with differing levels of illness severity, which may have contributed to the lower acuity ICU patient observed. Although we advocate the simplicity of the CARING criteria, the NHPCO noncancer hospice guidelines are more complicated, as they incorporates 8 broad categories of chronic illness. The hospice guidelines may not be general knowledge to the hospitalist or other primary providers. ePrognosis (
CONCLUSION
The CARING criteria are a simple, practical prognostic tool predictive of death within 1 year that has been validated in a broad population of hospitalized patients. The criteria hold up in a younger, healthier population that is more diverse by age, gender, and ethnicity than the VA population. With ready access to critical prognostic information on hospital admission, clinicians will be better informed to make decisions that are aligned with their patients' values, preferences, and goals of care.
Disclosure
Nothing to report.
- Predicting death: an empirical evaluation of predictive tools for mortality. Arch Intern Med. 2011;171:1721–1726. , , .
- Extent and determinants of error in physicians' prognoses in terminally ill patients: prospective cohort study. West J Med. 2000;172:310–313. , .
- A systematic review of physicians' survival predictions in terminally ill cancer patients. BMJ. 2003;327:195–198. , , , et al.
- Attitude and self‐reported practice regarding prognostication in a national sample of internists. Arch Intern Med. 1998;158:2389–2395. , .
- Discussing prognosis: balancing hope and realism. Cancer J. 2010;16:461–466. , , , et al.
- Acute Physiology and Chronic Health Evaluation (APACHE) IV: hospital mortality assessment for today's critically ill patients. Crit Care Med. 2006;34:1297–1310. , , , .
- SAPS 3 admission score: an external validation in a general intensive care population. Intensive Care Med. 2008;34:1873–1877. , , , , .
- Prospective validation of the intensive care unit admission Mortality Probability Model (MPM0‐III). Crit Care Med. 2009;37:1619–1623. , , , , , .
- A practical tool to identify patients who may benefit from a palliative approach: the CARING criteria. J Pain Symptom Manage. 2006;31:285–292. , , , , , .
- Prediction of survival for older hospitalized patients: the HELP survival model. J Am Geriatr Soc. 2000;48:S16–S24. , , , et al.
- Development and validation of a multidimensional prognostic index for one‐year mortality from comprehensive geriatric assessment in hospitalized older patients. Rejuvenation Res. 2008;11:151–161. , , , et al.
- Burden of illness score for elderly persons: risk adjustment incorporating the cumulative impact of diseases, physiologic abnormalities, and functional impairments. Med Care. 2003;41:70–83. , , , et al.
- The SUPPORT prognostic model. Objective estimates of survival for seriously ill hospitalized adults. Study to understand prognoses and preferences for outcomes and risks of treatments. Ann Intern Med. 1995;122:191–203. , , , et al.
Prognostication continues to be a challenge to the clinician despite over 100 prognostic indices that have been developed during the past few decades to inform clinical practice and medical decision making.[1] Physicians are not accurate in prognostication of patients' risk of death and tend to overestimate survival.[2, 3] In addition, many physicians do not feel comfortable offering a prognosis to patients, despite patients' wishes to be informed.[4, 5] Regardless of the prevalence in the literature and value in improving physicians' prognostic accuracy, prognostic indices of survival are not regularly utilized in the hospital setting. Prognostic tools available for providers are often complicated and may require data about patients that are not readily available.[6, 7, 8] Prognostic indices may be too specific to a patient population, too difficult to remember, or too time consuming to use. A simple, rapid, and practical prognostic index is important in the hospital setting to assist in identifying patients at high risk of death so that primary palliative interventions can be incorporated into the plan of care early in the hospital stay. Patient and family education, advance care planning, formulating the plan of care based on patientfamily goals, and improved resource utilization could be better executed by more accurate risk of death prediction on hospital admission.
The CARING criteria are the only prognostic index to our knowledge that evaluates a patient's risk of death in the next year, with information readily available at the time of hospital admission (Table 1).[9] The CARING criteria are a unique prognostic tool: (1) CARING is a mnemonic acronym, making it more user friendly to the clinician. (2) The 5 prognostic indicators are readily available from the patient's chart on admission; gathering further data by patient or caretaker interviews or by obtaining laboratory data is not needed. (3) The timing for application of the tool on admission to the hospital is an ideal opportunity to intervene and introduce palliative interventions early on the hospital stay. The CARING criteria were developed and validated in a Veteran's Administration hospital setting by Fischer et al.[9] We sought to validate the CARING criteria in a broader patient populationmedical and surgical patients from a tertiary referral university hospital setting and a safety‐net hospital setting.
METHODS
Study Design
This study was a retrospective observational cohort study. The study was approved by the Colorado Multiple Institutional Review Board and the University of Colorado Hospital Research Review Committee.
Study Purpose
To validate the CARING criteria in a tertiary referral university hospital (University of Colorado Hospital [UCH]) and safety‐net hospital (Denver Health and Hospitals [DHH]) setting using similar methodology to that employed by the original CARING criteria study.[9]
Study Setting/Population
All adults (18 years of age) admitted as inpatients to the medical and surgical services of internal medicine, hospitalist, pulmonary, cardiology, hematology/oncology, hepatology, surgery, intensive care unit, and intermediary care unit at UCH and DHH during the study period of July 2005 through August 2005. The only exclusion criteria were those patients who were prisoners or pregnant. Administrative admission data from July 2005 to August 2005 were used to identify names of all persons admitted to the medicine and surgical services of the study hospitals during the specified time period.
The 2 study hospitals, UCH and DHH, provide a range of patients who vary in ethnicity, socioeconomic status, and medical illness. This variability allows for greater generalizability of the results. Both hospitals are affiliated with the University of Colorado School of Medicine internal medicine residency training program and are located in Denver, Colorado.
At the time of the study, UCH was a licensed 550‐bed tertiary referral, academic hospital serving the Denver metropolitan area and the Rocky Mountain region as a specialty care and referral center. DHH was a 398‐bed, academic, safety‐net hospital serving primarily the Denver metropolitan area. DHH provides 42% of the care for the uninsured in Denver and 26% of the uninsured care for the state of Colorado.
Measures
The CARING criteria were developed and validated in a Veteran's Administration (VA) hospital setting by Fischer et al.[9] The purpose of the CARING criteria is to identify patients, at the time of hospital admission, who are at higher risk of death in the following year. The prognostic index uses 5 predictors that can be abstracted from the chart at time of admission. The CARING criteria were developed a priori, and patients were evaluated using only the medical data available at the time of admission. The criteria include items that are already part of the routine physician admission notes and do not require additional data collection or assessments. The criteria include: C=primary diagnosis of cancer, A=2 admissions to the hospital for a chronic illness within the last year; R=resident in a nursing home; I=intensive care unit (ICU) admission with multiorgan failure (MOF), NG=noncancer hospice guidelines (meeting 2 of the National Hospice and Palliative Care Organization's [NHPCO] guidelines).
Patients were identified using name, date of birth, social security number, address, and phone number. This identifying information was then used for tracing death records 1 year after hospital admission.
Mortality at 1 year following the index hospitalization was the primary end point. To minimize missing data and the number of subjects lost to follow‐up, 3 determinants of mortality were used. First, electronic medical records of the 2 participating hospitals and their outpatient clinics were reviewed to determine if a follow‐up appointment had occurred past the study's end point of 1 year (August 2006). For those without a confirmed follow‐up visit, death records from the Colorado Department of Public Health and Vital Records were obtained. For those patients residing outside of Colorado or whose mortality status was still unclear, the National Death Index was accessed.
Medical Record Review
Medical records for all study participants were reviewed by J.Y. (UCH) and B.C. (DHH). Data collection was completed using direct data entry into a Microsoft Access (Microsoft Corp., Redmond, WA) database utilizing a data entry form linked with the database table. This form utilized skip patterns and input masks to ensure quality of data entry and minimize missing or invalid data. Inter‐rater reliability was assessed by an independent rereview (S.F.) of 5% of the total charts. Demographic variables were collected using hospital administrative data. These included personal identifiers of the participants for purposes of mortality follow‐up. Clinical data including the 5 CARING variables and additional descriptive variables were abstracted from the paper hospital chart and the electronic record of the chart (together these constitute the medical record).
Death Follow‐up
A search of Colorado death records was conducted in February 2011 for all subjects. Death records were used to determine mortality and time to death from the index hospitalization. The National Death Index was then searched for any subjects without or record of death in Colorado.
Analysis
All analyses were conducted using the statistical application software SAS for Windows version 9.3 (SAS Institute, Cary, NC). Simple frequencies and means ( standard deviation) were used to describe the baseline characteristics. Multiple logistic regression models were used to model 1‐year mortality. The models were fitted using all of the CARING variables and age. As the aim of the study was to validate the CARING criteria, the variables for the models were selected a priori based on the original index. Two hospital cohorts (DHH and UCH) were modeled separately and as a combined sample. Kaplan‐Meier survival analysis was conducted to compare those subjects who met 1 of the CARING criteria with those who did not through the entire period of mortality follow‐up (20052011). Finally, using the probabilities from the logistic regression models, we again developed a scoring rule appropriate for a non‐VA setting to allow clinicians to easily identify patient risk for 1‐year mortality at the time of hospital admission.
RESULTS
There were a total of 1064 patients admitted to the medical and surgical services during the study period568 patients at DHH and 496 patients at UCH. Sample characteristics of each individual hospital cohort and the entire combined study cohort are detailed in Table 2. Overall, slightly over half the population were male, with a mean age of 50 years, and the ethnic breakdown roughly reflects the population in Denver. A total of 36.5% (n=388) of the study population met 1 of the CARING criteria, and 12.6% (n=134 among 1063 excluding 1 without an admit date) died within 1 year of the index hospitalization. These were younger and healthier patients compared to the VA sample used in developing the CARING criteria.
| |
Renal | Dementia |
Stop/decline dialysis | Unable to ambulate independently |
Not candidate for transplant | Urinary or fecal incontinence |
Urine output < 40cc/24 hours | Unable to speak with more than single words |
Creatinine > 8.0 (>6.0 for diabetics) | Unable to bathe independently |
Creatinine clearance 10cc/min | Unable to dress independently |
Uremia | Co‐morbid conditions: |
Persistent serum K + > 7.0 | Aspiration pneumonia |
Co‐morbid conditions: | Pyelonephritis |
Cancer CHF | Decubitus ulcer |
Chronic lung disease AIDS/HIV | Difficulty swallowing or refusal to eat |
Sepsis Cirrhosis | |
Cardiac | Pulmonary |
Ejection fraction < 20% | Dyspnea at rest |
Symptomatic with diuretics and vasodilators | FEV1 < 30% |
Not candidate for transplant | Frequent ER or hospital admits for pulmonary infections or respiratory distress |
History of cardiac arrest | Cor pulmonale or right heart failure |
History of syncope | 02 sat < 88% on 02 |
Systolic BP < 120mmHG | PC02 > 50 |
CVA cardiac origin | Resting tachycardia > 100/min |
Co‐morbid conditions as listed in Renal | Co‐morbid conditions as listed in Renal |
Liver | Stroke/CVA |
End stage cirrhosis | Coma at onset |
Not candidate for transplant | Coma >3 days |
Protime > 5sec and albumin <2.5 | Limb paralysis |
Ascites unresponsive to treatment | Urinary/fecal incontinence |
Hepatorenal syndrome | Impaired sitting balance |
Hepatic encephalopathy | Karnofsky < 50% |
Spontaneous bacterial peritonitis | Recurrent aspiration |
Recurrent variceal bleed | Age > 70 |
Co‐morbid conditions as listed in Renal | Co‐morbid conditions as listed in Renal |
HIV/AIDS | Neuromuscular |
Persistent decline in function | Diminished respiratory function |
Chronic diarrhea 1 year | Chosen not to receive BiPAP/vent |
Decision to stop treatment | Difficulty swallowing |
CNS lymphoma | Diminished functional status |
MAC‐untreated | Incontinence |
Systemic lymphoma | Co‐morbid conditions as listed in Renal |
Dilated cardiomyopathy | |
CD4 < 25 with disease progression | |
Viral load > 100,000 |
Safety‐Net Hospital Cohort, N=568 | Academic Center Cohort, N=496 | Study Cohort,N=1064 | Original CARING Cohort, N=8739 | |
---|---|---|---|---|
| ||||
Mean age ( SD), y | 47.8 (16.5) | 54.4 (17.5) | 50.9 (17.3) | 63 (13) |
Male gender | 59.5% (338) | 50.1% (248) | 55.1% (586) | 98% (856) |
Ethnicity | ||||
African American | 14.1% (80) | 13.5% (65) | 13.8% (145) | 13% (114) |
Asian | 0.4% (2) | 1.5% (7) | 0.9% (9) | Not reported |
Caucasian | 41.7% (237) | 66.3% (318) | 53.0 % (555) | 69% (602) |
Latino | 41.9% (238) | 9.6% (46) | 27.1% (284) | 8% (70) |
Native American | 0.5% (3) | 0.4% (2) | 0.5% (5) | Not reported |
Other | 0.5% (3) | 0.6% (3) | 0.6% (6) | 10% (87) |
Unknown | 0.9% (5) | 8.1% (39) | 4.2% (44) | Not reported |
CARING criteria | ||||
Cancer | 6.2% (35) | 19.4% (96) | 12.3% (131) | 23% (201) |
Admissions to the hospital 2 in past year | 13.6% (77) | 42.7% (212) | 27.2% (289) | 36% (314) |
Resident in a nursing home | 1.8% (10) | 3.4% (17) | 2.5% (27) | 3% (26) |
ICU with MOF | 3.7% (21) | 1.2% (6) | 2.5% (27) | 2% (17) |
NHPCO (2) noncancer guidelines | 1.6% (9) | 5.9% (29) | 3.6% (38) | 8% (70) |
Reliability testing demonstrated excellent inter‐rater reliability. Kappa for each criterion is as follows: (1) primary diagnosis of cancer=1.0, (2) 2 admissions to the hospital in the past year=0.91, (3) resident in a nursing home=1.0, (4) ICU admission with MOF=1.0, and (5) 2 noncancer hospice guidelines=0.78.
This study aimed to validate the CARING criteria9; therefore, all original individual CARING criterion were included in the validation logistic regression models. The 1 exception to this was in the university hospital study cohort, where the ICU criterion was excluded from the model due to small sample size and quasiseparation in the model. The model results are presented in Table 3 for the individual hospitals and combined study cohort.
Safety Net Hospital Cohort, C Index=0.76 | Academic Center Cohort, C Index=0.76 | Combined Hospital Cohort, C Index=0.79 | ||||
---|---|---|---|---|---|---|
Estimate | Odds Ratio (95% CI) | Estimate | Odds Ratio (95% CI) | Estimate | Odds Ratio (95% CI) | |
| ||||||
Cancer | 1.92 | 6.85 (2.83‐16.59)a | 1.85 | 6.36 (3.54‐11.41)a | 1.98 | 7.23 (4.45‐11.75)a |
Admissions to the hospital 2 in past year | 0.55 | 1.74 (0.76‐3.97) | 0.14 | 0.87 (0.51‐1.49) | 0.20 | 1.22 (0.78‐1.91) |
Resident in a nursing home | 0.49 | 0.61 (0.06‐6.56) | 0.27 | 1.31 (0.37‐4.66) | 0.09 | 1.09 (0.36‐3.32) |
ICU with MOF | 1.85 | 6.34 (2.0219.90)a | 1.94 | 6.97 (2.75‐17.68)a | ||
NHPCO (2) noncancer guidelines | 3.04 | 20.86 (4.25102.32)a | 2.62 | 13.73 (5.86‐32.15)a | 2.74 | 15.55 (7.2833.23)a |
Ageb | 0.38 | 1.46 (1.05‐2.03)a | 0.45 | 1.56 (1.23‐1.98)a | 0.47 | 1.60 (1.32‐1.93)a |
In the safety‐net hospital, admission to the hospital with a primary diagnosis related to cancer, 2 noncancer hospice guidelines, ICU admission with MOF, and age by category all were significant predictors of 1‐year mortality. In the university hospital cohort, primary diagnosis of cancer, 2 noncancer hospice guidelines, and age by category were predictive of 1‐year mortality. Finally, in the entire study cohort, primary diagnosis of cancer, ICU with MOF, 2 noncancer hospice guidelines, and age were all predictive of 1‐year mortality. Parameter estimates were similar in 3 of the criteria compared to the VA setting. Differences in patient characteristics may have caused the differences in the estimates. Gender was additionally tested but not significant in any model. One‐year survival was significantly lower for those who met 1 of the CARING criteria versus those who did not (Figure 1).
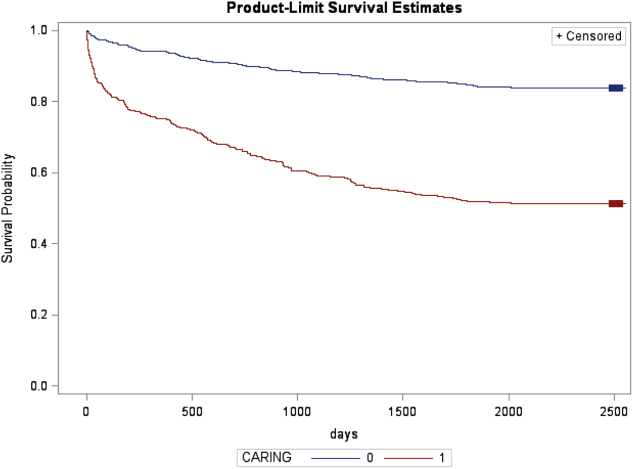
Based on the framework from the original CARING criteria analysis, a scoring rule was developed using the regression results of this validation cohort. To predict a high probability of 1‐year mortality, sensitivity was set to 58% and specificity was set at 86% (error rate=17%). Medium to high probability was set with a sensitivity of 73% and specificity of 72% (error rate=28%). The coefficients from the regression model of the entire study cohort were converted to scores for each of the CARING criteria. The scores are as follows: 0.5 points for admission from a nursing home, 1 point for 2 hospital admissions in the past year for a chronic illness, 10 points for primary diagnosis of cancer, 10 points for ICU admission with MOF, and 14 points for 2 noncancer hospice guidelines. For every age category increase, 2 points are assigned so that 0 points for age <55 years, 2 points for ages 56 to 65 years, 4 points for ages 66 to 75 years, and 6 points for >75 years. Points for individual risk factors were proportional to s (ie, log odds) in the logistic regression model for death at 1 year. Although no linear transformation exists between s and probabilities (of death at 1 year), the aggregated points for combinations of risk factors shown in Table 4 follow the probabilities in an approximately linear fashion, so that different degrees of risk of death can be represented contiguously (as highlighted by differently shaded regions in the scoring matrix) (Table 4). The scoring matrix allows for quick identification for patients at high risk for 1‐year mortality. In this non‐VA setting with healthier patients, low risk is defined at a lower probability threshold (0.1) compared to the VA setting (0.175).
CARING Criteria Components | |||||||
---|---|---|---|---|---|---|---|
None | Resident in a Nursing Home | Admitted to the Hospital 2 Times in the Past Year | Resident in a Nursing Home Admitted to the Hospital 2 Times in the Past Year | Primary Diagnosis of Cancer | ICU Admission With MOF | Noncancer Hospice Guidelines | |
| |||||||
Age | |||||||
55 years | 0 | 0.5 | 1 | 1.5 | 10 | ||
5565 years | 2 | 2.5 | 3 | 3.5 | 10 | ||
6675 years | 4 | 4.5 | 5 | 5.5 | 10 | ||
>75 years | 6 | 6.5 | 7 | 7.5 | 10 | ||
Risk | |||||||
Low | 3.5 | Probability<0.1 | |||||
Medium | 46.5 | 0.1probability <0.175 | |||||
High | 7 | Probability0.175 |
DISCUSSION
The CARING criteria are a practical prognostic tool that can be easily and rapidly applied to patients admitted to the hospital to estimate risk of death in 1 year, with the goal of identifying patients who may benefit most from incorporating palliative interventions into their plan of care. This study validated the CARING criteria in a tertiary referral university hospital and safety‐net hospital setting, demonstrating applicability in a much broader population than the VA hospital of the original CARING criteria study. The population studied represented a younger population by over 10 years, a more equitable proportion of males to females, a broader ethnic diversity, and lower 1‐year deaths rates than the original study. Despite the broader representation of the population, the significance of each of the individual CARING criterion was maintained except for 2 hospital admissions in the past year for a chronic illness (admission from a nursing home did not meet significance in either study as a sole criterion). As with the original study, meeting 2 of the NHPCO noncancer hospice guidelines demonstrated the highest risk of 1‐year mortality following index hospitalization, followed by primary diagnosis of cancer and ICU admission with MOF. Advancing age, also similar to the original study, conferred increased risk across the criterion.
Hospitalists could be an effective target for utilizing the CARING criteria because they are frequently the first‐line providers in the hospital setting. With the national shortage of palliative care specialists, hospitalists need to be able to identify when a patient has a limited life expectancy so they will be better equipped to make clinical decisions that are aligned with their patients' values, preferences, and goals of care. With the realization that not addressing advance care planning and patient goals of care may be considered medical errors, primary palliative care skills become alarmingly more important as priorities for hospitalists to obtain and feel comfortable using in daily practice.
The CARING criteria are directly applicable to patients who are seen by hospitalists. Other prognostic indices have focused on select patient populations, such as the elderly,[10, 11, 12] require collection of data that are not readily available on admission or would not otherwise be obtained,[10, 13] or apply to patients post‐hospital discharge, thereby missing the opportunity to make an impact earlier in the disease trajectory and incorporate palliative care into the hospital plan of care when key discussions about goals of care and preferences should be encouraged.
Additionally, the CARING criteria could easily be incorporated as a trigger for palliative care consults on hospital admission. Palliative care consults tend to happen late in a hospital stay, limiting the effectiveness of the palliative care team. A trigger system for hospitalists and other primary providers on hospital admission would lend to more effective timing of palliative measures being incorporated into the plan of care. Palliative care consults would not only be initiated earlier, but could be targeted for the more complex and sick patients with the highest risk of death in the next year.
In the time‐pressured environment, the presence of any 1 of the CARING criteria can act as a trigger to begin incorporating primary palliative care measures into the plan of care. The admitting hospitalist provider (ie, physician, nurse practitioner, physician assistant) could access the CARING criteria through an electronic health record prompt when admitting patients. When a more detailed assessment of mortality risk is helpful, the hospitalist can use the scoring matrix, which combines age with the individual criterion to calculate patients at medium or high risk of death within 1 year. Limited resources can then be directed to the patients with the greatest need. Patients with a focused care need, such as advance care planning or hospice referral, can be directed to the social worker or case manager. More complicated patients may be referred to a specialty palliative care team.
Several limitations to this study are recognized, including the small sample size of patients meeting criterion for ICU with MOF in the academic center study cohort. The patient data were collected during a transition time when the university hospital moved to a new campus, resulting in an ICU at each campus that housed patients with differing levels of illness severity, which may have contributed to the lower acuity ICU patient observed. Although we advocate the simplicity of the CARING criteria, the NHPCO noncancer hospice guidelines are more complicated, as they incorporates 8 broad categories of chronic illness. The hospice guidelines may not be general knowledge to the hospitalist or other primary providers. ePrognosis (
CONCLUSION
The CARING criteria are a simple, practical prognostic tool predictive of death within 1 year that has been validated in a broad population of hospitalized patients. The criteria hold up in a younger, healthier population that is more diverse by age, gender, and ethnicity than the VA population. With ready access to critical prognostic information on hospital admission, clinicians will be better informed to make decisions that are aligned with their patients' values, preferences, and goals of care.
Disclosure
Nothing to report.
Prognostication continues to be a challenge to the clinician despite over 100 prognostic indices that have been developed during the past few decades to inform clinical practice and medical decision making.[1] Physicians are not accurate in prognostication of patients' risk of death and tend to overestimate survival.[2, 3] In addition, many physicians do not feel comfortable offering a prognosis to patients, despite patients' wishes to be informed.[4, 5] Regardless of the prevalence in the literature and value in improving physicians' prognostic accuracy, prognostic indices of survival are not regularly utilized in the hospital setting. Prognostic tools available for providers are often complicated and may require data about patients that are not readily available.[6, 7, 8] Prognostic indices may be too specific to a patient population, too difficult to remember, or too time consuming to use. A simple, rapid, and practical prognostic index is important in the hospital setting to assist in identifying patients at high risk of death so that primary palliative interventions can be incorporated into the plan of care early in the hospital stay. Patient and family education, advance care planning, formulating the plan of care based on patientfamily goals, and improved resource utilization could be better executed by more accurate risk of death prediction on hospital admission.
The CARING criteria are the only prognostic index to our knowledge that evaluates a patient's risk of death in the next year, with information readily available at the time of hospital admission (Table 1).[9] The CARING criteria are a unique prognostic tool: (1) CARING is a mnemonic acronym, making it more user friendly to the clinician. (2) The 5 prognostic indicators are readily available from the patient's chart on admission; gathering further data by patient or caretaker interviews or by obtaining laboratory data is not needed. (3) The timing for application of the tool on admission to the hospital is an ideal opportunity to intervene and introduce palliative interventions early on the hospital stay. The CARING criteria were developed and validated in a Veteran's Administration hospital setting by Fischer et al.[9] We sought to validate the CARING criteria in a broader patient populationmedical and surgical patients from a tertiary referral university hospital setting and a safety‐net hospital setting.
METHODS
Study Design
This study was a retrospective observational cohort study. The study was approved by the Colorado Multiple Institutional Review Board and the University of Colorado Hospital Research Review Committee.
Study Purpose
To validate the CARING criteria in a tertiary referral university hospital (University of Colorado Hospital [UCH]) and safety‐net hospital (Denver Health and Hospitals [DHH]) setting using similar methodology to that employed by the original CARING criteria study.[9]
Study Setting/Population
All adults (18 years of age) admitted as inpatients to the medical and surgical services of internal medicine, hospitalist, pulmonary, cardiology, hematology/oncology, hepatology, surgery, intensive care unit, and intermediary care unit at UCH and DHH during the study period of July 2005 through August 2005. The only exclusion criteria were those patients who were prisoners or pregnant. Administrative admission data from July 2005 to August 2005 were used to identify names of all persons admitted to the medicine and surgical services of the study hospitals during the specified time period.
The 2 study hospitals, UCH and DHH, provide a range of patients who vary in ethnicity, socioeconomic status, and medical illness. This variability allows for greater generalizability of the results. Both hospitals are affiliated with the University of Colorado School of Medicine internal medicine residency training program and are located in Denver, Colorado.
At the time of the study, UCH was a licensed 550‐bed tertiary referral, academic hospital serving the Denver metropolitan area and the Rocky Mountain region as a specialty care and referral center. DHH was a 398‐bed, academic, safety‐net hospital serving primarily the Denver metropolitan area. DHH provides 42% of the care for the uninsured in Denver and 26% of the uninsured care for the state of Colorado.
Measures
The CARING criteria were developed and validated in a Veteran's Administration (VA) hospital setting by Fischer et al.[9] The purpose of the CARING criteria is to identify patients, at the time of hospital admission, who are at higher risk of death in the following year. The prognostic index uses 5 predictors that can be abstracted from the chart at time of admission. The CARING criteria were developed a priori, and patients were evaluated using only the medical data available at the time of admission. The criteria include items that are already part of the routine physician admission notes and do not require additional data collection or assessments. The criteria include: C=primary diagnosis of cancer, A=2 admissions to the hospital for a chronic illness within the last year; R=resident in a nursing home; I=intensive care unit (ICU) admission with multiorgan failure (MOF), NG=noncancer hospice guidelines (meeting 2 of the National Hospice and Palliative Care Organization's [NHPCO] guidelines).
Patients were identified using name, date of birth, social security number, address, and phone number. This identifying information was then used for tracing death records 1 year after hospital admission.
Mortality at 1 year following the index hospitalization was the primary end point. To minimize missing data and the number of subjects lost to follow‐up, 3 determinants of mortality were used. First, electronic medical records of the 2 participating hospitals and their outpatient clinics were reviewed to determine if a follow‐up appointment had occurred past the study's end point of 1 year (August 2006). For those without a confirmed follow‐up visit, death records from the Colorado Department of Public Health and Vital Records were obtained. For those patients residing outside of Colorado or whose mortality status was still unclear, the National Death Index was accessed.
Medical Record Review
Medical records for all study participants were reviewed by J.Y. (UCH) and B.C. (DHH). Data collection was completed using direct data entry into a Microsoft Access (Microsoft Corp., Redmond, WA) database utilizing a data entry form linked with the database table. This form utilized skip patterns and input masks to ensure quality of data entry and minimize missing or invalid data. Inter‐rater reliability was assessed by an independent rereview (S.F.) of 5% of the total charts. Demographic variables were collected using hospital administrative data. These included personal identifiers of the participants for purposes of mortality follow‐up. Clinical data including the 5 CARING variables and additional descriptive variables were abstracted from the paper hospital chart and the electronic record of the chart (together these constitute the medical record).
Death Follow‐up
A search of Colorado death records was conducted in February 2011 for all subjects. Death records were used to determine mortality and time to death from the index hospitalization. The National Death Index was then searched for any subjects without or record of death in Colorado.
Analysis
All analyses were conducted using the statistical application software SAS for Windows version 9.3 (SAS Institute, Cary, NC). Simple frequencies and means ( standard deviation) were used to describe the baseline characteristics. Multiple logistic regression models were used to model 1‐year mortality. The models were fitted using all of the CARING variables and age. As the aim of the study was to validate the CARING criteria, the variables for the models were selected a priori based on the original index. Two hospital cohorts (DHH and UCH) were modeled separately and as a combined sample. Kaplan‐Meier survival analysis was conducted to compare those subjects who met 1 of the CARING criteria with those who did not through the entire period of mortality follow‐up (20052011). Finally, using the probabilities from the logistic regression models, we again developed a scoring rule appropriate for a non‐VA setting to allow clinicians to easily identify patient risk for 1‐year mortality at the time of hospital admission.
RESULTS
There were a total of 1064 patients admitted to the medical and surgical services during the study period568 patients at DHH and 496 patients at UCH. Sample characteristics of each individual hospital cohort and the entire combined study cohort are detailed in Table 2. Overall, slightly over half the population were male, with a mean age of 50 years, and the ethnic breakdown roughly reflects the population in Denver. A total of 36.5% (n=388) of the study population met 1 of the CARING criteria, and 12.6% (n=134 among 1063 excluding 1 without an admit date) died within 1 year of the index hospitalization. These were younger and healthier patients compared to the VA sample used in developing the CARING criteria.
| |
Renal | Dementia |
Stop/decline dialysis | Unable to ambulate independently |
Not candidate for transplant | Urinary or fecal incontinence |
Urine output < 40cc/24 hours | Unable to speak with more than single words |
Creatinine > 8.0 (>6.0 for diabetics) | Unable to bathe independently |
Creatinine clearance 10cc/min | Unable to dress independently |
Uremia | Co‐morbid conditions: |
Persistent serum K + > 7.0 | Aspiration pneumonia |
Co‐morbid conditions: | Pyelonephritis |
Cancer CHF | Decubitus ulcer |
Chronic lung disease AIDS/HIV | Difficulty swallowing or refusal to eat |
Sepsis Cirrhosis | |
Cardiac | Pulmonary |
Ejection fraction < 20% | Dyspnea at rest |
Symptomatic with diuretics and vasodilators | FEV1 < 30% |
Not candidate for transplant | Frequent ER or hospital admits for pulmonary infections or respiratory distress |
History of cardiac arrest | Cor pulmonale or right heart failure |
History of syncope | 02 sat < 88% on 02 |
Systolic BP < 120mmHG | PC02 > 50 |
CVA cardiac origin | Resting tachycardia > 100/min |
Co‐morbid conditions as listed in Renal | Co‐morbid conditions as listed in Renal |
Liver | Stroke/CVA |
End stage cirrhosis | Coma at onset |
Not candidate for transplant | Coma >3 days |
Protime > 5sec and albumin <2.5 | Limb paralysis |
Ascites unresponsive to treatment | Urinary/fecal incontinence |
Hepatorenal syndrome | Impaired sitting balance |
Hepatic encephalopathy | Karnofsky < 50% |
Spontaneous bacterial peritonitis | Recurrent aspiration |
Recurrent variceal bleed | Age > 70 |
Co‐morbid conditions as listed in Renal | Co‐morbid conditions as listed in Renal |
HIV/AIDS | Neuromuscular |
Persistent decline in function | Diminished respiratory function |
Chronic diarrhea 1 year | Chosen not to receive BiPAP/vent |
Decision to stop treatment | Difficulty swallowing |
CNS lymphoma | Diminished functional status |
MAC‐untreated | Incontinence |
Systemic lymphoma | Co‐morbid conditions as listed in Renal |
Dilated cardiomyopathy | |
CD4 < 25 with disease progression | |
Viral load > 100,000 |
Safety‐Net Hospital Cohort, N=568 | Academic Center Cohort, N=496 | Study Cohort,N=1064 | Original CARING Cohort, N=8739 | |
---|---|---|---|---|
| ||||
Mean age ( SD), y | 47.8 (16.5) | 54.4 (17.5) | 50.9 (17.3) | 63 (13) |
Male gender | 59.5% (338) | 50.1% (248) | 55.1% (586) | 98% (856) |
Ethnicity | ||||
African American | 14.1% (80) | 13.5% (65) | 13.8% (145) | 13% (114) |
Asian | 0.4% (2) | 1.5% (7) | 0.9% (9) | Not reported |
Caucasian | 41.7% (237) | 66.3% (318) | 53.0 % (555) | 69% (602) |
Latino | 41.9% (238) | 9.6% (46) | 27.1% (284) | 8% (70) |
Native American | 0.5% (3) | 0.4% (2) | 0.5% (5) | Not reported |
Other | 0.5% (3) | 0.6% (3) | 0.6% (6) | 10% (87) |
Unknown | 0.9% (5) | 8.1% (39) | 4.2% (44) | Not reported |
CARING criteria | ||||
Cancer | 6.2% (35) | 19.4% (96) | 12.3% (131) | 23% (201) |
Admissions to the hospital 2 in past year | 13.6% (77) | 42.7% (212) | 27.2% (289) | 36% (314) |
Resident in a nursing home | 1.8% (10) | 3.4% (17) | 2.5% (27) | 3% (26) |
ICU with MOF | 3.7% (21) | 1.2% (6) | 2.5% (27) | 2% (17) |
NHPCO (2) noncancer guidelines | 1.6% (9) | 5.9% (29) | 3.6% (38) | 8% (70) |
Reliability testing demonstrated excellent inter‐rater reliability. Kappa for each criterion is as follows: (1) primary diagnosis of cancer=1.0, (2) 2 admissions to the hospital in the past year=0.91, (3) resident in a nursing home=1.0, (4) ICU admission with MOF=1.0, and (5) 2 noncancer hospice guidelines=0.78.
This study aimed to validate the CARING criteria9; therefore, all original individual CARING criterion were included in the validation logistic regression models. The 1 exception to this was in the university hospital study cohort, where the ICU criterion was excluded from the model due to small sample size and quasiseparation in the model. The model results are presented in Table 3 for the individual hospitals and combined study cohort.
Safety Net Hospital Cohort, C Index=0.76 | Academic Center Cohort, C Index=0.76 | Combined Hospital Cohort, C Index=0.79 | ||||
---|---|---|---|---|---|---|
Estimate | Odds Ratio (95% CI) | Estimate | Odds Ratio (95% CI) | Estimate | Odds Ratio (95% CI) | |
| ||||||
Cancer | 1.92 | 6.85 (2.83‐16.59)a | 1.85 | 6.36 (3.54‐11.41)a | 1.98 | 7.23 (4.45‐11.75)a |
Admissions to the hospital 2 in past year | 0.55 | 1.74 (0.76‐3.97) | 0.14 | 0.87 (0.51‐1.49) | 0.20 | 1.22 (0.78‐1.91) |
Resident in a nursing home | 0.49 | 0.61 (0.06‐6.56) | 0.27 | 1.31 (0.37‐4.66) | 0.09 | 1.09 (0.36‐3.32) |
ICU with MOF | 1.85 | 6.34 (2.0219.90)a | 1.94 | 6.97 (2.75‐17.68)a | ||
NHPCO (2) noncancer guidelines | 3.04 | 20.86 (4.25102.32)a | 2.62 | 13.73 (5.86‐32.15)a | 2.74 | 15.55 (7.2833.23)a |
Ageb | 0.38 | 1.46 (1.05‐2.03)a | 0.45 | 1.56 (1.23‐1.98)a | 0.47 | 1.60 (1.32‐1.93)a |
In the safety‐net hospital, admission to the hospital with a primary diagnosis related to cancer, 2 noncancer hospice guidelines, ICU admission with MOF, and age by category all were significant predictors of 1‐year mortality. In the university hospital cohort, primary diagnosis of cancer, 2 noncancer hospice guidelines, and age by category were predictive of 1‐year mortality. Finally, in the entire study cohort, primary diagnosis of cancer, ICU with MOF, 2 noncancer hospice guidelines, and age were all predictive of 1‐year mortality. Parameter estimates were similar in 3 of the criteria compared to the VA setting. Differences in patient characteristics may have caused the differences in the estimates. Gender was additionally tested but not significant in any model. One‐year survival was significantly lower for those who met 1 of the CARING criteria versus those who did not (Figure 1).
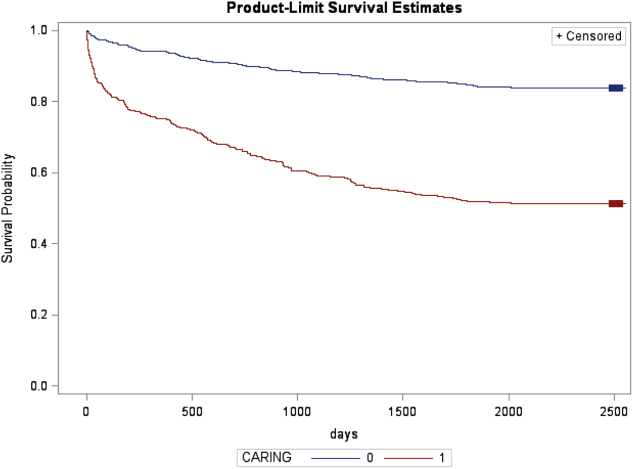
Based on the framework from the original CARING criteria analysis, a scoring rule was developed using the regression results of this validation cohort. To predict a high probability of 1‐year mortality, sensitivity was set to 58% and specificity was set at 86% (error rate=17%). Medium to high probability was set with a sensitivity of 73% and specificity of 72% (error rate=28%). The coefficients from the regression model of the entire study cohort were converted to scores for each of the CARING criteria. The scores are as follows: 0.5 points for admission from a nursing home, 1 point for 2 hospital admissions in the past year for a chronic illness, 10 points for primary diagnosis of cancer, 10 points for ICU admission with MOF, and 14 points for 2 noncancer hospice guidelines. For every age category increase, 2 points are assigned so that 0 points for age <55 years, 2 points for ages 56 to 65 years, 4 points for ages 66 to 75 years, and 6 points for >75 years. Points for individual risk factors were proportional to s (ie, log odds) in the logistic regression model for death at 1 year. Although no linear transformation exists between s and probabilities (of death at 1 year), the aggregated points for combinations of risk factors shown in Table 4 follow the probabilities in an approximately linear fashion, so that different degrees of risk of death can be represented contiguously (as highlighted by differently shaded regions in the scoring matrix) (Table 4). The scoring matrix allows for quick identification for patients at high risk for 1‐year mortality. In this non‐VA setting with healthier patients, low risk is defined at a lower probability threshold (0.1) compared to the VA setting (0.175).
CARING Criteria Components | |||||||
---|---|---|---|---|---|---|---|
None | Resident in a Nursing Home | Admitted to the Hospital 2 Times in the Past Year | Resident in a Nursing Home Admitted to the Hospital 2 Times in the Past Year | Primary Diagnosis of Cancer | ICU Admission With MOF | Noncancer Hospice Guidelines | |
| |||||||
Age | |||||||
55 years | 0 | 0.5 | 1 | 1.5 | 10 | ||
5565 years | 2 | 2.5 | 3 | 3.5 | 10 | ||
6675 years | 4 | 4.5 | 5 | 5.5 | 10 | ||
>75 years | 6 | 6.5 | 7 | 7.5 | 10 | ||
Risk | |||||||
Low | 3.5 | Probability<0.1 | |||||
Medium | 46.5 | 0.1probability <0.175 | |||||
High | 7 | Probability0.175 |
DISCUSSION
The CARING criteria are a practical prognostic tool that can be easily and rapidly applied to patients admitted to the hospital to estimate risk of death in 1 year, with the goal of identifying patients who may benefit most from incorporating palliative interventions into their plan of care. This study validated the CARING criteria in a tertiary referral university hospital and safety‐net hospital setting, demonstrating applicability in a much broader population than the VA hospital of the original CARING criteria study. The population studied represented a younger population by over 10 years, a more equitable proportion of males to females, a broader ethnic diversity, and lower 1‐year deaths rates than the original study. Despite the broader representation of the population, the significance of each of the individual CARING criterion was maintained except for 2 hospital admissions in the past year for a chronic illness (admission from a nursing home did not meet significance in either study as a sole criterion). As with the original study, meeting 2 of the NHPCO noncancer hospice guidelines demonstrated the highest risk of 1‐year mortality following index hospitalization, followed by primary diagnosis of cancer and ICU admission with MOF. Advancing age, also similar to the original study, conferred increased risk across the criterion.
Hospitalists could be an effective target for utilizing the CARING criteria because they are frequently the first‐line providers in the hospital setting. With the national shortage of palliative care specialists, hospitalists need to be able to identify when a patient has a limited life expectancy so they will be better equipped to make clinical decisions that are aligned with their patients' values, preferences, and goals of care. With the realization that not addressing advance care planning and patient goals of care may be considered medical errors, primary palliative care skills become alarmingly more important as priorities for hospitalists to obtain and feel comfortable using in daily practice.
The CARING criteria are directly applicable to patients who are seen by hospitalists. Other prognostic indices have focused on select patient populations, such as the elderly,[10, 11, 12] require collection of data that are not readily available on admission or would not otherwise be obtained,[10, 13] or apply to patients post‐hospital discharge, thereby missing the opportunity to make an impact earlier in the disease trajectory and incorporate palliative care into the hospital plan of care when key discussions about goals of care and preferences should be encouraged.
Additionally, the CARING criteria could easily be incorporated as a trigger for palliative care consults on hospital admission. Palliative care consults tend to happen late in a hospital stay, limiting the effectiveness of the palliative care team. A trigger system for hospitalists and other primary providers on hospital admission would lend to more effective timing of palliative measures being incorporated into the plan of care. Palliative care consults would not only be initiated earlier, but could be targeted for the more complex and sick patients with the highest risk of death in the next year.
In the time‐pressured environment, the presence of any 1 of the CARING criteria can act as a trigger to begin incorporating primary palliative care measures into the plan of care. The admitting hospitalist provider (ie, physician, nurse practitioner, physician assistant) could access the CARING criteria through an electronic health record prompt when admitting patients. When a more detailed assessment of mortality risk is helpful, the hospitalist can use the scoring matrix, which combines age with the individual criterion to calculate patients at medium or high risk of death within 1 year. Limited resources can then be directed to the patients with the greatest need. Patients with a focused care need, such as advance care planning or hospice referral, can be directed to the social worker or case manager. More complicated patients may be referred to a specialty palliative care team.
Several limitations to this study are recognized, including the small sample size of patients meeting criterion for ICU with MOF in the academic center study cohort. The patient data were collected during a transition time when the university hospital moved to a new campus, resulting in an ICU at each campus that housed patients with differing levels of illness severity, which may have contributed to the lower acuity ICU patient observed. Although we advocate the simplicity of the CARING criteria, the NHPCO noncancer hospice guidelines are more complicated, as they incorporates 8 broad categories of chronic illness. The hospice guidelines may not be general knowledge to the hospitalist or other primary providers. ePrognosis (
CONCLUSION
The CARING criteria are a simple, practical prognostic tool predictive of death within 1 year that has been validated in a broad population of hospitalized patients. The criteria hold up in a younger, healthier population that is more diverse by age, gender, and ethnicity than the VA population. With ready access to critical prognostic information on hospital admission, clinicians will be better informed to make decisions that are aligned with their patients' values, preferences, and goals of care.
Disclosure
Nothing to report.
- Predicting death: an empirical evaluation of predictive tools for mortality. Arch Intern Med. 2011;171:1721–1726. , , .
- Extent and determinants of error in physicians' prognoses in terminally ill patients: prospective cohort study. West J Med. 2000;172:310–313. , .
- A systematic review of physicians' survival predictions in terminally ill cancer patients. BMJ. 2003;327:195–198. , , , et al.
- Attitude and self‐reported practice regarding prognostication in a national sample of internists. Arch Intern Med. 1998;158:2389–2395. , .
- Discussing prognosis: balancing hope and realism. Cancer J. 2010;16:461–466. , , , et al.
- Acute Physiology and Chronic Health Evaluation (APACHE) IV: hospital mortality assessment for today's critically ill patients. Crit Care Med. 2006;34:1297–1310. , , , .
- SAPS 3 admission score: an external validation in a general intensive care population. Intensive Care Med. 2008;34:1873–1877. , , , , .
- Prospective validation of the intensive care unit admission Mortality Probability Model (MPM0‐III). Crit Care Med. 2009;37:1619–1623. , , , , , .
- A practical tool to identify patients who may benefit from a palliative approach: the CARING criteria. J Pain Symptom Manage. 2006;31:285–292. , , , , , .
- Prediction of survival for older hospitalized patients: the HELP survival model. J Am Geriatr Soc. 2000;48:S16–S24. , , , et al.
- Development and validation of a multidimensional prognostic index for one‐year mortality from comprehensive geriatric assessment in hospitalized older patients. Rejuvenation Res. 2008;11:151–161. , , , et al.
- Burden of illness score for elderly persons: risk adjustment incorporating the cumulative impact of diseases, physiologic abnormalities, and functional impairments. Med Care. 2003;41:70–83. , , , et al.
- The SUPPORT prognostic model. Objective estimates of survival for seriously ill hospitalized adults. Study to understand prognoses and preferences for outcomes and risks of treatments. Ann Intern Med. 1995;122:191–203. , , , et al.
- Predicting death: an empirical evaluation of predictive tools for mortality. Arch Intern Med. 2011;171:1721–1726. , , .
- Extent and determinants of error in physicians' prognoses in terminally ill patients: prospective cohort study. West J Med. 2000;172:310–313. , .
- A systematic review of physicians' survival predictions in terminally ill cancer patients. BMJ. 2003;327:195–198. , , , et al.
- Attitude and self‐reported practice regarding prognostication in a national sample of internists. Arch Intern Med. 1998;158:2389–2395. , .
- Discussing prognosis: balancing hope and realism. Cancer J. 2010;16:461–466. , , , et al.
- Acute Physiology and Chronic Health Evaluation (APACHE) IV: hospital mortality assessment for today's critically ill patients. Crit Care Med. 2006;34:1297–1310. , , , .
- SAPS 3 admission score: an external validation in a general intensive care population. Intensive Care Med. 2008;34:1873–1877. , , , , .
- Prospective validation of the intensive care unit admission Mortality Probability Model (MPM0‐III). Crit Care Med. 2009;37:1619–1623. , , , , , .
- A practical tool to identify patients who may benefit from a palliative approach: the CARING criteria. J Pain Symptom Manage. 2006;31:285–292. , , , , , .
- Prediction of survival for older hospitalized patients: the HELP survival model. J Am Geriatr Soc. 2000;48:S16–S24. , , , et al.
- Development and validation of a multidimensional prognostic index for one‐year mortality from comprehensive geriatric assessment in hospitalized older patients. Rejuvenation Res. 2008;11:151–161. , , , et al.
- Burden of illness score for elderly persons: risk adjustment incorporating the cumulative impact of diseases, physiologic abnormalities, and functional impairments. Med Care. 2003;41:70–83. , , , et al.
- The SUPPORT prognostic model. Objective estimates of survival for seriously ill hospitalized adults. Study to understand prognoses and preferences for outcomes and risks of treatments. Ann Intern Med. 1995;122:191–203. , , , et al.
© 2013 Society of Hospital Medicine
Low Concordance for Site of Death
At the turn of the 20th century, most deaths in the United States occurred at home. By the 1960s, over 70% of deaths occurred in an institutional setting, reflecting an evolution of medical technology.[1, 2, 3] With the birth of the hospice movement in the 1970s, dying patients had the opportunity to have both death at home and aggressive symptom control at the end of life. Although there has been a slow decline in the proportion of deaths that occur in the hospital over the past 2 decades,[3] the overwhelming majority of persons state that they would prefer to die at home. However, recent findings suggest that most people will die in an institutional setting.[3, 4, 5, 6]
Although good data exist describing population preferences for location of death, and we know, based on death records, where deaths occur in the United States, there are few studies that examine concordance between preferred and actual site of death at the individual patient level. Furthermore, although factors have been identified that predict death at home, factors predicting concordance between preferred and actual site of death are not well described.[3, 6, 7, 8, 9, 10, 11, 12, 13]
Regardless of where death ultimately occurs, most adults will experience multiple hospitalizations within the last years of their life. Understanding the preferences and subsequent experiences of this population is of particular relevance to hospitalist physicians who are in a unique position to elicit goals from seriously ill patients and help match patient preferences with their medical care. In this observational study, we sought to determine preferences for site of death in a cohort of adult patients admitted to the hospital for medical illness, and then follow those patients to determine where death occurred for those who died. We also sought to explore factors that may predict concordance between preferred and actual site of death. We hypothesized that ethnic diversity and lower socioeconomic status would be associated with a lower likelihood of concordance between preferred and actual site of death. We also hypothesized that advanced care planning would be associated with a higher likelihood of concordance. The Colorado Multi‐Institutional Review Board approved this study.
METHODS
Participants were recruited from 3 hospitals affiliated with the University of Colorado School of Medicine Internal Medicine Residency program, including the Denver Veterans' Administration Center (DVAMC), Denver Health Medical Center (DHMC), and University of Colorado Hospital (UCH). The DVAMC is a large urban Veterans Administration hospital, serving veterans from the Denver metro area, and is a tertiary referral center for veterans in rural Colorado, Wyoming, and parts of Montana. DHMC, the safety‐net hospital for the Denver area, serves over 25% of the residents in the city and county of Denver, including such special populations as the indigent, chronically mentally ill, and persons with polysubstance dependence. UCH had 350 licensed beds at the time of our study and serves as the Rocky Mountain region's only academic tertiary, specialty care, and referral center. At the time of this study, there was limited inpatient palliative care services at the DVAMC and UH, and no palliative care services at DHMC. Participants were screened on the first day following admission to the adult general medical service. Participants were recruited on 96 postadmission days between February 2004 and June 2006. Recruitment days varied from Monday through Friday, to include admissions from the weekend and throughout the year to reduce potential bias due to seasonal trends of diseases such as influenza. Patients were excluded if they died or were discharged within the first 24 hours of admission, were pregnant, jailed, or unable to give informed consent. All other patients were approached and invited to participate in a brief survey.
After informed consent was obtained, participants completed a bedside interview that included self‐identified ethnicity and the Berkman‐Syme Social Network Index,[14] a brief questionnaire quantifying social support from spouse or domestic partner, family, friends, and other religious or secular organizations. Baseline socioeconomic measures (eg, income, employment, home ownership, car ownership) and questions related to the last days of life were also included. Participants were asked the following question, If you were very sick, with an illness that could not be cured, and in bed most of the time, where would you spend the last days of your life if you could chose?
For each participant, we performed a detailed chart review to determine demographic data, presence of advance directives, and CARING criteria (Cancer, Admissions 2, Residence in a nursing home, Intensive care unit admit with multiorgan failure, 2 Noncancer hospice Guidelines), a set of prognostic criteria identifying patients at an index hospitalization who have a high burden of illness and are at risk for death in the following year.[15] We then followed patients for 5 years. If participants died within the follow‐up period, we collected the date and location of death using medical records, death certificates, or in a few cases when official death records were unavailable, direct contact with the family. Participants were considered alive if they had a clinic visit or MD/RN phone contact within 3 months prior to the final collection point date.
Analysis
SAS 9.1 (SAS Institute Inc., Cary, NC) was used for all analyses. Simple frequencies and means statistics were used to determine rates of descriptive characteristics of the sample as well as rates of the measured outcomes, preferred place to spend last days of life, and actual site of death. Agreement or concordance between preferred and actual site of death was calculated. For the purposes of the analysis, we assumed all persons who stated they had no preference died in a place concordant with their wishes. To calculate agreement by preferred and actual site, participants who expressed a preference and died (n=111) in hospital, nursing home, home, or hospice setting were included in the analysis, and participants (n=4) who died in an unknown or other locations were excluded (eg, motel room).
Logistic Regression Modeling
2 tests were performed for all categorical variables to determine a significant association with outcome variables. Preferred place of death and concordance between preferred and actual site of death were modeled using predictive variables selected if univariable association demonstrated a P0.25. This standard cutoff was selected to broadly identify candidate variables for logistic regression modeling.[16] A stepwise algorithm was used to select significant predictors that would remain in the model.
In lieu of fitting a multinomial logit model for preferred site of death of home vs hospital vs nursing home or hospice facility as preferred site of death, 3 logit models (although only 2 may be sufficient to estimate the underlying multinomial logit model[17]) were considered with outcome categories: home vs nursing home or hospice facility, and hospital vs nursing home or hospice facility and home vs hospital.
For the logistic regression modeling of concordance, we included the full sample of patients who died during the follow‐up period (n=123). We classified participants as dying in a place concordant with their wishes or not concordant.
RESULTS
Study Population
Subjects were recruited on 96 post‐admission days totaling 842 admissions. Three hundred thirty‐one patients (39%) were ineligible for study participation (n=175 discharged within 24 hours, n=76 unable to consent, n=78 ineligible for other reasons [eg, prisoner, pregnant, under 18 years old], n=2 died within 24 hours of admission). Only 53 of the remaining 511 (10%) patients refused; 458 patients (90%) gave informed consent to participate. Characteristics of the study population are depicted in Table 1. There were very few missing cases (<3%), that is persons without a recent clinic follow‐up date, contact, or a confirmed date of death. These persons were considered alive. Overall, the sample population was ethnically diverse, slightly older than middle age, mostly male (due to the inclusion of the Veterans Administration hospital), and of low socioeconomic status.
Mean age (SD), y | 57.9 (14.8) |
---|---|
| |
Mean time to death (SD), d | 339.5 (348.4) |
Ethnicity | |
African American | 19% (88) |
Caucasian | 52% (239) |
Latino | 22% (102) |
Other | 6% (29) |
Spanish language only | 6% (27) |
Female gender | 35% (159) |
Admitted to DVAMC | 41% (188) |
Admitted to DHMC | 38% (174) |
Admitted to UCH | 21% (96) |
CARING criteria | |
Cancer diagnosis | 11% (51) |
Admitted to hospital 2 times in the past year for chronic illness | 40% (181) |
Resident in a nursing home | 2% (9) |
Noncancer hospice guidelines (meeting 2) | 13% (59) |
Income <$30,000/year | 84% (377) |
No greater than high school education | 55% (248) |
Home owner | 26% (120) |
Rents home | 39% (177) |
Unstable living situationa | 34% (156) |
Low social supportb | 36% (165) |
Uninsured | 18% (81) |
Regular primary care provider | 73% (330) |
Preferred Site of Death
When asked where they preferred to spend the last days of their life, 75% of patients (n=343) stated they would like to be at home. In the hospital was the preferred location for 10% of patients, whereas 6% stated a nursing home and 4% a hospice inpatient facility. Two percent stated they had no preference, and 3% refused to answer (Figure 1)
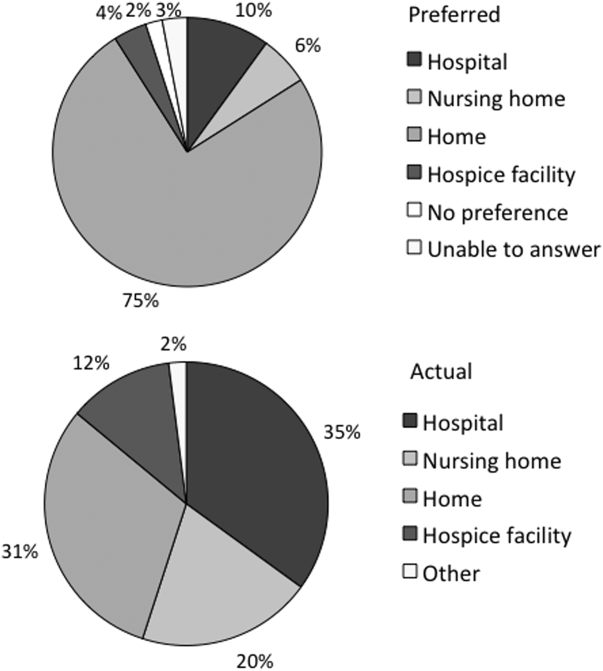
We found that in the univariable analysis the following factors were associated with preference for site of death at a significance level of P<0.25: unstable housing, hospital setting, income level, ethnicity, CARING criteria, presence of an advance directive, education level, married, primary care provider, and presence of public insurance. Results of the logit models (home vs nursing home or hospice facility, and hospital vs nursing home or hospice facility and home vs hospital) are presented in Table 2.
Adjusted Odds Ratio (95% Confidence Interval) | |||
---|---|---|---|
Home vs Nursing Home/Hospice Facility | Hospital vs Nursing Home/Hospice Facility | Home vs Hospital | |
| |||
Low income | 2.71 (1.305.67) | 3.05 (1.019.24) | 0.99 (0.422.37) |
Married | 2.44 (1.145.21) | 2.40 (0.876.62) | 0.82 (0.421.57) |
CARING criteria | 0.58 (0.301.14) | 0.44 (0.181.09) | 0.89 (0.471.66) |
Patients with income <$30,000/year were more likely to prefer home (or hospital) over a nursing home or hospice facility. Being married was predictive of preferring home over nursing home or hospice facility. Patients meeting 1 of the CARING criteria trended toward being less likely (P=0.11 for home and P=0.08 for hospital) to prefer home (or hospital) vs nursing home or hospice facility. However, there were no significant predictors for a preference for home or hospital when directly comparing the 2 locations, as expected from observing similar effects of variables in the other 2 logit models.
Actual Site of Death
One hundred twenty‐three patients died during the follow‐up period (26% of the total sample). Of those who died, the mean age was 64 years (standard deviation 13), 82% had annual incomes <$30,000, 73% were men, and 77% met at least 1 of the CARING criteria suggesting advanced medical illness. The distribution of ethnicities of the deceased subsample was similar to that of the overall cohort. Complete death records were obtained for 121 patients. Only 31% (n=38) died at home, whereas 35% (n=42) died in a hospital, 20% (n=24) died in a nursing home, and 12% (n=14) died in an inpatient hospice facility (Figure 1).
In univariable analysis, there were no associations at a 25% significance level between actual site of death and ethnicity, gender, age, severity of illness, high vs low social support, high or low socioeconomic status, stable vs unstable housing, or presence of a completed advance directive in the medical record.
Concordance Between Preferred and Actual Site of Death
Overall, 37% of the patients died where they stated they would prefer to die, including the 2 with no preference. Concordance rates for each site of death are presented in Table 3. We examined sociodemographic variables, disease severity, advance‐care planning, primary care provider, health insurance, and hospital site to look for associations with concordance. We found that female gender was positively associated with concordance (odds ratio [OR], 3.30; 95% confidence interval [CI], 1.25‐8.72). CARING criteria (P=0.06) and Latino ethnicity (vs all other ethnicity categories, P=0.12) also showed trends for association. Restricting to those who preferred home, the associations became stronger (OR, 4.62; 95% CI, 1.44‐14.79 for female; OR, 7.72; 95% CI, 1.67‐35.71 for CARING criteria), and the trend for the negative association between Latino ethnicity and concordance remained (P=0.12). Results of the model are shown in Table 4.
Actual Site of Death, n (Row %) | Row Total, % Out of 111 | ||||
---|---|---|---|---|---|
Hospital | Nursing Home | Home | Hospice Facility | ||
Preferred hospital | 5 (42%) | 3 (25%) | 2 (17%) | 2 (17%) | 12 (11%) |
Preferred nursing home | 1 (13%) | 5 (63%) | 2 (25%) | 0 | 8 (7%) |
Preferred home | 30 (34%) | 15 (17%) | 31 (35%) | 12 (14%) | 88 (79%) |
Preferred hospice facility | 3 (100%) | 0 | 0 | 0 | 3 (3%) |
Adjusted Odds Ratio (95% Confidence Interval) | |||
---|---|---|---|
All | Home (Using Same Variables) | Home (Using Only Significant Variables) | |
| |||
Female gender | 3.30 (1.258.72) | 4.62 (1.4414.79) | 3.57 (1.2410.34) |
CARING criteria | 3.09 (0.979.81) | 7.72 (1.6735.71) | 5.93 (1.4124.91) |
Latino vs African American/Caucasian/other | 0.43 (0.151.24) | 0.35 (0.091.30) |
DISCUSSION
We found, similarly to previous reports in the literature, the majority of patients preferred to die at home. We did not find a significant difference in preferences or location of death by ethnicity or illness severity. Lower‐income patients and married patients were more likely to prefer to be at home over a nursing home or a hospice facility in the last days of life. We found that the minority of patients died at their stated preferred site of death, and female gender was the only predictive variable we found to distinguish those patients who died in a place concordant with their wishes compared to those who did not.
In the literature, previous studies have reported concordance rates between preferred and actual site of death that range from 30% to 90%.[12, 13, 18, 19, 20, 21, 22, 23, 24] We found a concordance rate at the lowest end of this spectrum. In trying to understand our findings and place them in context, it is helpful to examine other studies. Many of these studies focused solely on cancer patients.[13, 18, 19, 20, 21, 22, 23] Cancer follows a more predictable trajectory of decline compared to other common life‐threatening illnesses, such as cardiac disease, emphysema, or liver failure, that often involve periods of acute deteriorations and plateaus throughout illness progression. The more predictable trajectory may explain the overall higher concordance rates found in the studies involving cancer patients.
The majority of studies in the literature examining concordance between preferred and actual site of death recruited the study sample from home health or home palliative care programs that were providing support and care to participants.[10, 12, 13, 18, 22, 25, 26, 27] The high concordance rates reported may be the result of the patients in the sample receiving services at home aimed at eliciting preferences and providing support at home. Our observational study is unique in that we elicited patient preferences from a diverse group of hospitalized adults. Patients had a broad range of medical illness and were at various stages in their disease trajectory. This allowed our findings to be more generalizable, a major strength of our study.
The only variable associated with concordance that we identified to predict concordance between preferred and actual site of death was female gender. Women have been shown to be more active in medical decision making, which may explain our findings.[28] Female gender and illness severity (as measured by the CARING criteria) were found to be associated with concordance when the preference is for death at home. For persons with more advanced medical illness, they may have had more opportunity to consider their preferences and talk about these preferences. It is even possible that our interview prompted some participants to have discussions with their families or providers.
Variables with high face validity, such as high social support, higher education, and completing an advance directive, did not demonstrate any effect on the outcome of concordance. Other studies have shown that low functional status, Caucasian ethnicity, home care, higher education, and social support have been associated with a greater likelihood for a home death.[3, 6, 9] However, although studies specifically examining concordance between preferred and actual site of death have looked at predictors for home death, we were unable to find predictors for concordance across all preferences in the literature. We can conclude from our findings that the factors that influence concordance of preferences for site of death are extremely complex and difficult to capture and measure. This is extremely unsatisfying in the face of the low concordance rate of 30% we identified.
Latino ethnicity showed a trend toward having a negative association with concordance between preferred and actual site of death. This trend persisted whether it was concordance overall or for concordance with those who preferred a death at home. In the literature, Latinos have been found to be less likely to complete advance directives, use hospice services at the end of life, and are more likely to experience a hospital death.[29, 30, 31, 32, 33] As care at the end of life continues to improve, careful attention should be paid to ensure that these kinds of gaps do not widen any further.
We interviewed patients at an index hospitalization. Patients had an acute medical illness or an exacerbation of a chronic medical illness and required at least 24 hours of hospitalization to be eligible for inclusion. Our bedside interview made use of an opportune time to question patients, a time when it may have been easier for patients to visualize severe illness at the end of life, rather than asking this question during a time of wellness. Although participants overwhelmingly stated they preferred to be at home, for those who died, decisions were made in their care that did not allow for this preference.
Our follow‐up after the initial bedside interview only included death records of where and when participants died. We do not have the details and narrative of the conversations that may have taken place that led to the care decisions that determined participants' actual place of death. We do not know if preferences were elicited or discussed, and care decisions then negotiated, to best meet the goals and preferences expressed at that time. We also do not know if the conversations did not occur and the default of medical intervention and cure‐focused care dictated how participants spent the last days of their life. There is evidence that when conversations about goals and preferences do occur, concordance between preferences and care received are high.[12, 21]
We were unable to identify any predictors beyond gender in this cohort of adults hospitalized with a broad spectrum of severe medical illness to predict concordance with stated preferences and actual site of death. We can conclude then, based on our findings and supported by the literature, that the default trends toward institutional end‐of‐life experiences. To shift to a more patient‐centered approach, away from the default, healthcare providers need to embrace a palliative approach and incorporate preferences and goals into the discussions about next steps of care to facilitate the peaceful death that the majority of patients imagine for themselves. Hospitalist physicians have a unique opportunity at an index hospitalization to start the conversation about preferences for care including where patients would want to spend the last days of their life.
Our study does have some limitations. We elicited preferences at a single point in time, at an index hospitalization. It is possible that participants' preferences changed over the course of their illness. However, in Agar et al.'s study of longitudinal patient preferences for site of death and place of care, most preferences remained stable over time.[18] We also did not have data that included palliative care involvement, homecare or hospice utilization, or cause of death. All of these variables may be important predictors of concordance. Issues of symptom management and lack of caregiver may also dictate place of death, even when goals and care are aligned. We do not have data to address these components of end‐of‐life decision making.
CONCLUSION
Patients continue to express a preference for death at home. However, the majority of patients experienced an institutional death. Furthermore, few participants achieved concordance with where they preferred to die and where they actually died. Female gender was the sole factor associated with concordance between preferred and actual site of death. Incorporating a palliative approach that elicits goals and helps match goals to care, may offer the best opportunity to help people die where they chose.
Disclosures: This research was supported by the Brookdale National Fellowship Award and the NIA/Beeson grant 5K23AG028957. All authors have seen and agree with the contents of the article. This submission was not under review by any other publication. The authors have no financial interest or other potential conflicts of interest.
- Field MJ, Cassel CK, eds. Committee on Care at the End of Life. Approaching Death: Improving Care at the End of Life. Washington DC: National Academy Press; 1997.
- Demography and epidemiology of dying in the U.S. with emphasis on deaths of older persons. Hosp J. 1998;13:49–60. , .
- Factors associated with site of death: a national study of where people die. Med Care. 2003;41:323–335. , , , .
- Terminal cancer care and patient's preferences for place of death: a prospective study. BMJ. 1990;301:415–417. , , , , , .
- Influence of patient preferences and local health system characteristics on the place of death. SUPPORT Investigators. Study to Understand Prognoses and Preferences for Risks and Outcomes of Treatment. J Am Geriatr Soc. 1998;46:1242–1250. , , , et al.
- Where people die: a multilevel approach to understanding influences on site of death in America. Med Care Res Rev. 2007;64:351–378. , , , , , .
- Dying at home or in an institution using death certificates to explore the factors associated with place of death. Health Policy. 2006;78:319–329. , , , , , .
- How do cancer patients who die at home differ from those who die elsewhere? Palliat Med. 1998;12:279–286. , .
- Factors influencing death at home in terminally ill patients with cancer: systematic review [published correction appears in BMJ. 2006;332:1012]. BMJ 2006;332:515–521. , .
- Predictive factors for home deaths among cancer patients in Swedish palliative home care. Support Care Cancer. 2003;11:560–567. , , , , , .
- Systemic adenosine infusions alleviated neuropathic pain. Pain. 2001;94:121–122. , .
- Prevalence, effectiveness, and predictors of planning their place of death among older persons followed in community‐based long term care. J Am Geriatr Soc. 2000;48:943–948. , , .
- Preferences for place of care and place of death among informal caregivers of the terminally ill. Palliat Med. 2005;19:492–499. , , , , .
- Social networks, host resistance, and mortality: a nine‐year follow‐up study of Alameda County residents. Am J Epidemiol. 1979;109:186–204. , .
- A practical tool to identify patients who may benefit from a palliative approach: the CARING criteria. J Pain Symptom Manage. 2006;31:285–292. , , , , , .
- Applied Logistic Regression. 2nd ed. New York, NY: Wiley‐Interscience; 2000. , .
- Calculation of polychotmous logistic regression parameters using individualized regressions. Biometrika. 1984;71:11–18. , .
- Preference for place of care and place of death in palliative care: are these different questions? Palliat Med. 2008;22(7):787–795. , , , , , .
- Place of death: preferences among cancer patients and their carers. Soc Sci Med. 2004;58:2431–2444. , , .
- Determinants of congruence between the preferred and actual place of death for terminally ill cancer patients. J Palliat Care. 2003;19:230–237. , .
- Factors associated with location of death (home or hospital) of patients referred to a palliative care team. CMAJ. 1995;152:361–367. , , .
- Proxy perspectives regarding end‐of‐life care for persons with cancer. Cancer. 2008;112:1854–1861. , , , et al.
- Actual and preferred place of death of cancer patients. Results from the Italian survey of the dying of cancer (ISDOC). J Epidemiol Community Health. 2006;60:412–416. , , , , , .
- Family reports of barriers to optimal care of the dying. Nurs Res. 2000;49:310–317. , , , .
- Place of death: preferences among cancer patients and their carers. Soc Sci Med. 2004;58(12):2431–2444. , , .
- Where do elderly patients prefer to die? Place of death and patient characteristics of 100 elderly patients under the care of a home health care team. J Am Geriatr Soc. 1983;31:457–461. , .
- Predictors of home death in palliative care cancer patients. J Palliat Care. 2000;16:23–28. , , , , , .
- Patient preferences for medical decision making: who really wants to participate? Med Care. 2000;38:335–341. , .
- Racial and ethnic differences in advance care planning among patients with cancer: impact of terminal illness acknowledgment, religiousness, and treatment preferences. J Clin Oncol. 2008;26:4131–4137. , , , et al.
- Influence of ethnicity on advance directives and end‐of‐life decisions. JAMA. 1997;277:298–299. , , , .
- Differences in end‐of‐life decision making among black and white ambulatory cancer patients. J Gen Intern Med. 1996;11:651–656. , , , .
- Hospice usage by minorities in the last year of life: results from the National Mortality Feedback Survey. J Am Geriatr Soc. 2003;51:970–978. , , .
- Place of death: correlations with quality of life of patients with cancer and predictors of bereaved caregivers' mental health. J Clin Oncol. 2010;28:4457–4464. , , , , , .
At the turn of the 20th century, most deaths in the United States occurred at home. By the 1960s, over 70% of deaths occurred in an institutional setting, reflecting an evolution of medical technology.[1, 2, 3] With the birth of the hospice movement in the 1970s, dying patients had the opportunity to have both death at home and aggressive symptom control at the end of life. Although there has been a slow decline in the proportion of deaths that occur in the hospital over the past 2 decades,[3] the overwhelming majority of persons state that they would prefer to die at home. However, recent findings suggest that most people will die in an institutional setting.[3, 4, 5, 6]
Although good data exist describing population preferences for location of death, and we know, based on death records, where deaths occur in the United States, there are few studies that examine concordance between preferred and actual site of death at the individual patient level. Furthermore, although factors have been identified that predict death at home, factors predicting concordance between preferred and actual site of death are not well described.[3, 6, 7, 8, 9, 10, 11, 12, 13]
Regardless of where death ultimately occurs, most adults will experience multiple hospitalizations within the last years of their life. Understanding the preferences and subsequent experiences of this population is of particular relevance to hospitalist physicians who are in a unique position to elicit goals from seriously ill patients and help match patient preferences with their medical care. In this observational study, we sought to determine preferences for site of death in a cohort of adult patients admitted to the hospital for medical illness, and then follow those patients to determine where death occurred for those who died. We also sought to explore factors that may predict concordance between preferred and actual site of death. We hypothesized that ethnic diversity and lower socioeconomic status would be associated with a lower likelihood of concordance between preferred and actual site of death. We also hypothesized that advanced care planning would be associated with a higher likelihood of concordance. The Colorado Multi‐Institutional Review Board approved this study.
METHODS
Participants were recruited from 3 hospitals affiliated with the University of Colorado School of Medicine Internal Medicine Residency program, including the Denver Veterans' Administration Center (DVAMC), Denver Health Medical Center (DHMC), and University of Colorado Hospital (UCH). The DVAMC is a large urban Veterans Administration hospital, serving veterans from the Denver metro area, and is a tertiary referral center for veterans in rural Colorado, Wyoming, and parts of Montana. DHMC, the safety‐net hospital for the Denver area, serves over 25% of the residents in the city and county of Denver, including such special populations as the indigent, chronically mentally ill, and persons with polysubstance dependence. UCH had 350 licensed beds at the time of our study and serves as the Rocky Mountain region's only academic tertiary, specialty care, and referral center. At the time of this study, there was limited inpatient palliative care services at the DVAMC and UH, and no palliative care services at DHMC. Participants were screened on the first day following admission to the adult general medical service. Participants were recruited on 96 postadmission days between February 2004 and June 2006. Recruitment days varied from Monday through Friday, to include admissions from the weekend and throughout the year to reduce potential bias due to seasonal trends of diseases such as influenza. Patients were excluded if they died or were discharged within the first 24 hours of admission, were pregnant, jailed, or unable to give informed consent. All other patients were approached and invited to participate in a brief survey.
After informed consent was obtained, participants completed a bedside interview that included self‐identified ethnicity and the Berkman‐Syme Social Network Index,[14] a brief questionnaire quantifying social support from spouse or domestic partner, family, friends, and other religious or secular organizations. Baseline socioeconomic measures (eg, income, employment, home ownership, car ownership) and questions related to the last days of life were also included. Participants were asked the following question, If you were very sick, with an illness that could not be cured, and in bed most of the time, where would you spend the last days of your life if you could chose?
For each participant, we performed a detailed chart review to determine demographic data, presence of advance directives, and CARING criteria (Cancer, Admissions 2, Residence in a nursing home, Intensive care unit admit with multiorgan failure, 2 Noncancer hospice Guidelines), a set of prognostic criteria identifying patients at an index hospitalization who have a high burden of illness and are at risk for death in the following year.[15] We then followed patients for 5 years. If participants died within the follow‐up period, we collected the date and location of death using medical records, death certificates, or in a few cases when official death records were unavailable, direct contact with the family. Participants were considered alive if they had a clinic visit or MD/RN phone contact within 3 months prior to the final collection point date.
Analysis
SAS 9.1 (SAS Institute Inc., Cary, NC) was used for all analyses. Simple frequencies and means statistics were used to determine rates of descriptive characteristics of the sample as well as rates of the measured outcomes, preferred place to spend last days of life, and actual site of death. Agreement or concordance between preferred and actual site of death was calculated. For the purposes of the analysis, we assumed all persons who stated they had no preference died in a place concordant with their wishes. To calculate agreement by preferred and actual site, participants who expressed a preference and died (n=111) in hospital, nursing home, home, or hospice setting were included in the analysis, and participants (n=4) who died in an unknown or other locations were excluded (eg, motel room).
Logistic Regression Modeling
2 tests were performed for all categorical variables to determine a significant association with outcome variables. Preferred place of death and concordance between preferred and actual site of death were modeled using predictive variables selected if univariable association demonstrated a P0.25. This standard cutoff was selected to broadly identify candidate variables for logistic regression modeling.[16] A stepwise algorithm was used to select significant predictors that would remain in the model.
In lieu of fitting a multinomial logit model for preferred site of death of home vs hospital vs nursing home or hospice facility as preferred site of death, 3 logit models (although only 2 may be sufficient to estimate the underlying multinomial logit model[17]) were considered with outcome categories: home vs nursing home or hospice facility, and hospital vs nursing home or hospice facility and home vs hospital.
For the logistic regression modeling of concordance, we included the full sample of patients who died during the follow‐up period (n=123). We classified participants as dying in a place concordant with their wishes or not concordant.
RESULTS
Study Population
Subjects were recruited on 96 post‐admission days totaling 842 admissions. Three hundred thirty‐one patients (39%) were ineligible for study participation (n=175 discharged within 24 hours, n=76 unable to consent, n=78 ineligible for other reasons [eg, prisoner, pregnant, under 18 years old], n=2 died within 24 hours of admission). Only 53 of the remaining 511 (10%) patients refused; 458 patients (90%) gave informed consent to participate. Characteristics of the study population are depicted in Table 1. There were very few missing cases (<3%), that is persons without a recent clinic follow‐up date, contact, or a confirmed date of death. These persons were considered alive. Overall, the sample population was ethnically diverse, slightly older than middle age, mostly male (due to the inclusion of the Veterans Administration hospital), and of low socioeconomic status.
Mean age (SD), y | 57.9 (14.8) |
---|---|
| |
Mean time to death (SD), d | 339.5 (348.4) |
Ethnicity | |
African American | 19% (88) |
Caucasian | 52% (239) |
Latino | 22% (102) |
Other | 6% (29) |
Spanish language only | 6% (27) |
Female gender | 35% (159) |
Admitted to DVAMC | 41% (188) |
Admitted to DHMC | 38% (174) |
Admitted to UCH | 21% (96) |
CARING criteria | |
Cancer diagnosis | 11% (51) |
Admitted to hospital 2 times in the past year for chronic illness | 40% (181) |
Resident in a nursing home | 2% (9) |
Noncancer hospice guidelines (meeting 2) | 13% (59) |
Income <$30,000/year | 84% (377) |
No greater than high school education | 55% (248) |
Home owner | 26% (120) |
Rents home | 39% (177) |
Unstable living situationa | 34% (156) |
Low social supportb | 36% (165) |
Uninsured | 18% (81) |
Regular primary care provider | 73% (330) |
Preferred Site of Death
When asked where they preferred to spend the last days of their life, 75% of patients (n=343) stated they would like to be at home. In the hospital was the preferred location for 10% of patients, whereas 6% stated a nursing home and 4% a hospice inpatient facility. Two percent stated they had no preference, and 3% refused to answer (Figure 1)
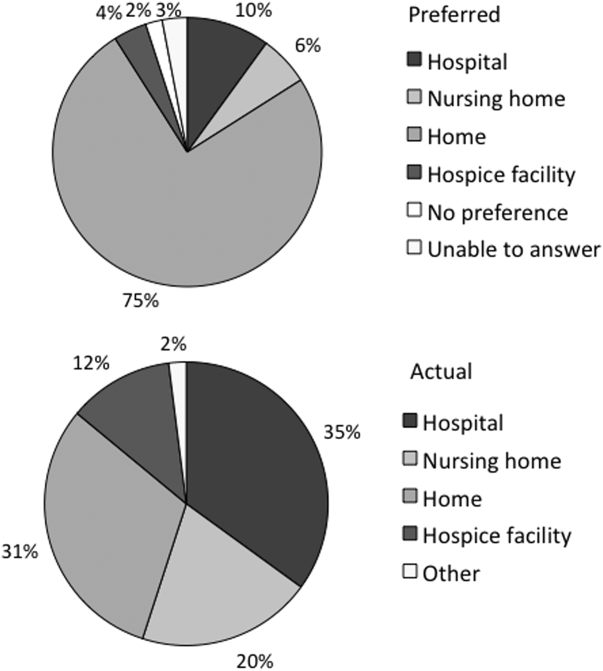
We found that in the univariable analysis the following factors were associated with preference for site of death at a significance level of P<0.25: unstable housing, hospital setting, income level, ethnicity, CARING criteria, presence of an advance directive, education level, married, primary care provider, and presence of public insurance. Results of the logit models (home vs nursing home or hospice facility, and hospital vs nursing home or hospice facility and home vs hospital) are presented in Table 2.
Adjusted Odds Ratio (95% Confidence Interval) | |||
---|---|---|---|
Home vs Nursing Home/Hospice Facility | Hospital vs Nursing Home/Hospice Facility | Home vs Hospital | |
| |||
Low income | 2.71 (1.305.67) | 3.05 (1.019.24) | 0.99 (0.422.37) |
Married | 2.44 (1.145.21) | 2.40 (0.876.62) | 0.82 (0.421.57) |
CARING criteria | 0.58 (0.301.14) | 0.44 (0.181.09) | 0.89 (0.471.66) |
Patients with income <$30,000/year were more likely to prefer home (or hospital) over a nursing home or hospice facility. Being married was predictive of preferring home over nursing home or hospice facility. Patients meeting 1 of the CARING criteria trended toward being less likely (P=0.11 for home and P=0.08 for hospital) to prefer home (or hospital) vs nursing home or hospice facility. However, there were no significant predictors for a preference for home or hospital when directly comparing the 2 locations, as expected from observing similar effects of variables in the other 2 logit models.
Actual Site of Death
One hundred twenty‐three patients died during the follow‐up period (26% of the total sample). Of those who died, the mean age was 64 years (standard deviation 13), 82% had annual incomes <$30,000, 73% were men, and 77% met at least 1 of the CARING criteria suggesting advanced medical illness. The distribution of ethnicities of the deceased subsample was similar to that of the overall cohort. Complete death records were obtained for 121 patients. Only 31% (n=38) died at home, whereas 35% (n=42) died in a hospital, 20% (n=24) died in a nursing home, and 12% (n=14) died in an inpatient hospice facility (Figure 1).
In univariable analysis, there were no associations at a 25% significance level between actual site of death and ethnicity, gender, age, severity of illness, high vs low social support, high or low socioeconomic status, stable vs unstable housing, or presence of a completed advance directive in the medical record.
Concordance Between Preferred and Actual Site of Death
Overall, 37% of the patients died where they stated they would prefer to die, including the 2 with no preference. Concordance rates for each site of death are presented in Table 3. We examined sociodemographic variables, disease severity, advance‐care planning, primary care provider, health insurance, and hospital site to look for associations with concordance. We found that female gender was positively associated with concordance (odds ratio [OR], 3.30; 95% confidence interval [CI], 1.25‐8.72). CARING criteria (P=0.06) and Latino ethnicity (vs all other ethnicity categories, P=0.12) also showed trends for association. Restricting to those who preferred home, the associations became stronger (OR, 4.62; 95% CI, 1.44‐14.79 for female; OR, 7.72; 95% CI, 1.67‐35.71 for CARING criteria), and the trend for the negative association between Latino ethnicity and concordance remained (P=0.12). Results of the model are shown in Table 4.
Actual Site of Death, n (Row %) | Row Total, % Out of 111 | ||||
---|---|---|---|---|---|
Hospital | Nursing Home | Home | Hospice Facility | ||
Preferred hospital | 5 (42%) | 3 (25%) | 2 (17%) | 2 (17%) | 12 (11%) |
Preferred nursing home | 1 (13%) | 5 (63%) | 2 (25%) | 0 | 8 (7%) |
Preferred home | 30 (34%) | 15 (17%) | 31 (35%) | 12 (14%) | 88 (79%) |
Preferred hospice facility | 3 (100%) | 0 | 0 | 0 | 3 (3%) |
Adjusted Odds Ratio (95% Confidence Interval) | |||
---|---|---|---|
All | Home (Using Same Variables) | Home (Using Only Significant Variables) | |
| |||
Female gender | 3.30 (1.258.72) | 4.62 (1.4414.79) | 3.57 (1.2410.34) |
CARING criteria | 3.09 (0.979.81) | 7.72 (1.6735.71) | 5.93 (1.4124.91) |
Latino vs African American/Caucasian/other | 0.43 (0.151.24) | 0.35 (0.091.30) |
DISCUSSION
We found, similarly to previous reports in the literature, the majority of patients preferred to die at home. We did not find a significant difference in preferences or location of death by ethnicity or illness severity. Lower‐income patients and married patients were more likely to prefer to be at home over a nursing home or a hospice facility in the last days of life. We found that the minority of patients died at their stated preferred site of death, and female gender was the only predictive variable we found to distinguish those patients who died in a place concordant with their wishes compared to those who did not.
In the literature, previous studies have reported concordance rates between preferred and actual site of death that range from 30% to 90%.[12, 13, 18, 19, 20, 21, 22, 23, 24] We found a concordance rate at the lowest end of this spectrum. In trying to understand our findings and place them in context, it is helpful to examine other studies. Many of these studies focused solely on cancer patients.[13, 18, 19, 20, 21, 22, 23] Cancer follows a more predictable trajectory of decline compared to other common life‐threatening illnesses, such as cardiac disease, emphysema, or liver failure, that often involve periods of acute deteriorations and plateaus throughout illness progression. The more predictable trajectory may explain the overall higher concordance rates found in the studies involving cancer patients.
The majority of studies in the literature examining concordance between preferred and actual site of death recruited the study sample from home health or home palliative care programs that were providing support and care to participants.[10, 12, 13, 18, 22, 25, 26, 27] The high concordance rates reported may be the result of the patients in the sample receiving services at home aimed at eliciting preferences and providing support at home. Our observational study is unique in that we elicited patient preferences from a diverse group of hospitalized adults. Patients had a broad range of medical illness and were at various stages in their disease trajectory. This allowed our findings to be more generalizable, a major strength of our study.
The only variable associated with concordance that we identified to predict concordance between preferred and actual site of death was female gender. Women have been shown to be more active in medical decision making, which may explain our findings.[28] Female gender and illness severity (as measured by the CARING criteria) were found to be associated with concordance when the preference is for death at home. For persons with more advanced medical illness, they may have had more opportunity to consider their preferences and talk about these preferences. It is even possible that our interview prompted some participants to have discussions with their families or providers.
Variables with high face validity, such as high social support, higher education, and completing an advance directive, did not demonstrate any effect on the outcome of concordance. Other studies have shown that low functional status, Caucasian ethnicity, home care, higher education, and social support have been associated with a greater likelihood for a home death.[3, 6, 9] However, although studies specifically examining concordance between preferred and actual site of death have looked at predictors for home death, we were unable to find predictors for concordance across all preferences in the literature. We can conclude from our findings that the factors that influence concordance of preferences for site of death are extremely complex and difficult to capture and measure. This is extremely unsatisfying in the face of the low concordance rate of 30% we identified.
Latino ethnicity showed a trend toward having a negative association with concordance between preferred and actual site of death. This trend persisted whether it was concordance overall or for concordance with those who preferred a death at home. In the literature, Latinos have been found to be less likely to complete advance directives, use hospice services at the end of life, and are more likely to experience a hospital death.[29, 30, 31, 32, 33] As care at the end of life continues to improve, careful attention should be paid to ensure that these kinds of gaps do not widen any further.
We interviewed patients at an index hospitalization. Patients had an acute medical illness or an exacerbation of a chronic medical illness and required at least 24 hours of hospitalization to be eligible for inclusion. Our bedside interview made use of an opportune time to question patients, a time when it may have been easier for patients to visualize severe illness at the end of life, rather than asking this question during a time of wellness. Although participants overwhelmingly stated they preferred to be at home, for those who died, decisions were made in their care that did not allow for this preference.
Our follow‐up after the initial bedside interview only included death records of where and when participants died. We do not have the details and narrative of the conversations that may have taken place that led to the care decisions that determined participants' actual place of death. We do not know if preferences were elicited or discussed, and care decisions then negotiated, to best meet the goals and preferences expressed at that time. We also do not know if the conversations did not occur and the default of medical intervention and cure‐focused care dictated how participants spent the last days of their life. There is evidence that when conversations about goals and preferences do occur, concordance between preferences and care received are high.[12, 21]
We were unable to identify any predictors beyond gender in this cohort of adults hospitalized with a broad spectrum of severe medical illness to predict concordance with stated preferences and actual site of death. We can conclude then, based on our findings and supported by the literature, that the default trends toward institutional end‐of‐life experiences. To shift to a more patient‐centered approach, away from the default, healthcare providers need to embrace a palliative approach and incorporate preferences and goals into the discussions about next steps of care to facilitate the peaceful death that the majority of patients imagine for themselves. Hospitalist physicians have a unique opportunity at an index hospitalization to start the conversation about preferences for care including where patients would want to spend the last days of their life.
Our study does have some limitations. We elicited preferences at a single point in time, at an index hospitalization. It is possible that participants' preferences changed over the course of their illness. However, in Agar et al.'s study of longitudinal patient preferences for site of death and place of care, most preferences remained stable over time.[18] We also did not have data that included palliative care involvement, homecare or hospice utilization, or cause of death. All of these variables may be important predictors of concordance. Issues of symptom management and lack of caregiver may also dictate place of death, even when goals and care are aligned. We do not have data to address these components of end‐of‐life decision making.
CONCLUSION
Patients continue to express a preference for death at home. However, the majority of patients experienced an institutional death. Furthermore, few participants achieved concordance with where they preferred to die and where they actually died. Female gender was the sole factor associated with concordance between preferred and actual site of death. Incorporating a palliative approach that elicits goals and helps match goals to care, may offer the best opportunity to help people die where they chose.
Disclosures: This research was supported by the Brookdale National Fellowship Award and the NIA/Beeson grant 5K23AG028957. All authors have seen and agree with the contents of the article. This submission was not under review by any other publication. The authors have no financial interest or other potential conflicts of interest.
At the turn of the 20th century, most deaths in the United States occurred at home. By the 1960s, over 70% of deaths occurred in an institutional setting, reflecting an evolution of medical technology.[1, 2, 3] With the birth of the hospice movement in the 1970s, dying patients had the opportunity to have both death at home and aggressive symptom control at the end of life. Although there has been a slow decline in the proportion of deaths that occur in the hospital over the past 2 decades,[3] the overwhelming majority of persons state that they would prefer to die at home. However, recent findings suggest that most people will die in an institutional setting.[3, 4, 5, 6]
Although good data exist describing population preferences for location of death, and we know, based on death records, where deaths occur in the United States, there are few studies that examine concordance between preferred and actual site of death at the individual patient level. Furthermore, although factors have been identified that predict death at home, factors predicting concordance between preferred and actual site of death are not well described.[3, 6, 7, 8, 9, 10, 11, 12, 13]
Regardless of where death ultimately occurs, most adults will experience multiple hospitalizations within the last years of their life. Understanding the preferences and subsequent experiences of this population is of particular relevance to hospitalist physicians who are in a unique position to elicit goals from seriously ill patients and help match patient preferences with their medical care. In this observational study, we sought to determine preferences for site of death in a cohort of adult patients admitted to the hospital for medical illness, and then follow those patients to determine where death occurred for those who died. We also sought to explore factors that may predict concordance between preferred and actual site of death. We hypothesized that ethnic diversity and lower socioeconomic status would be associated with a lower likelihood of concordance between preferred and actual site of death. We also hypothesized that advanced care planning would be associated with a higher likelihood of concordance. The Colorado Multi‐Institutional Review Board approved this study.
METHODS
Participants were recruited from 3 hospitals affiliated with the University of Colorado School of Medicine Internal Medicine Residency program, including the Denver Veterans' Administration Center (DVAMC), Denver Health Medical Center (DHMC), and University of Colorado Hospital (UCH). The DVAMC is a large urban Veterans Administration hospital, serving veterans from the Denver metro area, and is a tertiary referral center for veterans in rural Colorado, Wyoming, and parts of Montana. DHMC, the safety‐net hospital for the Denver area, serves over 25% of the residents in the city and county of Denver, including such special populations as the indigent, chronically mentally ill, and persons with polysubstance dependence. UCH had 350 licensed beds at the time of our study and serves as the Rocky Mountain region's only academic tertiary, specialty care, and referral center. At the time of this study, there was limited inpatient palliative care services at the DVAMC and UH, and no palliative care services at DHMC. Participants were screened on the first day following admission to the adult general medical service. Participants were recruited on 96 postadmission days between February 2004 and June 2006. Recruitment days varied from Monday through Friday, to include admissions from the weekend and throughout the year to reduce potential bias due to seasonal trends of diseases such as influenza. Patients were excluded if they died or were discharged within the first 24 hours of admission, were pregnant, jailed, or unable to give informed consent. All other patients were approached and invited to participate in a brief survey.
After informed consent was obtained, participants completed a bedside interview that included self‐identified ethnicity and the Berkman‐Syme Social Network Index,[14] a brief questionnaire quantifying social support from spouse or domestic partner, family, friends, and other religious or secular organizations. Baseline socioeconomic measures (eg, income, employment, home ownership, car ownership) and questions related to the last days of life were also included. Participants were asked the following question, If you were very sick, with an illness that could not be cured, and in bed most of the time, where would you spend the last days of your life if you could chose?
For each participant, we performed a detailed chart review to determine demographic data, presence of advance directives, and CARING criteria (Cancer, Admissions 2, Residence in a nursing home, Intensive care unit admit with multiorgan failure, 2 Noncancer hospice Guidelines), a set of prognostic criteria identifying patients at an index hospitalization who have a high burden of illness and are at risk for death in the following year.[15] We then followed patients for 5 years. If participants died within the follow‐up period, we collected the date and location of death using medical records, death certificates, or in a few cases when official death records were unavailable, direct contact with the family. Participants were considered alive if they had a clinic visit or MD/RN phone contact within 3 months prior to the final collection point date.
Analysis
SAS 9.1 (SAS Institute Inc., Cary, NC) was used for all analyses. Simple frequencies and means statistics were used to determine rates of descriptive characteristics of the sample as well as rates of the measured outcomes, preferred place to spend last days of life, and actual site of death. Agreement or concordance between preferred and actual site of death was calculated. For the purposes of the analysis, we assumed all persons who stated they had no preference died in a place concordant with their wishes. To calculate agreement by preferred and actual site, participants who expressed a preference and died (n=111) in hospital, nursing home, home, or hospice setting were included in the analysis, and participants (n=4) who died in an unknown or other locations were excluded (eg, motel room).
Logistic Regression Modeling
2 tests were performed for all categorical variables to determine a significant association with outcome variables. Preferred place of death and concordance between preferred and actual site of death were modeled using predictive variables selected if univariable association demonstrated a P0.25. This standard cutoff was selected to broadly identify candidate variables for logistic regression modeling.[16] A stepwise algorithm was used to select significant predictors that would remain in the model.
In lieu of fitting a multinomial logit model for preferred site of death of home vs hospital vs nursing home or hospice facility as preferred site of death, 3 logit models (although only 2 may be sufficient to estimate the underlying multinomial logit model[17]) were considered with outcome categories: home vs nursing home or hospice facility, and hospital vs nursing home or hospice facility and home vs hospital.
For the logistic regression modeling of concordance, we included the full sample of patients who died during the follow‐up period (n=123). We classified participants as dying in a place concordant with their wishes or not concordant.
RESULTS
Study Population
Subjects were recruited on 96 post‐admission days totaling 842 admissions. Three hundred thirty‐one patients (39%) were ineligible for study participation (n=175 discharged within 24 hours, n=76 unable to consent, n=78 ineligible for other reasons [eg, prisoner, pregnant, under 18 years old], n=2 died within 24 hours of admission). Only 53 of the remaining 511 (10%) patients refused; 458 patients (90%) gave informed consent to participate. Characteristics of the study population are depicted in Table 1. There were very few missing cases (<3%), that is persons without a recent clinic follow‐up date, contact, or a confirmed date of death. These persons were considered alive. Overall, the sample population was ethnically diverse, slightly older than middle age, mostly male (due to the inclusion of the Veterans Administration hospital), and of low socioeconomic status.
Mean age (SD), y | 57.9 (14.8) |
---|---|
| |
Mean time to death (SD), d | 339.5 (348.4) |
Ethnicity | |
African American | 19% (88) |
Caucasian | 52% (239) |
Latino | 22% (102) |
Other | 6% (29) |
Spanish language only | 6% (27) |
Female gender | 35% (159) |
Admitted to DVAMC | 41% (188) |
Admitted to DHMC | 38% (174) |
Admitted to UCH | 21% (96) |
CARING criteria | |
Cancer diagnosis | 11% (51) |
Admitted to hospital 2 times in the past year for chronic illness | 40% (181) |
Resident in a nursing home | 2% (9) |
Noncancer hospice guidelines (meeting 2) | 13% (59) |
Income <$30,000/year | 84% (377) |
No greater than high school education | 55% (248) |
Home owner | 26% (120) |
Rents home | 39% (177) |
Unstable living situationa | 34% (156) |
Low social supportb | 36% (165) |
Uninsured | 18% (81) |
Regular primary care provider | 73% (330) |
Preferred Site of Death
When asked where they preferred to spend the last days of their life, 75% of patients (n=343) stated they would like to be at home. In the hospital was the preferred location for 10% of patients, whereas 6% stated a nursing home and 4% a hospice inpatient facility. Two percent stated they had no preference, and 3% refused to answer (Figure 1)
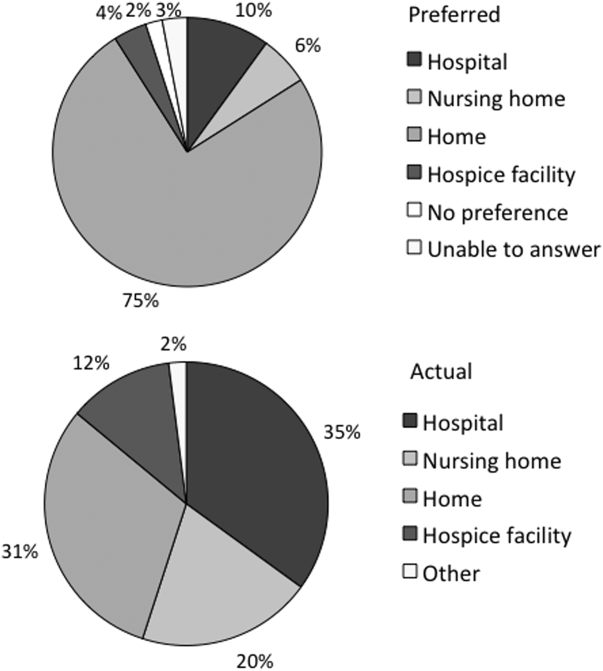
We found that in the univariable analysis the following factors were associated with preference for site of death at a significance level of P<0.25: unstable housing, hospital setting, income level, ethnicity, CARING criteria, presence of an advance directive, education level, married, primary care provider, and presence of public insurance. Results of the logit models (home vs nursing home or hospice facility, and hospital vs nursing home or hospice facility and home vs hospital) are presented in Table 2.
Adjusted Odds Ratio (95% Confidence Interval) | |||
---|---|---|---|
Home vs Nursing Home/Hospice Facility | Hospital vs Nursing Home/Hospice Facility | Home vs Hospital | |
| |||
Low income | 2.71 (1.305.67) | 3.05 (1.019.24) | 0.99 (0.422.37) |
Married | 2.44 (1.145.21) | 2.40 (0.876.62) | 0.82 (0.421.57) |
CARING criteria | 0.58 (0.301.14) | 0.44 (0.181.09) | 0.89 (0.471.66) |
Patients with income <$30,000/year were more likely to prefer home (or hospital) over a nursing home or hospice facility. Being married was predictive of preferring home over nursing home or hospice facility. Patients meeting 1 of the CARING criteria trended toward being less likely (P=0.11 for home and P=0.08 for hospital) to prefer home (or hospital) vs nursing home or hospice facility. However, there were no significant predictors for a preference for home or hospital when directly comparing the 2 locations, as expected from observing similar effects of variables in the other 2 logit models.
Actual Site of Death
One hundred twenty‐three patients died during the follow‐up period (26% of the total sample). Of those who died, the mean age was 64 years (standard deviation 13), 82% had annual incomes <$30,000, 73% were men, and 77% met at least 1 of the CARING criteria suggesting advanced medical illness. The distribution of ethnicities of the deceased subsample was similar to that of the overall cohort. Complete death records were obtained for 121 patients. Only 31% (n=38) died at home, whereas 35% (n=42) died in a hospital, 20% (n=24) died in a nursing home, and 12% (n=14) died in an inpatient hospice facility (Figure 1).
In univariable analysis, there were no associations at a 25% significance level between actual site of death and ethnicity, gender, age, severity of illness, high vs low social support, high or low socioeconomic status, stable vs unstable housing, or presence of a completed advance directive in the medical record.
Concordance Between Preferred and Actual Site of Death
Overall, 37% of the patients died where they stated they would prefer to die, including the 2 with no preference. Concordance rates for each site of death are presented in Table 3. We examined sociodemographic variables, disease severity, advance‐care planning, primary care provider, health insurance, and hospital site to look for associations with concordance. We found that female gender was positively associated with concordance (odds ratio [OR], 3.30; 95% confidence interval [CI], 1.25‐8.72). CARING criteria (P=0.06) and Latino ethnicity (vs all other ethnicity categories, P=0.12) also showed trends for association. Restricting to those who preferred home, the associations became stronger (OR, 4.62; 95% CI, 1.44‐14.79 for female; OR, 7.72; 95% CI, 1.67‐35.71 for CARING criteria), and the trend for the negative association between Latino ethnicity and concordance remained (P=0.12). Results of the model are shown in Table 4.
Actual Site of Death, n (Row %) | Row Total, % Out of 111 | ||||
---|---|---|---|---|---|
Hospital | Nursing Home | Home | Hospice Facility | ||
Preferred hospital | 5 (42%) | 3 (25%) | 2 (17%) | 2 (17%) | 12 (11%) |
Preferred nursing home | 1 (13%) | 5 (63%) | 2 (25%) | 0 | 8 (7%) |
Preferred home | 30 (34%) | 15 (17%) | 31 (35%) | 12 (14%) | 88 (79%) |
Preferred hospice facility | 3 (100%) | 0 | 0 | 0 | 3 (3%) |
Adjusted Odds Ratio (95% Confidence Interval) | |||
---|---|---|---|
All | Home (Using Same Variables) | Home (Using Only Significant Variables) | |
| |||
Female gender | 3.30 (1.258.72) | 4.62 (1.4414.79) | 3.57 (1.2410.34) |
CARING criteria | 3.09 (0.979.81) | 7.72 (1.6735.71) | 5.93 (1.4124.91) |
Latino vs African American/Caucasian/other | 0.43 (0.151.24) | 0.35 (0.091.30) |
DISCUSSION
We found, similarly to previous reports in the literature, the majority of patients preferred to die at home. We did not find a significant difference in preferences or location of death by ethnicity or illness severity. Lower‐income patients and married patients were more likely to prefer to be at home over a nursing home or a hospice facility in the last days of life. We found that the minority of patients died at their stated preferred site of death, and female gender was the only predictive variable we found to distinguish those patients who died in a place concordant with their wishes compared to those who did not.
In the literature, previous studies have reported concordance rates between preferred and actual site of death that range from 30% to 90%.[12, 13, 18, 19, 20, 21, 22, 23, 24] We found a concordance rate at the lowest end of this spectrum. In trying to understand our findings and place them in context, it is helpful to examine other studies. Many of these studies focused solely on cancer patients.[13, 18, 19, 20, 21, 22, 23] Cancer follows a more predictable trajectory of decline compared to other common life‐threatening illnesses, such as cardiac disease, emphysema, or liver failure, that often involve periods of acute deteriorations and plateaus throughout illness progression. The more predictable trajectory may explain the overall higher concordance rates found in the studies involving cancer patients.
The majority of studies in the literature examining concordance between preferred and actual site of death recruited the study sample from home health or home palliative care programs that were providing support and care to participants.[10, 12, 13, 18, 22, 25, 26, 27] The high concordance rates reported may be the result of the patients in the sample receiving services at home aimed at eliciting preferences and providing support at home. Our observational study is unique in that we elicited patient preferences from a diverse group of hospitalized adults. Patients had a broad range of medical illness and were at various stages in their disease trajectory. This allowed our findings to be more generalizable, a major strength of our study.
The only variable associated with concordance that we identified to predict concordance between preferred and actual site of death was female gender. Women have been shown to be more active in medical decision making, which may explain our findings.[28] Female gender and illness severity (as measured by the CARING criteria) were found to be associated with concordance when the preference is for death at home. For persons with more advanced medical illness, they may have had more opportunity to consider their preferences and talk about these preferences. It is even possible that our interview prompted some participants to have discussions with their families or providers.
Variables with high face validity, such as high social support, higher education, and completing an advance directive, did not demonstrate any effect on the outcome of concordance. Other studies have shown that low functional status, Caucasian ethnicity, home care, higher education, and social support have been associated with a greater likelihood for a home death.[3, 6, 9] However, although studies specifically examining concordance between preferred and actual site of death have looked at predictors for home death, we were unable to find predictors for concordance across all preferences in the literature. We can conclude from our findings that the factors that influence concordance of preferences for site of death are extremely complex and difficult to capture and measure. This is extremely unsatisfying in the face of the low concordance rate of 30% we identified.
Latino ethnicity showed a trend toward having a negative association with concordance between preferred and actual site of death. This trend persisted whether it was concordance overall or for concordance with those who preferred a death at home. In the literature, Latinos have been found to be less likely to complete advance directives, use hospice services at the end of life, and are more likely to experience a hospital death.[29, 30, 31, 32, 33] As care at the end of life continues to improve, careful attention should be paid to ensure that these kinds of gaps do not widen any further.
We interviewed patients at an index hospitalization. Patients had an acute medical illness or an exacerbation of a chronic medical illness and required at least 24 hours of hospitalization to be eligible for inclusion. Our bedside interview made use of an opportune time to question patients, a time when it may have been easier for patients to visualize severe illness at the end of life, rather than asking this question during a time of wellness. Although participants overwhelmingly stated they preferred to be at home, for those who died, decisions were made in their care that did not allow for this preference.
Our follow‐up after the initial bedside interview only included death records of where and when participants died. We do not have the details and narrative of the conversations that may have taken place that led to the care decisions that determined participants' actual place of death. We do not know if preferences were elicited or discussed, and care decisions then negotiated, to best meet the goals and preferences expressed at that time. We also do not know if the conversations did not occur and the default of medical intervention and cure‐focused care dictated how participants spent the last days of their life. There is evidence that when conversations about goals and preferences do occur, concordance between preferences and care received are high.[12, 21]
We were unable to identify any predictors beyond gender in this cohort of adults hospitalized with a broad spectrum of severe medical illness to predict concordance with stated preferences and actual site of death. We can conclude then, based on our findings and supported by the literature, that the default trends toward institutional end‐of‐life experiences. To shift to a more patient‐centered approach, away from the default, healthcare providers need to embrace a palliative approach and incorporate preferences and goals into the discussions about next steps of care to facilitate the peaceful death that the majority of patients imagine for themselves. Hospitalist physicians have a unique opportunity at an index hospitalization to start the conversation about preferences for care including where patients would want to spend the last days of their life.
Our study does have some limitations. We elicited preferences at a single point in time, at an index hospitalization. It is possible that participants' preferences changed over the course of their illness. However, in Agar et al.'s study of longitudinal patient preferences for site of death and place of care, most preferences remained stable over time.[18] We also did not have data that included palliative care involvement, homecare or hospice utilization, or cause of death. All of these variables may be important predictors of concordance. Issues of symptom management and lack of caregiver may also dictate place of death, even when goals and care are aligned. We do not have data to address these components of end‐of‐life decision making.
CONCLUSION
Patients continue to express a preference for death at home. However, the majority of patients experienced an institutional death. Furthermore, few participants achieved concordance with where they preferred to die and where they actually died. Female gender was the sole factor associated with concordance between preferred and actual site of death. Incorporating a palliative approach that elicits goals and helps match goals to care, may offer the best opportunity to help people die where they chose.
Disclosures: This research was supported by the Brookdale National Fellowship Award and the NIA/Beeson grant 5K23AG028957. All authors have seen and agree with the contents of the article. This submission was not under review by any other publication. The authors have no financial interest or other potential conflicts of interest.
- Field MJ, Cassel CK, eds. Committee on Care at the End of Life. Approaching Death: Improving Care at the End of Life. Washington DC: National Academy Press; 1997.
- Demography and epidemiology of dying in the U.S. with emphasis on deaths of older persons. Hosp J. 1998;13:49–60. , .
- Factors associated with site of death: a national study of where people die. Med Care. 2003;41:323–335. , , , .
- Terminal cancer care and patient's preferences for place of death: a prospective study. BMJ. 1990;301:415–417. , , , , , .
- Influence of patient preferences and local health system characteristics on the place of death. SUPPORT Investigators. Study to Understand Prognoses and Preferences for Risks and Outcomes of Treatment. J Am Geriatr Soc. 1998;46:1242–1250. , , , et al.
- Where people die: a multilevel approach to understanding influences on site of death in America. Med Care Res Rev. 2007;64:351–378. , , , , , .
- Dying at home or in an institution using death certificates to explore the factors associated with place of death. Health Policy. 2006;78:319–329. , , , , , .
- How do cancer patients who die at home differ from those who die elsewhere? Palliat Med. 1998;12:279–286. , .
- Factors influencing death at home in terminally ill patients with cancer: systematic review [published correction appears in BMJ. 2006;332:1012]. BMJ 2006;332:515–521. , .
- Predictive factors for home deaths among cancer patients in Swedish palliative home care. Support Care Cancer. 2003;11:560–567. , , , , , .
- Systemic adenosine infusions alleviated neuropathic pain. Pain. 2001;94:121–122. , .
- Prevalence, effectiveness, and predictors of planning their place of death among older persons followed in community‐based long term care. J Am Geriatr Soc. 2000;48:943–948. , , .
- Preferences for place of care and place of death among informal caregivers of the terminally ill. Palliat Med. 2005;19:492–499. , , , , .
- Social networks, host resistance, and mortality: a nine‐year follow‐up study of Alameda County residents. Am J Epidemiol. 1979;109:186–204. , .
- A practical tool to identify patients who may benefit from a palliative approach: the CARING criteria. J Pain Symptom Manage. 2006;31:285–292. , , , , , .
- Applied Logistic Regression. 2nd ed. New York, NY: Wiley‐Interscience; 2000. , .
- Calculation of polychotmous logistic regression parameters using individualized regressions. Biometrika. 1984;71:11–18. , .
- Preference for place of care and place of death in palliative care: are these different questions? Palliat Med. 2008;22(7):787–795. , , , , , .
- Place of death: preferences among cancer patients and their carers. Soc Sci Med. 2004;58:2431–2444. , , .
- Determinants of congruence between the preferred and actual place of death for terminally ill cancer patients. J Palliat Care. 2003;19:230–237. , .
- Factors associated with location of death (home or hospital) of patients referred to a palliative care team. CMAJ. 1995;152:361–367. , , .
- Proxy perspectives regarding end‐of‐life care for persons with cancer. Cancer. 2008;112:1854–1861. , , , et al.
- Actual and preferred place of death of cancer patients. Results from the Italian survey of the dying of cancer (ISDOC). J Epidemiol Community Health. 2006;60:412–416. , , , , , .
- Family reports of barriers to optimal care of the dying. Nurs Res. 2000;49:310–317. , , , .
- Place of death: preferences among cancer patients and their carers. Soc Sci Med. 2004;58(12):2431–2444. , , .
- Where do elderly patients prefer to die? Place of death and patient characteristics of 100 elderly patients under the care of a home health care team. J Am Geriatr Soc. 1983;31:457–461. , .
- Predictors of home death in palliative care cancer patients. J Palliat Care. 2000;16:23–28. , , , , , .
- Patient preferences for medical decision making: who really wants to participate? Med Care. 2000;38:335–341. , .
- Racial and ethnic differences in advance care planning among patients with cancer: impact of terminal illness acknowledgment, religiousness, and treatment preferences. J Clin Oncol. 2008;26:4131–4137. , , , et al.
- Influence of ethnicity on advance directives and end‐of‐life decisions. JAMA. 1997;277:298–299. , , , .
- Differences in end‐of‐life decision making among black and white ambulatory cancer patients. J Gen Intern Med. 1996;11:651–656. , , , .
- Hospice usage by minorities in the last year of life: results from the National Mortality Feedback Survey. J Am Geriatr Soc. 2003;51:970–978. , , .
- Place of death: correlations with quality of life of patients with cancer and predictors of bereaved caregivers' mental health. J Clin Oncol. 2010;28:4457–4464. , , , , , .
- Field MJ, Cassel CK, eds. Committee on Care at the End of Life. Approaching Death: Improving Care at the End of Life. Washington DC: National Academy Press; 1997.
- Demography and epidemiology of dying in the U.S. with emphasis on deaths of older persons. Hosp J. 1998;13:49–60. , .
- Factors associated with site of death: a national study of where people die. Med Care. 2003;41:323–335. , , , .
- Terminal cancer care and patient's preferences for place of death: a prospective study. BMJ. 1990;301:415–417. , , , , , .
- Influence of patient preferences and local health system characteristics on the place of death. SUPPORT Investigators. Study to Understand Prognoses and Preferences for Risks and Outcomes of Treatment. J Am Geriatr Soc. 1998;46:1242–1250. , , , et al.
- Where people die: a multilevel approach to understanding influences on site of death in America. Med Care Res Rev. 2007;64:351–378. , , , , , .
- Dying at home or in an institution using death certificates to explore the factors associated with place of death. Health Policy. 2006;78:319–329. , , , , , .
- How do cancer patients who die at home differ from those who die elsewhere? Palliat Med. 1998;12:279–286. , .
- Factors influencing death at home in terminally ill patients with cancer: systematic review [published correction appears in BMJ. 2006;332:1012]. BMJ 2006;332:515–521. , .
- Predictive factors for home deaths among cancer patients in Swedish palliative home care. Support Care Cancer. 2003;11:560–567. , , , , , .
- Systemic adenosine infusions alleviated neuropathic pain. Pain. 2001;94:121–122. , .
- Prevalence, effectiveness, and predictors of planning their place of death among older persons followed in community‐based long term care. J Am Geriatr Soc. 2000;48:943–948. , , .
- Preferences for place of care and place of death among informal caregivers of the terminally ill. Palliat Med. 2005;19:492–499. , , , , .
- Social networks, host resistance, and mortality: a nine‐year follow‐up study of Alameda County residents. Am J Epidemiol. 1979;109:186–204. , .
- A practical tool to identify patients who may benefit from a palliative approach: the CARING criteria. J Pain Symptom Manage. 2006;31:285–292. , , , , , .
- Applied Logistic Regression. 2nd ed. New York, NY: Wiley‐Interscience; 2000. , .
- Calculation of polychotmous logistic regression parameters using individualized regressions. Biometrika. 1984;71:11–18. , .
- Preference for place of care and place of death in palliative care: are these different questions? Palliat Med. 2008;22(7):787–795. , , , , , .
- Place of death: preferences among cancer patients and their carers. Soc Sci Med. 2004;58:2431–2444. , , .
- Determinants of congruence between the preferred and actual place of death for terminally ill cancer patients. J Palliat Care. 2003;19:230–237. , .
- Factors associated with location of death (home or hospital) of patients referred to a palliative care team. CMAJ. 1995;152:361–367. , , .
- Proxy perspectives regarding end‐of‐life care for persons with cancer. Cancer. 2008;112:1854–1861. , , , et al.
- Actual and preferred place of death of cancer patients. Results from the Italian survey of the dying of cancer (ISDOC). J Epidemiol Community Health. 2006;60:412–416. , , , , , .
- Family reports of barriers to optimal care of the dying. Nurs Res. 2000;49:310–317. , , , .
- Place of death: preferences among cancer patients and their carers. Soc Sci Med. 2004;58(12):2431–2444. , , .
- Where do elderly patients prefer to die? Place of death and patient characteristics of 100 elderly patients under the care of a home health care team. J Am Geriatr Soc. 1983;31:457–461. , .
- Predictors of home death in palliative care cancer patients. J Palliat Care. 2000;16:23–28. , , , , , .
- Patient preferences for medical decision making: who really wants to participate? Med Care. 2000;38:335–341. , .
- Racial and ethnic differences in advance care planning among patients with cancer: impact of terminal illness acknowledgment, religiousness, and treatment preferences. J Clin Oncol. 2008;26:4131–4137. , , , et al.
- Influence of ethnicity on advance directives and end‐of‐life decisions. JAMA. 1997;277:298–299. , , , .
- Differences in end‐of‐life decision making among black and white ambulatory cancer patients. J Gen Intern Med. 1996;11:651–656. , , , .
- Hospice usage by minorities in the last year of life: results from the National Mortality Feedback Survey. J Am Geriatr Soc. 2003;51:970–978. , , .
- Place of death: correlations with quality of life of patients with cancer and predictors of bereaved caregivers' mental health. J Clin Oncol. 2010;28:4457–4464. , , , , , .
Copyright © 2013 Society of Hospital Medicine
AD Myths in Healthcare Debate
All new legislation concerning advance care planning was removed from the Affordable Care Act, signed into law in March 2010. However, through a Medicare payment regulation, Centers for Medicare and Medicaid Services (CMS) was able to add a provision allowing compensation to physicians for advance directive (AD) discussions as part of the annual Medicare wellness exam. Previously, under President George W. Bush, funding for AD discussions was already part of the Welcome to Medicare visit. Once again, the provision was misrepresented and distorted in the media, talk radio shows, and social networking sites. Within days of the announcement, the White House removed the regulation stating that the controversy surrounding the provision was distracting from the overall debate about healthcare. The term death panels has now entered our national lexicon and serves to undermine the efforts of the palliative care field which, through discussions with patients and families, attempts to provide care consistent with patients' goals.
In fact, ADs have been a cornerstone of ethical decision making, by supporting patient autonomy and allowing patient wishes to be respected when decisional capacity is lacking. Advance directives may include a living will, a Medical Durable Power of Attorney, or may be a broader more comprehensive document outlining goals, values, and preferences for care in the event of decisional incapacity. ADs allow patients to express preferences that incorporate both quantity and quality of life, as there are times when interventions at the end of life may increase length of life to the detriment of quality of life. In this context, patients may chose to value quality of life and request the interventions be withdrawn that focus on maintaining life without hope for quality of life. ADs also permit patients who prefer quantity over quality of life to communicate these wishes. These conversations are complex and time‐consuming. Patients may have profound misperceptions about the benefits offered by interventions at the end of life. Having detailed conversations with healthcare providers about actual benefits, risks, and alternatives has been shown to impact that decision‐making process.1 In our current payment system, these time‐consuming conversations are not compensated by private or public insurers, and are incompatible with 20‐minute appointments, so they rarely occur.24 While Nancy Cruzan and Terri Schiavo brought national attention to the issue for a brief time, recent data suggest that only 30% of adults have completed an AD,57 however, 93% of adults would like to discuss ADs with their physician.8 Furthermore, Silveira et al. showed that older adults with ADs are more likely than those without ADs to receive care that is consistent with their preferences at the end of life.9 ADs were the sole predictor of concordance between preferred and actual site of death in a cohort of seriously ill, hospitalized patients.10 Patients with advanced cancer who discussed their end of life wishes with their physician were more likely to receive care consistent with their preferences.11
Advance directives are based on the ethical principle of autonomy and, with the growing evidence that ADs may improve care at the end of life, public understanding of the issue is critical. We had presented early preliminary data in a letter to the editor showing that having had an advance directive discussion or an AD in the medical record was not associated with an increased risk of death.12 This research, along with the work of Silveira and colleagues,9 was cited by the Obama administration when they decided to add the regulation for including advance care planning as part of the annual Medicare wellness exams. This brief report presents a more comprehensive examination of the relationship of AD discussions and AD documentation with survival in a group of hospitalized patients.
METHODS
Study Sites and Participant Recruitment
This was a multisite, prospective study of patients admitted to the hospital for medical illness. The Colorado Multi‐Institutional Review Board approved this study.
Over a 17‐month period starting in February 2004, participants were recruited from 3 hospitals affiliated with the University of ColoradoDenver Internal Medicine Residency program: the Denver Veterans' Administration Center (DVAMC); Denver Health Medical Center (DHMC), the city's safety net hospital; and University of Colorado Hospital (UCH), an academic tertiary, specialty care and referral center. Exclusion criteria included: admission <24 hours, pregnancy, age <18 years, incarceration, spoke neither English nor Spanish, lack of decisional capacity. Recruitment was done on the day following admission to the hospital throughout the year, to reduce potential bias due to seasonal trends. A trained assistant recruited on variable weekdays (to allow inclusion of weekend admissions). Of 842 admissions occurring during the recruitment, 331 (39%) were ineligible (175 discharged and 2 died within 24 hours postadmission; 76 lacked decisional capacity; and 78 met other exclusion criteria listed above). All other patients (n = 511) were invited to participate and 458 patients consented.
Participant Interview and Measures
Fifty‐three (10%) refused; 458 gave informed consent and participated in a bedside interview, including questions related to advance care planning. In this interview, participants were first asked to define an AD. Their response was either confirmed or corrected using a standard simple explanation that defined and described ADs:
An advance directive is a document that lets your healthcare providers know who you would want to make decisions for you if you were unable to make them for yourself. It can also tell your healthcare providers what types of medical treatments you would and would not want if you were unable to speak for yourself.
They were then asked if any healthcare provider had ever discussed ADs with them (AD discussion is a primary variable of interest).
Chart Review and Vital Records Data Collection
We reviewed each medical record to determine admitting diagnoses, CARING criteria (a set of simple criteria developed by our group to score the need for palliative care, which has been shown to predict death at 1 year),13 socioeconomic and demographic information, and the presence of ADs in the medical record (documentation of AD is a primary variable of interest). We defined ADs broadly, including: living will, durable power of attorney for healthcare, or a comprehensive advance care planning document (eg, Five Wishes). The CARING criteria are validated criteria that accurately predict death at 1 year, and were developed to identify patients who would be appropriate for a palliative care intervention. It is based on the following variables: Cancer as a primary admitting diagnosis, Admitted 2 times to the hospital in the past year for a chronic medical illness, Resident of a nursing home, ICU admission with >2 organ systems in failure, and 2 Non‐Cancer hospice Guidelines as well as age. Scores range from 4 = low risk of death, 5‐12 = medium risk of death, and 13 = high risk of death at 1 year. We accessed hospital records and state Vital Records from 2003 to 2009 to determine which patients died within a 12‐month follow‐up period, and their date of death (primary outcome).
Cohort Risk Stratification
Based on their CARING score, participants were classified as being at low, medium, or high risk of death at 1 year.13 The probability of imminent death in the group of high‐risk patients is the main indication for an advance directive, and therefore the analysis of this high‐risk group would be confounded. Therefore, those at high (and unclassified) risk of death (89 [and 13] out of 458 interviewed patients) were excluded from the survival analysis. Including persons at high risk of death in this analysis would lead to confounding by indicationthat physicians are most likely to address ADs with patients that they perceive are likely to die in the near future. An example of this in the literature is the timing of do‐not‐attempt‐resuscitation orders (DNAR). It is well documented that most DNAR orders are written within 1 to 2 days of death.1416 The DNAR orders do not cause or lead to death, they are simply finally written for patients that are actively dying.
Statistical Analysis
SAS 9.1 (SAS Institute, Cary, NC) was used for all analyses. Survival analysis was conducted to examine time to death. Interaction effects of the variable of interest with patient risk were assessed by estimating Kaplan‐Meier survival curves for low and medium risk groups separately. The Wilcoxon and log‐rank tests were employed to compare those with and without AD discussions (and accounting for clustering within hospitals) and documentation. Since the stratification into risk groups involves the use of the CARING criteria, which were the main confounders, additional risk adjustment in each risk group was not performed. Post hoc power analysis showed an ability to detect a 13 percentage points difference in mortality rate, with 80% power for a 2‐sided test and alpha = 0.05, assuming a 20% death rate for the group without AD discussion (adjusting for the covariate distribution difference between those with and without AD discussion).
RESULTS
Characteristics of the 356 study subjects are listed in Table 1. Overall, the sample population was ethnically diverse, slightly above middle‐aged, mostly male, and of lower socioeconomic status, reflecting the hospitals' populations. Using the CARING criteria, 297 subjects were found to be at low risk, and 59 subjects at medium risk, of death at 1 year.
Percent (n) or Mean SD | |
---|---|
| |
Ethnicity | |
African American | 19% (69) |
Caucasian | 55% (194) |
Latino | 19% (66) |
Other | 8% (27) |
Age (years) | 57.2 15 |
Female gender | 34% (122) |
Admitted to | |
DVAMC | 41% (147) |
DHMC | 34% (122) |
UCH | 24% (87) |
CARING criteria | |
Cancer diagnosis | 4% (15) |
Admitted to hospital 2 times in the past year for chronic illness | 31% (109) |
Resident in a nursing home | 2% (7) |
Non‐cancer hospice guidelines (meeting 2) | 1% (4) |
Income less than $30,000/yr | 81% (284) |
No greater than high school education | 53% (188) |
Living situation | |
Home owner | 36% (125) |
Rents home | 38% (132) |
Unstable living situation | 27% (94) |
Low social support | 37% (169) |
Uninsured | 14% (51) |
Regular primary care provider | 72% (254) |
Overall, 206 (45%) reported a discussion about ADs with a healthcare provider. However, we found that only 56 (10%) had an AD document on their chart. Twenty‐eight (6%) had a living will, 43 (9%) had a durable power of attorney, and 30 (7%) had a broader AD document. Between 2003 and 2009, 121 (26%) patients died. Unadjusted mortality rates for those with and without documentation and discussions of ADs are displayed in Figure 1.
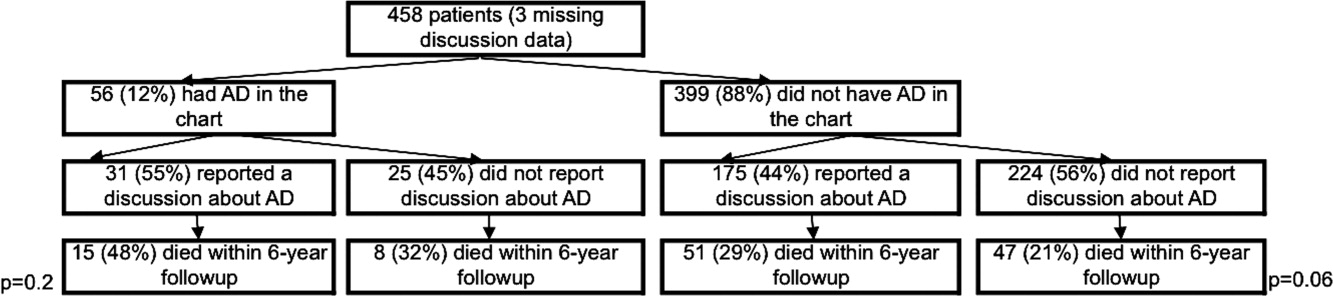
Kaplan‐Meier survival curves showed that, for subjects with a low or medium risk of death at 1 year, having had an AD discussion or having an AD in the medical record did not affect survival in subjects (Figures 2 and 3). Cox proportional hazards models adjusting for other covariates confirmed the results of the survival analysis (data not shown). Minimal intraclass correlation coefficients (0.005) were observed for the outcomes. Therefore, no models accounting for clustering within hospitals were developed.
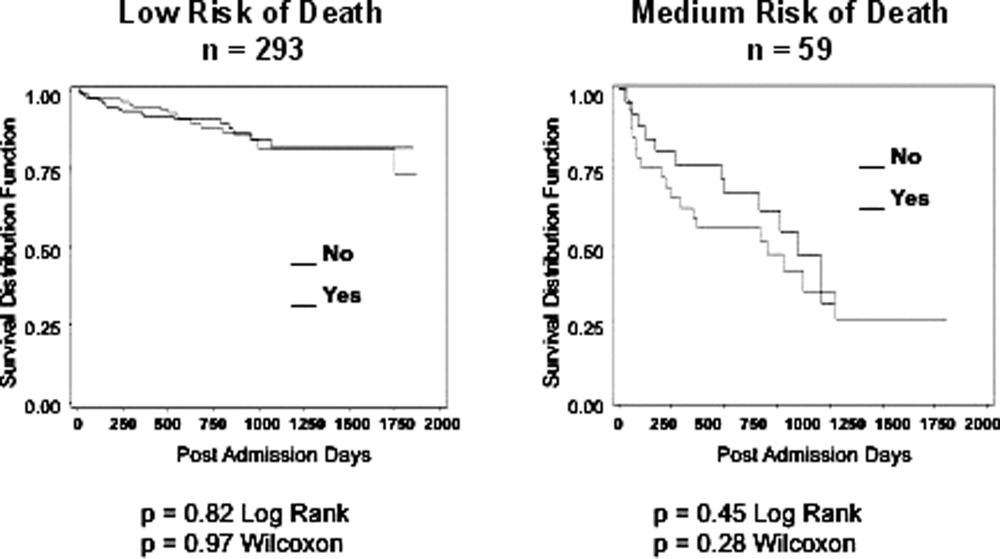
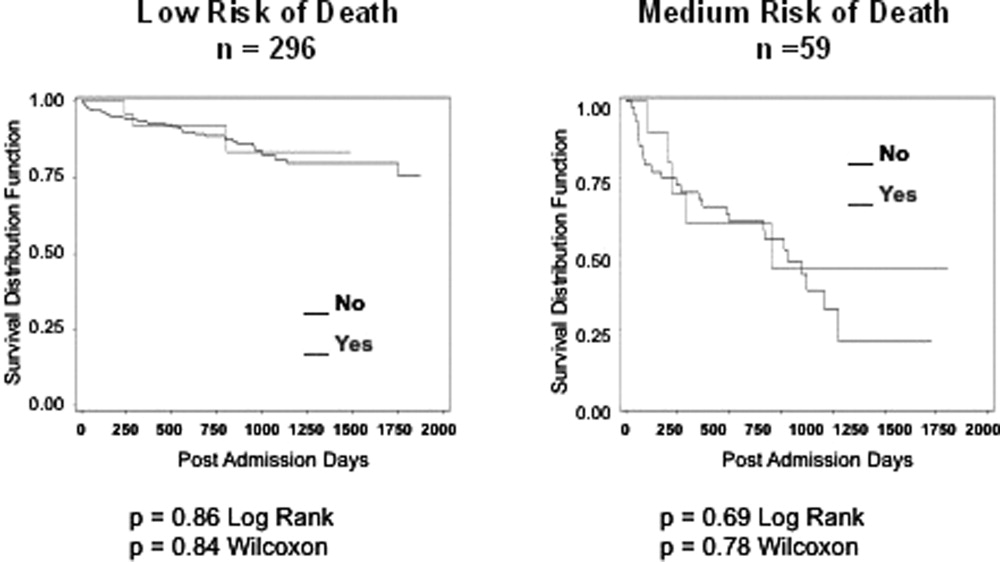
DISCUSSION
We found no decrease in survival for patients at low and medium 1‐year risk of death who reported having discussed ADs or who had an AD in their medical record, providing important evidence that having advance care planning discussions do not hasten death in this group of adults. However, it is possible that ADs, when implemented properly, may dictate withdrawal or withholding of interventions that may extend quantity of life at a quality unacceptable for the person executing the directive. For example, a feeding tube delivering artificial nutrition and hydration may grant years to someone in a persistent vegetative state, but those years, without the ability to be aware or interact with surroundings and loved ones, may not be a life worth living for some individuals. One explanation for our negative findings may be that the circumstances in which an AD may have an effect on outcomes may not yet have occurred among this lower risk population.
Opposition to the process of advance care planning may be considered unethical, by removing the opportunity for individuals to express their desires in the event of decisional incapacity, therefore disregarding patient autonomy. Furthermore, with the growing evidence that AD discussions and documentation help patients achieve care consistent with their wishes at the end of life,9, 11, 17 preventing advance care planning may worsen end of life outcomes.
Another important finding in our study was that only about 10% of the patients interviewed had completed an AD document, although nearly half reported they had discussed ADs with a healthcare provider. The patients we interviewed in this study had been admitted to the hospital in the previous 24 hours. As part of the Patient Self‐Determination Act, all patients admitted to a healthcare facility should receive information and counseling on AD. Less than half of our cohort reported any discussions about ADs and only 10% had completed an AD, suggesting that huge opportunities exist for improvement in advance care planning. As this study demonstrates, there was no increased mortality from advance care planning among those at low and medium risk of death, and others have shown benefits from the process. AD discussions and documentation should be fostered, especially as the burden of chronic disease increases and the population ages. In targeted studies to improve advance care planning, completion rates of up to 85% have been achieved.17
Our decision to focus solely on patients at low or medium risk of death, and exclude those at a high risk of death, is based on both clinical and methodological judgment. First, it is important to note that ADs are important even for those at lower risk of deaththe 3 critical cases that have shaped AD policy in this country, Karen Ann Quinlan, Nancy Cruzan, and Terry Schiavo, were all otherwise healthy young women.
Our study does have limitations. First, the sample size is small and not powered to detect small differences in survival. In addition, we only examined Vital Records within Colorado, although all participants had either a date of death or recent date of last contact. It is also conceivable that some patients discussed or completed ADs at a later time in their illness trajectory. However, the generalizability of this study is a major strength, by including a population and healthcare settings that are ethnically and socioeconomically diverse. Generalization of results beyond the three types of hospitals should be limited even with the low intraclass correlation. The major limitation of this research is that we do not have data on participant quality of life or whether completing an AD led to increased use of palliative care. During the time the research was conducted, 2 of the 3 hospitals involved had small palliative care services and the third remains without a palliative care service.
In conclusion, our study provides limited data to counteract the misleading claims of those opposed to the advance care planning process. Our results underscore the importance of educating the public on the importance of ADs and cast doubt on the death myth surrounding advance care planning. However, further, preferably longitudinal, study is needed to prospectively understand both the benefits and risks of advance care planning.
- The influence of the probability of survival on patient's preferences regarding cardiopulmonary resuscitation.N Engl J Med.1994;330:545–549. , , .
- Physician reluctance to discuss advance directives. An empiric investigation of potential barriers.Arch Intern Med.1994;154(20):2311–2318. , , .
- Advance directives and advance care planning: report to Congress. US Department of Health 82(12):1487–1490.
- Facts on dying: policy relevant data on care at the end of life, USA and state statistics. Dartmouth Atlas of Health Care Web site. Available at: http://www.chcr.brown.edu/dying/usastatistics.htm. Accessed September 20,2010.
- The quest to reform end of life care: rethinking assumptions and setting new directions.Hastings Cent Rep. November—December2005;S52–S57. , .
- End‐of‐life care and outcomes.Summary, Evidence Report/Technology Assessment No 110.Rockville, MD:Agency for Healthcare Research and Quality; December2004;1–6. , , , et al.
- Advance directives for medical care—a case for greater use.N Engl J Med.1991;324(13):889–895. , , , , .
- Advance directives and outcomes of surrogate decision making before death.N Engl J Med.2010;362(13):1211–1218. , , .
- Advance directives: the best predictor of congruence between preferred and actual site of death [Research Poster Abstracts].Journal of Hospital Medicine2010;5(S1):1–81. , , .
- End‐of‐life discussions, goal attainment, and distress at the end of life: predictors and outcomes of receipt of care consistent with preferences.J Clin Oncol2010;28(7):1203–1208. , , , , .
- Advance directive discussions do not lead to death.J Am Geriatr Soc.2010;58(2):400–401. , , .
- Practical tool to identify patients who may benefit from a palliative approach: the CARING criteria.J Pain Symptom Manage.2005;31(4):285–292. , , , , , .
- Do not resuscitate orders and the cost of death.Arch Intern Med.1993;153(10):1249–1253. , , .
- The do‐not‐resuscitate order: associations with advance directives, physician specialty and documentation of discussion 15 years after the Patient Self‐Determination Act.J Med Ethics.2008;34(9):642–647. , , , , .
- Factors associated with do‐not‐resuscitate orders: patients' preferences, prognoses, and physicians' judgments. SUPPORT Investigators. Study to Understand Prognoses and Preferences for Outcomes and Risks of Treatment.Ann Intern Med.1996;125(4):284–293. , , , et al.
- Death and end‐of‐life planning in one midwestern community.Arch Intern Med.1998;158(4):383–390. , .
All new legislation concerning advance care planning was removed from the Affordable Care Act, signed into law in March 2010. However, through a Medicare payment regulation, Centers for Medicare and Medicaid Services (CMS) was able to add a provision allowing compensation to physicians for advance directive (AD) discussions as part of the annual Medicare wellness exam. Previously, under President George W. Bush, funding for AD discussions was already part of the Welcome to Medicare visit. Once again, the provision was misrepresented and distorted in the media, talk radio shows, and social networking sites. Within days of the announcement, the White House removed the regulation stating that the controversy surrounding the provision was distracting from the overall debate about healthcare. The term death panels has now entered our national lexicon and serves to undermine the efforts of the palliative care field which, through discussions with patients and families, attempts to provide care consistent with patients' goals.
In fact, ADs have been a cornerstone of ethical decision making, by supporting patient autonomy and allowing patient wishes to be respected when decisional capacity is lacking. Advance directives may include a living will, a Medical Durable Power of Attorney, or may be a broader more comprehensive document outlining goals, values, and preferences for care in the event of decisional incapacity. ADs allow patients to express preferences that incorporate both quantity and quality of life, as there are times when interventions at the end of life may increase length of life to the detriment of quality of life. In this context, patients may chose to value quality of life and request the interventions be withdrawn that focus on maintaining life without hope for quality of life. ADs also permit patients who prefer quantity over quality of life to communicate these wishes. These conversations are complex and time‐consuming. Patients may have profound misperceptions about the benefits offered by interventions at the end of life. Having detailed conversations with healthcare providers about actual benefits, risks, and alternatives has been shown to impact that decision‐making process.1 In our current payment system, these time‐consuming conversations are not compensated by private or public insurers, and are incompatible with 20‐minute appointments, so they rarely occur.24 While Nancy Cruzan and Terri Schiavo brought national attention to the issue for a brief time, recent data suggest that only 30% of adults have completed an AD,57 however, 93% of adults would like to discuss ADs with their physician.8 Furthermore, Silveira et al. showed that older adults with ADs are more likely than those without ADs to receive care that is consistent with their preferences at the end of life.9 ADs were the sole predictor of concordance between preferred and actual site of death in a cohort of seriously ill, hospitalized patients.10 Patients with advanced cancer who discussed their end of life wishes with their physician were more likely to receive care consistent with their preferences.11
Advance directives are based on the ethical principle of autonomy and, with the growing evidence that ADs may improve care at the end of life, public understanding of the issue is critical. We had presented early preliminary data in a letter to the editor showing that having had an advance directive discussion or an AD in the medical record was not associated with an increased risk of death.12 This research, along with the work of Silveira and colleagues,9 was cited by the Obama administration when they decided to add the regulation for including advance care planning as part of the annual Medicare wellness exams. This brief report presents a more comprehensive examination of the relationship of AD discussions and AD documentation with survival in a group of hospitalized patients.
METHODS
Study Sites and Participant Recruitment
This was a multisite, prospective study of patients admitted to the hospital for medical illness. The Colorado Multi‐Institutional Review Board approved this study.
Over a 17‐month period starting in February 2004, participants were recruited from 3 hospitals affiliated with the University of ColoradoDenver Internal Medicine Residency program: the Denver Veterans' Administration Center (DVAMC); Denver Health Medical Center (DHMC), the city's safety net hospital; and University of Colorado Hospital (UCH), an academic tertiary, specialty care and referral center. Exclusion criteria included: admission <24 hours, pregnancy, age <18 years, incarceration, spoke neither English nor Spanish, lack of decisional capacity. Recruitment was done on the day following admission to the hospital throughout the year, to reduce potential bias due to seasonal trends. A trained assistant recruited on variable weekdays (to allow inclusion of weekend admissions). Of 842 admissions occurring during the recruitment, 331 (39%) were ineligible (175 discharged and 2 died within 24 hours postadmission; 76 lacked decisional capacity; and 78 met other exclusion criteria listed above). All other patients (n = 511) were invited to participate and 458 patients consented.
Participant Interview and Measures
Fifty‐three (10%) refused; 458 gave informed consent and participated in a bedside interview, including questions related to advance care planning. In this interview, participants were first asked to define an AD. Their response was either confirmed or corrected using a standard simple explanation that defined and described ADs:
An advance directive is a document that lets your healthcare providers know who you would want to make decisions for you if you were unable to make them for yourself. It can also tell your healthcare providers what types of medical treatments you would and would not want if you were unable to speak for yourself.
They were then asked if any healthcare provider had ever discussed ADs with them (AD discussion is a primary variable of interest).
Chart Review and Vital Records Data Collection
We reviewed each medical record to determine admitting diagnoses, CARING criteria (a set of simple criteria developed by our group to score the need for palliative care, which has been shown to predict death at 1 year),13 socioeconomic and demographic information, and the presence of ADs in the medical record (documentation of AD is a primary variable of interest). We defined ADs broadly, including: living will, durable power of attorney for healthcare, or a comprehensive advance care planning document (eg, Five Wishes). The CARING criteria are validated criteria that accurately predict death at 1 year, and were developed to identify patients who would be appropriate for a palliative care intervention. It is based on the following variables: Cancer as a primary admitting diagnosis, Admitted 2 times to the hospital in the past year for a chronic medical illness, Resident of a nursing home, ICU admission with >2 organ systems in failure, and 2 Non‐Cancer hospice Guidelines as well as age. Scores range from 4 = low risk of death, 5‐12 = medium risk of death, and 13 = high risk of death at 1 year. We accessed hospital records and state Vital Records from 2003 to 2009 to determine which patients died within a 12‐month follow‐up period, and their date of death (primary outcome).
Cohort Risk Stratification
Based on their CARING score, participants were classified as being at low, medium, or high risk of death at 1 year.13 The probability of imminent death in the group of high‐risk patients is the main indication for an advance directive, and therefore the analysis of this high‐risk group would be confounded. Therefore, those at high (and unclassified) risk of death (89 [and 13] out of 458 interviewed patients) were excluded from the survival analysis. Including persons at high risk of death in this analysis would lead to confounding by indicationthat physicians are most likely to address ADs with patients that they perceive are likely to die in the near future. An example of this in the literature is the timing of do‐not‐attempt‐resuscitation orders (DNAR). It is well documented that most DNAR orders are written within 1 to 2 days of death.1416 The DNAR orders do not cause or lead to death, they are simply finally written for patients that are actively dying.
Statistical Analysis
SAS 9.1 (SAS Institute, Cary, NC) was used for all analyses. Survival analysis was conducted to examine time to death. Interaction effects of the variable of interest with patient risk were assessed by estimating Kaplan‐Meier survival curves for low and medium risk groups separately. The Wilcoxon and log‐rank tests were employed to compare those with and without AD discussions (and accounting for clustering within hospitals) and documentation. Since the stratification into risk groups involves the use of the CARING criteria, which were the main confounders, additional risk adjustment in each risk group was not performed. Post hoc power analysis showed an ability to detect a 13 percentage points difference in mortality rate, with 80% power for a 2‐sided test and alpha = 0.05, assuming a 20% death rate for the group without AD discussion (adjusting for the covariate distribution difference between those with and without AD discussion).
RESULTS
Characteristics of the 356 study subjects are listed in Table 1. Overall, the sample population was ethnically diverse, slightly above middle‐aged, mostly male, and of lower socioeconomic status, reflecting the hospitals' populations. Using the CARING criteria, 297 subjects were found to be at low risk, and 59 subjects at medium risk, of death at 1 year.
Percent (n) or Mean SD | |
---|---|
| |
Ethnicity | |
African American | 19% (69) |
Caucasian | 55% (194) |
Latino | 19% (66) |
Other | 8% (27) |
Age (years) | 57.2 15 |
Female gender | 34% (122) |
Admitted to | |
DVAMC | 41% (147) |
DHMC | 34% (122) |
UCH | 24% (87) |
CARING criteria | |
Cancer diagnosis | 4% (15) |
Admitted to hospital 2 times in the past year for chronic illness | 31% (109) |
Resident in a nursing home | 2% (7) |
Non‐cancer hospice guidelines (meeting 2) | 1% (4) |
Income less than $30,000/yr | 81% (284) |
No greater than high school education | 53% (188) |
Living situation | |
Home owner | 36% (125) |
Rents home | 38% (132) |
Unstable living situation | 27% (94) |
Low social support | 37% (169) |
Uninsured | 14% (51) |
Regular primary care provider | 72% (254) |
Overall, 206 (45%) reported a discussion about ADs with a healthcare provider. However, we found that only 56 (10%) had an AD document on their chart. Twenty‐eight (6%) had a living will, 43 (9%) had a durable power of attorney, and 30 (7%) had a broader AD document. Between 2003 and 2009, 121 (26%) patients died. Unadjusted mortality rates for those with and without documentation and discussions of ADs are displayed in Figure 1.
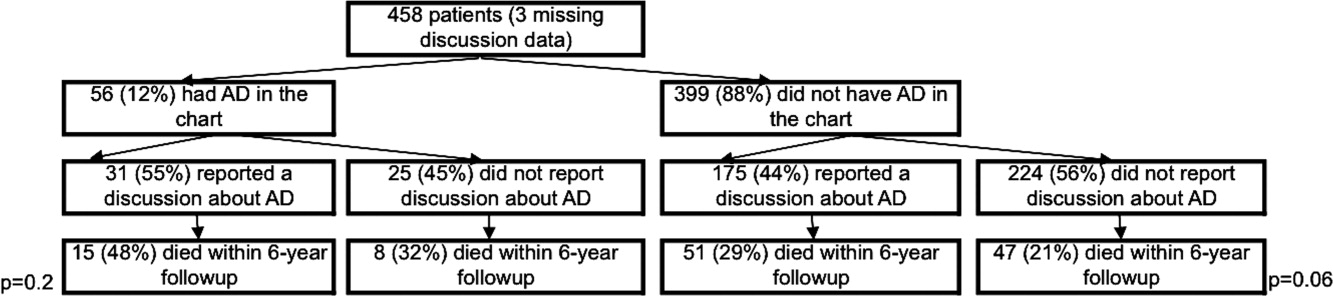
Kaplan‐Meier survival curves showed that, for subjects with a low or medium risk of death at 1 year, having had an AD discussion or having an AD in the medical record did not affect survival in subjects (Figures 2 and 3). Cox proportional hazards models adjusting for other covariates confirmed the results of the survival analysis (data not shown). Minimal intraclass correlation coefficients (0.005) were observed for the outcomes. Therefore, no models accounting for clustering within hospitals were developed.
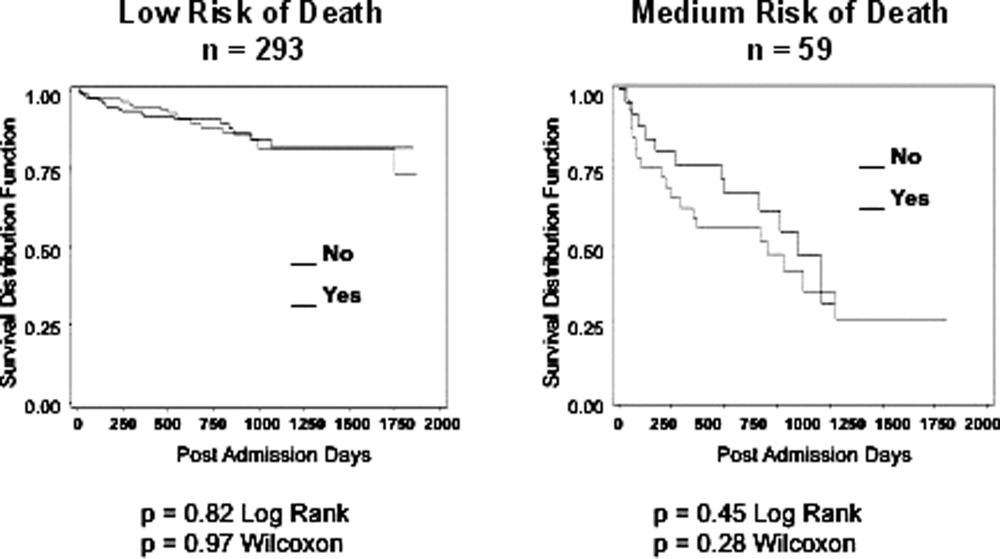
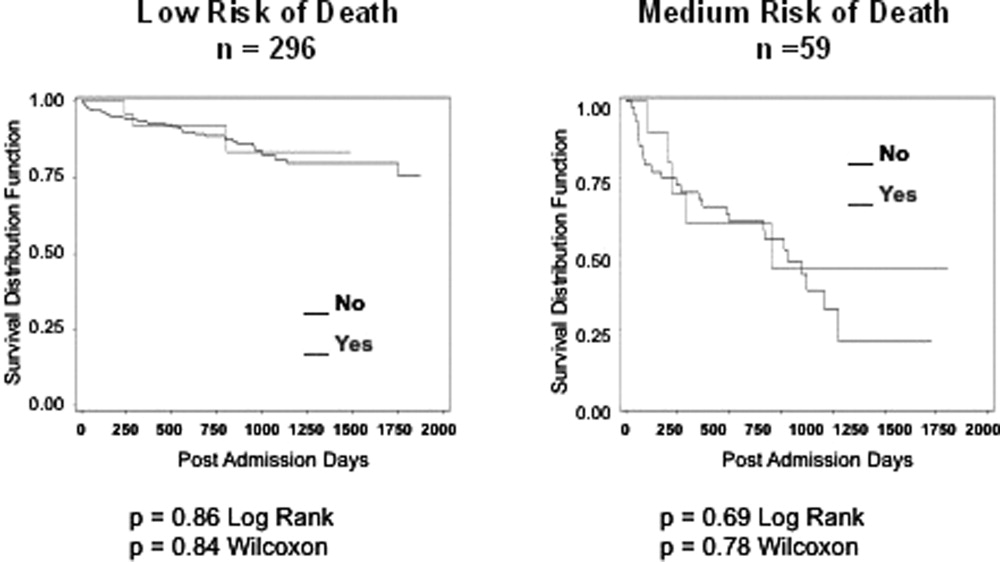
DISCUSSION
We found no decrease in survival for patients at low and medium 1‐year risk of death who reported having discussed ADs or who had an AD in their medical record, providing important evidence that having advance care planning discussions do not hasten death in this group of adults. However, it is possible that ADs, when implemented properly, may dictate withdrawal or withholding of interventions that may extend quantity of life at a quality unacceptable for the person executing the directive. For example, a feeding tube delivering artificial nutrition and hydration may grant years to someone in a persistent vegetative state, but those years, without the ability to be aware or interact with surroundings and loved ones, may not be a life worth living for some individuals. One explanation for our negative findings may be that the circumstances in which an AD may have an effect on outcomes may not yet have occurred among this lower risk population.
Opposition to the process of advance care planning may be considered unethical, by removing the opportunity for individuals to express their desires in the event of decisional incapacity, therefore disregarding patient autonomy. Furthermore, with the growing evidence that AD discussions and documentation help patients achieve care consistent with their wishes at the end of life,9, 11, 17 preventing advance care planning may worsen end of life outcomes.
Another important finding in our study was that only about 10% of the patients interviewed had completed an AD document, although nearly half reported they had discussed ADs with a healthcare provider. The patients we interviewed in this study had been admitted to the hospital in the previous 24 hours. As part of the Patient Self‐Determination Act, all patients admitted to a healthcare facility should receive information and counseling on AD. Less than half of our cohort reported any discussions about ADs and only 10% had completed an AD, suggesting that huge opportunities exist for improvement in advance care planning. As this study demonstrates, there was no increased mortality from advance care planning among those at low and medium risk of death, and others have shown benefits from the process. AD discussions and documentation should be fostered, especially as the burden of chronic disease increases and the population ages. In targeted studies to improve advance care planning, completion rates of up to 85% have been achieved.17
Our decision to focus solely on patients at low or medium risk of death, and exclude those at a high risk of death, is based on both clinical and methodological judgment. First, it is important to note that ADs are important even for those at lower risk of deaththe 3 critical cases that have shaped AD policy in this country, Karen Ann Quinlan, Nancy Cruzan, and Terry Schiavo, were all otherwise healthy young women.
Our study does have limitations. First, the sample size is small and not powered to detect small differences in survival. In addition, we only examined Vital Records within Colorado, although all participants had either a date of death or recent date of last contact. It is also conceivable that some patients discussed or completed ADs at a later time in their illness trajectory. However, the generalizability of this study is a major strength, by including a population and healthcare settings that are ethnically and socioeconomically diverse. Generalization of results beyond the three types of hospitals should be limited even with the low intraclass correlation. The major limitation of this research is that we do not have data on participant quality of life or whether completing an AD led to increased use of palliative care. During the time the research was conducted, 2 of the 3 hospitals involved had small palliative care services and the third remains without a palliative care service.
In conclusion, our study provides limited data to counteract the misleading claims of those opposed to the advance care planning process. Our results underscore the importance of educating the public on the importance of ADs and cast doubt on the death myth surrounding advance care planning. However, further, preferably longitudinal, study is needed to prospectively understand both the benefits and risks of advance care planning.
All new legislation concerning advance care planning was removed from the Affordable Care Act, signed into law in March 2010. However, through a Medicare payment regulation, Centers for Medicare and Medicaid Services (CMS) was able to add a provision allowing compensation to physicians for advance directive (AD) discussions as part of the annual Medicare wellness exam. Previously, under President George W. Bush, funding for AD discussions was already part of the Welcome to Medicare visit. Once again, the provision was misrepresented and distorted in the media, talk radio shows, and social networking sites. Within days of the announcement, the White House removed the regulation stating that the controversy surrounding the provision was distracting from the overall debate about healthcare. The term death panels has now entered our national lexicon and serves to undermine the efforts of the palliative care field which, through discussions with patients and families, attempts to provide care consistent with patients' goals.
In fact, ADs have been a cornerstone of ethical decision making, by supporting patient autonomy and allowing patient wishes to be respected when decisional capacity is lacking. Advance directives may include a living will, a Medical Durable Power of Attorney, or may be a broader more comprehensive document outlining goals, values, and preferences for care in the event of decisional incapacity. ADs allow patients to express preferences that incorporate both quantity and quality of life, as there are times when interventions at the end of life may increase length of life to the detriment of quality of life. In this context, patients may chose to value quality of life and request the interventions be withdrawn that focus on maintaining life without hope for quality of life. ADs also permit patients who prefer quantity over quality of life to communicate these wishes. These conversations are complex and time‐consuming. Patients may have profound misperceptions about the benefits offered by interventions at the end of life. Having detailed conversations with healthcare providers about actual benefits, risks, and alternatives has been shown to impact that decision‐making process.1 In our current payment system, these time‐consuming conversations are not compensated by private or public insurers, and are incompatible with 20‐minute appointments, so they rarely occur.24 While Nancy Cruzan and Terri Schiavo brought national attention to the issue for a brief time, recent data suggest that only 30% of adults have completed an AD,57 however, 93% of adults would like to discuss ADs with their physician.8 Furthermore, Silveira et al. showed that older adults with ADs are more likely than those without ADs to receive care that is consistent with their preferences at the end of life.9 ADs were the sole predictor of concordance between preferred and actual site of death in a cohort of seriously ill, hospitalized patients.10 Patients with advanced cancer who discussed their end of life wishes with their physician were more likely to receive care consistent with their preferences.11
Advance directives are based on the ethical principle of autonomy and, with the growing evidence that ADs may improve care at the end of life, public understanding of the issue is critical. We had presented early preliminary data in a letter to the editor showing that having had an advance directive discussion or an AD in the medical record was not associated with an increased risk of death.12 This research, along with the work of Silveira and colleagues,9 was cited by the Obama administration when they decided to add the regulation for including advance care planning as part of the annual Medicare wellness exams. This brief report presents a more comprehensive examination of the relationship of AD discussions and AD documentation with survival in a group of hospitalized patients.
METHODS
Study Sites and Participant Recruitment
This was a multisite, prospective study of patients admitted to the hospital for medical illness. The Colorado Multi‐Institutional Review Board approved this study.
Over a 17‐month period starting in February 2004, participants were recruited from 3 hospitals affiliated with the University of ColoradoDenver Internal Medicine Residency program: the Denver Veterans' Administration Center (DVAMC); Denver Health Medical Center (DHMC), the city's safety net hospital; and University of Colorado Hospital (UCH), an academic tertiary, specialty care and referral center. Exclusion criteria included: admission <24 hours, pregnancy, age <18 years, incarceration, spoke neither English nor Spanish, lack of decisional capacity. Recruitment was done on the day following admission to the hospital throughout the year, to reduce potential bias due to seasonal trends. A trained assistant recruited on variable weekdays (to allow inclusion of weekend admissions). Of 842 admissions occurring during the recruitment, 331 (39%) were ineligible (175 discharged and 2 died within 24 hours postadmission; 76 lacked decisional capacity; and 78 met other exclusion criteria listed above). All other patients (n = 511) were invited to participate and 458 patients consented.
Participant Interview and Measures
Fifty‐three (10%) refused; 458 gave informed consent and participated in a bedside interview, including questions related to advance care planning. In this interview, participants were first asked to define an AD. Their response was either confirmed or corrected using a standard simple explanation that defined and described ADs:
An advance directive is a document that lets your healthcare providers know who you would want to make decisions for you if you were unable to make them for yourself. It can also tell your healthcare providers what types of medical treatments you would and would not want if you were unable to speak for yourself.
They were then asked if any healthcare provider had ever discussed ADs with them (AD discussion is a primary variable of interest).
Chart Review and Vital Records Data Collection
We reviewed each medical record to determine admitting diagnoses, CARING criteria (a set of simple criteria developed by our group to score the need for palliative care, which has been shown to predict death at 1 year),13 socioeconomic and demographic information, and the presence of ADs in the medical record (documentation of AD is a primary variable of interest). We defined ADs broadly, including: living will, durable power of attorney for healthcare, or a comprehensive advance care planning document (eg, Five Wishes). The CARING criteria are validated criteria that accurately predict death at 1 year, and were developed to identify patients who would be appropriate for a palliative care intervention. It is based on the following variables: Cancer as a primary admitting diagnosis, Admitted 2 times to the hospital in the past year for a chronic medical illness, Resident of a nursing home, ICU admission with >2 organ systems in failure, and 2 Non‐Cancer hospice Guidelines as well as age. Scores range from 4 = low risk of death, 5‐12 = medium risk of death, and 13 = high risk of death at 1 year. We accessed hospital records and state Vital Records from 2003 to 2009 to determine which patients died within a 12‐month follow‐up period, and their date of death (primary outcome).
Cohort Risk Stratification
Based on their CARING score, participants were classified as being at low, medium, or high risk of death at 1 year.13 The probability of imminent death in the group of high‐risk patients is the main indication for an advance directive, and therefore the analysis of this high‐risk group would be confounded. Therefore, those at high (and unclassified) risk of death (89 [and 13] out of 458 interviewed patients) were excluded from the survival analysis. Including persons at high risk of death in this analysis would lead to confounding by indicationthat physicians are most likely to address ADs with patients that they perceive are likely to die in the near future. An example of this in the literature is the timing of do‐not‐attempt‐resuscitation orders (DNAR). It is well documented that most DNAR orders are written within 1 to 2 days of death.1416 The DNAR orders do not cause or lead to death, they are simply finally written for patients that are actively dying.
Statistical Analysis
SAS 9.1 (SAS Institute, Cary, NC) was used for all analyses. Survival analysis was conducted to examine time to death. Interaction effects of the variable of interest with patient risk were assessed by estimating Kaplan‐Meier survival curves for low and medium risk groups separately. The Wilcoxon and log‐rank tests were employed to compare those with and without AD discussions (and accounting for clustering within hospitals) and documentation. Since the stratification into risk groups involves the use of the CARING criteria, which were the main confounders, additional risk adjustment in each risk group was not performed. Post hoc power analysis showed an ability to detect a 13 percentage points difference in mortality rate, with 80% power for a 2‐sided test and alpha = 0.05, assuming a 20% death rate for the group without AD discussion (adjusting for the covariate distribution difference between those with and without AD discussion).
RESULTS
Characteristics of the 356 study subjects are listed in Table 1. Overall, the sample population was ethnically diverse, slightly above middle‐aged, mostly male, and of lower socioeconomic status, reflecting the hospitals' populations. Using the CARING criteria, 297 subjects were found to be at low risk, and 59 subjects at medium risk, of death at 1 year.
Percent (n) or Mean SD | |
---|---|
| |
Ethnicity | |
African American | 19% (69) |
Caucasian | 55% (194) |
Latino | 19% (66) |
Other | 8% (27) |
Age (years) | 57.2 15 |
Female gender | 34% (122) |
Admitted to | |
DVAMC | 41% (147) |
DHMC | 34% (122) |
UCH | 24% (87) |
CARING criteria | |
Cancer diagnosis | 4% (15) |
Admitted to hospital 2 times in the past year for chronic illness | 31% (109) |
Resident in a nursing home | 2% (7) |
Non‐cancer hospice guidelines (meeting 2) | 1% (4) |
Income less than $30,000/yr | 81% (284) |
No greater than high school education | 53% (188) |
Living situation | |
Home owner | 36% (125) |
Rents home | 38% (132) |
Unstable living situation | 27% (94) |
Low social support | 37% (169) |
Uninsured | 14% (51) |
Regular primary care provider | 72% (254) |
Overall, 206 (45%) reported a discussion about ADs with a healthcare provider. However, we found that only 56 (10%) had an AD document on their chart. Twenty‐eight (6%) had a living will, 43 (9%) had a durable power of attorney, and 30 (7%) had a broader AD document. Between 2003 and 2009, 121 (26%) patients died. Unadjusted mortality rates for those with and without documentation and discussions of ADs are displayed in Figure 1.
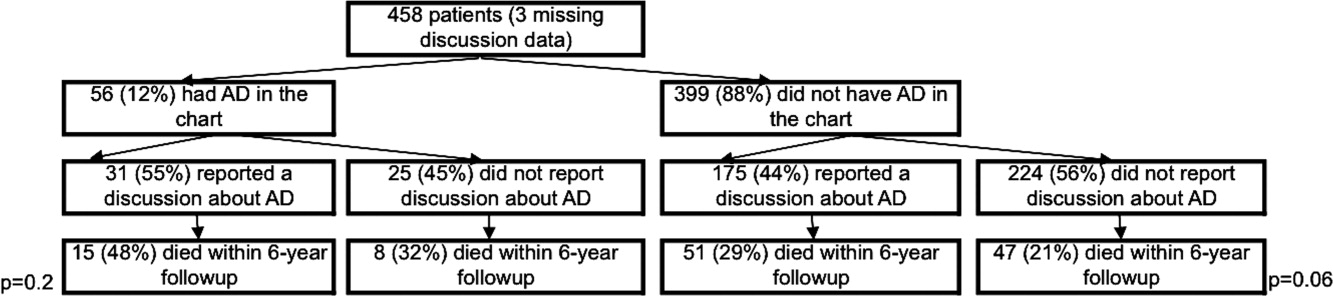
Kaplan‐Meier survival curves showed that, for subjects with a low or medium risk of death at 1 year, having had an AD discussion or having an AD in the medical record did not affect survival in subjects (Figures 2 and 3). Cox proportional hazards models adjusting for other covariates confirmed the results of the survival analysis (data not shown). Minimal intraclass correlation coefficients (0.005) were observed for the outcomes. Therefore, no models accounting for clustering within hospitals were developed.
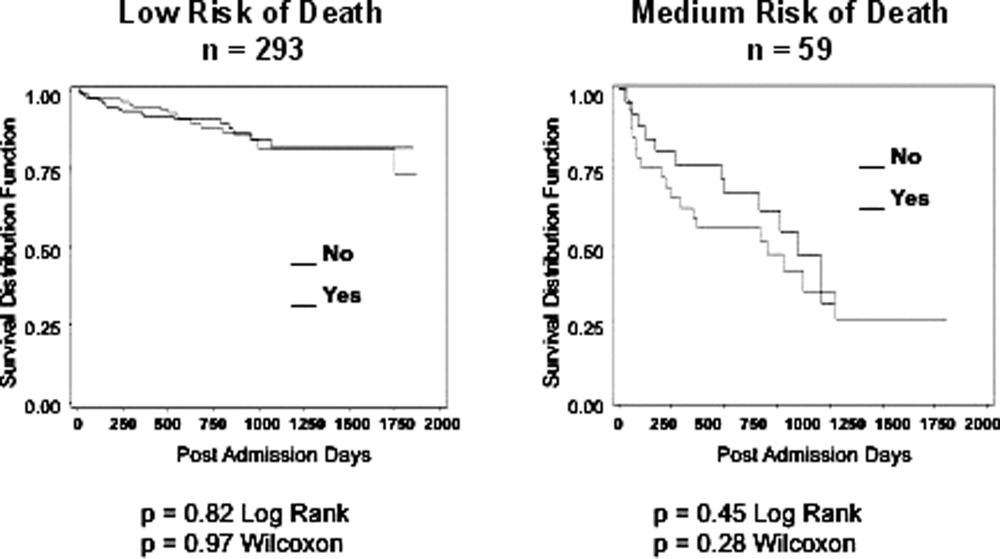
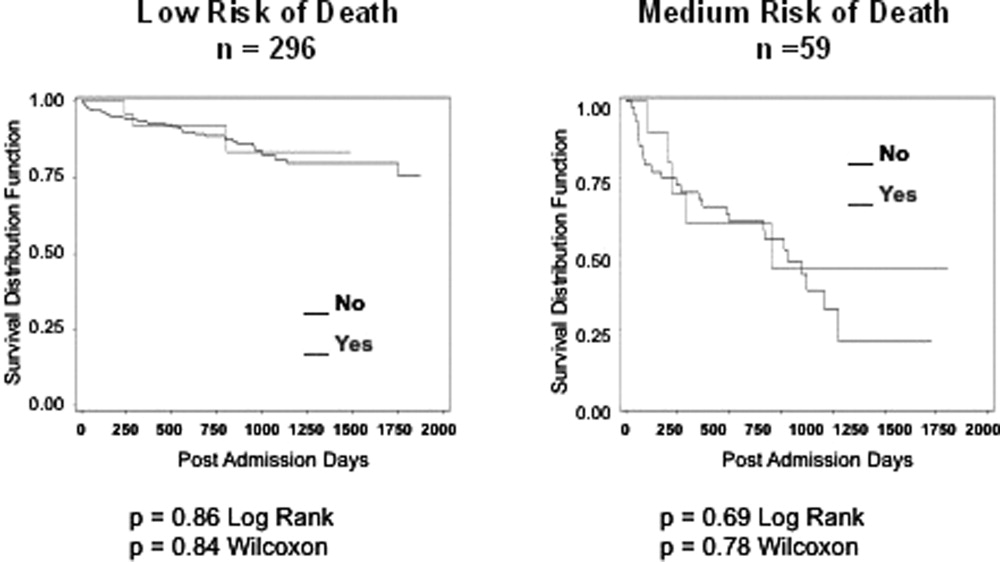
DISCUSSION
We found no decrease in survival for patients at low and medium 1‐year risk of death who reported having discussed ADs or who had an AD in their medical record, providing important evidence that having advance care planning discussions do not hasten death in this group of adults. However, it is possible that ADs, when implemented properly, may dictate withdrawal or withholding of interventions that may extend quantity of life at a quality unacceptable for the person executing the directive. For example, a feeding tube delivering artificial nutrition and hydration may grant years to someone in a persistent vegetative state, but those years, without the ability to be aware or interact with surroundings and loved ones, may not be a life worth living for some individuals. One explanation for our negative findings may be that the circumstances in which an AD may have an effect on outcomes may not yet have occurred among this lower risk population.
Opposition to the process of advance care planning may be considered unethical, by removing the opportunity for individuals to express their desires in the event of decisional incapacity, therefore disregarding patient autonomy. Furthermore, with the growing evidence that AD discussions and documentation help patients achieve care consistent with their wishes at the end of life,9, 11, 17 preventing advance care planning may worsen end of life outcomes.
Another important finding in our study was that only about 10% of the patients interviewed had completed an AD document, although nearly half reported they had discussed ADs with a healthcare provider. The patients we interviewed in this study had been admitted to the hospital in the previous 24 hours. As part of the Patient Self‐Determination Act, all patients admitted to a healthcare facility should receive information and counseling on AD. Less than half of our cohort reported any discussions about ADs and only 10% had completed an AD, suggesting that huge opportunities exist for improvement in advance care planning. As this study demonstrates, there was no increased mortality from advance care planning among those at low and medium risk of death, and others have shown benefits from the process. AD discussions and documentation should be fostered, especially as the burden of chronic disease increases and the population ages. In targeted studies to improve advance care planning, completion rates of up to 85% have been achieved.17
Our decision to focus solely on patients at low or medium risk of death, and exclude those at a high risk of death, is based on both clinical and methodological judgment. First, it is important to note that ADs are important even for those at lower risk of deaththe 3 critical cases that have shaped AD policy in this country, Karen Ann Quinlan, Nancy Cruzan, and Terry Schiavo, were all otherwise healthy young women.
Our study does have limitations. First, the sample size is small and not powered to detect small differences in survival. In addition, we only examined Vital Records within Colorado, although all participants had either a date of death or recent date of last contact. It is also conceivable that some patients discussed or completed ADs at a later time in their illness trajectory. However, the generalizability of this study is a major strength, by including a population and healthcare settings that are ethnically and socioeconomically diverse. Generalization of results beyond the three types of hospitals should be limited even with the low intraclass correlation. The major limitation of this research is that we do not have data on participant quality of life or whether completing an AD led to increased use of palliative care. During the time the research was conducted, 2 of the 3 hospitals involved had small palliative care services and the third remains without a palliative care service.
In conclusion, our study provides limited data to counteract the misleading claims of those opposed to the advance care planning process. Our results underscore the importance of educating the public on the importance of ADs and cast doubt on the death myth surrounding advance care planning. However, further, preferably longitudinal, study is needed to prospectively understand both the benefits and risks of advance care planning.
- The influence of the probability of survival on patient's preferences regarding cardiopulmonary resuscitation.N Engl J Med.1994;330:545–549. , , .
- Physician reluctance to discuss advance directives. An empiric investigation of potential barriers.Arch Intern Med.1994;154(20):2311–2318. , , .
- Advance directives and advance care planning: report to Congress. US Department of Health 82(12):1487–1490.
- Facts on dying: policy relevant data on care at the end of life, USA and state statistics. Dartmouth Atlas of Health Care Web site. Available at: http://www.chcr.brown.edu/dying/usastatistics.htm. Accessed September 20,2010.
- The quest to reform end of life care: rethinking assumptions and setting new directions.Hastings Cent Rep. November—December2005;S52–S57. , .
- End‐of‐life care and outcomes.Summary, Evidence Report/Technology Assessment No 110.Rockville, MD:Agency for Healthcare Research and Quality; December2004;1–6. , , , et al.
- Advance directives for medical care—a case for greater use.N Engl J Med.1991;324(13):889–895. , , , , .
- Advance directives and outcomes of surrogate decision making before death.N Engl J Med.2010;362(13):1211–1218. , , .
- Advance directives: the best predictor of congruence between preferred and actual site of death [Research Poster Abstracts].Journal of Hospital Medicine2010;5(S1):1–81. , , .
- End‐of‐life discussions, goal attainment, and distress at the end of life: predictors and outcomes of receipt of care consistent with preferences.J Clin Oncol2010;28(7):1203–1208. , , , , .
- Advance directive discussions do not lead to death.J Am Geriatr Soc.2010;58(2):400–401. , , .
- Practical tool to identify patients who may benefit from a palliative approach: the CARING criteria.J Pain Symptom Manage.2005;31(4):285–292. , , , , , .
- Do not resuscitate orders and the cost of death.Arch Intern Med.1993;153(10):1249–1253. , , .
- The do‐not‐resuscitate order: associations with advance directives, physician specialty and documentation of discussion 15 years after the Patient Self‐Determination Act.J Med Ethics.2008;34(9):642–647. , , , , .
- Factors associated with do‐not‐resuscitate orders: patients' preferences, prognoses, and physicians' judgments. SUPPORT Investigators. Study to Understand Prognoses and Preferences for Outcomes and Risks of Treatment.Ann Intern Med.1996;125(4):284–293. , , , et al.
- Death and end‐of‐life planning in one midwestern community.Arch Intern Med.1998;158(4):383–390. , .
- The influence of the probability of survival on patient's preferences regarding cardiopulmonary resuscitation.N Engl J Med.1994;330:545–549. , , .
- Physician reluctance to discuss advance directives. An empiric investigation of potential barriers.Arch Intern Med.1994;154(20):2311–2318. , , .
- Advance directives and advance care planning: report to Congress. US Department of Health 82(12):1487–1490.
- Facts on dying: policy relevant data on care at the end of life, USA and state statistics. Dartmouth Atlas of Health Care Web site. Available at: http://www.chcr.brown.edu/dying/usastatistics.htm. Accessed September 20,2010.
- The quest to reform end of life care: rethinking assumptions and setting new directions.Hastings Cent Rep. November—December2005;S52–S57. , .
- End‐of‐life care and outcomes.Summary, Evidence Report/Technology Assessment No 110.Rockville, MD:Agency for Healthcare Research and Quality; December2004;1–6. , , , et al.
- Advance directives for medical care—a case for greater use.N Engl J Med.1991;324(13):889–895. , , , , .
- Advance directives and outcomes of surrogate decision making before death.N Engl J Med.2010;362(13):1211–1218. , , .
- Advance directives: the best predictor of congruence between preferred and actual site of death [Research Poster Abstracts].Journal of Hospital Medicine2010;5(S1):1–81. , , .
- End‐of‐life discussions, goal attainment, and distress at the end of life: predictors and outcomes of receipt of care consistent with preferences.J Clin Oncol2010;28(7):1203–1208. , , , , .
- Advance directive discussions do not lead to death.J Am Geriatr Soc.2010;58(2):400–401. , , .
- Practical tool to identify patients who may benefit from a palliative approach: the CARING criteria.J Pain Symptom Manage.2005;31(4):285–292. , , , , , .
- Do not resuscitate orders and the cost of death.Arch Intern Med.1993;153(10):1249–1253. , , .
- The do‐not‐resuscitate order: associations with advance directives, physician specialty and documentation of discussion 15 years after the Patient Self‐Determination Act.J Med Ethics.2008;34(9):642–647. , , , , .
- Factors associated with do‐not‐resuscitate orders: patients' preferences, prognoses, and physicians' judgments. SUPPORT Investigators. Study to Understand Prognoses and Preferences for Outcomes and Risks of Treatment.Ann Intern Med.1996;125(4):284–293. , , , et al.
- Death and end‐of‐life planning in one midwestern community.Arch Intern Med.1998;158(4):383–390. , .
Copyright © 2011 Society of Hospital Medicine